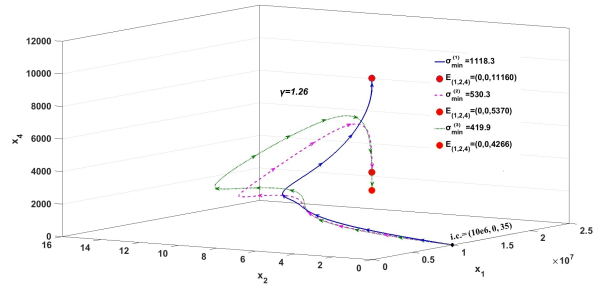
Citation: Konstantin E. Starkov, Giovana Andres Garfias. Dynamics of the tumor-immune-virus interactions: Convergence conditions to tumor-only or tumor-free equilibrium points[J]. Mathematical Biosciences and Engineering, 2019, 16(1): 421-437. doi: 10.3934/mbe.2019020
[1] | Alexander P. Krishchenko, Konstantin E. Starkov . The four-dimensional Kirschner-Panetta type cancer model: How to obtain tumor eradication?. Mathematical Biosciences and Engineering, 2018, 15(5): 1243-1254. doi: 10.3934/mbe.2018057 |
[2] | Prathibha Ambegoda, Hsiu-Chuan Wei, Sophia R-J Jang . The role of immune cells in resistance to oncolytic viral therapy. Mathematical Biosciences and Engineering, 2024, 21(5): 5900-5946. doi: 10.3934/mbe.2024261 |
[3] | Maria Vittoria Barbarossa, Christina Kuttler, Jonathan Zinsl . Delay equations modeling the effects of phase-specific drugs and immunotherapy on proliferating tumor cells. Mathematical Biosciences and Engineering, 2012, 9(2): 241-257. doi: 10.3934/mbe.2012.9.241 |
[4] | Yuyang Xiao, Juan Shen, Xiufen Zou . Mathematical modeling and dynamical analysis of anti-tumor drug dose-response. Mathematical Biosciences and Engineering, 2022, 19(4): 4120-4144. doi: 10.3934/mbe.2022190 |
[5] | Ge Song, Tianhai Tian, Xinan Zhang . A mathematical model of cell-mediated immune response to tumor. Mathematical Biosciences and Engineering, 2021, 18(1): 373-385. doi: 10.3934/mbe.2021020 |
[6] | Dan Liu, Shigui Ruan, Deming Zhu . Stable periodic oscillations in a two-stage cancer model of tumor and immune system interactions. Mathematical Biosciences and Engineering, 2012, 9(2): 347-368. doi: 10.3934/mbe.2012.9.347 |
[7] | Yuting Ding, Gaoyang Liu, Yong An . Stability and bifurcation analysis of a tumor-immune system with two delays and diffusion. Mathematical Biosciences and Engineering, 2022, 19(2): 1154-1173. doi: 10.3934/mbe.2022053 |
[8] | K. E. Starkov, Svetlana Bunimovich-Mendrazitsky . Dynamical properties and tumor clearance conditions for a nine-dimensional model of bladder cancer immunotherapy. Mathematical Biosciences and Engineering, 2016, 13(5): 1059-1075. doi: 10.3934/mbe.2016030 |
[9] | Urszula Foryś, Yuri Kheifetz, Yuri Kogan . Critical-Point Analysis For Three-Variable Cancer Angiogenesis Models. Mathematical Biosciences and Engineering, 2005, 2(3): 511-525. doi: 10.3934/mbe.2005.2.511 |
[10] | H. J. Alsakaji, F. A. Rihan, K. Udhayakumar, F. El Ktaibi . Stochastic tumor-immune interaction model with external treatments and time delays: An optimal control problem. Mathematical Biosciences and Engineering, 2023, 20(11): 19270-19299. doi: 10.3934/mbe.2023852 |
The elaboration of mathematical models that describe interactions between cancer cells and the immune system is an actively developing field in mathematical medicine. One of the important goals of this modeling is to analyze the ultimate dynamics which is necessary for monitoring the long-term tumor dynamics and, in the most optimistic case, for the creation of tumor eradication algorithms as well. Oncolytic virotherapy of cancer attracts attention of many researchers, see e.g. papers [1,2,3,4,5,6,8,14] and so on. Eftimie et al. in [5] described and studied the seven-dimensional model characterizing behavior of the cancer population in the case when the dual immunization protocol, by vaccine viruses and oncolytic viruses, is applied. They obtained local stability conditions for equilibrium points of their model and numerically investigated multi-stability phenomenon which may exist in the case of four types of equilibrium points. Later, in [6], Eftimie et al. presented the five-dimensional system which is obtained by a simplification of the model from [5]. This system was derived from an experimental protocol of the paper [3] and can be written as
⋅x1=rx1(1−x1+x2κ)−a1x1x5b1+x1−a2x1x4b2+x4⋅x2=a1x1x5b1+x1−δx2−a2x2x4b2+x4⋅x3=p1x3x5b3+x5(1−x3m)⋅x4=p2x3x5b3+x5−a3x4−a4x1x4+σ⋅x5=δβx2−γx5. | (1.1) |
Below the system (1.1) is considered on the nonnegative orthant R5+,0 and it is denoted by x1/x2/x3 /x4/ x5 the density of uninfected tumor cells/ infected tumor cells/ memory cells/ effector immune cells/ oncolytic (Vesicular Stomatitis) virus particles (OVPs) correspondingly. Parameters are supposed to be positive excepting σ, σ≥0, and are defined as follows: r is proliferation rate for uninfected tumor cells; κ is carrying capacity for tumor cells; a1 is infection rate of tumor cells with the oncolytic virus; b1 is half-saturation constant for the tumor cells infected with the oncolytic virus; δ is rate at which the oncolytic virus kills the tumor cells; a2 is lysis rate of tumor cells (infected and uninfected) by the immune cells; b2 is half-saturation constant for the effector cells that support half the maximum killing rate; p1 is proliferation rate of memory cells following secondary encounter with tumor antigens carried by virus particles; b3 is half-saturation constant of viral antigens that induce half the maximum proliferation rate of immune cells; m is carrying capacity for memory cells; p2 is rate at which memory cells become effector cells following secondary encounter with tumor antigens carried by virus particles; effector cells die at rate a3; a4 is inactivation rate of effector immune cells by tumor cells; β is number of OVPs released from an infected cell, capable of forming plaques; γ is decay rate for the concentration of OVPs in the blood; σ is the influx rate into the lymphoid tissue at which the naive cells become effector cells, see [5]. It is pointed out that σ has sufficiently small values and may be neglected, [6]. In this paper it is assumed that the rate σ reflects the presence of the influx of immune cells from the external source and the dependence of global behavior of (1.1) is explored with a change in σ.
Authors of [6] studied only the case σ=0. They found equilibrium points and performed local stability analysis. As well, authors of [6] fulfilled numerical studies transient and asymptotic behavior of the system which are based on using bifurcation diagrams. In particular, they showed the existence of periodic orbits and a chaotic attractor for some values of parameters. Nevertheless, the rigorous exploration of long time-dynamics is of interest for better understanding potentialities of cancer virotherapy described by equations (1.1).
The purpose of this paper and its novelty consist in studies of the case of convergence dynamics of (1.1), with σ≥0. In particular, conditions are found under which every trajectory in the nonnegative orthant tends to one of equilibrium points. It is worth to remind that systems with convergence dynamics have been investigated in articles of Hirsch; R.A. Smith; Li and Muldowney, see [7,15,16], in the broader perspective of studies of competitive or cooperative systems; higher dimensional generalizations of Bendixon's criterion and Dulac's criterion. Our results are based on the LaSalle theorem and the localization method of compact invariant sets (LMCIS), see [10,11]. It is worth to mention that the LMCIS has been efficiently utilized in papers [12,13,17,18,19,20] for analysis of ultimate dynamics of various cancer models.
The dynamics of (1.1) has some distinctive features: the unbounded set of equilibrium points, positive Lagrange stability of trajectories and several invariant planes that are useful in this analysis. In the case of a sufficiently large σ>0 it is found that (1.1) acquires a new global qualitative property consisting in the fact that all trajectories are attracted to the tumor-free plane x1=x2=0. This property may be characterized quantitatively as well. Namely, it is computed a bound σ(1)min called the first eradication bound such that if σ≥σ(1)min then each ω-limit set is located in x1=x2=0 plane. This signifies that the population of tumor cells is under control after sufficiently large time of observations. From the biological point of view, this case may correspond to the immunotherapy injection of some agent like IL-2 which is combined with virotherapy. Although the tumor may be cleared for σ≥σ(1)min, but the harmful side effects from an over-activated immune system may eliminate the benefits of tumor clearance. We propose an approach for reducing this risk. We show that there is the sequence of eradication bounds {σ(k)min},k=1,2,..,with the property that for σ≥σ(k)min each ω-limit set is located in x1=x2=0 plane. The value σ(k)min appears as the minimal positive root of some algebraic equation of degree k. The existence of σ(k)min is established in Theorem 5.1. The formula for σ(2)min is provided; here we have that σ(1)min>σ(2)min. It is demonstrated numerically that σ(2)min>σ(3)min. We expect that {σ(k)min} is a decreasing sequence. Figs. 1-3 show the tumor eradication dynamics with σ=σ(i)min,i=1,2,3.
In the present paper ultimate upper bounds are computed for variables of (1.1). The domain defined by these bounds contains all compact invariant sets including a chaotic attractor found in [6] for some values of parameters. Further, it is noted that in the case σ=0 tumor-free TF-equilibrium points are always locally unstable [6]. Main results of this paper are
1) Theorem 4.2 containing conditions under which the ω-limit set of any positive half trajectory in R5+ is either one of TF-equilibrium points or one of tumor-only TO-equilibrium points and
2) Propositions 3;4 and Theorem 5.1 in which we give minimal bounds for σ guaranteeing convergence dynamics of any positive half trajectory in R5+ to one of tumor-only TO-equilibrium points.
Therefore conditions of this paper may be utilized for a prediction of the possible health scenario for a sufficiently long time of observations.
The results of this work are stated in terms of inequalities imposed on parameters of the model. This may be important in applications, because in medical models, as a rule, biological parameters are known in a certain range.
The present paper is organized as follows. Helpful auxiliary results are provided in Section 2 and in Appendix. In Section 3 ultimate dynamics of the system (1.1) is studied via computing ultimate bounds in case σ≥0. These bounds form a domain in which the chaotic attractor is detected for some values of parameters given in [6]. Further, Section 4 contains conditions under which (1.1) possesses convergent dynamics to one of TO- or TF-equilibrium points in case σ=0. In Section 5 we describe the case when the immunotherapy is utilized σ>0. Here we show that the tumor clearance phenomenon may be achieved with a sufficiently large value of σ. The tumor eradication bounds for σ are derived. Section 6 contains concluding remarks. Appendix contains the derivation of the cubic equation for finding σ(3)min.
With the goal of describing helpful results the following objects are introduced:
1) a nonlinear system
˙x=v(x) | (2.1) |
where v is a C1−differentiable vector field; x∈Rn is the state vector;
2) a C1−differentiable function h(x) such that h is not the first integral of (2.1);
3) the set S(h):={x∈Rn∣Lvh(x)=0} where Lvh is the Lie derivative;
4) values hinf:=inf{h(x)∣x∈S(h)}; hsup:=sup{h(x)∣x∈S(h)}.
If it is set up the localization problem of compact invariant sets contained in the set M⊂Rn then the sets
S(h;M):=S(h)∩M={x∈M∣Lvh(x)=0};hinf(M):=inf{h(x)∣x∈S(h;M)};hsup(M):=sup{h(x)∣x∈S(h;M)}. |
are defined.
Assertion 1 [9,10,11] For any h(x)∈C1(Rn) all compact invariant sets of the system (2.1) located in M are contained in the localization set K(h;M) defined by the formula K(h;M)={x∈M∣hinf(M)≤h(x)≤hsup(M)} as well. If M∩S(h)=∅ then there are no compact invariant sets located in M.
The function h mentioned in this assertion is called localizing.
Below the following notations are used: if the system is examined on the positive orthant M=Rn+,0 then K(h):=K(h;M). If the system is considered on the domain M:=Rn+,0∩{x5>0} then this M is indicated.
Further, it is utilized
Assertion 2 [12]. Let h be a differential function which is bounded below on the set D⊂Rn and Lvh(x)∣D≤−ε for some ε>0. Then the solution φ(x,t) of the system (2.1), with initial condition φ(x,0)=x∈D, leaves the set D in a finite time.
To formulate the following useful result, the nonautonomous system
˙y=G(y,t),y∈Rn, | (2.2) |
is introduced; here G is a C1−differentiable vector function. The system (2.2) is called asymptotically autonomous with the limit system (2.1) if G(x,t)→v(x), with t→∞, for any compact subset in Rn, see e.g. [21].
Theorem 2.1. (Markus), see e.g. [21]. Let us consider systems (2.1) and (2.2) in case n=2. Now if Ω is the ω -limit set of a forward bounded solution x of (2.2) then Ω either contains equilibria of (2.1) or Ω is the union of periodic orbits of (2.1).
The Markus theorem is applicable to the nonnegative orthant R2+,0 in case when R2+,0 is a positively invariant domain.
The planes x1=0; x1=x2=0; x3=0; x3=m; x2=x5=0 are invariant.
Further, if σ=0 then there are four types of equilibrium points, [6]:
a) TF-equilibrium points are given by E1:=(0,0,e13,0,0)T;e13∈R1+,0;
b) TO-equilibrium points are given by E2:=(κ,0,e23,0,0)T;e23∈R1+,0;
c) a tumor with virus but no immune response TV-equilibrium point is given by E3:=(e31,e32,0,0,e35)T, with
e31=b1γa1β−γ;e32=rδβ(κ−e31)(b1+e31)a1δβκ+b1rγ+e31rγ;e35=e35γβδ; |
d) an internal tumor virus ITV-equilibrium point is given by E4:=(e41,e42,m,e44,e45)T, with components e4j,j=1,2,4,5, defined by very cumbersome formulas and omitted here.
Since TF-equilibrium points and TO-equilibrium points form an unbounded set the system (1.1) does not possess the bounded positively invariant domain.
It is noted that if σ=0 then each TF-equilibrium point (0,0,e13,0,0)T is connected with TO-equilibrium point (κ,0,e13,0,0)T by a heteroclinic orbit which is located in the invariant plane xi=0,i=2,4,5;x3=e13 and is an arc directed from (0,0,e13,0,0)T to (κ,0,e13,0,0)T. So heteroclinic orbits cover half strip x3≥0,x2=x4=x5=0.
Next, upper ultimate bounds are got for all state variables of the system (1.1) with help of computing the localization domain containing all compact invariant sets.
1. Bound x1max. The localizing function h1=x1 is applied. Then
S(h1)∩{x1>0}={r(1−x1+x2κ)=a1x5b1+x1+a2x4b2+x4≥0}⊂{r(1−x1+x2κ)≥0}. |
Therefore the localization set K1max:={0≤x1≤κ:=x1max} is derived.
2) Bound x2max. Here the function h2=x1+x2 is utilized. Then
Lfh2=rx1(1−x1+x2κ)−a2(x1+x2)x4b2+x4−δx2 |
Therefore the inequality
δ(x1+x2)≤(δ+r)x1−rκx21 |
holds on S(h2). Considering the last inequality within K1max the following inequality holds
δ(x1+x2)≤ζ:={δκ,δ>rκ(δ+r)24r,δ≤r}. |
Thus the localization set K21:={h2≤h2max:=ζδ−1} is derived. So the upper bound x2max given in
K2max:={x2≤ζδ−1:=x2max} | (3.2) |
is obtained.
3) Bound x5max. Here using h3=x5 it is noted that
S(h3)∩K2max⊂{x5≤δβγx2max} |
and so the localization set with the bound x5max is obtained:
K5max:={x5≤x5max:=δβγx2max}. | (3.3) |
4) The bound x3max is computed with respect to the domain R5+,0∩{x5>0}. The function h4=x3 provides the localization set:
K3max(h4,R5+,0∩{x5>0}):={0≤x3≤m;x5>0}. |
5) The bound x4max is computed with respect to the domain R5+,0∩{x5>0}. Here the function h5=x4 is applied. Then
Lfh5=p2x3x5b3+x5−a3x4−a4x1x4+σ |
and therefore the set S(h5)∩K5max∩{x5>0} is contained in the set defined by the inequality
x4≤p2x3x5a3(b3+x5)+σa3≤x4max:=p2mx5maxa3(b3+x5max)+σa3. |
Therefore the localization set:
K4max(h5,R5+,0∩{x5>0}):={0≤x4≤x4max;x5>0} |
is got.
As a result, it is established
Proposition 1. All compact invariant sets inR5+,0∩{x5>0} are located in the domain
Π:=∩i=1,2,5Kimax∩K3max(h4,R5+,0∩{x5>0})∩K4max(h5,R5+,0∩{x5>0}). |
Let φ(x,t) be a solution of (1.1), with φ(x,0)=x for all x. Further, the following fact is used in this paper:
Lemma 3.1. Let σ≥0. The system (1.1) is positively Lagrange stable in R5+,0, i.e. for any point x∈R5+,0 the time functions φi(x,t),t≥0, i=1,...,5, are upper bounded. So there are no escaping to infinity trajectories in positive time.
Proof. 1. Taking the function h1=x1 and the domain D1={x1≥κ+ε1}∩R5+,0 for ε1>0 it is easy to see that
Lfh1∣D1≤−rε1−rε21κ<0. |
So applying Assertion 2 it is established that each trajectory leaves D1 in a finite time. So φ1(x,t) is upper bounded as a time function.
2. Since x3=m is an invariant plane any trajectory with an initial condition satisfying the inequality x3≤m has the property that φ3(x,t) is upper bounded as a time function. From the other side, if a trajectory has an initial condition satisfying x3>m then since ˙x3(t)≤0 on the domain x3>m then φ3(x,t) is upper bounded as a time function.
3. We have in R5+,0 that
˙x4≤−a3x4+p2φ3(x,t)+σ. |
Integrating this inequality we obtain that the function φ4(x,t) is upper bounded.
4. Taking the function h2=x1+x2 and the domain
D2={x1+x2≥h2max+ε2;x1<κ+ε1}∩R5+,0, |
for ε2>0 it is easy to see that
Lfh2∣D2≤−rε1−rε21κ−a2(h2max+ε2)inft>0φ4(x,t)a2+φ4(x,t)<0. |
So applying Assertion 2 it is established that each trajectory leaves D2 in a finite time. So both of φi(x,t),i=1,2, are upper bounded time functions.
5. At last, it follows from integration of ˙x5 with the upper bounded time function φ2(x,t) that φ5(x,t) is the upper bounded time function as well.
The main purpose of this section is to present attraction conditions to either TF- equilibrium points or TO- equilibrium points in case σ=0. All statements are established in the form of simple inequalities imposed on the decay rate γ of VSV particles in the blood. Here γ can be considered as a control parameter. Firstly, it is introduced a system obtained from (1.1) by a restriction on the invariant plane x3=x4=0:
⋅x1=rx1(1−x1+x2κ)−a1x1x5b1+x1;⋅x2=a1x1x5b1+x1−δx2;⋅x5=δβx2−γx5. | (4.1) |
By g it is denoted its vector field. This system has the following equilibrium points
e1=(0,0,0)T;e2=(κ,0,0)T;e3=(e31,e32,e33)T, |
with
e31=b1γa1β−γ;e32=rb1γ(κ−e31)(e31+γ)rb1γ(e31+γ)+e31κa1βδ;e33=δβγe32. |
Before considering the system (1.1) it is formulated
Lemma 4.1. The system (4.1) has the localization domainΠ1 in R3+,0 defined by
x1≤x1max:=κ;x2≤x2max;x5≤x5max, |
where x2max is defined by (3.2). This polytope contains all compact invariant sets and the attracting set of (4.1).
These bounds are derived as in case of Proposition 1.
Proposition 2. Assume that
γ>γmin:=a1βκκ+b1. | (4.2) |
Then each trajectory of the system (4.1) in R3+,0 tends to e1 or e2.
Proof. Let us apply the function h6=x2+β−1x5. Then
Lgh6=(a1x1b1+x1−γβ)x5. | (4.3) |
It is noted that all ω-limit sets of the system (4.1) are located in Π1. So one may examine the equality (4.3) on Π1 and proceed as follows
Lgh6∣Π1≤(a1κb1+κ−γβ)x5∣Π1≤0. |
By using Lemma 4.1 and the LaSalle theorem it is established that the ω-limit set of any point of the system (4.1) is not empty and belongs to the set {x5=0}.Therefore, in order to analyze the ultimate dynamics of (4.1) it should be examined the ultimate dynamics of the system
⋅x1=rx1(1−x1+x2κ)−a1x1φ5(x,t)b1+x1⋅x2=a1x1φ5(x,t)b1+x1−δx2, | (4.4) |
with the input φ5(x,t) satisfying the condition
limt→∞φ5(x,t)=0. |
The system (4.4) is asymptotically autonomous with the limit system
⋅x1=rx1(1−x1+x2κ)⋅x2=−δx2, | (4.5) |
All solutions of the system (4.5) in R2+,0 are convergent to the equilibrium point (κ,0)T. In virtue of the Markus theorem, all solutions of the system (4.4) are convergent to the equilibrium point (κ,0)T. Therefore any solution of the system (4.1) tends to e1 or e2.
Remark 1. If the inequality
1<a1βγ<1+b1κ |
then there is the third equilibrium e3 which is not contained in R3+,0 because κ−e31<0 and, therefore, e32<0. It follows from formulas for equilibrium points.
Now it is established
Theorem 4.2. Suppose that (4.2) is fulfilled. Then theω -limit set in R5+,0 is either one of TF-equilibrium points or one of TO-equilibrium points.
It should be noted that the condition (4.2) is the condition of local stability of TO-equilibrium points, see [6].
Proof. Let us apply the function h6=x2+β−1x5. In this case
Lfh6=a1x1x5b1+x1−γβx5−a2x2x4b2+x4. | (4.6) |
Since all ω-limit sets of the system (1.1) in R5+,0∩{x5>0} are nonempty and are located in K1max one may restrict the equality (4.6) on K1max and proceed as follows
Lfh6∣K1max≤{(a1κb1+κ−γβ)x5−a2x2x4b2+x4}∣K1max≤0. |
Using the condition (4.2) and the LaSalle theorem it is possible to conclude that any ω-limit set belongs to the set {x2=x5=0}∪{x4=x5=0}. Hence, any ω-limit set is one of TF-equilibrium points or TO-equilibrium points.
Below everwhere we take the case a2>r. We shall utilize the notation x(1)1max:=x1max.
Proposition 3. Then all compact invariant sets are located in the domain K(1)4min∩K(2)1max, with
K(1)4min:={x4≥x(1)4min:=σa3+a4κ};K(2)1max:={x1≤x(2)1max:=κ−a2κx(1)4minr(b2+x(1)4min)}.{} |
Here these formulas are valid under condition
σ<σ(1)min:=b2r(a3+a4κ)a2−r. | (5.1) |
2) Further, if
σ≥σ(1)min | (5.2) |
then all ω -limit sets are located in the plane x1=0. Moreover, all ω -limit sets are located in the plane x1=0;x2=0 as well.
The value of σ(1)min is called the first eradication bound.
Proof. 1). Using the function h5 one may obtain inequalities
σ≤p2x3x5b3+x5+σ=a3x4+a4x1x4≤(a3+a4κ)x4 |
within the set K1max∩S(h5) and, as a result, the localization set K(1)4min is got. Next,
˙x1∣x1>0≤(r−rκx1−a2x4b2+x4)∣x1>0 | (5.3) |
and the inequality (5.3) on the domain K(1)4min leads to the inequality
0<rκx1≤r−a2x(1)4minb2+x(1)4min. |
This implies the desirable assertion.
2) It follows from (5.3), the LaSalle theorem and the fact that the second equation of (1.1) with x1≡0 is globally asymptotically stable.
Now we discuss how we can decrease the value for σ for which the tumor clearance can be reached.
One may iterate this argument and obtain sequences of localization sets
K(1)4min⊂K(2)4min⊂…K(2)1max⊃K(3)1max⊃…, |
with
x(k)1max(σ):=κ−a2κx(k−1)4min(σ)r(b2+x(k−1)4min)(σ),k=3,4,… |
and
x(k)1max(σ)>x(k+1)1max(σ),k=1,2,... |
So similarly as above, we establish
Proposition 4. If for given value of the influx rate σ there is k such that
x(1)1max(σ)>x(2)1max(σ)>...>x(k−1)1max(σ)>0 |
but x(k)1max(σ)≤0 then we have the tumor clearance for this value of σ.
The minimal positive root of the equation (5.4)
x(k)1max(σ)=0 | (5.4) |
is called the k−th eradication bound which will be denoted by σ(k)min. In what follows, we examine the issue concerning the existence of a positive root of (5.4) for any k. Finding bounds for the value σ(k)min encounters computational difficulties and is not the subject of this study. We notice that though the function x(k)1max(σ) may possess more than one positive root, this fact does not contradict the biological meaning of the use of the treatment σ. This is because in Propositions 3 and 4 only sufficient conditions of the tumor clearance are given.
Below we shall utilize notations
Ak(σ)=Pk(σ)Qk(σ)=x(k)1max(σ);Bk(σ)=x(k)4min(σ) | (5.5) |
and for simplicity we shall omit σ for functions introduced in (5.5). Further, our goal is to establish
Theorem 5.1. The equation (5.4) for k≥2 has at least one positive root.
Proof. Firstly, we prove
Lemma 5.2. We have for any k≥2 that 1) degPk=degQk=k−1; 2) coefficients of Pk and Qk satisfy the following condition:
coefσ0(Pk)>0;coefσ0(Qk)>0;coefσk−1(Pk)<0;coefσk−1(Qk)>0. |
Proof. We shall prove this statement by induction on k. For k=2 this result is valid since
A2=P2Q2=κ−κa2B1r(b2+B1)=rb2κ(a3+a4κ)+σκ(r−a2)r[b2(a3+a4κ)+σ]. |
Suppose that this assertion is proven for k. Let us consider this assertion for k+1. Then
Ak+1=Pk+1Qk+1=κ−κa2Bkr(b2+Bk)=κrb2+Bkκ(r−a2)r(b2+Bk). | (5.6) |
Using the formula
Bk=σa3+a4Ak=σQka3Qk+a4Pk |
we proceed (5.6) as follows:
Ak+1=κrb2(a3Qk+a4Pk)+σQkκ(r−a2)r[b2(a3Qk+a4Pk)+σQk]. | (5.7) |
Now we get from the inductive hypothesis and the formula (5.7) that the first assertion of this lemma is valid and, besides, we notice that
coefσ0(Pk+1)=κrb2[a3coefσ0(Qk)+a4coefσ0(Pk)]>0;coefσ0(Qk+1)=rb2[a3coefσ0(Qk)+a4coefσ0(Pk)]>0;coefσk(Pk+1)=κ(r−a2)coefσk−1(Qk)<0;coefσk(Qk+1)=rcoefσk−1(Qk)>0. |
Therefore Lemma 5.2 is proven.
As a result, in virtue of Lemma 5.2 and the formula (5.7) we obtain the desirable assertion.
Now we compute the value σ(2)min. Then we have that
B2=σr(b2+B1)r(a3+a4κ)(b2+B1)−a2a4κB1. |
Examining the equation A3(σ)=0 we get the equation
rb2=B2(a2−r). | (5.8) |
For our convenience we introduce notations
α1=b2a2−r;α2=rb2(a3+a4κ)2;α3=r(a3+a4κ)−a2a4κ |
in (5.8) and after this we come to the equation
σ2+σ(b2(a3+a4κ)−α1α3)−α1α2=0. |
Its unique positive root has the form
σ+=α1α3−b2(a3+a4κ)2+√(α1α3−b2(a3+a4κ))24+α1α2 |
We notice that σ(2)min=σ+. Finally, by the routine computations omitted here it is easy to establish that
σ(1)min+b2(a3+a4κ)−α1α32>0 |
and, its square exceeds
(α1α3−b2(a3+a4κ))24+α1α2. |
So, we have that σ(1)min>σ(2)min and in case of using σ≥σ(2)min we still achieve the tumor eradication.
Now taking parameters from Table 2 [6], we demonstrate by calculations that the tumor eradication bounds σ(1)min;σ(2)min;σ(3)min are decreasing: σ(1)min=1118.3;σ(2)min=530.3;σ(3)min=419.9.
On Figures 1; 2; 3 we show the tumor eradication dynamics corresponding these values of σ at γ=1.26;1.46 and 1.66:
It should be said that one can get improved bounds for x2 and x5 with help of using formulas (3.2)-(3.3). In this case the function h2=x1+x2 it should be employed in the same way as above in the formula (3.1). So considering S(h2) within K(1)1max∩K(1)4min it is easy to see that this set is located in the set defined by the inequality
(δ+a2x4minb2+x4min)(x1+x2)≤ζ. |
Thus the localization set K22:={h2≤h2max:=ζδ−11} is obtained, with
δ1=δ+a2x4minb2+x4min. |
As a result, one can get upper bounds indicated in the formula
K(1)2max:={x2≤ζδ−11:=x(1)2max}, K(1)5max:={x5≤x(1)5max:=σβγx(1)2max}. |
Hence one may conclude:
Proposition 5. All compact invariant sets are located in the domain K(1)1max∩K(1)2max∩K(1)5max∩K(1)4min.
Convergence conditions for trajectories of the model (1.1) are presented in this paper. Such kind of ultimate dynamics may be important for predicting the patient's health scenario, its monitoring, correction and a proper application of treatments. For this purpose one may vary the parameters γ (the decay rate for the concentration of OVPs in the blood) and σ (the rate of influx of immune cells from the external source).
1) Ultimate upper bounds for x1-, x2-cells populations and for concentration of OVPs (x5) are obtained. Upper bounds for x3-, x4-cells populations are given provided we examine compact invariant sets outside the plane x5=0. As a result, it is shown that ultimate dynamics of the system (1.1) outside the plane x5=0 is contained in the domain defined by these bounds. Then it is established that in case σ>0 upper bounds for x1-, x2-, x5- variables and the lower bound for x4- variable can be improved by some number of iterations.
2) In the case σ=0 we examine one specific type of the convergence dynamics (1.1), which excludes the existence of TV-, ITV-equilibrium points, periodic orbits and the chaotic attractor. All results are expressed in terms of γ. It is computed the lower bound γmin such that for γ>γmin any trajectory is attracted to either one of TO-equilibrium points or one of TF-equilibrium points. This problem is solved in Theorem 4.2.
3) In Proposition 3 conditions for the global tumor elimination are presented for sufficiently large values of the influx rate σ of immune cells from the external source σ,σ≥σ(1)min. The formula (5.2) describes how the eradication bound σ(1)min depends on parameters of the model. It is shown that the bound σ(1)min may be decreased if we apply the iterational procedure. This leads to a reduced risk of over-immunization. As a result, we get the sequence of bounds σ(k)min which are characterized in Theorem 5.1. It is demonstrated that σ(2)min<σ(1)min. The decrease of the sequence of eradication bounds {σ(k)min} seems clear from the point of view of common sense and is confirmed by calculations. However, the additional analysis is still required and deserves a separate research. On Figures 1; 2; 3 it is shown numerically that there is the tumor eradication dynamics at different values of γ and σ=σ(1)min;σ(2)min;σ(3)min.Our statements about the tumor eradication are presented in a convenient form for applications and may be helpful in administering immunotherapy.
Further, let us derive an equation for finding σ(3)min. With this goal we notice that .
A2=θ1+σθ2θ3+σθ4, | (6.1) |
with
θ1=κrb2(a3+a4κ);abcdθ2=κ(r−a2);θ3=rb2(a3+a4κ);abcdeθ4=r. |
Utilizing these formulas for B2 we have that
B2=σa3+a4A2=θ3σ+θ4σ2θ5+θ6σ, |
with
θ5=a3θ3+a4θ1;abcdθ6=a3θ4+a4θ2. |
Next, we compute
A3=κ−κa2B2rb2+rB2=κθ7+σθ8+σ2θ9θ10+σθ11+σ2θ12, |
with
θ7=rb2θ5;abcdθ8=rb2θ6+(r−a2)θ3;abcdθ9=(r−a2)θ4;abcdθ10=rb2θ6+rθ3;abcdθ11=rθ4. |
Further,
B3=σa3+a4A3=σθ7+σ2θ10+σ3θ11θ12+σθ13+σ2θ14, |
with
θ12=(a3+a4κ)θ7;θ13=a3θ10+a4κθ8;θ14=a3θ11+a4θ9 |
Finally, we notice that σ(3)min is a minimal positive root of the cubic equation rb2+B3(r−a2)=0 which can be written in the form
σ3θ11(r−a2)+σ2(rb2θ14+(r−a2)θ10)+σ(rb2θ13+(r−a2)θ7)+rb2θ12=0. |
All authors declare no conflicts of interest in this paper.
[1] | Ž. Bajzer, T. Carr, K. Josić, S.J Russell and D. Dingli, Modeling of cancer virotherapy with recombinant measles viruses, J. Theor. Biol. 252 (2008), 109–122. |
[2] | M. Biesecker, J.H. Kimn, H. Lu, D. Dingli and Ž. Bajzer, Optimization of virotherapy for cancer, Bulletin Math. Biol., 72 (2010), 469–489. |
[3] | B.W. Bridle, K.B. Stephenson, J.E. Boudreau, S. Koshy, N. Kazdhan, E. Pullenayegum, J. Brunellière, J.L. Bramson, B.D. Lichty and Y. Wan, Potentiating cancer immunotherapy using an oncolytic virus, Mol. Ther., 18 (2010), 1430–1439. |
[4] | R.M. Diaz, F. Galivo, T. Kottke, P.Wongthida, J. Qiao, J. Thompson, M. Valdes, G. Barber and R.G Vile, Oncolytic immunovirotherapy for melanoma using vesicular stomatitis virus, Cancer Res., 67 (2007), 2840–2848. |
[5] | R. Eftimie, J. Dushoff, B.M. Bridle, J.L. Bramson and D.J.D. Earn, Multi-stability and multiinstability phenomena in a mathematical model of tumor-immune-virus interactions, Bulletin Math. Biol., 73 (2011), 2932–2961. |
[6] | R. Eftimie, C.K. Macnamara, J. Dushoff, J.L. Bramson and D.J.D. Earn, Bifurcations and chaotic dynamics in a tumour-immune-virus system, Math. Modell. Nat. Pheno., 11 (2016), 65–85. |
[7] | M.W. Hirsch, Systems of differential equations that are competitive or cooperative II: Convergence almost everywhere, SIAM J. Math. Analysis, 16 (1985), 423–439. |
[8] | B.Y. Hwang and D.V. Schaffer, Engineering a serum-resistant and thermostable vesicular stomatitis virus G glycoprotein for pseudotyping retroviral and lentiviral vectors, Gene Ther., 20 (2013), 807– 815. |
[9] | A.P. Krishchenko, Estimation of domains with cycles, Computer Math. Appl., 34 (1997), 325–332. |
[10] | A.P. Krishchenko, Localization of invariant compact sets of dynamical systems, Differ. Equations, 41 (2005), 1669–1676. |
[11] | A.P. Krishchenko and K.E. Starkov, Localization of compact invariant sets of the Lorenz system, Phys. Lett. A, 353 (2006), 383–388. |
[12] | A.P. Krishchenko and K.E. Starkov, On the global dynamics of a chronic myelogenous leukemia model, Commun. Nonlin. Sci. Numer. Simul., 33 (2016), 174–183. |
[13] | A.P. Krishchenko and K.E. Starkov, The four-dimensional Kirschner-Panetta type cancer model: how to obtain tumor eradication? Math. Biosci. Eng., 15 (2018), 1243–1254. |
[14] | S. Varghese and S.D. Rabkin, Oncolytic herpes simplex virus vectors for cancer virotherapy, Cancer Gene Ther., 9 (2002), 967–978. |
[15] | M.Y. Li and S.J. Muldowney, On R.A. Smith's autonomous convergence theorem, Rocky Mountain J. Math., 25 (1995), 365–379. |
[16] | R.A. Smith, Some applications of Hausdorff dimension inequalities for ordinary differential equations, Proceed. Royal Soc. Edinburgh Sec. A: Math., 104 (1986), 235–259. |
[17] | K.E. Starkov, On dynamic tumor eradication conditions under combined chemical/anti-angiogenic therapies, Phys. Lett. A, 382 (2018), 387–393. |
[18] | K.E. Starkov and S. Bunimovich-Mendrazitsky, Dynamical properties and tumor clearance conditions for a nine-dimensional model of bladder cancer immunotherapy, Math. Biosci. Eng., 13 (2016), 1059–1075. |
[19] | K.E. Starkov and L. Jimenez Beristain, Dynamic analysis of the melanoma model: from cancer persistence to its eradication, Internat. J. Bifur. Chaos Appl. Sci. Engrg., 27 (2017), 1750151-1– 1750151-11. |
[20] | K.E. Starkov and A.P. Krishchenko, Ultimate dynamics of the Kirschner-Panetta model: Tumor eradication and related problems, Phys. Lett. A, 381 (2017), 3409–3016. |
[21] | H.R. Thieme, Asymptotically autonomous differential equations in the plane, Rocky Mountain J. Math., 34 (1994), 351–380. |
1. | Konstantin E. Starkov, Anatoly N. Kanatnikov, Giovana Andres, Ultimate tumor dynamics and eradication using oncolytic virotherapy, 2021, 92, 10075704, 105469, 10.1016/j.cnsns.2020.105469 | |
2. | A. N. Kanatnikov, Localizing Sets and Behavior of Trajectories of Time-Varying Systems, 2019, 55, 0012-2661, 1420, 10.1134/S00122661190110028 | |
3. | Christine E. Engeland, Johannes P.W. Heidbuechel, Robyn P. Araujo, Adrianne L. Jenner, Improving immunovirotherapies: the intersection of mathematical modelling and experiments, 2022, 6, 26671190, 100011, 10.1016/j.immuno.2022.100011 | |
4. | Alexander P. Krishchenko, Konstantin E. Starkov, 5D model of pancreatic cancer: Key features of ultimate dynamics, 2021, 103, 10075704, 105997, 10.1016/j.cnsns.2021.105997 |