Special Issue: Artificial Neural Networks to Aid Prediction and Diagnosis of Neurological Diseases
Guest Editors
Dr. Diego Oliva
Depto. de Innovación Basada en la Información y el Conocimiento, Universidad de Guadalajara, CUCEI, Guadalajara, Mexico
Email: diego.oliva@cucei.udg.mx ; doliva@ucm.es
Dr. Renjith V Ravi
Department of Electronics and Communication Engineering, M.E.A. Engineering College, Kerala, India
Email: renjithravi@meaec.edu.in
Dr. Seyyed Jalaleddin Mousavirad
Hakim Sabzevari University, Iran
Email: Jalalmoosavirad@gmail.com
Dr. Gerald Schaefer
Department of Computer Science, Loughborough University, Loughborough, UK
Email: gerald.schaefer@ieee.org
Dr. Marco Perez-Cisneros
Depto. de Ciencias Computacionales, Universidad de Guadalajara, CUCEI, Guadalajara, Mexico
Email: marco.perez@cucei.udg.mx
Manuscript Topics
Our understanding of intelligence is based on the skills of evolved biological creatures, especially humans. Consequently, artificial intelligence (AI) research has primarily focussed on developing algorithms that can perceive, learn, and reason, intending to create artificial general intelligence (AGI) systems that can emulate human intelligence, also known as Turing-powerful systems. Given this goal, it is unsurprising that scientists, mathematicians, and philosophers working in artificial intelligence have drawn inspiration from the brain's mechanical, structural, and functional features.
Computer-aided diagnosis (CAD) has been shown to be able to improve the prediction, diagnosis, and treatment of neurological illness. Various CAD methods have been devised to help identify subjects suffering from epilepsy, Alzheimer's disease, Parkinson's disease, multiple sclerosis, autism spectrum disorders, alcoholism-related illnesses, sleep problems, etc. Recent developments in artificial neural networks (ANNs) based on deep learning approaches allow powerful analysis of diverse medical imaging data, such as MRIs of Alzheimer's patients or CT scans of suspected brain tumours.
Approaches such as deep learning can aid to identify characteristics specific to diverse neurological illnesses and can outperform humans' ability to categorise such data. Such intelligent systems may thus enhance neurological illness treatment by lowering work load of clinical experts and by improving diagnostic accuracy including helping to avoid human errors. The use of emerging technologies in this sector is important for improved diagnosis, which substantially influences preventative measures and therapy.
In this special issue, we seek original research and review articles on artificial neural networks, and in particular deep learning approaches, to aid the prediction, diagnosis, and treatment of neurological diseases. Topics include but are not limited to:
• Deep learning aided diagnosis of neural disorders
• Deep learning approaches for Parkinson's disease diagnosis
• Deep learning-based Alzheimer disease detection
• Deep learning applications to classification of psychiatric disorders
• Diagnosis of autism spectrum disorders using deep learning
• Deep learning for brain disorder diagnosis
• Brain tumour detection using deep learning
• Deep learning approachs for brain cancer classification
• Deep learning models for automated sleep stage classification
• Deep learning for automatic seizure detection
• Brain age prediction using deep learning
• Deep learning methods for analysing Covid-19 stress
Instructions for authors
https://www.aimspress.com/mbe/news/solo-detail/instructionsforauthors
Please submit your manuscript to online submission system
https://aimspress.jams.pub/
Paper Submission
All manuscripts will be peer-reviewed before their acceptance for publication. The deadline for manuscript submission is 30 June 2024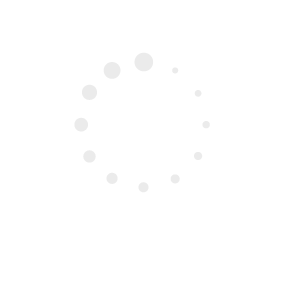