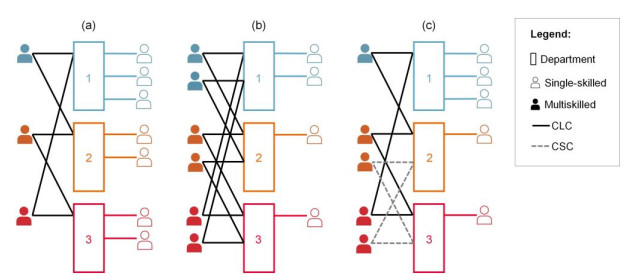
Citation: Kin Keung Lai, Shashi Kant Mishra, Geetanjali Panda, Md Abu Talhamainuddin Ansary, Bhagwat Ram. On q-steepest descent method for unconstrained multiobjective optimization problems[J]. AIMS Mathematics, 2020, 5(6): 5521-5540. doi: 10.3934/math.2020354
[1] | Zhaoxiang Zhang, Xuehua Yang, Song Wang . The alternating direction implicit difference scheme and extrapolation method for a class of three dimensional hyperbolic equations with constant coefficients. Electronic Research Archive, 2025, 33(5): 3348-3377. doi: 10.3934/era.2025148 |
[2] | Junseok Kim . Maximum principle preserving the unconditionally stable method for the Allen–Cahn equation with a high-order potential. Electronic Research Archive, 2025, 33(1): 433-446. doi: 10.3934/era.2025021 |
[3] | Yunxia Niu, Chaoran Qi, Yao Zhang, Wahidullah Niazi . Numerical analysis and simulation of the compact difference scheme for the pseudo-parabolic Burgers' equation. Electronic Research Archive, 2025, 33(3): 1763-1791. doi: 10.3934/era.2025080 |
[4] | Lot-Kei Chou, Siu-Long Lei . High dimensional Riesz space distributed-order advection-dispersion equations with ADI scheme in compression format. Electronic Research Archive, 2022, 30(4): 1463-1476. doi: 10.3934/era.2022077 |
[5] | Hongsong Feng, Shan Zhao . A multigrid based finite difference method for solving parabolic interface problem. Electronic Research Archive, 2021, 29(5): 3141-3170. doi: 10.3934/era.2021031 |
[6] | Chang Hou, Hu Chen . Stability and pointwise-in-time convergence analysis of a finite difference scheme for a 2D nonlinear multi-term subdiffusion equation. Electronic Research Archive, 2025, 33(3): 1476-1489. doi: 10.3934/era.2025069 |
[7] | Yiyuan Qian, Haiming Song, Xiaoshen Wang, Kai Zhang . Primal-dual active-set method for solving the unilateral pricing problem of American better-of options on two assets. Electronic Research Archive, 2022, 30(1): 90-115. doi: 10.3934/era.2022005 |
[8] | Cheng Wang . Convergence analysis of Fourier pseudo-spectral schemes for three-dimensional incompressible Navier-Stokes equations. Electronic Research Archive, 2021, 29(5): 2915-2944. doi: 10.3934/era.2021019 |
[9] | Leilei Wei, Xiaojing Wei, Bo Tang . Numerical analysis of variable-order fractional KdV-Burgers-Kuramoto equation. Electronic Research Archive, 2022, 30(4): 1263-1281. doi: 10.3934/era.2022066 |
[10] | Noelia Bazarra, José R. Fernández, Ramón Quintanilla . Numerical analysis of a problem in micropolar thermoviscoelasticity. Electronic Research Archive, 2022, 30(2): 683-700. doi: 10.3934/era.2022036 |
Retail companies must face constant changes in staffing demand caused by the seasonality and uncertainty of demand [1,2,3,4]. In general, retailers seek to reduce their labor costs and simultaneously improve the service levels offered to their customers [5,6]. However, even retail companies with effective personnel scheduling tools can have difficulty in managing staff surpluses or shortages due to the seasonality and uncertainty of demand [7,8,9]. Various authors addressed the problems of staff surpluses and shortages through the use of an optimal multiskilled staff (e.g., [10,11,12,13]).
In a retail store, multiskilled staff can work in multiple departments, allowing store supervisors to relocate multiskilled workers from departments with staff surpluses to departments with staff shortages [14]. Several articles have reported that the most profitable staff is one where multiskilled workers are skilled to perform up to a total of two store departments (e.g., [15,16,17]). This is known in the multiskilling literature as a 2-flexibility policy, and based on this policy, the 2-chaining strategy has been the most recommended to design training plans (e.g., [18,19,20,21]).
In a retail store context, 2-chaining requires that a set of workers be skilled up to a maximum of two departments and training schemes must be designed through closed long chains and/or closed short chains. In one closed long chain (CLC), each store department has one multiskilled worker, such that these workers connect all the departments through a single closed chain. In turn, in one closed short chain (CSC), only a subset of departments is connected through a closed chain, since only some of them have multiskilled workers. Particularly, Henao et al. [1,2] and Porto et al. [6,21] indicated that staff training plans built through of CLCs and CSCs provide maximum efficiency to assign and reassign workers in scenarios with unscheduled staff absenteeism and/or uncertainty in the staff demand.
In Figure 1 three examples of 2-chaining structures are shown. In each of the three structures, demand nodes (departments) are in the middle, whereas supply nodes (workers) are positioned on the right (single-skilled workers) and the left (multiskilled workers). Figure 1(a) shows that in one CLC, there is only one multiskilled worker at each store department. In contrast, Figure 1(b) shows that in two similar CLCs, each department has two multiskilled workers forming two CLCs that are indistinguishable. Finally, Figure 1(c) shows three multiskilled workers connecting departments 1, 2, and 3 by using of one CLC, and also shows two additional multiskilled workers connecting departments 2 and 3 by using of one CSC.
For the same retail case study and assuming uncertainty in the staff demand (at the store level), Henao et al. [1] and Henao et al. [2] solved a weekly multiskilled personnel assignment problem (MPAP). They calculated the number of single-skilled workers who should become multiskilled and in which additional department they should be trained. In addition, both studies designed training plans through a 2-chaining strategy to minimize the total cost of under/overstaffing and training. In the first study, Henao et al. [1] developed a single-stage robust optimization approach to calculate the optimal number of multiskilled workers per store's department, whereas in the second study, Henao et al. [2] derived a closed-form equation to deliver an estimation of the optimal quantity of multiskilled workers per store's department. Even with different solution approaches, both studies showed that the most profitable staff is one where there is a mix of single-skilled and multiskilled workers. Their results also showed that the best 2-chaining structures combine CLCs and CSCs of different lengths.
The literature on personnel scheduling problems shows several approaches for optimizing under uncertainty that have received substantial theoretical development, among these are: (1) Closed-form equation (CF); under this approach, it is possible to develop an analytic expression to calculate the optimal solution (or an approximation of the optimal solution) for the addressed problem. This approach requires knowing the probability distribution function associated with the parameter under uncertainty1. (2) Two-stage stochastic optimization (TSSO); this approach assumes complete knowledge of the distribution of probability associated with the parameter under uncertainty and includes (in an explicit way) such uncertainty into the mathematical model [22]. (3) Robust optimization (RO); unlike the previous approaches, complete knowledge of the stochastic parameters is not required [23,24]. Stochastic parameters are represented by the so-called uncertainty set, i.e., a set where parameters can take any value. RO limits the variability in the parameter under uncertainty to an acceptable and predefined range to avoid unreasonable scenarios. (4) Distributionally robust optimization (DRO); this approach is known as a generalization of the RO and TSSO approaches. DRO tackles the uncertainty by including additional information into the uncertainty set (e.g., mean and covariance), which can be estimated from expert knowledge or historical data [25]. In short, CF and TSSO assume complete distributional information, DRO assumes partial distributional information, whereas RO assumes minimal distributional knowledge about the uncertain parameters. Although all of these approaches guarantee robust solutions, they cannot ensure the same level of reliability and conservatism in the solutions. Note that, in a retail store context, a robust solution is not necessarily the best in every possible scenario of demand variability, but it remains good over a wide range of changes in staffing demand.
1 In the personnel scheduling literature, we have not found a closed-form equation with partial knowledge of the stochastic parameter. On the contrary, all the studies assumed complete knowledge. Thus, without loss of generality, the closed-form approach is considered to have access to full distributional knowledge of stochastic parameters in this paper.
The current work builds on top of our previous findings from Henao et al. [1] and Henao et al. [2]. We also propose to solve the MPAP with 2-chaining in this paper. However, unlike other references in the state-of-the-art and our previous works, we formulate a TSSO model to calculate the multiskilling requirements that minimize the training costs and the expected costs of staff surplus/shortage. Particularly, this work presents two main contributions. First, we solve the MPAP using a TSSO approach. Second, we evaluate and compare the performance of three different optimization approaches under uncertainty (i.e., TSSO vs. RO vs. CF). Note that, the benchmark RO and CF approaches were already published in Henao et al. [1] and Henao et al. [2], respectively. Besides, these three optimization approaches under uncertainty will be compared with the deterministic (DT) approach and with two multiskilling approaches considered as myopic: zero multiskilling (ZM) and total multiskilling (TM). Such that, under a ZM approach, each worker is skilled to perform in a single store department (i.e., single-skilled), whereas under a TM approach, all workers are skilled to perform in all departments. The methodology is tested for a case study associated with a retail store operating in Chile, which has already been presented and solved by Henao et al. [1,2]. The performance of all approaches is assessed by means of out-of-sample simulations. Each approach is tested against different levels of variability in staff demand per store's department. We also provide insights on how to choose the most suitable approach according to the balance between conservatism and reliability associated with each of them.
Personnel scheduling is a research area extensively studied. In this topic, literature review articles such as Ernst et al. [26], Brucker et al. [27], Van den Bergh et al. [28], and Özder et al. [29] addressed various problems and solution methodologies associated with multiple industries. Table 1 presents previous articles that have used optimization approaches under uncertainty to solve personnel scheduling problems in the service industries. The principal features of these articles are described below. We remark that each of the articles classified in Table 1 considered demand uncertainty, but none of them included supply uncertainty (i.e., unscheduled staff absenteeism).
Reference | PSP | MS | 2-CH | SM | AMU | Application |
Bard et al. [30] | S + TS | No | – | IP + H | TSSO | Postal service |
Zhu & Sherali [31] | S + SS | Var | No | MIP + BD | TSSO | Service centers |
Robbins & Harrison [32] | S + TS | No | – | MIP + BD | TSSO | Call Centers |
Campbell [33] | DOS | Par | No | IP + H | TSSO | Health |
Liao et al. [34] | S + A | No | – | MIP | TSSO, 1RO | Call Centers |
Liao et al. [35] | S + SS | No | – | MIP | TSSO, DRO | Call Centers |
Gnanlet & Gilland [36] | S + A | Var | Yes | MIP + AN | TSSO, CF | Health |
Paul & MacDonald [37] | S + A | Var | Yes | MIP + H + AN | CF | Health |
Kim & Mehrotra [38] | S + TS | No | – | MIP + M | TSSO | Health |
Parisio & Jones [39] | TS | No | – | MIP | TSSO | Retail |
Henao et al. [1] | A | Var | Yes | MIP + H | 1RO | Retail |
Bodur & Luedtke [40] | S + SS | Par | Yes | MIP + BD + B & C | TSSO | Call Centers |
Liu [18] | S + A | Par | Yes | IP + AN | TSSO, CF | – |
Mattia et al. [41] | S + SS | No | – | IP + BD + B & C | 2RO | Call Centers |
Restrepo et al. [42] | TS | Par | No | MIP + M | TSSO | – |
Altner et al. [43] | S + SS | No | – | IP + CG | TSSO | CSOC |
Altner et al. [44] | DOS | Var | No | MIP + M | TSSO | CSOC |
Henao et al. [2] | A | Var | Yes | AN + H + LP | CF | Retail |
Fontalvo Echavez et al. [20] | DOS | Var | Yes | MIP | TSSO | Retail |
This paper | A | Var | Yes | MIP + H + AN | TSSO, RO, CF | Retail |
1. Type of personnel scheduling problem (PSP) studied: May be classified as: (a) Staffing (S), that is, deciding the number of workers needed per task type and per labor contract type. (b) Shift scheduling (SS), that is, deciding what shift each worker should perform on their working days. (c) Days-off scheduling (DOS), that is, deciding the sequence of working and resting days that should be assigned to each worker (e.g., weekly, biweekly). (d) Tour scheduling (TS), where shifts and days-off are scheduled simultaneously (e.g., weekly, biweekly). (e) Assignment (A), where workers are assigned to particular task types, but it is not required to schedule shifts or rest days (e.g., daily, weekly, monthly). Note that, the last type of problem is known as the personnel assignment problem (PAP), and when it involves the use of multiskilled staff, it is known as the MPAP.
2. Multiskilling (MS): Specifies whether or not the PSP incorporates multiskilling as a source of labor flexibility. If the study includes multiskilling, we identify two alternatives. That is, the skill(s) of a worker can be incorporated into the math model as a parameter (par) or as a variable (var).
3. 2-chaining (2-CH): Specifies when a study incorporated the multiskilling using the 2-chaining strategy.
4. Solution method (SM) used: May be: (a) linear programming (LP); (b) integer programming (IP); (c) mixed integer programming (MIP); (d) heuristics (H); (e) metaheuristics (M); (f) benders decomposition (BD); (g) branch-and-cut (B & C); (h) column generation (CG); or (i) analytic (AN).
5. Approach to model uncertainty (AMU): Specifies the optimization approach under uncertainty employed to handle the uncertain demand in the study. It can be classified as: (a) two-stage stochastic optimization (TSSO); (b) distributionally robust optimization (DRO); (c) single-stage robust optimization (1RO); (d) two-stage robust optimization (2RO); or (e) closed-form equation (CF).
6. Application: Service industry where the study has been applied.
Below, we present a more detailed review of various articles listed in Table 1. We begin with those that did not incorporate multiskilling as a source of flexibility and then consider those that did. Then, we discuss those articles that solved the addressed problem using two or more optimization approaches under uncertainty.
Table 1 shows that various articles did not use multiskilled staff to minimize overstaffing/understaffing levels (i.e., [30,32,34,35,38,39,41,43]). This is common in studies where the personnel scheduling problem only considers a single type of task (or store department). In Bard et al. [30], a TSSO model is developed to solve a shift scheduling problem in a post office. Decisions on the number of required workers were modeled in the first stage, and weekly shift structures were modeled in the second stage. In Kim and Mehrotra [38], the authors proposed a TSSO model to determine the staff size and the shift structures in the first stage, whereas these shift structures were adjusted once new information about incoming demand was available in the second stage. Similarly, Parisio and Jones [39] proposed a TSSO model to solve a tour scheduling problem in retail. Optimal decisions on workers' schedules (i.e., rest days and shifts) were modeled in the first stage, while overtime decisions after demand realization were modeled in the second stage.
Table 1 indicates that various articles included multiskilling as a source of flexibility. Thus, such articles addressed a personnel scheduling problem with at least two types of tasks (or store departments). The literature review shows that multiskilling can be incorporated into mathematical modeling in three ways.
First, by considering multiple multiskilling structures as an input parameter in the math model and then the model solution must identify the best performing structure (i.e., [18,33,40,42]). In Campbell [33], a TSSO model is developed to solve a days-off scheduling problem with multiskilled workers and a multi-department service environment. Decisions on rest days were modeled in the first stage, whereas reassigns available workers according to the observed demands were modeled in the second stage. In Restrepo et al. [42], the authors addressed a tour scheduling problem considering workers with identical skill sets. Decisions on rest days and shifts were represented in the first stage, while break times and task assignments were described in the second stage.
Second, by modeling the multiskilling as a decision variable into the math model (i.e., [31,44]). In Zhu and Sherali [31], the authors proposed a TSSO model to solve a staffing and shift scheduling problem. Decisions on staffing levels and shifts were modeled in the first stage, while in the second stage, worker reassignment decisions were taken. In Altner et al. [44], the authors studied a TSSO model to solve a days-off scheduling problem. The first stage modeled decisions on which workers should be multiskilled and which days each worker should rest considering a bi-weekly planning horizon. Then, for each day, the second stage considered how task types could be assigned to workers once the uncertain demand is revealed.
Third, by modeling the multiskilling as a decision variable into the math model and ensuring the formation of closed-chained multiskilling structures by including of a set of constraints into the model (i.e., [1,2,20,36,37]). Particularly in the retail industry, Henao et al. [1] and Henao et al. [2] solved a weekly MPAP with 2-chaining using different optimization approaches under uncertainty; RO and CF, respectively. Note that, to our knowledge, these are the only articles that solved the MPAP with 2-chaining (see Table 1). The MPAP allows adding and simplifying scheduling decisions while focusing on answering the multiskilling tactical questions. In both articles, the authors proposed two methodological stages to sequentially answer the following three tactical questions: (i) How many multiskilled workers are needed per department? (ii) In which second department should be trained each of the workers identified as multiskilled? (iii) How many CLCs and/or CSCs are needed, and which store departments should belong to each of the chains? In Henao et al. [1], the first stage of the methodology proposed a single-stage robust optimization model to answer the question (i), while the second stage proposed a constructive heuristic to answer the questions (ii) and (iii). In Henao et al. [2], the first stage of the methodology derived a closed-form equation to answer the question (i), while the second stage used the constructive heuristic developed by Henao et al. [1] to answer the questions (ii) and (iii). However, these sequential methodologies could lead to suboptimal solutions; thus, integrated approaches are needed to guarantee optimality.
Table 1 shows various articles that solved a personnel scheduling problem using two optimization approaches under uncertainty (i.e., [18,34,35,36]). On one side, Liao et al. [34] and Liao et al. [35] did not incorporate multiskilling in the mathematical modeling. On the other hand, Liu [18] incorporated multiskilling in the mathematical modeling as a parameter, while Gnanlet and Gilland [36] incorporated multiskilling as a variable, but they also used 2-chaining.
In Liao et al. [34], the authors addressed a call center staffing problem considering single-length shifts. They compared the performance of a stochastic programming approach and a robust programming formulation. Regarding the average cost, they explained that the TSSO approach was more efficient than the RO approach. This is because the RO approach is based on a worst-case-type analysis. Thus, the optimal RO solution generates higher staffing levels, which decreases the staff shortage costs but also increases the direct salary costs. In Liao et al. [35], for a case study in call centers, the authors proposed a methodology that combined the TSSO and DRO approaches to solve simultaneously a staffing and shift scheduling problem considering service level constraints. Liu [18] and Gnanlet and Gilland [36] proposed TSSO models to solve a staffing problem with only two task types for the health sector. In Liu [18], the authors developed an analytic expression to calculate the optimal number of workers. In Gnanlet and Gilland [36], the authors also developed analytic expressions to determine the optimal number of multiskilled nurses. Note that none of these four papers solved the MPAP, and they did not address the retail industry as a case study.
Our study seeks to fill two gaps evidenced in the literature review. First, for the MPAP with 2-chaining in the retail industry, we develop a TSSO model to simultaneously answer the tactical questions (i), (ii), and (iii) described previously, instead of solving them sequentially, as proposed by Henao et al. [1] and Henao et al. [2]. It is important to note that, Table 1 indicates that most of the authors used a TSSO approach to solve the PSP addressed, but none of them studied the MPAP with 2-chaining.
Second, we propose to evaluate and compare the performance of three different optimization approaches under uncertainty (i.e., TSSO vs. RO vs. CF). Thus, using an extensive computational study, we will quantify the reliability of the TSSO model by comparing it with the benchmark RO and CF approaches presented in Henao et al. [1] and Henao et al. [2], respectively. However, we will not make comparisons with additional benchmarks from the literature, because except for Henao et al. [1] and Henao et al. [2], the other articles in Table 1 studied different PSPs. In addition to these three optimization approaches, we propose to compare them with the deterministic (DT) approach and with the two myopic approaches ZM and TM.
Our problem considers a retail store with a known number of departments, such that each of them has contracted a group of workers initially single-skilled. Working in a store department requires specific skills, which differ among all departments. Although the working hours of workers are determined in their contracts, the store's departments face uncertainty in demand. Here, the skill training of workers plays a strategic role in handling the uncertainty in demands. That is, the costs associated with staff shortages and surpluses in the different store departments may be prevented by assigning some multiskilled workers from departments with staff surpluses to departments with staff shortages. Therefore, the goal of the problem is to decide how many workers should be multiskilled and in what additional department, such that they get ready to work in other departments in case needed.
In short, in this paper, we will solve a weekly MPAP that at the same time determines how many multiskilled workers are needed, in what second department, and how to assign working hours to each worker over a one-week planning horizon. In addition, our formulation will provide a training plan that ensures the formation of CLCs and/or CSCs, which have shown to be especially useful in minimizing overstaffing and understaffing under uncertain demand. Thus, the solution of the MPAP with 2-chaining, at the store level, must minimize the training costs and the expected costs of staff surplus/shortage. Table 2 presents the mathematical notation employed to formulate the MPAP with 2-chaining. It will also be possible to find additional notation at each subsection of the proposed methodology in Section 4.
Sets | |
I | Workers, indexed by i |
L | Departments of the retail store, indexed by l |
S | Scenarios of demand, indexed by s |
Il | Set of single-skilled workers initially hired in department l, indexed by i |
Parameters | |
rl(s) | Random parameter of staff demand in weekly hours per store's department l, ∀l∈L,s∈S |
¯rl | Average value of staff demand in weekly hours per store's department l, ∀l∈L |
c | Cost of workers training in any department; [US$-week /Worker] |
u | Staff shortage cost per store's department; [US$/Hour] |
b | Staff surplus cost per store's department; [US$/Hour] |
h | Weekly hours that workers have to work in accordance with their employment contract |
mi | Department in which the worker i is initially trained, ∀i∈I |
Variables | |
xil | 1 if worker i is trained in department l, 0 otherwise, ∀i∈I,l∈L |
vi | 1 if worker i is multiskilled, 0 otherwise, ∀i∈I |
λl | Number of multiskilled workers belonging to department l, ∀l∈L |
Ω | Number of generated closed-chains |
ωil | Number of weekly working hours allocated to worker i in department l, ∀i∈I,l∈L |
κl | Number of staff shortage hours per week in department l,∀l∈L |
δl | Number of staff surplus hours per week in department l,∀l∈L |
Solution approaches for the MPAP with 2-chaining | |
DT | Deterministic optimization |
RO | Robust optimization |
CF | Closed-form equation |
TSSO | Two-stage stochastic optimization |
Similar to Henao et al. [1] and Henao et al. [2], the formulation of the MPAP with 2-chaining considers various assumptions: (1) The demand in weekly hours per store's department, rl(s), is a random parameter. In this paper, it is assumed two different levels of knowledge about the uncertainty of the parameter rl(s): (i) for the TSSO and CF approaches, the probability distribution of the random parameter is completely known; (ii) for the RO approach, only the average value and the maximum value allowed of the random parameter are known. (2) There is no correlation between the demand of different pairs of departments. (3) Staffing demand per store's department may not be fully covered (i.e., unsatisfied demand). Thus, the objective function incorporates a staff shortage cost, and it is assumed that this cost does not differ between departments. (4) Staff surplus also has an associated cost, and it is also assumed that this cost does not vary between departments. Mattia et al. [41] and Henao et al. [1] indicated that this cost represents an opportunity cost, which is incurred when there are idle workers in the store. (5) The objective function also incorporates staff training costs, and they do not vary by department. (6) All store workers have the same type of contract and thus they must perform an equal number of weekly working hours. (7) It is assumed that, for each department, the available assignment weekly hours, (|Il|h), are equal to the average value of weekly hours demand (¯rl). Notice that this assumption imposes a trivial solution to the deterministic formulation for the MPAP. That is, since there is no imbalance between staffing and staff demand in each store department, the solution of the deterministic MPAP formulation will not require any multiskilled worker. That is, the DT approach solution will be equivalent to the ZM approach solution (i.e., zero multiskilling). (8) All departments have contracted a group of workers initially single-skilled. (9) Workers can be trained in a single additional department and, thus, they could perform up to a total of two store departments. (10) Workers have equal productivity in those departments where they are skilled. Henao et al. [1,2,9] and Porto et al. [6,21], who also addressed multiskilled personnel scheduling problems for retail stores, explain that this last assumption is reasonable when multiskilled workers can only perform up to a maximum of two store departments, and the types of tasks performed have a low and uniform level of complexity.
This section proposes a framework to solve the MPAP with 2-chaining and uncertain demand, as described above. First, in Subsection 4.1, we develop a TSSO model to calculate the multiskilling requirements that minimize the training costs and the expected costs of staff surplus/shortage. Then, in Subsection 4.2, we present the two alternative optimization approaches under uncertainty - RO and CF - to solve the addressed problem. These two alternative approaches were already published in Henao et al. [1] and Henao et al. [2], respectively. Recall that the main contributions of this work are: (1) to solve the MPAP with 2-chaining using a TSSO approach and (2) to evaluate and compare the performance of three different optimization approaches under uncertainty (i.e., TSSO vs. RO vs. CF).
In this subsection, we present a brief background on the TSSO approach, and then we present the TSSO version of the deterministic MPAP model (DT) developed by Henao et al. [1]. In Subsection 4.1.1, we make abuse of notation for the sake of consistency with the standard nomenclature used on the Stochastic Optimization literature. All symbols are described within the context.
In a TSSO approach, decisions are modeled in two stages. The first-stage decisions are often denoted by the set of variables, x, and are called the "here-and-now" decisions. These decisions are made before the uncertain parameters become known. The second-stage decisions or recourse decisions are often described by the vector of variables, y(x,ξ), and are defined as the "wait and see" decisions. These decisions are made once the uncertainty is exposed. Uncertain parameters are represented by ξ, and are assumed to be known. Thus, the second-stage decisions depend not only on first-stage decisions but also on the knowledge of the parameters under uncertainty, that is, y(x,ξ). In addition, in the TSSO framework, non-anticipativity constraints are implicitly modeled to ensure that the first-stage decisions are not affected by the knowledge of the uncertain parameters. For details about the theory of TSSO, the reader is referred to Birge and Louveaux [22].
A general form for the classical TSSO model is expressed in Equation (1), distinguishing between first-stage and second-stage decisions.
Minx,y(x,ξ)cTx+Ep[f(y(x,ξ))]s.t.x∈Xy(x,ξ)∈Y(x,ξ), | (1) |
Note that the first term in the objective function, cTx, corresponds cost incurred by the first-stage decisions. The second-stage term is the recourse expected cost function, Ep[f(y(x,ξ))], which is represented as the expectation of the second-stage objective taken over the stochastic parameter ξ within a given probability measure p. Such that f(y(x,ξ)) is the operational value function.
The uncertain parameters, ξ, are usually continuous; thus, the TSSO framework is in most cases intractable. Sample Average Approximation (SAA) approach is commonly used to overcome this issue. The SAA is based on the generation of a set of random samples from the true stochastic parameter ξ that would represent them. Birge and Louveaux [22] explain that, for each possible scenario, the SAA clones the variables set of second-stage. Thus, by taking independent and identically distributed (iid) random samples, ξ, from the assumed probability distribution, it is possible to approximate the problem (1). By doing so, we represent each generated sample by ξ(s)∈Ξs, where Ξs is the set of all iid random samples generated and |Ξs| represents the cardinality of Ξs, that is, number of scenarios. Such that, when |Ξs| is large enough (but a finite number), the SAA approach has a high quality. However, for a large set of scenarios in the second-stage, the SAA can be too expensive to solve. Below, for a finite set of scenarios, the SAA formulation of (1) is defined.
Minx,y(x,ξ(s))cTx+1|Ξs|∑ξ(s)∈Ξsf(y(x,ξ(s)))s.t.x∈Xy(x,ξ(s))∈Y(x,ξ(s)),ξ(s)∈Ξs | (2) |
Then, (2) is a finite-dimensional problem equivalent to (1).
For the MPAP problem, we assume complete knowledge of the distribution of probability associated with the uncertain demand, which is accessible from historical data. The deterministic linear MIP model presented by Henao et al. [1] can be extended to add such uncertainty. For full details on the DT model, the reader is referred to Subsection 3.1 in Henao et al. [1].
In this subsection, we proposed a TSSO model for the MPAP with 2-chaining. To facilitate comparisons of the TSSO version with the DT version developed by Henao et al. [1], we use the same mathematical notation. The first-stage variables, xil,vi, and λl are tactical decisions associated with the workers training to work in one additional store department. These decisions are taken before the uncertainty in weekly demands has been revealed. The second-stage continuous recourse variables, ωil(s),κl(s),δl(s), are operational decisions associated with the workers allocation to minimize staff surplus/shortage levels. These decisions are taken after the uncertainty in weekly demands has been revealed. To solve the TSSO version for the MPAP, we implemented an SAA approach.
Below, the mathematical formulation of our TSSO model is presented. Also recall that, in Table 2 was defined the math notation for each of the sets, parameters, and variables used in the TSSO model.
Min∑i∈I∑l∈L:l≠micxil⏟first−stage+1|S|[∑s∈S∑l∈Luκl(s)+∑s∈S∑l∈Lbδl(s)]⏟second−stage | (3) |
s.t.
∑i∈Iωil(s)+κl(s)−δl(s)=rl(s)∀l∈L,s∈S | (4) |
∑l∈Lωil(s)=h∀i∈I,s∈S | (5) |
ωil(s)≤hxil∀i∈I,l∈L,s∈S | (6) |
xil=1∀i∈I,l∈L:l=mi | (7) |
vi=∑l∈L:l≠mixil∀i∈I | (8) |
λl=∑l∈Ilvi∀l∈L | (9) |
vi≤1∀i∈I | (10) |
∑i∈Ilvi=∑i∈{I−Il}xil∀l∈L | (11) |
xil∈{0,1}∀i∈I,l∈L | (12) |
vi≥0∀i∈I | (13) |
λl≥0∀l∈L | (14) |
ωil(s)≥0∀i∈I,l∈L,s∈S | (15) |
κl(s)≥0∀l∈L,s∈S | (16) |
δl(s)≥0∀l∈L,s∈S | (17) |
The objective function (3) minimizes the total weekly cost. On the one hand, and associated with the first-stage decisions, the first term in the objective function minimizes the total training cost. On the other hand, and associated with the second-stage decisions, the next two terms in the objective function minimize the total expected cost of staff shortages and surpluses. In the TSSO version for the MPAP, the decisions about how many workers should be multiskilled in the first-stage will impact the staff surplus/shortage levels in the second-stage. Besides, the second-stage decisions are recourse variables that seek to take corrective actions in the workers' assignment after the uncertainty is revealed, but such corrective actions have a cost. Thus, a TSSO approach seeks to select the variables of first-stage that minimizes both first-stage costs and expected second-stage random costs.
Constraints (4) account for demand fulfillment for each department and demand scenario. They also calculate the staff surplus/shortages levels produced by the mismatch between the staffing and staff demand. Constraints (5) guarantee that, for each demand scenario, all workers are assigned to work the working hours stipulated in their contracts. Constraints (6) ensure that, for each demand scenario, workers can only be assigned to work in those departments where they received training. Constraints (7) indicate the department where each worker was originally trained. Constraints (8) indicate whether a worker was trained to be multiskilled, or he/she continues as a single-skilled. Constraints (9) calculate the optimal number of multiskilled workers belonging to each department. Constraints (10) ensure that workers can be trained in a single additional department. Constraints (11) ensure that the sum of multiskilled workers originally trained in the department l and who were also trained to perform in another second department (∑i∈Ilvi), is equal to the sum of multiskilled workers originally trained in a department different to l but that were also trained to perform in l as additional department (∑i∈{I−Il}xil). Constraints (10) and (11) guarantee a 2-chaining policy, and thus, the generation of CLCs or CSCs that allow the transfer of multiskilled workers among store departments. Note that, Henao et al. [1] explained that for both CLCs and CSCs formation, it is required that the outdegree of every one demand nodes belonging to a chain must be equal to the indegree of its corresponding supply node. The constraints (11) guarantee the satisfaction of such requirement. Constraints (12)-(17) indicate the possible values that each decision variable can take. Note that, given the auxiliary variables vi must take values less than or equal to one (see constraints (10)) and they are calculated through the sum of the binary variables xil (see constraints (8)), such variables do not require to be declared as binary. Finally, note that constraints (4)-(6) and (15)-(17) were accordingly extended from Henao et al. [1] to model that the second-stage variables depend on the random demand scenarios.
The optimal solution of the TSSO simultaneously answer the following three tactical questions: (i) How many multiskilled workers are needed per department, λl? (ii) In which second department should be trained each of the workers identified as multiskilled, xil?, and (iii) How many CLCs and/or CSCs are needed, and which store departments should belong to each of the chains, Ω?
In this subsection, we present the alternative optimization approaches, RO and CF, presented in Henao et al. [1] and Henao et al. [2] respectively, for solving the MPAP with 2-chaining and uncertain demand. Later, in Section 6, the solution of the proposed TSSO model will be compared with the benchmark approaches RO and CF.
Robust optimization is widely studied in the literature. In Beyer and Sendhoff [45], Kouvelis and Yu [46], and Gabrel et al. [47], the authors exhaustively reviewed and discussed articles that present methodological advances in RO as well as a range of problems that have used RO. In this subsection, we present the single-stage RO approach developed by Henao et al. [1] to solve the MPAP with 2-chaining and uncertain demand. The classic first-stage RO is an alternative approach to TSSO, where the probability distribution associated with the random parameter is not known; instead, the uncertainty of the parameter is modeled using the so-called uncertainty sets that usually are obtained by using historical data or estimates. In the RO approach from Henao et al. [1], it is assumed that the uncertain demand of each store department has a known average value, ¯rl, and the maximum value allowed for the uncertain demand is equal to ˆrl=α¯rl. Note that, this maximum allowed variation is defined as a fraction of the average value, where α is equivalent to the coefficient of variation (CV) for the demand in a department, 0≤α≤1. Thus, the weekly hours demand rl is a random variable that can only take values within the interval [¯rl−ˆrl,¯rl+ˆrl].
Henao et al. [1] also included into the RO model the set J, which refers to the set of departments whose staff demands can vary simultaneously. They assumed that |J|=|L|, so that, the staff demand in each department is subject to uncertainty. In order to address issues of overconservative solutions in the RO framework, it was possible to define an uncertainty budget parameter or level of conservatism, Γ, which was stated as a fraction, 0≤Γ≤1, of all departments with uncertain demand,|J|. If Γ=0, RO model is equivalent to DT model, since uncertainty is not considered and demand values are the nominal values of the uncertainty set. If Γ=1, solutions are optimized accordingly for the worst-case scenario, which considers the maximum allowed uncertainty.
Considering the previous assumptions and the approaches developed by Bertsimas and Sim [24], Bertsimas and Thiele [48] and Bohle et al. [49]; Henao et al. [1] presented a RO version of their DT formulation. The RO model seeks to minimize the worst-case operational costs of staff shortages and surpluses for the training decisions, xil. For full details on the RO version, the reader is referred to Subsection 3.2.2 in Henao et al. [1].
Finally, we found two key methodological differences between the TSSO approach and the RO approach. First, while the TSSO approach solutions are driven by the best-expected cost, solutions of the RO approach are driven by the best performance in the worst-case scenario. Second, the TSSO approach simultaneously answers the tactical questions (i), (ii), and (iii) established at the end of Subsection 4.1.2, which ensures consistency in the decision approach. On the contrary, the single-stage RO approach answers these questions sequentially through two methodological stages. The first stage of the methodology proposed a RO model to answer the question (i). The second stage proposed a constructive heuristic to answer the questions (ii) and (iii). For full details on the constructive heuristic, the reader is referred to Subsection 3.3 in Henao et al. [1]. In short, the first stage determines the optimal number of multiskilled workers needed per department (λl). Then, the second stage considering the solution provided by the first stage decides in which second department should be trained each of the workers identified as multiskilled (xil), whereas the minimum number of closed chains is built (Ω).
In this subsection, we present the approach developed by Henao et al. [2] for solving the MPAP with 2-chaining and uncertain demand. Similar to Henao et al. [1], Henao et al. [2] answered the tactical questions (i), (ii), and (iii) sequentially through two methodological stages. The first stage of the methodology proposed a CF equation to estimate: (i) the number of multiskilled workers needed per department (λl). The second stage used the constructive heuristic developed by Henao et al. [1] to determine: (ii) in which second department should be trained each of the multiskilled workers (xil); and (iii) the number of generated closed-chains (Ω). Note that, the second stage considers the solution provided by the first stage.
The CF expression developed by Henao et al. [2] was motivated by various problems in the literature where the authors seek to find an approximate solution using local optimality criteria. In Robuste et al. [50], Del Castillo [51], and Daganzo [52], the authors present a range of complex logistics problems (e.g., distribution centers location and vehicle routing) that have used a closed-form equation. In particular, in Henao et al. [2], the authors derived a novel CF equation that examines every single department in separate to deliver an estimation of the optimal quantity of multiskilled workers. Note that, under a CF approach, it is assumed that the local conditions are considered to be explicable for the complete region under evaluation.
CF is an alternative framework to TSSO and RO, where is assumed complete knowledge of the distribution of probability associated with the uncertain demand. The CF approach considered the following two key assumptions: (1) the probability density function for the staff demand per department is independently and normally distributed with known average value ¯rl and standard deviation σl; (2) the staff hours supply per store's department are equal to the average value of staff hours demand (|Il|h=¯rl). Note that the second assumption was also considered in the TSSO and RO approaches.
Under these assumptions, Henao et al. [2] developed a closed-form equation to estimate the number of multiskilled workers needed per department (λl). The CF equation seeks to minimize the incurred training cost (but per-hour instead of per-week) and the expected staff shortage cost. For further details about the development of the CF equation, the reader is referred to Subsection 4.2.1 in Henao et al. [2].
We also found two key methodological differences between the TSSO approach and the CF approach. First, the TSSO approach solutions are driven by the best-expected cost. In contrast, the solutions of the CF approach are driven by the best performance in the average-case scenario. Second, the TSSO approach simultaneously answers the tactical questions (i), (ii), and (iii), whereas the CF approach answers these questions sequentially. Finally, unlike TSSO and RO approaches that propose sophisticated optimization models for assessing the benefits of the 2-chaining structures, the CF approach offers decision-makers with a valuable and practical formula to estimate cost-effectiveness levels of multiskilling.
We propose a case study to validate the methodology previously presented. Such case study is associated with a home improvement store in Santiago de Chile, only for its sales personnel. In Subsection 5.1, we present the performance metrics used in Section 6 to assess and compare the 2-chaining structures constructed by the optimization approaches under uncertainty (i.e., TSSO, RO, and CF). Besides, in Section 6, these three optimization approaches under uncertainty will be compared with the two myopic approaches ZM and TM. Recall that the ZM approach represents the solution of the DT formulation. Also, note that the TM approach will achieve the lower bound of the total weekly staff surplus/shortage cost, whereas the ZM approach will obtain the upper bound. Later, we explain the data requirements of the MPAP model in Subsection 5.2.
To evaluate the performance of the solution approaches presented (i.e., DT, TSSO, RO, CF, ZM, and TM), we use metrics to measure: the required multiskilling levels (%ME,%TM), the incurred costs (φ,ψ), and the reliability (%SSS) associated with the solutions delivered by each approach. Below, we describe each of these metrics in more detail.
1) Multiskilled workers in percentage. It is calculated as the ratio between the total number of multiskilled workers and the total number of hired workers.
%ME=∑i∈Lλl|I|.100 |
2) Total multiskilling in percentage. It is calculated as the ratio between the number of additional departments in which workers were trained and the maximum number feasible.
%TM=∑i∈I∑l∈L:l≠mixil|I|(|L|−1).100 |
3) Total weekly cost. It is equivalent to the sum of incurred costs of training and staff surplus/ shortage.
φ=∑i∈I∑l∈L:l≠micxil+∑l∈Lukl+∑l∈Lbδl |
4) Total weekly staff surplus/shortage cost. It is defined as the incurred cost of staff surplus/ shortage associated with any approach.
ψ=∑l∈Lukl+∑l∈Lbδl |
5) Savings on the total weekly cost of staff surplus/shortage for an approach. Consider that, ψZM indicates the incurred cost of staff surplus/shortage when all workers are single-skilled, whereas ψTM indicates the incurred cost when each worker is trained to work in all departments. Then, the difference between ψZM and ψTM represents the maximum possible savings in this cost when there is total multiskilling.
%SSS=ψZM−ψψZM−ψTM.100 |
This subsection describes the data used in this study. To fairly compare the three optimization approaches under uncertainty (i.e., TSSO, RO, CF), we used the same case study that was already presented and solved by Henao et al. [1] and Henao et al. [2]. The database includes real and simulated data that were derived from a retail store operating in Chile. The real dataset indicates the store size, the number of single-skilled workers initially hired in each store department, working hours established in the employment contract, average weekly staff hours demand per department, and the cost parameters values. The simulated datasets describe the random weekly staff hours demand per department. Below we present a description of both dataset types. For more details of the database, the reader is referred to the data article presented by Henao et al. [53].
The case study evaluates a store with |I|=30 and |L|=6. Such that each worker has a full-time contract according to Chilean labor legislation, that is, h=45. Let ¯rl be the average value of demand in weekly hours per store's department l, ∀l∈L. Such that, ¯r1=315 (i.e., 7 workers), ¯r2=225, ¯r3=135, ¯r4=135, ¯r5=180, and ¯r6=360. Regarding the cost parameters, the training cost per worker is assumed to be the same minimum value for each store department (c=US$1−week/worker). Henao et al. [1,2,9] and Porto et al. [6,21,54] indicate that the results obtained under this assumption can be interpreted as an upper bound of the requirements multiskilling. The staff shortage cost is equal to u=US$60/h, whereas the staff surplus cost is equal to b=US$15/h. Note that the TSSO, RO, and CF approaches use the same real dataset.
The case study considers in-sample data along with out-of-sample data, both associated with the uncertain demand. Regarding the in-sample data, these consider the data utilized to find the in-sample solutions with the TSSO approach. Regarding the out-of-sample data, these consider the data utilized to make fair comparisons between the performance of the solutions obtained by the optimization approaches under uncertainty (i.e., TSSO, RO, and CF) and the approaches considered as myopic (i.e., ZM and TM). In both sample data types, to evaluate how the 2-chaining structures work in the face of different levels of variability demand, we test six coefficients of variation: CV=5,10,20,30,40,50%. In addition, in both sample data types, we consider that the random realizations of the weekly staff hours demand per store's department are independent and normally distributed with known average value ¯rl and standard deviation σl=¯rlCV. Note that, in Henao et al. [1,2], Abello et al. [3], Fontalvo Echavez et al. [20], Porto et al. [6,21], and Mercado et al. [14], articles with case studies in the retail industry, authors also used the same probability density function (pdf) to test the benefits of using multiskilled workers.
For the in-sample data, because an SAA method is used for solving the TSSO model (3)-(17), we employ a Monte Carlo simulation to randomly create 2000 scenarios for the staff demand in weekly hours per store's department rl(s), ∀l∈L,s∈S, such that |S|=2000. This simulation is performed for six different CVs of demand. To determine the appropriate sample size of |S|, we performed a data sample evaluation and identified that the solutions stabilize from 1000 scenarios. We choose the value of |S|=2000, considered as a representative sample size for this case study. Note that 2000 weekly demand scenarios are equivalent to a sample of 38.5 years. Later, we created two different datasets to evaluate and compare the conservatism level in the TSSO approach solutions. Each dataset has a different truncation type in the pdf's of the departments' demand. The first dataset considers that the pdfs are truncated between the percentiles 5th and 95th. That is, 10% of the data distribution is excluded, which avoids the generation of extreme demand values. In addition, the pdfs are truncated at zero. The second dataset considers that the pdfs are zero-truncated, which avoids the generation of negative demand values. However, in this second dataset, extreme demand values could be generated more frequently (in both pdf's tails) as the %CV of demand increases. Thus, the second dataset offers a more conservative point of view than the first dataset. That is, TSSO approach solutions obtained under a more conservative truncation may require higher multiskilling levels than those obtained under less conservative truncation. Note that the CF approach also assumes that the pdf for each department's demand follows a normal distribution truncated at zero.
For the out-of-sample data, to simplify the analyses, we employ a Monte Carlo simulation to create a single dataset for the realizations of the random staff demand in weekly hours in all departments. In this case, following a zero-truncated normal distribution and, for each store department and CV, we generated 10000 scenarios for the demand parameters.
Summarizing, we use three simulated datasets. Two in-sample datasets, of which the first is percentile-truncated and the second is zero-truncated; whereas the out-of-sample dataset is zero-truncated only. There are six files in each dataset (one for each CV), and each of them contains random realizations of the weekly staff hours demand for six departments (2000 for in-sample and 10000 for out-of-sample). The simulated datasets are presented in 18 files classified according to: (i) sample data type (in-sample, out-of-sample); (ii) In which way the normal distribution was truncated (percentile-truncated, zero-truncated); and (iii) coefficient of variation for demand in a department (5, 10, 20, 30, 40, 50%). As mentioned before, these files are available in the data article presented in Henao et al. [53].
This section presents the computational experiments, the results, and the discussions associated with the case study. In Subsection 6.1, the in-sample solutions in terms of the training decisions of the TSSO approach are compared with the solutions of RO, CF, and DT approaches. In Subsection 6.2, we carry out an out-of-sample analysis to evaluate cost and reliability benchmarking the TSSO approach to the alternative optimization approaches under uncertainty (i.e., RO and CF), the deterministic (DT) approach, and the two myopic approaches ZM and TM. The models were written in the AMPL language and solved through ILOG CPLEX 12.9.0, such that for all instances, we obtained optimality gaps of less than 0.1%. Additionally, all test instances were run on Intel Xeon Gold 6148, 2.4GHz - 2.39GHz (two processors) with 256 GB of RAM.
We compared the proposed TSSO model with the benchmark approaches RO, CF, and DT. The DT model presented in Henao et al. [1] was solved using the nominal values of the uncertain demand. The RO and CF approaches were solved as we defined in Subsection 4.2.1 and 4.2.2, respectively. Regarding the RO approach, we evaluated two levels of conservatism or uncertainty budget; Γ = 0.6 and 1. On one side, Γ = 1 represents the worst-case scenario and, therefore, the problem's most conservative solution. On the other hand, Henao et al. [1] indicated in their results that Γ = 0.6 represents a cost-effective value of Γ, since such value achieves savings of at least a 95% on the average total weekly cost of staff surplus/shortage (i.e., %¯SSS≥95%) in the face of different levels of demand uncertainty. The TSSO model (3)-(17) was solved using an SAA approach, as described in Subsection 5.2. The TSSO model solutions are presented considering two in-sample datasets: (i) zero-truncated and (ii) percentile-truncated.
Summarizing, the in-sample analysis compares the performance of the following approaches: (i) two-stage stochastic optimization with a pdf zero-truncated (TSSO-ZT); (ii) two-stage stochastic optimization with a pdf percentile-truncated (TSSO-PT); (iii) robust optimization with Γ = 1 (RO-1); (iv) robust optimization with Γ = 0.6 (RO-0.6); (v) closed-form equation (CF); and (vi) deterministic optimization (DT). To compare in-sample solutions in terms of the training decisions, and only for the approaches TSSO-ZT, TSSO-PT, RO-1, RO-0.6 and CF, we evaluate six different demand variability levels: CV=5,10,20,30,40,50%.
Below, we present the results and discussions of the in-sample analysis in three subsections.
Table 3 reports the computational times and size for each model and CV. We observe that the computational times of the TSSO model are much greater than the RO and CF models, which is because the size of the TSSO model is also larger than the others. TSSO-ZT and TSSO-PT models take an average of 39.2 hours and 28.1 hours, respectively, to solve to optimality. Despite the computational time difference compared to the other models, the TSSO approach has acceptable computational times for real-life applications since decisions related to workers training are considered as tactical-strategic. Thus, staff training decisions are taken over planning horizons of medium and long-term (e.g., months, seasons, semesters).
Approach | CPU time | Average CPU time | Constraints | Binary variables | Continuous variables | |||||
5% | 10% | 20% | 30% | 40% | 50% | |||||
TSSO-ZT | 41.9 h | 109.6 h | 23.3 h | 41.1 h | 13.6 h | 5.4 h | 39.2 h | 384,054 | 150 | 396,048 |
TSSO-PT | 11.3 h | 18.0 h | 37.7 h | 32.8 h | 15.3 h | 53.4 h | 28.1 h | 384,054 | 150 | 396,048 |
RO-1 | 0.39 s | 0.33 s | 0.3 s | 3.83 s | 4.56 s | 0.53 s | 1.66 s | 254 | 150 | 247 |
RO-0.6 | 0.23 s | 0.23 s | 0.7 s | 0.06 s | 0.3 s | 5.66 s | 1.20 s | 254 | 150 | 247 |
CF | 0.02 s | 0.02 s | 0.02 s | 0.02 s | 0.02 s | 0.02 s | 0.02 s | - | - | - |
DT | 0.01 s | 0.01 s | 240 | 150 | 240 |
In addition, as was expected, the DT model has the lowest computational time. However, the computational times of the RO and CF models are also quite short. Regarding RO models, these have very low computational times since their size is almost identical to the DT model. Finally, the computational times of the CF model are also very low because this approach is solved using conventional spreadsheets, which demonstrates its high practical value. Thus, the DT, CF, and RO approaches have the same order of magnitude for finding an optimal solution, while the TSSO approach is several orders of magnitude larger, but reasonable for the tactical MPAP.
The objective in this subsection is to respond the following tactical question: (i) How many multiskilled workers are needed per department? Given this purpose, we calculate the optimal number of multiskilled workers per department (λ∗l) considering six coefficients of variation (CV) in demand and five optimization approaches under uncertainty (TSSO-ZT, TSSO-PT, RO-1, RO-0.6, CF). Figure 2 presents the multiskilling requirements associated with each combination between optimization approach and CV. Recall that, our case study considers a store with six department sizes (DS), whose average values of weekly staff hours are respectively 315,225,135,135,180, and 360. Finally, the DT approach was not considered in this comparison, since its solution does not require any multiskilled worker (i.e., zero multiskilling). This is because, for each department, the available assignment weekly hours (|Il|h) are equal to the average value of weekly hours demand (¯rl). Thus, there is no imbalance between staffing and staff demand in each store department.
In Figure 2, the colored area shows the optimal number of multiskilled workers in each department size (λ∗l). It is observed that as the %CV of demand and the DS increase, λ∗lalso increases. Similarly, for each approach, the sum of the multiskilling requirements for all departments (∑l∈Lλ∗l,%ME,%TM) increase progressively as %CV of demand rises. There are several aspects worth discussing. It is observed that, for each of the five approaches, when CV = 5% (i.e., the minimum value tested for the %CV) a single multiskilled worker is required per department even when the training cost was considered almost free (c=1). Such a result can be seen as the required minimum multiskilling level (i.e., ME=20% and TM=4%). In contrast, for the RO-1, CF, and TSSO-ZT approaches, when CV = 50% (i.e., the maximum value tested for the %CV) all store workers are required to be multiskilled. Such result can be seen as the theoretical maximum (i.e., ∑l∈Lλ∗l=|I|, ME=100%, TM=20%). In fact, once the CV is greater than or equal to 40%, the TSSO-ZT approach requires that 100% of the single-skilled workers be trained to be multiskilled.
Note that when %ME and %TM reach their theoretical maximum, this may involve three possible conclusions. First, ME=100% and TM=20% represent sufficient multiskilling levels to minimize the staff surplus/shortage costs. Second, for very high demand variability levels, 2-chaining structures could not be enough to minimize the staff surplus/shortage costs, and thus, k-chaining structures with k≥2may be explored. That is, chaining structures where a number of workers are trained to perform in two or more departments. Third, for very high demand variability levels, increases in labor flexibility are required but also increases in staffing levels. That is, more workers should be hired.
It is also noteworthy that the conservatism levels in the solutions vary according to the demand variability and the selected approach. Note that, the higher are the required multiskilling levels (%ME, %TM) associated with an approach, the higher is its conservatism level. Considering this criterion, from Figure 2, we order the five approaches according to its conservatism level: (1) TSSO-ZT (the most conservative), (2) RO-1, (3) CF, (4) TSSO-PT, and (5) RO-0.6 (the least conservative). The solutions of the RO-0.6 are the least conservative, mainly because it considers an intermediate uncertainty budget (i.e., Γ = 0.6). In contrast, the RO-1 approach presents solutions more conservative than the solutions of the CF and TSSO-PT approaches. This intuitive result is explained by the fact that the RO-1 approach aims to protect against the worst-case scenario. That is to say, the RO approach as presented in Subsection 4.2.1, implies that for each CV, the weekly hours demand rl is a bound random variable taking the maximum permitted variation, ˆrl=CV¯rl, on the interval [¯rl−ˆrl,¯rl+ˆrl].
Finally, a less intuitive result indicates that the solutions of the TSSO-ZT are more conservative than the solutions of the RO-1 approach, although not very different. However, this result can be explained because the TSSO-ZT approach considers a range of possible demand realizations wider than the RO-1 approach. Recall that, although under the RO-1 approach the demand realizations take the maximum or minimum value on the interval [¯rl−ˆrl,¯rl+ˆrl], the TSSO-ZT uses a dataset where the pdf's of the departments' demand are zero-truncated. Such a dataset represents the maximum theoretical range for the demand realizations in our case of study.
The objective in this subsection is to respond the following two tactical questions: (ii) In which second department should be trained each of the workers identified as multiskilled? and (iii) How many CLCs and/or CSCs are needed, and which store departments should belong to each of the chains? Given this purpose, we analyzed the 2-chaining structures generated according to the demand variability (CV) and the evaluated approach (TSSO-ZT, TSSO-PT, RO-1, RO-0.6, CF). Regarding the RO and CF approaches, once the question (i) has been answered in Subsection 6.1.2, it is required to apply the constructive heuristic developed by Henao et al. [1] to answer the questions (ii) and (iii). The constructive heuristic builds the minimum required number of closed chains, replicating the CLC concept. Unlike RO and CF approaches, the TSSO approach simultaneously answers the tactical questions (i), (ii), and (iii).
As an example, Table 4 presents the set of 2-chaining structures obtained for each approach and a CV of 20%. For each approach, Table 4 shows the optimal total number of multiskilled workers (∑l∈Lλ∗l), the constructed closed-chains, and what types of closed chains were built.
Approach | ∑l∈Lλ∗l | Closed chains | Type of closed chain |
TSSO-ZT | 19 | D1-D3-D5-D6-D4-D2-D1 | CLC |
D5-D4-D1-D6-D2-D5 | CSC | ||
D2-D3-D6-D2 | CSC | ||
D1-D5-D6-D1 | CSC | ||
D1-D6-D1 | CSC | ||
RO-1 | 15 | D3-D1-D4-D5-D6-D2-D3 | CLC |
D1-D2-D6-D3-D5-D1 | CSC | ||
D4-D6-D4 | CSC | ||
D1-D6-D1 | CSC | ||
CF | 12 | D3-D1-D4-D5-D6-D2-D3 | CLC |
D1-D5-D2-D6-D1 | CSC | ||
D1-D6-D1 | CSC | ||
TSSO-PT | 12 | D1-D3-D6-D5-D4-D1 | CSC |
D1-D2-D5-D6-D1 | CSC | ||
D1-D6-D2-D1 | CSC | ||
RO-0.6 | 11 | D1-D2-D4-D3-D6-D5-D1 | CLC |
D2-D1-D6-D2 | CSC | ||
D1-D6-D1 | CSC |
Table 4 shows that under the TSSO-ZT approach, five closed chains were built, the first one is a CLC while the other four are CSCs. For example, the constructed chain "D1-D5-D6-D1" indicated that a worker from Department 1 was also trained in Department 5, a worker from Department 5 was also trained in Department 6, and a worker from Department 6 was also trained in Department 1. The RO-1, CF, and RO-0.6 approaches also combine the two closed-chain types (CSCs of not the same length and CLCs). In addition, the TSSO-PT approach constructs three closed short chains of not the same length but no closed long chain. This is a valuable result since it indicates that under a TSSO approach, where all tactical questions were answered simultaneously, various CSCs, each one connecting a significant number of departments, perform as well as a combination of CLCs and CSCs. This result extends the findings reported by Henao et al. [1] and Henao et al. [2].
In addition, for the RO-1, CF, and R0-0.6 approaches, the constructive heuristic seeks to maximize flexibility in the training plans. That is, if possible, it is avoided that the multiskilled workers belonging to a given department have been trained in the same additional departments. The solutions of the TSSO-ZT and TSSO-PT approaches show also that the flexibility is maximized. However, in the set of constructed closed-chains for the TSSO-ZT approach, four training arcs are repeated: "D1-D6", "D5-D6", "D6-D2", and "D6-D1". This is also a valuable result since it indicates that, under a TSSO approach, connecting pairs of departments with large demands more than once is a good strategy. Recall that D1, D2, D5, and D6 represent the departments with the largest sizes in our case study. This result also extends the findings reported by Henao et al. [1] and Henao et al. [2].
Finally, it is important to highlight that, given the methodology used by the RO and CF approaches, it would not have been possible to find these new findings with such approaches. That is, the second stage of the RO and CF approaches consists of using a constructive heuristic to answer the questions (ii) and (iii), and thus, such a heuristic uses a set of intuitive decision rules (set by the modeler) to create an attractive set of CLCs and CSCs. However, being a heuristic procedure, it explores only a portion of the entire feasible region of solutions. This does not happen with the simultaneous TSSO approach, which explores the entire feasible set of solutions. This feature of the TSSO approach, although it comes with a high computational cost, allowed us to identify and provide new managerial insights to practitioners and retail decision-makers.
The out-of-sample evaluation presented in this subsection aims to fairly compare the solutions of the TSSO-ZT and TSSO-PT approaches with the solutions of the alternative optimization approaches, RO-1, RO-0.6, and CF. Besides, we evaluate the myopic approaches, i.e., ZM and TM. We remark that, in our case study, the solution of the DT approach is equivalent to the ZM approach. The benchmark evaluation is made by measuring and comparing the performance solutions of these seven approaches. We employ metrics that measure the incurred costs (φ,ψ) and the reliability (%SSS) associated with the solutions delivered by each approach. We use a Monte Carlo simulation to generate 10000 random realizations of the weekly demand per store's department and CV of demand using a normal probability distribution truncated at zero. Then, for each approach and CV, we compute the total weekly cost (φ) and the weekly staff surplus/shortage cost (ψ) related to each of the 10000 demand scenarios by using the multiskilling structures obtained in Subsection 6.1. After, the average costs, ¯φ and ¯ψ, are calculated.
For each approach and CV, Table 5 presents in-sample results along with out-of-sample results. Columns 2 and 3 show the requirements of multiskilling (%ME,%TM) obtained in the in-sample results. Columns 4, 5, and 6 show the metrics related to the out-of-sample results. Column 4 indicates the percentage savings on the average total weekly cost of staff surplus/shortage (%¯SSS); which represents a measure of reliability. Column 5 reports the average total weekly cost (¯φ). Finally, Column 6 shows a metric that we call homogeneous group metric. This metric summarizes the results of an inferential analysis performed on the average total costs (¯φ) of the seven approaches. Following the Fisher's least significant difference (LSD) procedure, we construct 21 confidence intervals for the difference of the average total costs, as a result of the possible combinations between each pair of approaches (C72=7!/2!(7−2)!=21). The LSD procedure allows to detect significant difference between each pair of approaches. Considering a confidence level equal to 95%, the approaches with X's in the same subcolumn do not present significant differences among them, whereas those that are not in the same subcolumn do present significant differences. Note that, approaches with X's (in bold) in subcolumn "A" have the best performance (i.e., the most cost-effective approaches) in the face of uncertain demand.
In-sample metrics | Out-of-sample metrics | |||||||
Approaches | %ME | %TM | %¯SSS | ¯φ (US$) | Homogeneous groups | |||
A | B | C | D | |||||
CV = 5% | ||||||||
TSSO-ZT | 20 | 4 | 100 | 882 | X | |||
RO-1 | 20 | 4 | 100 | 882 | X | |||
CF | 20 | 4 | 100 | 882 | X | |||
TSSO-PT | 20 | 4 | 100 | 882 | X | |||
RO-0.6 | 20 | 4 | 100 | 882 | X | |||
TM | 100 | 100 | 100 | 1,026 | X | |||
ZM (DT) | 0 | 0 | 0 | 2,013 | X | |||
CV = 10% | ||||||||
TSSO-ZT | 33 | 7 | 100 | 1,798 | X | |||
TSSO-PT | 27 | 5 | 100 | 1,806 | X | |||
RO-1 | 27 | 5 | 99 | 1,813 | X | X | ||
CF | 27 | 5 | 99 | 1,813 | X | X | ||
RO-0.6 | 23 | 5 | 97 | 1,859 | X | |||
TM | 100 | 100 | 100 | 1,937 | X | |||
ZM (DT) | 0 | 0 | 0 | 4,046 | X | |||
CV = 20% | ||||||||
TSSO-ZT | 63 | 13 | 100 | 3,561 | X | |||
RO-1 | 50 | 10 | 100 | 3,573 | X | |||
TSSO-PT | 40 | 8 | 98 | 3,649 | X | X | ||
CF | 40 | 8 | 98 | 3,660 | X | X | ||
TM | 100 | 100 | 100 | 3,692 | X | |||
RO-0.6 | 37 | 7 | 94 | 3,811 | X | |||
ZM (DT) | 0 | 0 | 0 | 8,124 | X | |||
CV = 30% | ||||||||
TSSO-ZT | 87 | 17 | 100 | 5,324 | X | |||
RO-1 | 67 | 13 | 99 | 5,363 | X | |||
CF | 63 | 13 | 99 | 5,371 | X | |||
TSSO-PT | 60 | 12 | 99 | 5,413 | X | |||
TM | 100 | 100 | 100 | 5,445 | X | X | ||
RO-0.6 | 53 | 11 | 96 | 5,594 | X | |||
ZM (DT) | 0 | 0 | 0 | 12,154 | X | |||
CV = 40% | ||||||||
TSSO-ZT | 100 | 20 | 100 | 7,190 | X | |||
RO-1 | 90 | 18 | 99 | 7,237 | X | |||
CF | 87 | 17 | 99 | 7,238 | X | |||
TM | 100 | 100 | 100 | 7,297 | X | X | ||
TSSO-PT | 73 | 15 | 98 | 7,344 | X | X | ||
RO-0.6 | 67 | 13 | 97 | 7,464 | X | |||
ZM (DT) | 0 | 0 | 0 | 16,104 | X | |||
CV = 50% | ||||||||
TSSO-ZT | 100 | 20 | 99 | 9,309 | X | |||
RO-1 | 100 | 20 | 99 | 9,326 | X | |||
CF | 100 | 20 | 99 | 9,326 | X | |||
TSSO-PT | 93 | 19 | 99 | 9,354 | X | |||
TM | 100 | 100 | 100 | 9,365 | X | |||
RO-0.6 | 87 | 17 | 98 | 9,508 | X | |||
ZM (DT) | 0 | 0 | 0 | 20,170 | X |
From Table 5, several aspects can be highlighted. First, the TSSO-ZT approach always delivers the solution with the lowest average total cost (i.e., ¯φ), whereas the ZM approach always delivers the solution with the highest average total cost. This result is expected given that, except for the TM approach, the TSSO-ZT approach has the highest conservatism level, and the ZM approach does not provide any protection for its solutions since %ME = %TM = 0%.
Second, for each CV, the metric %¯SSS allows us to assess the reliability and robustness of the solutions. As expected, the TM approach exhibits the highest possible reliability and robustness. That is, the TM approach reaches the maximum feasible reduction on the average total weekly cost of staff surplus/shortage (%¯SSS = 100%), although it also needs the maximum investment in multiskilling since %ME = %TM = 100%. Conversely, the ZM approach represents no investment in multiskilling, such that %ME = %TM = %¯SSS = 0%. In addition, when the approaches TSSO-ZT, RO-1, CF, TSSO-PT, and RO-0.6 are compared, we found that there are similar values of %¯SSS among these five approaches. In turn, the values of this metric were also very close to those reported for the TM approach.
Third, for each CV, the homogeneous group metric indicates that TSSO-ZT, RO-1, CF, and TSSO-PT always belong to the set of approaches with the lowest average total cost (see subcolumn A). In this group, there are not statistically significant differences from one approach to another, so these four approaches are the most cost-effective. Also, the RO-0.6 approach is shown as cost-effective only when CV = 5% or CV = 50%, i.e., the minimum and maximum values tested for the %CV. In turn, the TM approach is cost-effective only when the CV is high (≥30%). This result is interesting because it can reinforce the hypothesis described in the in-sample analysis, in which it was expressed that for very high demand variability levels, 2-chaining structures could not be enough to minimize the staff surplus/shortage costs. Therefore, it would be valuable to study two additional policies: (i) evaluate k-chaining structures with k≥2; and (ii) consider multiskilling and staff size decisions simultaneously.
Fourth, we carry out an analysis to provide understanding on how to select the most suitable approach(es) according to the balance between the conservatism (i.e., %ME reported in the in-sample analysis) and the reliability (i.e., %¯SSS reported in the out-of-sample analysis) associated with the solutions delivered by each approach. Figure 3 shows that as the CV of demand rises, the percentage of multiskilled workers (%ME) also increases for each approach. In addition, for each combination of %CV and approach, Figure 3 also shows the value of the %¯SSS metric. Note that, the TM and ZM (DT) approaches represent the upper and lower bound of the level of conservatism, respectively. In addition, it is interesting to note that, as indicated in Table 5 and Figure 3, even when a minimal training cost was assumed, the approaches TSSO-ZT, RO-1, CF, and TSSO-PT achieve between 98% and 100% of the possible benefits of total multiskilling but requiring much lower investments. Besides, the RO-0.6 approach achieves savings of at least a 94% on the average total weekly cost of staff surplus/shortage (i.e., %¯SSS≥94%,∀ CV), even with a lower multiskilling investment than the one required by the other four optimization approaches under uncertainty.
Finally, from Figure 3, we order the approaches according to the level of conservatism as reported in the solutions: (1) TM (the most conservative), (2) TSSO-ZT, (3) RO-1, (4) CF, (5) TSSO-PT, (6) RO-0.6, and (7) ZM (DT) (the least conservative). We remark that the TSSO-ZT, TSSO-PT, and CF approaches assume complete knowledge of the distributional information, whereas the RO-1 and RO-0.6 approaches do not require this full knowledge of the stochastic parameters. In addition, the TSSO approach can simultaneously answer the tactical questions (i), (ii), and (iii), and not sequentially as RO and CF approaches do. Thus, from the results, we observe that the suitable approach under demand uncertainty will depend on the preferred solution method (simultaneous or sequential), the decision-maker's knowledge on the probability distribution, and his/her degree of risk-aversion over the decision.
This article studies the weekly multiskilled personnel assignment problem (MPAP) considering 2-chaining and uncertain demand. The solution of the MPAP generates staff training plans structured through closed chains (CLCs, and CSCs) and where the workers could be trained for only one additional task type. The contribution of this paper is twofold. Firstly, we developed a TSSO approach to calculate the multiskilling requirements that minimize the training costs and the expected costs of staff surplus/shortage. Secondly, we evaluated and compared the performance of the solutions generated by the TSSO approach with the solutions of alternative optimization approaches under uncertainty (i.e., RO and CF), the solution of deterministic (DT) approach, and the solutions of myopic approaches (i.e., ZM and TM).
The methodology was tested for a case study associated with a retail store operating in Chile. Through in-sample and out-of-sample analyses employing real and simulated data, we evaluated and compared the different approaches by testing six representative levels of staff demand per department. On the in-sample analysis, we evaluated the performance of the optimization approaches under uncertainty TSSO, RO, and CF, such that the findings can be grouped under the following two aspects. First, given the reported computational times, the RO and CF approaches can be useful for larger problems; more store departments and workers. Second, the obtained results under the TSSO approach and its comparison with the other approaches allow us to incorporate new guidelines to design practical and effective policies that answer the following three tactical questions: (i) How many multiskilled workers are needed per department? (ii) In which second department should be trained each of the workers identified as multiskilled? (iii) How many CLCs and/or CSCs are needed, and which store departments should belong to each of the chains? We remark that, unlike RO and CF approaches, the TSSO approach simultaneously answers the tactical questions (i), (ii), and (iii).
Regarding the first question, the results of the TSSO, RO, and CF approaches showed that, even when the cost of training was considered almost free, total multiskilling is impractical and is not required to achieve the full possible benefits associated with multiskilling. In addition, our results (intuitively) showed that the required number of multiskilled workers rises as the department size and the level of demand variability grow. Finally, we found a less intuitive result when the solutions to the optimization approaches under uncertainty were compared. The solutions of the TSSO approach where the demand parameters follow a zero-truncated normal distribution (i.e., TSSO-ZT) were more conservative than the solutions of the RO approach with the maximum uncertainty budget (i.e., worst-case scenario). Note that, the higher are the required multiskilling levels associated with an approach, the higher is its level of conservatism.
Regarding the second and third questions, we found that the most profitable staff is one where there is a mix of single-skilled and multiskilled workers. This result is observed with all optimization approaches under uncertainty. In turn, and in a novel way, we also found that under a TSSO approach, various CSCs, each one connecting a significant number of departments, perform as well as a combination of CLCs and CSCs. Finally, under the RO and CF approaches, it seeks to maximize flexibility in the training plans, that is, avoiding that the multiskilled workers belonging to a given department were trained in the same additional departments. However, under the TSSO approach, we discovered that closed chains connecting pairs of departments with large demands more than once also exhibited excellent performance.
On the out-of-sample analysis, we evaluated the performance of the optimization approaches under uncertainty TSSO, RO, and CF, the deterministic approach DT, and the two myopic approaches TM and ZM. The findings provided understanding on how to select the most suitable approach(es) according to the balance between the conservatism and the reliability associated with the solutions delivered by each approach. Particularly, it was shown that the TSSO-ZT approach always delivers the solution with the lowest average total cost. However, the main finding was that TSSO-ZT, RO-1, CF, and TSSO-PT always belong to the set of approaches with the lowest average total cost. That is, in this group, there are not statistical differences from one approach to another, so these approaches are the most cost-effective. This finding showed that there is not only one approach to effectively solve the MPAP with 2-chaining under uncertain demand. Finally, considering the overall results, we concluded that the choice of the most suitable approach would depend on the preferred solution method (i.e., simultaneous or sequential methodology), the available information of the distribution of probability associated with the uncertain demand, and the level of risk tolerance of the store manager.
As for future research, the methodology could incorporate new additional features, or some assumptions could be relaxed. (1) Assess k-chaining structures with k≥2where a number of workers are trained to perform in two or more departments. (2) Solve simultaneously a staffing and personnel assignment problem considering closed-chained multiskilling structures. (3) Solve the MPAP under uncertain demand by a distributionally robust optimization, which is a suitable approach for ambiguity-averse decision-makers.
The authors thank Shift's colleagues for supplying the real data used in this investigation. Authors also thank Dr. David Pozo at Skoltech Faculty for his recommendations and valuable support in defining of the solution methodology in this study. Finally, this investigation was aided by "Fundación para la Promoción de la Investigación y la Tecnología (FPIT)" with the Grant Number 4.523.
All authors declare no conflicts of interest in this paper.
[1] | Marusciac, On Fritz John type optimality criterion in multi-objective optimization, Anal. Numer. Theor. Approx., 11 (1982), 109-114. |
[2] | G. Palermo, C. Silvano, S. Valsecchi, et al. A system-level methodology for fast multi-objective design space exploration, ACM, New York, 2003. |
[3] | M. Tavana, A subjective assessment of alternative mission architectures for the human exploration of mars at NASA using multicriteria decision making, Comput. Oper. Res., 31 (2004), 1147-1164. |
[4] | H. Eschenauer, J. Koski, A. Osyczka, Multicriteria design optimization: Procedures and applications, Springer-Verlag, Berlin, 1990. |
[5] | I. Das, J. E. Dennis, Normal-Boundary intersection: A new method for generating the Pareto surface in nonlinear multicriteria optimization problems, SIAM J. Optim.,8 (1998), 631-657. |
[6] | I. Y. Kim, O. L. de Weck, Adaptive weighted-sum method for bi-objective optimization: Pareto front generation, Struct. Multidisc. Optim., 29 (2005), 149-158. |
[7] | Y. Fu, U. M. Diwekar, An efficient sampling approach to multiobjective optimization, Ann. Oper. Res., 132 (2004), 109-134. |
[8] | A. Jüschke, J. Jahn, A. Kirsch, A bicriterial optimization problem of antenna design, Comput. Optim. Appl., 7 (1997), 261-276. |
[9] | T. M. Leschine, H. Wallenius, W. A. Verdini, Interactive multiobjective analysis and assimilative capacity-based ocean disposal decisions, Eur. J. Oper. Res., 56 (1992), 278-289. |
[10] | J. Fliege, OLAF-A general modeling system to evaluate and optimize the location of an air polluting facility, OR Spektrum, 23 (2001), 117-136. |
[11] | E. Carrizosa, J. B. G. Frenk, Dominating sets for convex functions with some applications, J. Optim. Theory Appl., 96 (1998), 281-295. |
[12] | G. W. Evan, An overview of techniques for solving multiobjective mathematical programs, Manage. Sci., 30 (1984), 1268-1282. |
[13] | D. Prabuddha, J. B. Ghosh, C. E. Wells, On the minimization of completion time variance with a bicriteria extension, Oper. Res., 40 (1992), 1148-1155. |
[14] | D. J. White, Epsilon-dominating solutions in mean-variance portfolio analysis, Eur. J. Oper. Res., 105 (1998), 457-466. |
[15] | M. G. C. Tapia, C. A. C. Coello, Applications of multi-objective evolutionary algorithms in economics and finance: A survey, IEEE Congr. Evol. Comput., (2007), 532-539. |
[16] | E. Zitzler, K. Deb, L. Thiele, Comparison of multiobjective evolutionary algorithms: Empirical results, Evol. Comput., 8 (2000), 173-195. |
[17] | M. Ehrgott, Multicriteria optimization, Springer, Berlin, 2005. |
[18] | K. Miettinen, M. M. Makelä, Interactive bundle-based method for nondifferentiable multiobjeective optimization: NIMBUS, Optimization, 34 (1995), 231-246. |
[19] | L. M. G. Drummond, B. F. Svaiter, A steepest descent method for vector optimization, J. Comput. Appl. Math., 175 (2005), 395-414. |
[20] | J. Fliege, L. M. G. Drummond, B. F. Svaiter, Newton's method for multiobjective optimization, SIAM J. Optim., 20 (2009), 602-626. |
[21] | A. Cauchy, Méthode générale pour la résolution des systèms d'équations simultanées, Comp. Rend. Sci. Paris, 25 (1847), 536-538. |
[22] | H. Zhao, J. Zheng, W. Deng, et al. Semi-supervised broad learning system based on manifold regularization and broad network, IEEE Trans. Circuits Syst., 67 (2020), 983-994. |
[23] | W. Deng, J. Xu, Y. Song, et al. An effective improved co-evolution ant colony optimization algorithm with multi-strategies and its application, Int. J. Bio-Inspir. Com., (2020) 1-10. |
[24] | W. Deng, J. Xu, H. Zhao, An improved ant colony optimization algorithm based on hybrid strategies for scheduling problem, IEEE access, 7 (2019), 20281-20292. |
[25] | W. Deng, H. Liu, J. Xu, et al. An improved quantum-inspired differential evolution algorithm for deep belief network, IEEE Trans. Instrum. Meas., 2020. |
[26] | S. K. Mishra, B. Ram, Introduction to Unconstrained Optimization with R, Springer Nature, Singapore, 2019. |
[27] | J. Fliege, B. F. Svaiter, Steepest descent methods for multicriteria optimization, Math. Method Oper. Res., 51 (2000), 479-494. |
[28] | T. D. Chuong, J. C. Yao, Steepest descent methods for critical points in vector optimization problems, Appl. Anal., 91 (2012), 1811-1829. |
[29] | J. Fliege, A. I. F. Vaz, L. N. Vicente, Complexity of gradient descent for multiobjective optimization, Optim. Method Softw., 34 (2019), 949-959. |
[30] | F. H. Jackson, On q-functions and a certain difference operator, Trans. Roy Soc. Edin., 46 (1908) 253-281. |
[31] | F. H. Jackson, On q-Definite Integrals, Pure Appl. Math. Q., 41 (1910), 193-203. |
[32] | A. Aral, V. Gupta, R. P. Agarwal, Applications of q-calculus in operator theory, Springer, New York, 2013. |
[33] | P. M. Rajković, M. S. Stanković, S. D. Marinković, Mean value theorems in q-calculus, Mat. Vesn., 54 (2002), 171-178. |
[34] | M. E. Ismail, D. Stanton, Applications of q-Taylor theorems, J. Comput. Appl. Math., 153 (2003), 259-272. |
[35] | S. C. Jing, H. Y. Fan, q-Taylor's formula with its q-remainder, Commun. Theor. Phys., 23 (1995), 117. |
[36] | P. M. Rajković, S. D. Marinković, M. S. Stanković, Fractional integrals and derivatives in qcalculus, Appl. Anal. Discrete Math., 1 (2007), 311-323. |
[37] | H. Gauchman, Integral inequalities in q-calculus, Comput. Math. Appl., 47 (2004), 281-300. |
[38] | G. Bangerezako, Variational q-calculus, J. Math. Anal. Appl., 289 (2004), 650-665. |
[39] | L. Abreu, A q-sampling theorem related to the q-Hankel transform, Proc. Am. Math. Soc., 133 (2005), 1197-1203 |
[40] | T. H. Koornwinder, R. F. Swarttouw, On q-analogues of the Fourier and Hankel transforms, Trans. Am. Math. Soc., 333 (1992), 445-461. |
[41] | J. Ablinger, A. K. Uncu, qFunctions-A Mathematica package for q-series and partition theory applications, arXiv preprint arXiv:1910.12410, 2019. |
[42] | A. C. Soterroni, R. L. Galski, F. M. Ramos, The q-gradient vector for unconstrained continuous optimization problems, In: Operations research proceedings 2010. Springer-Verlag Berlin Heidelberg, (2011), 365-370. |
[43] | A. C. Soterroni, R. L. Galski, F. M. Ramos, The q-gradient method for global optimization, arXiv:1209.2084, 2012. |
[44] | A. C. Soterroni1, R. L. Galski, M. C. Scarabello1, et al. The q-G method: A q-version of the steepest descent method for global optimization, SpringerPlus, 4 (2015), 647. |
[45] | A. C. Soterroni, R. L. Galski, F. M. Ramos, Satellite constellation design using the q-G global optimization method, Third World Conference on Complex Systems (WCCS), IEEE, (2015), 1-6. |
[46] | U. M. Al-Saggaf, M. Moinuddin, M. Arif, et al. The q-least mean squares algorithm, Signal Process., 111 (2015), 50-60. |
[47] | A. Sadiq, S. Khan, I. Naseem, et al. Enhanced q-least mean square, Circ. Syst. Signal Process., 38 (2019), 4817-4839. |
[48] | P. M. Rajković, S. D. Marinković, M. S. Stanković, On q-Newton-Kantorovich method for solving systems of equations, Appl. Math. Comput., 168 (2005), 1432-1448. |
[49] | S. K. Chakraborty, G. Panda, Newton like line search method using q-calculus, In: D. Giri, R. N. Mohapatra, H. Begehr, M. Obaidat, Communications in Computer and Information Science, Singapore: Springer, 2017. |
[50] | S. K. Mishra, G. Panda, M. A. T. Ansary, et al. On q-Newton's method for unconstrained multiobjective optimization problems, J. Appl. Math. Comput., (2020), 1-20. |
[51] | Ž. Povalej, Quasi-Newton's method for multiobjective optimization, J. Comput. Appl. Math., 255 (2014), 765-777. |
[52] | K. K. Lai, S. K. Mishra, B. Ram, On q-Quasi-Newton's Method for Unconstrained Multiobjective Optimization Problems, Mathematics, 8 (2020), 616. |
[53] | M. A. T. Ansary, G. Panda, A sequential quadratically constrained quadratic programming technique for a multi-objective optimization problem, Eng. Optim., 51 (2019), 22-41. |
[54] | W. E. Karush, Minima of functions of several variables with inequalities as side conditions. PhD thesis, Univ. Chicago, 1939. 55. M. A. Ansary, G. Panda, A modified quasi-Newton method for vector optimization problem, Optimization, 64 (2015), 2289-2306. |
[55] | M. A. T. Ansary, G. Panda, A modified quasi-Newton method for vector optimization problem, Optimization, 64 (2015), 2289-2306. |
[56] | K. Deb, L. Thiele, M. Laumanns, et al. Scalable multi-objective optimization test problems, IEEE, 1 (2002), 825-830. |
[57] | O. P. Ferreira, M. S. Louzeiro, L. F. Prudente, Iteration-complexity and asymptotic analysis of steepest descent method for multiobjective optimization on Riemannian manifolds, J. Optim. Theory Appl., 184 (2020), 507-533. |
1. | Hojjatollah Amiri Kayvanloo, Mohammad Mehrabinezhad, Mahnaz Khanehgir, Reza Allahyari, Mohammad Mursaleen, Solvability of an infinite system of n-th order differential equations in a paranormed sequence space and its numerical solution, 2025, 10, 2473-6988, 16804, 10.3934/math.2025755 |
Reference | PSP | MS | 2-CH | SM | AMU | Application |
Bard et al. [30] | S + TS | No | – | IP + H | TSSO | Postal service |
Zhu & Sherali [31] | S + SS | Var | No | MIP + BD | TSSO | Service centers |
Robbins & Harrison [32] | S + TS | No | – | MIP + BD | TSSO | Call Centers |
Campbell [33] | DOS | Par | No | IP + H | TSSO | Health |
Liao et al. [34] | S + A | No | – | MIP | TSSO, 1RO | Call Centers |
Liao et al. [35] | S + SS | No | – | MIP | TSSO, DRO | Call Centers |
Gnanlet & Gilland [36] | S + A | Var | Yes | MIP + AN | TSSO, CF | Health |
Paul & MacDonald [37] | S + A | Var | Yes | MIP + H + AN | CF | Health |
Kim & Mehrotra [38] | S + TS | No | – | MIP + M | TSSO | Health |
Parisio & Jones [39] | TS | No | – | MIP | TSSO | Retail |
Henao et al. [1] | A | Var | Yes | MIP + H | 1RO | Retail |
Bodur & Luedtke [40] | S + SS | Par | Yes | MIP + BD + B & C | TSSO | Call Centers |
Liu [18] | S + A | Par | Yes | IP + AN | TSSO, CF | – |
Mattia et al. [41] | S + SS | No | – | IP + BD + B & C | 2RO | Call Centers |
Restrepo et al. [42] | TS | Par | No | MIP + M | TSSO | – |
Altner et al. [43] | S + SS | No | – | IP + CG | TSSO | CSOC |
Altner et al. [44] | DOS | Var | No | MIP + M | TSSO | CSOC |
Henao et al. [2] | A | Var | Yes | AN + H + LP | CF | Retail |
Fontalvo Echavez et al. [20] | DOS | Var | Yes | MIP | TSSO | Retail |
This paper | A | Var | Yes | MIP + H + AN | TSSO, RO, CF | Retail |
Sets | |
I | Workers, indexed by i |
L | Departments of the retail store, indexed by l |
S | Scenarios of demand, indexed by s |
Il | Set of single-skilled workers initially hired in department l, indexed by i |
Parameters | |
rl(s) | Random parameter of staff demand in weekly hours per store's department l, ∀l∈L,s∈S |
¯rl | Average value of staff demand in weekly hours per store's department l, ∀l∈L |
c | Cost of workers training in any department; [US$-week /Worker] |
u | Staff shortage cost per store's department; [US$/Hour] |
b | Staff surplus cost per store's department; [US$/Hour] |
h | Weekly hours that workers have to work in accordance with their employment contract |
mi | Department in which the worker i is initially trained, ∀i∈I |
Variables | |
xil | 1 if worker i is trained in department l, 0 otherwise, ∀i∈I,l∈L |
vi | 1 if worker i is multiskilled, 0 otherwise, ∀i∈I |
λl | Number of multiskilled workers belonging to department l, ∀l∈L |
Ω | Number of generated closed-chains |
ωil | Number of weekly working hours allocated to worker i in department l, ∀i∈I,l∈L |
κl | Number of staff shortage hours per week in department l,∀l∈L |
δl | Number of staff surplus hours per week in department l,∀l∈L |
Solution approaches for the MPAP with 2-chaining | |
DT | Deterministic optimization |
RO | Robust optimization |
CF | Closed-form equation |
TSSO | Two-stage stochastic optimization |
Approach | CPU time | Average CPU time | Constraints | Binary variables | Continuous variables | |||||
5% | 10% | 20% | 30% | 40% | 50% | |||||
TSSO-ZT | 41.9 h | 109.6 h | 23.3 h | 41.1 h | 13.6 h | 5.4 h | 39.2 h | 384,054 | 150 | 396,048 |
TSSO-PT | 11.3 h | 18.0 h | 37.7 h | 32.8 h | 15.3 h | 53.4 h | 28.1 h | 384,054 | 150 | 396,048 |
RO-1 | 0.39 s | 0.33 s | 0.3 s | 3.83 s | 4.56 s | 0.53 s | 1.66 s | 254 | 150 | 247 |
RO-0.6 | 0.23 s | 0.23 s | 0.7 s | 0.06 s | 0.3 s | 5.66 s | 1.20 s | 254 | 150 | 247 |
CF | 0.02 s | 0.02 s | 0.02 s | 0.02 s | 0.02 s | 0.02 s | 0.02 s | - | - | - |
DT | 0.01 s | 0.01 s | 240 | 150 | 240 |
Approach | ∑l∈Lλ∗l | Closed chains | Type of closed chain |
TSSO-ZT | 19 | D1-D3-D5-D6-D4-D2-D1 | CLC |
D5-D4-D1-D6-D2-D5 | CSC | ||
D2-D3-D6-D2 | CSC | ||
D1-D5-D6-D1 | CSC | ||
D1-D6-D1 | CSC | ||
RO-1 | 15 | D3-D1-D4-D5-D6-D2-D3 | CLC |
D1-D2-D6-D3-D5-D1 | CSC | ||
D4-D6-D4 | CSC | ||
D1-D6-D1 | CSC | ||
CF | 12 | D3-D1-D4-D5-D6-D2-D3 | CLC |
D1-D5-D2-D6-D1 | CSC | ||
D1-D6-D1 | CSC | ||
TSSO-PT | 12 | D1-D3-D6-D5-D4-D1 | CSC |
D1-D2-D5-D6-D1 | CSC | ||
D1-D6-D2-D1 | CSC | ||
RO-0.6 | 11 | D1-D2-D4-D3-D6-D5-D1 | CLC |
D2-D1-D6-D2 | CSC | ||
D1-D6-D1 | CSC |
In-sample metrics | Out-of-sample metrics | |||||||
Approaches | %ME | %TM | %¯SSS | ¯φ (US$) | Homogeneous groups | |||
A | B | C | D | |||||
CV = 5% | ||||||||
TSSO-ZT | 20 | 4 | 100 | 882 | X | |||
RO-1 | 20 | 4 | 100 | 882 | X | |||
CF | 20 | 4 | 100 | 882 | X | |||
TSSO-PT | 20 | 4 | 100 | 882 | X | |||
RO-0.6 | 20 | 4 | 100 | 882 | X | |||
TM | 100 | 100 | 100 | 1,026 | X | |||
ZM (DT) | 0 | 0 | 0 | 2,013 | X | |||
CV = 10% | ||||||||
TSSO-ZT | 33 | 7 | 100 | 1,798 | X | |||
TSSO-PT | 27 | 5 | 100 | 1,806 | X | |||
RO-1 | 27 | 5 | 99 | 1,813 | X | X | ||
CF | 27 | 5 | 99 | 1,813 | X | X | ||
RO-0.6 | 23 | 5 | 97 | 1,859 | X | |||
TM | 100 | 100 | 100 | 1,937 | X | |||
ZM (DT) | 0 | 0 | 0 | 4,046 | X | |||
CV = 20% | ||||||||
TSSO-ZT | 63 | 13 | 100 | 3,561 | X | |||
RO-1 | 50 | 10 | 100 | 3,573 | X | |||
TSSO-PT | 40 | 8 | 98 | 3,649 | X | X | ||
CF | 40 | 8 | 98 | 3,660 | X | X | ||
TM | 100 | 100 | 100 | 3,692 | X | |||
RO-0.6 | 37 | 7 | 94 | 3,811 | X | |||
ZM (DT) | 0 | 0 | 0 | 8,124 | X | |||
CV = 30% | ||||||||
TSSO-ZT | 87 | 17 | 100 | 5,324 | X | |||
RO-1 | 67 | 13 | 99 | 5,363 | X | |||
CF | 63 | 13 | 99 | 5,371 | X | |||
TSSO-PT | 60 | 12 | 99 | 5,413 | X | |||
TM | 100 | 100 | 100 | 5,445 | X | X | ||
RO-0.6 | 53 | 11 | 96 | 5,594 | X | |||
ZM (DT) | 0 | 0 | 0 | 12,154 | X | |||
CV = 40% | ||||||||
TSSO-ZT | 100 | 20 | 100 | 7,190 | X | |||
RO-1 | 90 | 18 | 99 | 7,237 | X | |||
CF | 87 | 17 | 99 | 7,238 | X | |||
TM | 100 | 100 | 100 | 7,297 | X | X | ||
TSSO-PT | 73 | 15 | 98 | 7,344 | X | X | ||
RO-0.6 | 67 | 13 | 97 | 7,464 | X | |||
ZM (DT) | 0 | 0 | 0 | 16,104 | X | |||
CV = 50% | ||||||||
TSSO-ZT | 100 | 20 | 99 | 9,309 | X | |||
RO-1 | 100 | 20 | 99 | 9,326 | X | |||
CF | 100 | 20 | 99 | 9,326 | X | |||
TSSO-PT | 93 | 19 | 99 | 9,354 | X | |||
TM | 100 | 100 | 100 | 9,365 | X | |||
RO-0.6 | 87 | 17 | 98 | 9,508 | X | |||
ZM (DT) | 0 | 0 | 0 | 20,170 | X |
Reference | PSP | MS | 2-CH | SM | AMU | Application |
Bard et al. [30] | S + TS | No | – | IP + H | TSSO | Postal service |
Zhu & Sherali [31] | S + SS | Var | No | MIP + BD | TSSO | Service centers |
Robbins & Harrison [32] | S + TS | No | – | MIP + BD | TSSO | Call Centers |
Campbell [33] | DOS | Par | No | IP + H | TSSO | Health |
Liao et al. [34] | S + A | No | – | MIP | TSSO, 1RO | Call Centers |
Liao et al. [35] | S + SS | No | – | MIP | TSSO, DRO | Call Centers |
Gnanlet & Gilland [36] | S + A | Var | Yes | MIP + AN | TSSO, CF | Health |
Paul & MacDonald [37] | S + A | Var | Yes | MIP + H + AN | CF | Health |
Kim & Mehrotra [38] | S + TS | No | – | MIP + M | TSSO | Health |
Parisio & Jones [39] | TS | No | – | MIP | TSSO | Retail |
Henao et al. [1] | A | Var | Yes | MIP + H | 1RO | Retail |
Bodur & Luedtke [40] | S + SS | Par | Yes | MIP + BD + B & C | TSSO | Call Centers |
Liu [18] | S + A | Par | Yes | IP + AN | TSSO, CF | – |
Mattia et al. [41] | S + SS | No | – | IP + BD + B & C | 2RO | Call Centers |
Restrepo et al. [42] | TS | Par | No | MIP + M | TSSO | – |
Altner et al. [43] | S + SS | No | – | IP + CG | TSSO | CSOC |
Altner et al. [44] | DOS | Var | No | MIP + M | TSSO | CSOC |
Henao et al. [2] | A | Var | Yes | AN + H + LP | CF | Retail |
Fontalvo Echavez et al. [20] | DOS | Var | Yes | MIP | TSSO | Retail |
This paper | A | Var | Yes | MIP + H + AN | TSSO, RO, CF | Retail |
Sets | |
I | Workers, indexed by i |
L | Departments of the retail store, indexed by l |
S | Scenarios of demand, indexed by s |
Il | Set of single-skilled workers initially hired in department l, indexed by i |
Parameters | |
rl(s) | Random parameter of staff demand in weekly hours per store's department l, ∀l∈L,s∈S |
¯rl | Average value of staff demand in weekly hours per store's department l, ∀l∈L |
c | Cost of workers training in any department; [US$-week /Worker] |
u | Staff shortage cost per store's department; [US$/Hour] |
b | Staff surplus cost per store's department; [US$/Hour] |
h | Weekly hours that workers have to work in accordance with their employment contract |
mi | Department in which the worker i is initially trained, ∀i∈I |
Variables | |
xil | 1 if worker i is trained in department l, 0 otherwise, ∀i∈I,l∈L |
vi | 1 if worker i is multiskilled, 0 otherwise, ∀i∈I |
λl | Number of multiskilled workers belonging to department l, ∀l∈L |
Ω | Number of generated closed-chains |
ωil | Number of weekly working hours allocated to worker i in department l, ∀i∈I,l∈L |
κl | Number of staff shortage hours per week in department l,∀l∈L |
δl | Number of staff surplus hours per week in department l,∀l∈L |
Solution approaches for the MPAP with 2-chaining | |
DT | Deterministic optimization |
RO | Robust optimization |
CF | Closed-form equation |
TSSO | Two-stage stochastic optimization |
Approach | CPU time | Average CPU time | Constraints | Binary variables | Continuous variables | |||||
5% | 10% | 20% | 30% | 40% | 50% | |||||
TSSO-ZT | 41.9 h | 109.6 h | 23.3 h | 41.1 h | 13.6 h | 5.4 h | 39.2 h | 384,054 | 150 | 396,048 |
TSSO-PT | 11.3 h | 18.0 h | 37.7 h | 32.8 h | 15.3 h | 53.4 h | 28.1 h | 384,054 | 150 | 396,048 |
RO-1 | 0.39 s | 0.33 s | 0.3 s | 3.83 s | 4.56 s | 0.53 s | 1.66 s | 254 | 150 | 247 |
RO-0.6 | 0.23 s | 0.23 s | 0.7 s | 0.06 s | 0.3 s | 5.66 s | 1.20 s | 254 | 150 | 247 |
CF | 0.02 s | 0.02 s | 0.02 s | 0.02 s | 0.02 s | 0.02 s | 0.02 s | - | - | - |
DT | 0.01 s | 0.01 s | 240 | 150 | 240 |
Approach | ∑l∈Lλ∗l | Closed chains | Type of closed chain |
TSSO-ZT | 19 | D1-D3-D5-D6-D4-D2-D1 | CLC |
D5-D4-D1-D6-D2-D5 | CSC | ||
D2-D3-D6-D2 | CSC | ||
D1-D5-D6-D1 | CSC | ||
D1-D6-D1 | CSC | ||
RO-1 | 15 | D3-D1-D4-D5-D6-D2-D3 | CLC |
D1-D2-D6-D3-D5-D1 | CSC | ||
D4-D6-D4 | CSC | ||
D1-D6-D1 | CSC | ||
CF | 12 | D3-D1-D4-D5-D6-D2-D3 | CLC |
D1-D5-D2-D6-D1 | CSC | ||
D1-D6-D1 | CSC | ||
TSSO-PT | 12 | D1-D3-D6-D5-D4-D1 | CSC |
D1-D2-D5-D6-D1 | CSC | ||
D1-D6-D2-D1 | CSC | ||
RO-0.6 | 11 | D1-D2-D4-D3-D6-D5-D1 | CLC |
D2-D1-D6-D2 | CSC | ||
D1-D6-D1 | CSC |
In-sample metrics | Out-of-sample metrics | |||||||
Approaches | %ME | %TM | %¯SSS | ¯φ (US$) | Homogeneous groups | |||
A | B | C | D | |||||
CV = 5% | ||||||||
TSSO-ZT | 20 | 4 | 100 | 882 | X | |||
RO-1 | 20 | 4 | 100 | 882 | X | |||
CF | 20 | 4 | 100 | 882 | X | |||
TSSO-PT | 20 | 4 | 100 | 882 | X | |||
RO-0.6 | 20 | 4 | 100 | 882 | X | |||
TM | 100 | 100 | 100 | 1,026 | X | |||
ZM (DT) | 0 | 0 | 0 | 2,013 | X | |||
CV = 10% | ||||||||
TSSO-ZT | 33 | 7 | 100 | 1,798 | X | |||
TSSO-PT | 27 | 5 | 100 | 1,806 | X | |||
RO-1 | 27 | 5 | 99 | 1,813 | X | X | ||
CF | 27 | 5 | 99 | 1,813 | X | X | ||
RO-0.6 | 23 | 5 | 97 | 1,859 | X | |||
TM | 100 | 100 | 100 | 1,937 | X | |||
ZM (DT) | 0 | 0 | 0 | 4,046 | X | |||
CV = 20% | ||||||||
TSSO-ZT | 63 | 13 | 100 | 3,561 | X | |||
RO-1 | 50 | 10 | 100 | 3,573 | X | |||
TSSO-PT | 40 | 8 | 98 | 3,649 | X | X | ||
CF | 40 | 8 | 98 | 3,660 | X | X | ||
TM | 100 | 100 | 100 | 3,692 | X | |||
RO-0.6 | 37 | 7 | 94 | 3,811 | X | |||
ZM (DT) | 0 | 0 | 0 | 8,124 | X | |||
CV = 30% | ||||||||
TSSO-ZT | 87 | 17 | 100 | 5,324 | X | |||
RO-1 | 67 | 13 | 99 | 5,363 | X | |||
CF | 63 | 13 | 99 | 5,371 | X | |||
TSSO-PT | 60 | 12 | 99 | 5,413 | X | |||
TM | 100 | 100 | 100 | 5,445 | X | X | ||
RO-0.6 | 53 | 11 | 96 | 5,594 | X | |||
ZM (DT) | 0 | 0 | 0 | 12,154 | X | |||
CV = 40% | ||||||||
TSSO-ZT | 100 | 20 | 100 | 7,190 | X | |||
RO-1 | 90 | 18 | 99 | 7,237 | X | |||
CF | 87 | 17 | 99 | 7,238 | X | |||
TM | 100 | 100 | 100 | 7,297 | X | X | ||
TSSO-PT | 73 | 15 | 98 | 7,344 | X | X | ||
RO-0.6 | 67 | 13 | 97 | 7,464 | X | |||
ZM (DT) | 0 | 0 | 0 | 16,104 | X | |||
CV = 50% | ||||||||
TSSO-ZT | 100 | 20 | 99 | 9,309 | X | |||
RO-1 | 100 | 20 | 99 | 9,326 | X | |||
CF | 100 | 20 | 99 | 9,326 | X | |||
TSSO-PT | 93 | 19 | 99 | 9,354 | X | |||
TM | 100 | 100 | 100 | 9,365 | X | |||
RO-0.6 | 87 | 17 | 98 | 9,508 | X | |||
ZM (DT) | 0 | 0 | 0 | 20,170 | X |