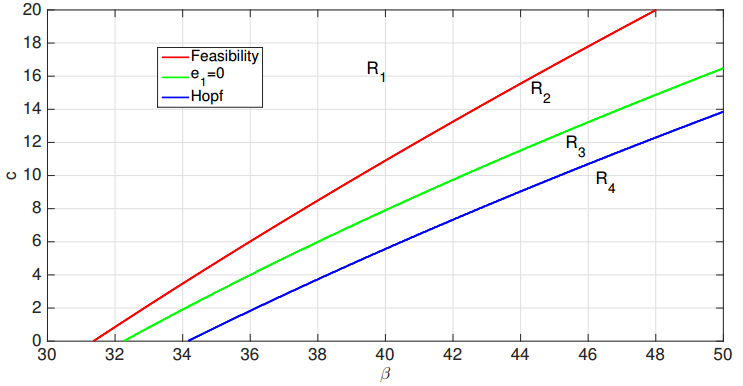
Citation: Raimund Bürger, Gerardo Chowell, Elvis Gavilán, Pep Mulet, Luis M. Villada. Numerical solution of a spatio-temporal predator-prey model with infected prey[J]. Mathematical Biosciences and Engineering, 2019, 16(1): 438-473. doi: 10.3934/mbe.2019021
[1] | Raimund BÜrger, Gerardo Chowell, Elvis GavilÁn, Pep Mulet, Luis M. Villada . Numerical solution of a spatio-temporal gender-structured model for hantavirus infection in rodents. Mathematical Biosciences and Engineering, 2018, 15(1): 95-123. doi: 10.3934/mbe.2018004 |
[2] | Ilse Domínguez-Alemán, Itzel Domínguez-Alemán, Juan Carlos Hernández-Gómez, Francisco J. Ariza-Hernández . A predator-prey fractional model with disease in the prey species. Mathematical Biosciences and Engineering, 2024, 21(3): 3713-3741. doi: 10.3934/mbe.2024164 |
[3] | Kwadwo Antwi-Fordjour, Folashade B. Agusto, Isabella Kemajou-Brown . Modeling the effects of lethal and non-lethal predation on the dynamics of tick-borne disease. Mathematical Biosciences and Engineering, 2025, 22(6): 1428-1463. doi: 10.3934/mbe.2025054 |
[4] | Paulo Amorim, Bruno Telch, Luis M. Villada . A reaction-diffusion predator-prey model with pursuit, evasion, and nonlocal sensing. Mathematical Biosciences and Engineering, 2019, 16(5): 5114-5145. doi: 10.3934/mbe.2019257 |
[5] | Sangeeta Kumari, Sidharth Menon, Abhirami K . Dynamical system of quokka population depicting Fennecaphobia by Vulpes vulpes. Mathematical Biosciences and Engineering, 2025, 22(6): 1342-1363. doi: 10.3934/mbe.2025050 |
[6] | Xiaoying Wang, Xingfu Zou . Pattern formation of a predator-prey model with the cost of anti-predator behaviors. Mathematical Biosciences and Engineering, 2018, 15(3): 775-805. doi: 10.3934/mbe.2018035 |
[7] | Sourav Kumar Sasmal, Jeet Banerjee, Yasuhiro Takeuchi . Dynamics and spatio-temporal patterns in a prey–predator system with aposematic prey. Mathematical Biosciences and Engineering, 2019, 16(5): 3864-3884. doi: 10.3934/mbe.2019191 |
[8] | Yun Kang, Sourav Kumar Sasmal, Komi Messan . A two-patch prey-predator model with predator dispersal driven by the predation strength. Mathematical Biosciences and Engineering, 2017, 14(4): 843-880. doi: 10.3934/mbe.2017046 |
[9] | Aniello Buonocore, Luigia Caputo, Enrica Pirozzi, Amelia G. Nobile . A non-autonomous stochastic predator-prey model. Mathematical Biosciences and Engineering, 2014, 11(2): 167-188. doi: 10.3934/mbe.2014.11.167 |
[10] | Lazarus Kalvein Beay, Agus Suryanto, Isnani Darti, Trisilowati . Hopf bifurcation and stability analysis of the Rosenzweig-MacArthur predator-prey model with stage-structure in prey. Mathematical Biosciences and Engineering, 2020, 17(4): 4080-4097. doi: 10.3934/mbe.2020226 |
Mathematical models are powerful tools to characterize and gain insights into the spatial-temporal dynamics of complex systems. More specifically, mathematical models are frequently used to gain a qualitative understanding of the dynamics and control of natural and social dynamic phenomena [44]. For example, systems of mathematical models are often simple and crude approximations that aim to capture key mechanisms underlying the dynamics of infectious disease transmission in human, animal, and plant populations. Such first approximation models can be subsequently refined to study the effects of additional factors and processes including spatial heterogeneity in density of hosts with different characteristics (e.g., fitness, susceptibility, infectivity) as well as the role of specific host behaviors (e.g, migration and diffusion patterns) in response to local host density or disease prevalence.
It is the purpose of this contribution to advance a spatio-temporal predator-prey model taking into account infected prey with a particular focus on its efficient numerical solution and dynamic characteristics. The resulting eco-epidemiological model combines several submodels that have been proposed in the recent literature. The population of prey is described by a spatio-temporal SIR-type epidemiological model similar to the one studied by Sun [45] while the predator-prey interaction follows the treatment by Greenhalgh and Haque [22] (formulated in a non-spatial setting in that paper). The movement of predators is based on the non-local velocity model advanced for the predator movement in a spatio-temporal predator-prey model by Colombo and Rossi [16]. That velocity model was also used for the male compartments in a recently published model of hantavirus infection [11]. In particular the implicit-explicit numerical technique for efficiently solving the governing partial differential equations (PDEs) is adapted to the new model presented herein.
In most general terms, the governing model considers a prey population with a susceptible and infective compartment, denoted by S and I, and a variable Y denoting the population of the predator. In the spatio-temporal context, these variables are understood as local densities that are functions of position x∈Ω and time t∈T:=[0,T] on a bounded domain Ω⊂R2. The final model for
u:=(u1,u2,u3)T:=(S,I,Y)T,u=u(x,t) |
is given by a convection-diffusion reaction system of the type
∂u∂t+∇⋅Fc(u)=DΔu+s(u), | (1.1) |
supplied with initial and boundary conditions, where the convective fluxes Fc(u), the diffusion matrix D, and the vector of reaction terms s(u) are specified in later parts of the paper.
It is proposed to describe the movement of the predators in a particular way that depends non-locally on the density I of infected prey, while the movement of infected and susceptible prey, that is of members of the compartments I and S, is standard diffusion. This property calls for numerical methods that allow the efficient computation of numerical fluxes based on the non-local evaluation of data but avoid the severe time step restriction incurred by explicit time discretizations of the diffusion term DΔu. As in [11] the first goal can be achieved by a technique based on Fast Fourier Transforms (FFT) in combination with an implicit-explicit (IMEX) discretization to handle the second. The numerical method constructed in this way is applied to simulate several scenarios that allow comparing the effect of initial conditions and different parameter values. The numerical results exhibit a rich variety of behavior, including formation of patterns taking the shapes of spots, stripes, both from randomized perturbations of steady states and in a scenario that describes the invasion of a prey habitat by the predator. The structure of solutions obtained in the latter case are reminiscent of permanent wave fronts.
The spatial spread of infectious diseases is described mathematically in a number of monographs that include [18,35,36,41,48]. The temporal evolution of diseases is also treated in [10,21]. Our assumption that all epidemiological compartments are distributed over the whole spatial domain is opposed to the alternative metapopulation approach that describes spatial structure through well-identified sub-populations or "patches" (cf., e.g., [1,2,3,12,46,47]). The description of spatial structure by explicitly specifying the mobilities between "patches" is typical for characterizing the behavior of humans who undertake directed travels, while a description through a convection-diffusion-reaction mechanism is more suitable for non-human infectious agents such as spores, insects, and bacteria that would disperse [18,36,37]. A decisive advantage of the spatially continuous approach, namely its amenability to mathematical analysis, is emphasized in [49].
The introduction of [22] and Hadeler and Freedman [24] provide extensive references to real-world examples of three-species eco-epidemiological systems of sound prey, infected prey, and predators. Specifically, in [24] a predator-prey model is described in which the prey is infected by a parasite and the prey in turn infects the predator with that parasite. The models studied in [22] and herein are based on the general assumption that the infection weakens the prey and increases its susceptibility to predation. Furthermore, we mention that the eco-epidemiological system of the Salton Sea in the desert of Southern California, New Mexico, is an important eco-epidemiological system where birds (particularly pelicans) prey on fish (particularly tilapia). The mechanism of predation is explained in [23]: "The vibrio class of bacteria is very common in salt water fish, and those infected with vibrio may have salt water present in their tissue. As the fish struggle in their death process, they tend to rise to the surface of the sea for oxygen. When they do, they become very attractive to fish-eating birds, specifically the pelicans." Mathematical models of this system were proposed and analyzed by Chattopadhyay and collaborators in a series of papers [14,15,30,40].
A less common ingredient in mathematical epidemiology is the convective term ∇⋅Fc(u). Related advection terms, for which the essential functional dependence for one compartment is Fc=Fc(x,u)=b(x)u with a given velocity b(x), arise if the population under study is transported (as is the case with wind-borne infectious agents, plankton, etc.). In our model the main role of the convective term is similar to that of [36,Ch. 14] in that it imposes a preferred direction of movement of predators, where the global dependence of the direction is determined by convolution with population data within a certain horizon of the current position. This idea of non-local dependence of biological fluxes goes back to a non-local predator-prey model introduced and analyzed by Colombo and Rossi [16], and for which a numerical scheme was analyzed in [39]. The scheme of [39] is based on the Lax-Friedrichs scheme for the ease of demonstrating convergence properties; we here employ higher-order weighted essentially non-oscillatory (WENO) reconstructions [26,34] to achieve high-order spatial accuracy.
Furthermore, IMEX Runge-Kutta (IMEX-RK) schemes play an important role for the efficient numerical solution of (1.1). Roughly speaking, an IMEX-RK method for the convection-diffusion-reaction equation (1.1) consists of a Runge-Kutta scheme with an implicit discretization of the diffusive term DΔu combined with an explicit one for the convective and reactive terms, Fc(u) and s(u), respectively. To introduce the main idea, we assume that
dvdt=Φ∗(v)+Φ(v) | (1.2) |
represents a method-of-lines semi-discretization of (1.1), where Φ∗(v) and Φ(v) are spatial discretizations of the convective and reactive terms and of the diffusive term, respectively. Assume that the spatial mesh width is h>0 in both the x- and y-direction. Then the stability restriction on the time step Δt that explicit schemes impose when applied to (1.2) is very severe (Δt must be proportional to h2), due to the presence of Φ(v). The implicit treatment of both Φ∗(v) and Φ(v) would remove any stability restriction on Δt. However, the upwind nonlinear discretization of the convective terms in Φ∗(v) that is needed for stability makes its implicit treatment extremely involved. This situation becomes even more complicated due to the use of WENO reconstructions [26,34,42,51]. However, numerical integrators that deal implicitly with Φ(v) and explicitly with Φ∗(v) can be used with a time step restriction dictated by the convective-reactive term alone. These schemes have been profusely used in convection-diffusion problems and convection problems with stiff reaction terms [4,6,7,8,9,17,19,28,38,52].
The remainder of the paper is organized as follows. In Section 2 the governing mathematical model is introduced. To this end, we first formulate (in Section 2.1) a non-spatial predator-prey model, defined by three nonlinear ordinary differential equations (ODEs), in which an epidemic spreads in the prey. This model gives rise to five equilibrium points whose respective stability is discussed in Section 2.2, along with a discussion of the Hopf bifurcation associated with the unique stable one of the five equilibria that includes all three species. (These stability results will motivate the choice of parameters of the simulated spatio-temporal scenarios.) Then, in Section 2.3, we obtain the full spatio-temporal model by equipping the ODE model with diffusive terms for the prey species and a non-local convection term for predators. These ingredients are specified in detail in Section 2.4. Section 3 is devoted to the description of the numerical scheme proposed to solve the spatio-temporal model developed in Section 2.3. Specifically, the (standard) spatial discretization of local convection and diffusion terms is introduced in Section 3.1. The spatial discretization of the convolution defining the non-local predator velocities is described in Section 3.2. These velocities, in turn, are utilized in the definition of the final discretization of the convective flux by means of a fifth-order WENO discretization, as is detailed in Section 3.3. The time discretization of the complete model by an IMEX-RK scheme is outlined in Section 3.4. In Section 4, which is at the core of this paper, numerical solutions to the spatio-temporal model are presented. To motivate the choice of parameters, we focus on two equilibrium points of the three-equation ODE model of Section 2.1, as is briefly discussed in Section 4.1. In Section 4.2 we present a total number of 10 examples of numerical solutions of the spatio-temporal model that give rise to patterns. In Section 4.3 we present Example 11 that has been designed to demonstrate in a purely visual way the convergence of the numerical scheme to a definite solution upon refinement of the computational grid, while Example 12 illustrates the influence of the choice of the radius of the convolution kernel of the non-local prey velocity. Finally, some conclusions and possible directions of future work are collected in Section 5.
Combining the SIR epidemic model of [45] with a second species corresponding to a predator (as in the model of [22]), and assuming that the predator only eats infected prey. That is, illness arising from infection debilitates the prey, making it available for predation otherwise we assume that the predator is not sufficiently fit to access healthy prey. Accordingly, we obtain the following model:
dSdt=A−dS−βSI2, | (2.1a) |
dIdt=βSI2−(d+μ)I−cYImY+I, | (2.1b) |
dYdt=δY(1−hYI), | (2.1c) |
where t is time, S(t) is the population of susceptible prey, I(t) is the population of infected prey, A is the recruitment rate of the prey population, d is the natural death rate of the prey population, β is the force of infection or the rate of transmission, μ is the disease-related death from the infected, Y(t) is the population of predators, c is the search rate of the predators towards infected prey, δ is the per capita growth rate of the predators, h is a constant related to the density dependent mortality of the predator population, and m>0 is a constant.
The incidence rate in (2.1a) and (2.1b) is chosen here as the nonlinear expression βSI2 in agreement with Sun [45], who in turn appeals to the justification by Liu et al. [32,33]. The last term in the righthand side of (2.1b) is a ratio-dependent predation term; see, e.g., [29] for further justification of such expressions. Overall, the right-hand sides of (2.1a) and (2.1b) have been chosen such that they coincide with the reactive (algebraic, i.e., non-differential) terms of the governing model of [45] (Equations (1a) and (1b)) of that paper, so that results can in principle be compares. Moreover, the assumption of a constant recruitment rate A (rather than a per capita growth rate for the prey population) simplifies the subsequent stability analysis. Furthermore, note that (2.1c), that is the assumption of a logistical growth rate of the predator population with a carrying capacity proportional to the population size of prey, coincides with the predator equation [22,Eq. (1)], and that hY/I in that equation is the Leslie-Gower term [31] which measures the loss in the predator population due to the relative scarcity of the (infected) prey [23]. An example of a real biological scenario for which (2.1) would be an adequate description is the "pelican-tilapia" eco-epidemiological model of the Salton Sea mentioned in Section 1.2, where S and I correspond to the number of susceptible and infected tilapia (fish) and Y to that of the predating pelicans (birds). In particular, Chattopadhyay and Bairagi [14] and Sarkar et al. [40] argue that in this scenario the predator only eats infected prey (although later works study variants of the model advanced in [14] in which the predator also eats susceptible (sound) prey, see [15,23,30]).
It is well known that the use of a ratio-dependent terms requires carefully handling situations of zero denominators. The right-hand sides of the ODE version (2.1) are potentially ill-defined if I(0)=0. This issue is treated in [22] as follows: if I(0)=0 and Y(0)≥0, then (2.1c) is interpreted as implying that Y(t)=0 for t>0 and if I(0)=Y(0)=0, then (2.1b) is interpreted as implying that I(t)=0 for all t. With respect to the PDE version (2.15), we have not encountered any difficulty of division by zero in our numerical experiments since in all cases we choose the initial datum I(x,0)>0 for x∈Ω.
Theorem 1. The system (2.1) has the following equilibrium points:
i) The equilibrium E1=(A/d,0,0) corresponding to the extinction of the epidemic.
ii) The following equilibria in absence of the predator:
E2=(Aβ+√R2dβ,2d(d+μ)Aβ+√R,0),E3=(Aβ−√R2dβ,2d(d+μ)Aβ−√R,0), |
where we define R:=A2β2−4d3β−8d2βμ−4dβμ2. These equilibria are feasible if R>0, which occurs if β>4d(d+μ)2/A2.
iii) The following equilibria with presence of the predator:
E4=(Ad+βI24,I4,I4h),E5=(Ad+βI25,I5,I5h), | (2.2) |
where I4<I5 are the solutions of the quadratic equation
αβI2−βAI+dα=0,whereα=d+μ+cm+h. |
They are feasible if
β>4dα2/A2; | (2.3) |
if this condition is satisfied, then we have
I4=A2α−(A24α2−dβ)1/2,I5=A2α+(A24α2−dβ)1/2. | (2.4) |
The point E1 corresponds to the extinction of the epidemic. On the other hand, the detailed analysis in [45] shows that E2 is unstable and E3 is stable. In these states the predator is absent, but we are especially interested in equilibria in which the predator does participate. To handle the dynamics of the system around E4 and E5, we first recall that at Ek, k=4 or k=5, the stability matrix is given by
J∗(Ek)=[−βI2k−d−2cm+h−2d−2μ0βI2k2cm+h+d+μ−cm(m+h)2−ch2(m+h)20δ/h−δ]=[−βI2k−d−2α0βI2kα+ch(m+h)2−ch2(m+h)20δ/h−δ]. |
A straightforward calculation reveals that
detJ∗(Ek)=αδ(d−βI2k)fork=4,5. | (2.5) |
For I4 given by the first expression of (2.4), we obtain, after rearranging terms,
detJ∗(E4)=αβδ(A24α2−dβ)1/2[Aα−2(A24α2−dβ)1/2]. |
Since the term in squared brackets is positive, it follows that detJ∗(E4)>0, and since the entries of J∗(E4) are real, this means that at least one eigenvalue of J∗(E4) has positive real part. Thus, the equilibrium E4 is always unstable.
To analyze the stability of E5, we utilize the Routh-Hurwitz criterion. To this end, we write the characteristic equation of J∗ as
det(J∗−λI)=0⇔λ3+e1λ2+e2λ+e3=0, | (2.6) |
where e1=−tr(J∗), e2=∑3i=1Mi, where Mi is the determinant of the matrix obtained by eliminating row i and column i from J∗, and e3=−detJ∗. Here, the Routh-Hurwitz conditions (cf., e.g., [20,Sect. 6.4]; see also [50,Th. 2]) mean that there are only roots with strictly negative real parts, and hence the corresponding state is stable, if and only if
e1>0,e3>0,ande1e2>e3. | (2.7) |
This criterion is now applied to J∗(E5). From (2.5) for k=5 we get
detJ∗(E5)=αβδ(A24α2−dβ)1/2[−Aα−2(A24α2−dβ)1/2]<0, | (2.8) |
so we always have e3>0 for J∗(E5) wherever E5 is feasible.
The subsequent analysis of e1 and e2, as well as that of the existence and possible type of a Hopf bifurcation around E5, will be simplified if we fix the parameters
A=1,μ=1.8,d=1,m=30,h=0.1,δ=0.4, | (2.9) |
where the values of A, μ, and d are those proposed in [45] while the choices of m, h, and δ are assumed but are comparable with those utilized in [22]. In order identify scenarios of interest for the simulation of the spatio-temporal model we will only vary the infectious force of the epidemic expressed by the parameter β, and the search rate c of the predators towards infected prey. The stability of E5 will be analyzed under variations of β and c only.
To determine suitable choices of β and c, we assume that e1=e1(β,c), e2=e2(β,c) and e3=e3(β,c) and analyze satisfaction of the Routh-Hurwitz condition (2.7). In addition, and to make the discussion as short as possible, we utilize numerical evaluation to deduce that e1, e2, and a coefficient function γ that arises further below, are positive on a relevant (β,c)-region in each case. Combined with the feasibility condition (2.3) and the fact that e3>0 wherever (2.3) holds, this discussion gives rise to four distinct regions as subregions of the region of the (β,c)-plane where the feasibility condition (2.3), the first condition in (2.7), and all conditions of (2.7) are satisfied, see Figure 1.
Finally, we demonstrate that a Hopf bifurcation occurs across the curve that separates R3 from R4. Our reasoning partly follows that of [23,Th. 6]. Figure 1 illustrates that at points where the stability changes (either from unstable to stable or from stable to unstable), it is the constraint e1e2>e3 that changes rather than e1>0, where we recall that e3>0 is generically satisfied wherever E4 and E5 are feasible. Furthermore, for the parameters (2.9) and (β,c)∈R:=[30,50]×[0,20], we also get e2>0 wherever E4 and E5 are feasible. (We limit the further discussion to (β,c)∈R, and understand that R1 to R4 are subregions of R.)
Let us show that across the curve c↦βH(c), which is marked in blue in Figure 1, defined implicitly by e1(βH(c),c)e2(βH(c),c)−e3(βH(c),c)=0, a Hopf bifurcation occurs, i.e., we fix c as a parameter and then consider β as a bifurcation parameter. Clearly, the characteristic equation (2.6) takes the form (λ2+e2)(λ+e1)=0 with the three roots λ1=ie1/22, λ2=−ie1/22 and λ3=−e1. Thus, in a neighborhood of (βH(c),c), (2.6) cannot have real positive roots; rather, the roots are of the form λ1=u+iv, λ2=u−iv and λ3=−ϕ with ϕ>0, and where u,v∈R and ϕ depend on (βH(c),c). It remains to verify the transversality condition, i.e.,
(∂∂βℜλ1(β,c))|β=βH(c)=∂u(β,c)∂β|β=βH(c)≠0. |
To this end, we substitute λ=λ1=u+iv into (2.6), equate real and imaginary parts, and calculate the derivative (see [23]) to obtain the following equations, where u=u(β,c), u′=∂u(β,c)/∂β, and analogously for v, e1, e2, and e3 and quantities ρ=ρ(β,c), σ, τ and ν defined below. This yields
ρu′−σv′+τ=0,σu′+ρv′+ν=0, | (2.10) |
where
ρ=3u2+2e1u+e2−3v2,σ=6uv+2e1v,τ=u2e′1+e′2u+e′3−e′1v2,ν=2uve′1+e′2v. | (2.11) |
At β=βH(c), u(βH(c))=0 and v(βH(c))≠0. Since e1>0 in a neighborhood of (βH(c),c), it follows that σ≠0. Applying Cramer's rule to (2.10) we get
∂u(β,c)∂c|β=βH(c)=u′(β)|β=βH(c)=−σν+ρτρ2+σ2|β=βH(c). | (2.12) |
We wish to show that σν+ρτ≠0. Evaluating (2.11) at β=βH(c), we obtain at that state σν+ρτ=2e2(e1e′2+e2e′1−e′3). We already know that e2>0 in the region of interest. Furthermore,
e′1=∂(βI25)∂β,e′2=(α+δ−ch(m+h)2)∂(βI25)∂β,e′3=αδ∂(βI25)∂β; |
so that
e1e′2+e2e′1−e′3=γ(βH(c),c)∂(βI25)/∂β, where we define
γ(β,c):=e1(α+δ−ch(m+h)2)+e2−αδ. |
For the parameters (2.9), we obtain
γ(β,c)>0for all(β,c)∈R3∪R4, | (2.13) |
as we infer from the rightmost plot of Figure 2. (Indeed, γ is positive on a slightly larger subset of R. However, positivity on R3∪R4 is sufficient for the discussion of the bifurcation across the boundary between R3 and R4.) Furthermore, we observe that
∂(βI25)∂β=A22α2+Aα(A24α2−dβ)1/2+Aβ2α(A24α2−dβ)−1/2dβ2>0. | (2.14) |
Combining (2.13) and (2.14), we deduce from (2.12) that
∂u(β,c)/∂c|β=βH(c)<0, |
which proves the transversality condition. Thus, a Hopf bifurcation occurs across c↦βH(c). We have conducted this analysis for E5 as we are interested in the dynamics around an equilibrium when all three species are present. To make one of the eigenvalues of J∗(E5) zero, we must have detJ∗(E5)=0, but here we know that detJ∗(E5)<0 wherever E5 is feasible. In particular, it cannot happen that J∗(E5) has a complex conjugate pair of purely imaginary eigenvalues with the third one being zero. Thus, the system does not experience a supercritical bifurcation, and in particular (2.1) does not admit time periodic solutions. The latter property is a mere consequence of detJ∗(E5)<0, which has been established symbolically and is therefore valid for any choice of parameters (instead of (2.9)) and (β,c) for which E5 is feasible.
The ODE model (2.1) exhibits a rich solution behaviour, which motivates us to analyze a spatial variant that in turn generalizes the spatio-temporal hyperbolic-parabolic predator-prey model studied by Colombo and Rossi [16]. Following [11,16], we then replace (2.1c) by a non-local spatio-temporal PDE that contains a term describing the spatial movement of predators towards infected prey, and equip (2.1a) and (2.1b) with diffusion terms. The resulting spatio-temporal model is given by
∂S∂t−DSΔS=A−dS−βSI2, | (2.15a) |
∂I∂t−DIΔI=βSI2−(d+μ)I−cYImY+I, | (2.15b) |
∂Y∂t+∇⋅FY(S,I,Y)=δY(1−hYI), | (2.15c) |
where ∇⋅ denotes the (spatial) divergence operator. Here x and y are the space variables, Δ=∂2/∂x2+∂2/∂y2 is the two-dimensional Laplace operator, and DS and DI are the diffusion coefficients, i.e. we assume standard linear diffusion for the prey compartments S and I.
The right-hand sides of (2.15) are identical to that of the non-spatial ODE model (2.1), i.e., this model is recovered if all divergence terms on the left-hand sides are set to zero and variables are considered to depend on t only, and the unknowns represent suitably scaled densities.
The flux FY appearing in the left-hand side of (2.15c) is assumed to have the form FY(I,Y)=κYYV(I), where κY≥0 is constant and
V(w)=∇(w∗η)√1+‖∇(w∗η)‖2 | (2.16) |
is the non-local unscaled velocity function [16]. Here η denotes a radial convolution kernel with radius ε, i.e., η is a piecewise smooth function such that
η(x)=η(‖x‖2),η(x)≥0,η(x)=0for‖x‖>ε,and∫R2η(x)dx=1, | (2.17) |
i.e., for any function w defined on Ω×T and x∈Ω such that Bε(x):={y∈R2:‖y−x‖<ε}⊂Ω, we have
(w(⋅,t)∗η)(x)=∫Bε(x)w(y,t)η(x−y)dy=∫R2w(y,t)η(x−y)dy |
(with slight modifications for points x with dist(x,∂Ω)<ε.) Since ∇(w∗η)=w∗∇η, the velocity V(w) indeed depends (non-locally) on w and not on its gradient.
Summarizing, we obtain Fc(u)=(0,0,FY(I,Y))T and D=diiag(DS,DI,0). The vector s(u)=(s1(u),s2(u),s3(u))T is given by the right-hand sides of (2.15). The system (2.15) is considered on Ω×T along with the initial condition
u(x,0)=u0(x),x∈Ω, | (2.18) |
where u0 is a given function, and zero-flux boundary conditions
(Fc(u)−D∇u)⋅n=0,x∈∂Ω,t∈(0,T], | (2.19) |
where n is the unit exterior normal vector to the boundary ∂Ω of Ω.
We take Ω=[0,L]×[0,L] and use a Cartesian grid with nodes (xi,yj), i,j=1,…,M, with xi=yi=(i−1/2)h, h=L/M. We discretize ∇⋅Fc(u) by WENO finite differences and Δu by the standard second-order scheme with a five-point stencil to get a spatial semi-discretization of (1.1) for a 3×M×M-matrix v(t) of unknown approximations vℓ,i,j(t)≈uℓ(xi,yj,t) for i,j=1,…,M and ℓ=1,…,3. This spatial semi-discretization is given by
v′=−∇h⋅˜Fc(v)+Bv+S(v). | (3.1) |
Applying a suitable IMEX-RK scheme to (3.1) yields the final fully-discrete scheme (see Section 3.4). Here ∇h⋅˜Fc(v) is the discretization of ∇⋅Fc(u), to be defined in Section 3.3, and
(Bv)ℓ,i,j=μℓ(Δhvℓ)i,j,i,j=1,…,M,ℓ=1,…,3 | (3.2) |
denotes the discretization of the diffusion terms. Here vℓ denotes the M×M submatrix given by (vℓ)i,j=vℓ,i,j and Δh is the approximation of the standard two-dimensional Laplacian operator with Neumann boundary conditions. Furthermore, S(v) is the 3×M×M-matrix with components
S(v)ℓ,i,j=sℓ(vℓ,i,j),i,j=1,…,M,ℓ=1,…,3, |
with corresponding submatrices Sℓ(v), given by Sℓ(v)i,j=sℓ(vℓ,i,j).
The following identity arises from (2.16) if we take into account that ∇(w∗η)=w∗∇η:
V(w)=w∗v√1+‖w∗v‖2,v=(∂η∂x,∂η∂y). | (3.3) |
The corresponding convolutions w∗∂η/∂x and w∗∂η/∂y are calculated approximately on the discrete grid via a composite Newton-Cotes quadrature formula, such as the composite Simpson rule.
Since Bε(0)⊆[−rh,rh]2, r=⌈ε/h⌉<M, and according to boundary conditions, w is extended to the exterior of Ω by reflection, e.g. by setting w(−x,y)=w(x,y), (x,y)∈Ω, we obtain
(w∗χ)(xi,yj)≈r∑p=−rr∑q=−rβp,qw(xi−p,yj−q),βp,q=h2αpαqχ(xp,yq), | (3.4) |
where αp and αq are the coefficients in the quadrature rule (e.g., for the composite Simpson rule, α=(1,4,2,4,…,2,4,1)). Consequently, the approximation (3.4) for W=(wi,j)∈RM×M, wi,j≈w(xi,yj), is given by
(w∗χ)(xi,yj)≈(W∗hβ)i,j:=r∑p=−rr∑q=−rβp,qw[i−p]M,[j−q]M, | (3.5) |
where we define
[i]M:={−i+1for−r+1≤i≤0,ifor 1≤i≤M,2M+1−iforM+1≤i≤M+r. |
The discrete approximation of V(w) in (3.3) is then given by
Vh(W)=W∗hv√1+‖W∗hv‖2. |
Since r≈ε/h=εM/L, the computational cost of this discrete convolution is M2(2r+1)2≈4ε2M4/L2, which can be very high for large M. This cost can be substantially reduced to O(M2logM) by performing a convolution with periodic data by Fast Fourier Transforms (FFTs) (see [27,43]). To achieve this goal, we define from W=(wi,j)∈RM×M a matrix ˜W=(˜wi,j)∈R2M×2M such that
˜wi,j=w[i]M,[j]M,i,j=1,…,2M |
and use the notation [i]'_{2M} = \mod (i-1, 2M)+1, i.e., [i]'_{2M} = i+2kM, with k being the integer such that 1\leq [i]'_{2M}\leq 2M. It is then readily checked that
w_{[i]_{M}, [j]_{M}} = \tilde{w}_{[i]'_{2M}, [j]'_{2M}}, \quad i, j = -r+1, \dots, M+r. |
Therefore (3.5) for i, j = 1, \dots, M can be rewritten as
(\boldsymbol{W} \ast_h \beta)_{i, j} = \sum\limits_{p = -r}^{r} \sum\limits_{q = -r}^{r} \beta_{p, q} \tilde w_{[i-p]'_{2M}, [j-q]'_{2M}}. | (3.6) |
The convolution on the right-hand side of (3.6) can be performed by FFTs applied to the (2M)\times (2M) matrix \smash{\boldsymbol{\tilde{W}}}. To save further computational costs, the FFT of the kernel \beta_{p, q} is performed only once, son each convolution entails two two-dimensional FFT of (2M)\times (2M) matrices and a product of 4M^2 numbers, with an overall computational cost of \mathcal{O}(M^2\log M).
The convective flux for the prey compartments (S and I, corresponding to \ell\in\{1, 2\}) is zero and for the predator (species Y, \ell = 3) is given by \smash{\boldsymbol{F}^{\mathrm{c}}_{3} (\boldsymbol{u}) = u_{3} (\kappa_{3} \boldsymbol{V} (u_2))}. To discretize its divergence \nabla\cdot \boldsymbol{F}^{\mathrm{c}}_{3} (\boldsymbol{u}) for the approximation \boldsymbol{v}, we first approximate the convolution terms as expounded in Section 3.2 to obtain
\boldsymbol{\tilde{F}}^{\mathrm{c}}_{3} ( \boldsymbol{v} )_{i, j} = \boldsymbol{v}_{\ell, i, j} \bigl(\kappa_{3} \boldsymbol{V}_h\left(v_2\right)_{i, j} \bigr)\in\mathbb R^{2}. |
We introduce the notation {\smash (f_{i, j}^{x}, f_{i, j}^{y}): = \boldsymbol{\tilde{F}}^{\mathrm{c}}_{\ell} (\boldsymbol{v})_{i, j}}, where we have dropped the index \ell in \smash{f_{i, j}^{x}} etc. to obtain clearer expressions. Our purpose is to use a fifth-order WENO finite difference discretization [26,34,42] of \nabla\cdot \boldsymbol{F}^{\mathrm{c}}_{\ell} (\boldsymbol{u}) for which
\nabla\cdot \boldsymbol{F}^{\mathrm{c}}_{\ell} (\boldsymbol{u}) (x_i, y_j) \approx \nabla_h\cdot \tilde{\boldsymbol{F}}^{\mathrm{c}}(\boldsymbol{v})_{\ell, i, j}: = \frac{\hat f^{x}_{i+1/2, j}-\hat f^{x}_{i-1/2, j}}{h} + \frac{\hat f^{y}_{i, j+1/2}-\hat f^{y}_{i, j-1/2}}{h} |
for suitable numerical fluxes \smash{\hat f^{x}_{i+1/2, j}, \hat f^{y}_{i, j+1/2}} obtained by WENO reconstructions of split fluxes. For the numerical flux in the x-direction, the Lax-Friedrichs-type flux splitting f^{x, \pm} is given by
f^{x, \pm}_{i, j} = \frac12\big(f^{x}_{i, j}\pm \alpha^{x} v_{\ell, i, j} \big), \quad \alpha^{x} = \max\limits_{i, j} \bigl|\boldsymbol{V}_{h}^{x}(\boldsymbol{v})_{i, j} \bigr|, |
with an analogous formula for \smash{f^{y, \pm}_{i, j}}. If \mathcal{R}^{\pm} denotes fifth-order WENO upwind biased reconstructions and we use matlab-type notation for submatrices, then
\begin{align*} \hat f^{x}_{i+1/2, j}& = \mathcal{R}^{+} \bigl( f^{x, +}_{i-2:i+2, j} \bigr) +\mathcal{R}^{-} \bigl( f^{x, -}_{i-1:i+3, j}\bigr), \\ \hat f^{y}_{i, j+1/2} & = \mathcal{R}^{+} \bigl( f^{y, +}_{i, j-2:j+2} \bigr) +\mathcal{R}^{-} \bigl( f^{y, -}_{i, j-1:j+3} \bigr). \end{align*} |
To specify the IMEX-RK integrators for (3.1), we rewrite (3.1) as (1.2), where
\Phi^*(\boldsymbol{v}) : = -\nabla_{h}\cdot \boldsymbol{\tilde{F}}^{\mathrm{c}}( \boldsymbol{v})+ \boldsymbol{S}(\boldsymbol{v}), \qquad \Phi(\boldsymbol{v}) : = \boldsymbol{\mathcal{B}} \boldsymbol{v}. | (3.7) |
The diffusive part \Phi(\boldsymbol{v}) is handled by an implicit s-stage diagonally implicit (DIRK) scheme with coefficients \boldsymbol{A}\in\mathbb{R}^{s\times s}, \boldsymbol{b}, \boldsymbol{c}\in \mathbb{R}^s, in the common notation, where \boldsymbol{A} = (a_{ij}) with a_{ij} = 0 for j>i. For the term \Phi^*(\boldsymbol{v}) we employ an s-stage explicit scheme with coefficients \smash{\boldsymbol{\hat{A}}\in\mathbb{R}^{s\times s}}, \smash{\boldsymbol{\hat{b}}}, \boldsymbol{\hat{c}}\in\mathbb{R}^{s} and \smash{\boldsymbol{\hat{A}} = (\hat{a}_{ij})} with \hat{a}_{ij} = 0 for j\geq i. In our simulations, we limit ourselves to the second-order IMEX-RK scheme H-DIRK2(2, 2, 2) that corresponds to the pair of Butcher arrays
\begin{align*} \begin{array}{c|c} \boldsymbol{c} & \boldsymbol{A} \\ \hline & \boldsymbol{b}^{\mathrm{T}} \vphantom{X_X^{X^X}} \end{array} = \begin{array}{c|cc} 1/2 & 1/2 & 0\\[1mm] 1/2 & 0 & 1/2 \\[1mm] \hline & 1/2 & 1/2 \vphantom{\int\limits^.} \end{array}, \qquad \begin{array}{c|c} \boldsymbol{\hat{c}} & \boldsymbol{\hat{A}} \\ \hline & \boldsymbol{\hat{b}}^{\mathrm{T}} \vphantom{X_{X^X_X}^{X^{X^X}_X}} \end{array} = \begin{array}{c|cc} 0 & 0 & 0 \\ 1 & 1 & 0\\ \hline & 1/2 & 1/2 \vphantom{\int\limits^.} \end{array} \, . \end{align*} |
Alternative choices are provided and discussed in [5,6,38]. If applied to the equation (1.2), the IMEXRK scheme gives rise to the following algorithm (see [38]).
Algorithm 3.1.
Input: approximate solution vector \boldsymbol{v}^n of (1.2) for t = t^n
for p = 1, \dots, s
if p = 1 then
{\displaystyle \boldsymbol{\hat{v}}^{(1)} \leftarrow \boldsymbol{v}^n, \quad \bar{\boldsymbol{v}}^{(1)} \leftarrow \boldsymbol{v}^n }
else compute \boldsymbol{\hat{v}}^{(p)} and \bar{\boldsymbol{v}}^{(p)} from the known values \boldsymbol{K}_1, \dots, \boldsymbol{K}_{p-1}:
{\displaystyle \boldsymbol{\hat{v}}^{(p)} \leftarrow \boldsymbol{v}^n + \Delta t\sum_{j = 1}^{p-1}\hat{a}_{pj} \boldsymbol{K}_j}, \quad {\displaystyle \bar{\boldsymbol{v}}^{(p)} \leftarrow \boldsymbol{v}^n + \Delta t\sum_{j = 1}^{p-1} a_{pj} \boldsymbol{K}_j}
endif
solve for \boldsymbol{K}_p the linear system
{\boldsymbol{K}_p} = \Phi^*\bigl(\boldsymbol{\hat{v}}^{(p)}\bigr) + \Phi\bigl(\bar{\boldsymbol{v}}^{(p)} + \Delta t a_{pp} \boldsymbol{K}_p \bigr)\quad \quad \quad \quad \quad \quad (3.8)
endfor
{\displaystyle \boldsymbol{v}^{n+1} \leftarrow \boldsymbol{v}^n+\Delta t \sum_{j = 1}^sb_j\boldsymbol{K}_j}
Output: approximate solution vector \boldsymbol{v}^{n+1} of (1.2) for t = t^{n+1} = t^n+\Delta t.
To solve the linear equation (3.8) for \boldsymbol{K}_{p} , in view of (3.7) we rewrite it as
\bigl( \boldsymbol{I}-\Delta t a_{pp} \boldsymbol{\mathcal{B}}\bigr) \boldsymbol{K}_{p} = \boldsymbol{b}^{(p)}, \quad \boldsymbol{b}^{(p)}: = \Phi^*\bigl(\boldsymbol{\hat{v}}^{(p)}\bigr) + \boldsymbol{\mathcal{B}}\bar{\boldsymbol{v}}^{(p)}, | (3.9) |
where \boldsymbol{I} denotes the identity operator for {\rm{3}} \times M \times M matrices. From the definition of the matrix \boldsymbol{\mathcal{B}} in (3.2) and from the definition of \Phi^* in (3.7), if we equate the \ell submatrices along the first dimension of both sides of (3.9) we get
\bigl( \boldsymbol{I}_{M\times M}-\Delta t a_{pp} \mu_{\ell}\boldsymbol{\Delta}_h\bigr) (\boldsymbol{K}_{p})_{\ell} = -\nabla_{h}\cdot \tilde{\boldsymbol{F}}_{\ell}^{\mathrm{c}}( \boldsymbol{\hat{v}}^{(p)})+ \boldsymbol{S}_{\ell}(\boldsymbol{\hat{v}}^{(p)}) + \mu_{\ell}\boldsymbol{\Delta}_h\bar{\boldsymbol{v}}^{(p)}_{\ell}, \quad \ell = 1, 2, 3, | (3.10) |
where \boldsymbol{I}_{M\times M} is the M\times M identity operator and, e.g., (\boldsymbol{K}_{p})_{\ell} is the \ell submatrix of \boldsymbol{K}_p along the first dimension, i.e., ((\boldsymbol{K}_{p})_{\ell})_{i, j} = (\boldsymbol{K}_{p})_{\ell, i, j}. If \mu_{\ell} = 0 (for \ell = 3), then
(\boldsymbol{K}_{p})_{\ell} = -\bigl(\nabla_{h}\cdot \tilde{\boldsymbol{F}}^{\mathrm{c}}( \boldsymbol{\hat{v}} ^{(p)})\bigr)_{\ell}+ \boldsymbol{S}_{\ell}(\boldsymbol{v}), |
otherwise (3.10) is solved by Fast Cosine Transforms (due to boundary conditions), which entails a nearly optimal computational cost of \mathcal{O}(M^2\log M).
We wish to compare the ODE model (2.1) with the spatio-temporal model (2.15) in different scenarios.
For this model we consider parameter values (2.9) along with
(\beta, c) = (42, 5) \in R_4, | (4.1) |
in accordance with the region of stability shown in Figure 1 (these parameters will be varied within the numerical solution of spatio-temporal models). For the parameters (2.9) and (4.1), we obtain the following equilibria of Theorem 1 (besides E_1 = (1, 0, 0)) where the predator is absent:
E_2 = (0.7516611, 0.0886924, 0), \quad E_3 = (0.2483389, 0.2684504, 0), | (4.2) |
as well as the two points
E_4 = (0.7013155, 0.1006989, 1.0069894), \quad E_5 = (0.2986844, 0.2364426, 2.3644263). |
From Section 2.2 we know that all eigenvalues of \boldsymbol{J}^* (E_5) have negative real part, and that the equilibrium E_4 is unstable. As a consequence, whenever predators and prey are present, the solution orbits of (2.1) approach the stable equilibrium E_5, as is illustrated in Figure 3.
In Examples 1 to 10 we select the spatial domain \Omega = [0, L]\times[0, L] with L = 100. For the spatial discretization we use M = 400, such that \Delta x = L/M = 0.25. For the time discretization we employ Algorithm 3.1. The parameters \varepsilon = 4 and \kappa_Y = 1 are chosen in the non-local term, and the diffusion constants are chosen as D_{S} = 6 and D_{I} = 1, corresponding to the assumption that infected individuals of the prey population exhibit a lower degree of mobility than their susceptible counterparts. To observe the effect of the non-local movement of predators in the spatio-temporal dynamics of the prey, we consider two cases: Case Ⅰ, where the values of \beta and \mu in combination with D_{S} and D_{I} have been chosen such that they lie in the Turing region (see [45,Example 6]), and Case Ⅱ, where this combination is not in the Turing space. The initial condition for S and I is always a spatially distributed random perturbation of the respective values 0.3 and 0.2. For both cases, three scenarios display three different initial conditions of the distributions of the predators: (a) absence of predators, (b) a "triangular" initial condition for predators to describe how predators invade a region that is initially only occupied by the prey, and (c) a random initial distribution of the predators. These three scenarios are illustrated in Figure 4. The simulations are carried out until the non-local system (2.15) attains a stable non-homogeneous steady state.
Furthermore, we wish to compare the numerical results with the predictions obtained using the the non-spatial ODE model. To this end we determine for each compartment X \in \{ S, I, Y\} and time instants t^n = n \Delta t the following quantity:
\mathcal{I} (X) = \mathcal{I} (X, t^n) : = h^2 \sum\limits_{i, j = 1}^{\mathrm{N}} X^n_{i, j} \approx \int_{\Omega} X(\boldsymbol{x}, t^n) \, \mathrm{d} \boldsymbol{x}, | (4.3) |
which represents the approximate total number in \Omega of individuals of compartment X at time t^n. We recall that in the PDE model, the unknowns X \in \mathcal{C} are densities, and so an integration over \Omega is necessary to make results comparable with those of a model that predicts the total number of individuals in each compartment (as does the dynamical system (2.1)).
Example 1: Case Ⅰ in the absence of the predator.
Here, only the equations (2.15a), (2.15b) are considered, with the parameters given by (2.9) and (4.1). The values of \beta and \mu in combination with D_{S} = 6 and D_{I} = 1 have been chosen such that they lie in the Turing region, see [45,Example 6], which means that the formation of a permanent spatial pattern by the standard Turing mechanism in reaction-diffusion systems (see, e..g., [36]) is expected. In our simulation the initial conditions for S and I are those of Figure 4(a). In Figure 5 we display the numerical solution at three different times. We observe the formation of [45]. This distribution will remain constant over time. Moreover, Figure 6 shows the integral quantities \mathcal{I} (S), \mathcal{I} (I), and wherever appropriate \mathcal{I} (Y), for Examples 1 to 6. For Example 1, and consistently with the nearly stationary spatial pattern, we observe (in Figures 6(a) and (b)) that \mathcal{I} (S) and \mathcal{I} (I) become practically stationary after very short time, and assume values close to L^2 \times 0.2483389 \approx 2483 and L^2 \times 0.2684504 \approx 2684 that would be obtained if the solution were equal to the stable equilibrium E_3 (see 4.2) on the whole domain. In this sense, in this example the temporal behavior of the model is consistent with (2.1).
Example 2: Case Ⅰ with a "triangular" initial distribution of the predator.
Here we introduce predators through the "triangular" initial condition of Figure 4(b). All other parameters are the same as in Example 1. In Figure 7 we display the dynamics of model (2.15). The convective term in (2.15c) defined in terms of the non-local velocity function (2.16) allows the movement of the predators to places where infective prey are present. At simulated time t = 30 we observe that the distribution of prey varies in comparison with Example 1 in places where predators are present. At t = 90 we observe that in places where the predators are absent, preys are distributed in forming spots as in Example 1, however, where predators are present, these patterns take the form of stripes. At t = 200, predators are present in the whole domain, and this has motivated that the distribution of the prey in the domain varies drastically from its natural distribution without predator (Example 1). This distribution will remain constant over time. The corresponding plots of \mathcal{I} (S) and \mathcal{I} (I) (Figure 6(c)) and \mathcal{I} (Y) indicate a growth of the predator population over a very long period of time. Shortly before t = 200 these quantities become constant. It is worth noting that while \mathcal{I} (S) and \mathcal{I} (I) tend to values that are close to L^2 times the first and second component of E_5, namely 2986.8 and 2364.4, respectively, the limit value of \mathcal{I} (Y) exceeds L^2 times the Y-component of E_5, that is 23644.3, by more than a factor of two.
Example 3: Case Ⅰ with a random initial distribution of the predator.
Here we consider a random initial condition for predators with the same parameters of Example 1. The initial condition is shown in Figure 4(c). In Figure 8 we display the dynamics of the model with this initial condition. At simulated time t = 30 we observe that stripes and some spots patterns emerge mixed in the distribution of each species. After the stripe patterns form, they grow steadily with time until they reach a certain arm length, and the spatial patterns become distinct at t = 200. It is observed that these stripe patterns are not similar to those obtained in Example 1, instead these patterns in form of stripes or filaments are very similar to those observed in Example 2. Furthermore, Figures 6(e) and (f) indicate that \mathcal{I} (S), \mathcal{I} (I) and \mathcal{I} (Y) quickly attain steady states, with final values similar to those of Example 2.
Example 4: Case Ⅱ in the absence of the predator.
Here, we consider absence of predators like in Example 1 and we use here and in Examples 5 and 6 the parameter values
(\beta, c) = (46, 4) \in R_4. | (4.4) |
In this case the parameters \beta and \mu together with the diffusion constants are not in the Turing space. The steady states according to Theorem 1 are now, besides E_1 = (1, 0, 0),
E_2 = (0.7820731, 0.0778310, 0), \quad E_3 = (0.2179270, 0.2793118, 0), | (4.5) |
E_4 = (0.7510048, 0.0848976, 0.8489759), \quad E_5 = (0.2489953, 0.2560630, 2.5606299). | (4.6) |
Considering the initial conditions of Figure 4(a), we obtain in this case that no patterns are formed and the system quickly arrives at a constant equilibrium (uniform distribution for S and I in whole domain), which is maintained over time, as can be observed in Figure 9. This figure, as well as Figures 6(g) and (h), indicate that the global equilibrium is E_3 given by (4.5).
Example 5: Case Ⅱ with a "triangular" initial distribution of the predator.
In this case we consider the same scenario as in Example 2, namely the triangular initial condition of Figure 4(b), but utilize the parameters (4.4) instead of (4.1). In this case, as in Example 2, predators are heading towards the infected prey, and as is shown in Figure 10, the whole domain becomes successively filled with a pattern formed of stripes. Note that in regions occupied by the prey before the arrival of predators the solution is constant (see Example 4), and in particular does not exhibit any formed pattern (in contrast to Example 2). In a sense this scenario illustrates how the model (2.15), under the appropriate choice of parameters, predicts the formation of spatial patterns among the prey upon arrival of the predators. Furthermore, we observe that a "front" of predators is moving into the domain initially occupied by prey only, which seems to move at a lower velocity than in Example 2. It is worth noting that the final distribution of prey corresponds to total S and I populations close to L^2 times the corresponding entries of E_5 given by (4.6), while as in previous cases the final population of predators is much higher than L^2 times the Y-component of E_5.
Example 6: Case Ⅱ with a random initial distribution of the predator.
This case is the analogue of Example 2, namely we impose the initial condition of Figure 4(c). In Figure 11 we display the dynamics of the model with this initial condition. We observe that at simulated time t = 50, some stripes and spots emerge as patterns in the distribution of each species. Comparing the successive solutions for t = 50, t = 100 and t = 200, we observe that once the stripes form, they grow steadily in time incorporating "spots", until they reach a certain arm length, and the spatial patterns become slightly distinct at t = 200 since at this time there are strips and spots. It is observed that these patterns differ from those obtained in Example 5, where only stripe-like patterns are formed. The observations corresponding to the evolution of \mathcal{I}(S), \mathcal{I}(I) and \mathcal{I}(Y) are analogous to those of Example 3.
Example 7
Here and in Examples 8 and 9 we use the values
(\beta, c) = (46, c = 10) \in R_4. | (4.7) |
The equilibrium points are E_1 = (1, 0, 0), E_2 and E_3 given by (4.5), and
E_4 = (0.6916269, 0.984517, 0.9845173), \quad E_5 = (0.3083731, 0.2208100, 2.2081005). |
The integral quantities for this case and Examples 8 to 10 are plotted in Figure 13.
As in Example 4, the parameters \beta and \mu together with the diffusion constants are not in the Turing space. Considering the initial condition of Figure 4(a), in this case no patterns are formed and the system rapidly tends to a constant equilibrium which is maintained in time, see Figure 12. For this case we observe a behavior similar to that of Example 4.
Example 8
This case is based on the same initial "triangular" scenario (Figure 4(b)) as Examples 2 and 5. The numerical solution (Figure 14) shows that the predators are heading towards the infected prey, and for t = 50 and t = 150 we observe that the distribution of the prey varies in comparison with the uniform distribution of Example 7 in those places were the predators are present. The final spatial configuration is marked by spots and stripes for the prey as well as for the predators, and remains in time. However, the structure of patterns that are formed differs from the results of Example 5. It is interesting to note that the stripes visible at t = 600 are still roughly aligned with the original "front" of predators separating the triangular region from the rest of the domain. The conclusions concerning the evolution of \mathcal{I}(S), \mathcal{I} (I) and \mathcal{I} (Y) (see Figure 13(c) and (d)) are similar to those for Example 5.
We now consider an analogue of Examples 3 and 6 corresponding to the initial configuration of Figure 4(c). In Figure 15 we display the dynamics of the model with this initial condition. At t = 50 we observe that some stripes and spots patterns emerge mixed in the distribution of each species. The pattern distribution is maintained over time after its formation. It is observed that these patterns differ from those obtained in Examples 5, 6, and 8. As in Examples 3 and 6, the quantities \mathcal{I} (S), \mathcal{I} (I) and \mathcal{I} (Y) very quickly attain constant values (see Figures 13(e) and (f)).
Example 10: Effect of varying parameter c.
In this example, a constant value is considered for \beta = 48 and in combination with the three different values c = 4, c = 6 and c = 10 (all pairs (48, c) belong, again, to R_4, see Figure 1). For these three pairs a variation is obtained in the formation of patterns for the different values of c. The initial datum is given by Figure 4(c). In Figure 16 the corresponding numerical results are shown. It turns out that with increasing c the species tend to form more filament-like rather than spot-like spatial structures. Moreover, there is a marked difference in behavior of \mathcal{I}(S), \mathcal{I} (I) and \mathcal{I} (Y), as can be inferred from Figures 13(g) to (l). Note that the steady-state value of \mathcal{I} (I) decreases with increasing c, as does the steady-state value of \mathcal{I}(Y).
In Example 11, we investigate the sensitivity of (2.15) to the variation of discretization parameter \Delta x = L/M. We take L = 25 and the model parameters (2.9), (4.4) as in Examples 4 to 6. The initial datum is a random distribution (similar to Figure 4(c)) defined for a 50 \times 50 discretization, which is also utilized for finer discretizations with M = 100, 200, and 400, so the initial condition is exactly the same in all cases. We compute the solution at time t = 15 until we obtain a steady state. The results are displayed in Figure 17. It is observed that the resolution in the solution is improved as \Delta x is reduced, and that the steady-state solution is apparently the same (modulo, of course, sharpness of resolution) for all values of M. These results do not provide a rigorous convergence proof but indicate that the numerical simulator is reliable.
Finally, in Example 12 we utilize the same scenario as in Example 11, and now fix the spatial discretization M = 400 but vary the parameter \varepsilon, that is the radius of the convolution kernel of the non-local velocity function (see (2.17)). The corresponding results are given in Figures 18 and 19. We observe that the results for the prey compartments S and I are practically the same for all values of \varepsilon considered, while the prey distribution depends appreciably on \varepsilon. As \varepsilon decreases, the regions in which predators concentrate become smaller and the densities become higher. Moreover, Figures 19(b), (d), (f), and (h) indicate a decrease of the steady state value of \mathcal{I} as \varepsilon is decreased.
We have shown that a relatively simple three-species model can produce complex spatio-temporal patterns of predators and prey. As is stated in Section 1.1, the treatment combines the spatio-temporal epidemic model of [45], the non-spatial three-species predator-prey model analyzed in [22], the nonlocal velocity function introduced in [16] and the numerical method developed in [11]. The numerical method is not fully analyzed herein, and certainly leaves potential for further improvements. For instance, its accuracy deserves further investigation particularly to establish that its order of convergence corresponds to its design order. Further, we use a second-order spatial discretization of the Laplacian and a second-order time-stepping, which limit the high-order accuracy of the fifth-order WENO spatial semidiscretization. Nevertheless, on the basis of our (limited) evidence of robustness of the scheme (provided by Examples 11 and 12 along with the fact that numerical solutions of Examples 1, 4 and 7 are consistent with those of [45], where, however, the domain \Omega has slightly different dimensions), the numerical results allow us to draw some conclusions and conjectures about solutions of (2.15). Let us first point out that long-time stable patterned configurations obtained in our numerical experiments are formed by stripes. This contrasts with the results in [16] obtained for a predator-prey model with one single prey species only, where the system tends to an asymptotic state with regular arrangements of spots of high predator concentrations, in agreement with the radial structure introduced by the non-local velocity function and where the diffusion caused by the Laplacian in the prey equation counterbalances the first-order non-local differential operator in the predator equation [16]. A similar tendency was observed in some scenarios of the eight-compartment epidemiological model studied in [11], where male individuals are supposed to move non-locally in response to the sampled density of their female counterparts. Furthermore, it is calling to attention that in our Example 12, the dominant mechanism of pattern formation seems to be the interaction between susceptible and infected prey, since the solution (see Figure 18) for the prey compartments S and Y is practically independent of \varepsilon. That the effect of predator location on the formation of the prey pattern should be weak, at least for the parameters (2.9) and (4.4), can probably be explained by the relative smallness of the predation term on the right-hand side of (2.15b). Finally, it would be interesting to investigate whether the invasion of predators into a domain initially occupied by prey solely, as in Examples 2, 5 and 8, can possibly be modeled by simpler, spatially one-dimensional version of (2.15). Clearly, a more in-depth mathematical analysis to describe the mechanisms that compel these and other phenomena is necessary.
With respect to further extensions, we mention that the spatio-temporal approach could also be applied to the model by Greenhalgh et al.[23] that generalizes (2.1) and [22] in the sense that the assumption that the predator eats infected prey only is replaced by the assumption that the susceptible and infected populations are both exposed to the predator but to varying degrees. Another model variant whose predictions should be compared with the findings of the present work arises from replacing the constant recruitment rate A of susceptible prey (see (2.1a) or (2.15a)) by a per capita growth rate, for instance by a logistic growth term as is done in [13,14,15,23,22,25,30,40]. Concerning the numerical scheme, further analysis could compare results with higher-order discretizations of the diffusion operator and time-stepping scheme.
RB is supported by Fondecyt project 1170473; and Centro CRHIAM Proyecto Conicyt/Fondap/15130015. PM is supported by Spanish MINECO project MTM2017-83942-P and Conicyt (Chile), project PAI-MEC, folio 80150006. LMV is supported by Fondecyt project 1181511. RB and LMV are also supported by BASAL pro-ject CMM, Universidad de Chile and Centro de Investigación en Ingeniería Matemá-tica (CI^{\mathrm{2}}MA), Universidad de Concepción and the INRIA Associated Team "Efficient numerical schemes for non-local transport phenomena (NOLOCO; 2018--2020)". EG is supported by CONICYT scholarship. GC acknowledges financial support from grants NSF-IIS RAPID award #1518939, NSF grant 1318788 Ⅲ: Small: Data Management for Real-Time Data Driven Epidemic simulation, and Conicyt (Chile), project MEC80170119.
All authors declare no conflicts of interest in this paper.
[1] | L.J.S. Allen, B.M. Bolker, Y. Lou, and A.L. Nevai, Asymptotic profiles of the steady states for an SIS epidemic patch model, SIAM J. Appl. Math., 67 (2007), 1283–1309. |
[2] | J. Arino, Diseases in metapopulations. In Z. Ma, Y. Zhou, and J. Wu (Eds.), Modeling and Dynamics of Infectious Diseases, Higher Education Press, Beijing, 2009, 64–122. |
[3] | J. Arino, J.R. Davis, D. Hartley, R. Jordan, J.M. Miller, and P. van den Driessche, A multi-species epidemic model with spatial dynamics, Math. Med. Biol., 22 (2005), 129–142. |
[4] | U. Ascher, S. Ruuth, and J. Spiteri, Implicit-explicit Runge-Kutta methods for time dependent partial differential equations, Appl. Numer. Math., 25 (1997), 151–167. |
[5] | S. Boscarino, R. Bürger, P. Mulet, G. Russo, and L.M. Villada, Linearly implicit IMEX Runge- Kutta methods for a class of degenerate convection-diffusion problems, SIAM J. Sci. Comput., 37 (2015), B305–B331. |
[6] | S. Boscarino, F. Filbet, and G. Russo, High order semi-implicit schemes for time dependent partial differential equations, J. Sci. Comput., 68 (2016), 975–1001. |
[7] | S. Boscarino, P.G. LeFloch, and G. Russo, High-order asymptotic-preserving methods for fully nonlinear relaxation problems, SIAM J. Sci. Comput., 36 (2014), A377–A395. |
[8] | S. Boscarino and G. Russo, On a class of uniformly accurate IMEX Runge-Kutta schemes and applications to hyperbolic systems with relaxation, SIAM J. Sci. Comput., 31 (2009), 1926–1945. |
[9] | S. Boscarino and G. Russo, Flux-explicit IMEX Runge-Kutta schemes for hyperbolic to parabolic relaxation problems, SIAM J. Numer. Anal., 51 (2013), 163–190. |
[10] | F. Brauer and C. Kribs, Dynamical Systems for Biological Modeling: An Introduction, CRC Press, Boca Raton, FL, USA, 2016. |
[11] | R. Bürger, G. Chowell, E. Gavilán, P. Mulet, and L.M. Villada, Numerical solution of a spatiotemporal gender-structured model for hantavirus infection in rodents, Math. Biosci. Eng., 15 (2018), 95–123. |
[12] | R. Bürger, G. Chowell, P. Mulet, and L.M. Villada, Modelling the spatial-temporal progression of the 2009 A/H1N1 influenza pandemic in Chile, Math. Biosci. Eng., 13 (2016), 43–65. |
[13] | J. Chattopadhyay and O. Arino, A predator-prey model with disease in the prey, Nonlin. Anal., 36 (1999), 747–766. |
[14] | J. Chattopadhyay and N. Bairagi, Pelicans at risk in Salton sea-an eco-epidemiological model, Ecol. Model., 136 (2001), 103–112. |
[15] | J. Chattopadhyay, P.D.N. Srinivasu, and N. Bairagi, Pelicans at risk in Salton sea-an ecoepidemiological model-II, Ecol. Model., 167 (2003), 199-211. |
[16] | R.M. Colombo and E. Rossi, Hyperbolic predators versus parabolic preys, Commun. Math. Sci., 13 (2015), 369–400. |
[17] | M. Crouzeix, Une méthode multipas implicite-explicite pour l'approximation des équations d'évolution paraboliques, Numer. Math., 35 (1980), 257–276. |
[18] | O. Diekmann, H. Heesterbeek, and T. Britton, Mathematical Tools for Understanding Infectious Disease Dynamics, Princeton Series in Theoretical and Computational Biology, Princeton University Press, 2012. |
[19] | R. Donat and I. Higueras, On stability issues for IMEX schemes applied to 1D scalar hyperbolic equations with stiff reaction terms, Math. Comp., 80 (2011), 2097–2126. |
[20] | L. Edelstein-Keshet, Mathematical Models in Biology, reprint, SIAM, 2005. |
[21] | I.M. Foppa, A Historical Introduction to Mathematical Modeling of Infectious Diseases, Academic Press, London, UK, 2016. |
[22] | D. Greenhalgh and M. Haque, A predator-prey model with disease in the prey species only, Math. Meth. Appl. Sci., 30 (2007), 911–929. |
[23] | D. Greenhalgh, Q.J.A. Khan, and J.S. Pettigrew, An eco-epidemiological predator-prey modelmwhere predators distinguish between susceptible and infected prey, Math. Meth. Appl. Sci., 40 (2017), 146–166. |
[24] | K.P. Hadeler and H.I. Freedman, Predator-prey populations with parasitic infection, J. Math. Biol., 27 (1989), 609–631. |
[25] | H.W. Hethcote, W. Wang, L. Han, and Z. Ma, A predator-prey model with infected prey, Theor. Population Biol., 66 (2004), 259–268. |
[26] | G.S. Jiang and C.W. Shu, Efficient implementation of weighted ENO schemes, J. Comput. Phys., 126 (1996), 202–228. |
[27] | Y. Katznelson, An Introduction to Harmonic Analysis, Third Ed., Cambridge University Press, Cambridge, UK, 2004. |
[28] | C.A. Kennedy and M.H. Carpenter, Additive Runge-Kutta schemes for convection-diffusionreaction equations, Appl. Numer. Math., 44 (2003), 139–181. |
[29] | Y. Kuang and E. Beretta, Global qualitative analysis of a ratio-dependent predator-prey system, J. Math. Biol., 36 (1998), 389–406. |
[30] | K. Kundu and J. Chattopadhyay, A ratio-dependent eco-epidemiological model of the Salton Sea, Math. Meth. Appl. Sci., 29 (2006), 191–207. |
[31] | P.H. Leslie and J.C. Gower, The properties of a stochastic model for the predator-prey type of interaction between two species, Biometrika, 47 (1960), 219–234. |
[32] | W.M. Liu, H.W. Hethcote, and S.A. Levin, Dynamical behavior of epidemiological models with nonlinear incidence rates, J. Math. Biol., 25 (1987), 359–380. |
[33] | W.M. Liu, S.A. Levin, and Y. Iwasa, Influence of nonlinear incidence rates upon the behavior of SIRS epidemiological models, J. Math. Biol., 23 (1986), 187–204. |
[34] | X.D. Liu, S. Osher, and T. Chan, Weighted essentially non-oscillatory schemes, J. Comput. Phys., 115 (1994), 200–212. |
[35] | H. Malchow, S.V. Petrovskii, and E. Venturino, Spatial Patterns in Ecology and Epidemiology: Theory, Models, and Simulation. Chapman & Hall/CRC, Boca Raton, FL, USA, 2008. |
[36] | J.D. Murray, Mathematical Biology II: Spatial Models and Biomedical Applications. Third Edition. Springer, New York, 2003. |
[37] | A. Okubo and S.A. Levin, Diffusion and Ecological Problems: Modern Perspectives. Second Edition, Springer-Verlag, New York, 2001. |
[38] | L. Pareschi and G. Russo, Implicit-Explicit Runge-Kutta schemes and applications to hyperbolic systems with relaxation, J. Sci. Comput., 25 (2005), 129–155. |
[39] | E. Rossi and V. Schleper, Convergence of a numerical scheme for a mixed hyperbolic-parabolic system in two space dimensions, ESAIM Math. Modelling Numer. Anal., 50 (2016), 475–497. |
[40] | R. Sarkar, J. Chattopadhyay, and N. Bairagi, Effects of environmental fluctuation on an ecoepidemiological model of the Salton Sea, Environmetrics, 12 (2001), 289–300. |
[41] | L. Sattenspiel, The Geographic Spread of Infectious Diseases: Models and Applications, Princeton Series in Theoretical and Computational Biology, Princeton University Press, 2009. |
[42] | C.W. Shu and S. Osher, Efficient implementation of essentially non-oscillatory shock-capturing schemes, II, J. Comput. Phys., 83 (1988), 32–78. |
[43] | S.W. Smith, Digital Signal Processing: A Practical Guide for Engineers and Scientists. Demystifying technology series: by engineers, for engineers. Newnes, 2003. |
[44] | S.H. Strogatz, Nonlinear Dynamics and Chaos: with Applications to Physics, Biology, Chemistry, and Engineering, Second Edition, CRC Press, Boca Raton, FL, 2015. |
[45] | G. Q. Sun, Pattern formation of an epidemic model with diffusion, Nonlinear Dynamics, 69 (2012), 1097–1104. |
[46] | P. van den Driessche, Deterministic compartmental models: extensions of basic models. In F. Brauer, P. van den Driessche, and J. Wu (Eds.), Mathematical Epidemiology, Springer-Verlag, Berlin, 2008, 147–157. |
[47] | P. van den Driessche, Spatial structure: patch models. In F. Brauer, P. van den Driessche and J.Wu (Eds.), Mathematical Epidemiology, Springer-Verlag, Berlin, 2008, 179–189. |
[48] | E. Vynnycky and R.E. White, An Introduction to Infectious Disease Modelling, Oxford University Press, 2010. |
[49] | J. Wu, Spatial structure: partial differential equations models. In F. Brauer, P. van den Driessche and J. Wu (Eds.), Mathematical Epidemiology, Springer-Verlag, Berlin, 2008, 191–203. |
[50] | P. Yu, Closed-form conditions of bifurcation points for general differential equations, Internat. J. Bifur. Chaos Appl. Sci. Engrg., 15 (2005), 1467–1483. |
[51] | Y.T. Zhang and C.W. Shu, ENO and WENO schemes. Chapter 5 in R. Abgrall and C.W. Shu (eds.), Handbook of Numerical Methods for Hyperbolic Problems Basic and Fundamental Issues. Handbook of Numerical Analysis vol. 17, North Holland (2016), 103–122. |
[52] | X. Zhong, Additive semi-implicit Runge-Kutta methods for computing high-speed nonequilibrium reactive flows, J. Comput. Phys., 128 (1996), 19–31. |
1. | J. Alba-Pérez, J. E. Macías-Díaz, A positive and bounded convergent scheme for general space-fractional diffusion-reaction systems with inertial times, 2020, 0020-7160, 1, 10.1080/00207160.2020.1802018 | |
2. | Raimund Bürger, Rafael Ordoñez, Mauricio Sepúlveda, Luis Miguel Villada, Numerical analysis of a three-species chemotaxis model, 2020, 80, 08981221, 183, 10.1016/j.camwa.2020.03.008 | |
3. | Chi-Wang Shu, Essentially non-oscillatory and weighted essentially non-oscillatory schemes, 2020, 29, 0962-4929, 701, 10.1017/S0962492920000057 | |
4. | Raimund Bürger, Elvis Gavilán, Daniel Inzunza, Pep Mulet, Luis Miguel Villada, Exploring a Convection–Diffusion–Reaction Model of the Propagation of Forest Fires: Computation of Risk Maps for Heterogeneous Environments, 2020, 8, 2227-7390, 1674, 10.3390/math8101674 | |
5. | J.E. Macías-Díaz, Simple efficient simulation of the complex dynamics of some nonlinear hyperbolic predator–prey models with spatial diffusion, 2020, 77, 0307904X, 1373, 10.1016/j.apm.2019.09.003 | |
6. | Salihu S. Musa, Shi Zhao, Daozhou Gao, Qianying Lin, Gerardo Chowell, Daihai He, Mechanistic modelling of the large-scale Lassa fever epidemics in Nigeria from 2016 to 2019, 2020, 493, 00225193, 110209, 10.1016/j.jtbi.2020.110209 | |
7. | Alberto d’Onofrio, Malay Banerjee, Piero Manfredi, Spatial behavioural responses to the spread of an infectious disease can suppress Turing and Turing–Hopf patterning of the disease, 2020, 545, 03784371, 123773, 10.1016/j.physa.2019.123773 | |
8. | Raimund Bürger, Elvis Gavilán, Daniel Inzunza, Pep Mulet, Luis Miguel Villada, Implicit-Explicit Methods for a Convection-Diffusion-Reaction Model of the Propagation of Forest Fires, 2020, 8, 2227-7390, 1034, 10.3390/math8061034 | |
9. | J.E. Macías-Díaz, Héctor Vargas-Rodríguez, Analysis and simulation of numerical schemes for nonlinear hyperbolic predator–prey models with spatial diffusion, 2021, 03770427, 113636, 10.1016/j.cam.2021.113636 | |
10. | Rinaldo M. Colombo, Elena Rossi, Well‐posedness and control in a hyperbolic–parabolic parasitoid–parasite system, 2021, 147, 0022-2526, 839, 10.1111/sapm.12402 | |
11. | Nabeela Anwar, Shafaq Naz, Muhammad Shoaib, Reliable numerical treatment with Adams and BDF methods for plant virus propagation model by vector with impact of time lag and density, 2022, 8, 2297-4687, 10.3389/fams.2022.1001392 | |
12. | Bing Yang, Jizeng Wang, Xiaojing Liu, Youhe Zhou, High-order adaptive multiresolution wavelet upwind schemes for hyperbolic conservation laws, 2024, 269, 00457930, 106111, 10.1016/j.compfluid.2023.106111 | |
13. | Ritwika Mondal, Yasuhiro Takeuchi, Dipak Kesh, Debasis Mukherjee, Dynamical study on volatiles signaling in plant disease and pest-natural enemy interaction, 2025, 11, 2363-6203, 10.1007/s40808-024-02248-0 |