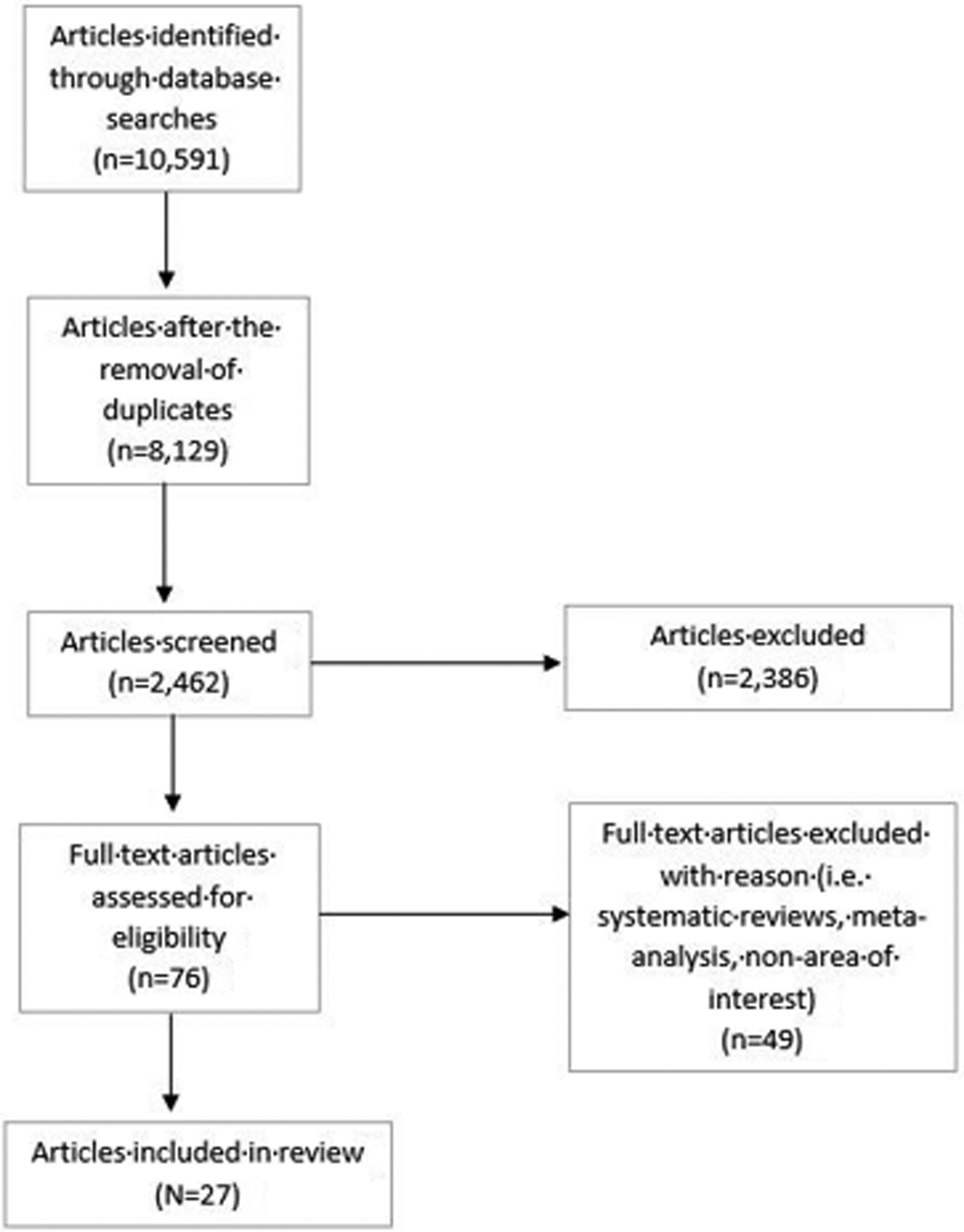
The fractional Bagley-Torvik system (FBTS) is initially created by utilizing fractional calculus to study the demeanor of real materials. It can be described as the dynamics of an inflexible plate dipped in a Newtonian fluid. In the present article, we aim for the first time to discuss the existence and uniqueness (E&U) theories of an unbounded solution for the proposed generalized FBTS involving Riemann-Liouville fractional derivatives in the half-line , by using fixed point theorems (FPTs). Moreover, the Hyers-Ulam stability (HUS), Hyers-Ulam-Rassias stability (HURS), and semi-Hyers-Ulam-Rassias stability (sHURS) are proved. Finally, two numerical examples are given for checking the validity of major findings. By investigating unbounded solutions for the FBTS, engineers gain a deeper understanding of the underlying physics, optimize performance, improve system design, and ensure the stability of the motion of real materials in a Newtonian fluid.
Citation: Sabri T. M. Thabet, Imed Kedim, Miguel Vivas-Cortez. Efficient results on unbounded solutions of fractional Bagley-Torvik system on the half-line[J]. AIMS Mathematics, 2024, 9(2): 5071-5087. doi: 10.3934/math.2024246
[1] | Anissa Guillemin, Michael P. H. Stumpf . Non-equilibrium statistical physics, transitory epigenetic landscapes, and cell fate decision dynamics. Mathematical Biosciences and Engineering, 2020, 17(6): 7916-7930. doi: 10.3934/mbe.2020402 |
[2] | Cristina De Ambrosi, Annalisa Barla, Lorenzo Tortolina, Nicoletta Castagnino, Raffaele Pesenti, Alessandro Verri, Alberto Ballestrero, Franco Patrone, Silvio Parodi . Parameter space exploration within dynamic simulations of signaling networks. Mathematical Biosciences and Engineering, 2013, 10(1): 103-120. doi: 10.3934/mbe.2013.10.103 |
[3] | Eugene Kashdan, Dominique Duncan, Andrew Parnell, Heinz Schättler . Mathematical methods in systems biology. Mathematical Biosciences and Engineering, 2016, 13(6): i-ii. doi: 10.3934/mbe.201606i |
[4] | Juexin Wang, Yan Wang . Towards Machine Learning in Molecular Biology. Mathematical Biosciences and Engineering, 2020, 17(4): 2822-2824. doi: 10.3934/mbe.2020156 |
[5] | Hilla Behar, Alexandra Agranovich, Yoram Louzoun . Diffusion rate determines balance between extinction and proliferationin birth-death processes. Mathematical Biosciences and Engineering, 2013, 10(3): 523-550. doi: 10.3934/mbe.2013.10.523 |
[6] | Janine Egert, Clemens Kreutz . Realistic simulation of time-course measurements in systems biology. Mathematical Biosciences and Engineering, 2023, 20(6): 10570-10589. doi: 10.3934/mbe.2023467 |
[7] | Gerardo Aquino, Andrea Rocco . Bimodality in gene expression without feedback: from Gaussian white noise to log-normal coloured noise. Mathematical Biosciences and Engineering, 2020, 17(6): 6993-7017. doi: 10.3934/mbe.2020361 |
[8] | Adam Peddle, William Lee, Tuoi Vo . Modelling chemistry and biology after implantation of a drug-eluting stent. Part Ⅱ: Cell proliferation. Mathematical Biosciences and Engineering, 2018, 15(5): 1117-1135. doi: 10.3934/mbe.2018050 |
[9] | Heyrim Cho, Ya-Huei Kuo, Russell C. Rockne . Comparison of cell state models derived from single-cell RNA sequencing data: graph versus multi-dimensional space. Mathematical Biosciences and Engineering, 2022, 19(8): 8505-8536. doi: 10.3934/mbe.2022395 |
[10] | Hal L. Smith . Tribute to Horst R. Thieme on the occasion of his 60th birthday. Mathematical Biosciences and Engineering, 2010, 7(1): i-iii. doi: 10.3934/mbe.2010.7.1i |
The fractional Bagley-Torvik system (FBTS) is initially created by utilizing fractional calculus to study the demeanor of real materials. It can be described as the dynamics of an inflexible plate dipped in a Newtonian fluid. In the present article, we aim for the first time to discuss the existence and uniqueness (E&U) theories of an unbounded solution for the proposed generalized FBTS involving Riemann-Liouville fractional derivatives in the half-line , by using fixed point theorems (FPTs). Moreover, the Hyers-Ulam stability (HUS), Hyers-Ulam-Rassias stability (HURS), and semi-Hyers-Ulam-Rassias stability (sHURS) are proved. Finally, two numerical examples are given for checking the validity of major findings. By investigating unbounded solutions for the FBTS, engineers gain a deeper understanding of the underlying physics, optimize performance, improve system design, and ensure the stability of the motion of real materials in a Newtonian fluid.
It has been estimated that by 2050 older adults (≥ 60 years old) will comprise 22% of the world's population [1]. Older adults face significant challenges related to their social, emotional, and physical health as well as other challenges such as ageism and life transitions (e.g., retirement and grandparent status) not experienced by younger people. Accelerometer data for older adults in the United States indicate that they engage in greater amounts of sedentary behavior than any other age group [2]. Globally, similar results have been reported (in more than 60 countries), whereby older adults are more likely to report sitting for four or more hours per day greater than any other age group [3].
Despite the findings that older adults report more sedentary behavior than other age group, they are underrepresented in studies examining health outcomes associated with sedentary behavior. The most recent Physical Activity Guidelines Report found limited data about the relationship between sedentary behavior, age, and mortality outcomes [4]. Furthermore, there was insufficient evidence about sedentary behavior and age related to cardiovascular disease, type 2 diabetes, and cancer [4]. Therefore, more studies are needed to clarify the relationship between sedentary behaviors and health outcomes in older adults.
In the general population, sedentary behavior has been identified as a risk factor independent of physical activity [5]–[8]. A central concern is the extent to which sedentary behavior affects the well-being of older adults. To address this issue, there is a need for studies that systematically and comprehensively review the literature. In a narrative review, measurement of sedentary behavior was evaluated, specific associations of sedentary time with geriatric-relevant health outcomes were presented, and interventions that target reducing sedentary time in older adults were analyzed; six health outcomes were reviewed [9]. To our knowledge, there has been only one comprehensive, systematic review of sedentary behavior and multiple health outcomes in older adults. In 2014, de Rezende et al [10] reported the associations between sedentary behaviors and twelve health outcomes in older adults: mortality, metabolic syndrome, cardiometabolic biomarkers, triglycerides, HDL cholesterol, blood pressure, plasma glucose/Hb1Ac/glucose intolerance, cholesterol ratio and total, other cardiometabolic biomarkers, waist circumference/waist-to-hip ratio/abdominal obesity, overweight/obesity, and cancer [10]. While this review was comprehensive, in the past six years, additional studies have been published; an update of this review is needed to advance the field. Therefore, the objectives of this systematic review were to: (1) assess the relationship among sedentary behaviors and twelve health outcomes in older adults; (2) compare our findings to previous reviews; and (3) present recommendations to advance the field.
Sedentary behavior is operationalized as any activity engaged in while sitting including leisure and occupational time. In addition, sedentary behavior is defined as any waking behavior accounting for energy in the range of 1.0 to 1.5 metabolic equivalents while in a sitting or reclining position [4],[11]. Sedentary behaviors include television viewing and computer use [11].
In our study, there was no contact with human participants; therefore, this research was exempt from Institutional Review Board approval.
We updated the 2014 review [10], which identified articles up to May of 2013. Therefore, we searched articles from 2013 to June 2019 (six years). Similar to the search strategy of the prior review [10], the following databases were used with the assistance of a professional librarian: Medline, Web of Science, PsycINFO, and Cumulative Index to Nursing and Allied Health Literature (CINAHL). Because of access issues, we did not search the following databases identified in the 2014 review: Excerpta Medica (EMBASE), SPORTDiscus, Literatura Latino-Americana e do Caribe em Ciências da Saúde (LILLACS), and Sedentary Behavior Research Database (SBRD). However, to extend and expand our search, we added the following databases not included in the prior review: PubMed, Google Scholar, Scopus, and Academic Search Premier. We chose outcomes that were most relevant for people 60 years and older which included the outcomes chosen in the previous review [10]. Our objective was to capture “geriatric-relevant health outcomes” [9].
As in the previous review [10], the same search keywords were used: “exposures (sedentary behavior, sedentary lifestyles, sitting time, television reviewing, driving, screen-time, video game, and computer); primary outcomes (mortality, cardiovascular disease, cancer, type 2 diabetes mellitus); and secondary outcomes (accidental falls, frail elderly, obesity, metabolic syndrome, mental disorders, musculoskeletal diseases)” . Studies that were excluded: review articles, studies with adults younger than 60 years of age, and descriptive data only studies. In two subsections, there were no recent empirical data. To fill this gap and to provide a context, two systematic reviews/meta-analyses were cited as exceptions to our exclusion criteria.
Data from eligible articles were extracted by one author and reviewed independently by a second author. The tables present: Author(s), Year, Title, Journal, Hypotheses/Study Aims, Research Design, Sample Size, Ages, Country, Measures, Primary Findings, Limitations, and Strengths.
Because of its credibility and reputation of having “state of art” and “gold standard” assessments, we rated each article based on the U.S. Department of Health & Human Services, National Institutes of Health, Study Quality Assessment Tools [12]. Each research design had a specific assessment tool to evaluate quality assessment. The research designs were controlled intervention studies (14 questions), systematic reviews and meta-analyses (8 questions), observational cohort and cross-sectional studies (14 questions), case-control studies (12 questions), before-after (pre-post) studies with no control group (12 questions), and case series studies (9 questions). For each category, the response choices were yes, no, or other (e.g., CD, cannot determine; NA, not applicable; NR, not reported). To ensure accurate reporting, each design was accompanied by a document called “guidance for assessing the quality”. This document provided guidelines, rules, and tips to answer specific questions for each research design. The final quality ratings were Good, Fair, or Poor. One of the coauthors rated each study independently. All coauthors rated the same study for consensus if there were concerns or ambiguity about any ratings.
As described in Figure 1, the search started with 10,591 potentially relevant articles. After screening articles for duplicates, review articles, meta-analyses, not relevant areas of interest, and other reasons, 27 articles were reviewed and described. Articles are presented in Tables 1 to 9. In the 2014 review, 23 articles met the inclusion criteria [10].
All-cause mortality is the most severe outcome associated with sedentary behavior. Across all studies, there was a consistent association between sedentary behavior and mortality [13]. Six prospective cohort studies were identified. In one prospective cohort of Spanish older adults, those who were consistently non-sedentary had a lower hazard ratio (HR = 0.74, 95% confidence ratio (CI) = 0.62–0.90) for mortality than those who were consistently sedentary [14]. Studies conducted in both older men and women yielded similar results with respect to mortality risk. Ensrud et al. [15] found that older men who were in the highest quartile of sedentary behavior had a higher risk for all-cause mortality (HR = 1.51, 95% CI: 1.10–2.08) when compared to those in the lowest quartile. Similarly, in a sample of Australian women, there was a dose response relationship between sitting and those who were not meeting physical activity guidelines [16]. In particular, the risk for mortality increased for non-active women who reported 8 or more hours of sedentary time (i.e., for 8 to 11 hours OR = 1.31 and for ≥ 11 hours OR = 1.47) when compared to those who sat less than 4 hours per day (p < 0.05). Similarly, in a sample of British men, each additional 30 minutes of sedentary behavior increased the risk for mortality (HR = 1.17 95% CI; 1.10 to 1.25 p < 0.05) [17]. Conversely, Klenk et al. [18] found that the association between sedentary behavior and mortality diminished after adjustments for several biomarkers (HR = 2.05 95% CI: 1.13, 3.73 p < 0.05). Furthermore, in a sample of older adults from Spain, being in the highest quartile for sedentary behavior increased the risk for mortality due to inflammatory (HR = 1.49 95% CI: 1.08, 2.06) and non-infectious inflammatory causes (HR = 1.59 95% CI: 1.08, 2.33) [19]. However, these models became non-significant when adjusting for physical activity. In examining patterns of physical activity and sedentary behavior together, high levels of sedentary behavior (> 7 hours per day), regardless of physical activity levels were associated with mortality from inflammatory (HR = 1.68 95% CI: 1.19, 2.37) and non-infectious inflammatory causes [19].
In our review, we identified six prospective cohort studies and Rezende et al. 2014 [10] described four prospective cohort studies. The findings are remarkably similar. For older adults, sedentary behavior (particularly sitting) six or more hours per day was consistently related to all-cause mortality. In the studies, the thresholds for sedentary behavior/sitting time ranged from 6, 8, 8 to 11, and 11 or more hours per day.
Reference Author(s), Year, Title, Journal |
Hypotheses/Study Aims | Research Design, Sample Size, Ages, Country | Measures | Primary Findings | Limitations | Strengths |
Cabanas-Sanchez, Guallar-Castillon et al., (2018); Physical activity, sitting time and mortality from inflammatory disease in older adults. Frontiers in Physiology | Study focused on infectious and non-infectious inflammatory causes. | Prospective Cohort study (N = 3,667) Men and women ages 60 years and older in Spain |
Sedentary data were self-reported. Mortality identified using the National Death Index. |
In nonlinear models, those who were sedentary > 7 hours per day had a higher mortality risk for total inflammatory and non-infectious inflammatory causes. Relationships were stronger for those who were the least active. | Sedentary time and physical activity were self-reported. No dietary data were collected. Inflammatory disease definition may not encompass all related conditions. |
Prospective cohort study Spanish population National database to confirm deaths Focus on mortality due to inflammatory and non-inflammatory causes |
Ensrud, Blackwell, Cauley et al., (2014); Objective measures of activity level and mortality in older men. Journal of the American Geriatrics Society | Study focused on developing objective measures of activity level for older adult men, related to all-cause mortality. | Prospective Cohort study (N = 2,918) Men ages 71 years and older in the United States |
Accelerometer determined sedentary behavior (ACTi Graph) Deaths confirmed via death certificate and follow-up calls. |
In adjusted models, men in the highest quartile for sedentary time had a higher risk for all-cause mortality. Subsequent analysis indicated that time spent sedentary was associated with non-cancer and non-CVD deaths. | Associations for women or racial and ethnic minority populations not determined | Objective assessment of behavior Cohort study Disease specific mortality |
Jefferis, Parsons, Sartini et al., (2018); Objectively measured physical activity, sedentary behavior and all-cause mortality in older men: Does volume of activity matter more than pattern of accumulation? Br J Sports Med | To examine the association between accelerometer determined sedentary behavior, physical activity, and all-cause mortality | Prospective Cohort study (N = 1,655) Men ages 71–92years old in the United Kingdom |
Accelerometer determined sedentary behavior (ACTi Graph) National database confirmed mortality |
In adjusted models, those in the highest quartile for sedentary time had a higher risk for all-cause mortality when compared to men in the lowest quartile. | No measure of postural allocation Associations for women or racial and ethnic minority populations not determined |
Prospective cohort study. An objective measure of sedentary behavior |
Klenk, Dallmeier, Denkinger et al., (2016); Objectively measured walking duration and sedentary behavior and four-year mortality in older people. Plos ONE | This study examined the effect of walking and sedentary time on mortality. | Randomly and geographically selected cohort of older adults in Germany (N = 1,271) aged 65 years or greater. | Physical activity levels were accelerometer determined sedentary behavior (ActivPAL) Mortality determined via local registration offices |
Sedentary duration association between sedentary time and mortality diminished in fully adjusted models. | Limited follow-up period Slower walking speeds may have different outcomes |
Use of ActivePAL to measure sedentary behavior |
Leon-Munoz, Martinez-Gomez, Balboa-Castillo et al., (2013); Continued sedentariness, change in sitting time, and mortality in older adults. Med Sci Sports Exerc | To examine the association between continued sedentary time and changes in sedentary time with mortality | Prospective Cohort (N = 2,635) of adults aged 60 years and older. | Self-reported sedentary time in different activities National Death Index confirmed deaths |
Those who were consistently sedentary had higher mortality rates when compared to those who were newly sedentary, formally sedentary, and consistently non-sedentary. Associations maintained in different SES strata. | Self-reported questionnaires Other factors may be related to mortality | Prospective cohort study Mortality confirmed via National Death Index. Analysis in different SES strata |
Pavey, Peeters, Brown (2015); Sitting-time and 9-year all-cause mortality in older women. Br J Sports Med | This study looked at the relationship between total sitting-time and all-cause mortality in older women. | Prospective cohort study (N = 6,656) of Australian Women ages 76–90. | Self-reported questionnaires Mortality data confirmed by the Australian National Death Index. |
In fully adjusted models there was a dose-response relationship between time spent sitting and mortality. However, only the p-value for the trend was significant. | Other factors may be related to mortality. Self-reported questionnaires |
Confirmed morality data Prospective cohort design |
Metabolic syndrome increases the risk of future chronic health conditions and premature mortality. Evidence from cross-sectional studies, longitudinal studies, and randomized controlled trials suggest that sedentary time, moderate-to-vigorous physical activity, and cardio-respiratory fitness are important predictors of various cardio-metabolic risk factors [20]. The main finding was that total daily time spent in sedentary behaviors was associated with increased odds of having metabolic syndrome without accounting for effect modifiers [20]. Evidence showed a 9% increase in the odds of having metabolic syndrome with each additional hour of sitting time. Findings showed a 58% (OR 1.58; 95% CI 1.01, 2.48) increased odds of metabolic syndrome when comparing the highest (> 9.49 h) and the lowest (< 6.70 h) quartiles [20].
In our review, we identified two studies published in 2015 related to sedentary behavior and metabolic syndrome. Rezende et al. described four studies [10]. The findings indicated that sedentary behavior is adversely and consistently related to metabolic syndrome.
One study found statistically significant interactions between sedentary behavior and moderate-to-vigorous intensity physical activity for triglycerides (p = 0.04), HDL-cholesterol (p = 0.01), fasting blood glucose. In addition, there were increased odds of having low HDL-cholesterol with each hour of sedentary behavior time for those who accumulated 150–300 and ≥ 300 min/week of moderate-to-vigorous intensity physical activity, respectively [21]. Similarly, increased odds of 13% were found (OR 1.13; 95% CI 1.01, 1.26) for having high fasting blood glucose with each hour of sedentary time for those who accumulated ≥ 300 min/week of moderate-to-vigorous intensity physical activity [21]. Each minute spent in sedentary behavior was significantly associated with reductions of 0.03 mmHg (95%CI: −0.05; −0.01) in systolic blood pressure and reductions of 0.02 mg/dL (95%CI: −0.02; −0.01) in HDL-cholesterol [22]. Beneficial associations of moderate-to-vigorous intensity physical activity and HDL (β = 0.10; 95%CI: 0.01; 0.18) and plasma glucose (β = −0.18; 95%CI: −0.33; −0.02) were observed [22]. Similarly, moderate-to-vigorous intensity physical activity was associated with higher levels of HDL (β = −0.35; 95%CI: 0.14; 0.54), but it was not related to the other cardiovascular risk markers [22]. In a randomized, sedentary behavior reduction trial, Aadahl et al. [23] found that the intervention condition experienced greater improvements in insulin (−5.9 pmol/L, p = 0.03), insulin resistance (−0.28, p = 0.03), and waist circumference (−1.42 cm, p = 0.01) when compared to the control condition participants.
Reference Author(s), Year, Title, Journal |
Hypotheses/Study Aims | Research Design, Sample Size, Ages, Country | Measures | Primary Findings | Limitations | Strengths |
Ekblom, Ö., Ekblom-Bak, E., Rosengren, A., Hallsten, M., Bergström, G., Börjesson, M., & Lazzeri, C. (2015); Cardiorespiratory fitness, sedentary behavior and physical activity are independently associated with the metabolic syndrome, Results from the SCAPIS Pilot Study. PLoS ONE | Examine the association between sedentary behavior and metabolic syndrome | Random stratified sample of adults aged 50 to 65 years living in Sweden (N = 1111) | Accelerometer determined sedentary behavior Serum samples used to detect metabolic syndrome |
In adjusted models, the highest quartile for sedentary time had higher odds for metabolic syndrome when compared to the first quartile. Sedentary time increased the odds of a larger waist circumference and higher triglyceride, and HDL cholesterol levels. | Cross-sectional design Possibility of multi-collinearity |
Adjusted for dietary intake and fitness Accelerometer determined sedentary behavior Random stratified sample |
Joseph, J., Echouffo-Tcheugui, J., Golden, S., Chen, H., Jenny, N., Carnethon, M., Bertoni, A. (2016); Physical activity, sedentary behaviors and the incidence of type 2 diabetes mellitus: The Multi-Ethnic Study of Atherosclerosis (MESA). BMJ Open Diabetes Research & Care | Examine the association between sedentary behavior and diabetes | Prospective cohort (N = 5829) of men and women from the United States aged 45–84 years old | Incident type II diabetes determined by fasting glucose Self-reported sedentary time |
In adjusted models, both television time and total sedentary behavior increased the odds for type II diabetes. Possible effect modification by family history of diabetes, race, and BMI were observed. | Physical activity and sedentary behaviors were self-reported, and the time frame queried was their typical activities within the last month. Therefore, discrepancies with actual activity levels may exist and self-reported sedentary behavior | Multiethnic population Serum-based biomarkers |
Wirth, K., Klenk, J., Brefka, S., Dallmeier, D., Faehling, K., Roqué, M., Denkinger, M. (2017); Biomarkers associated with sedentary behavior in older adults: A systematic review. Ageing Research Reviews | Patho-mechanisms of sedentary behavior (SB) are unclear. We conducted a systematic review to investigate the associations between SB and various biomarkers in older adults. | Electronic databases were searched (MEDLINE, EMBASE, CINAHL, AMED) up to July 2015 to identify studies with objective or subjective measures of SB, sample size ≥ 50, mean age ≥ 60years and accelerometer wear time ≥ 3days. Methodological quality was appraised with the CASP tool. | Randomized controlled trials found a positive correlation for SB with BMI, neck circumference, fat mass, HbA1C, cholesterol and insulin levels, cohort studies additionally for waist circumference, leptin, C-peptide, and low-density lipoprotein and a negative correlation for HDL. | The final sample (26 articles) 63 biomarkers were detected. Most investigated markers were body mass index (BMI, n = 15), waist circumference (WC, n = 15), blood pressure (n = 11), triglycerides (n = 12) and high-density lipoprotein (HDL, n = 15). Some inflammation markers were identified such as interleukin-6, C-reactive protein or tumor necrosis factor alpha. There was a lack of renal, muscle or bone biomarkers. | First, we identified relatively few high quality or longitudinal studies investigating biomarkers specifically in older adults. Therefore, we were not able to conduct a meta-analysis as we anticipated. | The highlighted results of the four “risk population” studies showed associations for SB with biomarkers in the same direction as the studies performed in non-risk populations. |
Figueiró, T. H., Arins, G., Santos, C., Cembranel, F., Medeiros, P. A., d'Orsi, E., & Rech, C. R. (2019); Association of objectively measured sedentary behavior and physical activity with cardiometabolic risk markers in older adults. Plos ONE | Examine the association between sedentary behavior and cardiometabolic markers | Cross-sectional study of Brazilians adults (N = 425) aged 60 years and older | Accelerometer determined sedentary behavior Waist circumference, blood pressure, and plasma-based cardio-metabolic markers |
Sedentary behavior negatively and linearly associated with systolic blood pressure and HDL cholesterol. Physically inactive and sedentary individuals had greater waist circumference and lower HDL cholesterol levels than active, non-sedentary subjects. | Cross-sectional study design Confounding variables (i.e., medications and BMI) not included in the analysis |
Objectively measured sedentary time Plasma-based biomarkers |
Aadahl, M., Linneberg, A., Møller, T., Rosenørn, S., Dunstan, D., Witte, D., & Jørgensen, T. (2014); Motivational counseling to reduce sitting time: A community-based randomized controlled trial in adults. American Journal of Preventive Medicine | Examine the effectiveness of a sedentary behavior intervention | Randomized trial of 166 adults aged adults aged 18–69 years old from Denmark | Objectively measured overall sitting time; secondary measures were breaks in sitting time; anthropometric Accelerometer determined sedentary behavior (ActivPAL Body fat percentage determined by Tanita BC-420MA Serum measures of insulin homeostasis model assessment, hepatic insulin resistance, and basil insulin secretion, glycosylated hemoglobin, total cholesterol, LDL and HDL cholesterol, and triglycerides |
The reduction in sedentary time did not differ between groups. The intervention group had greater improvements in standing time than the control group. Greater improvements in insulin resistance was observed in the intervention condition when compared to the control group. No other differences were observed. | No dietary information was included Uneven sample size in the intervention and control condition. |
Accelerometer determined sedentary behavior Serum biological markers Randomized design |
We identified three relevant studies and, in our review, with the exception of systolic blood pressure, sedentary behavior/sitting was associated with unfavorable biometric indices. In the 2014 review, for two of the three studies, the associations between time spent in sedentary behavior and high triglycerides was not statistically significant. Overall, the literature in this area is not consistent.
In a cross-sectional descriptive study (with 306 elderly Koreans with hypertension), levels and predictors of sedentary behavior were examined [24]. The participants spent an average of 8.59 hours per day in sedentary behavior. The analysis showed that sedentary behavior was significantly predicted by a longer duration since hypertension diagnosis, greater levels of depressive symptoms, and lower levels of perceived health, vigorous-intensity physical activity, and empowerment (i.e., a composite score of sense of control, self-efficacy, problem solving, psychosocial coping, support, motivation, and decision making). These variables explained 42.6% of the variance in sedentary behavior [24].
In our review, we found one study related to blood pressure. However, blood pressure was not analyzed as a predictor or consequence of sedentary behavior. In the prior review, two of the three publications reported that sedentary time was associated with high blood pressure [10]. This area of research is emerging and more definitive studies are needed.
Sedentary behavior has been associated with glucose levels and insulin secretions. Lifestyle interventions designed to reduce sedentary behaviors such as television watching have the potential to improve glucose metabolism. Joseph et al. [25] found that both sedentary behavior (< 2 versus > 6 hours/day: OR = 1.65, 95% CI = 1.26, 2.14) and television viewing (< 2 versus > 6 hours/day: OR = 2.68, 95% CI = 1.38, 5.21) were associated with risk of type II diabetes in adjusted models. The association remained after adjusting for BMI (OR = 1.39, 95% CI = 1.06, 1.81). Some of these relationships differed by racial and ethnic identity.
Since 2013, we found one study that examined the association between sedentary behavior and type 2 diabetes. A positive association was reported between type 2 diabetes risk, leisure sedentary behavior, and television viewing, which may be more pronounced in non-Hispanics whites. In the prior review [10], four studies were found. One study reported a positive association between television viewing, sedentary time and plasma glucose; in another study, a positive relationship was found in women only; in two studies, the findings were not statistically significant [10]. Overall, the findings are mixed and inconsistent related to sedentary behavior, HBA1C/Glucose Intolerance, and diabetes. Clearly, more research is needed.
Reference Author(s), Year, Title, Journal |
Hypotheses/Study Aims | Research Design, Sample Size, Ages, Country | Measures | Primary Findings | Limitations | Strengths |
Chang & Sok (2015); Predictors of sedentary behavior in elderly Koreans with hypertension, The Journal of Nursing Research | Examine predictors of sedentary behavior | Cross-sectional study (N = 306) of Korean adults aged 65 years and older | Self-reported sedentary behavior Psychosocial variables (i.e., empowerment, perceived health, depression, self-efficacy, and social support for physical activity) |
Stepwise regression analysis indicated that empowerment, duration since HBP diagnosis, perceived health, vigorous-intensity physical activity, and depressive symptoms were determinants of sedentary behavior. | Self-reported measures of sedentary behavior Cross-sectional design |
Validated measures |
Our review found one study with no statistically significant results. Rezende et al. 2014 reported three studies; two studies reported positive results between television viewing, high total-to-HDL cholesterol ratio and leisure-time sedentary behavior, television viewing, and cholesterol ratio [10]. A third study found no statistically significant findings between sedentary behavior and total cholesterol. Overall, two studies including the most recent one (2019) found no association and two earlier studies (2007 and 2012) found positive associations. To clarify the relationships between cholesterol and sedentary behaviors in this emerging area, more definitive research is recommended.
There is a scarcity of studies investigating inflammatory biomarkers and sedentary behavior. Therefore, we included one review article as an exception to our exclusion criteria. Wirth and colleagues (2017) [26] defined sedentary activity as waking behavior with an energy expenditure ≤ 1.5 METS while in a sitting or reclining posture. C-reactive protein, which is a protein made by your liver that is sent into your bloodstream in response to inflammation, was investigated most frequently; although, restricted to four cross-sectional studies and one randomized controlled trial, only two cross-sectional studies demonstrated that sedentary behavior was positively associated with C-reactive protein [26]. Neck circumference and fat mass were positively correlated to sedentary behavior but were investigated in only one randomized controlled trial [27]. There was limited or no evidence for the other anthropometric biomarkers [26].
In our review, the findings related to sedentary behavior and C-reactive protein were not consistent. Rezende et al. 2014 reported a study that found a positive relationship between sedentary behavior and C-reactive protein [10]. In three of the five studies, positive associations were found between C-reactive protein and sedentary behavior. Other cardio-metabolic biomarkers such as pericardial fat, coronary artery calcification, neck circumference, and fat mass merit further research.
An area of great interest is the impact of sedentary behavior on the waist circumference of older adults. Three cross-sectional studies were identified; sedentary behavior had strong positive associations with waist circumference. In models adjusted for moderate-to-vigorous intensity physical activity, sedentary behavior was positively associated with both weight and waist circumference [27]. Similarly, Bann et al. [28] found that sedentary behavior was inversely associated with body mass index (BMI). In addition, less time spent in sedentary behavior and more time spent in light-intensity physical activities resulted in lower BMIs. These results were partially supported by a cross-sectional study [22].
Reference Author(s), Year, Title, Journal |
Hypotheses/Study Aims | Research Design, Sample Size, Ages | Measures | Primary Findings | Limitations | Strengths |
Bann, Hire, Manini et al. (2015); Light intensity physical activity and sedentary behavior in relation to body mass index and grip strength in older adults: cross-sectional findings from the lifestyle interventions and independence for elders (LIFE) study. Plos ONE | Examine the association between sedentary behavior, BMI, and grip strength. | Cross-sectional study (N = 1,130) of adults aged 70–89 living in the United States. | Accelerometer determined sedentary time Jamar determined grip strength BMI and waist circumference measured by trained staff |
In adjusted models, sedentary behavior was associated with BMI. Less time spent sedentary and more time spent in light-intensity physical activities was associated with BMI | Cross sectional design | Use of BMI as a measure of body size |
Gennuso, Gangnon Matthews (2013); Sedentary behavior, physical activity, and markers of health in older adults. Medicine and science in sports and exercise | Examine the association between sedentary behavior, physical activity, and cardiometabolic markers | Population based cross-sectional study (N = 1914) of adults aged 65 years and older living in the United States | Accelerometer determined sedentary behavior Cardiometabolic markers (total, HDL, and LDL cholesterol, glucose, triglycerides, glycohemoglobin, waist circumference, blood pressure, and C-reactive protein) Physical function |
In adjusted models, higher quartiles of sedentary behavior were associated with weight, BMI, waist circumference, systolic blood pressure, and C-reactive protein among participants who were insufficiently active older adults. Among sufficiently active older adults, higher quartiles of sedentary behavior were associated with waist circumference and LDL cholesterol levels. In addition, insufficiently active older adults who were less sedentary had lower odds of functional limitations. | Cross-sectional design | Accelerometer determined sedentary behavior Population based sample Serum cardiometabolic markers |
Figueiró, T. H., Arins, G., Santos, C., Cembranel, F., Medeiros, P. A., d'Orsi, E., & Rech, C. R. (2019); Association of objectively measured sedentary behavior and physical activity with cardiometabolic risk markers in older adults. Plos ONE | Examine the association between sedentary behavior and cardiometabolic markers | Cross-sectional study of Brazilians adults (N = 425) aged 60 years and older | Accelerometer determined sedentary behavior Waist circumference, blood pressure, and plasma based cardiometabolic markers |
Sedentary behavior was negatively and linearly associated with systolic blood pressure and HDL cholesterol. Physically inactive and sedentary individuals had greater waist circumference and lower HDL cholesterol levels than active, non-sedentary subjects. | Cross-sectional study design Confounding variables (i.e., medications and BMI) not included in the analysis |
Objectively measured sedentary time Plasma-based biomarkers |
In our review, the findings were consistent; in three studies, sedentary behavior was related to greater waist circumference in older adults. Similarly, in five of six studies, sedentary behavior was related to high waist circumference and high waist-to-hip ratio as reported in the prior review [10]. The only exception to the consistent findings is a study in a colorectal cancer population in which sedentary time was not associated with waist circumference. Overall, however, the research is consistent; sedentary time is a risk factor for greater waist circumference in older adults.
The Centers for Disease Control and Prevention classify individuals as obese with a body mass index (BMI) ≥30.0 kg/m2. A systematic review study indicated that sedentary behavior was linked to increased risk of obesity in various populations [29]. We identified three cross-sectional studies in our search. In an earlier study of older adults in the United States [27], positive associations were found between sedentary behavior and BMI. Similarly, Bann et al. [28] found that sedentary behavior was inversely associated with BMI, especially among those with lower levels of light-intensity physical activity. Furthermore, these results were supported by strong associations observed between sedentary behavior and waist circumference among older adults in Portugal. In particular, for each additional 10- to 20- minutes of sedentary time, the odds of abdominal obesity increased 6.8%. The odds increased up to 48% for each additional 60 minutes of time spent in sedentary behavior [30].
In our review, we found that all three studies reported positive associations between sedentary time and obesity, measured by BMI or waist circumference. In the prior review [10], five of the six studies reported a positive association between sedentary behavior and obesity. The one exception was a study examining the association between automobile-based sedentary behavior and obesity [10]. Overall, the research is consistent in that eight of nine studies reported a positive relationship between sedentary behavior, overweight, and obesity in older adults.
Reference Author(s), Year, Title, Journal |
Hypotheses/Study Aims | Research Design, Sample Size, Ages | Measures | Primary Findings | Limitations | Strengths |
Bann, Hire, Manini et al. (2015); Light intensity physical activity and sedentary behavior in relation to body mass index and grip strength in older adults: cross-sectional findings from the lifestyle interventions and independence for elders (LIFE) study. Plos ONE | Examine the association between sedentary behavior, BMI, and grip strength. | Cross-sectional study (N = 1,130) of adults aged 70–89 living in the United States. | Accelerometer determined sedentary time Jamar determined grip strength BMI and waist circumference measured by trained staff |
In adjusted models, sedentary behavior was associated with BMI. Less time spent sedentary and more time spent in light-intensity physical activities were associated with BMI | Cross sectional design | Use of BMI as a measure of body size |
Gennuso, Gangnon, Matthews (2013); Sedentary behavior, physical activity, and markers of health in older adults. Medicine and science in sports and exercise | Examine the association between sedentary behavior, physical activity, and cardiometabolic markers | Population based cross-sectional study (N=1914) of adults aged 65 years and older living in the United States | Accelerometer determined sedentary behavior Cardiometabolic markers (total, HDL, and LDL cholesterol, glucose, triglycerides, glycohemoglobin, waist circumference, blood pressure, and C-reactive protein) Physical function |
In adjusted models, higher quartiles of sedentary behavior were associated with weight, BMI, waist circumference, systolic blood pressure, and C-reactive protein among participants who were insufficiently active older adults. Among sufficiently active older adults, higher quartiles of sedentary behavior were associated with waist circumference and LDL cholesterol levels. In addition, insufficiently active older adults who were less sedentary had lower odds of functional limitations. | Cross-sectional design |
Accelerometer determined sedentary behavior Population based sample Serum cardiometabolic markers |
Judice, Silva, & Sardinha (2015); Sedentary bout durations are associated with abdominal obesity in older adults. The Journal of Nutrition, Health & Aging | Examine the association between sedentary time and patterns of abdominal obesity | Cross-sectional study (N = 351) of older adults (aged 65–103 years) from Portugal | Accelerometer determined sedentary behavior Waist circumference measured by trained staff |
No association was observed with total sedentary time and abdominal obesity. However, for bouts of sedentary time that ranged from 10–20 minutes up to <60 minutes, each bout significantly increased the odds of abdominal obesity. | Cross sectional design Dietary variables not considered in models |
Objectively measured sedentary time |
We identified eight articles published since 2013 that examined the association between sedentary behavior and mental and cognitive health. Two of the studies focused on dementia [31],[32]. In one study, the authors reported that institutionalized versus community-dwelling dementia patients had 23.5% lower daily physical activity levels (p = 0.004) and spent 9.3% more time in sedentary behavior (p = 0.032). Community-dwelling dementia patients had daily physical activity levels and sedentary time 21.6% lower (p = 0.007) and 8.9% longer (p = 0.078) than healthy older adults [31]. In another study, sedentary time and sedentary bout duration were significantly greater in dementia patients than in age- and sex-matched cognitively healthy older adults [32].
Mild cognitive impairment (MCI) is defined as cognitive decline greater than expected for age and education level, which does not interfere with independence. Mild cognitive impairment is associated with up to a 30% increased risk of developing dementia within 5 years [33]. In contrast, older adults without mild cognitive impairment develop dementia at a rate of 1% to 2% within 5 years [33]. Therefore, mild cognitive impairment is a critical phase to intervene because it is a transitional stage between healthy cognition and dementia [33]. As observed by Falck et al. 2017, providing effective strategies to maintain cognitive health during this transition period might slow the conversion to dementia [33]. We identified four articles related to mild cognitive impairment. Engeroff et al. 2018 reported that brain-derived neurotrophic factor (BDNF) levels (measure of brain plasticity) were detrimentally associated with sedentary time but beneficially related to accelerometer total activity counts and moderate-to-vigorous intensity physical activity [34]. Falck et al. [33] found that participants with probable mild cognitive impairment had lower physical activity and greater sedentary behavior compared to participants without mild cognitive impairment.
Parkinson's disease is a progressive, neurodegenerative disorder characterized by rigidity, tremor, impaired postural stability, decreased walking ability, and an increased risk of falls [35]. People with mild-to-moderate Parkinson's disease reported 75% of waking hours spent in sedentary behaviors and 18% engaged in physical activity [35].
Subjective cognitive complaints are a meaningful indicator of dementia onset or mild cognitive impairment [36]. Nemoto et al. (2018) found that moderate-to-vigorous intensity physical activity was significantly related with a lower risk of subjective cognitive complaints and that reading showed a dose-response relationship with subjective cognitive complaints [36]. The group who reported > 150 min/week physical activity and > 30 min/day reading showed 60% lower subjective cognitive complaints than the group who reported < 150 min/week physical activity and < 10 min/day reading [36].
Subjective well-being refers to personal evaluations of the degree or extent to which a person considers his/her life meaningful, satisfying, worthwhile, and rewarding [37]. A key to measure a person's wellbeing is the perception of quality of life being experienced [37]. We found two studies related to well-being and sedentary behavior. Okely et al. (2019) reported no association between wellbeing or symptoms of anxiety and sedentary time. On the other hand, symptoms of depression were positively associated with sedentary time [38]. Ku et al. (2015) found that participants who had greater frequencies of leisure-time physical activity and sedentary behaviors, especially engaging in walking, yard/gardening, group exercise, television watching, social chatting, and reading recorded greater levels of well-being. Other types of activities, such as solitary exercise, listening to radio and playing chess/cards were not related to subjective well-being [37]. Clearly, the type of sedentary behavior makes a difference related to health outcomes.
In our review, we found eight articles. Dementia was related to sedentary behavior. In three of the four studies, sedentary behavior was related to mild cognitive impairment. In one study, reading was related to lower subjective cognitive complaints. Symptoms of depression were positively associated with sedentary time. In contrast, participants who watched television, chatted socially, and read, recorded greater levels of well-being. Rezende et al. 2014 presented six studies [10]. Individuals who played board games and read were less likely to develop dementia. Similarly, reading books, playing board games, craft activities, computer activities, and watching television were significantly associated with decreased odds of having mild-cognitive impairment (two studies). In contrast, two studies found that sedentary time was negatively associated with psychological well-being and the highest quartile of sitting time was negatively associated with mental health. On the other hand, one study found no association between four or more hours per day of sedentary behavior and successful aging. Considering both reviews, the type of sedentary behavior can be important in understanding the findings. A taxonomy of sedentary behaviors for older adults is needed to elucidate the divergent findings related to sedentary behavior and mental health.
We found one study related to cancer [39]. The purpose of the study was to determine the impact of a mixed exercise program compared to stretching only to improve physical capacity and decrease sedentary behavior time in older adults during cancer treatment. The mixed physical exercise combined aerobic and resistance training. A greater decrease in sedentary behavior time was observed in favor of the mixed exercise program compared to the stretching only program [39].
Both reviews identified only one study related to cancer. In the Rezende et al. 2014 review [10], no association was found between time watching television or videos and renal cell carcinoma. Cancer and sedentary behavior in older adults are underdeveloped areas of research.
Reference Author(s), Year, Title, Journal |
Hypotheses/Study Aims | Research Design, Sample Size, Ages, Country | Measures | Primary Findings | Limitations | Strengths |
van Alphen et al. (2016); Older adults with dementia are sedentary for most of the day. Plos ONE | To objectively assess the physical activity levels of community dwelling and institutionalized ambulatory patients with dementia and to compare with the physical activity levels of cognitive healthy older adults. | The research design was a longitudinal study that examined the effects of regular physical activity on the health of dementia patients; participants were recruited via medical staff of aged care facilities. There were 83 institutionalized [mean age 83.0 (7.6)] and 37 community-dwelling dementia patients [mean age 77.3 (5.6)], and 26 healthy older adults [mean age 79.5 (5.6)]. The study was conducted in The Netherlands. | Actigraphy was used to assess physical activity levels that were based on the raw data. Sedentary behavior was classified < 100 counts/min. | Institutionalized vs. community-dwelling dementia patients had 23.5% lower daily physical activity levels (p = .004) and spent 9.3% more time in sedentary behavior (p = .032). Community-dwelling dementia patients vs healthy older adults' daily physical activity levels and sedentary time were 21.6% lower (p = .007) and 8.9% longer (p = .078). | One limitation is that all activity counts per minute represent the same physical activity intensity for healthy as well as adults with dementia. Another limitation is the selection procedure of institutions and daycare centers and homes based on existing collaborations. | The first study to objectively characterize institutionalized and community-dwelling dementia patients' physical activity and sedentary behavior levels. |
Hartman et al. (2018); Dementia patients are more sedentary and less physically active than age-and sex-matched cognitively healthy older adults. Dementia Geriatric Cognitive Disorders | To examine physical activity and sedentary behavior characteristics of ambulatory and community-dwelling patients with dementia compared to cognitively healthy age-, sex- and weight-matched controls. | Cross-sectional study - persons with a dementia diagnosis aged > 60 years who were ambulatory and community dwelling were included. (n = 45, age 79.6 ± 5.9 years), and matched controls (n = 49, age 80.0 ± 7.7 years). Cognitively healthy controls were age, sex, and weight matched to dementia patients and had no history of cognitive impairment. The study was conducted in The Netherlands. | Dementia diagnosis was based on comprehensive clinical assessment by a physician. Mini-Mental State Examination indicated severity of cognitive impairment. Participants wore a wrist accelerometer for 7 days to assess sedentary time, sedentary bout duration and time spent in very light, light-to-moderate and moderate-to-vigorous physical activities. |
Relative sedentary time and sedentary bout duration were significantly greater in dementia patients than in controls. In addition, dementia patients spent a lower percentage of their waking time in light-to-moderate and moderate-to-vigorous intensity physical activities. Secondly, older age was associated with a decline in physical activity and increase in sedentary behavior in controls; however, no such age-related changes were found in dementia patients. | The design of the study could not answer the question whether differences in physical activity and sedentary behavior are simply a consequence of dementia. Only community-dwelling patients were included; therefore, results cannot be generalized to institutionalized dementia patients. Furthermore, a potential bias related to the study enrollment should be considered. Dementia patients in the study were enrolled in an exercise trial. | An accelerometer is a validated measure of physical activity and sedentary behavior. A novel finding was that differences between dementia patients and controls remain when corrected for sleep time. |
Reference Author(s), Year, Title, Journal |
Hypotheses/Study Aims | Research Design, Sample Size, Ages, Country | Measures | Primary Findings | Limitations | Strengths |
Engeroff et al. (2018); Is objectively assessed sedentary behavior, physical activity and cardiorespiratory fitness linked to brain plasticity outcomes in old age? Neuroscience | Examine the association between brain plasticity outcomes and brain-derived neurotrophic factor | Cross-sectional study (N = 50) of older adults (aged 73–77 years old) in Germany | Accelerometer determined sedentary behavior Serum markers of brain metabolism and brain-derived neurotrophic factors (BDNF) Cardiorespiratory fitness |
Sedentary time was associated with BDNF, but not brain metabolism measures | Small sample Cognitively healthy sample |
Well controlled study Novel methods |
Falck et al. (2017); Cross-sectional relationships of physical activity and sedentary behavior with cognitive function in older adults with probable mild cognitive impairment. Physical Therapy |
To examine differences in physical activity and sedentary behavior between people with probable mild cognitive impairment and people without mild cognitive impairment and to assess whether associations of physical activity and sedentary behavior with cognitive function differed by mild cognitive impairment status. | The study was cross sectional. The sample size was 150. The mean age was 71.11 years old (7.22). The location was Vancouver, British Columbia, Canada. | Physical activity and sedentary behavior in adults dwelling in the community were measured using a wrist-worn actigraphy unit. The Montreal Cognitive Assessment categorized participants with probable mild cognitive impairment and participants without the condition. Cognitive function was indexed using the Alzheimer Disease Assessment Scale-Cognitive-Plus. | Participants with probable mild cognitive impairment had lower physical activity and higher sedentary behavior than participants without mild cognitive impairment. Higher physical activity and lower sedentary behavior were associated with better Alzheimer Disease Assessment Scale-Cognitive-Plus performance in participants without mild cognitive impairment but not in participants with probable mild cognitive impairment. | This study was cross-sectional and therefore causal associations should not be inferred. In addition, the diagnosis of mild cognitive impairment was not confirmed with a physician. | First study to report that older adults with probable mild cognitive impairment were less active and more sedentary than their cognitively healthy peers. Objective assessments of physical activity and sedentary behavior. |
Walle'n et al. (2015); Levels and patterns of physical activity and sedentary behavior in elderly people with mild to moderate Parkinson's disease. Physical Therapy | To describe levels and patterns of physical activity and sedentary behaviors in elderly people with mild to moderate Parkinson's Disease. | This cross-sectional study involved a free-living setting and 53 men and 42 women (mean age 73.4 years; (SD-5.7 years) with mild to moderate idiopathic Parkinson's Disease. The country was Sweden. | Time spent in physical activity and sedentary behaviors were assessed for one week with accelerometers. | The main finding was that approximately 75% of all time awake was spent in sedentary behaviors; 18% was spent in physical activity. The proportion of individuals meeting the goal of achieving 150 minutes of moderate- to vigorous-intensity PA per week was 27%; 16% achieved 7,000 or more steps per day; participants spent 589 minutes daily in sedentary behaviors. | The participants were not randomly selected; therefore, generalizability is limited. | Based on objective assessment (accelerometers), described the levels and patterns of physical activity, including diurnal patterns, and estimated the extent to which physical activity recommendations were met. |
Nemoto et al. (2018); The association of single and combined factors of sedentary behavior and physical activity with subjective cognitive complaints among community-dwelling older adults: Cross-sectional study. Plos ONE | To examine the association of type of sedentary behavior, physical activity, or their combination with subjective cognitive complaints among community dwelling older adults. | The cross-sectional study targeted independently living individuals aged 65 years and older who resided in Tsuru, Yamanashi Prefecture, Japan (n = 5,328). | Participants responded to the National Functional Survey Questionnaire (Kihon checklist) to assess subjective cognitive complaints. Do your family or friends point out your memory loss? Do you make a call by looking up phone numbers? Do you find yourself not knowing today's date? To evaluate physical activity, the Japanese version of the International Physical Activity Questionnaire Short-version (IPAQ-SV) was conducted. Sedentary behavior time was assessed as subjective average duration of television viewing and reading books or newspapers during the last seven days. | The primary findings were that moderate-to-vigorous intensity physical activity was significantly related with a lower risk of subjective cognitive complaints and that reading showed a dose-response relationship with subjective cognitive complaints. The combined group who reported >150 min/week physical activity and >30 min/day reading showed 60% lower subjective cognitive complaints than the combined group who reported <150 min/week physical activity and <10 min/day reading. | The cross-sectional design limits causal inferences. Physical activity and sedentary behavior were assessed subjectively. Participants who developed dementia or mild cognitive impairment during the study were not necessarily completely excluded. | First study to report the association of the combined effects of sedentary time and physical activity with subjective cognitive complaints; demographic variables, health behavior, and health status were included in the analyses. The data were from a complete survey with a high response rate of 79.8% and missing values were accounted for by multiple imputation. |
Reference Author(s), Year, Title, Journal |
Hypotheses/Study Aims | Research Design, Sample Size, Ages, Country | Measures | Primary Findings | Limitations | Strengths |
Okely et al. (2019); Positive and negative well-being and objectively measured sedentary behavior in older adults: evidence from three cohorts. BMC Geriatrics | To examine the association between sedentary behavior and both positive and negative effect | Three prospective (N = 698) of older adults in Scotland | Accelerometer determined sedentary behavior (ActivPAL) Validated scales for positive and negative effect, depression, and anxiety |
Sedentary behavior was associated with depression symptoms | Waking time was self-reported | Accelerometer determined sedentary behavior Validated measures of mental health |
Ku et al. (2015); Leisure-time physical activity, sedentary behaviors and subjective well-being in older adults: An eight-year longitudinal research. Social Indicators Research | Examine the association between self-reported sedentary time and subjective well-being | Longitudinal fixed cohort (N = 1,268) of adults aged 70 years or older in Taiwan. | Self-reported leisure time in sedentary behavior Subjective well-being (i.e., Life Satisfaction Index) |
Television viewing, social chatting, reading, and listening to the radio were significantly associated with subjective well-being | Self-reported sedentary time | Longitudinal design |
Reference Author(s), Year, Title, Journal |
Hypotheses/Study Aims | Research Design, Sample Size, Ages, Country | Measures | Primary Findings | Limitations | Strengths |
Maréchal et al. (2019); Effect of a mixed-exercise program on physical capacity and sedentary behavior in older adults during cancer treatments. Aging Clinical and Experimental Research | To examine the impact of an intervention on increasing physical activity and reducing sedentary behavior during cancer treatment | Two-group (i.e., mixed exercise vs. stretching) pre- and post-test design (N = 14) of older patients in Canada | Self-reported sedentary time Senior fitness test – functional performance test (i.e., chair stands, Up and Go, Arm Curl, Sit and Reach, and 6-minute walk test) |
Mixed exercise group had greater improvements in chair stands and global physical capacity scores when compared to those in the stretching group. No other differences were observed | Small sample Sedentary behavior was not the focus |
Objective assessments of functional performance |
The purpose of the quality ratings was to assess risk of bias. As described earlier, we used the National Institute of Health ratings system for each research design [12]. Among 20 observational cohort and cross-sectional studies, three studies were rated as fair and seventeen studies were rated as good. Among the four other research designs [i.e., before-after (pre-post) studies with no control group; case-control studies; controlled intervention studies; and systematic reviews/meta-analyses], there were seven studies. Five were rated as fair, one as good, and one was rated poor—controlled intervention study. Most of the studies adjusted for confounders. Most of the studies were cross-sectional. More randomized controlled trial studies are needed.
Each article in our review was published in a peer-reviewed journal; therefore, only one article was rated as poor. Our quality assessment of articles probably would be different if we had included the grey literature. Given the overall quality assessment of our articles, we are confident in the findings of our review. It is not likely that risk of bias compromises our major conclusions.
Research design | Reference | Quality assessment from National Institute of Health ratings criteria (good, fair, poor) |
Observational Cohort and Cross-Sectional Studies | ||
Bann et al. 2015 | Good | |
Benka Wallén et al. 2015 | Good | |
Cabanas-Sanchez et al. 2018 | Fair | |
Chang et al. 2015 | Fair | |
Ekblom et al. 2015 | Good | |
Engeroff et al. 2018 | Fair | |
Ensrud et al. 2014 | Good | |
Figueiró et al. 2019 | Good | |
Gennuso et al. 2013 | Good | |
Gennuso et al. 2015 | Good | |
Jefferis et al. 2019 | Good | |
Joseph et al. 2016 | Good | |
Judice et al. 2015 | Good | |
Klenk et al. 2016 | Good | |
Ku et al. 2016 | Good | |
Leon-Munoz et al. 2013 | Good | |
Matthews et al. 2012 | Good | |
Nemoto et al. 2018 | Good | |
Okely et al. 2019 | Good | |
Pavey et al. 2015 | Good | |
Before-After (Pre-Post) Studies with No Control Group | ||
Hartman et al. 2018 | Fair | |
Case-Control Studies | ||
Falck et al. 2017 | Fair | |
van Alphen et al. 2016 | Fair | |
Controlled Intervention Studies | ||
Aadahl et al. 2019 | Fair | |
Maréchal et al. 2019 | Poor | |
Systematic Reviews and Meta-Analyses | ||
Thorp et al. 2011 | Fair | |
Wirth et al. 2017 | Good |
By 2030, there will be nearly one billion older adults worldwide [40]. According to Harvey et al. (2013), almost 60% of older adults reported sitting for more than four hours per day; 65% sat in front of a screen for more than three hours per day; and over 55% reported watching television more than two hours. The previous findings were based on self-report; when measured objectively, 67% of older adults were sedentary for > 8.5 hours daily [40],[41].
The most consistent associations with increased risk between sedentary behaviors and health outcomes in older adults were: all-cause mortality, metabolic syndrome, triglycerides/high density lipoprotein cholesterol/blood glucose, HBA1C/glucose intolerance, waist circumference, and obesity/overweight. The inconsistent associations were for blood pressure, cancer, and other cardio-metabolic biomarkers. Mixed findings (positive and negative associations) were found for mental health (dementia, mild cognitive impairment, psychological well-being). A systematic review concluded that limiting sedentary time and participating in regular moderate-to-vigorous intensity physical activity promote healthy cognitive aging. Sedentary behavior was associated with lower cognitive performance, “although the attributable risk of sedentary time to all-cause dementia incidence is unclear” [31].
Our findings compared to an earlier review [10] were similar for all-cause mortality, metabolic syndrome, other cardio-metabolic biomarkers, waist circumference, obesity/overweight, cancer, and mental health (dementia, mild cognitive impairment, psychological well-being). These consistent associations may be attributable to robust results, sufficient literature to draw conclusions, and/or clear outcome measures. Our findings were dissimilar for triglycerides/high density lipoprotein cholesterol/blood glucose, blood pressure, and HBA1C/glucose intolerance. The dissimilar findings may be accounted for by our recent update (i.e., different times for which studies were conducted), a limited number of studies in the area, or absence of consistent or robust results. Another possibility that merits further study is that heterogeneity in clinical outcomes, methods, and statistical approaches may explain dissimilar results.
Rezende et al. 2014 [10] reported detrimental effects of sedentary behaviors on physical health in older adults. However, it is noteworthy that there are studies documenting positive psychological and mental effects at least for some forms of sedentary behavior [37]. Another review concluded that some sedentary behaviors (e.g., reading or use of computers) were beneficial for older adults [9]. As noted earlier, based on our review, we recommend a taxonomy of sedentary behavior for older adults to disentangle the web of seemingly discrepant findings. Further research is needed to provide greater insights.
Based on our review and the earlier review [10], another emerging area of research for older adults is cancer and sedentary behavior; only two studies were reported. Clearly, cancer, sedentary behavior, and older adults is an understudied research area [39]. Aging is regarded as an important cause of cancer and studies show that aging and cancer together contribute to an increased risk of deconditioning [42],[43]. In addition, the combination of aging and cancer is associated with an increase in sedentary behaviors. In the context of cancer treatment such as chemotherapy, patients are more likely to adopt sedentary behaviors and decrease physical activity levels that exacerbate deconditioning which hinders recovery and wellbeing [44]–[46]. This cycle may be particularly evident in older populations [45]–[47]. Unfortunately, health care professionals recommend resting and to avoid physical activity during cancer treatments believing this strategy limits cancer-related symptoms especially cancer-related fatigue [48]. More research is required to understand better how to disrupt this health-compromising cycle and improve the health of older adults during cancer treatments.
From another perspective, the biological pathways that underlie the associations between sedentary behavior and cancer is important [49]. In 10 of 18 articles, a review found that sedentary behavior was associated with increased colorectal, endometrial, ovarian, and prostate cancer risk and cancer mortality in women [49]. The biological pathways or mechanisms that produce the association between sedentary behavior and cancer were adiposity and metabolic dysfunction. The author recommended that reducing sedentary behavior is a viable cancer control strategy [49]. For the health outcomes reported in our systematic review, more research is needed documenting biological pathways.
Limitations—The limitations of this review are a publication bias because we did search for non-published papers and the grey literature. We did not include technical reports, papers from government agencies and other scientific groups, conference proceedings, and unpublished manuscripts. However, we acknowledge that searching the grey literature is important because, typically, only positive, significant findings are published in peer-reviewed journals. Another limitation is that findings were not categorized by physical activity patterns and levels, age groups (e.g., old and very old - over 80 years of age), gender, racial and ethnic identity, or by dose and type of sedentary behavior. All studies should adjust for confounders. Because our systematic review was not a meta-analysis, we did not assess clinical, methodological, and statistical heterogeneity; differences that can influence conclusions. In a narrative review, geriatric-relevant health outcomes included physical function, cognitive function, incontinence, mental health, quality of life and wellbeing as well as sleep. In our review, we did not review research related to incontinence and sleep. Another limitation is the extent to which the literature is dependent on self-report measures. More objective assessments of sedentary behaviors are recommended.
Strengths—In spite of these limitations, our systematic review is the most recent and comprehensive study of this topic including a full description of each article and an evaluation of the quality of each article. Furthermore, we summarize the findings for twelve different health conditions ranging from mortality to mental health and then compared our findings to a previous review. Each study was evaluated for quality and risk of bias based on the National Institute of Health standardized approach for rating the quality of studies [12]. Key characteristics of each study were described and presented in both narrative and table formats.
Future research, policy, and practice – Given the growing population of older adults and the prevalence of sedentary behavior in this population, the effects of sedentary behavior both positive and negative on health outcomes merit careful study. Worldwide, the number of dementia patients has been rapidly increasing during the last few decades, and is expected to reach over 100 million in the year 2050 [36]. Age is the greatest risk factor for dementia [31]. Effective lifestyle interventions are critical because non-pharmacological therapies can be used to treat dementia. The true efficacy of these approaches is not known [31]. Therefore, to prevent dementia or cognitive decline is an urgent, global public health crisis.
To improve the methodological quality of future research, we recommend greater use of diaries and global position systems (GPS) (to provide a context) and accelerometers, which are regarded as a valid and reliable method for evaluating sedentary behavior. In addition, more subetaoup analyses including gender, racial and ethnic identity, and geography would improve our understanding of sedentary behavior and health outcomes in older adults. Similarly, dose-response gradients between sedentary behavior, physical activity, and health outcomes would advance the field. We recommend that future research include meta-analyses.
As noted in previous reviews, chronic diseases and all-cause mortality and the effects of sedentary behavior are important [9],[10]. However, from a geriatric perspective, the effects of sedentary behavior on independence, quality of life, and overall impairment (e.g., mobility) are high priority concerns for older adults [9]. More research is needed in these areas.
The next stage of research is to identify modifiable determinants of sedentary behavior among older adults to inform the development of behavior change interventions to reduce health-compromising sedentary behaviors and increase physical activity to improve the health and wellbeing of older adults. An earlier review evaluated the effectiveness of sedentary behavior interventions for older adults and reported non-significant findings [9].
In terms of policy and practice, The United Kingdom advises those 65 years of age and older to minimize the time they spend being sedentary for extended periods [40]. Any proposed policies and practices should be evaluated in randomized controlled trials to evaluate the effects on mental, psychological, and physical health. Policies and practices should discourage health-compromising sedentary behaviors and promote health-enhancing sedentary behaviors (i.e., for mental and psychological health) with the objective to improve the overall wellbeing of older adults.
There is a global trend in population aging that embraces terms such as “successful aging”, “active aging,” and “healthy aging” which imply adding life to years and not just living longer (i.e., adding years to life) [37]. To achieve this objective, given the high levels of sedentary behavior among older adults, documenting the health effects of sedentary behavior is important. In our systematic review, we found that sedentary behaviors were adversely associated with six health outcomes ranging from obesity to mortality. Contrary to expectations, depending on the dose and type of sedentary behavior, older adults may benefit from particular kinds of sedentary pursuits that are stimulating and cognitively engaging. Our hope is that this review can be serve as a springboard for more rigorous studies to advance the field of sedentary behavior and health effects on older adults.
[1] | A. A. Kilbas, H. M. Srivastava, J. J. Trujillo, Theory and applications of fractional differential equations, Elsevier, 2006. |
[2] | R. Hilfer, Applications of fractional calculus in physics, World Scientific, 2000. |
[3] |
A. Ali, K. Shah, T. Abdeljawad, Study of implicit delay fractional differential equations under anti-periodic boundary conditions, Adv. Differ. Equ., 2020 (2020), 139. https://doi.org/10.1186/s13662-020-02597-x doi: 10.1186/s13662-020-02597-x
![]() |
[4] |
J. O. Alzabut, Almost periodic solutions for an impulsive delay Nicholson's blowflies model, J. Comput. Appl. Math., 234 (2010), 233–239. https://doi.org/10.1016/j.cam.2009.12.019 doi: 10.1016/j.cam.2009.12.019
![]() |
[5] | S. T. M. Thabet, M. B. Dhakne, On nonlinear fractional integro-differential equations with two boundary conditions, Adv. Stud. Contemp. Math., 26 (2016), 513–526. |
[6] |
M. I. Ayari, S. T. M. Thabet, Qualitative properties and approximate solutions of thermostat fractional dynamics system via a nonsingular kernel operator, Arab J. Math. Sci., 2023. https://doi.org/10.1108/AJMS-06-2022-0147 doi: 10.1108/AJMS-06-2022-0147
![]() |
[7] |
S. T. M. Thabet, M. M. Matar, M. A. Salman, M. E. Samei, M. Vivas-Cortez, I. Kedim, On coupled snap system with integral boundary conditions in the -Caputo sense, AIMS Mathematics, 8 (2023), 12576–12605. https://doi.org/10.3934/math.2023632 doi: 10.3934/math.2023632
![]() |
[8] |
S. T. M. Thabet, M. Vivas-Cortez, I. Kedim, M. E. Samei, M. I. Ayari, Solvability of a -Hilfer fractional snap dynamic system on unbounded domains, Fractal Fract., 7 (2023), 607. https://doi.org/10.3390/fractalfract7080607 doi: 10.3390/fractalfract7080607
![]() |
[9] |
P. J. Torvik, R. L. Bagley, On the appearance of the fractional derivative in the behavior of real materials, J. Appl. Mech., 51 (1984), 294–298. https://doi.org/10.1115/1.3167615 doi: 10.1115/1.3167615
![]() |
[10] |
M. I. Syam, A. Alsuwaidi, A. Alneyadi, S. Al Refai, S. Al Khaldi, An implicit hybrid method for solving fractional Bagley-Torvik boundary value problem, Mathematics, 6 (2018), 109. https://doi.org/10.3390/math6070109 doi: 10.3390/math6070109
![]() |
[11] |
V. Saw, S. Kumar, Numerical solution of fraction Bagley-Torvik boundary value problem based on Chebyshev collocation method, Int. J. Appl. Comput. Math., 5 (2019), 68. https://doi.org/10.1007/s40819-019-0653-8 doi: 10.1007/s40819-019-0653-8
![]() |
[12] |
H. M. Srivastava, F. A. Shah, R. Abass, An application of the Gegenbauer Wavelet method for the numerical solution of the fractional Bagley-Torvik equation, Russ. J. Math. Phys., 26 (2019), 77–93. https://doi.org/10.1134/S1061920819010096 doi: 10.1134/S1061920819010096
![]() |
[13] |
H. M. Srivastava, R. M. Jena, S. Chakraverty, S. K. Jena, Dynamic response analysis of fractionally-damped generalized Bagley–Torvik equation subject to external loads, Russ. J. Math. Phys., 27 (2020), 254–268. https://doi.org/10.1134/S1061920820020120 doi: 10.1134/S1061920820020120
![]() |
[14] |
S. Yüzbaşı, M. Karaçayır, A Galerkin-type fractional approach for solutions of Bagley-Torvik equations, Comput. Model. Eng. Sci., 123 (2020), 941–956. https://doi.org/10.32604/cmes.2020.08938 doi: 10.32604/cmes.2020.08938
![]() |
[15] |
M. El-Gamel, M. A. El-Hady, Numerical solution of the Bagley-Torvik equation by Legendre-collocation method, SeMA J., 74 (2017), 371–383. https://doi.org/10.1007/s40324-016-0089-6 doi: 10.1007/s40324-016-0089-6
![]() |
[16] |
A. B. Deshi, G. A. Gudodagi, Numerical solution of Bagley–Torvik, nonlinear and higher order fractional differential equations using Haar wavelet, SeMA J., 79 (2021), 663–675. https://doi.org/10.1007/s40324-021-00264-z doi: 10.1007/s40324-021-00264-z
![]() |
[17] |
A. G. Atta, G. M. Moatimid, Y. H. Youssri, Generalized Fibonacci operational tau algorithm for fractional Bagley-Torvik equation, Prog. Fract. Differ. Appl., 6 (2020), 215–224. http://doi.org/10.18576/pfda/060305 doi: 10.18576/pfda/060305
![]() |
[18] |
Y. H. Youssri, A new operational matrix of Caputo fractional derivatives of Fermat polynomials: An application for solving the Bagley-Torvik equation, Adv. Differ. Equ., 2017 (2017), 73. http://doi.org/10.1186/s13662-017-1123-4 doi: 10.1186/s13662-017-1123-4
![]() |
[19] |
S. Stanek, Two-point boundary value problems for the generalized Bagley-Torvik fractional differential equation, Cent. Eur. J. Math., 11 (2013), 574–593. https://doi.org/10.2478/s11533-012-0141-4 doi: 10.2478/s11533-012-0141-4
![]() |
[20] |
W. Labecca, O. Guimaraes, J. R. C. Piqueira, Analytical solution of general Bagley-Torvik equation, Math. Probl. Eng., 2015 (2015), 591715. https://doi.org/10.1155/2015/591715 doi: 10.1155/2015/591715
![]() |
[21] |
H. Fazli, J. J. Nieto, An investigation of fractional Bagley-Torvik equation, Open Math., 17 (2019), 499–512. https://doi.org/10.1515/math-2019-0040 doi: 10.1515/math-2019-0040
![]() |
[22] |
D. Pang, W. Jiang, J. Du, A. U. K. Niazi, Analytical solution of the generalized Bagley-Torvik equation, Adv. Differ. Equ., 2019 (2019), 207. https://doi.org/10.1186/s13662-019-2082-8 doi: 10.1186/s13662-019-2082-8
![]() |
[23] |
H. Baghani, M. Feckan, J. Farokhi-Ostad, J. Alzabut, New existence and uniqueness result for fractional Bagley-Torvik differential equation, Miskolc Math. Notes, 23 (2022), 537–549. http://doi.org/10.18514/MMN.2022.3702 doi: 10.18514/MMN.2022.3702
![]() |
[24] |
A. A. Zafar, G. Kudra, J. Awrejcewicz, An investigation of fractional Bagley-Torvik equation, Entropy, 22 (2020), 28. https://doi.org/10.3390/e22010028 doi: 10.3390/e22010028
![]() |
[25] |
J. Zhou, S. Zhang, Y. He, Existence and stability of solution for nonlinear differential equations with -Hilfer fractional derivative, Appl. Math. Lett., 121, (2021), 107457. https://doi.org/10.1016/j.aml.2021.107457 doi: 10.1016/j.aml.2021.107457
![]() |
[26] |
Y. Liu, Existence and unboundedness of positive solutions for singular boundary value problems on half-line, Appl. Math. Comput., 144 (2003), 543–556. https://doi.org/10.1016/S0096-3003(02)00431-9 doi: 10.1016/S0096-3003(02)00431-9
![]() |
[27] |
S. T. M. Thabet, I. Kedim, Study of nonlocal multiorder implicit differential equation involving Hilfer fractional derivative on unbounded domains, J. Math., 2023 (2023), 8668325. https://doi.org/10.1155/2023/8668325 doi: 10.1155/2023/8668325
![]() |
[28] |
S. T. M. Thabet, S. Al-Sadi, I. Kedim, A. Sh. Rafeeq, S. Rezapour, Analysis study on multi-order -Hilfer fractional pantograph implicit differential equation on unbounded domains, AIMS Mathematics, 8 (2023), 18455–18473. https://doi.org/10.3934/math.2023938 doi: 10.3934/math.2023938
![]() |
[29] | J. B. Diaz, B. Margolis, A fixed point theorem of the alternative, for contractions on a generalized complete metric space, Bull. Amer. Math. Soc., 74 (1968), 305–309. |
[30] | Y. Zhou, Basic theory of fractional differential equations, World Scientific, 2014. |
[31] |
X. Su, S. Zhang, Unbounded solutions to a boundary value problem of fractional order on the halfline, Comput. Math. Appl., 61 (2011), 1079–1087. https://doi.org/10.1016/j.camwa.2010.12.058 doi: 10.1016/j.camwa.2010.12.058
![]() |
[32] |
X. Su, Solutions to boundary value problem of fractional order on unbounded domains in a Banach space, Nonlinear Anal., 74 (2011), 2844–2852. https://doi.org/10.1016/j.na.2011.01.006 doi: 10.1016/j.na.2011.01.006
![]() |
[33] | L. Cdariu, L. Gvruta, P. Gvruta, Weighted space method for the stability of some nonlinear equations, Appl. Anal. Discr. Math., 6 (2012), 126–139. |
[34] |
E. C. de Oliveira, J. V. da C. Sousa, Ulam-Hyers-Rassias stability for a class of fractional integro-differential equations, Results Math., 73 (2018), 111. https://doi.org/10.1007/s00025-018-0872-z doi: 10.1007/s00025-018-0872-z
![]() |
1. | TARRAH MITCHELL, KELSEY BORNER, JONATHAN FINCH, JACQUELINE KERR, JORDAN A. CARLSON, Using Activity Monitors to Measure Sit-to-Stand Transitions in Overweight/Obese Youth, 2017, 49, 0195-9131, 1592, 10.1249/MSS.0000000000001266 | |
2. | QU TANG, DINESH JOHN, BINOD THAPA-CHHETRY, DIEGO JOSE ARGUELLO, STEPHEN INTILLE, Posture and Physical Activity Detection: Impact of Number of Sensors and Feature Type, 2020, 52, 1530-0315, 1834, 10.1249/MSS.0000000000002306 | |
3. | Anna G.M. Rojer, Keenan A. Ramsey, Marijke C. Trappenburg, Natascha M. van Rijssen, René H.J. Otten, Martijn W. Heymans, Mirjam Pijnappels, Carel G.M. Meskers, Andrea B. Maier, Instrumented measures of sedentary behaviour and physical activity are associated with mortality in community-dwelling older adults: A systematic review, meta-analysis and meta-regression analysis, 2020, 61, 15681637, 101061, 10.1016/j.arr.2020.101061 | |
4. | Roman P. Kuster, Daniel Baumgartner, Maria Hagströmer, Wilhelmus J.A. Grooten, Where to Place Which Sensor to Measure Sedentary Behavior? A Method Development and Comparison Among Various Sensor Placements and Signal Types, 2020, 3, 2575-6605, 274, 10.1123/jmpb.2019-0060 | |
5. | Saejong Park, Mihyun Lee, Validation of Physical Activity Measured by Accelerometers Worn on Waist and Wrist, 2017, 56, 1738-964X, 563, 10.23949/kjpe.2017.07.56.4.38 | |
6. | Gil Boudet, Pierre Chausse, David Thivel, Sylvie Rousset, Martial Mermillod, Julien S. Baker, Lenise M. Parreira, Yolande Esquirol, Martine Duclos, Frédéric Dutheil, How to Measure Sedentary Behavior at Work?, 2019, 7, 2296-2565, 10.3389/fpubh.2019.00167 | |
7. | E. J. Smits, E. A. H. Winkler, G. N. Healy, P. M. Dall, M. H. Granat, P. W. Hodges, Comparison of single- and dual-monitor approaches to differentiate sitting from lying in free-living conditions, 2018, 28, 09057188, 1888, 10.1111/sms.13203 | |
8. | Nitin Nair, Chinchu Thomas, Dinesh Babu Jayagopi, 2018, Human Activity Recognition Using Temporal Convolutional Network, 9781450364874, 1, 10.1145/3266157.3266221 | |
9. | Vahid Farrahi, Maisa Niemela, Petra Tjurin, Maarit Kangas, Raija Korpelainen, Timo Jamsa, Evaluating and Enhancing the Generalization Performance of Machine Learning Models for Physical Activity Intensity Prediction From Raw Acceleration Data, 2020, 24, 2168-2194, 27, 10.1109/JBHI.2019.2917565 | |
10. | Abdul Hamid, Michael J. Duncan, Emma L. J. Eyre, Yanguo Jing, Predicting children’s energy expenditure during physical activity using deep learning and wearable sensor data, 2020, 1746-1391, 1, 10.1080/17461391.2020.1789749 | |
11. | Alexander H.K. Montoye, James M. Pivarnik, Lanay M. Mudd, Subir Biswas, Karin A. Pfeiffer, Evaluation of the activPAL accelerometer for physical activity and energy expenditure estimation in a semi-structured setting, 2017, 20, 14402440, 1003, 10.1016/j.jsams.2017.04.011 | |
12. | Anantha Narayanan, Farzanah Desai, Tom Stewart, Scott Duncan, Lisa Mackay, Application of Raw Accelerometer Data and Machine-Learning Techniques to Characterize Human Movement Behavior: A Systematic Scoping Review, 2020, 17, 1543-3080, 360, 10.1123/jpah.2019-0088 | |
13. | Jesus D. Ceron, Diego M. Lopez, Gustavo A. Ramirez, A mobile system for sedentary behaviors classification based on accelerometer and location data, 2017, 92-93, 01663615, 25, 10.1016/j.compind.2017.06.005 | |
14. | Vahid Farrahi, Maisa Niemelä, Maarit Kangas, Raija Korpelainen, Timo Jämsä, Calibration and validation of accelerometer-based activity monitors: A systematic review of machine-learning approaches, 2019, 68, 09666362, 285, 10.1016/j.gaitpost.2018.12.003 | |
15. | Hanneke C. van Dijk-Huisman, Wouter Bijnens, Rachel Senden, Johannes M. N. Essers, Kenneth Meijer, Jos Aarts, Antoine F. Lenssen, Optimization and Validation of a Classification Algorithm for Assessment of Physical Activity in Hospitalized Patients, 2021, 21, 1424-8220, 1652, 10.3390/s21051652 | |
16. | Anis Davoudi, Kumar Rohit Malhotra, Benjamin Shickel, Scott Siegel, Seth Williams, Matthew Ruppert, Emel Bihorac, Tezcan Ozrazgat-Baslanti, Patrick J. Tighe, Azra Bihorac, Parisa Rashidi, Intelligent ICU for Autonomous Patient Monitoring Using Pervasive Sensing and Deep Learning, 2019, 9, 2045-2322, 10.1038/s41598-019-44004-w | |
17. | Rashmika Nawaratne, Damminda Alahakoon, Daswin De Silva, Paul D O’Halloran, Alexander HK Montoye, Kiera Staley, Matthew Nicholson, Michael IC Kingsley, Deep Learning to Predict Energy Expenditure and Activity Intensity in Free Living Conditions using Wrist-specific Accelerometry, 2021, 39, 0264-0414, 683, 10.1080/02640414.2020.1841394 | |
18. | Michael I.C. Kingsley, Rashmika Nawaratne, Paul D. O’Halloran, Alexander H.K. Montoye, Damminda Alahakoon, Daswin De Silva, Kiera Staley, Matthew Nicholson, Wrist-specific accelerometry methods for estimating free-living physical activity, 2019, 22, 14402440, 677, 10.1016/j.jsams.2018.12.003 | |
19. | Katja Krustrup Pedersen, Esben Lykke Skovgaard, Ryan Larsen, Mikkel Stengaard, Søren Sørensen, Kristian Overgaard, The Applicability of Thigh-Worn vs. Hip-Worn ActiGraph Accelerometers During Walking and Running, 2019, 2, 2575-6605, 209, 10.1123/jmpb.2018-0043 | |
20. | Alexander H. K. Montoye, Bradford S. Westgate, Morgan R. Fonley, Karin A. Pfeiffer, Cross-validation and out-of-sample testing of physical activity intensity predictions with a wrist-worn accelerometer, 2018, 124, 8750-7587, 1284, 10.1152/japplphysiol.00760.2017 | |
21. | Jennifer L. Huberty, Jeni L. Matthews, Meynard Toledo, Lindsay Smith, Catherine L. Jarrett, Benjamin Duncan, Matthew P. Buman, Vinyasa Flow: Metabolic Cost and Validation of Hip- and Wrist-Worn Wearable Sensors, 2018, 1, 2575-6605, 174, 10.1123/jmpb.2017-0010 | |
22. | Li-Tang Tsai, Eleanor Boyle, Jan C. Brønd, Gry Kock, Mathias Skjødt, Lars G. Hvid, Paolo Caserotti, Associations between objectively measured physical activity, sedentary behaviour and time in bed among 75+ community-dwelling Danish older adults, 2021, 21, 1471-2318, 10.1186/s12877-020-01856-6 | |
23. | Joost Oomen, Dennis Arts, Michel Sperling, Steven Vos, A stepwise science-industry collaboration to optimize the calculation of energy expenditure during walking and running with a consumer-based activity device, 2019, 56, 0160791X, 1, 10.1016/j.techsoc.2018.09.001 | |
24. | Marco Giurgiu, Johannes B.J. Bussmann, Holger Hill, Bastian Anedda, Marcel Kronenwett, Elena D. Koch, Ulrich W. Ebner-Priemer, Markus Reichert, Validating Accelerometers for the Assessment of Body Position and Sedentary Behavior, 2020, 3, 2575-6605, 253, 10.1123/jmpb.2019-0068 | |
25. | Udeni Jayasinghe, William S. Harwin, Faustina Hwang, Comparing Clothing-Mounted Sensors with Wearable Sensors for Movement Analysis and Activity Classification, 2019, 20, 1424-8220, 82, 10.3390/s20010082 | |
26. | ALEXANDER H. K. MONTOYE, M. BENJAMIN NELSON, JOSHUA M. BOCK, MARY T. IMBODEN, LEONARD A. KAMINSKY, KELLY A. MACKINTOSH, MELITTA A. MCNARRY, KARIN A. PFEIFFER, Raw and Count Data Comparability of Hip-Worn ActiGraph GT3X+ and Link Accelerometers, 2018, 50, 0195-9131, 1103, 10.1249/MSS.0000000000001534 | |
27. | Alexander H. K. Montoye, Scott A. Conger, Christopher P. Connolly, Mary T. Imboden, M. Benjamin Nelson, Josh M. Bock, Leonard A. Kaminsky, Validation of Accelerometer-Based Energy Expenditure Prediction Models in Structured and Simulated Free-Living Settings, 2017, 21, 1091-367X, 223, 10.1080/1091367X.2017.1337638 | |
28. | Justin Aunger, Janelle Wagnild, Objective and subjective measurement of sedentary behavior in human adults: A toolkit, 2020, 1042-0533, 10.1002/ajhb.23546 | |
29. | Alec PL Tolley, Keenan A Ramsey, Anna GM Rojer, Esmee M Reijnierse, Andrea B Maier, Objectively measured physical activity is associated with frailty in community-dwelling older adults: A systematic review, 2021, 08954356, 10.1016/j.jclinepi.2021.04.009 | |
30. | Joana Chong, Petra Tjurin, Maisa Niemelä, Timo Jämsä, Vahid Farrahi, Machine-learning models for activity class prediction: A comparative study of feature selection and classification algorithms, 2021, 89, 09666362, 45, 10.1016/j.gaitpost.2021.06.017 | |
31. | Sonia W.M. Cheng, Jennifer A. Alison, Emmanuel Stamatakis, Sarah M. Dennis, Zoe J. McKeough, Validity and Accuracy of Step Count as an Indicator of a Sedentary Lifestyle in People With Chronic Obstructive Pulmonary Disease, 2023, 00039993, 10.1016/j.apmr.2023.01.020 | |
32. | Mary C. Hidde, Kate Lyden, Kim Henry, Heather J. Leach, Reallocating Time to Physical Activity and Sleep: Associations with Body Mass Index in Cancer Survivors, 2023, 1070-5503, 10.1007/s12529-023-10152-7 | |
33. | Mary C. Hidde, Mary E. Crisafio, Emma Gomes, Kate Lyden, Heather J. Leach, An Evaluation of Participant Perspectives and Wear-Time Compliance for a Wrist-Worn Versus Thigh-Worn Accelerometer in Cancer Survivors, 2023, 20, 1543-3080, 129, 10.1123/jpah.2022-0346 | |
34. | Alexander H.K. Montoye, Bradford S. Westgate, Kimberly A. Clevenger, Karin A. Pfeiffer, Joseph D. Vondrasek, Morgan R. Fonley, Joshua M. Bock, Leonard A. Kaminsky, Individual versus Group Calibration of Machine Learning Models for Physical Activity Assessment Using Body-Worn Accelerometers, 2021, Publish Ahead of Print, 0195-9131, 10.1249/MSS.0000000000002752 | |
35. | Ayda Karaca, Necip Demirci, Vedat Yılmaz, Sinem Hazır Aytar, Sema Can, Evrim Ünver, Validation of the ActiGraph wGT3X-BT Accelerometer for Step Counts at Five Different Body Locations in Laboratory Settings, 2022, 26, 1091-367X, 63, 10.1080/1091367X.2021.1948414 | |
36. | Aleksandra Makarewicz, Małgorzata Jamka, Maria Wasiewicz-Gajdzis, Joanna Bajerska, Anna Miśkiewicz-Chotnicka, Jarosław Kwiecień, Aleksandra Lisowska, Dominque Gagnon, Karl-Heinz Herzig, Edyta Mądry, Jarosław Walkowiak, Comparison of Subjective and Objective Methods to Measure the Physical Activity of Non-Depressed Middle-Aged Healthy Subjects with Normal Cognitive Function and Mild Cognitive Impairment—A Cross-Sectional Study, 2021, 18, 1660-4601, 8042, 10.3390/ijerph18158042 | |
37. | Shu Ning Ch’ng, Joanne A. McVeigh, David Manners, Terry Boyle, Carolyn J. Peddle-McIntyre, Rajesh Thomas, Jeanie Leong, Samantha Bowyer, Kirsten Mooney, Leon Straker, Daniel A. Galvão, Vinicius Cavalheri, Sedentary Behaviour, Physical Activity, and Their Associations with Health Outcomes at the Time of Diagnosis in People with Inoperable Lung Cancer, 2022, 11, 2077-0383, 5870, 10.3390/jcm11195870 | |
38. | Fangyu Liu, Amal A Wanigatunga, Jennifer A Schrack, Assessment of Physical Activity in Adults Using Wrist Accelerometers, 2021, 43, 1478-6729, 65, 10.1093/epirev/mxab004 | |
39. | Riccardo Femiano, Charlotte Werner, Matthias Wilhelm, Prisca Eser, Validation of open-source step-counting algorithms for wrist-worn tri-axial accelerometers in cardiovascular patients, 2022, 92, 09666362, 206, 10.1016/j.gaitpost.2021.11.035 | |
40. | Matthew C. Ruder, Roger Zauel, Brian J. Diefenbach, Michael J. Bey, Quantifying shoulder activity after rotator cuff repair: Technique and preliminary results, 2022, 40, 0736-0266, 917, 10.1002/jor.25122 | |
41. | MIKAEL ANNE GREENWOOD-HICKMAN, SUPUN NAKANDALA, MARTA M. JANKOWSKA, DORI E. ROSENBERG, FATIMA TUZ-ZAHRA, JOHN BELLETTIERE, JORDAN CARLSON, PAUL R. HIBBING, JINGJING ZOU, ANDREA Z. LACROIX, ARUN KUMAR, LOKI NATARAJAN, The CNN Hip Accelerometer Posture (CHAP) Method for Classifying Sitting Patterns from Hip Accelerometers: A Validation Study, 2021, 53, 1530-0315, 2445, 10.1249/MSS.0000000000002705 | |
42. | Thomas D. Griffiths, Diane Crone, Mike Stembridge, Rachel N. Lord, Co-Production at Work: The Process of Breaking Up Sitting Time to Improve Cardiovascular Health. A Pilot Study, 2021, 19, 1660-4601, 361, 10.3390/ijerph19010361 | |
43. | Willie Leung, Layne Case, Ming-Chih Sung, Jaehun Jung, A meta-analysis of Fitbit devices: same company, different models, different validity evidence, 2022, 46, 0309-1902, 102, 10.1080/03091902.2021.2006350 | |
44. | Charlotte L Edwardson, Benjamin D Maylor, Nathan P Dawkins, Tatiana Plekhanova, Alex V Rowlands, Comparability of Postural and Physical Activity Metrics from Different Accelerometer Brands Worn on the Thigh: Data Harmonization Possibilities, 2022, 26, 1091-367X, 39, 10.1080/1091367X.2021.1944154 | |
45. | Mikael Anne Greenwood-Hickman, Jing Zhou, Andrea Cook, Kayne D. Mettert, Bev Green, Jennifer McClure, David Arterburn, Stefani Florez-Acevedo, Dori E. Rosenberg, Exploring Differences in Older Adult Accelerometer-Measured Sedentary Behavior and Resting Blood Pressure Before and During the COVID-19 Pandemic, 2022, 8, 2333-7214, 233372142210960, 10.1177/23337214221096007 | |
46. | Alexander H.K. Montoye, Olivia Coolman, Amberly Keyes, Megan Ready, Jaedyn Shelton, Ethan Willett, Brian C. Rider, Evaluation of Two Thigh-Worn Accelerometer Brands in Laboratory and Free-Living Settings, 2022, 5, 2575-6605, 233, 10.1123/jmpb.2022-0012 | |
47. | Karin A Pfeiffer, Kimberly A Clevenger, Andrew Kaplan, Cailyn A Van Camp, Scott J Strath, Alexander H K Montoye, Accessibility and use of novel methods for predicting physical activity and energy expenditure using accelerometry: a scoping review, 2022, 43, 0967-3334, 09TR01, 10.1088/1361-6579/ac89ca | |
48. | James D. Amor, Christopher J. James, Validation of a Commercial Android Smartwatch as an Activity Monitoring Platform, 2018, 22, 2168-2194, 968, 10.1109/JBHI.2017.2732678 | |
49. | Nolan Gall, Ruopeng Sun, Matthew Smuck, A Comparison of Wrist- Versus Hip-Worn ActiGraph Sensors for Assessing Physical Activity in Adults: A Systematic Review, 2022, 5, 2575-6605, 252, 10.1123/jmpb.2021-0045 | |
50. | Thomas Bilterys, Eveline Van Looveren, Olivier Mairesse, Jo Nijs, Mira Meeus, Kelly Ickmans, Barbara Cagnie, Dorien Goubert, Ward Willaert, Robby De Pauw, Lieven Danneels, Maarten Moens, Anneleen Malfliet, Predictors for physical activity and its change after active physical therapy in people with spinal pain and insomnia: Secondary analysis of a randomized controlled trial, 2022, 26, 14133555, 100456, 10.1016/j.bjpt.2022.100456 | |
51. | Xiaoping Zheng, Michiel F Reneman, Rita HR Schiphorst Preuper, Egbert Otten, Claudine JC Lamoth, Relationship between physical activity and central sensitization in chronic low back pain: Insights from machine learning, 2023, 232, 01692607, 107432, 10.1016/j.cmpb.2023.107432 | |
52. | Anne-Christine Auge, Gabriel Blouin-Demers, Dennis L. Murray, William David Halliday, Developing a classification system to assign activity states to two species of freshwater turtles, 2022, 17, 1932-6203, e0277491, 10.1371/journal.pone.0277491 | |
53. | Mayara S. Bianchim, Melitta A. McNarry, Rachel Evans, Lena Thia, Alan R. Barker, Craig A. Williams, Sarah Denford, Kelly A Mackintosh, Calibration and Cross-validation of Accelerometry in Children and Adolescents with Cystic Fibrosis, 2023, 27, 1091-367X, 51, 10.1080/1091367X.2022.2065919 | |
54. | Vincenza Gianfredi, Pietro Ferrara, Flavia Pennisi, Giulia Casu, Andrea Amerio, Anna Odone, Daniele Nucci, Monica Dinu, Association between Daily Pattern of Physical Activity and Depression: A Systematic Review, 2022, 19, 1660-4601, 6505, 10.3390/ijerph19116505 | |
55. | Zeynep S. Akinci, Oriol Marquet, Xavier Delclòs-Alió, Carme Miralles-Guasch, Urban vitality and seniors’ outdoor rest time in Barcelona, 2022, 98, 09666923, 103241, 10.1016/j.jtrangeo.2021.103241 | |
56. | Duncan S Buchan, Benjamin D Maylor, Comparison of physical activity metrics from two research-grade accelerometers worn on the non-dominant wrist and thigh in children, 2023, 0264-0414, 1, 10.1080/02640414.2023.2197726 | |
57. | John P. Rech, Priyanka Chaudhary, Michaela Schenkelberg, Danae Dinkel, A Convergent Mixed Methods Study to Explore Physical Activity Among Teachers and Children During the Preschool Day, 2023, 1082-3301, 10.1007/s10643-023-01486-8 | |
58. | Naureen Akber Ali Meghani, Joanne Hudson, Gareth Stratton, Jane Mullins, Deepak Sahoo, A multi-method feasibility trial of a multi-component behaviour change intervention to reduce sedentary behaviour and increase physical activity among ethnically diverse older adults, 2024, 14, 2044-6055, e084645, 10.1136/bmjopen-2024-084645 | |
59. | Avirup Roy, Hrishikesh Dutta, Amit Kumar Bhuyan, Subir Biswas, On-Device Semi-Supervised Activity Detection: A New Privacy-Aware Personalized Health Monitoring Approach, 2024, 24, 1424-8220, 4444, 10.3390/s24144444 | |
60. | Guilherme Moraes Balbim, Ryan S Falck, Nárlon Cássio Boa Sorte Silva, Arthur F Kramer, Michelle Voss, Teresa Liu-Ambrose, Lewis A Lipsitz, The Association of the 24-Hour Activity Cycle Profiles With Cognition in Older Adults With Mild Cognitive Impairment: A Cross-Sectional Study, 2024, 79, 1079-5006, 10.1093/gerona/glae099 | |
61. | Udeni Jayasinghe, Faustina Hwang, William S. Harwin, Inertial measurement data from loose clothing worn on the lower body during everyday activities, 2023, 10, 2052-4463, 10.1038/s41597-023-02567-4 | |
62. | Benjamin D. Maylor, Charlotte L. Edwardson, Alexandra M. Clarke-Cornwell, Melanie J. Davies, Nathan P. Dawkins, David W. Dunstan, Kamlesh Khunti, Tom Yates, Alex V. Rowlands, Physical Activity Assessed by Wrist and Thigh Worn Accelerometry and Associations with Cardiometabolic Health, 2023, 23, 1424-8220, 7353, 10.3390/s23177353 | |
63. | Zhi Cao, Jiahao Min, Yabing Hou, Keyi Si, Mingwei Wang, Chenjie Xu, Association of accelerometer-derived physical activity with all-cause and cause-specific mortality among individuals with cardiovascular diseases: a prospective cohort study, 2024, 2047-4873, 10.1093/eurjpc/zwae248 | |
64. | Li‐Tang Tsai, Eleanor Boyle, Sussi F Buhl, Gry Kock, Jan C Brønd, Marjolein Visser, Nuno Mendonça, Eric J Shiroma, Paolo Caserotti, Associations between appetite, physical activity and sedentary behaviour from hip‐ and wrist‐worn accelerometers in community‐dwelling older adults, 2023, 23, 1444-1586, 411, 10.1111/ggi.14588 | |
65. | Priyanka Chaudhary, John P Rech, Gaurav Kumar, Danae Dinkel, Perceived barriers and facilitators of childcare providers’ physical activity: A mixed-methods study, 2024, 83, 0017-8969, 137, 10.1177/00178969231222543 | |
66. | Inge de Wolf, Anne Elevelt, Femke van Nassau, Vera Toepoel, Ellen de Hollander, Maaike E. Kompier, Annemieke Luiten, Barry Schouten, G. C. Wanda Wendel-Vos, Hidde P. van der Ploeg, Comparing national device-based physical activity surveillance systems: a systematic review, 2024, 21, 1479-5868, 10.1186/s12966-024-01612-8 | |
67. | Kirkke Reisberg, Eva-Maria Riso, Liina Animägi, Jaak Jürimäe, Longitudinal Relationships of Physical Activity, Sedentary Time, Cardiorespiratory Fitness, and Muscular Fitness with Body Fatness in Preschoolers, 2024, 12, 2075-4663, 237, 10.3390/sports12090237 | |
68. | Kyle R. Leister, Sara E. Burke, Joon Young Kim, Victor H. Duenas, Tiago V. Barreira, Comparison of daily step count between the Fitbit Inspire 3 and the activPAL 3 in adults with transtibial amputation, 2024, 5, 2673-6861, 10.3389/fresc.2024.1331005 | |
69. | Iris Parés-Salomón, Anna M. Señé-Mir, Fernando Martín-Bozas, Bette Loef, Alan Coffey, Kieran P. Dowd, Guillem Jabardo-Camprubí, Karin I. Proper, Anna Puig-Ribera, Judit Bort-Roig, Effectiveness of workplace interventions with digital elements to reduce sedentary behaviours in office employees: a systematic review and meta-analysis, 2024, 21, 1479-5868, 10.1186/s12966-024-01595-6 | |
70. | Taylor McCoy, Anthony J. Sochan, Andrea M. Spaeth, The Relationship between Sleep and Physical Activity by Age, Race, and Gender, 2024, 25, 1530-6550, 10.31083/j.rcm2510378 | |
71. | Franziska Beck, Isabel Marzi, Alina Eisenreich, Selina Seemüller, Clara Tristram, Anne K. Reimers, Determination of cut-off points for the Move4 accelerometer in children aged 8–13 years, 2023, 15, 2052-1847, 10.1186/s13102-023-00775-4 | |
72. | S.A. Pomeshkina, E.A. Demchenko, Methods of sedentary lifestyle study: possibilities and limitations, 2024, 27, 2305-4948, 97, 10.17116/profmed20242705197 | |
73. | Agus Salim, Christian J. Brakenridge, Dulari Hakamuwa Lekamlage, Erin Howden, Ruth Grigg, Hayley T. Dillon, Howard D. Bondell, Julie A. Simpson, Genevieve N. Healy, Neville Owen, David W. Dunstan, Elisabeth A. H. Winkler, Detection of sedentary time and bouts using consumer-grade wrist-worn devices: a hidden semi-Markov model, 2024, 24, 1471-2288, 10.1186/s12874-024-02311-5 | |
74. | Andrea Weber, Daniela Schmid, Michael F. Leitzmann, 2023, Chapter 67-1, 978-1-4614-6625-3, 1, 10.1007/978-1-4614-6625-3_67-1 | |
75. | S. M. J. Janssen, M. A. Spruit, R. S. Djamin, J. C. C. M in ‘t Veen, H. A. C. van Helvoort, A. J. van ‘t Hul, Reliability of 24-h measurement of physical activity, sleep, and sedentary time in adult patients with asthma using a triaxial accelerometer, 2024, 0277-0903, 1, 10.1080/02770903.2024.2420758 | |
76. | Amie Wallman-Jones, Carina Nigg, Valentin Benzing, Mirko Schmidt, Leave the screen: The influence of everyday behaviors on self-reported interoception, 2023, 181, 03010511, 108600, 10.1016/j.biopsycho.2023.108600 | |
77. | Elyse Letts, Josephine S Jakubowski, Sara King-Dowling, Kimberly Clevenger, Dylan Kobsar, Joyce Obeid, Accelerometer techniques for capturing human movement validated against direct observation: a scoping review, 2024, 45, 0967-3334, 07TR01, 10.1088/1361-6579/ad45aa | |
78. | Songtao Lu, Jun Sun, Zhiguang Guo, Mingyu Yi, Yuheng Zhang, Jiali Wang, Yue Wang, Instrumented measures of sedentary behavior and physical activity are associated with depression among children and adolescents: a systematic review and dose–response meta-analysis of observational studies, 2024, 15, 1664-1078, 10.3389/fpsyg.2024.1465974 | |
79. | Anis Davoudi, Jacek K. Urbanek, Lacey Etzkorn, Romil Parikh, Elsayed Z. Soliman, Amal A. Wanigatunga, Kelley Pettee Gabriel, Josef Coresh, Jennifer A. Schrack, Lin Yee Chen, Validation of a Zio XT Patch Accelerometer for the Objective Assessment of Physical Activity in the Atherosclerosis Risk in Communities (ARIC) Study, 2024, 24, 1424-8220, 761, 10.3390/s24030761 | |
80. | Arto J. Pesola, Samad Esmaeilzadeh, Pirjo Hakala, Nina Kallio, Päivi Berg, Marko Havu, Tiina Rinne, Sensitivity and specificity of measuring children's free-living cycling with a thigh-worn Fibion® accelerometer, 2023, 5, 2624-9367, 10.3389/fspor.2023.1113687 | |
81. | Johan Y. Y. Ng, Joni H. Zhang, Stanley S. Hui, Guanxian Jiang, Fung Yau, James Cheng, Amy S. Ha, Yaodong Gu, Development of a multi-wear-site, deep learning-based physical activity intensity classification algorithm using raw acceleration data, 2024, 19, 1932-6203, e0299295, 10.1371/journal.pone.0299295 | |
82. | Julie Riopel-Meunier, Paul Poirier, Jean-Pierre Després, Marie-Eve Piché, Is the time right for preventive cardiology guidelines on sedentary behaviors and sitting time?, 2024, 0828282X, 10.1016/j.cjca.2024.12.024 | |
83. | Kimberly A. Clevenger, Melitta A. McNarry, Kelly A. Mackintosh, Karin A. Pfeiffer, M. Benjamin Nelson, Joshua M. Bock, Mary T. Imboden, Leonard A. Kaminsky, Alexander H.K. Montoye, A Consensus Method for Estimating Moderate to Vigorous Physical Activity Levels in Adults Using Wrist-Worn Accelerometers, 2024, 7, 2575-6605, 10.1123/jmpb.2024-0013 | |
84. | Brandon D. Willingham, Marcos Daou, Jake VanArsdale, Meghan Thomas, Patrick G. Saracino, Energy Availability in Female Collegiate Beach Volleyball Athletes, 2024, 1064-8011, 10.1519/JSC.0000000000004884 | |
85. | Dawei Wang, Yuheng Zhang, Zhiguang Guo, Songtao Lu, Sedentary behavior and physical activity are associated with risk of depression among adult and older populations: a systematic review and dose–response meta-analysis, 2025, 16, 1664-1078, 10.3389/fpsyg.2025.1542340 | |
86. | Barbara Engels, Manon A. T. Bloemen, Richard Felius, Karlijn Damen, Eline A. M. Bolster, Harriët Wittink, Raoul H. H. Engelbert, Jan Willem Gorter, Monitoring of child-specific activities in ambulatory children with and without developmental disabilities, 2025, 25, 1471-2431, 10.1186/s12887-025-05489-9 | |
87. | Arto J. Pesola, Marko Havu, Automated Algorithm for Accurate Waking Sitting and Physical Activity Estimates Without Diaries Using Thigh-Worn Fibion Accelerometers in 10- to 12-Year-Old Children, 2025, 8, 2575-6605, 10.1123/jmpb.2024-0022 | |
88. | Reagan E. Garcia, Anne B. Newman, Eileen Johnson, Yujia Susanna Qiao, Peggy M. Cawthon, Barbara J. Nicklas, Bret H. Goodpaster, Nancy W. Glynn, Using Wearable Devices to Examine the Associations of Sedentary Behavior with Perceived and Performance Fatigability Among Older Adults: The Study of Muscle, Mobility and Aging (SOMMA), 2025, 25, 1424-8220, 2722, 10.3390/s25092722 |
Reference Author(s), Year, Title, Journal |
Hypotheses/Study Aims | Research Design, Sample Size, Ages, Country | Measures | Primary Findings | Limitations | Strengths |
Cabanas-Sanchez, Guallar-Castillon et al., (2018); Physical activity, sitting time and mortality from inflammatory disease in older adults. Frontiers in Physiology | Study focused on infectious and non-infectious inflammatory causes. | Prospective Cohort study (N = 3,667) Men and women ages 60 years and older in Spain |
Sedentary data were self-reported. Mortality identified using the National Death Index. |
In nonlinear models, those who were sedentary > 7 hours per day had a higher mortality risk for total inflammatory and non-infectious inflammatory causes. Relationships were stronger for those who were the least active. | Sedentary time and physical activity were self-reported. No dietary data were collected. Inflammatory disease definition may not encompass all related conditions. |
Prospective cohort study Spanish population National database to confirm deaths Focus on mortality due to inflammatory and non-inflammatory causes |
Ensrud, Blackwell, Cauley et al., (2014); Objective measures of activity level and mortality in older men. Journal of the American Geriatrics Society | Study focused on developing objective measures of activity level for older adult men, related to all-cause mortality. | Prospective Cohort study (N = 2,918) Men ages 71 years and older in the United States |
Accelerometer determined sedentary behavior (ACTi Graph) Deaths confirmed via death certificate and follow-up calls. |
In adjusted models, men in the highest quartile for sedentary time had a higher risk for all-cause mortality. Subsequent analysis indicated that time spent sedentary was associated with non-cancer and non-CVD deaths. | Associations for women or racial and ethnic minority populations not determined | Objective assessment of behavior Cohort study Disease specific mortality |
Jefferis, Parsons, Sartini et al., (2018); Objectively measured physical activity, sedentary behavior and all-cause mortality in older men: Does volume of activity matter more than pattern of accumulation? Br J Sports Med | To examine the association between accelerometer determined sedentary behavior, physical activity, and all-cause mortality | Prospective Cohort study (N = 1,655) Men ages 71–92years old in the United Kingdom |
Accelerometer determined sedentary behavior (ACTi Graph) National database confirmed mortality |
In adjusted models, those in the highest quartile for sedentary time had a higher risk for all-cause mortality when compared to men in the lowest quartile. | No measure of postural allocation Associations for women or racial and ethnic minority populations not determined |
Prospective cohort study. An objective measure of sedentary behavior |
Klenk, Dallmeier, Denkinger et al., (2016); Objectively measured walking duration and sedentary behavior and four-year mortality in older people. Plos ONE | This study examined the effect of walking and sedentary time on mortality. | Randomly and geographically selected cohort of older adults in Germany (N = 1,271) aged 65 years or greater. | Physical activity levels were accelerometer determined sedentary behavior (ActivPAL) Mortality determined via local registration offices |
Sedentary duration association between sedentary time and mortality diminished in fully adjusted models. | Limited follow-up period Slower walking speeds may have different outcomes |
Use of ActivePAL to measure sedentary behavior |
Leon-Munoz, Martinez-Gomez, Balboa-Castillo et al., (2013); Continued sedentariness, change in sitting time, and mortality in older adults. Med Sci Sports Exerc | To examine the association between continued sedentary time and changes in sedentary time with mortality | Prospective Cohort (N = 2,635) of adults aged 60 years and older. | Self-reported sedentary time in different activities National Death Index confirmed deaths |
Those who were consistently sedentary had higher mortality rates when compared to those who were newly sedentary, formally sedentary, and consistently non-sedentary. Associations maintained in different SES strata. | Self-reported questionnaires Other factors may be related to mortality | Prospective cohort study Mortality confirmed via National Death Index. Analysis in different SES strata |
Pavey, Peeters, Brown (2015); Sitting-time and 9-year all-cause mortality in older women. Br J Sports Med | This study looked at the relationship between total sitting-time and all-cause mortality in older women. | Prospective cohort study (N = 6,656) of Australian Women ages 76–90. | Self-reported questionnaires Mortality data confirmed by the Australian National Death Index. |
In fully adjusted models there was a dose-response relationship between time spent sitting and mortality. However, only the p-value for the trend was significant. | Other factors may be related to mortality. Self-reported questionnaires |
Confirmed morality data Prospective cohort design |
Reference Author(s), Year, Title, Journal |
Hypotheses/Study Aims | Research Design, Sample Size, Ages, Country | Measures | Primary Findings | Limitations | Strengths |
Ekblom, Ö., Ekblom-Bak, E., Rosengren, A., Hallsten, M., Bergström, G., Börjesson, M., & Lazzeri, C. (2015); Cardiorespiratory fitness, sedentary behavior and physical activity are independently associated with the metabolic syndrome, Results from the SCAPIS Pilot Study. PLoS ONE | Examine the association between sedentary behavior and metabolic syndrome | Random stratified sample of adults aged 50 to 65 years living in Sweden (N = 1111) | Accelerometer determined sedentary behavior Serum samples used to detect metabolic syndrome |
In adjusted models, the highest quartile for sedentary time had higher odds for metabolic syndrome when compared to the first quartile. Sedentary time increased the odds of a larger waist circumference and higher triglyceride, and HDL cholesterol levels. | Cross-sectional design Possibility of multi-collinearity |
Adjusted for dietary intake and fitness Accelerometer determined sedentary behavior Random stratified sample |
Joseph, J., Echouffo-Tcheugui, J., Golden, S., Chen, H., Jenny, N., Carnethon, M., Bertoni, A. (2016); Physical activity, sedentary behaviors and the incidence of type 2 diabetes mellitus: The Multi-Ethnic Study of Atherosclerosis (MESA). BMJ Open Diabetes Research & Care | Examine the association between sedentary behavior and diabetes | Prospective cohort (N = 5829) of men and women from the United States aged 45–84 years old | Incident type II diabetes determined by fasting glucose Self-reported sedentary time |
In adjusted models, both television time and total sedentary behavior increased the odds for type II diabetes. Possible effect modification by family history of diabetes, race, and BMI were observed. | Physical activity and sedentary behaviors were self-reported, and the time frame queried was their typical activities within the last month. Therefore, discrepancies with actual activity levels may exist and self-reported sedentary behavior | Multiethnic population Serum-based biomarkers |
Wirth, K., Klenk, J., Brefka, S., Dallmeier, D., Faehling, K., Roqué, M., Denkinger, M. (2017); Biomarkers associated with sedentary behavior in older adults: A systematic review. Ageing Research Reviews | Patho-mechanisms of sedentary behavior (SB) are unclear. We conducted a systematic review to investigate the associations between SB and various biomarkers in older adults. | Electronic databases were searched (MEDLINE, EMBASE, CINAHL, AMED) up to July 2015 to identify studies with objective or subjective measures of SB, sample size ≥ 50, mean age ≥ 60years and accelerometer wear time ≥ 3days. Methodological quality was appraised with the CASP tool. | Randomized controlled trials found a positive correlation for SB with BMI, neck circumference, fat mass, HbA1C, cholesterol and insulin levels, cohort studies additionally for waist circumference, leptin, C-peptide, and low-density lipoprotein and a negative correlation for HDL. | The final sample (26 articles) 63 biomarkers were detected. Most investigated markers were body mass index (BMI, n = 15), waist circumference (WC, n = 15), blood pressure (n = 11), triglycerides (n = 12) and high-density lipoprotein (HDL, n = 15). Some inflammation markers were identified such as interleukin-6, C-reactive protein or tumor necrosis factor alpha. There was a lack of renal, muscle or bone biomarkers. | First, we identified relatively few high quality or longitudinal studies investigating biomarkers specifically in older adults. Therefore, we were not able to conduct a meta-analysis as we anticipated. | The highlighted results of the four “risk population” studies showed associations for SB with biomarkers in the same direction as the studies performed in non-risk populations. |
Figueiró, T. H., Arins, G., Santos, C., Cembranel, F., Medeiros, P. A., d'Orsi, E., & Rech, C. R. (2019); Association of objectively measured sedentary behavior and physical activity with cardiometabolic risk markers in older adults. Plos ONE | Examine the association between sedentary behavior and cardiometabolic markers | Cross-sectional study of Brazilians adults (N = 425) aged 60 years and older | Accelerometer determined sedentary behavior Waist circumference, blood pressure, and plasma-based cardio-metabolic markers |
Sedentary behavior negatively and linearly associated with systolic blood pressure and HDL cholesterol. Physically inactive and sedentary individuals had greater waist circumference and lower HDL cholesterol levels than active, non-sedentary subjects. | Cross-sectional study design Confounding variables (i.e., medications and BMI) not included in the analysis |
Objectively measured sedentary time Plasma-based biomarkers |
Aadahl, M., Linneberg, A., Møller, T., Rosenørn, S., Dunstan, D., Witte, D., & Jørgensen, T. (2014); Motivational counseling to reduce sitting time: A community-based randomized controlled trial in adults. American Journal of Preventive Medicine | Examine the effectiveness of a sedentary behavior intervention | Randomized trial of 166 adults aged adults aged 18–69 years old from Denmark | Objectively measured overall sitting time; secondary measures were breaks in sitting time; anthropometric Accelerometer determined sedentary behavior (ActivPAL Body fat percentage determined by Tanita BC-420MA Serum measures of insulin homeostasis model assessment, hepatic insulin resistance, and basil insulin secretion, glycosylated hemoglobin, total cholesterol, LDL and HDL cholesterol, and triglycerides |
The reduction in sedentary time did not differ between groups. The intervention group had greater improvements in standing time than the control group. Greater improvements in insulin resistance was observed in the intervention condition when compared to the control group. No other differences were observed. | No dietary information was included Uneven sample size in the intervention and control condition. |
Accelerometer determined sedentary behavior Serum biological markers Randomized design |
Reference Author(s), Year, Title, Journal |
Hypotheses/Study Aims | Research Design, Sample Size, Ages, Country | Measures | Primary Findings | Limitations | Strengths |
Chang & Sok (2015); Predictors of sedentary behavior in elderly Koreans with hypertension, The Journal of Nursing Research | Examine predictors of sedentary behavior | Cross-sectional study (N = 306) of Korean adults aged 65 years and older | Self-reported sedentary behavior Psychosocial variables (i.e., empowerment, perceived health, depression, self-efficacy, and social support for physical activity) |
Stepwise regression analysis indicated that empowerment, duration since HBP diagnosis, perceived health, vigorous-intensity physical activity, and depressive symptoms were determinants of sedentary behavior. | Self-reported measures of sedentary behavior Cross-sectional design |
Validated measures |
Reference Author(s), Year, Title, Journal |
Hypotheses/Study Aims | Research Design, Sample Size, Ages | Measures | Primary Findings | Limitations | Strengths |
Bann, Hire, Manini et al. (2015); Light intensity physical activity and sedentary behavior in relation to body mass index and grip strength in older adults: cross-sectional findings from the lifestyle interventions and independence for elders (LIFE) study. Plos ONE | Examine the association between sedentary behavior, BMI, and grip strength. | Cross-sectional study (N = 1,130) of adults aged 70–89 living in the United States. | Accelerometer determined sedentary time Jamar determined grip strength BMI and waist circumference measured by trained staff |
In adjusted models, sedentary behavior was associated with BMI. Less time spent sedentary and more time spent in light-intensity physical activities was associated with BMI | Cross sectional design | Use of BMI as a measure of body size |
Gennuso, Gangnon Matthews (2013); Sedentary behavior, physical activity, and markers of health in older adults. Medicine and science in sports and exercise | Examine the association between sedentary behavior, physical activity, and cardiometabolic markers | Population based cross-sectional study (N = 1914) of adults aged 65 years and older living in the United States | Accelerometer determined sedentary behavior Cardiometabolic markers (total, HDL, and LDL cholesterol, glucose, triglycerides, glycohemoglobin, waist circumference, blood pressure, and C-reactive protein) Physical function |
In adjusted models, higher quartiles of sedentary behavior were associated with weight, BMI, waist circumference, systolic blood pressure, and C-reactive protein among participants who were insufficiently active older adults. Among sufficiently active older adults, higher quartiles of sedentary behavior were associated with waist circumference and LDL cholesterol levels. In addition, insufficiently active older adults who were less sedentary had lower odds of functional limitations. | Cross-sectional design | Accelerometer determined sedentary behavior Population based sample Serum cardiometabolic markers |
Figueiró, T. H., Arins, G., Santos, C., Cembranel, F., Medeiros, P. A., d'Orsi, E., & Rech, C. R. (2019); Association of objectively measured sedentary behavior and physical activity with cardiometabolic risk markers in older adults. Plos ONE | Examine the association between sedentary behavior and cardiometabolic markers | Cross-sectional study of Brazilians adults (N = 425) aged 60 years and older | Accelerometer determined sedentary behavior Waist circumference, blood pressure, and plasma based cardiometabolic markers |
Sedentary behavior was negatively and linearly associated with systolic blood pressure and HDL cholesterol. Physically inactive and sedentary individuals had greater waist circumference and lower HDL cholesterol levels than active, non-sedentary subjects. | Cross-sectional study design Confounding variables (i.e., medications and BMI) not included in the analysis |
Objectively measured sedentary time Plasma-based biomarkers |
Reference Author(s), Year, Title, Journal |
Hypotheses/Study Aims | Research Design, Sample Size, Ages | Measures | Primary Findings | Limitations | Strengths |
Bann, Hire, Manini et al. (2015); Light intensity physical activity and sedentary behavior in relation to body mass index and grip strength in older adults: cross-sectional findings from the lifestyle interventions and independence for elders (LIFE) study. Plos ONE | Examine the association between sedentary behavior, BMI, and grip strength. | Cross-sectional study (N = 1,130) of adults aged 70–89 living in the United States. | Accelerometer determined sedentary time Jamar determined grip strength BMI and waist circumference measured by trained staff |
In adjusted models, sedentary behavior was associated with BMI. Less time spent sedentary and more time spent in light-intensity physical activities were associated with BMI | Cross sectional design | Use of BMI as a measure of body size |
Gennuso, Gangnon, Matthews (2013); Sedentary behavior, physical activity, and markers of health in older adults. Medicine and science in sports and exercise | Examine the association between sedentary behavior, physical activity, and cardiometabolic markers | Population based cross-sectional study (N=1914) of adults aged 65 years and older living in the United States | Accelerometer determined sedentary behavior Cardiometabolic markers (total, HDL, and LDL cholesterol, glucose, triglycerides, glycohemoglobin, waist circumference, blood pressure, and C-reactive protein) Physical function |
In adjusted models, higher quartiles of sedentary behavior were associated with weight, BMI, waist circumference, systolic blood pressure, and C-reactive protein among participants who were insufficiently active older adults. Among sufficiently active older adults, higher quartiles of sedentary behavior were associated with waist circumference and LDL cholesterol levels. In addition, insufficiently active older adults who were less sedentary had lower odds of functional limitations. | Cross-sectional design |
Accelerometer determined sedentary behavior Population based sample Serum cardiometabolic markers |
Judice, Silva, & Sardinha (2015); Sedentary bout durations are associated with abdominal obesity in older adults. The Journal of Nutrition, Health & Aging | Examine the association between sedentary time and patterns of abdominal obesity | Cross-sectional study (N = 351) of older adults (aged 65–103 years) from Portugal | Accelerometer determined sedentary behavior Waist circumference measured by trained staff |
No association was observed with total sedentary time and abdominal obesity. However, for bouts of sedentary time that ranged from 10–20 minutes up to <60 minutes, each bout significantly increased the odds of abdominal obesity. | Cross sectional design Dietary variables not considered in models |
Objectively measured sedentary time |
Reference Author(s), Year, Title, Journal |
Hypotheses/Study Aims | Research Design, Sample Size, Ages, Country | Measures | Primary Findings | Limitations | Strengths |
van Alphen et al. (2016); Older adults with dementia are sedentary for most of the day. Plos ONE | To objectively assess the physical activity levels of community dwelling and institutionalized ambulatory patients with dementia and to compare with the physical activity levels of cognitive healthy older adults. | The research design was a longitudinal study that examined the effects of regular physical activity on the health of dementia patients; participants were recruited via medical staff of aged care facilities. There were 83 institutionalized [mean age 83.0 (7.6)] and 37 community-dwelling dementia patients [mean age 77.3 (5.6)], and 26 healthy older adults [mean age 79.5 (5.6)]. The study was conducted in The Netherlands. | Actigraphy was used to assess physical activity levels that were based on the raw data. Sedentary behavior was classified < 100 counts/min. | Institutionalized vs. community-dwelling dementia patients had 23.5% lower daily physical activity levels (p = .004) and spent 9.3% more time in sedentary behavior (p = .032). Community-dwelling dementia patients vs healthy older adults' daily physical activity levels and sedentary time were 21.6% lower (p = .007) and 8.9% longer (p = .078). | One limitation is that all activity counts per minute represent the same physical activity intensity for healthy as well as adults with dementia. Another limitation is the selection procedure of institutions and daycare centers and homes based on existing collaborations. | The first study to objectively characterize institutionalized and community-dwelling dementia patients' physical activity and sedentary behavior levels. |
Hartman et al. (2018); Dementia patients are more sedentary and less physically active than age-and sex-matched cognitively healthy older adults. Dementia Geriatric Cognitive Disorders | To examine physical activity and sedentary behavior characteristics of ambulatory and community-dwelling patients with dementia compared to cognitively healthy age-, sex- and weight-matched controls. | Cross-sectional study - persons with a dementia diagnosis aged > 60 years who were ambulatory and community dwelling were included. (n = 45, age 79.6 ± 5.9 years), and matched controls (n = 49, age 80.0 ± 7.7 years). Cognitively healthy controls were age, sex, and weight matched to dementia patients and had no history of cognitive impairment. The study was conducted in The Netherlands. | Dementia diagnosis was based on comprehensive clinical assessment by a physician. Mini-Mental State Examination indicated severity of cognitive impairment. Participants wore a wrist accelerometer for 7 days to assess sedentary time, sedentary bout duration and time spent in very light, light-to-moderate and moderate-to-vigorous physical activities. |
Relative sedentary time and sedentary bout duration were significantly greater in dementia patients than in controls. In addition, dementia patients spent a lower percentage of their waking time in light-to-moderate and moderate-to-vigorous intensity physical activities. Secondly, older age was associated with a decline in physical activity and increase in sedentary behavior in controls; however, no such age-related changes were found in dementia patients. | The design of the study could not answer the question whether differences in physical activity and sedentary behavior are simply a consequence of dementia. Only community-dwelling patients were included; therefore, results cannot be generalized to institutionalized dementia patients. Furthermore, a potential bias related to the study enrollment should be considered. Dementia patients in the study were enrolled in an exercise trial. | An accelerometer is a validated measure of physical activity and sedentary behavior. A novel finding was that differences between dementia patients and controls remain when corrected for sleep time. |
Reference Author(s), Year, Title, Journal |
Hypotheses/Study Aims | Research Design, Sample Size, Ages, Country | Measures | Primary Findings | Limitations | Strengths |
Engeroff et al. (2018); Is objectively assessed sedentary behavior, physical activity and cardiorespiratory fitness linked to brain plasticity outcomes in old age? Neuroscience | Examine the association between brain plasticity outcomes and brain-derived neurotrophic factor | Cross-sectional study (N = 50) of older adults (aged 73–77 years old) in Germany | Accelerometer determined sedentary behavior Serum markers of brain metabolism and brain-derived neurotrophic factors (BDNF) Cardiorespiratory fitness |
Sedentary time was associated with BDNF, but not brain metabolism measures | Small sample Cognitively healthy sample |
Well controlled study Novel methods |
Falck et al. (2017); Cross-sectional relationships of physical activity and sedentary behavior with cognitive function in older adults with probable mild cognitive impairment. Physical Therapy |
To examine differences in physical activity and sedentary behavior between people with probable mild cognitive impairment and people without mild cognitive impairment and to assess whether associations of physical activity and sedentary behavior with cognitive function differed by mild cognitive impairment status. | The study was cross sectional. The sample size was 150. The mean age was 71.11 years old (7.22). The location was Vancouver, British Columbia, Canada. | Physical activity and sedentary behavior in adults dwelling in the community were measured using a wrist-worn actigraphy unit. The Montreal Cognitive Assessment categorized participants with probable mild cognitive impairment and participants without the condition. Cognitive function was indexed using the Alzheimer Disease Assessment Scale-Cognitive-Plus. | Participants with probable mild cognitive impairment had lower physical activity and higher sedentary behavior than participants without mild cognitive impairment. Higher physical activity and lower sedentary behavior were associated with better Alzheimer Disease Assessment Scale-Cognitive-Plus performance in participants without mild cognitive impairment but not in participants with probable mild cognitive impairment. | This study was cross-sectional and therefore causal associations should not be inferred. In addition, the diagnosis of mild cognitive impairment was not confirmed with a physician. | First study to report that older adults with probable mild cognitive impairment were less active and more sedentary than their cognitively healthy peers. Objective assessments of physical activity and sedentary behavior. |
Walle'n et al. (2015); Levels and patterns of physical activity and sedentary behavior in elderly people with mild to moderate Parkinson's disease. Physical Therapy | To describe levels and patterns of physical activity and sedentary behaviors in elderly people with mild to moderate Parkinson's Disease. | This cross-sectional study involved a free-living setting and 53 men and 42 women (mean age 73.4 years; (SD-5.7 years) with mild to moderate idiopathic Parkinson's Disease. The country was Sweden. | Time spent in physical activity and sedentary behaviors were assessed for one week with accelerometers. | The main finding was that approximately 75% of all time awake was spent in sedentary behaviors; 18% was spent in physical activity. The proportion of individuals meeting the goal of achieving 150 minutes of moderate- to vigorous-intensity PA per week was 27%; 16% achieved 7,000 or more steps per day; participants spent 589 minutes daily in sedentary behaviors. | The participants were not randomly selected; therefore, generalizability is limited. | Based on objective assessment (accelerometers), described the levels and patterns of physical activity, including diurnal patterns, and estimated the extent to which physical activity recommendations were met. |
Nemoto et al. (2018); The association of single and combined factors of sedentary behavior and physical activity with subjective cognitive complaints among community-dwelling older adults: Cross-sectional study. Plos ONE | To examine the association of type of sedentary behavior, physical activity, or their combination with subjective cognitive complaints among community dwelling older adults. | The cross-sectional study targeted independently living individuals aged 65 years and older who resided in Tsuru, Yamanashi Prefecture, Japan (n = 5,328). | Participants responded to the National Functional Survey Questionnaire (Kihon checklist) to assess subjective cognitive complaints. Do your family or friends point out your memory loss? Do you make a call by looking up phone numbers? Do you find yourself not knowing today's date? To evaluate physical activity, the Japanese version of the International Physical Activity Questionnaire Short-version (IPAQ-SV) was conducted. Sedentary behavior time was assessed as subjective average duration of television viewing and reading books or newspapers during the last seven days. | The primary findings were that moderate-to-vigorous intensity physical activity was significantly related with a lower risk of subjective cognitive complaints and that reading showed a dose-response relationship with subjective cognitive complaints. The combined group who reported >150 min/week physical activity and >30 min/day reading showed 60% lower subjective cognitive complaints than the combined group who reported <150 min/week physical activity and <10 min/day reading. | The cross-sectional design limits causal inferences. Physical activity and sedentary behavior were assessed subjectively. Participants who developed dementia or mild cognitive impairment during the study were not necessarily completely excluded. | First study to report the association of the combined effects of sedentary time and physical activity with subjective cognitive complaints; demographic variables, health behavior, and health status were included in the analyses. The data were from a complete survey with a high response rate of 79.8% and missing values were accounted for by multiple imputation. |
Reference Author(s), Year, Title, Journal |
Hypotheses/Study Aims | Research Design, Sample Size, Ages, Country | Measures | Primary Findings | Limitations | Strengths |
Okely et al. (2019); Positive and negative well-being and objectively measured sedentary behavior in older adults: evidence from three cohorts. BMC Geriatrics | To examine the association between sedentary behavior and both positive and negative effect | Three prospective (N = 698) of older adults in Scotland | Accelerometer determined sedentary behavior (ActivPAL) Validated scales for positive and negative effect, depression, and anxiety |
Sedentary behavior was associated with depression symptoms | Waking time was self-reported | Accelerometer determined sedentary behavior Validated measures of mental health |
Ku et al. (2015); Leisure-time physical activity, sedentary behaviors and subjective well-being in older adults: An eight-year longitudinal research. Social Indicators Research | Examine the association between self-reported sedentary time and subjective well-being | Longitudinal fixed cohort (N = 1,268) of adults aged 70 years or older in Taiwan. | Self-reported leisure time in sedentary behavior Subjective well-being (i.e., Life Satisfaction Index) |
Television viewing, social chatting, reading, and listening to the radio were significantly associated with subjective well-being | Self-reported sedentary time | Longitudinal design |
Reference Author(s), Year, Title, Journal |
Hypotheses/Study Aims | Research Design, Sample Size, Ages, Country | Measures | Primary Findings | Limitations | Strengths |
Maréchal et al. (2019); Effect of a mixed-exercise program on physical capacity and sedentary behavior in older adults during cancer treatments. Aging Clinical and Experimental Research | To examine the impact of an intervention on increasing physical activity and reducing sedentary behavior during cancer treatment | Two-group (i.e., mixed exercise vs. stretching) pre- and post-test design (N = 14) of older patients in Canada | Self-reported sedentary time Senior fitness test – functional performance test (i.e., chair stands, Up and Go, Arm Curl, Sit and Reach, and 6-minute walk test) |
Mixed exercise group had greater improvements in chair stands and global physical capacity scores when compared to those in the stretching group. No other differences were observed | Small sample Sedentary behavior was not the focus |
Objective assessments of functional performance |
Research design | Reference | Quality assessment from National Institute of Health ratings criteria (good, fair, poor) |
Observational Cohort and Cross-Sectional Studies | ||
Bann et al. 2015 | Good | |
Benka Wallén et al. 2015 | Good | |
Cabanas-Sanchez et al. 2018 | Fair | |
Chang et al. 2015 | Fair | |
Ekblom et al. 2015 | Good | |
Engeroff et al. 2018 | Fair | |
Ensrud et al. 2014 | Good | |
Figueiró et al. 2019 | Good | |
Gennuso et al. 2013 | Good | |
Gennuso et al. 2015 | Good | |
Jefferis et al. 2019 | Good | |
Joseph et al. 2016 | Good | |
Judice et al. 2015 | Good | |
Klenk et al. 2016 | Good | |
Ku et al. 2016 | Good | |
Leon-Munoz et al. 2013 | Good | |
Matthews et al. 2012 | Good | |
Nemoto et al. 2018 | Good | |
Okely et al. 2019 | Good | |
Pavey et al. 2015 | Good | |
Before-After (Pre-Post) Studies with No Control Group | ||
Hartman et al. 2018 | Fair | |
Case-Control Studies | ||
Falck et al. 2017 | Fair | |
van Alphen et al. 2016 | Fair | |
Controlled Intervention Studies | ||
Aadahl et al. 2019 | Fair | |
Maréchal et al. 2019 | Poor | |
Systematic Reviews and Meta-Analyses | ||
Thorp et al. 2011 | Fair | |
Wirth et al. 2017 | Good |
Reference Author(s), Year, Title, Journal |
Hypotheses/Study Aims | Research Design, Sample Size, Ages, Country | Measures | Primary Findings | Limitations | Strengths |
Cabanas-Sanchez, Guallar-Castillon et al., (2018); Physical activity, sitting time and mortality from inflammatory disease in older adults. Frontiers in Physiology | Study focused on infectious and non-infectious inflammatory causes. | Prospective Cohort study (N = 3,667) Men and women ages 60 years and older in Spain |
Sedentary data were self-reported. Mortality identified using the National Death Index. |
In nonlinear models, those who were sedentary > 7 hours per day had a higher mortality risk for total inflammatory and non-infectious inflammatory causes. Relationships were stronger for those who were the least active. | Sedentary time and physical activity were self-reported. No dietary data were collected. Inflammatory disease definition may not encompass all related conditions. |
Prospective cohort study Spanish population National database to confirm deaths Focus on mortality due to inflammatory and non-inflammatory causes |
Ensrud, Blackwell, Cauley et al., (2014); Objective measures of activity level and mortality in older men. Journal of the American Geriatrics Society | Study focused on developing objective measures of activity level for older adult men, related to all-cause mortality. | Prospective Cohort study (N = 2,918) Men ages 71 years and older in the United States |
Accelerometer determined sedentary behavior (ACTi Graph) Deaths confirmed via death certificate and follow-up calls. |
In adjusted models, men in the highest quartile for sedentary time had a higher risk for all-cause mortality. Subsequent analysis indicated that time spent sedentary was associated with non-cancer and non-CVD deaths. | Associations for women or racial and ethnic minority populations not determined | Objective assessment of behavior Cohort study Disease specific mortality |
Jefferis, Parsons, Sartini et al., (2018); Objectively measured physical activity, sedentary behavior and all-cause mortality in older men: Does volume of activity matter more than pattern of accumulation? Br J Sports Med | To examine the association between accelerometer determined sedentary behavior, physical activity, and all-cause mortality | Prospective Cohort study (N = 1,655) Men ages 71–92years old in the United Kingdom |
Accelerometer determined sedentary behavior (ACTi Graph) National database confirmed mortality |
In adjusted models, those in the highest quartile for sedentary time had a higher risk for all-cause mortality when compared to men in the lowest quartile. | No measure of postural allocation Associations for women or racial and ethnic minority populations not determined |
Prospective cohort study. An objective measure of sedentary behavior |
Klenk, Dallmeier, Denkinger et al., (2016); Objectively measured walking duration and sedentary behavior and four-year mortality in older people. Plos ONE | This study examined the effect of walking and sedentary time on mortality. | Randomly and geographically selected cohort of older adults in Germany (N = 1,271) aged 65 years or greater. | Physical activity levels were accelerometer determined sedentary behavior (ActivPAL) Mortality determined via local registration offices |
Sedentary duration association between sedentary time and mortality diminished in fully adjusted models. | Limited follow-up period Slower walking speeds may have different outcomes |
Use of ActivePAL to measure sedentary behavior |
Leon-Munoz, Martinez-Gomez, Balboa-Castillo et al., (2013); Continued sedentariness, change in sitting time, and mortality in older adults. Med Sci Sports Exerc | To examine the association between continued sedentary time and changes in sedentary time with mortality | Prospective Cohort (N = 2,635) of adults aged 60 years and older. | Self-reported sedentary time in different activities National Death Index confirmed deaths |
Those who were consistently sedentary had higher mortality rates when compared to those who were newly sedentary, formally sedentary, and consistently non-sedentary. Associations maintained in different SES strata. | Self-reported questionnaires Other factors may be related to mortality | Prospective cohort study Mortality confirmed via National Death Index. Analysis in different SES strata |
Pavey, Peeters, Brown (2015); Sitting-time and 9-year all-cause mortality in older women. Br J Sports Med | This study looked at the relationship between total sitting-time and all-cause mortality in older women. | Prospective cohort study (N = 6,656) of Australian Women ages 76–90. | Self-reported questionnaires Mortality data confirmed by the Australian National Death Index. |
In fully adjusted models there was a dose-response relationship between time spent sitting and mortality. However, only the p-value for the trend was significant. | Other factors may be related to mortality. Self-reported questionnaires |
Confirmed morality data Prospective cohort design |
Reference Author(s), Year, Title, Journal |
Hypotheses/Study Aims | Research Design, Sample Size, Ages, Country | Measures | Primary Findings | Limitations | Strengths |
Ekblom, Ö., Ekblom-Bak, E., Rosengren, A., Hallsten, M., Bergström, G., Börjesson, M., & Lazzeri, C. (2015); Cardiorespiratory fitness, sedentary behavior and physical activity are independently associated with the metabolic syndrome, Results from the SCAPIS Pilot Study. PLoS ONE | Examine the association between sedentary behavior and metabolic syndrome | Random stratified sample of adults aged 50 to 65 years living in Sweden (N = 1111) | Accelerometer determined sedentary behavior Serum samples used to detect metabolic syndrome |
In adjusted models, the highest quartile for sedentary time had higher odds for metabolic syndrome when compared to the first quartile. Sedentary time increased the odds of a larger waist circumference and higher triglyceride, and HDL cholesterol levels. | Cross-sectional design Possibility of multi-collinearity |
Adjusted for dietary intake and fitness Accelerometer determined sedentary behavior Random stratified sample |
Joseph, J., Echouffo-Tcheugui, J., Golden, S., Chen, H., Jenny, N., Carnethon, M., Bertoni, A. (2016); Physical activity, sedentary behaviors and the incidence of type 2 diabetes mellitus: The Multi-Ethnic Study of Atherosclerosis (MESA). BMJ Open Diabetes Research & Care | Examine the association between sedentary behavior and diabetes | Prospective cohort (N = 5829) of men and women from the United States aged 45–84 years old | Incident type II diabetes determined by fasting glucose Self-reported sedentary time |
In adjusted models, both television time and total sedentary behavior increased the odds for type II diabetes. Possible effect modification by family history of diabetes, race, and BMI were observed. | Physical activity and sedentary behaviors were self-reported, and the time frame queried was their typical activities within the last month. Therefore, discrepancies with actual activity levels may exist and self-reported sedentary behavior | Multiethnic population Serum-based biomarkers |
Wirth, K., Klenk, J., Brefka, S., Dallmeier, D., Faehling, K., Roqué, M., Denkinger, M. (2017); Biomarkers associated with sedentary behavior in older adults: A systematic review. Ageing Research Reviews | Patho-mechanisms of sedentary behavior (SB) are unclear. We conducted a systematic review to investigate the associations between SB and various biomarkers in older adults. | Electronic databases were searched (MEDLINE, EMBASE, CINAHL, AMED) up to July 2015 to identify studies with objective or subjective measures of SB, sample size ≥ 50, mean age ≥ 60years and accelerometer wear time ≥ 3days. Methodological quality was appraised with the CASP tool. | Randomized controlled trials found a positive correlation for SB with BMI, neck circumference, fat mass, HbA1C, cholesterol and insulin levels, cohort studies additionally for waist circumference, leptin, C-peptide, and low-density lipoprotein and a negative correlation for HDL. | The final sample (26 articles) 63 biomarkers were detected. Most investigated markers were body mass index (BMI, n = 15), waist circumference (WC, n = 15), blood pressure (n = 11), triglycerides (n = 12) and high-density lipoprotein (HDL, n = 15). Some inflammation markers were identified such as interleukin-6, C-reactive protein or tumor necrosis factor alpha. There was a lack of renal, muscle or bone biomarkers. | First, we identified relatively few high quality or longitudinal studies investigating biomarkers specifically in older adults. Therefore, we were not able to conduct a meta-analysis as we anticipated. | The highlighted results of the four “risk population” studies showed associations for SB with biomarkers in the same direction as the studies performed in non-risk populations. |
Figueiró, T. H., Arins, G., Santos, C., Cembranel, F., Medeiros, P. A., d'Orsi, E., & Rech, C. R. (2019); Association of objectively measured sedentary behavior and physical activity with cardiometabolic risk markers in older adults. Plos ONE | Examine the association between sedentary behavior and cardiometabolic markers | Cross-sectional study of Brazilians adults (N = 425) aged 60 years and older | Accelerometer determined sedentary behavior Waist circumference, blood pressure, and plasma-based cardio-metabolic markers |
Sedentary behavior negatively and linearly associated with systolic blood pressure and HDL cholesterol. Physically inactive and sedentary individuals had greater waist circumference and lower HDL cholesterol levels than active, non-sedentary subjects. | Cross-sectional study design Confounding variables (i.e., medications and BMI) not included in the analysis |
Objectively measured sedentary time Plasma-based biomarkers |
Aadahl, M., Linneberg, A., Møller, T., Rosenørn, S., Dunstan, D., Witte, D., & Jørgensen, T. (2014); Motivational counseling to reduce sitting time: A community-based randomized controlled trial in adults. American Journal of Preventive Medicine | Examine the effectiveness of a sedentary behavior intervention | Randomized trial of 166 adults aged adults aged 18–69 years old from Denmark | Objectively measured overall sitting time; secondary measures were breaks in sitting time; anthropometric Accelerometer determined sedentary behavior (ActivPAL Body fat percentage determined by Tanita BC-420MA Serum measures of insulin homeostasis model assessment, hepatic insulin resistance, and basil insulin secretion, glycosylated hemoglobin, total cholesterol, LDL and HDL cholesterol, and triglycerides |
The reduction in sedentary time did not differ between groups. The intervention group had greater improvements in standing time than the control group. Greater improvements in insulin resistance was observed in the intervention condition when compared to the control group. No other differences were observed. | No dietary information was included Uneven sample size in the intervention and control condition. |
Accelerometer determined sedentary behavior Serum biological markers Randomized design |
Reference Author(s), Year, Title, Journal |
Hypotheses/Study Aims | Research Design, Sample Size, Ages, Country | Measures | Primary Findings | Limitations | Strengths |
Chang & Sok (2015); Predictors of sedentary behavior in elderly Koreans with hypertension, The Journal of Nursing Research | Examine predictors of sedentary behavior | Cross-sectional study (N = 306) of Korean adults aged 65 years and older | Self-reported sedentary behavior Psychosocial variables (i.e., empowerment, perceived health, depression, self-efficacy, and social support for physical activity) |
Stepwise regression analysis indicated that empowerment, duration since HBP diagnosis, perceived health, vigorous-intensity physical activity, and depressive symptoms were determinants of sedentary behavior. | Self-reported measures of sedentary behavior Cross-sectional design |
Validated measures |
Reference Author(s), Year, Title, Journal |
Hypotheses/Study Aims | Research Design, Sample Size, Ages | Measures | Primary Findings | Limitations | Strengths |
Bann, Hire, Manini et al. (2015); Light intensity physical activity and sedentary behavior in relation to body mass index and grip strength in older adults: cross-sectional findings from the lifestyle interventions and independence for elders (LIFE) study. Plos ONE | Examine the association between sedentary behavior, BMI, and grip strength. | Cross-sectional study (N = 1,130) of adults aged 70–89 living in the United States. | Accelerometer determined sedentary time Jamar determined grip strength BMI and waist circumference measured by trained staff |
In adjusted models, sedentary behavior was associated with BMI. Less time spent sedentary and more time spent in light-intensity physical activities was associated with BMI | Cross sectional design | Use of BMI as a measure of body size |
Gennuso, Gangnon Matthews (2013); Sedentary behavior, physical activity, and markers of health in older adults. Medicine and science in sports and exercise | Examine the association between sedentary behavior, physical activity, and cardiometabolic markers | Population based cross-sectional study (N = 1914) of adults aged 65 years and older living in the United States | Accelerometer determined sedentary behavior Cardiometabolic markers (total, HDL, and LDL cholesterol, glucose, triglycerides, glycohemoglobin, waist circumference, blood pressure, and C-reactive protein) Physical function |
In adjusted models, higher quartiles of sedentary behavior were associated with weight, BMI, waist circumference, systolic blood pressure, and C-reactive protein among participants who were insufficiently active older adults. Among sufficiently active older adults, higher quartiles of sedentary behavior were associated with waist circumference and LDL cholesterol levels. In addition, insufficiently active older adults who were less sedentary had lower odds of functional limitations. | Cross-sectional design | Accelerometer determined sedentary behavior Population based sample Serum cardiometabolic markers |
Figueiró, T. H., Arins, G., Santos, C., Cembranel, F., Medeiros, P. A., d'Orsi, E., & Rech, C. R. (2019); Association of objectively measured sedentary behavior and physical activity with cardiometabolic risk markers in older adults. Plos ONE | Examine the association between sedentary behavior and cardiometabolic markers | Cross-sectional study of Brazilians adults (N = 425) aged 60 years and older | Accelerometer determined sedentary behavior Waist circumference, blood pressure, and plasma based cardiometabolic markers |
Sedentary behavior was negatively and linearly associated with systolic blood pressure and HDL cholesterol. Physically inactive and sedentary individuals had greater waist circumference and lower HDL cholesterol levels than active, non-sedentary subjects. | Cross-sectional study design Confounding variables (i.e., medications and BMI) not included in the analysis |
Objectively measured sedentary time Plasma-based biomarkers |
Reference Author(s), Year, Title, Journal |
Hypotheses/Study Aims | Research Design, Sample Size, Ages | Measures | Primary Findings | Limitations | Strengths |
Bann, Hire, Manini et al. (2015); Light intensity physical activity and sedentary behavior in relation to body mass index and grip strength in older adults: cross-sectional findings from the lifestyle interventions and independence for elders (LIFE) study. Plos ONE | Examine the association between sedentary behavior, BMI, and grip strength. | Cross-sectional study (N = 1,130) of adults aged 70–89 living in the United States. | Accelerometer determined sedentary time Jamar determined grip strength BMI and waist circumference measured by trained staff |
In adjusted models, sedentary behavior was associated with BMI. Less time spent sedentary and more time spent in light-intensity physical activities were associated with BMI | Cross sectional design | Use of BMI as a measure of body size |
Gennuso, Gangnon, Matthews (2013); Sedentary behavior, physical activity, and markers of health in older adults. Medicine and science in sports and exercise | Examine the association between sedentary behavior, physical activity, and cardiometabolic markers | Population based cross-sectional study (N=1914) of adults aged 65 years and older living in the United States | Accelerometer determined sedentary behavior Cardiometabolic markers (total, HDL, and LDL cholesterol, glucose, triglycerides, glycohemoglobin, waist circumference, blood pressure, and C-reactive protein) Physical function |
In adjusted models, higher quartiles of sedentary behavior were associated with weight, BMI, waist circumference, systolic blood pressure, and C-reactive protein among participants who were insufficiently active older adults. Among sufficiently active older adults, higher quartiles of sedentary behavior were associated with waist circumference and LDL cholesterol levels. In addition, insufficiently active older adults who were less sedentary had lower odds of functional limitations. | Cross-sectional design |
Accelerometer determined sedentary behavior Population based sample Serum cardiometabolic markers |
Judice, Silva, & Sardinha (2015); Sedentary bout durations are associated with abdominal obesity in older adults. The Journal of Nutrition, Health & Aging | Examine the association between sedentary time and patterns of abdominal obesity | Cross-sectional study (N = 351) of older adults (aged 65–103 years) from Portugal | Accelerometer determined sedentary behavior Waist circumference measured by trained staff |
No association was observed with total sedentary time and abdominal obesity. However, for bouts of sedentary time that ranged from 10–20 minutes up to <60 minutes, each bout significantly increased the odds of abdominal obesity. | Cross sectional design Dietary variables not considered in models |
Objectively measured sedentary time |
Reference Author(s), Year, Title, Journal |
Hypotheses/Study Aims | Research Design, Sample Size, Ages, Country | Measures | Primary Findings | Limitations | Strengths |
van Alphen et al. (2016); Older adults with dementia are sedentary for most of the day. Plos ONE | To objectively assess the physical activity levels of community dwelling and institutionalized ambulatory patients with dementia and to compare with the physical activity levels of cognitive healthy older adults. | The research design was a longitudinal study that examined the effects of regular physical activity on the health of dementia patients; participants were recruited via medical staff of aged care facilities. There were 83 institutionalized [mean age 83.0 (7.6)] and 37 community-dwelling dementia patients [mean age 77.3 (5.6)], and 26 healthy older adults [mean age 79.5 (5.6)]. The study was conducted in The Netherlands. | Actigraphy was used to assess physical activity levels that were based on the raw data. Sedentary behavior was classified < 100 counts/min. | Institutionalized vs. community-dwelling dementia patients had 23.5% lower daily physical activity levels (p = .004) and spent 9.3% more time in sedentary behavior (p = .032). Community-dwelling dementia patients vs healthy older adults' daily physical activity levels and sedentary time were 21.6% lower (p = .007) and 8.9% longer (p = .078). | One limitation is that all activity counts per minute represent the same physical activity intensity for healthy as well as adults with dementia. Another limitation is the selection procedure of institutions and daycare centers and homes based on existing collaborations. | The first study to objectively characterize institutionalized and community-dwelling dementia patients' physical activity and sedentary behavior levels. |
Hartman et al. (2018); Dementia patients are more sedentary and less physically active than age-and sex-matched cognitively healthy older adults. Dementia Geriatric Cognitive Disorders | To examine physical activity and sedentary behavior characteristics of ambulatory and community-dwelling patients with dementia compared to cognitively healthy age-, sex- and weight-matched controls. | Cross-sectional study - persons with a dementia diagnosis aged > 60 years who were ambulatory and community dwelling were included. (n = 45, age 79.6 ± 5.9 years), and matched controls (n = 49, age 80.0 ± 7.7 years). Cognitively healthy controls were age, sex, and weight matched to dementia patients and had no history of cognitive impairment. The study was conducted in The Netherlands. | Dementia diagnosis was based on comprehensive clinical assessment by a physician. Mini-Mental State Examination indicated severity of cognitive impairment. Participants wore a wrist accelerometer for 7 days to assess sedentary time, sedentary bout duration and time spent in very light, light-to-moderate and moderate-to-vigorous physical activities. |
Relative sedentary time and sedentary bout duration were significantly greater in dementia patients than in controls. In addition, dementia patients spent a lower percentage of their waking time in light-to-moderate and moderate-to-vigorous intensity physical activities. Secondly, older age was associated with a decline in physical activity and increase in sedentary behavior in controls; however, no such age-related changes were found in dementia patients. | The design of the study could not answer the question whether differences in physical activity and sedentary behavior are simply a consequence of dementia. Only community-dwelling patients were included; therefore, results cannot be generalized to institutionalized dementia patients. Furthermore, a potential bias related to the study enrollment should be considered. Dementia patients in the study were enrolled in an exercise trial. | An accelerometer is a validated measure of physical activity and sedentary behavior. A novel finding was that differences between dementia patients and controls remain when corrected for sleep time. |
Reference Author(s), Year, Title, Journal |
Hypotheses/Study Aims | Research Design, Sample Size, Ages, Country | Measures | Primary Findings | Limitations | Strengths |
Engeroff et al. (2018); Is objectively assessed sedentary behavior, physical activity and cardiorespiratory fitness linked to brain plasticity outcomes in old age? Neuroscience | Examine the association between brain plasticity outcomes and brain-derived neurotrophic factor | Cross-sectional study (N = 50) of older adults (aged 73–77 years old) in Germany | Accelerometer determined sedentary behavior Serum markers of brain metabolism and brain-derived neurotrophic factors (BDNF) Cardiorespiratory fitness |
Sedentary time was associated with BDNF, but not brain metabolism measures | Small sample Cognitively healthy sample |
Well controlled study Novel methods |
Falck et al. (2017); Cross-sectional relationships of physical activity and sedentary behavior with cognitive function in older adults with probable mild cognitive impairment. Physical Therapy |
To examine differences in physical activity and sedentary behavior between people with probable mild cognitive impairment and people without mild cognitive impairment and to assess whether associations of physical activity and sedentary behavior with cognitive function differed by mild cognitive impairment status. | The study was cross sectional. The sample size was 150. The mean age was 71.11 years old (7.22). The location was Vancouver, British Columbia, Canada. | Physical activity and sedentary behavior in adults dwelling in the community were measured using a wrist-worn actigraphy unit. The Montreal Cognitive Assessment categorized participants with probable mild cognitive impairment and participants without the condition. Cognitive function was indexed using the Alzheimer Disease Assessment Scale-Cognitive-Plus. | Participants with probable mild cognitive impairment had lower physical activity and higher sedentary behavior than participants without mild cognitive impairment. Higher physical activity and lower sedentary behavior were associated with better Alzheimer Disease Assessment Scale-Cognitive-Plus performance in participants without mild cognitive impairment but not in participants with probable mild cognitive impairment. | This study was cross-sectional and therefore causal associations should not be inferred. In addition, the diagnosis of mild cognitive impairment was not confirmed with a physician. | First study to report that older adults with probable mild cognitive impairment were less active and more sedentary than their cognitively healthy peers. Objective assessments of physical activity and sedentary behavior. |
Walle'n et al. (2015); Levels and patterns of physical activity and sedentary behavior in elderly people with mild to moderate Parkinson's disease. Physical Therapy | To describe levels and patterns of physical activity and sedentary behaviors in elderly people with mild to moderate Parkinson's Disease. | This cross-sectional study involved a free-living setting and 53 men and 42 women (mean age 73.4 years; (SD-5.7 years) with mild to moderate idiopathic Parkinson's Disease. The country was Sweden. | Time spent in physical activity and sedentary behaviors were assessed for one week with accelerometers. | The main finding was that approximately 75% of all time awake was spent in sedentary behaviors; 18% was spent in physical activity. The proportion of individuals meeting the goal of achieving 150 minutes of moderate- to vigorous-intensity PA per week was 27%; 16% achieved 7,000 or more steps per day; participants spent 589 minutes daily in sedentary behaviors. | The participants were not randomly selected; therefore, generalizability is limited. | Based on objective assessment (accelerometers), described the levels and patterns of physical activity, including diurnal patterns, and estimated the extent to which physical activity recommendations were met. |
Nemoto et al. (2018); The association of single and combined factors of sedentary behavior and physical activity with subjective cognitive complaints among community-dwelling older adults: Cross-sectional study. Plos ONE | To examine the association of type of sedentary behavior, physical activity, or their combination with subjective cognitive complaints among community dwelling older adults. | The cross-sectional study targeted independently living individuals aged 65 years and older who resided in Tsuru, Yamanashi Prefecture, Japan (n = 5,328). | Participants responded to the National Functional Survey Questionnaire (Kihon checklist) to assess subjective cognitive complaints. Do your family or friends point out your memory loss? Do you make a call by looking up phone numbers? Do you find yourself not knowing today's date? To evaluate physical activity, the Japanese version of the International Physical Activity Questionnaire Short-version (IPAQ-SV) was conducted. Sedentary behavior time was assessed as subjective average duration of television viewing and reading books or newspapers during the last seven days. | The primary findings were that moderate-to-vigorous intensity physical activity was significantly related with a lower risk of subjective cognitive complaints and that reading showed a dose-response relationship with subjective cognitive complaints. The combined group who reported >150 min/week physical activity and >30 min/day reading showed 60% lower subjective cognitive complaints than the combined group who reported <150 min/week physical activity and <10 min/day reading. | The cross-sectional design limits causal inferences. Physical activity and sedentary behavior were assessed subjectively. Participants who developed dementia or mild cognitive impairment during the study were not necessarily completely excluded. | First study to report the association of the combined effects of sedentary time and physical activity with subjective cognitive complaints; demographic variables, health behavior, and health status were included in the analyses. The data were from a complete survey with a high response rate of 79.8% and missing values were accounted for by multiple imputation. |
Reference Author(s), Year, Title, Journal |
Hypotheses/Study Aims | Research Design, Sample Size, Ages, Country | Measures | Primary Findings | Limitations | Strengths |
Okely et al. (2019); Positive and negative well-being and objectively measured sedentary behavior in older adults: evidence from three cohorts. BMC Geriatrics | To examine the association between sedentary behavior and both positive and negative effect | Three prospective (N = 698) of older adults in Scotland | Accelerometer determined sedentary behavior (ActivPAL) Validated scales for positive and negative effect, depression, and anxiety |
Sedentary behavior was associated with depression symptoms | Waking time was self-reported | Accelerometer determined sedentary behavior Validated measures of mental health |
Ku et al. (2015); Leisure-time physical activity, sedentary behaviors and subjective well-being in older adults: An eight-year longitudinal research. Social Indicators Research | Examine the association between self-reported sedentary time and subjective well-being | Longitudinal fixed cohort (N = 1,268) of adults aged 70 years or older in Taiwan. | Self-reported leisure time in sedentary behavior Subjective well-being (i.e., Life Satisfaction Index) |
Television viewing, social chatting, reading, and listening to the radio were significantly associated with subjective well-being | Self-reported sedentary time | Longitudinal design |
Reference Author(s), Year, Title, Journal |
Hypotheses/Study Aims | Research Design, Sample Size, Ages, Country | Measures | Primary Findings | Limitations | Strengths |
Maréchal et al. (2019); Effect of a mixed-exercise program on physical capacity and sedentary behavior in older adults during cancer treatments. Aging Clinical and Experimental Research | To examine the impact of an intervention on increasing physical activity and reducing sedentary behavior during cancer treatment | Two-group (i.e., mixed exercise vs. stretching) pre- and post-test design (N = 14) of older patients in Canada | Self-reported sedentary time Senior fitness test – functional performance test (i.e., chair stands, Up and Go, Arm Curl, Sit and Reach, and 6-minute walk test) |
Mixed exercise group had greater improvements in chair stands and global physical capacity scores when compared to those in the stretching group. No other differences were observed | Small sample Sedentary behavior was not the focus |
Objective assessments of functional performance |
Research design | Reference | Quality assessment from National Institute of Health ratings criteria (good, fair, poor) |
Observational Cohort and Cross-Sectional Studies | ||
Bann et al. 2015 | Good | |
Benka Wallén et al. 2015 | Good | |
Cabanas-Sanchez et al. 2018 | Fair | |
Chang et al. 2015 | Fair | |
Ekblom et al. 2015 | Good | |
Engeroff et al. 2018 | Fair | |
Ensrud et al. 2014 | Good | |
Figueiró et al. 2019 | Good | |
Gennuso et al. 2013 | Good | |
Gennuso et al. 2015 | Good | |
Jefferis et al. 2019 | Good | |
Joseph et al. 2016 | Good | |
Judice et al. 2015 | Good | |
Klenk et al. 2016 | Good | |
Ku et al. 2016 | Good | |
Leon-Munoz et al. 2013 | Good | |
Matthews et al. 2012 | Good | |
Nemoto et al. 2018 | Good | |
Okely et al. 2019 | Good | |
Pavey et al. 2015 | Good | |
Before-After (Pre-Post) Studies with No Control Group | ||
Hartman et al. 2018 | Fair | |
Case-Control Studies | ||
Falck et al. 2017 | Fair | |
van Alphen et al. 2016 | Fair | |
Controlled Intervention Studies | ||
Aadahl et al. 2019 | Fair | |
Maréchal et al. 2019 | Poor | |
Systematic Reviews and Meta-Analyses | ||
Thorp et al. 2011 | Fair | |
Wirth et al. 2017 | Good |