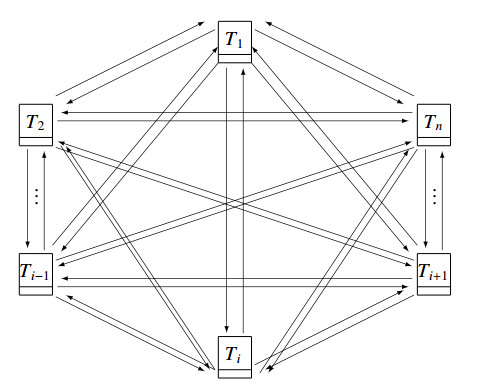
Cardiac arrhythmias are serious myocardial electrical disturbances that affect the rate and rhythm of heartbeats. Despite the rapidly accumulating data about the pathophysiology and the treatment, new insights are required to improve the overall clinical outcome of patients with cardiac arrhythmias. Three major arrhythmogenic processes can contribute to the pathogenesis of cardiac arrhythmias; 1) enhanced automaticity, 2) afterdepolarization-triggered activity and 3) reentry circuits. The mathematical model of the quantum tunneling of ions is used to investigate these mechanisms from a quantum mechanical perspective. The mathematical model focuses on applying the principle of quantum tunneling to sodium and potassium ions. This implies that these ions have a non-zero probability of passing through the gate, which has an energy that is higher than the kinetic energy of ions. Our mathematical findings indicate that, under pathological conditions, which affect ion channels, the quantum tunneling of sodium and potassium ions is augmented. This augmentation creates a state of hyperexcitability that can explain the enhanced automaticity, after depolarizations that are associated with triggered activity and a reentry circuit. Our mathematical findings stipulate that the augmented and thermally assisted quantum tunneling of sodium and potassium ions can depolarize the membrane potential and trigger spontaneous action potentials, which may explain the automaticity and afterdepolarization. Furthermore, the quantum tunneling of potassium ions during an action potential can provide a new insight regarding the formation of a reentry circuit. Introducing these quantum mechanical aspects may improve our understanding of the pathophysiological mechanisms of cardiac arrhythmias and, thus, contribute to finding more effective anti-arrhythmic drugs.
Citation: Mohammed I. A. Ismail, Abdallah Barjas Qaswal, Mo'ath Bani Ali, Anas Hamdan, Ahmad Alghrabli, Mohamad Harb, Dina Ibrahim, Mohammad Nayel Al-Jbour, Ibrahim Almobaiden, Khadija Alrowwad, Esra'a Jaibat, Mira Alrousan, Mohammad Banifawaz, Mohammed A. M. Aldrini, Aya Daikh, Nour Aldarawish, Ahmad Alabedallat, Ismail M. I. Ismail, Lou'i Al-Husinat. Quantum mechanical aspects of cardiac arrhythmias: A mathematical model and pathophysiological implications[J]. AIMS Biophysics, 2023, 10(3): 401-439. doi: 10.3934/biophy.2023024
[1] | Christian E. Vazquez, Catherine Cubbin . Associations between breastfeeding duration and overweight/obese among children aged 5–10: a focus on racial/ethnic disparities in California. AIMS Public Health, 2019, 6(4): 355-369. doi: 10.3934/publichealth.2019.4.355 |
[2] | Catherine Cubbin, Katherine Heck, Tara Powell, Kristen Marchi, Paula Braveman . Racial/Ethnic Disparities in Depressive Symptoms Among Pregnant Women Vary by Income and Neighborhood Poverty. AIMS Public Health, 2015, 2(3): 411-425. doi: 10.3934/publichealth.2015.3.411 |
[3] | Wanda M. Williams, Michelle M. Yore, Melicia C. Whitt-Glover . Estimating physical activity trends among blacks in the United States through examination of four national surveys. AIMS Public Health, 2018, 5(2): 144-157. doi: 10.3934/publichealth.2018.2.144 |
[4] | Keith Elder, Keon Gilbert, Louise Meret Hanke, Caress Dean, Shahida Rice, Marquisha Johns, Crystal Piper, Jacqueline Wiltshire, Tondra Moore, Jing Wang . Disparities in Confidence to Manage Chronic Diseases in Men. AIMS Public Health, 2014, 1(3): 123-136. doi: 10.3934/publichealth.2014.3.123 |
[5] | Marybeth Gasman, Tiffany Smith, Carmen Ye, Thai-Huy Nguyen . HBCUs and the Production of Doctors. AIMS Public Health, 2017, 4(6): 579-589. doi: 10.3934/publichealth.2017.6.579 |
[6] | Salimah H. Meghani, Eeeseung Byun, Jesse Chittams . Conducting Research with Vulnerable Populations: Cautions and Considerations in Interpreting Outliers in Disparities Research. AIMS Public Health, 2014, 1(1): 25-32. doi: 10.3934/publichealth.2014.1.25 |
[7] | Elspeth Molony, Christine Duncan . Income, Wealth and Health Inequalities — A Scottish Social Justice Perspective. AIMS Public Health, 2016, 3(2): 255-264. doi: 10.3934/publichealth.2016.2.255 |
[8] | Wenjun Li, Elizabeth Procter-Gray, Gretchen A. Youssef, Scott E. Crouter, Jie Cheng, Kristen Brown, Linda Churchill, Anthony Clarke, Judith K. Ockene, Michelle F. Magee . Racial Differences in Neighborhood Perceptions and their Influences on Physical Activity among Urban Older Women. AIMS Public Health, 2017, 4(2): 149-170. doi: 10.3934/publichealth.2017.2.149 |
[9] | Yelena Ogneva-Himmelberger, Tyler Dahlberg, Kristen Kelly, Tiffany A. Moore Simas . Using Geographic Information Science to Explore Associations between Air Pollution, Environmental Amenities, and Preterm Births. AIMS Public Health, 2015, 2(3): 469-486. doi: 10.3934/publichealth.2015.3.469 |
[10] | Carmen Giurgescu, Lara Fahmy, Jaime Slaughter-Acey, Alexandra Nowak, Cleopatra Caldwell, Dawn P Misra . Can support from the father of the baby buffer the adverse effects of depressive symptoms on risk of preterm birth in Black families?. AIMS Public Health, 2018, 5(1): 89-98. doi: 10.3934/publichealth.2018.1.89 |
Cardiac arrhythmias are serious myocardial electrical disturbances that affect the rate and rhythm of heartbeats. Despite the rapidly accumulating data about the pathophysiology and the treatment, new insights are required to improve the overall clinical outcome of patients with cardiac arrhythmias. Three major arrhythmogenic processes can contribute to the pathogenesis of cardiac arrhythmias; 1) enhanced automaticity, 2) afterdepolarization-triggered activity and 3) reentry circuits. The mathematical model of the quantum tunneling of ions is used to investigate these mechanisms from a quantum mechanical perspective. The mathematical model focuses on applying the principle of quantum tunneling to sodium and potassium ions. This implies that these ions have a non-zero probability of passing through the gate, which has an energy that is higher than the kinetic energy of ions. Our mathematical findings indicate that, under pathological conditions, which affect ion channels, the quantum tunneling of sodium and potassium ions is augmented. This augmentation creates a state of hyperexcitability that can explain the enhanced automaticity, after depolarizations that are associated with triggered activity and a reentry circuit. Our mathematical findings stipulate that the augmented and thermally assisted quantum tunneling of sodium and potassium ions can depolarize the membrane potential and trigger spontaneous action potentials, which may explain the automaticity and afterdepolarization. Furthermore, the quantum tunneling of potassium ions during an action potential can provide a new insight regarding the formation of a reentry circuit. Introducing these quantum mechanical aspects may improve our understanding of the pathophysiological mechanisms of cardiac arrhythmias and, thus, contribute to finding more effective anti-arrhythmic drugs.
In recent years, the addition-min fuzzy relation inequality (FRI) was first introduced in [1,2] for describing the flow constraints in the peer-to-peer (P2P) network system. Since the minimal solutions play a key role in constructing the complete solution set for the addition-min FRIs [3,4,5], Li et al. [1] proposed a feasible approach to find some specific minimal solutions. However, as shown in [2], the addition-min FRIs usually have an infinite number of minimal solutions, and it is difficult to determine all the minimal solutions. To obtain specific minimal solutions, various approaches have been developed. In [6], the lexicographic minimum solution was defined and studied. An effective resolution algorithm and some illustrative numerical examples were provided. The concept of the lexicographic minimum solution was also extended to random-term-absent addition-min FRIs [7]. It can be formally proven that any lexicography minimum solution should also be a minimal solution in the addition-min FRI system. In [8], Li et al. investigated another kind of minimal solution for addition-min FRIs. The authors attempted to find a minimal solution that was less than or equal to a given solution [8]. Moreover, to obtain specific minimal solutions, solving an optimization problem is also an effective approach. For instance, the optimization problem with a linear objective function was studied in [9,10], with addition-min FRIs constraints. Considering the fairness among the terminals in the P2P network system, Yang et al. [11] and Chiu et al. [12] further investigated fuzzy relation minimax programming with addition-min composition. However, it was shown that its optimal solutions were usually nonunique. Thus, Wu et al. [13] and Yang et al. [14] further searched for the minimal optimal solutions. By adding some weighted factors to the terminals, the corresponding fuzzy relation weighted minimax programming was also investigated [15,16,17].
In references [1,2,3,4,5,6,7,8,9,10,11,12,13,14,15,16,17], addition-min FRIs were introduced to investigate the P2P network system. As noted in [18,19,20,21], by applying the addition-min FRIs to model the P2P network system, the authors considered the total download speed of each terminal and downloaded its requested data from other terminals. However, it was also indicated in [18,19,20,21] that, in some cases, the highest download speed should be considered. Next, we describe the requirements of the highest download speed for the terminals in a P2P network system. Similarly, it is assumed that there are n terminals in the system, represented by T1,T2,⋯,Tn (see Figure 1). After accepting the downloading request from other terminals, we suppose that terminal Tj transmits its local file to any other terminal at quality level tj. If the bandwidth between Ti and Tj (exactly from Tj to Ti) is aij (see Figure 2), then the actual download traffic of Ti from Tj is aij∧tj.
Considering the total download traffic of each terminal, the P2P network system can be modeled by the addition-min FRIs. However, in some cases, the specific file should be downloaded from a single terminal. In such cases, Ti downloads its requested file from the terminal with the highest download traffic, i.e., ai1∧t1∨ai2∧t2∨⋯∨ain∧tn. Let us suppose that the requirement of the highest download traffic of Ti is no less than bi, i=1,2,⋯,m. Then the requirements of all the terminals in a P2P network system should be modeled by the following max-min FRIs:
ai1∧t1∨ai2∧t2∨⋯∨ain∧tn≥bi,i=1,2,⋯,m. | (1.1) |
Moreover, if the requirement of each terminal for the highest download speed has both an upper bound and a lower bound, then the corresponding max-min FRIs should be further written as
bi≤ai1∧t1∨ai2∧t2∨⋯∨ain∧tn≤di,i=1,2,⋯,m. | (1.2) |
For inconsistent system (1.1), Yang established an evaluation model for a given vector, based on which the approximate solution was defined and investigated [22]. For the consistent system (1.1), Zhong et al. [23] focused on the lexicographic minimum solution. Xiao et al. investigated the evaluation and derived a classification of system solutions (1.1) [24]. A resolution algorithm was developed with an illustrative example. Moreover, the lexicographic minimum solution was also introduced for the above system (1.2) [25]. Then considering the stability of the P2P network system, Chen et al. [26] defined the concept of interval solutions for system (1.2). The authors proposed an effective algorithm to find the so-called widest interval solution of system (1.2) in [26].
As mentioned above, with the constraint system of addition-min FRIs, weighted minimax programming, or even minimax programming as its specific form, has been studied, providing some efficient resolution algorithms [11,12,13,14,15,16,17]. However, when considering the highest download speed, the relevant weighted minimax programming has not been studied for the corresponding max-min system (1.2). Consequently, we focus on such an optimization problem in this work. The formulistic form of the weighted minimax programming subject to system (1.2) is
minz(t)=c1t1∨c2t2∨⋯∨cntn,s.t.{bi≤ai1∧t1∨ai2∧t2∨⋯∨ain∧tn≤di,i=1,2,⋯,m. | (1.3) |
As shown in [11,12,13,14,15,16,17], in system (1.2) or problem (1.3), tj (measure: Mbps) represents the quality level on which the jth terminal shares (sends out) its local resources with the other terminals. To decrease network congestion, system managers usually try to minimize the values of t1,t2,⋯,tn. However, in most cases, these values cannot be minimized simultaneously. Instead of minimizing all these variables, minimizing a specific function with the variables t1,t2,⋯,tn is much more realistic. For instance, in problem (1.3), we adopt the weighted minimax function. In such a weighted minimax function in (1.3), the parameter cj represents the weighted factor of the jth terminal in the P2P network system. In this work, we design several effective algorithms for identifying the optimal solution.
In summary, the innovations and contributions of this work can be summarized as follows:
(ⅰ) Instead of the total download traffic of the terminal, we consider the highest download traffic. Consequently, the corresponding max-min FRIs are employed.
(ⅱ) To decrease network congestion in the P2P network system, we consider a given weighted factor for each terminal. Moreover, we further establish a relevant weighted minimax programming problem with max-min FRI constraints.
(ⅲ) To solve our established weighted minimax optimization model, we propose the so-called single-constraint programming approach for identifying the optimal solution.
The following content is organized as follows. In Section 2, we describe some foundational concepts and results on max-min FRIs, i.e., system (1.2). Our major results are presented in Section 3. In this section, we introduce the single-constraint programming (SCP) approach for addressing our studied problem (1.3). The original problem (1.3) is separated into several subproblems and solved. Moreover, our proposed approach is a step-by-step SCP-based algorithm. In Section 4, a detailed numerical example is provided to demonstrate the SCP-based algorithm. In Section 5, we compare our problem and the proposed method to the existing ones. Section 6 provides a simple conclusion.
In this section, we provide some foundational results on the max-min fuzzy relation inequalities, i.e., system (1.2). These existing results are helpful for the resolution of problem (1.3).
Let us denote
A=(aij)m×n,t=(t1,⋯,tn),b=(b1,⋯,bm),d=(d1,⋯,dm). |
Then we represent system (1.2) as
b≤A∘t≤d. | (2.1) |
Moreover, the solution set of system (1.2) is indeed
TA,b,d={t∈[0,1]n|b≤A∘t≤d}. |
For convenience, we let
I={1,2,⋯,m},J={1,2,⋯,n}. |
Definition 1 (Consistent; Maximum solution [25]) System (1.2) is said to be consistent when its solution set is nonempty, i.e., TA,b,d≠∅; otherwise, system (1.2) is inconsistent. When system (1.2) is consistent and there exists a solution ¯t∈TA,b,d such that ¯t≥t for any t∈TA,b,d, then we say ¯t is the maximum solution of (1.2).
In system (1.2), we construct the vector ˆt=(ˆt1,ˆt2,⋯,ˆtn) as follows:
ˆtj={⋀i∈Ijdi,ifIj≠∅,1,ifIj=∅, | (2.2) |
where Ij={i∈I|aij>di}, ∀j∈J. The vector ˆt is important to determine whether system (1.2) is consistent.
Proposition 1. [25] Let t∈TA,b,d be a solution of system (1.2), and the vector ˆt is defined by (2.2). Then, we have t≤ˆt.
Theorem 1. [25] System (1.2) is consistent, i.e., TA,b,d≠∅, if and only if ˆt∈TA,b,d.
According to Proposition 1 and Theorem 1, we know that when TA,b,d≠∅, ˆt should be the unique maximum solution of system (1.2). The potential maximum solution ˆt can be used to check the consistency of (1.2).
Definition 2. (Minimal solution [25]) Let (1.2) be consistent and ˇt∈TA,b,d. We say that ˇt is a minimal solution if there is no t∈TA,b,d such that t≤ˇt and t≠ˇt.
The conservative path approach was proposed in [27,28] to obtain all the minimal solutions of the system (1.2). Moreover, it was shown that system (1.2) has a finite number of minimal solutions when it is consistent. For system (1.2), we denote the set of all minimal solutions by
ˇTA,b,d={ˇt∈TA,b,d|ˇtis a minimal solution}. |
Based on the maximum solution ˆt and the minimal solution set ˇTA,b,d, the complete solution set to (1.2) can be characterized by Theorem 2.
Theorem 2. [25] When system (1.2) is consistent, the solution set TA,b,d is
TA,b,d=⋃ˇt∈ˇTA,b,d[ˇt,ˆt]. | (2.3) |
Since all minimal solutions can be found by the conservative path approach [27,28], one can obtain the complete solution set TA,b,d for system (1.2).
In this subsection, we first illustrate the existence of the optimal solution for problem (1.3). The optimal solution exists if and only if the feasible domain, i.e., TA,b,d, is nonempty. Afterward, we assume TA,b,d≠∅, and we attempt to solve problem (1.3). The original problem (1.3) is separated into m subproblems according to the constraints, i.e., system (1.2). Each subproblem has the same objective function as problem (1.3) and a single inequality in the constraint. Thus, each subproblem is indeed a single-constraint programming (SCP) problem. The optimal solution of problem (1.3) can be generated by the optimal solutions of the subproblems. Since problem (1.3) is solved via single-constraint programming, we refer to our resolution method as the SCP-based approach.
Theorem 3. (Existence of the optimal solution) System (1.2) is consistent, i.e., TA,b,d≠∅, if and only if problem (1.3) has at least one optimal solution.
Proof. It has been shown previously that system (1.2) has a finite number of minimal solutions since it is consistent. Without loss of generality, we denote the minimal solution set by
ˇTA,b,d={ˇt1,ˇt2,⋯,ˇts}. |
The objective function values of {ˇt1,ˇt2,⋯,ˇts} can be found as {z(ˇt1),z(ˇt2),⋯,z(ˇts)}. Let us denote
z∗=min{z(ˇt1),z(ˇt2),⋯,z(ˇts)}. | (3.1) |
Moreover, there exists l∗∈{1,2,⋯,s} such that z(ˇtl∗)=z∗, and
z∗≤z(ˇtl),∀l∈{1,2,⋯,s}. | (3.2) |
Obviously, the minimal solution ˇtl∗ is also a feasible solution to problem (1.3). Next, we further verified that it is an optimal solution.
Let t∈TA,b,d be an arbitrary solution of (1.2). According to Theorem 2, l′∈{1,2,⋯,s} and ˇtl′∈ˇTA,b,d, such that t∈[ˇtl′,ˆt], i.e., ˇtl′≤t≤ˆt. Thus,
z(ˇtl′)=c1ˇtl′1∨⋯∨cnˇtl′n≤c1t1∨⋯∨cntn=z(t). | (3.3) |
Let us note that l′∈{1,2,⋯,s}. The inequalities (3.2) and (3.3) imply that z∗≤z(t), i.e., z(ˇtl∗)≤z(t). As a result, ˇtl∗ is an optimal solution of (1.3).
It has been shown in Theorem 3 that the optimal solution of problem (1.3) should exist when system (1.2) is consistent. Next, we consider the resolution of problem (1.3) with the assumption that TA,b,d≠∅.
Based on the maximum solution ˆt and the formulae in problem (1.3), we construct the following single-constraint programming problem:
(Pi)minz(t)=c1t1∨c2t2∨⋯∨cntn,s.t.{bi≤ai1∧t1∨ai2∧t2∨⋯∨ain∧tn≤di,t≤ˆt, | (3.4) |
for each i∈I. Then, we obtain m subproblems, denoted by {(P1),(P2),⋯,(Pm)}, corresponding to the original problem (1.3).
In fact, the optimal solution of problem (1.3) can be generated by the optimal solutions of these subproblems, as indicated in what follows. Next, we provide an algorithm to obtain the optimal solution of each subproblem, and then we show the relationship between the original problem (1.3) and the subproblems {(P1),(P2),⋯,(Pm)}.
Let i∈I be an arbitrary index in I. In this subsection, we propose an effective approach to obtain an optimal solution of subproblem (Pi), i.e., problem (3.4). In the remainder of this subsection, we always assume that i is a given index.
We denote the index set as
Jˆti={j∈J|aij≥bi,ˆtj≥bi}. | (3.5) |
It is clear that j∈Jˆti if and only if aij∧ˆtj≥bi.
Proposition 2. ˆt is a solution of system (1.2), if and only if Jˆtk≠∅ holds for any k∈I.
Proof. (⇒) If ˆt is a solution of system (1.2), we have
ak1∧ˆt1∨ak2∧ˆt2∨⋯∨akn∧ˆtn≥bk,∀k∈I. | (3.6) |
Hence, there exists j′∈J such that akj′∧ˆtj′≥bk. This implies that j′∈Jˆtk, i.e., Jˆtk≠∅.
(⇐) If Jˆtk≠∅, ∀k∈I, then there exists jk∈Jˆtk for each k. Since jk∈Jˆtk⊆J, by (3.5) we have
ak1∧ˆt1∨ak2∧ˆt2∨⋯∨akn∧ˆtn≥akjk∧ˆtjk≥bk,∀k∈I. | (3.7) |
Therefore, ˆt is a solution of system (1.2).
According to Theorem 1 and Proposition 2, we find Corollary 1.
Corollary 1. TA,b,d≠∅ if and only if Jˆtkneq∅ holds for any k∈I.
Obviously, Corollary 1 can also be used to check the consistency of system (1.2) through the index sets {Jˆt1,Jˆt2,⋯,Jˆtm}.
Based on the index set Jˆti, defined by (3.5), we find the optimal index as
p∗i=argminj∈Jˆti{cj}. | (3.8) |
Then we have p∗i∈Jˆti and cp∗i=min{cj|j∈Jˆti}. Furthermore, we construct the vector t∗i=(t∗i1,t∗i2,⋯,t∗in) as
t∗ij={bi,ifj=p∗i,0,ifj≠p∗i. | (3.9) |
We show that the vector t∗i is indeed an optimal solution of the subproblem (Pi).
Theorem 4. (Optimal solution of (Pi)) Let us suppose TA,b,d≠∅. Then, the vector t∗i defined above is an optimal solution of the subproblem (Pi).
Proof. (Feasibility) Let j′=p∗i∈Jˆti. By (3.5), we have
aij′≥bi,ˆtj′≥bi. | (3.10) |
By (3.9), we also have
t∗ij′=bi, | (3.11) |
and
t∗ij=0,∀j≠j′,j∈J. | (3.12) |
Since aij′≥bi, it is immediate that aij′∧bi=bi. Considering (3.12), we have
ai1∧t∗i1∨⋯∨ain∧t∗in=aij′∧bi=bi. | (3.13) |
According to (3.10)–(3.12), we have
ˆtj′≥bi=t∗ij′, | (3.14) |
and
ˆtj≥0=t∗ij,∀j≠j′,j∈J. | (3.15) |
Formulae (3.14) and (3.15) imply that t∗i≤ˆt. Combining formula (3.13), t∗i is a feasible solution of subproblem (Pi).
According to (3.11) and (3.12), z(t∗i)=cj′bi. Let us take an arbitrary feasible solution of (1.3) as t. Then, it holds that
{bi≤ai1∧t1∨ai2∧t2∨⋯∨ain∧tn≤di,t≤ˆt. | (3.16) |
There exists j″∈J such that aij″∧tj″≥bi, i.e., aij″≥bi and tj″≥bi. By (3.5), we have j″∈Jˆti. Since j′=p∗i, by (3.8) we have
cj′=cp∗i=min{cj|j∈Jˆti}≤cj″. | (3.17) |
However, considering tj″≥bi, we have
z(t)=c1t1∨⋯∨cntn≥cj″tj″≥cj′bi=z(t∗i). | (3.18) |
As a consequence, t∗i is an optimal solution of subproblem (Pi).
Summarizing the above results, we develop Algorithm Ⅰ to solve subproblem (Pi).
Algorithm Ⅰ: To calculate an optimal solution of the subproblem (Pi)
Step 1: Construct the vector ˆt=(ˆt1,ˆt2,⋯,ˆtn) following (2.2).
Step 2: Apply the vector ˆt; check the consistency of system (1.2) following Theorem 1. If ˆt∈TA,b,d, then TA,b,d≠∅ and problem (1.3) is solvable. Continue to the next step. Otherwise, problem (1.3) is unsolvable; stop.
Step 3: Find the index set Jˆti according to (3.5).
Step 4: Find the optimal index p∗i according to Jˆti and (3.8).
Step 5: Find the vector t∗i=(t∗i1,t∗i2,⋯,t∗in) according to the optimal indices p∗i and (3.9). Then, by Theorem 4, t∗i is an optimal solution of subproblem (Pi).
Example 1. Let us consider the following single-constraint programming problem:
(P0)minz(t)=0.4t1∨0.5t2∨0.7t3∨0.3t4∨0.5t5∨0.6t6,s.t.{0.38≤0.9∧t1∨0.6∧t2∨0.8∧t3∨0.2∧t4∨0.3∧t5∨0.4∧t6≤0.76,t≤(0.76,0.77,0.76,0.75,0.85,1). | (3.19) |
Algorithm Ⅰ is applied to find an optimal solution to problem (3.19), i.e., problem (P0).
Solution. Steps 1 and 2: The constraint is extracted from system (4.1) appearing in Example 2 below. We verify that system (4.1) is consistent, with the maximum solution ˆt=(0.76,0.77,0.76,0.75,0.85,1). Hence, we go directly to Step 3.
Step 3: Since
{0.9∧ˆt1=0.9∧0.76=0.76>0.38,0.6∧ˆt2=0.6∧0.77=0.6>0.38,0.8∧ˆt3=0.8∧0.76=0.76>0.38,0.2∧ˆt4=0.2∧0.75=0.2<0.38,0.3∧ˆt5=0.3∧0.85=0.3<0.38,0.4∧ˆt6=0.4∧1=0.4>0.38, | (3.20) |
by (3.5) we have Jˆt0={1,2,3,6}.
Step 4: Here, c=(0.4,0.5,0.7,0.3,0.5,0.6). Since
p∗0=argminj∈Jˆt0{cj}=argmin{c1,c2,c3,c6}=argmin{0.4,0.5,0.7,0.6}=0.4=c1, | (3.21) |
by (3.8), we find the optimal index as p∗0=1.
Step 5: Since p∗0=1, we find the vector t∗0 by (3.9) as t∗0=(0.38,0,0,0,0,0). According to Theorem 4, t∗0=(0.38,0,0,0,0,0) is an optimal solution of subproblem (P0), i.e., problem (3.19).
In Subsection 3.2, we find an optimal solution for each subproblem (Pi), denoted by t∗i. Based on these optimal solutions {t∗1,t∗2,⋯,t∗m}, we generate the optimal solution of problem (1.3) in this subsection.
For x1=(x11,⋯,x1n),x2=(x21,⋯,x2n)∈[0,1]n, let
x1∨x2=(x11∨x21,⋯,x1n∨x2n). | (3.22) |
Lemma 1. For arbitrary x1,x2,⋯,xm∈[0,1]n, we have z(x1∨x2∨⋯∨xm)=z(x1)∨z(x2)∨⋯∨z(xm).
Proof. In fact, we have to prove only that z(x1∨x2)=z(x1)∨z(x2).
z(x1∨x2)=c1(x11∨x21)∨⋯∨cn(x1n∨x2n)=(c1x11∨c1x21)∨⋯∨(cnx1n∨cnx2n)=(c1x11∨⋯∨cnx1n)∨(c1x21∨⋯∨cnx2n)=z(x1)∨z(x2). | (3.23) |
Lemma 2. Let t∈[0,1] be an arbitrary real number. Then, we have t∈TA,b,d if and only if A∘t≥b and t≤ˆt.
Proof. (⇒) This is evident, according to Proposition 1 and the expression of system (1.2).
(⇐) Let us consider, arbitrarily, i′∈I and j′∈J. Let us recall that Ij′={i∈I|aij′>di}.
If i′∉Ij′, then we have
ai′j′∧ˆtj′≤ai′j′≤di′. | (3.24) |
If i′∈Ij′, then by (2.2) we have Ij′≠∅ and ˆtj′=⋀i∈Ij′di≤di′. Thus,
ai′j′∧ˆtj′≤ˆtj′≤di′. | (3.25) |
Due to the arbitrariness of i and j, we have
ai′j′∧ˆtj′≤di′,∀i′∈I,j′∈J. | (3.26) |
Hence,
ai′1∧ˆt1∨⋯∨ai′n∧ˆtn≤di′,∀i′∈I. | (3.27) |
That is, A∘ˆt≤d. Since t≤ˆt, we have A∘t≤A∘ˆt≤d. Combining A∘t≥b, it is immediate that t∈TA,b,d.
Theorem 5. Let t∗i be an optimal solution of subproblem (Pi) for each i∈I. Then, t∗=t∗1∨t∗2∨⋯∨t∗m is an optimal solution of problem (1.3).
Proof. (Feasibility) For arbitrarily given i∈I, we verified in Theorem 4 that t∗i≤ˆt. Hence,
t∗=t∗1∨t∗2∨⋯∨t∗m≤ˆt. | (3.28) |
Since t∗=t∗1∨t∗2∨⋯∨t∗m, it is obvious that t∗j=⋁k∈It∗kj≥t∗ij, ∀i∈I,j∈J. Hence, by (3.13),
ai1∧t∗1∨⋯∨ain∧t∗n≥ai1∧t∗i1∨⋯∨ain∧t∗in=bi,∀i∈I. | (3.29) |
That is, A∘t∗≥b. Considering t∗≤ˆt, it follows from Lemma 2 that t∗∈TA,b,d. That is, t∗ is a feasible solution to problem (1.3).
(Optimality) Let t∈TA,b,d be an arbitrary feasible solution of problem (1.3). By observing system (1.2), it is clear that
bi≤ai1∧t1∨ai2∧t2∨⋯∨ain∧tn≤di,∀i∈I. | (3.30) |
Moreover, by Proposition 1, we have t≤ˆt. Hence, t is a feasible solution of subproblem (Pi) for any i∈I. Let us note that t∗i is the optimal solution of (Pi). We have
z(t)≥z(t∗i),∀i∈I. | (3.31) |
Following Lemma 1, we have
z(t)≥z(t∗1)∨z(t∗2)∨⋯∨z(t∗m)=z(t∗1∨t∗2∨⋯∨t∗m)=z(t∗). | (3.32) |
As a consequence, t∗ is an optimal solution of problem (1.3).
According to Theorem 5, if we can determine the optimal solutions of all the subproblems {P1,P2,⋯,Pm}, then the optimal solution of problem (1.3) can be generated by those m optimal solutions. The resolution approach indicated in Theorem 5 is based on single-constraint programming (SCP), i.e., subproblems {P1,P2,⋯,Pm}. Thus, we call this the SCP-based resolution approach. Moreover, we summarize the resolution approach as the following SCP-based algorithm.
SCP-based Algorithm to obtain an optimal solution of problem (1.3)
Step 1: Construct the vector ˆt=(ˆt1,ˆt2,⋯,ˆtn) following (2.2).
Step 2: Apply the vector ˆt; check the consistency of system (1.2) following Theorem 1. If ˆt∈TA,b,d, then TA,b,d≠∅ and problem (1.3) is solvable. Continue to the next step. Otherwise, problem (1.3) is unsolvable; stop.
Step 3: Construct m subproblems as (3.4), denoted by {(P1),(P2),⋯,(Pm)}.
Step 4: For each i∈I, find an optimal solution of the subproblem (Pi) by applying the proposed Algorithm Ⅰ presented in Subsection 3.2. Suppose the obtained optimal solution of (Pi) is t∗i, ∀i∈I. Then, find m optimal solutions as {t∗1,t∗2,⋯,t∗m}.
Step 5: Generate the vector t∗=t∗1∨t∗2∨⋯∨t∗m. Then, by Theorem 5, t∗ is an optimal solution of problem (1.3).
In this section, we provide an illustrative example of our proposed SCP-based algorithm.
Example 2. We consider a P2P network system with 6 terminals. Let us suppose the P2P network system is described by the max-min FRI system as
b≤A∘t≤d, | (4.1) |
where
A=(0.30.70.60.80.60.70.80.20.60.30.40.60.30.40.20.50.90.60.90.60.80.20.30.40.40.80.60.30.20.50.40.50.80.20.80.3), | (4.2) |
b=(0.45,0.37,0.52,0.38,0.42,0.48), d=(0.75,0.78,0.85,0.76,0.77,0.89), t=(t1,t2,⋯,t6). We find an optimal solution of the following weighted minimax programming problem:
minz(t)=c1t1∨c2t2∨⋯∨c6t6,s.t.b≤A∘t≤d, | (4.3) |
where c=(c1,c2,⋯,c6)=(0.5,0.6,0.8,0.4,0.6,0.7).
Solution. Step 1: According to Ij={i∈I|aij>di}, I1={2,4}, I2={5}, I3={4}, I4={1}, I5={3}, and I6=∅. Based on these index sets, we can calculate the vector ˆt by (2.2). After calculation, we have ˆt=(ˆt1,ˆt2,⋯,ˆt6)=(0.76,0.77,0.76,0.75,0.85,1).
Step 2: We compute A∘ˆt as follows:
A∘ˆt=(0.30.70.60.80.60.70.80.20.60.30.40.60.30.40.20.50.90.60.90.60.80.20.30.40.40.80.60.30.20.50.40.50.80.20.80.3)∘(0.76,0.77,0.76,0.75,0.85,1)=(0.75,0.76,0.85,0.76,0.77,0.8). | (4.4) |
We know that b≤A∘ˆt≤d, i.e., ˆt fulfills system (4.1). Hence, system (4.1) is consistent, and problem (4.3) is solvable. We continue to the next step.
Step 3: Following (3.4), we construct the subproblems as follows.
(P1)minz(t)=0.5t1∨0.6t2∨0.8t3∨0.4t4∨0.6t5∨0.7t6,s.t.{0.45≤0.3∧t1∨0.7∧t2∨0.6∧t3∨0.8∧t4∨0.6∧t5∨0.7∧t6≤0.75,t≤(0.76,0.77,0.76,0.75,0.85,1). |
(P2)minz(t)=0.5t1∨0.6t2∨0.8t3∨0.4t4∨0.6t5∨0.7t6,s.t.{0.37≤0.8∧t1∨0.2∧t2∨0.6∧t3∨0.3∧t4∨0.4∧t5∨0.6∧t6≤0.78,t≤(0.76,0.77,0.76,0.75,0.85,1). |
(P3)minz(t)=0.5t1∨0.6t2∨0.8t3∨0.4t4∨0.6t5∨0.7t6,s.t.{0.52≤0.3∧t1∨0.4∧t2∨0.2∧t3∨0.5∧t4∨0.9∧t5∨0.6∧t6≤0.85,t≤(0.76,0.77,0.76,0.75,0.85,1). |
(P4)minz(t)=0.5t1∨0.6t2∨0.8t3∨0.4t4∨0.6t5∨0.7t6,s.t.{0.38≤0.9∧t1∨0.6∧t2∨0.8∧t3∨0.2∧t4∨0.3∧t5∨0.4∧t6≤0.76,t≤(0.76,0.77,0.76,0.75,0.85,1). |
(P5)minz(t)=0.5t1∨0.6t2∨0.8t3∨0.4t4∨0.6t5∨0.7t6,s.t.{0.42≤0.4∧t1∨0.8∧t2∨0.6∧t3∨0.3∧t4∨0.2∧t5∨0.5∧t6≤0.77,t≤(0.76,0.77,0.76,0.75,0.85,1). |
(P6)minz(t)=0.5t1∨0.6t2∨0.8t3∨0.4t4∨0.6t5∨0.7t6,s.t.{0.48≤0.4∧t1∨0.5∧t2∨0.8∧t3∨0.2∧t4∨0.8∧t5∨0.3∧t6≤0.89,t≤(0.76,0.77,0.76,0.75,0.85,1). |
Step 4: In this step, we apply our proposed Algorithm Ⅰ to find the optimal solutions of the subproblems {(P1),(P2),⋯,(P6)}.
According to (3.5), we find the index sets as Jˆt1={2,3,4,5,6}, Jˆt2={1,3,5,6}, Jˆt3={5,6}, Jˆt4={1,2,3,6}, Jˆt5={2,3,6}, and Jˆt1={2,3,5}.
Based on these index sets, we further compute the optimal indices by (3.8) as p∗1=4, p∗2=1, p∗3=5, p∗4=1, p∗5=2, and p∗6=2 or 5.
As a result, we can find the optimal solutions {t∗1,t∗2,⋯,t∗6} following (3.9). Since p∗1=4, we find the optimal solution to (P1) as
t∗1=(0,0,0,b1,0,0)=(0,0,0,0.45,0,0). |
Since p∗2=1, we find the optimal solution to (P2) as
t∗2=(b2,0,0,0,0,0)=(0.37,0,0,0,0,0). |
Since p∗3=5, we find the optimal solution to (P3) as
t∗3=(0,0,0,0,b3,0)=(0,0,0,0,0.52,0). |
Since p∗4=1, we find the optimal solution to (P4) as
t∗4=(b4,0,0,0,0,0)=(0.38,0,0,0,0,0). |
Since p∗5=2, we find the optimal solution to (P5) as
t∗5=(0,b5,0,0,0,0)=(0,0.42,0,0,0,0). |
Since p∗6=2 or 5, we find the optimal solution to (P6) as
t∗6=(0,b6,0,0,0,0)=(0,0.48,0,0,0,0), |
or
t∗6=(0,0,0,0,b6,0)=(0,0,0,0,0.48,0). |
Step 5: We generate the vector t∗=t∗1∨t∗2∨⋯∨t∗6. When t∗6=(0,b6,0,0,0,0)=(0,0.48,0,0,0,0), we have
t∗=t∗1∨t∗2∨⋯∨t∗6=(0,0,0,0.45,0,0)∨(0.37,0,0,0,0,0)∨(0,0,0,0,0.52,0)∨(0.38,0,0,0,0,0)∨(0,0.42,0,0,0,0)∨(0,0.48,0,0,0,0)=(0.38,0.48,0,0.45,0.52,0). |
When t∗6=(0,0,0,0,b6,0)=(0,0,0,0,0.48,0), we have
t∗′=t∗1∨t∗2∨⋯∨t∗6=(0,0,0,0.45,0,0)∨(0.37,0,0,0,0,0)∨(0,0,0,0,0.52,0)∨(0.38,0,0,0,0,0)∨(0,0.42,0,0,0,0)∨(0,0,0,0,0.48,0)=(0.38,0.42,0,0.45,0.52,0). |
According to Theorem 5, both t∗ and t∗′ are the optimal solutions of problem (4.3).
In this work, we establish a minimax program with max-min FRI constraints. Moreover, we propose the so-called SCP-based algorithm to identify an optimal solution. In the following section, we compare our problem and the proposed resolution algorithm to those presented in several existing works.
(ⅰ) The optimization problem investigated in this work has not been studied previously. This result is different from those reported in existing research.
In fact, minimax programming problems subject to addition-min FRIs were studied in [11,12,13,14,15,16,17]. In those problems, the optimization objective was a minimax function, but the constraints were the addition-min FRIs; they are different from those in our studied problem. Moreover, [28,29,30,31,32,33,34] minimized a linear objective function under the constraints of max-min FRIs, while [18,19,35,36,37] optimized a geometric objective function under the same constraints. Although the constraints are the same as those in our studied problem, the linear or geometric objective function is different from the minimax objective function, which appears in our problem. Consequently, our minimax programming problem with the addition-min FRI constraints is different from those presented in [11,12,13,14,15,16,17,18,19,28,29,30,31,32,33,34,35,36,37].
(ⅱ) The feasible domain of our optimization model is different from those employed in some relevant published works.
In the minimax optimization problems studied in [11,12,13,14,15,16,17], the feasible domain is the solution set to the addition-min FRIs. It has been formally proven that such a feasible domain is a convex set [2]. However, the feasible domain of our problem, i.e., the solution set to the max-min FRIs, should be nonconvex when the minimum is not unique [38]. In addition, a system of addition-min FRIs usually has infinitely many minimal solutions [2], in most cases. However, the number of minimal solutions to the max-min FRIs is always finite. Consequently, the properties of the feasible domain with an addition-min composition are much different from those with a max-min composition.
(ⅲ) The SCP-based algorithm is proposed for our optimization model; this approach is different from the existing resolution methods adopted for the relevant fuzzy relation optimization models in [11,12,13,14,15,16,17,18,19,28,29,30,31,32,33,34,35,36,37].
In [15], the dichotomy algorithm was proposed for searching for optimal solutions. The subproblem and single-variable approach was also proposed in [11,12,13,16,17]. In addition, [14] developed the so-called optimal-vector-based algorithm for minimax programming with addition-min FRI constraints. However, the branch and bound method [28,29,30,31,32,33,34], which is suitable for linear programming with max-min FRI constraints, and the value-matrix-based iterative method [35,36,37], which is suitable for geometric programming with max-min FRI constraints, are ineffective for our minimax programming problem with max-min FRI constraints. All of these existing methods for the relevant fuzzy relation optimization models are no longer effective for our problem due to their different optimization scenarios and properties of the feasible domains.
The P2P network system has been reduced to the max-min FRIs, i.e., system (1.2). To decrease network congestion in the P2P network system, we constructed and investigated a weighted minimax programming problem subject to system (1.2), i.e., problem (1.3). The purpose of this work is to propose an effective algorithm to produce an optimal solution of (1.3). We divided the original problem (1.3) into m subproblems. Each subproblem involves single-constraint programming. Algorithm Ⅰ was designed to find one of the optimal solutions of each subproblem. The optimal solution can be generated by the optimal solutions from those m subproblems. We further proposed the SCP-based algorithm to find an optimal solution to the original problem (1.3). A numerical example was given to verify the validity of the SCP-based algorithm. Moreover, Example 2 showed that the optimal solutions might not be unique.
In the future, based on the max-min FRIs for modeling the P2P network system, we plan to further consider the stability with respect to some given solutions of the max-min FRIs.
The authors declare they have not used Artificial Intelligence (AI) tools in the creation of this article.
This work was supported by the National Natural Science Foundation of China (12271132) and the Guangdong Basic and Applied Basic Research Foundation (2024A1515010532, 2023A1515011093, 2022A1515011460, 2023KQNCX041, 2021ZDJS044, QD202211, PNB2103).
The authors declare that they have no conflict of interest.
[1] |
Huikuri HV, Castellanos A, Myerburg RJ (2001) Sudden death due to cardiac arrhythmias. New Engl J Med 345: 1473-1482. https://doi.org/10.1056/NEJMra000650 ![]() |
[2] |
Srinivasan NT, Schilling RJ (2018) Sudden cardiac death and arrhythmias. Arrhyth Electrophysi Rev 7: 111. https://doi.org/10.15420/aer.2018:15:2 ![]() |
[3] |
John RM, Tedrow UB, Koplan BA, et al. (2012) Ventricular arrhythmias and sudden cardiac death. Lancet 380: 1520-1529. https://doi.org/10.1016/S0140-6736(12)61413-5 ![]() |
[4] |
Janse MJ, Wit AL (1989) Electrophysiological mechanisms of ventricular arrhythmias resulting from myocardial ischemia and infarction. Physiol Rev 69: 1049-1169. https://doi.org/10.1152/physrev.1989.69.4.1049 ![]() |
[5] |
Peretto G, Sala S, Rizzo S, et al. (2019) Arrhythmias in myocarditis: state of the art. Heart Rhythm 16: 793-801. https://doi.org/10.1016/j.hrthm.2018.11.024 ![]() |
[6] |
Kumar S, Stevenson WG, John RM (2015) Arrhythmias in dilated cardiomyopathy. Card Electrophy Clin 7: 221-233. https://doi.org/10.1016/j.hrthm.2018.11.024 ![]() |
[7] |
Tisdale JE, Chung MK, Campbell KB, et al. (2020) Drug-induced arrhythmias: a scientific statement from the American Heart Association. Circulation 142: e214-233. https://doi.org/10.1161/CIR.0000000000000905 ![]() |
[8] |
Behere SP, Weindling SN (2015) Inherited arrhythmias: The cardiac channelopathies. Ann Pediat Cardiol 8: 210. https://doi.org/10.4103/0974-2069.164695 ![]() |
[9] |
FISCH C (1973) Relation of electrolyte disturbances to cardiac arrhythmias. Circulation 47: 408-419. https://doi.org/10.1161/01.CIR.47.2.408 ![]() |
[10] |
Tse G (2016) Mechanisms of cardiac arrhythmias. J Arrhythm 32: 75-81. https://doi.org/10.1016/j.joa.2015.11.003 ![]() |
[11] |
Antzelevitch C, Burashnikov A (2011) Overview of basic mechanisms of cardiac arrhythmia. Card Electrophy Clin 3: 23-45. https://doi.org/10.1016/j.ccep.2010.10.012 ![]() |
[12] |
Marbán E (2002) Cardiac channelopathies. Nature 415: 213-218. https://doi.org/10.1038/415213a ![]() |
[13] |
Franz MR, Cima R, Wang D, et al. (1992) Electrophysiological effects of myocardial stretch and mechanical determinants of stretch-activated arrhythmias. Circulation 86: 968-978. https://doi.org/10.1161/01.CIR.86.3.968 ![]() |
[14] | Morand J, Arnaud C, Pepin JL, et al. (2018) Chronic intermittent hypoxia promotes myocardial ischemia-related ventricular arrhythmias and sudden cardiac death. Sci Rep 8: 1-8. https://doi.org/10.1038/s41598-018-21064-y |
[15] |
Orchard CH, Cingolani HE (1994) Acidosis and arrhythmias in cardiac muscle. Card Res 28: 1312-1319. https://doi.org/10.1093/cvr/28.9.1312 ![]() |
[16] |
Morris CE (2011) Voltage-gated channel mechanosensitivity: fact or friction?. Front Physiol 2: 25. https://doi.org/10.3389/fphys.2011.00025 ![]() |
[17] | Dehghani-Samani A, Madreseh-Ghahfarokhi S, Dehghani-Samani A (2019) Mutations of voltage-gated ionic channels and risk of severe cardiac arrhythmias. Acta Cardiol Sin 35: 99. https://doi.org/10.6515%2FACS.201903_35(2).20181028A |
[18] |
Li Q, Huang H, Liu G, et al. (2009) Gain-of-function mutation of Nav1. 5 in atrial fibrillation enhances cellular excitability and lowers the threshold for action potential firing. Biochem Biophys Res Commun 380: 132-137. https://doi.org/10.1016/j.bbrc.2009.01.052 ![]() |
[19] | Moskalenko A (2014) Cardiac Arrhythmias Mechanisms, Pathophysiology, and Treatment: 1-162. https://doi.org/10.5772/57008 |
[20] | Cardiac Arrhythmia Suppression Trial (CAST) Investigators.Preliminary report: effect of encainide and flecainide on mortality in a randomized trial of arrhythmia suppression after myocardial infarction. N Engl J Med (1989) 321: 406-412. https://doi.org/10.1056/nejm198908103210629 |
[21] |
Brooks MM, Gorkin L, Schron EB, et al. (1994) Moricizine and quality of life in the Cardiac Arrhythmia Suppression Trial II (CAST II). Control Clin Trials 15: 437-449. https://doi.org/10.1016/0197-2456(94)90002-7 ![]() |
[22] |
Kurian TK, Efimov IR (2010) Mechanisms of fibrillation: Neurogenic or myogenic? reentrant or focal? multiple or single?: Still puzzling after 160 years of inquiry. J Card Electrophysiol 21: 1274. https://doi.org/10.1111%2Fj.1540-8167.2010.01820.x ![]() |
[23] |
Calvillo L, Redaelli V, Ludwig N, et al. (2022) Quantum biology research meets pathophysiology and therapeutic mechanisms: a biomedical perspective. Quantum Rep 4: 148-172. https://www.mdpi.com/2624-960X/4/2/11 ![]() |
[24] |
Kim Y, Bertagna F, D'souza EM, et al. (2021) Quantum biology: An update and perspective. Quantum Rep 3: 80-126. https://www.mdpi.com/2624-960X/3/1/6 ![]() |
[25] |
Cao J, Cogdell RJ, Coker DF, et al. (2020) Quantum biology revisited. Sci Adv 6: eaaz4888. https://doi.org/10.1126/sciadv.aaz4888 ![]() |
[26] |
Slocombe L, Sacchi M, Al-Khalili (2022) An open quantum systems approach to proton tunnelling in DNA. Commun Phys 5: 109. https://doi.org/10.1038/s42005-022-00881-8 ![]() |
[27] |
Sutcliffe MJ, Scrutton NS (2002) A new conceptual framework for enzyme catalysis: Hydrogen tunneling coupled to enzyme dynamics in flavoprotein and quinoprotein enzymes. Eur J Biochem 269: 3096-3102. https://doi.org/10.1046/j.1432-1033.2002.03020.x ![]() |
[28] |
Qaswal AB (2019) Quantum tunneling of ions through the closed voltage-gated channels of the biological membrane: A mathematical model and implications. Quantum Rep 1: 219-225. https://www.mdpi.com/2624-960X/1/2/19 ![]() |
[29] | Miller DA (2008). Quantum mechanics for scientists and engineers. Cambridge University Press |
[30] |
Aryal P, Sansom MS, Tucker SJ (2015) Hydrophobic gating in ion channels. J Mol Biol 427: 121-130. https://doi.org/10.1016/j.jmb.2014.07.030 ![]() |
[31] |
Oelstrom K, Goldschen-Ohm MP, Holmgren M, et al. (2014) Evolutionarily conserved intracellular gate of voltage-dependent sodium channels. Nat Commun 5: 3420. https://doi.org/10.1038/ncomms4420 ![]() |
[32] |
Jensen MØ, Borhani DW, Lindorff-Larsen K, et al. (2010) Principles of conduction and hydrophobic gating in K+ channels. Proceedings of the National Academy of Sciences 107: 5833-5838. https://doi.org/10.1073/pnas.0911691107 ![]() |
[33] |
Trick JL, Aryal P, Tucker SJ, et al. (2015) Molecular simulation studies of hydrophobic gating in nanopores and ion channels. Biochem Society Transact 43: 146-150. https://doi.org/10.1042/BST20140256 ![]() |
[34] |
Rao S, Klesse G, Lynch CI, et al. (2021) Molecular simulations of hydrophobic gating of pentameric ligand gated ion channels: insights into water and ions. J Phys Chem B 125: 981-994. https://doi.org/10.1021/acs.jpcb.0c09285 ![]() |
[35] |
Chandra AK (1974). |
[36] |
Miyazaki T (2004). |
[37] | Serway RA, Moses CJ, Moyer CA (2004). Modern physics |
[38] |
Eckart C (1930) The penetration of a potential barrier by electrons. Phys Rev 35: 1303. https://doi.org/10.1103/PhysRev.35.1303 ![]() |
[39] |
Zhu F, Hummer G (2012) Drying transition in the hydrophobic gate of the GLIC channel blocks ion conduction. Biophys J 103: 219-227. http://dx.doi.org/10.1016/j.bpj.2012.06.003 ![]() |
[40] |
Rao S, Lynch CI, Klesse G, et al. (2018) Water and hydrophobic gates in ion channels and nanopores. Faraday Discuss 209: 231-247. https://doi.org/10.1039/C8FD00013A ![]() |
[41] |
Neale C, Chakrabarti N, Pomorski P, et al. (2015) Hydrophobic gating of ion permeation in magnesium channel CorA. PLoS Comput Biol 11: e1004303. https://doi.org/10.1371/journal.pcbi.1004303 ![]() |
[42] |
Khavrutskii IV, Gorfe AA, Lu B, et al. (2009) Free energy for the permeation of Na+ and Cl− ions and their ion-pair through a zwitterionic dimyristoyl phosphatidylcholine lipid bilayer by umbrella integration with harmonic fourier beads. J Am Chem Society 131: 1706-1716. https://doi.org/10.1021/ja8081704 ![]() |
[43] |
Vorobyov I, Olson TE, Kim JH, et al. (2014) Ion-induced defect permeation of lipid membranes. Biophys J 106: 586-597. http://dx.doi.org/10.1016/j.bpj.2013.12.027 ![]() |
[44] |
Leontiadou H, Mark AE, Marrink SJ (2007) Ion transport across transmembrane pores. Biophys J 92: 4209-4215. http://dx.doi.org/10.1529/biophysj.106.101295 ![]() |
[45] |
Zhang HY, Xu Q, Wang YK, et al. (2016) Passive transmembrane permeation mechanisms of monovalent ions explored by molecular dynamics simulations. J Chem Theory Comput 12: 4959-4969. https://doi.org/10.1021/acs.jctc.6b00695 ![]() |
[46] |
Chen F, Hihath J, Huang Z, et al. (2007) Measurement of single-molecule conductance. Annu Rev Phys Chem 58: 535-564. https://doi.org/10.1146/annurev.physchem.58.032806.104523 ![]() |
[47] |
Qaswal AB (2020) Quantum electrochemical equilibrium: Quantum version of the Goldman–Hodgkin–Katz equation. Quantum Rep 2: 266-277. https://www.mdpi.com/2624-960X/2/2/17 ![]() |
[48] |
Qaswal AB (2021) The role of quantum tunneling of ions in the pathogenesis of the cardiac arrhythmias due to channelopathies, ischemia, and mechanical stretch. Biophysics 66: 637-641. https://doi.org/10.1134/S0006350921040072 ![]() |
[49] |
Ababneh O, Qaswal AB, Alelaumi A, et al. (2021) Proton quantum tunneling: Influence and relevance to acidosis-induced cardiac arrhythmias/cardiac arrest. Pathophysiology 28: 400-436. https://www.mdpi.com/1873-149X/28/3/27 ![]() |
[50] |
Zhang XC, Yang H, Liu Z, et al. (2018) Thermodynamics of voltage-gated ion channels. Biophys Rep 4: 300-319. https://doi.org/10.1016/j.celrep.2021.109931 ![]() |
[51] |
Summhammer J, Salari V, Bernroider G (2012) A quantum-mechanical description of ion motion within the confining potentials of voltage-gated ion channels. J Integr Neurosci 11: 123-135. https://doi.org/10.1142/S0219635212500094 ![]() |
[52] |
Summhammer J, Sulyok G, Bernroider G (2018) Quantum dynamics and non-local effects behind ion transition states during permeation in membrane channel proteins. Entropy 20: 558. https://doi.org/10.1142/S0219635212500094 ![]() |
[53] |
Summhammer J, Sulyok G, Bernroider G (2020) Quantum mechanical coherence of K+ ion wave packets increases conduction in the KcsA ion channel. Appl Sci 10: 4250. https://www.mdpi.com/2076-3417/10/12/4250 ![]() |
[54] |
Wang K, Wang S, Yang L, et al. (2021) THz trapped ion model and THz spectroscopy detection of potassium channels. Nano Res 15: 3825-3833. https://doi.org/10.1007/s12274-021-3965-z ![]() |
[55] |
Karandashev K, Xu ZH, Meuwly M, et al. (2017) Kinetic isotope effects and how to describe them. Struct Dynam 4: 061501. https://doi.org/10.1063/1.4996339 ![]() |
[56] |
Sen A, Kohen A (2010) Enzymatic tunneling and kinetic isotope effects: chemistry at the crossroads. J Phys Org Chem 23: 613-619. https://doi.org/10.1002/poc.1633 ![]() |
[57] |
Eckhardt AK, Gerbig D, Schreiner PR (2018) Heavy atom secondary kinetic isotope effect on H-tunneling. J Phys Chem A 122: 1488-1495. https://doi.org/10.1021/acs.jpca.7b12118 ![]() |
[58] |
Nappi P, Miceli F, Soldovieri MV, et al. (2020) Epileptic channelopathies caused by neuronal Kv7 (KCNQ) channel dysfunction. Pflüg Arch-Eur J Phy 472: 881-898. https://doi.org/10.1007/s00424-020-02404-2 ![]() |
[59] | Niday Z, Tzingounis AV (2018) Potassium channel gain of function in epilepsy: an unresolved paradox. Neurosci 24: 368-380. https://doi.org/10.1177/1073858418763752 |
[60] |
Miceli F, Soldovieri MV, Ambrosino P, et al. (2015) Early-onset epileptic encephalopathy caused by gain-of-function mutations in the voltage sensor of Kv7. 2 and Kv7. 3 potassium channel subunits. J Neurosci 35: 3782-3793. https://doi.org/10.1523/JNEUROSCI.4423-14.2015 ![]() |
[61] |
Du W, Bautista JF, Yang H, et al. (2005) Calcium-sensitive potassium channelopathy in human epilepsy and paroxysmal movement disorder. Nat Genet 37: 733-738. https://doi.org/10.1038/ng1585 ![]() |
[62] |
Robinson RB, Siegelbaum SA (2003) Hyperpolarization-activated cation currents: from molecules to physiological function. Annu Rev Physiol 65: 453-480. https://doi.org/10.1146/annurev.physiol.65.092101.142734 ![]() |