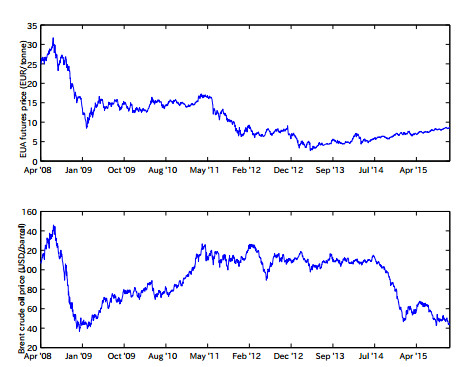
Citation: Mikael Lantz, Emma Kreuger, Lovisa Björnsson. An economic comparison of dedicated crops vs agricultural residues as feedstock for biogas of vehicle fuel quality[J]. AIMS Energy, 2017, 5(5): 838-863. doi: 10.3934/energy.2017.5.838
[1] | Ke Liu, Changqing Luo, Zhao Li . Investigating the risk spillover from crude oil market to BRICS stock markets based on Copula-POT-CoVaR models. Quantitative Finance and Economics, 2019, 3(4): 754-771. doi: 10.3934/QFE.2019.4.754 |
[2] | Emmanuel Assifuah-Nunoo, Peterson Owusu Junior, Anokye Mohammed Adam, Ahmed Bossman . Assessing the safe haven properties of oil in African stock markets amid the COVID-19 pandemic: a quantile regression analysis. Quantitative Finance and Economics, 2022, 6(2): 244-269. doi: 10.3934/QFE.2022011 |
[3] | Lucjan T. Orlowski, Monika Sywak . Wavering interactions between commodity futures prices and us dollar exchange rates. Quantitative Finance and Economics, 2019, 3(2): 221-243. doi: 10.3934/QFE.2019.2.221 |
[4] | Albert A. Agyemang-Badu, Fernando Gallardo Olmedo, José María Mella Márquez . Conditional macroeconomic and stock market volatility under regime switching: Empirical evidence from Africa. Quantitative Finance and Economics, 2024, 8(2): 255-285. doi: 10.3934/QFE.2024010 |
[5] | Xiaoling Yu, Kaitian Xiao, Javier Cifuentes-Faura . Closer is more important: The impact of Chinese and global macro-level determinants on Shanghai crude oil futures volatility. Quantitative Finance and Economics, 2024, 8(3): 573-609. doi: 10.3934/QFE.2024022 |
[6] | Raéf Bahrini, Assaf Filfilan . Impact of the novel coronavirus on stock market returns: evidence from GCC countries. Quantitative Finance and Economics, 2020, 4(4): 640-652. doi: 10.3934/QFE.2020029 |
[7] | Lorna Katusiime . Time-Frequency connectedness between developing countries in the COVID-19 pandemic: The case of East Africa. Quantitative Finance and Economics, 2022, 6(4): 722-748. doi: 10.3934/QFE.2022032 |
[8] | Xiaohang Ren, Weixi Xu, Kun Duan . Fourier transform based LSTM stock prediction model under oil shocks. Quantitative Finance and Economics, 2022, 6(2): 342-358. doi: 10.3934/QFE.2022015 |
[9] | Saeed Sazzad Jeris, Ridoy Deb Nath . Covid-19, oil price and UK economic policy uncertainty: evidence from the ARDL approach. Quantitative Finance and Economics, 2020, 4(3): 503-514. doi: 10.3934/QFE.2020023 |
[10] | Donatien Hainaut . Continuous Mixed-Laplace Jump Diffusion Models for Stocks and Commodities. Quantitative Finance and Economics, 2017, 1(2): 145-173. doi: 10.3934/QFE.2017.2.145 |
Commodity transaction including energy often starts with long-term contracts from supply-side perspective of commodities. This is reasonable because the supply sides certainly attempt to recover the production costs in the early stage of commodity trading. It also fits well to the demand side preference to desire the commodity purchase with a stable constant price in the absence of the highly liquid spot markets even if the constant price is more or less expensive for consumers. For example, natural gas trading is known to employ long-term contracts between supply and demand sides, resulting in crude oil-linked pricing (see, e.g., Stern (2007)). When the liquidity of energy trading fully increases, as the next step of long-term contracts energy tends to be evaluated in the market using supply and demand-based pricing. For example, the US natural gas prices are determined by the supply and demand in the relatively highly liquid market (see, e.g., Kanamura (2009b)). On the other hand in contrast to energy commodities, emissions trading politically and artificially starts with the markets whose prices are determined by the supply and demand relations for emissions, as observed in the EU ETS. That is to say, carbon assets are transacted in a supply and demand-based market regardless of low liquidity of carbon trading, which is different from market development processes of energy commodities. This can be a reason why carbon markets do not work well, resulting in low prices less than 10 EUR/tonne (see the recent years of Figure 1), i.e., a recent low price issue in carbon markets. Low carbon prices are quite problematic for accelerating the developments of carbon reduction technologies because inexpensive carbon assets are favored by EU ETS covered or compliance entities more than the introduction of new carbon emission reduction technologies with high marginal abatement costs. We revisit carbon pricing to solve a recent low carbon price issue. Crude oil markets are closely related to economic fundamentals as in e.g., Chevallier (2011b). It implies that high crude oil prices can be driven by strong economy, resulting in large carbon emissions and high carbon prices. Thus in a first order approximation it is assumed that carbon prices can positively be affected by crude oil prices. In order to examine the relation between carbon and energy, we show scatter plots between Brent crude oil and EUA futures prices in Figure 2. It seems an upward-sloping nonlinear relation between Brent crude oil and EUA futures prices, resulting in the positive support of crude oil-linked pricing of carbon products.
In the last decade, the development of carbon markets has attracted great academic interest. Fehr and Hinz (2006) propose an equilibrium price model for EUA prices taking into account fuel switching between natural gas and coal fired power plants. Benz and Trück (2009) employ an AR-GARCH Markov switching price return model to capture regime changes between different phases of the EU ETS and heteroskedasticty. Daskalakis, Psychoyios, and Markellos (2009) compare existing popular diffusion and jump diffusion models, with the results in favor of the Geometric Brownian motion with jumps to fit historical EUA spot prices, unlike the mean-reverting processes often used for commodity price modeling. Seifert, Uhrig-Homburg, and Wagner (2008) propose a stochastic model of CO2 prices which does not have any seasonal pattern, as often observed in commodity markets. Paolella and Taschini (2008) also propose mixed normal and mixed stable GARCH models to capture the heavy tails and volatility clustering in the U.S. SO2 permits and EUA price returns, which are not modeled using any mean-reversion and seasonality. Uhrig-Homburg and Wagner (2009) examine the relation between carbon spot and futures prices traded on the Powernext and the European Climate Exchange. Trück, Härdle, and Weron (2015) conduct empirical analyses of EUA convenience yields using the spot and futures prices traded on the EEX and presenting a convenience yield model based on the spot prices and volatilities. Kanamura (2009a) also investigates the characteristics of carbon asset prices, resulting in the possibility of classifying carbon assets into non commodity assets. Gorenflo (2013) analyzes the pricing and lead–lag relation between EUA spot and futures prices.
Other papers for carbon markets focus on price determination in carbon markets. The relations between EUA futures prices and macroeconomic factors, including stock and bond market variables, are found in Chevallier (2009). Fezzi and Bunn (2009) show that carbon prices accompanied by natural gas prices drive electricity prices in the UK. Hintermann (2010) investigates whether marginal abatement costs explain EUA prices in the first phase of the EU ETS or not. Bredin and Muckley (2011) examine the impacts of economic growth, energy prices, and weather conditions on EUA futures prices. Chevallier (2011a) suggests that yearly compliance events and growing uncertainties in post-Kyoto international agreements may explain the instability in carbon price volatilities. Chevallier (2011b) also develops a carbon pricing model considering two fundamental EUA price drivers of economic activity and energy prices. Gronwald, Ketterer, and Trück (2011) find a strong dependence between EUA futures price returns and those of other financial assets and commodities during the period of the financial crisis. Aatola, Ollikainen, and Toppinen (2013) discover a strong relation between EUA prices and energy prices, including German electricity prices and gas and coal prices. Koch, Fuss, Grosjean, and Edenhofer (2014) conduct an ex-post empirical analysis of the reasons for the EUA price slump. Kanamura (2016) theoretically and empirically examines the role of carbon swap trading and energy prices in volatilities and price correlations between the EU and Kyoto Protocol emissions trading schemes. Gil-Alana, Gupta, and de Gracia (2016) analyze the persistence property of CO2 allowance price accounting for structural breaks and non-linearities with CO2 data from the three Phases of the EU ETS. Ji, Zhang, and Geng (2018) investigate information linkages and dynamic spillover effects between the carbon and energy markets by using a systemic time-series approach. Dutta, Bouri, and Noor (2018) study return and volatility linkages between EUA and clean energy stock indices using the bivariate VAR-GARCH approach. Dutta (2018) assesses whether outliers or extreme observations occur in EUA data, examines if time-varying jumps are present in the carbon emission market using the GARCH-jump models, and investigates the effect of oil market uncertainty on the emission prices using the crude oil volatility index.
While these studies keep eyes on carbon price models and the empirical analyses of carbon markets, they do not seem to pay attention to direct modeling of carbon prices using the linkage of carbon prices to crude oil prices. This paper theoretically and empirically revisits carbon pricing from the supply-side perspective for carbon assets to solve the recent low price issue which may delay the development of emission reduction technologies in the sense of marginal abatement costs. We propose a carbon pricing model linked to crude oil prices, which is employed in supply-side driven pricing of long-term contracts for early-stage energy trading. Since the model is designed to hold carbon prices between certain lower and upper boundaries using S-shaped carbon price linkage to crude oil prices, it can be useful to overcome a recent low carbon price issue. In addition, it is shown that the model can alleviate the difficulties of carbon derivative pricing in selecting market price of risk. Empirical studies using EUA and Brent crude oil futures prices estimate the parameters of the Brent crude oil-linked EUA price model. The comparison of EUA prices simulated from the model with historical EUA prices suggests that simulated EUA prices be kept relatively higher than historical EUA prices. This is preferable for accelerating carbon emission reductions in that it can make emission reduction technologies with high marginal abatement costs affordable. It may imply that EUA must be priced using a crude oil-linked carbon price model in the early stage of EUA trading until EUA markets mature. This is a sharp contrast to current carbon markets employing premature market-based or supply and demand based pricing models. To show usefulness of crude oil-linked carbon pricing, we also give a numerical example of European carbon option pricing based on the Brent crude oil-linked EUA price model by using the Crank-Nicolson finite difference method. Finally we discuss the relation between crude oil-linked carbon pricing and emission reduction risk.
The remainder of this paper is organized as follows. Section 2 proposes a carbon pricing model which is linked to crude oil prices. Section 3 conducts empirical studies of EUA and Brent crude oil prices using the crude oil-linked carbon price model. Section 4 discusses the relation between crude oil-linked carbon pricing and emission reduction risk. Section 5 concludes.
It is observed that energy transaction including natural gas is conducted using long-term contracts in the early stage where prices are linked to crude oil prices from the supply-side perspective for energy. For example in practice, Japanese liquefied natural gas (LNG) is priced using the linkage to crude oil prices. Then after the liquidity of energy transaction increases, the pricing mechanism is transferred from the oil-linked pricing to market-based pricing where the supply and demand for energy determine the prices, e.g., the US natural gas market. On the other hand, while carbon assets are considered as energy-related assets, the pricing employs market-based pricing from the beginning where the supply and demand for emissions determine the prices, not based on energy-linked pricing. We consider that the development process of carbon markets is different from the other energy markets in the sense of pricing mechanism. When energy prices increase, the world economy is likely to grow rapidly as observed during commodity boom period from 2005 to 2007. It will increase the output of emissions in the world, resulting in high appreciation of carbon assets. Thus carbon prices may also have positive linkage to energy prices. Following this idea, we propose a carbon pricing model which is linked to crude oil prices. Crude oil price (Pt) model is given by
Pt=(1+aVtc)1a, | (1) |
dVt=μVdt+σVdwt. | (2) |
The inverse Box-Cox transformation function in Equation (1) and the stochastic process in Equation (2) represent a crude oil supply curve and the demand fluctuation of Vt, respectively. Assuming demand inelasticity to prices, i.e., a vertical demand curve, the equilibrium prices are obtained from the intersections of the supply and demand curves.
By using Ito's lemma, we have crude oil price model:
dPtPt=μPdt+σPdwt, | (3) |
μP=μVσVσP+12(1−a)σ2P, | (4) |
σP=σVcP−at. | (5) |
Carbon price Ct is defined using S-shaped logit model which has price cap (A) and floor (B).
Ct=Aeα+βPt+B1+eα+βPt | (6) |
This is referred to as "a crude oil-linked carbon price model" we propose. Since the model holds carbon prices between certain lower and upper boundaries, it can accelerate to develop emission reduction technologies in the sense of marginal abatement costs by resolving a recent low carbon price issue. Note that
∂Ct∂Pt=(A−B)βeα+βPt(1+eα+βPt)2. | (7) |
Taking into account A−B>0 by definition, if β>0, then carbon price Ct is an increasing function of crude oil price Pt.*
* The EU ETS does neither cover the oil demand from transportation sector nor the demand from the residential and most commercial oil combustion for heating or small-scale electricity generation purposes. One may put to question the assumed validity of a link between oil and carbon prices. However, since positive correlations of EUA prices with oil prices, which are determined by the supply and demand, have been confirmed as in e.g., Kanamura (2016), this model based on oil demand will hold in the first order approximation.
It is in general difficult to price carbon derivatives due to the illiquidity of carbon derivative markets, resulting in incomplete market pricing of the carbon assets and the difficulties in selecting carbon market price of risk.† As the advantage of the crude oil-linked carbon price model, the market price of risk for carbon derivatives can be selected as crude oil market price of risk, which can be more reliable than carbon market price of risk in the sense of market liquidity. Thus in a first order approximation we can avoid the issue of incomplete market pricing of carbon assets, i.e., the issue of the selection of carbon market price of risk. The European call option price (ft) on carbon prices from crude oil prices is calculated using the following equation.
ft=Et[ΛTΛt(CT−K)+] | (8) |
† We recognize the praise to its early liquidity in the EU ETS, e.g., Ellerman and Joskow (2008) and the World Bank's report of the much bigger transaction volume in EUA futures contracts than EUA spot contracts. However, the carbon market does not have enough liquidity to employ complete market pricing based on the perfect replication of risk as assumed in stock market option pricing.
where CT is carbon price at time T using crude oil price (PT), Λt is a stochastic discount factor at time t, and K is the strike of the call option. Here we assume the stochastic discount factor is given by
dΛtΛt=−rdt−ϕPdwt. | (9) |
Note that ϕP=μP−rσP is crude oil market price of risk. By using Ito's Lemma, we have the partial differential equation (PDE) for the option prices:
∂ft∂t+rPt∂ft∂Pt+12σ2PP2t∂2ft∂P2t−rft=0, | (10) |
fT=(CT−K)+. | (11) |
By solving the PDE from time T to t numerically, we can obtain the call option price at time t. It was shown that the crude oil-linked carbon pricing model can alleviate the difficulties of carbon derivative pricing in the sense of the selection of the market price of risk.
We use daily Brent crude oil and EUA futures price data of spot-month continuous products obtained from the website of Quandl. It covers from April 8, 2008 to June 5, 2009 whose sample size is 300. The motivation for the selected sample period is that the carbon market is considered to have been successful during the period because the prices are maintained as relatively high prices greater than around 10 EUR/tonne. The basic statistics are reported in Table 1. The skewness of Brent crude oil prices is positive while the skewness of EUA prices is negative, resulting in right-skewed and left-skewed distributions, respectively.
Brent | EUA | |
Mean | 81.14 | 20.14 |
Maximum | 146.08 | 31.71 |
Minimum | 36.61 | 8.43 |
Std. Dev. | 35.52 | 6.44 |
Skewness | 0.37 | -0.05 |
Kurtosis | 1.48 | 1.50 |
Assuming A=31.71 and B=8.43 which are maximum and minimum of the sample carbon price data, respectively in Table 1, we consider the following nonlinear regression model for Equation (6), i.e., a modified logit regression model.
Ct=Aeα+βPt+B1+eα+βPt+ut | (12) |
where ut represents an error term. The estimation results of the non-linear regression model for Equation (12) are reported in Table 2 based on non-linear least squares. The parameters are statistically significant judging from the standard errors. Since β>0, carbon prices increase in line with crude oil prices. In addition we draw the model estimation results on the scatter plots between Brent crude oil and EUA futures prices in Figure 3, which suggests that the model fit well to the historical scatter plots.
Parameters | α | β |
Estimates | -2.733 | 3.492E-02 |
Standard errors | 0.077 | 9.787E-04 |
Log likelihood | 290.695 | |
AIC | -577.389 | |
SIC | -569.982 |
Then we estimate crude oil price model in Equation (3) to (5), which are discretized as
logPt+1−logPt=(kσP−12aσ2P)Δt+σPϵt, | (13) |
σP=ˉσPP−at. | (14) |
Note that k=μVσV, ˉσP=σVc, Δt=1252, and ϵt∼N(0,1252). The results using log likelihood estimation are reported in Table 3. Inverse Box-Cox parameter for the supply curve (a) and volatility parameter for crude oil price (ˉσP) are positive and statistically significant while the drift parameter (k) is not statistically significant judging from the standard errors. The positive a suggests leverage effect for crude oil prices because the volatilities in crude oil prices decrease in line with the prices. This characteristic of crude oil is the same as those of financial products, which may come from the fact that crude oil futures are traded like financial products.
Parameters | k | a | ˉσP |
Estimates | -0.682 | 0.606 | 7.450 |
Standard errors | 0.865 | 0.009 | 0.295 |
Log likelihood | 613 | ||
AIC | -1,220 | ||
SIC | -1,226 |
We simulate EUA prices from crude oil prices by using the Brent crude oil-linked EUA price model with the model parameter estimates obtained in the previous subsection. As out of sample data, we employ the data covering from June 8, 2009 to November 23, 2015. The simulation results accompanied by out of sample carbon price data are shown in Figure 4. It suggests that EUA prices simulated from the Brent crude oil-linked EUA price model are kept relatively higher than historical prices. The characteristics of simulated EUA prices are helpful to accelerate the developments of emission reduction technologies because high carbon prices allow emission reduction technologies with expensive marginal abatement costs. It may imply that EUA must be priced using a crude oil-linked carbon price model, not employing a premature market-based or supply and demand-based carbon price model.
We finally show ten sample paths simulated by using both Brent crude oil price model and the Brent crude oil-linked EUA price model in Equation (3) and (6), respectively. The results are reported in Figure 5. Both figures demonstrate that even if Brent crude oil prices are fluctuated dramatically as time goes by, EUA prices are kept between lower and upper boundaries. The simulation results using both the Brent crude oil price model and the Brent crude oil-linked EUA price model also suggest that a carbon price modeling linked to crude oil prices with some boundaries work well to promote the development of emission reduction technologies from the point of the relation between carbon prices and marginal abatement costs of new emission reduction technologies.
Recently, EUA prices have been double that level, recently reaching around 23 EUR/tonne. This rise in EUA prices is largely due to the supply-constraining effect of the market stability reserve (MSR), which is initially only anticipated and now also actual. However, these changing fundamentals and ETS design do not necessarily guarantee the underlying support of carbon prices to avoid carbon price declines in the future. In that sense, the model proposed in this paper will contribute to the robustness of carbon markets.
Regarding the link between crude oil and the other commodities prices, including carbon prices, that underlies the "crude oil-linked carbon price model, " the link between natural gas and oil prices has come under pressure because supply and demand fundamentals of both are increasingly divorced from each other: e.g., for supply side hydraulic fracturing in North America and elsewhere has partly disrupted the traditional correlation between oil and gas production while for demand side oil consumption may stagnate or drop in some jurisdictions due to fuel efficiency and electric vehicle mandates for transportation, yet natural gas demand may rise if coal to gas switching is encouraged in the power sector. Similarly, the stated link between oil demand growth and carbon emissions growth may seem tenuous. As the power and transportation sectors further decarbonize, the fundamental link between oil prices and carbon prices may weaken even further-in scenarios that see global policy developments aligned with committed decarbonization targets, oil prices should be very low by 2050 due to policy-induced demand constraints, i.e., the supply will be steady and the demand near zero, while carbon prices should be in the triple or even quadruple digits. Additionally, the weak link here is the assumed correlation between economic (and emissions) growth and high oil prices. This may suggest a very pessimistic outlook on the continued innovation in and penetration of low- and zero-carbon technologies that may sever that correlation. While economic growth may continue to go hand in hand with increased energy demand, this increased energy demand may not necessarily translate into emissions growth, as it has in the past. Committed energy efficiency improvements and energy intensity reductions may also change the historical link between economic growth and energy demand. However, the carbon price model proposed in this research is useful on a short-term basis as seen in other commodity markets such as natural gas markets when the carbon market is immature and the imbalance between supply and demand leads to low carbon prices. Therefore, this model is not semi-permanent and if it is possible to maintain an appropriate carbon price level as a result of the supply and demand being reflected in the price, the crude oil-linked carbon pricing will shift to pricing based on supply and demand as with other commodity markets.
In order to show usefulness of crude oil-linked carbon pricing, we give a numerical example of European carbon option pricing based on the Brent crude oil-linked EUA price model by using the Crank-Nicolson finite difference method. The PDE in Equation (10) is solved backwards in time from 0.25 year (3 months) to 0 by setting the terminal condition in Equation (11). Note that we assume r=0.05 per annum. The results are reported in Figure 6 where the strike is 20 EUR/tonne for EUA price, which is close to the sample average of Table 1. The results empirically suggest that the model can alleviate the difficulties of carbon derivative pricing in the sense of selecting the market price of risk by showing that the call option prices are numerically obtained based on the crude oil-linked carbon pricing.
When we look at the track records of the relations between crude oil and carbon prices as in Figure 3, carbon prices move in line with crude oil prices. This is reasonable in the sense of the relations between world economic development and carbon emission outputs. If energy consumption, in particular crude oil consumption, increases, not only will crude oil price rise due to the supply and demand, but carbon prices will also rise due to the increase in energy consumption. It implies that energy consumers will economically have burdens from the rise in carbon prices as well as those from the rise in energy prices. Thus energy consumers have strong incentives to reduce energy usage in order to alleviate both impacts of energy and carbon prices on their businesses as well as to accelerate new emission reduction technologies with lower marginal abatement costs than carbon prices. In contrast if energy consumption level is low, then emissions themselves become small. In this case we do not need any new emission reduction technologies. In summary, when emissions increase due to energy consumption, the introduction of a crude oil-linked carbon price model to carbon markets forces energy consumers to reduce the emissions. In contrast, when emissions decrease due to energy consumption, the introduction of the model makes energy consumers release their burdens from emission reductions. In this sense, the introduction of a crude oil-linked carbon price model to carbon markets may offer a natural hedge of emission reduction risk for energy consumers.
This paper theoretically and empirically revisited carbon pricing from the supply-side perspective for carbon assets to solve the recent low price issue which may delay the development of emission reduction technologies in the sense of marginal abatement costs. We proposed a carbon pricing model linked to crude oil prices, which is employed in supply-side driven pricing of long-term contracts for early-stage energy trading. Since the model is designed to hold carbon prices between certain lower and upper boundaries using S-shaped carbon price linkage to crude oil prices, it can be useful to overcome a recent low carbon price issue. In addition, it was shown that the model can alleviate the difficulties of carbon derivative pricing in selecting market price of risk. Empirical studies using EUA and Brent crude oil futures prices estimated the parameters of the Brent crude oil-linked EUA price model. The comparison of EUA prices simulated from the model with historical EUA prices suggests that simulated EUA prices be kept relatively higher than historical EUA prices. This is preferable for accelerating carbon emission reductions in that it can make emission reduction technologies with high marginal abatement costs affordable. It may imply that EUA must be priced using a crude oil-linked carbon price model in the early stage of EUA trading until EUA markets mature. This is a sharp contrast to current carbon markets employing premature market-based or supply and demand based pricing models. To show usefulness of crude oil-linked carbon pricing, we also offered a numerical example of European carbon option pricing based on the Brent crude oil-linked EUA price model by using the Crank-Nicolson finite difference method. Finally we discussed the relation between crude oil-linked carbon pricing and emission reduction risk and showed that the introduction of a crude oil-linked carbon price model to carbon markets may offer a natural hedge of emission reduction risk for energy consumers. These studies may suggest carbon policy makers take account of crude oil-linked carbon pricing to tackle low price and low liquidity issues of carbon assets.
Views expressed in this paper are those of the author. I am grateful to John Constable, Toshiki Honda, Yu Li, Matteo Manera, Ryozo Miura, Nobuhiro Nakamura, Kazuhiko Ōhashi, Stefan Trück, the participants in Commodity and Energy Markets Conference 2017, and three anonymous referees for their useful comments and suggestions. All remaining errors are mine.
The author declares no conflict of interest.
[1] | EU (2009) DIRECTIVE 2009/28/EC OF THE EUROPEAN PARLIAMENT AND OF THE COUNCIL of 23 April 2009 on the promotion of the use of energy from renewable sources and amending and subsequently repealing Directives 2001/77/EC and 2003/30/EC. Official Journal of the European Union. |
[2] | Eurostat (2017) Energy from renewable sources. Available from: http://ec.europa.eu/eurostat/statistics-explained/index.php/Energy_from_renewable_sources. |
[3] | EurObservÉR (2016) Biofuels Barometer 2016. EurObservÉR. |
[4] | Fritsche UR, Wiegmann K (2011) Indirect land use change and biofuels. Committee on Environment, Public Health and Food Safety, European Parliament, Brussels. |
[5] | EU (2015) DIRECTIVE (EU) 2015/1513 OF THE EUROPEAN PARLIAMENT AND OF THE COUNCIL of 9 September 2015 amending Directive 98/70/EC relating to the quality of petrol and diesel fuels and amending Directive 2009/28/EC on the promotion of the use of energy from renewable sources. Official Journal of the European Union. |
[6] | SEA (2016) Transportsektorns energianvändning 2015 [Use of energy in the transportation sector 2015]. The Swedish Energy Agency. ES 2016: 03. |
[7] | SEA (2016) Produktion och användning av biogas och rötrester 2015 [Production and utilization of biogas and digestate 2015]. The Swedish Energy Agency. |
[8] | EurObservÉR (2016) The state of renewable energies in Europe-Edition 2016, 16th EurObservÉR Report. EurObservÉR. |
[9] | FNR (2016) Bioenergy in Germany-Facts and Figures 2016. Fachagentur Nachwachsende Rohstoffe e.V. |
[10] |
Grahn M, Hansson J (2015) Prospects for domestic biofuels for transport in Sweden 2030 based on current production and future plans. Wires Energy Environ 4: 290–306. doi: 10.1002/wene.138
![]() |
[11] | STA (2016) Styrmedel och åtgärder för att minska transportsystemets utsläpp av växthusgaser–med fokus på transportinfrastrukturen [Policy instruments and measures to reduce emissions of GHG from the transport system-with focus on transport infrastructure]. The Swedish Transport Administration. |
[12] | STA (2016) Åtgärder för att minska transportsektorns utsläpp av växthusgaser–ett regeringsuppdrag [Measures to reduce GHG emissions from the transport sector-a government assignment]. The Swedish Transport Administration. |
[13] | SOU (2016) En klimat-och luftvårdsstrategi för Sverige [A climate and air pollution strategi] Swedish Government Official Report. |
[14] |
Gissén C, Prade T, Kreuger E, et al. (2014) Comparing energy crops for biogas production–yields, energy input and costs in cultivation using digestate and mineral fertilisation. Biomass Bioenerg 64: 199–210. doi: 10.1016/j.biombioe.2014.03.061
![]() |
[15] |
Börjesson P, Prade T, Lantz M, et al. (2015) Energy crop-based biogas as vehicle fuel: the impact of crop selection on energy efficiency and greenhouse gas performance. Energies 8: 6033–6058. doi: 10.3390/en8066033
![]() |
[16] | FNR (2010) Biogas Messprogramm II-61 Biogasanlagen im Vergleich. Gulzow: Bundesministerium fur Ernährung, Landwirtshafts und Verbraucherschutz. |
[17] | Hjort-Gregersen K (2015) Udvikling og effektivisering af biogasproduktionen i Danmark - Ökonomi, nögletal og benchmark, Energistyrelsens Biogas Taskforce. AgroTech. |
[18] | Möller HB, Nielsen KJ (2015) Udvikling og effektivisering af biogasproduktionen i Danmark, Faglig rapport, Biogas Taskforce. Inst. for Ingeniörvidenskab, Aarhus Universitet. |
[19] | SJV (2016) Rötning av animaliska biprodukter, 2016-10-24 Jordbruksverket. |
[20] | Lantz M, Ekman A, Börjesson P (2009) Systemoptimerad produktion av fordonsgas, Report 69. Lund, Sweden: Department of Technology and Society, Lund University. 69. 110 p. |
[21] | Petersson A, Wellinger A (2009) Biogas upgrading technologies–development and innovations. IEA Bioenergy Task 37, European Commission Joint Research Centre, Petten, The Netherlands. |
[22] | IEA (2016) Biogas upgrading plant list, data up to the end of 2015. IEA Bioenergy Task 37, European Commission Joint Research Centre, Petten, The Netherlands. |
[23] | Bauer F, Hultenberg C, Persson T, et al. (2013) Biogas upgrading–Review of commercial technologies. Malmö: Svenskt Gastekniskt Center. Rapport 2013: 270. |
[24] | Hoyer K, Hultenberg C, Svensson M, et al. (2016) Biogas Upgrading–Technical Review. Energiforsk. |
[25] | Urban W, Girod K, Lohmann H (2008) Technologien und Kosten der Biogasaufbereitung und Einspeisung in das Erdgasnetz. Ergebnisse der Markterhebung 2007–2008. Fraunhofer-Institut für Umwelt- Sicherheits- und Energietechnik, Oberhausen, Germany. |
[26] | Berglund P, Bohman M, Svensson M, et al. (2012) Teknisk och ekonomisk utvärdering av lantbruksbaserad fordonsgasproduktion. Swedish Gas Technology Centre. |
[27] | Colnerud GS, Gåverud H, Glimhall A (2010) Förändrade marknadsvillkor för biogasproduktion. The Energy Market Inspectorate. |
[28] |
Walla C, Schneeberger W (2008) The optimal size for biogas plants. Biomass Bioenerg 32: 551–557. doi: 10.1016/j.biombioe.2007.11.009
![]() |
[29] | Börjesson P, Lantz M, Andersson J, et al. (2016) METHANE AS VEHICLE FUEL–A WELL-TO-WHEEL ANALYSIS (METDRIV), report 2016:06 f3 The Swedish Knowledge Centre for Renewable Transportation Fuels, Sweden. |
[30] | Berglund M, Börjesson P (2006) Assesment of energy performance in the life-cycle of biogas production. Biomass Bioenerg 30: 254–266. |
[31] |
Brown J, Nizami AS, Thamsiriroj T, et al. (2011) Assessing the cost of biofuel production with increasing penetration of the transport fuel market: A case study of gaseous biomethane in Ireland. Renew Sust Energ Rev 15: 4537–4547. doi: 10.1016/j.rser.2011.07.098
![]() |
[32] | Jury C, Benetto E, Koster D, et al. (2010) Life cycle assessment of biogas production by monofermentation of energy crops and injection into the natural gas grid. Biomass Bioenerg 34: 54–66. |
[33] | FNR (2006) Handreichung Biogasgewinnung und nutzung. Fachagentur Nachwachsende Rohstoffe e.V., Bundesministerium für Ernährung, Landwirtschaft and Verbraucherschutz, Gülzow-Prüzen, German. |
[34] | Murphy J, Braun R, Weiland P, et al. (2011) Biogas from Crop digestion. Available from: https://www.nachhaltigwirtschaften.at/resources/iea_pdf/reports/iea_bioenergy_task37_biogas_from_crop_digestion.pdf. |
[35] | FNR (2005) Ergebnisse des Biogas-Messprogram. Fachagentur Nachwachsende Rohstoffe e.V., Bundesministerium für Ernährung, Landwirtschaft and Verbraucherschutz, Gülzow-Prüzen, Germany. |
[36] | Lundqvist P (2009) Fjärrvärme för utökad biogasproduktion–Teknisk och ekonomisk utvärdering av fjärrvärme för uppvärmning av biogasprocesser. Thermal Engineering Research Institute, Stockholm, Sweden. |
[37] | Norin E (2007) Alternativa hygieniseringsmetoder. Swedish Gas Technology Centre, Malmö, Sweden. |
[38] | Rosenqvist H (2017) Kalkyler för energigrödor 2017-fastbränsle, biogas, spannmål och raps. Jordbruksverket. |
[39] | Björnsson L, Prade T, Lantz M (2016) Grass for biogas-Arable land as carbon sink, Report 2016:280. Energiforsk. |
[40] | Valin H, Peters D, Berg Mvd, et al. (2015) The land use change impact of biofuels consumed in the EU - Quantification of area and greenhouse gas impacts. ECOFYS. |
[41] | Kreuger E, Prade T, Björnsson L, et al. (2014) Biogas från Skånsk betblast - potential, teknik och ekonomi [Biogas from beet tops in Scania-potential, technology and economy]. Environmental and Energy System Studies, Lund University. |
[42] | SCB (2017) Price Indices and Prices in the Food Sector-Annual and Monthly Statistics-2017:06. Statistics Sweden. JO 49 SM 1708. |
[43] | Energimyndigheten (2017) Trädbränsle- och torvpriser Nr 3 2017. Swedish Statistics. |
[44] | Hansen MT (2015) Standardforutsaetninger-til VE til process-ansögningsmateriale. FORCE Technology. |
[45] | Odhner PB, Svensson SE, Prade T (2015) Extruder för ökad biogasproduktion [Extrusion increases the production of biogas], Report 2015: 26 Sveriges lantbruksuniversitet, Fakulteten för landskapsarkitektur, trädgårds- och växtproduktionsvetenskap. |
[46] | FNR (2010) Guide for biogas-From production to use Nachwachsende Rohstoffe e.V., Bundesministerium für Ernährung, Landwirtschaft and Verbraucherschutz, Gülzow-Prüzen, Germany. |
[47] | FNR (2010) Leitfaden Biogas–Von der Gewinnung zur Nutzung. Nachwachsende Rohstoffe e.V. Gülzow-Prüzen, Germany: Bundesministerium für Ernährung, Landwirtschaft and Verbraucherschutz. |
[48] | FNR (2016) Leitfaden Biogas–Von der Gewinnung zur Nutzung. Nachwachsende Rohstoffe e.V. Gülzow-Prüzen, Germany: Bundesministerium für Ernährung, Landwirtschaft and Verbraucherschutz. |
[49] | Lantz M, Björnsson L (2011) Biogas från gödsel och vall-analys av föreslagna styrmedel. The Federation of Swedish Farmers, Stockholm, Sweden: LRF. |
[50] | Berglund P (2010) Biogödselhandbok–Biogödsel från storskaliga biogasanläggningar. Swedish Waste Management. |
[51] | SEA (2017) Trädbränsle och torvpriser [Wood fuel and peat prices], Nr 1 2017. The Swedish Energy Agency. |
[52] | SCB (2016) Priser på el för industrikunder [Electricity price for industries]. Available from: http://www.scb.se/hitta-statistik/statistik-efter-amne/energi/prisutvecklingen-inom-energiomradet/energipriser-pa-naturgas-och-el/pong/tabell-och-diagram/genomsnittspriser-per-halvar-2007/priser-pa-el-for-industrikunder-2007/, accessed 2017-05-23. |
[53] |
Smyth B, H S, Murphy J (2010) Can grass biomethane be an economically viable biofuel for the farmer and the consumer? Biofuel Bioprod Bio 4: 519–537. doi: 10.1002/bbb.238
![]() |
[54] | Lantz M (2013) Biogas in Sweden-opportunities and challenges from a systems perspective. Lund, Sweden: Lund University. |
[55] | SJV (2016) Rekommendationer för gödsling och kalkning 2017, Report JO16:24. The Swedish Board of Agriculture. Jönköping, Sweden. |
[56] | Nutrients Fo (2011) Stallgödselkalkyl (Manure calculator) version 2011-03-31. accessed 2012-10-18. |
[57] | Lantz M, Svensson M, Björnsson L, et al. (2007) The prospects for an expansion of biogas systems in Sweden–Incentives, barriers and potentials. Energy Policy 35: 1830–1843. |
[58] | EC (2015) Statligt stöd-Skattebefrielser och skattenedsättningar för flytande drivmedel, C(2015) 9344. European Commission. |
[59] | EC (2015) Statligt stöd-Skattebefrielser för biogas som används som motorbränsle, C(2015) 9345. European Commission. |
[60] | Regeringen (2017) Reduktion av växthusgasutsläpp genom inblandning av biodrivmedel i bensin och dieselbränslen, Lagrådsremiss In: energidepartementet M-o, editor: The government of Sweden. |
[61] | SJV (2017) Gödselgasstöd. Available from: http://www.jordbruksverket.se/amnesomraden/ stod/andrastod/godselgasstod.4.ac526c214a28250ac23333e.html, accessed 20170613. |
[62] | SJV (2017) Investeringsstöd till biogas. Available from: http://www.jordbruksverket.se/amnesomraden/stod/stodilandsbygdsprogrammet/investeringar/biogas.4.6ae223614dda2c3dbc44f95.html, accessed. |
[63] | EC (2014) COMMISSION REGULATION (EU) No 651/2014 of 17 June 2014 declaring certain categories of aid compatible with the internal market in application of Articles 107 and 108 of the Treaty In: COMMISSION TE, editor. Official Journal of the European Union. |
[64] | SPBI (2017) Priser och skatter. Available from: http://spbi.se/statistik/priser/, accessed. |
[65] | Karlsson S, Rodhe L (2002) Översyn av Statistiska Centralbyråns beräkning av ammoniakavgången i jordbruket–emissionsfaktorer för ammoniak vid lagring och spridning av stallgödsel. |
[66] | Bengtsson B, Rasic Z (2005) Kadmium i odlingssystem med tillförsel av rötslam. Jord-skörd- och markvatten-analyser. Department of plant sciences, Swedish University of Agricultural Sciences, Alnarp, Sweden. |
[67] | Roth U, Wulf S (2010) Gasausbeute in landwirtschaftlichen Biogasanlagen, KTBL-Heft 88. Kuratorium Fur Technik und Bauwesen in der Landwirtschaft e.V. (KTBL), Darmstadt, Germany. |
[68] | ECN (2014) The database Phyllis2: Biomass and waste. Available from: https://www.ecn.nl/phyllis2, accessed 2017-04-03. |
[69] |
Tamaki Y, Mazza G (2010) Measurement of structural carbohydrates, lignins, and micro-components of straw and shives: Effects of extractives, particle size and crop species. Ind Crop Prod 31: 534–541. doi: 10.1016/j.indcrop.2010.02.004
![]() |
[70] | Björnsson L, Castillo MdP, Gunnarsson C, et al. (2014) Förbehandling av lignocellulosarika råvaror för biogasproduktion-Nyckelaspekter vid jämförande utvärdering. Lund, Sweden: Environmental and Energy Systems Studies. |
[71] |
Moller HB, Sommer SG, Ahring B (2004) Methane productivity of manure, straw and solid fractions of manure. Biomass Bioenerg 26: 485–495. doi: 10.1016/j.biombioe.2003.08.008
![]() |
[72] | Amon T, Amon B, Kryvoruchko V, et al. (2007) Biogas production from maize and dairy cattle manure-Influence of biomass composition on the methane yield. Agr Ecosyst Enviro 118: 173–182. |
[73] | Mähnert P, Linke B (2009) Kinetic study of biogas production from energy crops and animal waste slurry: Effect of organic loading rate and reactor size. Environ Technol 30: 93–99. |
[74] | McCarty PL (1964) Anaerobic waste treatment fundamentals, Part one, Chemistry and microbiology. Public Works 95: 107–112. |
[75] | FNR (2010) Biogas-Messprogramm II, 61 Biogasanlagen im Vergleich. Fachagentur Nachwachsende Rohstoffe e.V., Bundesministerium für Ernährung, Landwirtschaft and Verbraucherschutz, Gülzow-Prüzen, Germany. |
[76] | Ljung E, Palm O, Rodhe L (2013) Ökad acceptans för biogödsel inom lantbruket. Uppsala, Sweden: JTI-Institutet för jordbruks-och miljöteknik. |
[77] |
Procházka J, Dolejš P, Máca J, et al. (2012) Stability and inhibition of anaerobic processes caused by insufficiency or excess of ammonia nitrogen. Appl Microbiol Biot 93: 439–447. doi: 10.1007/s00253-011-3625-4
![]() |
[78] | Schnürer A, Nordberg Å (2008) Ammonia, a selective agent for methane production by syntrophic acetate oxidation at mesophilic temperature. Water Sci Technol 57: 735–740. |
[79] | Scherer P, Lippert H, Wolff G (1983) Composition of the major elements and trace elements of 10 methanogenic bacteria determined by inductively coupled plasma emission spectrometry Biol Trace Elem Res 5: 149–163. |
[80] |
Banks CJ, Zhang Y, Jiang Y, et al. (2012) Trace element required for stable food waste digestion at elevated ammonia concentrations. Bioresource Technol 104: 127–135. doi: 10.1016/j.biortech.2011.10.068
![]() |
[81] |
Overend R (1982) Haul distance and transportation work factors for biomass. Biomass 2: 75–79. doi: 10.1016/0144-4565(82)90008-7
![]() |
[82] |
Börjesson P, Gustavsson L (1996) Regional production and utilization of biomass in Sweden. Energy 21: 747–764. doi: 10.1016/0360-5442(96)00029-1
![]() |
[83] | Björnsson L, Lantz M, Murto M, et al. (2011) Biogaspotential i Skåne-inventering och planeringsunderlag på översiktsnivå [The biogas potential in Scania-inventory and data for planning]. The County Administrative Board of Skåne. |
1. | Yang Wang, Meng Sun, Rui Xie, Xiangjie Chen, Multiplicative Structural Decomposition Analysis of Spatial Differences in Energy Intensity among G20 Countries, 2020, 10, 2076-3417, 2832, 10.3390/app10082832 | |
2. | Lili Zhao, Fenghua Wen, Xiong Wang, Interaction among China carbon emission trading markets: Nonlinear Granger causality and time-varying effect, 2020, 91, 01409883, 104901, 10.1016/j.eneco.2020.104901 | |
3. | Yanhong Feng, Shuanglian Chen, Pierre Failler, Productivity Effect Evaluation on Market-Type Environmental Regulation: A Case Study of SO2 Emission Trading Pilot in China, 2020, 17, 1660-4601, 8027, 10.3390/ijerph17218027 | |
4. | Takashi Kanamura, 2021, 9780128147122, 275, 10.1016/B978-0-12-814712-2.00007-5 | |
5. | Zhen Liu, Yuk Ming Tang, Ka Yin Chau, Fengsheng Chien, Wasim Iqbal, Muhammad Sadiq, Incorporating strategic petroleum reserve and welfare losses: A way forward for the policy development of crude oil resources in South Asia, 2021, 74, 03014207, 102309, 10.1016/j.resourpol.2021.102309 | |
6. | Lili Zhao, Wenhua Liu, Min Zhou, Fenghua Wen, Extreme event shocks and dynamic volatility interactions: The stock, commodity, and carbon markets in China, 2022, 47, 15446123, 102645, 10.1016/j.frl.2021.102645 | |
7. | Rui Feng, Peina Lin, Chenxue Hou, Shuaishuai Jia, Study of the Effect of China’s Emissions Trading Scheme on Promoting Regional Industrial Carbon Emission Reduction, 2022, 10, 2296-665X, 10.3389/fenvs.2022.947925 | |
8. | Jian Liu, Ziting Zhang, Lizhao Yan, Fenghua Wen, Forecasting the volatility of EUA futures with economic policy uncertainty using the GARCH-MIDAS model, 2021, 7, 2199-4730, 10.1186/s40854-021-00292-8 | |
9. | Tao Ge, Li Ma, Cang Wang, Spatial Effect of Economic Growth Targets on CO2 Emissions: Evidence From Prefectural-Level Cities in China, 2022, 10, 2296-665X, 10.3389/fenvs.2022.857225 | |
10. | Bin Mo, Zhenghui Li, Juan Meng, The dynamics of carbon on green energy equity investment: quantile-on-quantile and quantile coherency approaches, 2022, 29, 0944-1344, 5912, 10.1007/s11356-021-15647-y | |
11. | Shan Huang, Yan Ding, Pierre Failler, Does the Government’s Environmental Attention Affect Ambient Pollution? Empirical Research on Chinese Cities, 2022, 14, 2071-1050, 3242, 10.3390/su14063242 |
Brent | EUA | |
Mean | 81.14 | 20.14 |
Maximum | 146.08 | 31.71 |
Minimum | 36.61 | 8.43 |
Std. Dev. | 35.52 | 6.44 |
Skewness | 0.37 | -0.05 |
Kurtosis | 1.48 | 1.50 |
Parameters | α | β |
Estimates | -2.733 | 3.492E-02 |
Standard errors | 0.077 | 9.787E-04 |
Log likelihood | 290.695 | |
AIC | -577.389 | |
SIC | -569.982 |
Parameters | k | a | ˉσP |
Estimates | -0.682 | 0.606 | 7.450 |
Standard errors | 0.865 | 0.009 | 0.295 |
Log likelihood | 613 | ||
AIC | -1,220 | ||
SIC | -1,226 |
Brent | EUA | |
Mean | 81.14 | 20.14 |
Maximum | 146.08 | 31.71 |
Minimum | 36.61 | 8.43 |
Std. Dev. | 35.52 | 6.44 |
Skewness | 0.37 | -0.05 |
Kurtosis | 1.48 | 1.50 |
Parameters | α | β |
Estimates | -2.733 | 3.492E-02 |
Standard errors | 0.077 | 9.787E-04 |
Log likelihood | 290.695 | |
AIC | -577.389 | |
SIC | -569.982 |
Parameters | k | a | ˉσP |
Estimates | -0.682 | 0.606 | 7.450 |
Standard errors | 0.865 | 0.009 | 0.295 |
Log likelihood | 613 | ||
AIC | -1,220 | ||
SIC | -1,226 |