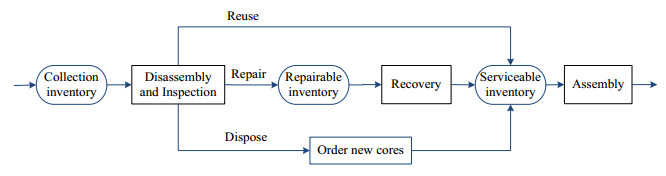
The connection between curvature and topology is a very well-studied theme in the subject of differential geometry. By suitably defining curvature on networks, the study of this theme has been extended into the domain of network analysis as well. In particular, this has led to curvature-based community detection algorithms. In this paper, we reveal the relation between community structure of a network and the curvature of its edges. In particular, we give apriori bounds on the curvature of intercommunity edges of a graph.
Citation: Sathya Rengaswami, Theodora Bourni, Vasileios Maroulas. On the relation between graph Ricci curvature and community structure[J]. Mathematics in Engineering, 2025, 7(2): 178-193. doi: 10.3934/mine.2025008
[1] | Guohui Zhang, Jinghe Sun, Xing Liu, Guodong Wang, Yangyang Yang . Solving flexible job shop scheduling problems with transportation time based on improved genetic algorithm. Mathematical Biosciences and Engineering, 2019, 16(3): 1334-1347. doi: 10.3934/mbe.2019065 |
[2] | Kongfu Hu, Lei Wang, Jingcao Cai, Long Cheng . An improved genetic algorithm with dynamic neighborhood search for job shop scheduling problem. Mathematical Biosciences and Engineering, 2023, 20(9): 17407-17427. doi: 10.3934/mbe.2023774 |
[3] | Jianguo Duan, Mengting Wang, Qinglei Zhang, Jiyun Qin . Distributed shop scheduling: A comprehensive review on classifications, models and algorithms. Mathematical Biosciences and Engineering, 2023, 20(8): 15265-15308. doi: 10.3934/mbe.2023683 |
[4] | Cong Zhao, Na Deng . An actor-critic framework based on deep reinforcement learning for addressing flexible job shop scheduling problems. Mathematical Biosciences and Engineering, 2024, 21(1): 1445-1471. doi: 10.3934/mbe.2024062 |
[5] | Zilong Zhuang, Zhiyao Lu, Zizhao Huang, Chengliang Liu, Wei Qin . A novel complex network based dynamic rule selection approach for open shop scheduling problem with release dates. Mathematical Biosciences and Engineering, 2019, 16(5): 4491-4505. doi: 10.3934/mbe.2019224 |
[6] | Yifan Gu, Hua Xu, Jinfeng Yang, Rui Li . An improved memetic algorithm to solve the energy-efficient distributed flexible job shop scheduling problem with transportation and start-stop constraints. Mathematical Biosciences and Engineering, 2023, 20(12): 21467-21498. doi: 10.3934/mbe.2023950 |
[7] | Shaofeng Yan, Guohui Zhang, Jinghe Sun, Wenqiang Zhang . An improved ant colony optimization for solving the flexible job shop scheduling problem with multiple time constraints. Mathematical Biosciences and Engineering, 2023, 20(4): 7519-7547. doi: 10.3934/mbe.2023325 |
[8] | Wenqiang Zhang, Chen Li, Mitsuo Gen, Weidong Yang, Zhongwei Zhang, Guohui Zhang . Multiobjective particle swarm optimization with direction search and differential evolution for distributed flow-shop scheduling problem. Mathematical Biosciences and Engineering, 2022, 19(9): 8833-8865. doi: 10.3934/mbe.2022410 |
[9] | Xuyin Wang, Weiguo Liu, Lu Li, Peizhen Zhao, Ruifeng Zhang . Resource dependent scheduling with truncated learning effects. Mathematical Biosciences and Engineering, 2022, 19(6): 5957-5967. doi: 10.3934/mbe.2022278 |
[10] | Yufan Zheng, Rafiq Ahmad . Feature extraction and process planning of integrated hybrid additive-subtractive system for remanufacturing. Mathematical Biosciences and Engineering, 2020, 17(6): 7274-7301. doi: 10.3934/mbe.2020373 |
The connection between curvature and topology is a very well-studied theme in the subject of differential geometry. By suitably defining curvature on networks, the study of this theme has been extended into the domain of network analysis as well. In particular, this has led to curvature-based community detection algorithms. In this paper, we reveal the relation between community structure of a network and the curvature of its edges. In particular, we give apriori bounds on the curvature of intercommunity edges of a graph.
Remanufacturing, as an industrial process of restoring discarded products/components back to their useful lives, is of growing importance due to the emerging pressure of legislation and increasing awareness of environmental conservation. As an ultimate form of recycling, remanufacturing maintains much of the value added from original material and reprocessing conservation, leading to lower production costs and improved profits [1]. A recent wall street journal revealed that large scale remanufacturing in the United States employs more than 500, 000 people and contributes to approximately $100 billion of goods sold each year [2]. More successful industry applications can be seen in automobile remanufacturing, aerospace remanufacturing and electronic remanufacturing [3, 4, 5].
Compared to manufacturing, remanufacturing is more complicated in the way that (1) the supply of returned products is unpredictable in timing and quantities; (2) the quality and composition of returned products varies; and (3) the process routings are not necessarily fixed but rather adapt to the actual conditions of products/components [6]. Such characteristics have led many new methodologies to deal with various operation management issues in remanufacturing as summarized in the survey literature [7]. One of the challenges, which is the focus of this paper, is remanufacturing job shop scheduling. With the high level of uncertainty and resource limitation, it becomes extremely difficult to carry out various remanufacturing tasks for different products and still achieve maximal system performance.
Guide and his colleagues are the first group of researchers that looked into the remanufacturing scheduling. Through simulation, they examined the performance of several static dispatching rules in a repair shop. They assumed the system has stochastic arrivals, exponentially distributed process times and probabilistic process routings [8]. Extended from this work, an analytical model was further developed to explore the impact of those rules on system performance in terms of weighted average sojourn time [9]. While the pursued simulation and model analysis provided insights to practicing managers in a repair shop, much of work is needed for complex job shop settings where various products/parts with uncertain remanufacturing routings compete for constrained resources.
A second line of work has focused on economic lot scheduling with random returns. For instance, Tang and Teunter considered a hybrid system where (re)manufacturing operations of multiple products are performed on the same production line [10]. The work formulated the problem as a mixed integer linear program to determine the optimal cycle time and production starting times for (re)manufacturing. The research was further extended to relax the constraint of common cycle time and a single (re)manufacturing lot per item per cycle [11]. By dividing the returns into different quality grades, Sun et al. investigated a lot scheduling problem where the remanufacturing rate increases as the quality grade increases and holding costs for serviceable products are higher than returns [12]. The objective is to minimize the average total cost by optimizing the acquisition lot size and scheduling the remanufacturing sequence. However, the deterministic nature of the assumptions (i.e., constant demand and returns, and constant remanufacturing rate) made in those works failed to address the uncertainty inherent in remanufacturing. Moreover, the authors considered remanufacturing as a single-stage process. Given that refurbishing used products up to like-new condition tends to involve a sequence of recovery operations, such single-stage scheduling methods present practical limitation.
A third line of researches have tackled the multi-stage and multi-product remanufacturing scheduling problem. For instance, Kim et al. considered a remanufacturing system with a single disassembly workstation, parallel flow-shop-type reprocessing lines, and a single reassembly workstation [13]. A hybrid scheduling heuristic is proposed to determine the sequences of products to be disassembled, to be reprocessed at each reprocessing lines and to be reassembled. The authors, however, treated the problem deterministic and static. Luh et al. presented another work that considered a similar remanufacturing system but tried to address the underlying uncertainty [14]. The main novelty of the research is that it considered stochastic asset arrival time and part repair time to model the dynamics of rotable inventory for optimization. Nevertheless, the authors presumed that each asset goes through a fixed series of overhaul operations. Giglio et al. studied a production system which produces multi-class single-level products through both manufacturing of raw materials and remanufacturing of return products [15]. A mixed-integer programming formulation is proposed to obtain the optimal lot size and job shop schedules with minimum total cost. This work presented the same limitation that the recovery process routings of returns are deterministic. By considering the alternatives of remanufacturing process routes for worn cores, Zhang et al. proposed a simulation based genetic algorithm approach for remanufacturing process planning and scheduling, where the processing time of each alternative routes are assume to be known a priori [16].
Summarizing the findings of the above discussion, no study has comprehensively dealt with remanufacturing job shop scheduling in subjecting to alternative recovery operations, random operation times and limited resource conflicts. In a practical remanufacturing system, the quality of returned products/cores varies, ranging from slightly used with minor blemishes to significantly damaged and requiring extensive repair. Such differences in the condition of products/cores strongly affect the set of recovery operations necessary to bring them up to a quality standard. In addition, the time that each recovery operation takes varies as well with respect to different damages of the cores. Those stochastic natures in remanufacturing triggers the potential conflicts of limited machine resources to a noticeable extend. Such characteristics have led a number of studies to investigate the operation management issues in remanufacturing environment by using different modeling methods, i.e., through analytic model [8, 9, 11, 12, 17], through Mixed Integer Programming model [10, 13, 15, 16], through Lagrangian Relaxation [14] and through Petri nets model [18, 19]. While our previous work [17] and [20] investigated the remanufacturing processes of worn cores with uncertain failure conditions and proposed an analytical model to study such dynamics in recovery process routings through probabilistic measures, how to incorporate those dynamic measures into remanufacturing scheduling has yet to be undertaken.
The colored timed Petri nets (CTPN) is an extension of ordinary Petri nets where the time and color are introduced to describe the logic structure and dynamic behavior of complex systems [21]. By taking advantage of both the well-formed formalism of CTPN and the robustness of Simulated Annealing (SA) for global optimization, the paper tackles this challenge and makes the following contributions. First, a colored timed Petri net is designed to explicitly model the dynamic of remanufacturing processes, such as various process routings, different uncertain operation times for cores, and real-time machine resource conflicts. With the color attributes in Petri nets, two types of decision points, recovery routing selection and resource dispatching, are introduced and embedded in places of CTPN model. With time attributes in Petri nets, the temporal aspect of recovery operations for cores as well as the evolution dynamics in cores' operational stages is mathematically analyzed. Second, a hybrid meta-heuristic algorithm embedded scheduling strategy over CTPN is proposed to search for the optimal recovery routings for worn cores and their recovery operation sequences on workstations, in minimizing the total production cost.
The rest of the paper is organized as follows. Section 2 presents the CTPN model. Section 3 describes the hybrid meta-heuristics based on SA and minimum slack time (MST) dispatching rule. A case study is conducted in Section 4, followed by the conclusion and future research in Section 5.
This paper considers a typical remanufacturing job shop where its clients drop their used products with an expectation to get them fully recovered within a fixed time frame. The remanufacturing processes are traditionally complicated including disassembly that disassembles a worn product into components/cores, cores' recovery operations and assembly that assembles recovered cores back into the product. Figure 1 gives an organizational structure of the remanufacturing job shop.
In this case, each used product (e.g., the lth used product) arrives at the job shop with an arrival time AT(l) and a due date Due(l). The used product is first disassembled into what so called "cores" that are disassembled subassemblies or components. The cores are then classified into "reusable", "repairable", and "disposal" through the inspection process as depicted in Figure 1. This paper focuses on the recovery activities only. The challenge arises when dealing with the repairable cores with different levels of damage that need to go through a set of recovery operations to restore their size and property.
In a remanufacturing job shop, the quality of repairable cores commonly ranges from slightly used with minor blemishes to significantly damaged and requiring extensive recovery. Such quality variations strongly affect the set of recovery operations necessary to bring them up to a quality standard. As exemplified in Figure 2, there are several process routings for the remanufacturing of used machine tool cores of different types and various damage conditions. For instance, the lathe spindles can be inspected and classified into either "abrasion", "corrosion", or "micro-cracks", on which a set of specific recovery operations are designated for their remanufacturing.
With a wide variety of recovery technologies being applied into remanufacturing industry, some recovery process routings for cores with a certain type of damage can be alternative. For instance, if a spindle is detected with severe abrasion, two typical process routings would be "grinding → chromium electroplating" or "grinding → cold welding → fine grind" to regain the surface accuracy. Each alternative process routing consists of a set of predesigned recovery operations, with each operation being performed by a certain kind of machine equipment in a workstation, resulting in a specified recovery quality and unit processing cost [22, 23]. As an upper level of process planning, different combinations of recovery process routings for cores would lead to much variation in remanufacturing cost and real-time machine workload in remanufacturing job shop.
With the recovery routings determined in the upper level, the core then travels to and is processed by a set of recovery workstations sequentially according to the designed process flow. However, the recovery operation time for a particular core in a given workstation is not fixed but rather dependent on the actual condition of the core. While different routings might demand operation times on the same workstation, various cores would compete for constrained machine resource to be recovered.
Our objective is to consider such variation for efficient resource utilization in fulfilling the demand with the minimum cost (i.e., operation cost and tardiness penalty). The remanufacturing job shop scheduling problem is strongly NP-hard due to: a) assignment decisions of reparable cores to recovery process routings and b) sequencing decisions of recovery operations of cores in each workstation. For that purpose, the paper makes the following assumptions:
(1) Each workstation has only one machine and each machine can process at most one task at a time.
(2) Each task is non-preemptive, requiring one and only one machine at a time.
(3) The transportation time between buffers is negligible.
(4) Penalty is charged if the system does not meet the due date of a product.
The ordinary of Petri nets (PN) does not include time and color, which limits its capability to describe the logic structure and behavior of modeled systems, but not its evolution over time and color [24, 25]. In view of the specific characteristics of remanufacturing system to be modeled, this section introduces time and color attributes to a Petri net and proposes a CTPN. The model considers not only recovery process routings and stochastic operation times, but also integrates recovery routing assignments and efficient resource dispatching.
Definition 1: A colored timed Petri net (CTPN) is defined as 7-tuple: CTPN = (P, T, I, O, M, U, C).
(1) P = S ∪ W, where the set of places in S represents buffers, the set of places in W stands for workstations.
(2) T = Ta ∪ Te, where the finite set of transitions in Ta represent recovery operations, and the ones in Te stand for transportation.
(3) I: P×T→{0, 1} is the input function that defines the set of ordered pairs (pi, tk), where I(pi, tk) = 1, if pi is an input place for tk (i.e., ∀pi ∈·tk), otherwise 0.
(4) O: P×T→{0, 1} is the output function that defines the set of ordered pairs (pi, tk), where O(pi, tk) = 1, if pi is an output place for tk (i.e., ∀pi ∈·tk·), otherwise 0.
(5) M: P→{0, 1, 2, …} is a marking vector, where M(pi) represents the number of tokens in pi.
(6) U: Ta→Θ is a non-zero time function that assigns to each recovery operation t∈Ta, while U: Tb→Θ is a zero time function such that the transportation time between buffers is negligible.
(7) C: P→Σ is a color function that assigns to each place p∈ S ∪W with a color set C(p).
Figure 3 gives an example of the proposed CTPN that corresponds to recovery process routings for used machine tools in Figure 2. The description for the places and transitions in CTPN are given Table 1. For the modeling and optimization purpose, some basic notations are introduced. Table 2 shows the index used in the CTPN model. Table 3 and Table 4 gives the model parameters and decision variables in the CTPN, respectively.
Places and transitions in CTPN | Description |
s0 | The output buffer of the inspection center |
si (i=1, 2, ..., 12) | The input buffer of each recovery workstation |
sz (z=13, 14, 15, 16) | The output buffer of each recovery workstation |
s17 | The input buffer of the reassembly center |
wk (k=1, 2, ..., 12) | Workstation places |
ti, j (i, j=1, 2, ..., 12, i≠j) | Recovery operations |
t0, i, ti, z, ti,17 (i=1, 2, ..., 12, z=13, 14, 15, 16) | Transportation transitions |
Index | Description |
L= {1, 2, ..., L} | index set of used products |
D= {1, 2, ..., D} | index set of component types |
K= {1, 2, ..., K} | index set of workstations |
H= {1, 2, ..., H} | index set of recovery process routings |
Parameters | Description |
ϑld | the dth core disassembled from the lth product |
wk | the kth workstation that performs a specific recovery operation |
Rh | the hth recovery process routing designed for worn cores and is represented as a set of recovery operations |
pre(tij, Rh) | the operational stages of recovery operation tij in Rh |
Last(Rh) | the last recovery operation in Rh |
aldh | a binary variable with a value 1 indicates the hth routing for the core ϑld |
u(tij, ϑld) | the time for a core ϑld to be processed in transition tij |
AT(si, ϑld) | the arrival time of the core ϑld at buffer place si |
ST(wk, ϑld) | the start time of the core ϑld being processed by wk |
Com(wk, ϑld) | the completion time of the core ϑld processed by wk |
ρ(ϑld) | the makespan of the component ϑld |
χ(ϑld) | the tardiness of the component ϑld |
ζ(l) | the tardiness of the lth product |
Probld | the probability of a core ϑld being selected for routing reassignment |
Πh,xld | the difference of the total average waiting time between the hth routing and the xth one for the core ϑld |
WTk | the average waiting time per core consumed in the kth workstation |
h | the total average waiting time per core consumed in the hth routing |
vh,xld | the probability of the xth routing being selected to replace the current hth routing for the core ϑld |
Slackxy | slack time of a core ϑxy in scheduling horizon |
Decision variables | Description |
rldh | a binary variable with a value 1 indicates that the hth process routing is assigned to a core ϑld |
Pri(wk, ϑld) | the priority for the dth component of the lthused product to be processed by the kth workstation |
(1) Modeling of recovery process routings
As stated earlier, the recovery process routing for a particular core is not deterministic but rather contingent on its damage level. When a core goes through inspection center and enter in the buffer place s0, it will be assigned with a specified process routing (i.e., Rh), which is represented as a set of recovery operations that a core should go through sequentially for its recovery.
For the cores with alternative process routings to be recovered, decisions should be made on which "reasonable" process routings to be assigned to them. In the proposed CTPN model, the buffer place s0 operates as a decision agent of choosing optimal recovery process routings for cores, by utilizing a hybrid scheduling algorithm elaborated in Section 3.
When the process routings for cores are determined, cores of different types and stochastic damage conditions require going through various recovery operations. In the CTPN model of Figure 3, different types of core tokens in s0 might go to transitions among t0, 12, t0, 5, t0, 1, t0, 3, t0, 2, t0, 6, t0, 8, t0, 11; tokens in s13 may either go to t13, 3, t13, 10 or t13, 17; tokens in s16 might enters into either t16, 17 or t16, 5. In addition, cores of the same type and the same damage condition might go through different recovery processes. For example, when a spindle is detected with abrasion, its process routing can either be R1 = {t03, t3, 14, t14, 9, t9, 17} or R2 = {t03, t3, 14, t14, 4, t4, 15, t15, 5, t5, 17}. Then in Figure 3, a token in s14 representing a spindle with abrasion might fire transition t14, 9 or t14, 4.
Thus, to make sure where the tokens in a buffer place should go, a unique color is introduced and associated with buffer places to distinguish the cores going through different recovery operations.
Definition 2: The color of a token ϑld in a buffer place si is defined as C(sj, ϑld) = cij if the token goes to tij after being released from si.
Let M(si) denote the number of tokens in place si regardless of token color, and M(si, cij) the number of tokens in place si at M that have the color cij. Therefore, M(si)=∑jM(si,cij).
Based on Definition 2, the color attached to a token in a buffer place changes through transition firings. Such color evolution represents the complex operational stages of a core according to its recovery process routing. For instance, if the recovery process routing of the core ϑld is determined (i.e., rldh is known), its color evolution can then be derived as follows.
C(sj,ϑld)=cjp, if {C(si,ϑld)=cijpre(tjp,Rh)=pre(tij,Rh)+1, ∀rldh=1si∈∙tij, sj∈∙tjp | (1) |
(2) Modeling of shared resources
In a remanufacturing job shop, various cores would compete for constrained resource to be recovered. An input buffer is then designated for each workstation in our CTPN model to accommodate all the cores that are waiting for processing. When multiple tokens occur in the input buffer place sk of the kth workstation, decisions should be made on which core to be processed next as soon as the machine resource is ready. In the proposed CTPN model, each input buffer place si (i = 1, 2, ..., 12) is considered as a decision agent for resource dispatching and determines the operation sequences (priority) for the cores to be processed by the workstation.
Definition 3: The priority for the core ϑld to be processed by the kth workstation is defined as Pri(wk, ϑld). The priority is a decision variable for scheduling optimization. The smaller the value is, the higher priority for the core to be processed by workstation wk.
Definition 4: A transition tij in the CTPN developed above is enabled at a marking M to process the core ϑld with a color cij, if and only if:
M(si,cij)⩾1,si∈∙tij | (2) |
M(wk)=1,wk∈∙tij∩t∙ij | (3) |
Pri(wk,ϑld)=min(Pri(wk,ϑxy))andC(si,ϑxy)=cij,∀x,y | (4) |
Definition 5: An enabled transition tij can fire in a marking M with respect to a color cij yielding a new marking M' denoted as M[(tij,cij)>M′:
M′(si,cij)=M(si,cij)−1,si∈∙tij | (5) |
M′(wk)=M′(wk),wk∈∙tij∩t∙ij | (6) |
Definition 6: When a transition tij is fired, the marking M' would transfer to M" according to:
M″ | (7) |
M''({w_k}) = M'({w_k}) + 1{,^{}}{w_k} \in {}^ \bullet {t_{ij}} \cap t_{ij}^ \bullet | (8) |
M''({{s}_{j}},{{c}_{jp}})=M'({{s}_{j}},{{c}_{jp}})+1\ \text{and}\ C({{s}_{j}},{{\vartheta }_{ld}})={{c}_{jp}}\ \text{if}\left\{ \begin{align} & {{s}_{j}}\in t_{ij}^{\bullet },\ {{s}_{j}}\in {}^{\bullet }{{t}_{jp}} \\ & pre({{t}_{jp}},{{R}_{h}})=pre({{t}_{ij}},{{R}_{h}})+1 \\ \end{align} \right. | (9) |
According to the above transition enabling and firing rules, the system always dispatch an available workstation wk to the core with the highest priority (i.e., the lowest Pri(wk, ϑld) value) in the queue to maximize the system performance.
(3) Modeling of recovery operation times
When associating time with operation transitions, the CTPN model is able to describe the temporal aspect of recovery operations.
Definition 7: The time needed for a token ϑld to be processed in transition tij is defined as u(tij, ϑld).
The processing times required to perform a necessary recovery operation is highly variable since the quality and composition of returned products/parts varies. It is reported that the processing time data obtained from a remanufacturing facility follows an exponential distribution in order to simulate the large range of possible values [8]. The amount of processing time for a remanufacturing operation is widely modeled as an exponential function of the wear associated with a part [19]. In this section, we models the recovery operation time as the sum of average operation time and extended time, where the latter is a exponential function of the quality condition of the core being processed. To that end, quality testing of a core is conducted before recovery, on which an inspection score, Score(ϑld)∈[0, 1], is assigned to the core based. It is assumed that the better the quality, the higher the score and the shorter the operation time. So the relationship between the quality score and the processing time is modeled as follows.
u({t_{ij}},{\vartheta _{ld}}) = \frac{{ - \ln (Score({\vartheta _{ld}}))}}{{\beta ({w_k})}} + \lambda ({w_k}){,_{}}{t_{ij}} \in w_k^ \bullet { \cap ^ \bullet }{w_k} | (10) |
where β(wk) is a control factor for workstation wk whose value is determined based on statistics of historical data, and λ(wk) is the average operation time of cores in wk. Whenever a component goes into a transition for processing, it must stay in transition tj for more thanu(tj, ϑld) time units and then can be released into another buffer place.
(4) Temporal aspect of recovery operations
In the CTPN model, with the core tokens dynamically entering the buffer places, a set of transitions are then enabled and fired according to the enabling and firing rules. With the dynamic behavior of the CTPN, the remanufacturing operations are subject to the following constraints.
Operation start time requirements: Recovery operations cannot be started until the core has arrived at the buffer sk of a corresponding workstation wk. When the core arrived in the buffer sk, it need waiting until all other cores with higher priorities are processed.
ST({w_k},{\vartheta _{ld}}) = {\text{max\{ }}A{\text{T}}({s_i},{\vartheta _{ld}}),Com({w_k},{\vartheta _{ij}}){\text{\} }}{{\text{,}}^{}}\forall Pri({w_k},{\vartheta _{ld}}) = Pri({w_k},{\vartheta _{ij}}) + 1,{s_i} \in {}^ \bullet {t_{ij}},{t_{ij}} \in {}^ \bullet {w_k} \cap w_k^ \bullet | (11) |
Processing completion time requirements: When a core goes into a workstation for processing, it must stay in a specific machine for more thanu(ϑld, wk) time units and then can be released into the successor buffer.
Com({w_k},{\vartheta _{ld}}) = ST({w_k},{\vartheta _{ld}}) + u({t_{ij}},{\vartheta _{ld}}){,^{}}\forall {t_{ij}} \in w_k^ \bullet { \cap ^ \bullet }{w_k} | (12) |
Arrival time constraints: When a core is finished processing by a workstation, it can be transported immediately into another buffer of the successor workstation by ignoring the transportation time.
A{\rm{T}}({s_i},{\vartheta _{ld}}) = Com({w_k},{\vartheta _{ld}}),\;\forall \left\{ \begin{array}{l} {r_{ldh}} = 1\\ {\rm{pre}}({w_v},{R_h}) = {\rm{pre}}({w_k},{R_h}) + 1\\ {s_i} \in {}^ \bullet {t_{ij}},\;{t_{ij}} \in {}^ \bullet {w_v} \cap w_v^ \bullet \end{array} \right. | (13) |
Using Eqs 11, 12 and 13 recursively, the makespan and tardiness of the component ϑld, i.e., ρ(ϑld) and \chi ({\vartheta _{ld}}), can be derived as shown in Eqs 14 and 15. The tardiness of the lth product is then calculated in Eq 16.
\rho ({\vartheta _{ld}}) = Com({w_k},{\vartheta _{ld}}) - AT(l){,^{}}\forall Last({R_h}) = {t_{ij}}{,^{}}{t_{ij}} \in w_k^ \bullet { \cap ^ \bullet }{w_k} | (14) |
\chi ({\vartheta _{ld}}) = \max \{ 0{,^{}}\rho ({\vartheta _{ld}}) - Due(l)\} | (15) |
\zeta (l) = \mathop {\max }\limits_d \chi ({\vartheta _{ld}}) | (16) |
Generally speaking, there are two ways to solve a scheduling problem by PN. One is to construct their reachability graph. However, it suffers from the state explosion problem [26]. Since we considered two types of decision variables in the proposed CTPN, using reachability graph for scheduling optimization might not be efficient. The other is to introduce scheduling algorithms into a PN model, which is adopted in this paper. The embedding style is optionally through places or transitions in a PN. This work uses the former mechanism and adopts two types of decision places in the proposed CTPN model. The decisions of choosing optimal recovery process routings for cores are embedded in buffer place s0, while the input buffer place si (I = 1, 2, ..., 12) makes decisions on dispatching machine resource in workstation to perform recovery operations for the cores that are waiting in queue. Considering the two types of scheduling decisions, a hybrid meta-heuristic algorithm using simulated annealing and minimum slack time rule is proposed and embedded in these decision places to search for global optimal process plans and schedules with a quick convergence speed.
The scheduling objective is to minimize both operation cost and tardiness penalty as shown in Eq 17. There are two types of decision variables: (1) recovery process routing for a given core (i.e., rldh), and (2) operation sequences for a given core in a workstation (i.e., Pri(wk, ϑld)).
Minimize:
TC = \sum\limits_l {\sum\limits_d {\sum\limits_{} {u({t_{ij}},{\vartheta _{ld}}) \cdot \phi ({w_k})} + \sum\limits_l {\zeta (l) \times \varpi } } } ,\;\forall \left\{ \begin{array}{l} {r_{ldh}} = 1\\ {t_{ij}} \in {R_h}\;{\rm{and}}\;{t_{ij}} \in w_k^ \bullet \end{array} \right. | (17) |
where φ(wk) is unit operation cost of the kth workstation and ϖ is unit penalty cost for product.
SA is a stochastic gradient method for global optimization that has been applied to a wide variety of sophisticated combinatorial problems [27, 28, 29]. While it conducts local searches, it is capable of exploring the solution space stochastically to prevent from being trapped in a local optimum. Starting from an initial solution g0, SA uses a certain mechanism to generate a neighborhood solution g' with an acceptance probability AP.
AP = \left\{ \begin{array}{l} 1,\;if\;\Delta \lt 0\\ \exp ( - \Delta /\Omega ),\;otherwise \end{array} \right. | (18) |
where Δ = f(g')-f(g0), Ω is called temperature, a global time-varying parameter, and f(g) the optimization objective function. In this paper, SA is used to determine the optimal process routings for cores. At each iteration when a neighbor solution is generated, MST to be elaborated in section 3.2 is then applied to determine the operation sequences of the cores in each workstation. The parameter Ω is reduced by a factor η at each iteration and the chance of choosing an inferior solution decreases as well. The nested SA-MST search process continues until the stopping criterion is met.
In this section, the CTPN model is integrated and embedded with SA algorithm and MST dispatching rules to obtain the optimal remanufacturing routings and schedules. At each iteration of SA algorithm, the feasible recovery routing is first generated by the Initial/Neighborhood Solution Generation process. Then the CTPN model is established based on the arrival time of parts, the predetermined recovery routings and the processing time of each operations. Whenever multiple parts arrived in a same buffer place in the CTPN, MST rules is used for resource dispatching. The CTPN model evolves to a final version until the resource conflicts in all the buffer places are tackled by the SMT. Finally, the operation cost and tardiness penalty of a SA solution at each iteration can be calculated according to the time aspects marked in the CTPN, and the next iteration of SA algorithm goes on until the stopping criterion is met. The flowchart of the CTPN-based hybrid meta-heuristics scheduler is shown in Figure 4.
(1) SA solution representation
In this paper, a 3-dimension matrix R = [rldh]L×D×H is proposed as representation of SA solutions. Each element rldh is a binary variable, one indicating the hth recovery process routing is assigned to the core ϑld and zero otherwise. Given that not all routings are feasible to a specific core, the viability of a randomly generated R is controlled via a logic AND operation in Eq 19:
R = \text{ }R\wedge A = \text{ }[{{r}_{ldh}}\wedge {{a}_{ldh}}] | (19) |
where A = [aldh] is a L-by-D-by-H mask whose element aldh = 1 indicates the feasibility of the hth routing for the core ϑld.
(2) Initial solution generation
The choice of an initial solution is vital to the performance of the algorithm [30]. To that end, a cost function of a routing (i.e., the hth routing) for a core ϑld is defined in Eq 20. The smaller the value of qldh, the greater chance the hth routing is selected for the core, i.e., rldh = 1.
{q_{ldh}} = \frac{{\sum\limits_j {u({t_{ij}},{\vartheta _{ld}}) \times \phi ({w_k})} }}{{\sum\limits_h {\sum\limits_j {u({t_{ij}},{\vartheta _{ld}}) \times \phi ({w_k})} } }},{t_{ij}} \in {R_h},{r_{ldh}} = 1,{t_{ij}} \in {}^ \bullet {w_k} \;{\rm{I}}\; w_k^ \bullet | (20) |
(3) Neighborhood solution generation
Traditional SA randomly generates a neighborhood solution g' from the current solution g by using a predefined move mechanism [31, 32]. In this section, a multiple moves mechanism is used for neighborhood solution generation [33, 34]. That is, for each current solution, randomly selects an element in the matrix R and uses one type of moves (i.e., ζ-opt, ζ∈{1, 2, …, H-h}) to exchange rldh with rld[h+ζ] for a new neighborhood solution.
To improve the convergence of SA, this work opts out the random selection process. Instead, at each annealing step, the proposed SA identifies cores with the potential to improve their routing assignment and to minimize their tardiness. To that end, χ(ϑld) is used to evaluate the urgency of routing reassignment for cores. The probability of a core being selected for routing reassignment is defined as follows.
pro{b_{ld}} = \chi ({\vartheta _{ld}})/\sum\limits_l {\sum\limits_d {\chi ({\vartheta _{ld}})} } | (21) |
For any identified core, the selection of an alternative yet better routing is determined based on an efficiency index, \Pi _{ld}^{h, x}, which calculates the difference of the total average waiting time between the current process routing (i.e., the hth routing) and the alternative one (i.e., xth routing):
\Pi _{ld}^{h,x} = {{\mathit{\Psi}} _h} - {{\mathit{\Psi}} _x},\forall \left\{ \begin{array}{l} {r_{ldh}} = 1\\ x \in \left\{ {1,2, \cdots ,H} \right\} - \left\{ h \right\}\\ {r_{ldx}} = 0,\;{a_{ldx}} = 1 \end{array} \right. | (22) |
{\Psi _h} = \sum\limits_{{t_{ij}} \in {R_h},{w_k} \in {}^ \bullet {t_{ij}}} {W{T_k}} \begin{array}{*{20}{c}} {}&{} \end{array}{\Psi _x} = \sum\limits_{{t_{ij}} \in {R_x},{w_k} \in {}^ \bullet {t_{ij}}} {W{T_k}} | (23) |
W{T_k} = \frac{{\sum\limits_l {\sum\limits_d {(ST({w_k},{\vartheta _{ld}}) - AT({s_i},{\vartheta _{ld}}))} } }}{{{N_k}}} | (24) |
where WTk is the average waiting time per core consumed in the kth workstation, Ψh and Ψx are the total average waiting time per core consumed in the hth and xth process routing, respectively, and Nk is the total number of cores being processed by workstation wk. When the core ϑld is switched from the current routing to the other one with a larger \Pi _{ld}^{h, x}, it is more likely to relieve resource conflict and accomplish the task with a greatly reduced tardiness. Therefore, the probability of the xth routing being selected to replace the current hth routing for the core is defined as
v_{ld}^{h,x} = \Pi _{ld}^{h,x}/\sum\limits_{\begin{array}{*{20}{c}} {x \in \left\{ {1,2, \cdots ,H} \right\} - \left\{ h \right\}}\\ {{a_{ldx}} = 1} \end{array}} {\Pi _{ld}^{h,x}} | (25) |
(4) Stopping criterion
In this proposed algorithm, two thresholds are pre-defined: the maximum number of inefficient iterations where the global optimum is not updated Imax, and the maximum number of outer loop iterations olmax. Our SA will stop whenever either of the thresholds is reached.
With a SA solution, each core follows the derived process routing to be recovered step by step. When multiple cores enter a workstation and compete for the same machine resource, a secondary optimization is conducted to determine the operation sequences of cores to be processed by the workstation, aiming to minimize the total tardiness penalty. The dispatching rules have been widely used for operation sequence optimization to address job shop scheduling problems [35]. Minimum Slack Time (MST), as one of typical heuristic sequencing rules, is widely used for the scheduling problems. MST measures the "urgency" of a job by its slack time, which is defined as the difference between its due date and production time. That is, the shorter the slack time, the higher priority to be processed. In the case of MST dispatching rules, the jobs might be processed as soon as possible in order to be finished right before its due date. As a result, the tardiness of all the jobs can be minimized. Recently, MST rule have been widely used for operation sequence optimization and are embedded with some meta-heuristic approaches to address various scheduling problems [36, 37]. In this section, MST is chosen for resource dispatching and is embedded with SA algorithm to search for the optimal schedules in minimizing both the operation cost and the penalty cost with related to tardiness.
For a specific core, the slack time of its recovery operation can be calculated as the temporal difference between the due date, the current time, and the remaining operation times, as formulated in Eq 26. MST sequences jobs in the descending order of slack time. Whenever a workstationwk releases a component ϑuv(∀Pri(wk, ϑuv) = q > 0) and turns back into idle state, it will pick up a core ϑld with the least slack time (\exists slackld = min{slackxy}) from the waiting queue (∀ϑxy, AT(si, ϑxy)-Com(wk, ϑld) ≤ 0), and then immediately process it in the (q + 1)th sequence, as shown in Eq 27.
slac{k_{xy}} = Due(l) - Com({w_k},{\vartheta _{uv}}) - \sum\limits_{pre({t_{cd}},{R_h}) \geqslant pre({t_{ij}},{R_h})} {u({t_{cd}},{\vartheta _{xy}})} \mathop ,\limits^{} \mathop {}\limits^{} \\\forall {r_{xyh}} = 1{,^{}}{t_{ij}} \in {R_h}{,^{}}{t_{cd}} \in {R_h}{,^{}}{t_{ij}} \in {}^ \bullet {w_k} \cap w_k^ \bullet {,^{}}Pri({w_k},{\vartheta _{uv}}) = q | (26) |
Pri({w_k},{\vartheta _{ld}}) = q + 1,\;if\;\\\left\{ \begin{array}{l} AT({s_i},{\vartheta _{xy}}) - Com({w_k},{\vartheta _{uv}}) \le 0,\;\forall {r_{uvh}} = 1,{t_{ij}} \in {R_h},{s_i} \in {}^ \bullet {t_{ij}},\;{t_{ij}} \in {}^ \bullet {w_k} \cap w_k^ \bullet ,\;Pri({w_k},{\vartheta _{uv}}) = q\\ slac{k_{ld}} = \min \left\{ {slac{k_{xy}}} \right\},\forall {r_{xyh}} = 1,{t_{ij}} \in {R_h} \end{array} \right. | (27) |
The solution obtained by MST is a 3-dimension matrix G = [gldk]L×D×K, where gldk, a non-negative integer, represents the operation sequence of the core ϑld to be performed by workstation wk. gldk = 0 means that the core ϑld is not processed by wk. Once the matrix G is derived, Pri(wk, ϑld) in CTPN are determined and thereby the total cost can be calculated.
With the recovery process routing R and operation sequence matrix G being derived through the proposed hybrid meta-heuristics, a set of transitions are then be enabled and fired as core tokens dynamically enter or release from the buffer places.
To fully understand the above concepts and algorithms, the remanufacturing of a batch of obsolete machine tools in an example system is used to demonstrate the remanufacturing scheduling. The workflows of cores in this remanufacturing shop and its corresponding CTPN are shown in Figs. 2 and 3. In order to verify the efficiency of the proposed method, three cases are considered for simulation. In the baseline case, cores are recovered through a set of fixed process routings. An available workstation takes MST rule for resource dispatching. In the second case, the traditional SA uses a standard neighborhood generation method and is embodied with MST rule for routing assignment and resource dispatching. In the third case, the recovery routing for a core and the resource dispatching for a workstation are guided through the proposed SA/MST by using the proposed neighborhood solution generation method.
All the algorithms are implemented in Matlab 2009. The simulation running duration is set to be 30 days. Everyday's work hours are 24 hours. Data of the first 3 days are thrown off as warm-up consideration. For the simulation, the input data is populated as follows.
(1) Since that the machine resources in the floor shop are fixed and limited, the machine workloads and the intension of resource conflicts increases with the rise of arrival rate, and vice versa. In order to simulated a set of resource conflict scenarios, the random arrival of used machine tools follows a Poisson distribution with an arrival rate per hour π \in {5, 6, 7, 8, 9, 10, 11}. The designed arrival rate can limit the machine workloads (i.e., the average length of waiting queue on the machines) in the floor shop within a satisfactory range [0.75, 3.6].
(2) The due date (in days) for each used machine tool is randomly generated by a uniform distribution, Due(l) ~ U[5, 10].
(3) Each core disassembled from used machine tools is inspected before its recovery and is assigned a quality score Score(ϑld) through an Exponential distribution, Score(ϑld))~ Γ(1, τ) where τ ∈ [0.08, 0.10].
(4) The control factor and average operation time for each workstation is set to β(wk) = 0.2 and λ(wk) = 15.
(5) The operation cost per hour in workstations 7 and 9 (i.e., chromium electroplating and laser cladding) is $100, while that in other workstations is $50.
(6) The penalty cost per day per machine tool is set to be ϖ ∈ {20, 60, 100} in dollars.
(7) The parameters in SA are set toolmax = 5000, ilmax = 100, Imax = 30, Ω = 169340, η = 0.998.
(8) Considering that the total number (L) of machine tools generated in each run of the simulation varies, our comparison focuses on normalized objective values. In particular, the comparison evaluates the total cost per product (tc), operation cost per product (pc), tardiness penalty per product (dc), and the average waiting time per core consumed in each workstation (wt).
Our first set of experiments evaluates how the three methods respond to system demand and various penalty cost rates. In each trial, we run the three methods independently. It should be noted that the baseline case only employs MST rule for resource scheduling, while the proposed SA/MST and the standard one run continuously until they meet the stopping criteria. As the arrival rate of used machine tools changes from 5 to 11, the average total cost tc is obtained and compared among the three methods. This process is repeated with different penalty cost rates (i.e., ϖ = 20, 60, and 100) as shown in Tables 5, 6, and 7, respectively.
Arrival rate | The average total cost per machine tool | Improvement of the standard method over the baseline one tc1–tc2 | Improvement of the proposed method over the standard one tc2–tc3 | ||
Baseline casetc1 | Standard SA/MSTtc2 | Proposed SA/MSTtc3 | |||
π=5 | 186.66 | 117.02 | 117.02 | 69.64 | 0 |
π=6 | 228.37 | 133.48 | 118.21 | 94.89 | 15.27 |
π=7 | 293.05 | 172.45 | 146.89 | 120.6 | 25.56 |
π=8 | 335.87 | 208.06 | 168.69 | 127.81 | 39.37 |
π=9 | 447.52 | 266.47 | 216.25 | 181.05 | 50.22 |
π=10 | 581.63 | 352.28 | 270.91 | 229.35 | 81.37 |
π=11 | 714.29 | 461.86 | 335.77 | 252.43 | 126.09 |
Sample mean | 398.20 | 244.52 | 196.25 | 153.68 | 48.27 |
Sample standard deviation | 193.44 | 125.46 | 82.67 | 68.93 | 43.15 |
Arrival rate | The average total cost per machine tool | Improvement of the standard method over the baseline onetc1–tc2 | Improvement of the proposed method over the standard onetc2–tc3 | ||
Baseline casetc1 | Standard SA/MSTtc2 | Proposed SA/MSTtc3 | |||
π=5 | 215.76 | 117.83 | 117.83 | 97.93 | 0 |
π=6 | 327.61 | 160.31 | 140.12 | 167.3 | 20.19 |
π=7 | 455.9 | 226.47 | 185.02 | 229.43 | 41.45 |
π=8 | 658.09 | 342.57 | 273.22 | 315.52 | 69.35 |
π=9 | 891.23 | 515.96 | 406.38 | 375.27 | 109.58 |
π=10 | 1137.96 | 738.41 | 559.67 | 399.55 | 178.74 |
π=11 | 1530.54 | 986.65 | 775.72 | 543.89 | 210.93 |
Sample mean | 745.30 | 441.17 | 351.14 | 304.13 | 90.03 |
Sample standard deviation | 472.28 | 324.23 | 244.76 | 151.99 | 80.25 |
Arrival rate | The average total cost per machine tool | Improvement of the standard method over the baseline onetc1–tc2 | Improvement of the proposed method over the standard onetc2–tc3 | ||
Baseline casetc1 | Standard SA/MSTtc2 | Proposed SA/MSTtc3 | |||
π=5 | 270.84 | 117.32 | 117.32 | 153.52 | 0 |
π=6 | 463.08 | 224.8 | 186.58 | 238.28 | 38.22 |
π=7 | 687.42 | 416.46 | 275.13 | 270.96 | 141.33 |
π=8 | 1063.8 | 655.68 | 453.47 | 408.12 | 202.21 |
π=9 | 1495.67 | 957.36 | 706.49 | 538.31 | 250.87 |
π=10 | 1917.52 | 1231.56 | 918.76 | 685.96 | 312.8 |
π=11 | 2403.39 | 1483.89 | 1120.77 | 919.5 | 363.12 |
Sample mean | 1185.96 | 726.72 | 539.79 | 459.24 | 186.94 |
Sample standard deviation | 789.17 | 517.36 | 385.07 | 273.81 | 135.61 |
The performance of the standard SA/MST and the baseline one is first evaluated and compared by calculating the cost difference under different arrival rates, as shown in Figure 5. It is clear that the proposed SA/MST outperforms the baseline method in terms of generating less cost, particularly in reacting to the larger arrival rates. This is reasonable since that when a substantial amount of cores are fighting for limited resources for to be recovered, the standard SA/MST operates on routings reassignment of cores to reduce the ever intensified resource conflicts and thus minimize a relatively large amount of tardiness penalty to lower the total cost, while the baseline method is not capable of addressing so by fixing process routings of cores. The results in Figure 5 can be also interpreted as evidence that the hybrid SA/MST is promising for remanufacturing scheduling optimization.
The differences of total cost per product obtained by the two hybrid methods are also calculated and plotted in Figure 6. The following remarks can be made regarding the performance of the proposed SA/MST over the standard one.
(1) When the arrival rate is low (e.g., π = 5), the system has sufficient enough resources to handle remanufacturing demand. Thus the benefit of our proposed SA/MST in comparison to the standard one is minor. As the system demand increases with the rising arrival rate, more and more used machine tools are fighting for the limited resource to be remanufactured. The proposed method then outperforms the standard method through a more targeted annealing process to properly reassign recovery routings of cores and resolve ever intensified resource conflict. Therefore, the advantage of the proposed method over the standard one becomes significant.
(2) In a similar fashion, by comparing the corresponding row in Tables 5, 6 and 7, the relative improvement of the proposed method over the standard one becomes more and more significant with the increasing penalty cost. Using π = 8 as an example, the improvement increases from 11% when ϖ = 20, to 17% when ϖ = 60, and to 30% when ϖ = 100.
To further show the significant difference between the performances of the two hybrid algorithms, a sample mean, a sample standard deviation and confident interval estimation are introduced. A relative value σ = –(tc3–tc2)/tc2 is defined to characterize the improvement of the best solution obtained by the proposed SA/MST over the one obtained by the standard one. A 95% confidence interval of the improvement rate σ is conducted using the data in Tables 5–7. The approximate 100(1–α)% confidence interval for σ is defined as
\begin{align} & \ \ \ \ \ \ \ \ \ \ \ \ \ \ \ \ \ \ \ \ \ \ \ \ \overset{\lower0.5em\hbox{$\smash{\scriptscriptstyle\frown}$}}{\sigma }\pm {{t}_{{1-\alpha }/{2}\;}}(n-1)\frac{SD}{\sqrt{n}} \\ & or\ \overset{\lower0.5em\hbox{$\smash{\scriptscriptstyle\frown}$}}{\sigma }-{{t}_{{1-\alpha }/{2}\;}}(n-1)\frac{SD}{\sqrt{n}}\le \sigma \le \overset{\lower0.5em\hbox{$\smash{\scriptscriptstyle\frown}$}}{\sigma }+{{t}_{{1-\alpha }/{2}\;}}(n-1)\frac{SD}{\sqrt{n}} \\ \end{align} | (28) |
where {\overset{\lower0.5em\hbox{$\smash{\scriptscriptstyle\frown}$}}{\sigma }} is the sample mean of σ based on a sample of size n; SD is the sample standard deviation; {{t}_{{1-\alpha }/{2}\; }}(n-1)is the 100(1–α)% percentage point of a t-distributed with n–1 degree of freedom. The 95% confidence intervals for the improvement σ under three scenarios (i.e., ϖ = 20, 60 and 100) are given in Eqs 29, 30 and 31, respectively. It is obvious that the 95% confidence interval for σ lies completely above zero (i.e., \overset{\lower0.5em\hbox{$\smash{\scriptscriptstyle\frown}$}}{\sigma } > 0), which provides strong evidence that the proposed SA/MST is better than the standard one in terms of generating less total cost.
0.0814 \leqslant {\overset{\lower0.5em\hbox{$\smash{\scriptscriptstyle\frown}$}}{\sigma } _1} \leqslant 0.2455 | (29) |
0.1042 \leqslant {\overset{\lower0.5em\hbox{$\smash{\scriptscriptstyle\frown}$}}{\sigma } _2} \leqslant 0.3118 | (30) |
0.1414 \leqslant {\overset{\lower0.5em\hbox{$\smash{\scriptscriptstyle\frown}$}}{\sigma } _3} \leqslant 0.3911 | (31) |
The results in Tables 5–7 represents significant enhancement of the proposed methodology in terms of overall total cost per product, especially when the system is over utilized and its responsiveness to fluctuating market demand is important.
In the second set of experiments, the performance of the two hybrid algorithms is further evaluated by calculating the normalized objective values achieved after pre-specified time breaks (Table 8), where the arrival rate and the penalty cost are set as π = 8 and ϖ = 60. The following remarks can be made regarding algorithms' performance.
Computation time breaks | Standard SA/MST | Proposed SA/MST | ||||||
tc1 | pc1 | dc1 | wt1 | tc2 | pc2 | dc2 | wt2 | |
After 0sec | 399.25 | 112.16 | 287.09 | 76.80 | 399.25 | 112.16 | 287.09 | 76.80 |
After 30sec | 380.38 | 116.78 | 263.60 | 72.37 | 371.14 | 114.62 | 256.51 | 55.21 |
After 60sec | 393.70 | 113.71 | 279.99 | 61.76 | 341.42 | 118.56 | 222.86 | 48.34 |
After 90sec | 393.70 | 113.71 | 279.99 | 58.90 | 311.93 | 120.51 | 191.43 | 46.49 |
After 120sec | 386.11 | 115.62 | 270.49 | 58.75 | 292.79 | 121.75 | 171.04 | 44.54 |
After 150sec | 385.56 | 119.96 | 265.60 | 58.42 | 280.06 | 123.28 | 156.78 | 41.52 |
After 180sec | 384.17 | 121.18 | 262.99 | 57.90 | 274.14 | 124.64 | 149.51 | 41.47 |
After 210sec | 383.35 | 122.86 | 260.49 | 56.51 | 273.33 | 126.31 | 147.03 | 38.40 |
After 240sec | 378.22 | 124.65 | 252.57 | 54.73 | 273.22 | 132.79 | 140.42 | 35.67 |
No time limits* | 342.57 | 129.41 | 213.16 | 48.07 | 273.22 | 132.79 | 140.42 | 35.67 |
* "No time limit" means the simulation keeps the algorithm running until it meets the stopping criteria elaborated above. |
(1) As the computation time increases, both algorithms strive to search for a better scheduling solution that maintains a good trade-off between operation cost and tardiness penalty. It should be noted that both algorithms start at the same initial solution (i.e., zero time break). Since the initial solution is generated with the goal of minimizing operation cost only, it presents a relatively larger penalty cost. The annealing step in both methods then begins to rearrange routings for cores that ultimately balances the overall workload of workstations and thus reduces waiting time to lower the tardiness penalty. As shown in Table 8, at the 210-second time break, the optimal solutions generated by both methods have a bit larger pc and a quite smaller dc, resulting in a much lower total cost per product.
(2) The proposed SA/MST method converges much rapidly than the standard one, providing a better solution within a given short time frame. This is intuitively understandable since the proposed SA/MST uses resource conflict and tardiness penalty to guide neighborhood solution generation while the random mechanism in the standard SA fails to address so, resulting in a relatively larger tardiness penalty.
All simulation output analysis verifies the effectiveness of the proposed hybrid SA/MST algorithm for the remanufacturing scheduling.
Remanufacturing systems are faced with a greater degree of uncertainty and complexity than traditional manufacturing systems, leading to the need for planning and control systems designed to deal with the added uncertainty and complexity. Although many new methodologies have been led up to deal with various operation management issues in remanufacturing environments, no study has comprehensively dealt with remanufacturing job shop scheduling in subjecting to alternative recovery operations, random operation times and limited resource conflicts. A CTPN is introduced to model the dynamics of remanufacturing process, such as various process routings, uncertain operations times and resource conflicts. With time and color attributes in PN, two types of decision variables are linked with places in CTPN and the evolution of system dynamics in recovery operations are mathematically analyzed. With the support of the computation model via CTPN, a hybrid meta-heuristic using SA and MST rule is proposed and embedded with CTPN to sample large search space efficiently and search for a good scheduling solution in minimizing total production cost. The performance of the proposed SA/MST algorithm is compared against another two cases: baseline case with fixed recovery process routings and case 2 using standard SA/MST. The comparison results provide strong evidence that the proposed scheduling method is of significant importance in achieving minimum production cost especially when the system is overloaded and its responsiveness to shared resource conflicts is important.
Our research can be also extended in several directions. For instance, the integration of alternative recovery routing selection and resource dispatching is a NP-hard problem. Therefore, it is worth of investigation to take the structure advantage of CTPN for a more efficient optimization heuristics. Another challenge is to take into consideration of unexpected system disruptions (i.e., random machine breakdown) in remanufacturing scheduling. The future work also includes further validation of our methodology using more factory data.
This work was supported in part by the Fundamental Research Funds for the Central Universities (SWU22300503), the National Natural Science Foundation of China (51875480) and the National Natural Science Foundation of China (51475059).
All authors declare that there is no conflict of interests in this paper.
[1] | F. Bauer, J. Jost, S. Liu, Ollivier-Ricci curvature and the spectrum of the normalized graph Laplace operator, arXiv, 2013. https://doi.org/10.48550/arXiv.1105.3803 |
[2] |
D. Cushing, S. Kamtue, J. Koolen, S. Liu, F. Münch, N. Peyerimhoff, Rigidity of the Bonnet-Myers inequality for graphs with respect to Ollivier Ricci curvature, Adv. Math., 369 (2020), 107188. https://doi.org/10.1016/j.aim.2020.107188 doi: 10.1016/j.aim.2020.107188
![]() |
[3] |
D. Cushing, S. Kamtue, R. Kangaslampi, S. Liu, N. Peyerimhoff, Curvatures, graph products and Ricci flatness, J. Graph Theory, 96 (2021), 522–553. https://doi.org/10.1002/jgt.22630 doi: 10.1002/jgt.22630
![]() |
[4] |
K. Devriendt, R. Lambiotte, Discrete curvature on graphs from the effective resistance, J. Phys.: Complex., 3 (2022), 025008. https://doi.org10.1088/2632-072X/ac730d doi: 10.1088/2632-072X/ac730d
![]() |
[5] |
R. Dunn, F. Dudbridge, C. M. Sanderson, The use of edge-betweenness clustering to investigate biological function in protein interaction networks, BMC Bioinf., 6 (2005), 39 https://doi.org/10.1186/1471-2105-6-39 doi: 10.1186/1471-2105-6-39
![]() |
[6] |
P. Elumalai, Y. Yadav, N. Williams, E. Saucan, J. Jürgen, A. Samal, Graph Ricci curvatures reveal atypical functional connectivity in autism spectrum disorder, Sci. Rep., 12 (2022), 8295. https://doi.org/10.1038/s41598-022-12171-y doi: 10.1038/s41598-022-12171-y
![]() |
[7] |
L. Fesser, S. S. de Haro Iváñez, K. Devriendt, M. Weber, R. Lambiotte, Augmentations of Forman's Ricci curvature and their applications in community detection, J. Phys.: Complex., 5 (2023), 035010. https://doi.org/10.1088/2632-072X/ad64a3 doi: 10.1088/2632-072X/ad64a3
![]() |
[8] |
Forman, Bochner's method for cell complexes and combinatorial Ricci curvature, Discrete Comput. Geom., 29 (2003), 323–374. https://doi.org/10.1007/s00454-002-0743-x doi: 10.1007/s00454-002-0743-x
![]() |
[9] |
S. Fortunato, Community detection in graphs, Phys. Rep., 486 (2010), 75–174. https://doi.org/10.1016/j.physrep.2009.11.002 doi: 10.1016/j.physrep.2009.11.002
![]() |
[10] |
S. Fortunato, D. Hric, Community detection in networks: a user guide, Phys. Rep., 659 (2016), 1–44. https://doi.org/10.1016/j.physrep.2016.09.002 doi: 10.1016/j.physrep.2016.09.002
![]() |
[11] |
A. Gosztolai, A. Arnaudon, Unfolding the multiscale structure of networks with dynamical Ollivier-Ricci curvature, Nat. Commun., 12 (2021), 4561. https://doi.org/10.1038/s41467-021-24884-1 doi: 10.1038/s41467-021-24884-1
![]() |
[12] |
R. Guimera, S. Mossa, A. Turtschi, L. A. N. Amaral, The worldwide air transportation network: anomalous centrality, community structure, and cities' global roles, Proc. Natl. Acad. Sci. U.S.A., 102 (2005), 7794–7799. https://doi.org/10.1073/pnas.0407994102 doi: 10.1073/pnas.0407994102
![]() |
[13] | V. Grout, S. Cunningham, A constrained version of a clustering algorithm for switch placement and interconnection in large networks, 19th ISCA International Conference on Computer Applications in Industry and Engineering, 2006,252–257. |
[14] |
P. Krishna, N. H. Vaidya, M. Chatterjee, D. K. Pradhan, A cluster-based approach for routing in dynamic networks, ACM SIGCOMM Comput. Commun. Rev., 27 (1997), 49–64. https://doi.org/10.1145/263876.263885 doi: 10.1145/263876.263885
![]() |
[15] | J. M. Lee, Introduction to Riemannian manifolds, 2 Eds., Springer, 2018. https://doi.org/10.1007/978-3-319-91755-9 |
[16] |
Y. Lin, L. Lu, S. T. Yau, Ricci curvature of graphs, Tohoku Math. J., Second Series, 63 (2011), 605–627. https://doi.org/10.2748/tmj/1325886283 doi: 10.2748/tmj/1325886283
![]() |
[17] |
F. Münch, R. K. Wojciechowski, Ollivier Ricci curvature for general graph Laplacians: heat equation, Laplacian comparison, non-explosion and diameter bounds, Adv. Math., 356 (2019), 106759. https://doi.org/10.1016/j.aim.2019.106759 doi: 10.1016/j.aim.2019.106759
![]() |
[18] |
C. C. Ni, Y. Y. Lin, F. Luo, J. Gao, Community detection on networks with Ricci flow, Sci. Rep., 9 (2019), 9984. https://doi.org/10.1038/s41598-019-46380-9 doi: 10.1038/s41598-019-46380-9
![]() |
[19] |
M. E. O'Kelly, A clustering approach to the planar hub location problem, Ann. Oper. Res., 40 (1992), 339–353. https://doi.org/10.1007/BF02060486 doi: 10.1007/BF02060486
![]() |
[20] |
Y. Ollivier, Ricci curvature of Markov chains on metric spaces, J. Funct. Anal., 256 (2009), 810–864. https://doi.org/10.1016/j.jfa.2008.11.001 doi: 10.1016/j.jfa.2008.11.001
![]() |
[21] |
O. Queen, G. A. McCarver, S. Thatigotla, B. P. Abolins, C. L. Brown, V. Maroulas, et al., Polymer graph neural networks for multitask property learning, npj Comput. Mater., 9 (2023), 90. https://doi.org/10.1038/s41524-023-01034-3 doi: 10.1038/s41524-023-01034-3
![]() |
[22] |
A. W. Rives, T. Galitski, Modular organization of cellular networks, Proc. Natl. Acad. Sci. U.S.A., 100 (2003), 1128–1133. https://doi.org/10.1073/pnas.0237338100 doi: 10.1073/pnas.0237338100
![]() |
[23] |
J. Sia, W. Zhang, E. Jonckheere, D. Cook, P. Bogdan, Inferring functional communities from partially observed biological networks exploiting geometric topology and side information, Sci. Rep., 12 (2022), 10883. https://doi.org/10.1038/s41598-022-14631-x doi: 10.1038/s41598-022-14631-x
![]() |
[24] |
J. Sia, E. Jonckheere, P. Bogdan, Ollivier-Ricci curvature-based method to community detection in complex networks, Sci. Rep., 9 (2019), 9800. https://doi.org/10.1038/s41598-019-46079-x doi: 10.1038/s41598-019-46079-x
![]() |
[25] |
V. Spirin, L. A. Mirny, Protein complexes and functional modules in molecular networks, Proc. Natl. Acad. Sci. U.S.A., 100 (2003), 12123–12128. https://doi.org/10.1073/pnas.2032324100 doi: 10.1073/pnas.2032324100
![]() |
[26] | S. Tan, J. Sia, P. Bogdan, R. Ivanov, Analyzing neural network robustness using graph curvature, 2024 International Conference on Assured Autonomy (ICAA), Nashville, TN, USA, 2024,110–113. https://doi.org/10.1109/ICAA64256.2024.00026 |
[27] | Y. Tian, Z. Lubberts, M. Weber, Mixed-membership community detection via line graph curvature, Proceedings of the 1st NeurIPS Workshop on Symmetry and Geometry in Neural Representations, 2023,219–233. |
[28] | J. Topping, F. Di Giovanni, B. P. Chamberlain, X. Dong, M. M. Bronstein, Understanding over-squashing and bottlenecks on graphs via curvature, arXiv, 2021. https://doi.org/10.48550/arXiv.2111.14522 |
[29] | Y. Tian, Z. Lubberts, M. Weber, Curvature-based clustering on graphs, arXiv, 2023. https://doi.org/10.48550/arXiv.2307.10155 |
[30] |
M. Weber, E. Saucan, J. Jürgen, Characterizing complex networks with Forman-Ricci curvature and associated geometric flows, J. Complex Netw., 5 (2017), 527–550. https://doi.org/10.1093/comnet/cnw030 doi: 10.1093/comnet/cnw030
![]() |
[31] |
J. Wee, K. Xia, Forman persistent Ricci curvature (FPRC)-based machine learning models for protein–ligand binding affinity prediction, Brief. Bioinform., 22 (2021), bbab136. https://doi.org/10.1093/bib/bbab136 doi: 10.1093/bib/bbab136
![]() |
[32] |
J. Wee, K. Xia, Ollivier persistent Ricci curvature-based machine learning for the protein–ligand binding affinity prediction, J. Chem. Inf. Model., 61 (2021), 1617–1626. https://doi.org/10.1021/acs.jcim.0c01415 doi: 10.1021/acs.jcim.0c01415
![]() |
[33] |
M. R. Znaidi, J. Sia, S. Ronquist, I. Rajapakse, E. Jonckheere, P. Bogdan, A unified approach of detecting phase transition in time-varying complex networks, Sci. Rep., 13 (2023), 17948. https://doi.org/10.1038/s41598-023-44791-3 doi: 10.1038/s41598-023-44791-3
![]() |
1. | Rudrajeet Pal, Yasaman Samie, Armaghan Chizaryfard, Demystifying process-level scalability challenges in fashion remanufacturing: An interdependence perspective, 2021, 286, 09596526, 125498, 10.1016/j.jclepro.2020.125498 | |
2. | Shuo Zhu, Hua Zhang, Zhigang Jiang, Bernard Hon, A carbon efficiency upgrading method for mechanical machining based on scheduling optimization strategy, 2020, 15, 2095-0233, 338, 10.1007/s11465-019-0572-8 | |
3. | Wenjie Wang, Guangdong Tian, Honghao Zhang, Kangkang Xu, Zheng Miao, Modeling and scheduling for remanufacturing systems with disassembly, reprocessing, and reassembly considering total energy consumption, 2021, 0944-1344, 10.1007/s11356-021-17292-x | |
4. | Guangyuan Zhang, Xiaonan Gao, Zhenfang Zhu, Fengyv Zhou, Dexin Yu, Determination of the location of the needle entry point based on an improved pruning algorithm, 2022, 19, 1551-0018, 7952, 10.3934/mbe.2022372 | |
5. | Xingwang Shen, Shimin Liu, Can Zhang, Jinsong Bao, Intelligent material distribution and optimization in the assembly process of large offshore crane lifting equipment, 2021, 159, 03608352, 107496, 10.1016/j.cie.2021.107496 | |
6. | Yaping Ren, Xinyu Lu, Hongfei Guo, Zhaokang Xie, Haoyang Zhang, Chaoyong Zhang, A Review of Combinatorial Optimization Problems in Reverse Logistics and Remanufacturing for End-of-Life Products, 2023, 11, 2227-7390, 298, 10.3390/math11020298 | |
7. | Wenyu Zhang, Jun Wang, Xiangqi Liu, Shuai Zhang, A new uncertain remanufacturing scheduling model with rework risk using hybrid optimization algorithm, 2023, 1614-7499, 10.1007/s11356-023-26219-7 | |
8. | Jun Guo, Junfeng Zou, Baigang Du, Kaipu Wang, Integrated scheduling for remanufacturing system considering component commonality using improved multi-objective genetic algorithm, 2023, 182, 03608352, 109419, 10.1016/j.cie.2023.109419 | |
9. | Wenyu Zhang, Jiaxuan Shi, Shuai Zhang, Mengjiao Chen, Scenario-Based Robust Remanufacturing Scheduling Problem Using Improved Biogeography-Based Optimization Algorithm, 2023, 53, 2168-2216, 3414, 10.1109/TSMC.2022.3225443 |
Places and transitions in CTPN | Description |
s0 | The output buffer of the inspection center |
si (i=1, 2, ..., 12) | The input buffer of each recovery workstation |
sz (z=13, 14, 15, 16) | The output buffer of each recovery workstation |
s17 | The input buffer of the reassembly center |
wk (k=1, 2, ..., 12) | Workstation places |
ti, j (i, j=1, 2, ..., 12, i≠j) | Recovery operations |
t0, i, ti, z, ti,17 (i=1, 2, ..., 12, z=13, 14, 15, 16) | Transportation transitions |
Index | Description |
L= {1, 2, ..., L} | index set of used products |
D= {1, 2, ..., D} | index set of component types |
K= {1, 2, ..., K} | index set of workstations |
H= {1, 2, ..., H} | index set of recovery process routings |
Parameters | Description |
ϑld | the dth core disassembled from the lth product |
wk | the kth workstation that performs a specific recovery operation |
Rh | the hth recovery process routing designed for worn cores and is represented as a set of recovery operations |
pre(tij, Rh) | the operational stages of recovery operation tij in Rh |
Last(Rh) | the last recovery operation in Rh |
aldh | a binary variable with a value 1 indicates the hth routing for the core ϑld |
u(tij, ϑld) | the time for a core ϑld to be processed in transition tij |
AT(si, ϑld) | the arrival time of the core ϑld at buffer place si |
ST(wk, ϑld) | the start time of the core ϑld being processed by wk |
Com(wk, ϑld) | the completion time of the core ϑld processed by wk |
ρ(ϑld) | the makespan of the component ϑld |
χ(ϑld) | the tardiness of the component ϑld |
\zeta (l) | the tardiness of the lth product |
Probld | the probability of a core ϑld being selected for routing reassignment |
\Pi _{ld}^{h, x} | the difference of the total average waiting time between the hth routing and the xth one for the core ϑld |
WTk | the average waiting time per core consumed in the kth workstation |
h | the total average waiting time per core consumed in the hth routing |
v_{ld}^{h, x} | the probability of the xth routing being selected to replace the current hth routing for the core ϑld |
Slackxy | slack time of a core ϑxy in scheduling horizon |
Decision variables | Description |
rldh | a binary variable with a value 1 indicates that the hth process routing is assigned to a core ϑld |
Pri(wk, ϑld) | the priority for the dth component of the lthused product to be processed by the kth workstation |
Arrival rate | The average total cost per machine tool | Improvement of the standard method over the baseline one tc1–tc2 | Improvement of the proposed method over the standard one tc2–tc3 | ||
Baseline casetc1 | Standard SA/MSTtc2 | Proposed SA/MSTtc3 | |||
π=5 | 186.66 | 117.02 | 117.02 | 69.64 | 0 |
π=6 | 228.37 | 133.48 | 118.21 | 94.89 | 15.27 |
π=7 | 293.05 | 172.45 | 146.89 | 120.6 | 25.56 |
π=8 | 335.87 | 208.06 | 168.69 | 127.81 | 39.37 |
π=9 | 447.52 | 266.47 | 216.25 | 181.05 | 50.22 |
π=10 | 581.63 | 352.28 | 270.91 | 229.35 | 81.37 |
π=11 | 714.29 | 461.86 | 335.77 | 252.43 | 126.09 |
Sample mean | 398.20 | 244.52 | 196.25 | 153.68 | 48.27 |
Sample standard deviation | 193.44 | 125.46 | 82.67 | 68.93 | 43.15 |
Arrival rate | The average total cost per machine tool | Improvement of the standard method over the baseline onetc1–tc2 | Improvement of the proposed method over the standard onetc2–tc3 | ||
Baseline casetc1 | Standard SA/MSTtc2 | Proposed SA/MSTtc3 | |||
π=5 | 215.76 | 117.83 | 117.83 | 97.93 | 0 |
π=6 | 327.61 | 160.31 | 140.12 | 167.3 | 20.19 |
π=7 | 455.9 | 226.47 | 185.02 | 229.43 | 41.45 |
π=8 | 658.09 | 342.57 | 273.22 | 315.52 | 69.35 |
π=9 | 891.23 | 515.96 | 406.38 | 375.27 | 109.58 |
π=10 | 1137.96 | 738.41 | 559.67 | 399.55 | 178.74 |
π=11 | 1530.54 | 986.65 | 775.72 | 543.89 | 210.93 |
Sample mean | 745.30 | 441.17 | 351.14 | 304.13 | 90.03 |
Sample standard deviation | 472.28 | 324.23 | 244.76 | 151.99 | 80.25 |
Arrival rate | The average total cost per machine tool | Improvement of the standard method over the baseline onetc1–tc2 | Improvement of the proposed method over the standard onetc2–tc3 | ||
Baseline casetc1 | Standard SA/MSTtc2 | Proposed SA/MSTtc3 | |||
π=5 | 270.84 | 117.32 | 117.32 | 153.52 | 0 |
π=6 | 463.08 | 224.8 | 186.58 | 238.28 | 38.22 |
π=7 | 687.42 | 416.46 | 275.13 | 270.96 | 141.33 |
π=8 | 1063.8 | 655.68 | 453.47 | 408.12 | 202.21 |
π=9 | 1495.67 | 957.36 | 706.49 | 538.31 | 250.87 |
π=10 | 1917.52 | 1231.56 | 918.76 | 685.96 | 312.8 |
π=11 | 2403.39 | 1483.89 | 1120.77 | 919.5 | 363.12 |
Sample mean | 1185.96 | 726.72 | 539.79 | 459.24 | 186.94 |
Sample standard deviation | 789.17 | 517.36 | 385.07 | 273.81 | 135.61 |
Computation time breaks | Standard SA/MST | Proposed SA/MST | ||||||
tc1 | pc1 | dc1 | wt1 | tc2 | pc2 | dc2 | wt2 | |
After 0sec | 399.25 | 112.16 | 287.09 | 76.80 | 399.25 | 112.16 | 287.09 | 76.80 |
After 30sec | 380.38 | 116.78 | 263.60 | 72.37 | 371.14 | 114.62 | 256.51 | 55.21 |
After 60sec | 393.70 | 113.71 | 279.99 | 61.76 | 341.42 | 118.56 | 222.86 | 48.34 |
After 90sec | 393.70 | 113.71 | 279.99 | 58.90 | 311.93 | 120.51 | 191.43 | 46.49 |
After 120sec | 386.11 | 115.62 | 270.49 | 58.75 | 292.79 | 121.75 | 171.04 | 44.54 |
After 150sec | 385.56 | 119.96 | 265.60 | 58.42 | 280.06 | 123.28 | 156.78 | 41.52 |
After 180sec | 384.17 | 121.18 | 262.99 | 57.90 | 274.14 | 124.64 | 149.51 | 41.47 |
After 210sec | 383.35 | 122.86 | 260.49 | 56.51 | 273.33 | 126.31 | 147.03 | 38.40 |
After 240sec | 378.22 | 124.65 | 252.57 | 54.73 | 273.22 | 132.79 | 140.42 | 35.67 |
No time limits* | 342.57 | 129.41 | 213.16 | 48.07 | 273.22 | 132.79 | 140.42 | 35.67 |
* "No time limit" means the simulation keeps the algorithm running until it meets the stopping criteria elaborated above. |
Places and transitions in CTPN | Description |
s0 | The output buffer of the inspection center |
si (i=1, 2, ..., 12) | The input buffer of each recovery workstation |
sz (z=13, 14, 15, 16) | The output buffer of each recovery workstation |
s17 | The input buffer of the reassembly center |
wk (k=1, 2, ..., 12) | Workstation places |
ti, j (i, j=1, 2, ..., 12, i≠j) | Recovery operations |
t0, i, ti, z, ti,17 (i=1, 2, ..., 12, z=13, 14, 15, 16) | Transportation transitions |
Index | Description |
L= {1, 2, ..., L} | index set of used products |
D= {1, 2, ..., D} | index set of component types |
K= {1, 2, ..., K} | index set of workstations |
H= {1, 2, ..., H} | index set of recovery process routings |
Parameters | Description |
ϑld | the dth core disassembled from the lth product |
wk | the kth workstation that performs a specific recovery operation |
Rh | the hth recovery process routing designed for worn cores and is represented as a set of recovery operations |
pre(tij, Rh) | the operational stages of recovery operation tij in Rh |
Last(Rh) | the last recovery operation in Rh |
aldh | a binary variable with a value 1 indicates the hth routing for the core ϑld |
u(tij, ϑld) | the time for a core ϑld to be processed in transition tij |
AT(si, ϑld) | the arrival time of the core ϑld at buffer place si |
ST(wk, ϑld) | the start time of the core ϑld being processed by wk |
Com(wk, ϑld) | the completion time of the core ϑld processed by wk |
ρ(ϑld) | the makespan of the component ϑld |
χ(ϑld) | the tardiness of the component ϑld |
\zeta (l) | the tardiness of the lth product |
Probld | the probability of a core ϑld being selected for routing reassignment |
\Pi _{ld}^{h, x} | the difference of the total average waiting time between the hth routing and the xth one for the core ϑld |
WTk | the average waiting time per core consumed in the kth workstation |
h | the total average waiting time per core consumed in the hth routing |
v_{ld}^{h, x} | the probability of the xth routing being selected to replace the current hth routing for the core ϑld |
Slackxy | slack time of a core ϑxy in scheduling horizon |
Decision variables | Description |
rldh | a binary variable with a value 1 indicates that the hth process routing is assigned to a core ϑld |
Pri(wk, ϑld) | the priority for the dth component of the lthused product to be processed by the kth workstation |
Arrival rate | The average total cost per machine tool | Improvement of the standard method over the baseline one tc1–tc2 | Improvement of the proposed method over the standard one tc2–tc3 | ||
Baseline casetc1 | Standard SA/MSTtc2 | Proposed SA/MSTtc3 | |||
π=5 | 186.66 | 117.02 | 117.02 | 69.64 | 0 |
π=6 | 228.37 | 133.48 | 118.21 | 94.89 | 15.27 |
π=7 | 293.05 | 172.45 | 146.89 | 120.6 | 25.56 |
π=8 | 335.87 | 208.06 | 168.69 | 127.81 | 39.37 |
π=9 | 447.52 | 266.47 | 216.25 | 181.05 | 50.22 |
π=10 | 581.63 | 352.28 | 270.91 | 229.35 | 81.37 |
π=11 | 714.29 | 461.86 | 335.77 | 252.43 | 126.09 |
Sample mean | 398.20 | 244.52 | 196.25 | 153.68 | 48.27 |
Sample standard deviation | 193.44 | 125.46 | 82.67 | 68.93 | 43.15 |
Arrival rate | The average total cost per machine tool | Improvement of the standard method over the baseline onetc1–tc2 | Improvement of the proposed method over the standard onetc2–tc3 | ||
Baseline casetc1 | Standard SA/MSTtc2 | Proposed SA/MSTtc3 | |||
π=5 | 215.76 | 117.83 | 117.83 | 97.93 | 0 |
π=6 | 327.61 | 160.31 | 140.12 | 167.3 | 20.19 |
π=7 | 455.9 | 226.47 | 185.02 | 229.43 | 41.45 |
π=8 | 658.09 | 342.57 | 273.22 | 315.52 | 69.35 |
π=9 | 891.23 | 515.96 | 406.38 | 375.27 | 109.58 |
π=10 | 1137.96 | 738.41 | 559.67 | 399.55 | 178.74 |
π=11 | 1530.54 | 986.65 | 775.72 | 543.89 | 210.93 |
Sample mean | 745.30 | 441.17 | 351.14 | 304.13 | 90.03 |
Sample standard deviation | 472.28 | 324.23 | 244.76 | 151.99 | 80.25 |
Arrival rate | The average total cost per machine tool | Improvement of the standard method over the baseline onetc1–tc2 | Improvement of the proposed method over the standard onetc2–tc3 | ||
Baseline casetc1 | Standard SA/MSTtc2 | Proposed SA/MSTtc3 | |||
π=5 | 270.84 | 117.32 | 117.32 | 153.52 | 0 |
π=6 | 463.08 | 224.8 | 186.58 | 238.28 | 38.22 |
π=7 | 687.42 | 416.46 | 275.13 | 270.96 | 141.33 |
π=8 | 1063.8 | 655.68 | 453.47 | 408.12 | 202.21 |
π=9 | 1495.67 | 957.36 | 706.49 | 538.31 | 250.87 |
π=10 | 1917.52 | 1231.56 | 918.76 | 685.96 | 312.8 |
π=11 | 2403.39 | 1483.89 | 1120.77 | 919.5 | 363.12 |
Sample mean | 1185.96 | 726.72 | 539.79 | 459.24 | 186.94 |
Sample standard deviation | 789.17 | 517.36 | 385.07 | 273.81 | 135.61 |
Computation time breaks | Standard SA/MST | Proposed SA/MST | ||||||
tc1 | pc1 | dc1 | wt1 | tc2 | pc2 | dc2 | wt2 | |
After 0sec | 399.25 | 112.16 | 287.09 | 76.80 | 399.25 | 112.16 | 287.09 | 76.80 |
After 30sec | 380.38 | 116.78 | 263.60 | 72.37 | 371.14 | 114.62 | 256.51 | 55.21 |
After 60sec | 393.70 | 113.71 | 279.99 | 61.76 | 341.42 | 118.56 | 222.86 | 48.34 |
After 90sec | 393.70 | 113.71 | 279.99 | 58.90 | 311.93 | 120.51 | 191.43 | 46.49 |
After 120sec | 386.11 | 115.62 | 270.49 | 58.75 | 292.79 | 121.75 | 171.04 | 44.54 |
After 150sec | 385.56 | 119.96 | 265.60 | 58.42 | 280.06 | 123.28 | 156.78 | 41.52 |
After 180sec | 384.17 | 121.18 | 262.99 | 57.90 | 274.14 | 124.64 | 149.51 | 41.47 |
After 210sec | 383.35 | 122.86 | 260.49 | 56.51 | 273.33 | 126.31 | 147.03 | 38.40 |
After 240sec | 378.22 | 124.65 | 252.57 | 54.73 | 273.22 | 132.79 | 140.42 | 35.67 |
No time limits* | 342.57 | 129.41 | 213.16 | 48.07 | 273.22 | 132.79 | 140.42 | 35.67 |
* "No time limit" means the simulation keeps the algorithm running until it meets the stopping criteria elaborated above. |