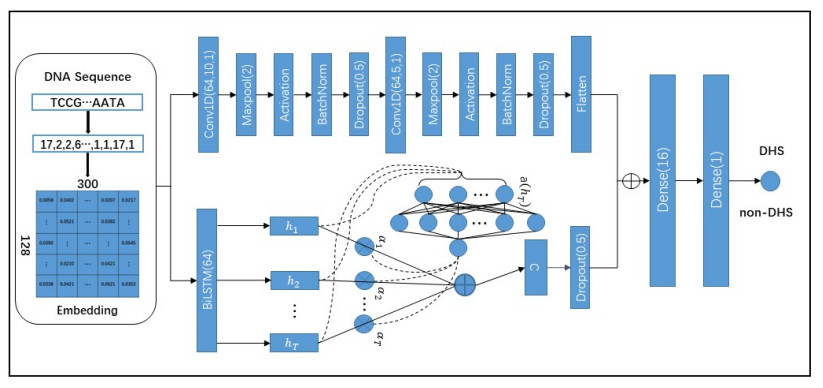
This study investigated the effect of Industrialization on carbon emissions through energy consumption for a panel of eight Organization of the Petroleum Exporting Countries (OPEC) and nine High Industrialised Countries over the period 1985 to 2020; the study employs the first generation and second-generation Unit root tests. The study further adopts the use of the Panel Autoregressive Distributed Lag Model, and Common Correlated Effect pooled mean group to estimate the parameters of the model for OPEC countries and High Industrialised Countries, respectively. In addition, the Dumitrescu-Hurlin Granger causality test is conducted to infer the direction of causality among the variables. The causality test result reveals that, in OPEC, energy consumed during industrial activity is not enough to cause carbon emission and carbon emission does not cause industrialisation to interact with energy consumption. Also, for highly industrialised countries, interaction of energy consumption and industrialization causes carbon emission, but carbon emission does not cause the interaction of energy consumption and industrialization. The estimated model shows that the interactive effect of Industrialization and energy consumption has no significant influence on carbon emissions in OPEC countries in the short and long run. In contrast, foreign direct investment and economic growth have a positive and significant effect on carbon emissions in the short run. However, for highly industrialised countries the study found that the interactive effect of energy industrialization and energy consumption has a positive and significant effect on carbon emissions in the short run. It is apparent from the study that energy consumption for industrial activities, particularly in highly industrialised countries, causes carbon emission and such policy makers should formulate policy that necessitate the use of green energy for industrial activities to improve environmental quality.
Citation: Ayodele Idowu, Obaika Micheal Ohikhuare, Munem Ahmad Chowdhury. Does industrialization trigger carbon emissions through energy consumption? Evidence from OPEC countries and high industrialised countries[J]. Quantitative Finance and Economics, 2023, 7(1): 165-186. doi: 10.3934/QFE.2023009
[1] | Fadoua El Moustaid, Amina Eladdadi, Lafras Uys . Modeling bacterial attachment to surfaces as an early stage of biofilm development. Mathematical Biosciences and Engineering, 2013, 10(3): 821-842. doi: 10.3934/mbe.2013.10.821 |
[2] | Yousef Rohanizadegan, Stefanie Sonner, Hermann J. Eberl . Discrete attachment to a cellulolytic biofilm modeled by an Itô stochastic differential equation. Mathematical Biosciences and Engineering, 2020, 17(3): 2236-2271. doi: 10.3934/mbe.2020119 |
[3] | Paolo Fergola, Marianna Cerasuolo, Edoardo Beretta . An allelopathic competition model with quorum sensing and delayed toxicant production. Mathematical Biosciences and Engineering, 2006, 3(1): 37-50. doi: 10.3934/mbe.2006.3.37 |
[4] | Nikodem J. Poplawski, Abbas Shirinifard, Maciej Swat, James A. Glazier . Simulation of single-species bacterial-biofilm growth using the Glazier-Graner-Hogeweg model and the CompuCell3D modeling environment. Mathematical Biosciences and Engineering, 2008, 5(2): 355-388. doi: 10.3934/mbe.2008.5.355 |
[5] | Ting Kang, Yanyan Du, Ming Ye, Qimin Zhang . Approximation of invariant measure for a stochastic population model with Markov chain and diffusion in a polluted environment. Mathematical Biosciences and Engineering, 2020, 17(6): 6702-6719. doi: 10.3934/mbe.2020349 |
[6] | Ana I. Muñoz, José Ignacio Tello . Mathematical analysis and numerical simulation of a model of morphogenesis. Mathematical Biosciences and Engineering, 2011, 8(4): 1035-1059. doi: 10.3934/mbe.2011.8.1035 |
[7] | Xiaomei Bao, Canrong Tian . Turing patterns in a networked vegetation model. Mathematical Biosciences and Engineering, 2024, 21(11): 7601-7620. doi: 10.3934/mbe.2024334 |
[8] | Chang-Yuan Cheng, Shyan-Shiou Chen, Xingfu Zou . On an age structured population model with density-dependent dispersals between two patches. Mathematical Biosciences and Engineering, 2019, 16(5): 4976-4998. doi: 10.3934/mbe.2019251 |
[9] | Jacques A. L. Silva, Flávia T. Giordani . Density-dependent dispersal in multiple species metapopulations. Mathematical Biosciences and Engineering, 2008, 5(4): 843-857. doi: 10.3934/mbe.2008.5.843 |
[10] | Yue Xia, Lijuan Chen, Vaibhava Srivastava, Rana D. Parshad . Stability and bifurcation analysis of a two-patch model with the Allee effect and dispersal. Mathematical Biosciences and Engineering, 2023, 20(11): 19781-19807. doi: 10.3934/mbe.2023876 |
This study investigated the effect of Industrialization on carbon emissions through energy consumption for a panel of eight Organization of the Petroleum Exporting Countries (OPEC) and nine High Industrialised Countries over the period 1985 to 2020; the study employs the first generation and second-generation Unit root tests. The study further adopts the use of the Panel Autoregressive Distributed Lag Model, and Common Correlated Effect pooled mean group to estimate the parameters of the model for OPEC countries and High Industrialised Countries, respectively. In addition, the Dumitrescu-Hurlin Granger causality test is conducted to infer the direction of causality among the variables. The causality test result reveals that, in OPEC, energy consumed during industrial activity is not enough to cause carbon emission and carbon emission does not cause industrialisation to interact with energy consumption. Also, for highly industrialised countries, interaction of energy consumption and industrialization causes carbon emission, but carbon emission does not cause the interaction of energy consumption and industrialization. The estimated model shows that the interactive effect of Industrialization and energy consumption has no significant influence on carbon emissions in OPEC countries in the short and long run. In contrast, foreign direct investment and economic growth have a positive and significant effect on carbon emissions in the short run. However, for highly industrialised countries the study found that the interactive effect of energy industrialization and energy consumption has a positive and significant effect on carbon emissions in the short run. It is apparent from the study that energy consumption for industrial activities, particularly in highly industrialised countries, causes carbon emission and such policy makers should formulate policy that necessitate the use of green energy for industrial activities to improve environmental quality.
DNase I hypersensitive sites (DHSs) are a specific genomic region in the chromatin, which is of hypersensitivity to cleavage by the DNase I enzyme [1]. DHSs untie its condensed structure, which makes the DNA exposed and accessible to the regulatory proteins. DHSs are functionally associated with the cis-regulatory elements such as promoters, enhancers, suppressors, insulators, as well as locus control regions [2]. Thus, mapping DHSs is becoming one of the most effective methods to precisely identify the location of many different regulatory elements in specific, well-studied genes [3]. Genetic variations in DHSs were found to be implicated in a wide spectrum of common diseases and traits, including Alzheimer's disease [4,5,6,7,8]. For example, DHSs were identified as driver distal regulatory elements in breast cancer, and were responsible for the aberrant expression of neighboring genes [9].
Identifying DHSs is of great interest to cis-regulatory element annotation. With advances in next-generation sequencing, many high-throughput techniques have been developed to detect DHSs in the past decades [5,10,11,12], such as the Southern blot approach [13] and DNase-seq [14]. Zhang et al. [15] developed a DNase-seq procedure for genome-wide mapping of DHSs in Arabidopsis thaliana and rice, while Wang et al. proposed a modified DNase-seq for genome-wide identification of DHSs in plants [16]. These experimentally verified DHSs were collected to be deposited in several public databases for further exploration [1].
Although these high-throughput techniques have contributed much to the discovery of thousands of DHSs, they have two inherent limitations: they are expensive and laborious, which make them insufficient to complete the challenging task of detecting DHSs from tremendous volumes of genomes. Computational identification is another routine to detect DHSs. The computational identification is defined as computational models or functions which are able to predict DHSs after they are trained by known DHSs. Computational identification is extremely cheaper and faster than high-throughput techniques for DHSs detection, and thus it is becoming an alternative to identify DHSs. Computational identification based on machine learning, especially deep learning, has extensively been applied to predict transcription factor binding sites [17,18,19,20,21,22,23] and to mine DNA/RNA motif [24]. For example, Wang et al. [17] created a hybrid convolutional recurrent neural network (RNN) for predicting transcription factor binding sites which obtained the state-of-the-art performance on 66 in vitro datasets. Zhang et al. [18] presented a deep learning-based method for transcription factor-DNA binding signal prediction that was able to deal with up to four transcription factor binding-related tasks. Wang et al. [19] employed fully convolutional neural networks (CNNs), along with gated recurrent units, to localize transcription factor binding sites. Following these successful practices, no less than 10 computational models or methods have been created for DHSs detection over the recent decade. These models or methods can be grouped into traditional machine learning-based methods [25,26,27,28], ensemble learning-based methods [29,30,31,32,33,34], and deep learning-based methods [35,36]. To the best of our knowledge, the first computational predictor for DHSs was the support vector machine-based method, which was proposed by Noble et al. [25] in 2005. This method used nucleotide composition as a representation of DNA sequences. Evidently, the nucleotide composition is unable to sufficiently represent DNA sequences because it drops out information about position and order. Feng et al. [37] used pseudo nucleotide composition [38,39,40] to integrate local and global sequence-order effects of DNA sequences. The pseudo nucleotide composition is similar to the pseudo amino acid composition which is a popular and effective representation for protein sequences. Liu et al. [30] computed nucleotide composition, reverse nucleotide composition, and pseudo dinucleotide composition to build three respective random forest-based classifiers. Three single random forest-based classifiers were fused as an ensemble classifier for DHSs prediction. Zhang et al. [41] employed reverse complement k-mer and dinucleotide-based auto covariance to represent DNA sequences. Zhang et al. [29] stacked multiple traditional machine learning algorithms to build an ensemble classifier for DHSs prediction. Zhang et al. [29] also employed the LASSO to reduce the dimension of representations and the SMOTE-Tomek to overcome imbalance between positive and negative samples. Zheng et al. [32] extracted composition information and physicochemical properties and used a boosting algorithm to optimize informative representations. Zhang et al. [33] proposed a dinucleotide-based spatial autocorrelation to represent DNA sequences. The aforementioned methods heavily rely on representations since it determines, to a great extent, whether methods are performed well or not. High effective representations are generally difficult to obtain in practice. Deep learning is merging as a representative of next-generation artificial intelligence, exhibiting vast potential to solve challenges unsolved in the past. Deep learning has been applied in a wide range of fields, including academic and industrial communities. Lyu et al. [36] developed a deep learning method for DHSs prediction which employed CNNs, along with the gate mechanism, to extract representation. To deal with variable-length inputs, Lyu et al. [36] used the spatial pyramid pooling [42], which was initially proposed to deal with variable-size images. The CNNs are able to capture local properties, and thus has extensively been used in the fields of image analysis and natural language processing. However, the CNNs are insufficient to capture dependencies between local parts, such as words. In the text sequences, dependencies between words are vital because they determine whether one understands it or not. Dao et al. [43] combined the CNNs and long-short term memory (LSTM), which is a special RNN for DHSs prediction. Dao et al. [43] stacked the CNNs and the LSTM in order, i.e., they first used the CNNs to capture local characteristics, and then used the LSTM to catch dependencies between local characteristics. The CNNs and the LSTM characterized different properties of sequences. Linearly stacking the CNN and the LSTM would lose their respective merits. In this paper, we stacked the CNNs and the LSTM in a parallel manner, which absorbed two respective representations learned by the CNN and the LSTM. In addition, we used feed-forward attention to improve representations by the LSTM.
We downloaded DHS datasets from 14 different tissues and 4 developmental stages in mouse which are available at the following website: http://lin-group.cn/server/iDHS-Deep/. These DHSs were collected according to the atlas of the DHSs created by Breeze et al. [44]. Dao et al. [43] further preprocessed these datasets for training of the iDHS-Deep model, including choosing the DHSs of 50 to 300 bp as positive samples, selecting specific DNA fragments as negative samples, removing or reducing the homology between sequences by using CD-Hit [45,46], which is a sequence-clustering tool, and dividing these datasets into the training set and the independent set at the ratio of 7 to 3. The numbers of positive and negative samples were not equal for the Stomach tissues, which were respectively 1062 and 2125 in the training set, and which were respectively 456 and 911 in the independent set. Except for Stomach tissues, the numbers of positive and negative samples were identical for each tissue or each developmental stage. The number of positive samples were respectively 7114, 10,299, 5766, 6519, 7424, 30,929, 6316, 4978, 1612, 2515, 3511, 2877, 1224, 7512, 52,418, 16,172 and 21,247 for 13 tissues (i.e., Forebrain, Midbrain, Hindbrain, Liver, Lung, Heart, Kidney, Limb, Thymus, Craniofacial, Retina, Muller retina, and Neural tube) and 4 developmental stages (i.e., ESC, Early-Fetal, Late-Fetal and Adult) in the training set, while they were respectively 3049, 4414, 2472, 2795, 3183, 13,256, 2708, 2134,692, 1078, 1506, 1234,525, 3224, 22,466, 6933, 9106 in the independent set.
As shown in Figure 1, the architecture of the proposed LangMoDHS mainly comprised the embedding, the CNNs, the Bi-LSTM followed by the feed-forward attention, the dropout, the fully-connected layer and the output layer. Unlike the iDHS-Deep [43], the LangMoDHS stacked the CNNs and the Bi-LSTM layer in a parallel manner. DNA sequences were first translated into integer sequences which were actually immediate input to the LangMoDHS. Each character in the DNA sequence was mapped into an integer as follows: A into 1, C into 2, G into 6 and T into 17 [43]. The same character-encoding scheme was adopted by the iDHS-Deep [43]. The integer sequences were embedded into continuous vectors, which were further characterized by the Bi-LSTM, CNNs and feed-forward attention. The output layer consisted of only a neuron that represented the probability of the input sequence containing the DHSs. The LangMoDHS is similar to the Deep6mAPred [47] in terms of the architecture, which is a deep learning method for predicting DNA N6-methyladenosine sites except that the former replaced one-hot encoding with an embedding layer and used two layers of CNN.
The embedding layer was intended to bridge the sequences of discrete variables and sequences of continuous vectors. In the deep neural network, the embedding layer is actually a specific neural network analogue to the Word2vec [48,49]. The embedding layer overcame the conventional issues, such as sparsity and no correlation between words. We used embedding in the Keras as the first layer in the LangMoDHS. The Keras is an open-source and extensively used deep learning toolkit.
The CNN is increasingly becoming one of the best popular neural networks, which was initially pioneered by Fukushima et al. [50] as an extension of the concept of receptive fields [51]. Since LeCun et al. [52] introduced the gradient back propagation algorithm to train the CNNs, the CNNs have attracted more and more attention, especially from deep learning communities. The CNNs contain two basic operations: convolution and pooling. The convolution is to multiply the input by a fixed convolutional kernel in the same layer. The convolutional kernel is like a filter in the digital signal field, which is shared by the same input. In addition, the CNNs use the pooling to reduce overfitting or computation. Actually, the pooling is a down-sampling method, including average pooling and max pooling. The CNNs are divided into 1D, 2D and 3D CNNs. The 2D CNN and the 3D CNN are generally applied for image data analysis, while the 1D CNN is applied to the field of text analysis. In this study, we used two 1D CNNs, each followed by the max pooling layer.
LSTM [53] is a special RNN that is different from the CNN. One of the main characteristics of LSTM is to share weights at all the time steps. LSTM is capable of preserving previous semantics by the cell state, which is controlled by the gate mechanism. For example, it uses an input gate to determine how much information is updated, and it uses a forget gate to decide what information is removed in the cell state. Therefore, LSTM is able to capture dependency between words in a sequence and thus is especially suitable to deal with sequence analysis. We used two LSTMs, i.e., Bi-LSTM, to capture the semantic relationship between words.
The attention mechanism is a newly developed technique of deep learning, and it has extensively been applied in the field of computer vision, natural language processing and bioinformatics. All of the deep learning-based language models, such as transformer and Bert employed attention mechanisms. Even Vaswani et al. declared that attention was all you need [54]. The attention mechanism is actually a scheme to allocate different weights to different parts. In the recent five years, many attention mechanisms have been proposed, including feed-forward attention [55] and self-attention [54]. Here, we used feed-forward attention to compensate for the deficiency of Bi-LSTM. The feed-forward attention is computed by
at=exp(et)∑Tk=1exp(ek), | (1) |
where
et=δ(ht). | (2) |
δ is the learnable parameter and ht is the hidden state at the time step of t in a Bi-LSTM. The output is a sum of the attentions multiplying corresponding hidden states, which is computed by
c=∑Tt=1θtht. | (3) |
We employed the Receiver Operator Characteristic (ROC) curve, Precision-Recall (PR) curve and F1-score to measure performance. The ROC curve plots the true positive rate (TPR) on the y-axis against the false positive rate (FPR) on the x-axis under the various thresholds. The PR curve plots the Precision on the y-axis against Recall on the x-axis. The F1-score is the summed mean of the Precision and Recall. These metrics are respectively defined by
FPR=FPFP+TN, | (4) |
TPR=Recall=TPTP+FN, | (5) |
Precision=TpTP+FP, | (6) |
F1−score=2×Precision×RecallPrecision+Recall. | (7) |
The more the ROC curve trends to the upper left, the better performance. The more upper right the PR curve trends to upper right, the better performance. The area under the ROC curve (AUROC) and the area under the PR curve (AUPRC) were used to quantitatively assess performance. The AUROC and AUPRC range from 0 to 1, where 1 means a perfect prediction, 0.5 is a random prediction, and 0 is a completely reverse prediction.
We performed a 5-fold cross-validation and an independent test to check the performance of the proposed method. In the 5-fold cross-validation, the training set was randomly divided into 5 parts of equal or approximate size. 4 parts were used to train the model, and the remaining part was used to test the trained model. The process was repeated five times. The independent test was to use the independent set to test the model trained by the training set. Figure 2A, B showed the AUROC values for the 5-fold cross-validations for the training sets from the 14 tissues and the 4 developmental stages, respectively. Obviously, all of the standard deviations of AUROC values over 5-fold cross-validation were less than 0.058, indicating that the LangMoDHS performed stably. Figure 3A, B showed the ROC curves for the independent set. The LangMoDHS achieved the best performance for the Heart tissue (AUROC = 0.960) and performed the worst for the Thymus tissue (AUROC = 0.770). The range of AUROC values for the 14 tissues was 0.19, implying that the LangMoDHS performed differently for different tissues. The LangMoDHS performed stably across the 4 stages. The highest AUROC value was 0.952, the lowest value was 0.910, and the range was 0.042, which was far smaller than that for the 14 tissues. Figures 4 and 5 respectively showed the PR curves and F1-scores for the independent set. The similar phenomenon was observed. For example, the LangMoDHS reached the best AUPRC value, as well as the best F1-score for the Heart tissue, and it achieved the best AUPRC value, as well as the best F1-score for the Early-Fetal stage.
We compared the LangMoDHS with the iDHS-Deep [43], which is a newly developed method for predicting DHSs. Table 1 listed the AUROC values of 5-fold cross-validation and independent test for the 14 tissues. The iDHS-Deep outperformed the LangMoDHS in both 5-fold cross-validation and independent test over 3 tissues: Stomach, Thymus, and Neural tube, while LangMoDHS completely outperformed iDHS-Deep over 4 tissues: Hindbrain, Liver, Lung and Heart. Over 3 tissues: Limb, Craniofacial, and Retina, iDHS-Deep performed better in 5-fold cross-validation, while LangMoDHS performed better in independent test. For the Kidney and Midbrain tissues, LangMoDHS was equal to iDHS-Deep in terms of the 5-fold cross-validation, while LangMoDHS performed better than iDHS-Deep in the independent test. Over the Forebrain and the Muller retina tissues, both methods is equivalent in the independent test. Table 2 listed all of the AUROC values in the 5-fold cross-validations and independent tests over 4 developmental stages. Although LangMoDHS performed worse than iDHS-Deep over two developmental stages: ESC and Late-Fetal in the 5-fold cross-validations, the former completely outperformed the latter over all 4 developmental stages in the independent tests.
DATASETS (TISSUES) | METHOD | |||
IDHS-Deep | LangMoDHS | IDHS-Deep | LangMoDHS | |
Training datasets | Independent datasets | |||
Forebrain | 0.934 | 0.938 | 0.939 | 0.939 |
Midbrain | 0.931 | 0.931 | 0.920 | 0.932 |
Hindbrain | 0.911 | 0.915 | 0.914 | 0.926 |
Liver | 0.927 | 0.932 | 0.924 | 0.935 |
Lung | 0.906 | 0.920 | 0.885 | 0.919 |
Heart | 0.955 | 0.957 | 0.949 | 0.960 |
Kidney | 0.934 | 0.934 | 0.923 | 0.938 |
Limb | 0.909 | 0.907 | 0.908 | 0.918 |
Stomach | 0.877 | 0.848 | 0.931 | 0.836 |
Thymus | 0.921 | 0.738 | 0.896 | 0.770 |
Craniofacial | 0.908 | 0.871 | 0.894 | 0.901 |
Retina | 0.902 | 0.900 | 0.894 | 0.911 |
Muller retina | 0.904 | 0.882 | 0.901 | 0.901 |
Neural tube | 0.896 | 0.763 | 0.900 | 0.804 |
DATASETS (STAGES) | METHOD | |||
IDHS-Deep | LangMoDHS | IDHS-Deep | LangMoDHS | |
Training datasets | Independent datasets | |||
ESC | 0.923 | 0.920 | 0.899 | 0.921 |
Early-Fetal | 0.949 | 0.950 | 0.940 | 0.952 |
Late-Fetal | 0.923 | 0.907 | 0.901 | 0.910 |
Adult | 0.916 | 0.925 | 0.905 | 0.930 |
We further tested LangMoDHS for the ability to predict DHSs across tissues (developmental stages). That is to say, the LangMoDHS trained by the dataset from A tissue (developmental stage), was used to predict DHSs from B tissue (developmental stage). Tables 3 and 4 listed the AUROC value of independent tests across tissues and developmental stages, respectively. Except for seven tissues: Heart, Kidney, Stomach, Thymus, Craniofacial, Muller retina, and Neural tube, LangMoDHS exhibited better performance over other tissues different from itself. For example, the LangMoDHS trained by the Forebrain training set achieved an AUROC value of 0.939 over the independent set from Forebrain, but obtained a better AUROC value (0.950) over the independent set from Heart tissue. This indicated that there existed a potential for LangMoDHS to predict DHSs across tissues. However, not all of the cross-tissue performance of LangMoDHS was better. For example, the LangMoDHS trained by Craniofacial training set reached an AUROC value of 0.901 over the Craniofacial independent set, which was better than those over all of the independent sets from other tissues. The similar phenomenon was observed in Table 4.
Training datasets | Forebr-ain | Midbr-ain | Hindb-rain | Liver | Lung | Heart | Kidn-ey | Limb | Stom-ach | Thym-us | Cranio-facial | Retina | Muller retina | Neural tube |
Independent datasets | ||||||||||||||
Forebrain | 0.939 | 0.939 | 0.922 | 0.923 | 0.926 | 0.944 | 0.904 | 0.930 | 0.789 | 0.721 | 0.796 | 0.914 | 0.708 | 0.791 |
Midbrain | 0.923 | 0.932 | 0.918 | 0.917 | 0.918 | 0.929 | 0.901 | 0.922 | 0.759 | 0.725 | 0.786 | 0.908 | 0.730 | 0.764 |
Hindbrain | 0.913 | 0.918 | 0.926 | 0.910 | 0.910 | 0.919 | 0.895 | 0.914 | 0.723 | 0.698 | 0.765 | 0.908 | 0.734 | 0.732 |
Liver | 0.901 | 0.907 | 0.907 | 0.935 | 0.924 | 0.907 | 0.922 | 0.910 | 0.671 | 0.658 | 0.728 | 0.901 | 0.747 | 0.672 |
Lung | 0.885 | 0.884 | 0.885 | 0.896 | 0.919 | 0.884 | 0.892 | 0.885 | 0.662 | 0.655 | 0.722 | 0.882 | 0.732 | 0.659 |
Heart | 0.950 | 0.953 | 0.938 | 0.938 | 0.939 | 0.960 | 0.920 | 0.947 | 0.797 | 0.710 | 0.789 | 0.934 | 0.700 | 0.792 |
Kidney | 0.889 | 0.896 | 0.901 | 0.919 | 0.913 | 0.884 | 0.938 | 0.894 | 0.594 | 0.608 | 0.673 | 0.903 | 0.755 | 0.624 |
Limb | 0.911 | 0.916 | 0.910 | 0.910 | 0.906 | 0.920 | 0.890 | 0.918 | 0.744 | 0.704 | 0.780 | 0.898 | 0.739 | 0.742 |
Stomach | 0.935 | 0.945 | 0.912 | 0.920 | 0.920 | 0.950 | 0.893 | 0.917 | 0.836 | 0.735 | 0.812 | 0.895 | 0.689 | 0.801 |
Thymus | 0.899 | 0.909 | 0.895 | 0.902 | 0.907 | 0.900 | 0.883 | 0.899 | 0.719 | 0.770 | 0.774 | 0.882 | 0.743 | 0.724 |
Craniofacial | 0.892 | 0.903 | 0.904 | 0.897 | 0.904 | 0.895 | 0.890 | 0.903 | 0.710 | 0.712 | 0.901 | 0.888 | 0.754 | 0.715 |
Retina | 0.888 | 0.895 | 0.897 | 0.899 | 0.892 | 0.887 | 0.894 | 0.893 | 0.673 | 0.674 | 0.742 | 0.911 | 0.747 | 0.680 |
Muller retina | 0.843 | 0.855 | 0.861 | 0.875 | 0.866 | 0.824 | 0.886 | 0.855 | 0.571 | 0.627 | 0.680 | 0.860 | 0.901 | 0.613 |
Neural tube | 0.931 | 0.933 | 0.913 | 0.915 | 0.918 | 0.933 | 0.895 | 0.926 | 0.750 | 0.711 | 0.788 | 0.910 | 0.747 | 0.804 |
Training datasets | ESC | Early-Fetal | Late-Fetal | Adult |
Independent datasets | ||||
ESC | 0.921 | 0.919 | 0.908 | 0.911 |
Early-Fetal | 0.940 | 0.952 | 0.937 | 0.934 |
Late-Fetal | 0.890 | 0.891 | 0.910 | 0.903 |
Adult | 0.905 | 0.902 | 0.908 | 0.930 |
LangMoDHS consisted mainly of three components: CNN, Bi-LSTM, and feed-forward attention. We investigated further how much the CNN, Bi-LSTM, and feed-forward attention contributed to the recognition of DHSs. Figure 6A–C showed the ROC curves of the independent tests by respectively removing the CNN, Bi-LSTM and attention from LangMoDHS. In contrast, Figure 6D showed the ROC curves of LangMoDHS over the independent tests. It was easy to observe that removing any one of 3 components caused the AUROC values to descend, implying that each contributed substantially to the recognition of DHSs. However, the contributions varied with the tissue (developmental stage) and component. That is to say, some components contributed more for some tissues or developmental stages than for other tissues or stages. For example, the AUROC value after removal of Bi-LSTM was higher than those after respective removal of the CNN and attention for Neural tube tissue, indicating that the CNN and attention contributed more than Bi-LSTM for Neural tube tissue. The AUROC value after removal of attention was more than those after the respective removal of the CNN and Bi-LSTM for Liver tissue, indicating that the CNN and Bi-LSTM contributed more than attention for the Liver tissue. The CNN contributed more than both the Bi-LSTM and attention for 4 developmental stages, the Bi-LSTM contributed more than the attention for 3 developmental stages: ESC, Late-Fetal, and Adult, while attention contributed more than Bi-LSTM for the Early-Fetal developmental stage.
We employed information entropy to analyze the motif of DHS sequences. The position-specific nucleotide matrix is defined by
Z=(z11z12⋯z1nz21z31z41z22z32⋯⋯z42⋯z2nz3nz4n), | (8) |
where Zin denotes the occurring probability of the nucleotide i at the position j, and n is the length of the sequence. The position-specific nucleotide matrix is estimated by computing the position-specific nucleotide frequencies over all DHS sequences in the benchmark dataset. The nucleotide information entropy and the position information entropy are respectively defined by
NPi=∑nj=1−Zijlog(Zij) | (9) |
and
PPj=∑4i=1−Zijlog(Zij). | (10) |
The lower the information entropy, the more certain the distribution of the nucleotides in the sequences. Tables 5 and 6 showed the nucleotide information entropy for all 14 the tissues and 4 developmental stages, respectively. The nucleotide information entropy of DHSs was lower than those of non-DHSs, indicating that the distribution of nucleotides of DHSs was more certain than those of non-DHSs. The nucleotide information entropy was tissue-specific and stage-specific. For example, the nucleotide information entropy for the Muller retina tissue was lower than those for the Kidney tissue, and the nucleotide information entropy for the Early-Fetal stage was lower than those for the Late-Fetal stage. This implied that the distribution of nucleotides was tissue-specific and stage-specific. Figures 7 and 8 showed the positions of entropy values for all 14 the tissues and all 4 stages, respectively. It can be obviously observed that the position information entropy in the range of 230 to 300 was far less than 2, implying that the distribution of nucleotides in these regions were not completely stochastic. In addition, at the nearby position of 100, the position information entropy for tissues was lower than 2, indicating that the distribution of nucleotides was more certain in these regions.
DATASETS (TISSUES) | Information entropy | |||||||||||
A | T | C | G | |||||||||
POS | NEG | ALL | POS | NEG | ALL | POS | NEG | ALL | POS | NEG | ALL | |
Forebrain | 7.782 | 7.997 | 7.922 | 7.793 | 8.001 | 7.914 | 7.782 | 7.999 | 7.909 | 7.789 | 7.997 | 7.925 |
Midbrain | 7.729 | 7.998 | 7.912 | 7.736 | 8.001 | 7.901 | 7.728 | 7.997 | 7.895 | 7.732 | 8.000 | 7.913 |
Hindbrain | 7.796 | 7.997 | 7.927 | 7.804 | 7.994 | 7.918 | 7.794 | 7.997 | 7.917 | 7.795 | 7.994 | 7.923 |
Liver | 7.775 | 8.001 | 7.918 | 7.769 | 7.998 | 7.908 | 7.758 | 7.998 | 7.904 | 7.768 | 8.000 | 7.915 |
Lung | 7.770 | 7.993 | 7.910 | 7.761 | 7.993 | 7.902 | 7.767 | 7.993 | 7.904 | 7.763 | 7.990 | 7.908 |
Heart | 7.842 | 7.992 | 7.935 | 7.837 | 7.994 | 7.924 | 7.831 | 7.992 | 7.919 | 7.844 | 7.993 | 7.936 |
Kidney | 7.732 | 7.990 | 7.895 | 7.724 | 7.994 | 7.894 | 7.725 | 7.988 | 7.890 | 7.727 | 7.988 | 7.891 |
Limb | 7.781 | 8.002 | 7.926 | 7.787 | 8.001 | 7.916 | 7.777 | 7.996 | 7.911 | 7.786 | 7.999 | 7.925 |
Stomach | 7.788 | 7.999 | 7.957 | 7.767 | 7.992 | 7.934 | 7.765 | 7.996 | 7.937 | 7.772 | 8.000 | 7.956 |
Thymus | 7.592 | 7.979 | 7.867 | 7.613 | 7.980 | 7.857 | 7.602 | 7.984 | 7.859 | 7.612 | 7.987 | 7.881 |
Craniofacial | 7.620 | 7.994 | 7.880 | 7.634 | 7.992 | 7.870 | 7.632 | 7.994 | 7.871 | 7.626 | 7.991 | 7.878 |
Retina | 7.714 | 7.984 | 7.891 | 7.705 | 7.986 | 7.882 | 7.718 | 7.986 | 7.886 | 7.717 | 7.982 | 7.891 |
Muller retina | 7.571 | 7.990 | 7.862 | 7.560 | 7.995 | 7.873 | 7.581 | 7.994 | 7.876 | 7.555 | 7.988 | 7.858 |
Neural tube | 7.770 | 8.001 | 7.908 | 7.695 | 7.997 | 7.890 | 7.694 | 7.994 | 7.888 | 7.690 | 7.994 | 7.901 |
DATASETS (STAGES) | Information Entropy | |||||||||||
A | T | C | G | |||||||||
POS | NEG | ALL | POS | NEG | ALL | POS | NEG | ALL | POS | NEG | ALL | |
ESC | 7.717 | 7.992 | 7.904 | 7.708 | 8.000 | 7.888 | 7.710 | 7.993 | 7.883 | 7.720 | 7.997 | 7.908 |
Early-Fetal | 7.830 | 7.995 | 7.934 | 7.828 | 7.998 | 7.924 | 7.821 | 7.996 | 7.920 | 7.832 | 7.996 | 7.935 |
Late-Fetal | 7.650 | 7.998 | 7.888 | 7.656 | 8.000 | 7.885 | 7.652 | 7.998 | 7.881 | 7.659 | 7.998 | 7.890 |
Adult | 7.763 | 7.992 | 7.908 | 7.754 | 7.994 | 7.900 | 7.757 | 7.993 | 7.900 | 7.755 | 7.992 | 7.905 |
To facilitate better using DHS sites and non-DHS sites, we have provided a useful web server at http:/www.biolscience.cn/LangMoDHS/. The web server interface is illustrated in Figure 9. Users first select the organization or developmental stage that needs to be predicted. Then, users input the sequence into the inputting box in the FASTA format. Alternatively, users upload a sequence file in the FASTA format. Finally, by clicking the submit button, users will get the predictive result in a certain amount of time which is determined by the number of inputted sequences.
DHSs play a key role in the cellular process. Sequence motif of DHSs is complicated, and thus, identifying DHSs is a challenging task at present. We have presented a deep language model for detecting DHSs in mouse genome. Extensive experiments showed that the LangMoDHS is an effective and efficient method for detecting DHSs. However, LangMoDHS performed differently with tissues and developmental stages. The LangMoDHS performed best for the Heart tissue, where the AUROC, AUPRC, and F1-score values were 0.960, 0.966, and 0.875, respectively, while it performed worse for 2 tissues, i.e., Thymus and Stomach, where the minimum AUROC and the minimum AUPRC values were 0.770 and 0.637, respectively. The range between the maximum and the minimum AUPRC value was up to 0.329, indicating that the sequence motif of DHSs would vary with tissue. This analysis also indicated that LangMoDHS is tissue-specific and stage-specific to predict DHSs.
It is desirable to develop a universal method which is able to detect DHSs in all tissues or species. However, there is a difference between tissues and species, so it is very difficult to develop such a universal method in practice. Like the iDHS-Deep [43], the LangMoDHS exhibited a certain ability to detect DHSs across tissues or developmental stages. The LangMoDHS achieved better or competitive performance across tissues and developmental stages, indicating that these tissues and stages would be of a similar mechanism of DHSs.
As mentioned previously, there are many computational approaches to detect DHSs. Compared with the methods [25,26,27,28,29,30,31,33,34,35,37], the LangMoDHS is an end-to-end method which requires no artificial design of features. The iDHS-Deep [43] is a newly developed deep-learning-based method to predict DHSs. The iDHS-Deep consisted mainly of two CNN layers and LSTM. The LSTM was attached at the end of the second CNN layer. The main difference between the iDHS-Deep and the LangMoDHS is that the latter used CNNs and Bi-LSTM in a parallel manner. The CNNs and the Bi-LSTM capture different characterization of the DHSs sequences respectively. Therefore, using CNNs and Bi-LSTM in a parallel manner would be more helpful to accumulate different characterization than stacking CNNs and Bi-LSTM in order. This might be a reason why the LangMoDHS performed better than the iDHS-Deep for most tissues and stages. Another difference is that the LangMoDHS to use feed-forward attention to improve the representations captured by the Bi-LSTM. Although the LangMoDHS exhibited competitive performance, the interpretability needs improving.
Due to limitations of the methods or techniques, precisely and high effectively identifying DHSs remains challenging. We have presented a deep learning-based language model for computationally predicting DHSs in mouse genomes. Our method achieved competitive performance with the state-of-the-art methods. We developed a user-friendly web server to facilitate the identification of DHSs. The LangMoDHS presented has certain ability to predict DHSs across tissues and across stages, and it is tissue-specific and stage-specific. The nucleotide distributions of DHSs in some regions, such as nearby the position of 100 and the range from 230 to 300, is more certain.
This work was supported by the National Natural Science Foundation of China (62272310, 62162025), Hunan Provincial Natural Science Foundation of China (2022JJ50177, 2020JJ4034), Scientific Research Fund of Hunan Provincial Education Department (21A0466, 19A215), and Shaoyang University Innovation Foundation for Postgraduate (CX2022SY046).
The authors declare there is no conflict of interest.
[1] |
Azam A, Rafiq M, Shafique M et al. (2021) Renewable electricity generation and economic growth nexus in developing countries: An ARDL approach. Econ Res-Ekonomska Istraživanja 34: 2423–2446. https://doi.org/10.1080/1331677X.2020.1865180 doi: 10.1080/1331677X.2020.1865180
![]() |
[2] | Akpan UF, Akpan GE (2011) The contribution of energy consumption to climate change: A feasible policy direction. Int J Energ Econ Policy 2: 21–33. |
[3] |
Antoci A, Russu P, Sordi S, et al. (2014) Industrialization and environmental externalities in a Solow-type model. J Econ Dyn Control 47: 211–224. http://dx.doi.org/10.1016/j.jedc.2014.08.009 doi: 10.1016/j.jedc.2014.08.009
![]() |
[4] |
Alam MS, Alam MN, Murshed M, et al. (2022) Pathways to securing environmentally sustainable economic growth through efficient use of energy: a bootstrapped ARDL analysis. Environ Sci Pollut R 29: 50025–50039. https://doi.org/10.1007/s11356-022-19410-9 doi: 10.1007/s11356-022-19410-9
![]() |
[5] |
Alam S, Fatima A, Butt MS (2007) Sustainable development in Pakistan in the context of energy consumption demand and environmental degradation. J Asian Econ 18: 825–837. https://doi.org/10.1016/j.asieco.2007.07.005 doi: 10.1016/j.asieco.2007.07.005
![]() |
[6] |
Bekun FV, Alola AA, Sarkodie SA (2019) Toward a sustainable environment: Nexus between CO2 emissions, resource rent, renewable and nonrenewable energy in 16-EU countries. Sci Total Environ 657: 1023–1029. https://doi.org/10.1016/j.scitotenv.2018.12.104 doi: 10.1016/j.scitotenv.2018.12.104
![]() |
[7] | Chudik A, Pesaran M, Hashem T (2013) Common Correlated Effects Estimation of Heterogeneous Dynamic Panel Data Models with Weakly Exogenous Regressors. Center for Economic Studies and ifo Institute (CESifo), Munich Working Paper, No. 4232 https://doi.org/10.2139/ssrn.2265296 |
[8] |
Chudik A, Pesaran MH (2015) Common correlated effects estimation of heterogeneous dynamic panel data models with weakly exogenous regressors. J Econometrics 188: 393–420. https://doi.org/10.1016/j.jeconom.2015.03.007 doi: 10.1016/j.jeconom.2015.03.007
![]() |
[9] |
Danmaraya IA, Jakada AH, Mahmood S, et al. (2021) Heterogeneous effect of oil production on environmental degradation: panel evidence from OPEC member countries. Int J Energ Sector Manage 16: 774–793. https://doi.org/10.1108/IJESM-04-2021-0009 doi: 10.1108/IJESM-04-2021-0009
![]() |
[10] |
De V Cavalcanti T, Mohaddes K, Raissi M (2014) Commodity Price Volatility and The Sources of Growth. J Appl Econometrics. 30: 857–873. https://doi.org/10.1002/jae.2407 doi: 10.1002/jae.2407
![]() |
[11] |
Dogan E, Inglesi-Lotz R (2020) The impact of economic structure to the environmental Kuznets curve (EKC) hypothesis: evidence from European countries. Environ Sci Pollut Res 27: 12717–12724. https://doi.org/10.1007/s11356-020-07878-2 doi: 10.1007/s11356-020-07878-2
![]() |
[12] |
Friedlingstein P, O'sullivan M, Jones M, et al. (2020) Global carbon budget 2020. Earth Syst Sci Data 12: 3269–3340. https://doi.org/10.5194/essd-12-3269-2020 doi: 10.5194/essd-12-3269-2020
![]() |
[13] | IEA (2021) Global Energy Review: CO2 Emissions in 2020, IEA, Paris. Available from: https://www.iea.org/articles/global-energy-review-co2-emissions-in-2020 |
[14] |
Levin A, Lin CF, Chu CSJ (2002) Unit root tests in panel data: Asymptotic and finite-sample properties. J Econometrics 108: 1–24. https://doi.org/10.1016/S0304-4076(01)00098-7 doi: 10.1016/S0304-4076(01)00098-7
![]() |
[15] |
Li K, Lin B (2015) Impacts of urbanisation and Industrialization on energy consumption/CO 2 emissions: Does the level of development matter? Renew Sust Energ Rev 52: 1107–1122. https://doi.org/10.1016/j.rser.2015.07.185 doi: 10.1016/j.rser.2015.07.185
![]() |
[16] |
Li L, Hong X, Tang D, et al. (2016) GHG Emissions, Economic Growth and Urbanization: A Spatial Approach. Sustainability 8: 462–477. https://doi.org/10.3390/su8050462 doi: 10.3390/su8050462
![]() |
[17] |
Lin B, Omojub OE, Okonkwo JC (2015) Impact of Industrialization on CO2 emissions in Nigeria. Renew Sust Energ Rev 52: 1228–1239. https://doi.org/10.1016/j.rser.2015.07.164 doi: 10.1016/j.rser.2015.07.164
![]() |
[18] |
Liu X, Bae J (2018) Urbanization and Industrialization Impact of CO2 Emissions in China. J Clean Prod 172:178–186. https://doi.org/10.1016/j.jclepro.2017.10.156 doi: 10.1016/j.jclepro.2017.10.156
![]() |
[19] |
Lu W (2017) Greenhouse Gas Emissions, Energy Consumption and Economic Growth: A Panel Cointegration Analysis for 16 Asian Countries. Int J Env Res Public He 14: 1436–1450. https://doi.org/10.3390/ijerph14111436 doi: 10.3390/ijerph14111436
![]() |
[20] | Magazzino C, Mele M (2022) A new machine learning algorithm to explore the CO2 emissions-energy use-economic growth trilemma. Ann Oper Res, 1–19. https://doi.org/10.1007/s10479-022-04787-0 |
[21] | Majeed M, Tauqir A (2020) Effects of Urbanization, Industrialization, Economic Growth, Energy Consumption and Financial Development on Carbon Emissions: An Extended STIRPAT Model for Heterogeneous Income Groups. Pak J Commer Soc Sci 14: 652–681. |
[22] |
Manigandan P, Alam MS, Alagirisamy K, et al. (2023) Realizing the Sustainable Development Goals through technological innovation: Juxtaposing the economic and environmental effects of financial development and energy use. Environ Sci Pollut Res 30: 8239–8256. https://doi.org/10.1007/s11356-022-22692-8 doi: 10.1007/s11356-022-22692-8
![]() |
[23] | Majeed MT, Luni T (2019) Renewable energy, water, and environmental degradation: A global panel data approach. Pak J Commer Soc Sci 13: 749–778. |
[24] |
Nusair SA (2019) Oil price and inflation dynamics in the Gulf Cooperation Council countries. Energy 181: 997–1011. https://doi.org/10.1016/j.energy.2019.05.208. doi: 10.1016/j.energy.2019.05.208
![]() |
[25] | Olivier J, Peters W (2020) Trends in global CO2 and total greenhouse gas emissions: 2020 Report. The Hague: PBL Netherlands Environmental Assessment Agency. |
[26] | OECD (2016) "Oil production", In: OECD Factbook 2015–2016: Economic, Environmental and Social Statistics, OECD Publishing, Paris. https://doi.org/10.1787/factbook-2015-44-en |
[27] | Pesaran MH, Shin Y (1999) An Autoregressive Distributed Lag Modelling Approach to Cointegration Analysis, In: Strom, S., Ed., Chapter 11 in Econometrics and Economic Theory in the 20th Century the Ragnar Frisch Centennial Symposium, Cambridge University Press, Cambridge, 371–413. https://doi.org/10.1017/CBO9781139052221.011 |
[28] |
Poumanyvong P, Kaneko S (2010) Does urbanization lead to less energy use and lower CO2 emissions? A cross-country analysis. Ecol Econ 70: 434–444. https://doi.org/10.1016/j.ecolecon.2010.09.029 doi: 10.1016/j.ecolecon.2010.09.029
![]() |
[29] |
Ross L, Arrow K, Cialdini R, et al. (2016) The Climate Change Challenge and Barriers to the Exercise of Foresight Intelligence. BioScience 66: 363–370. https://doi.org/10.1093/biosci/biw025 doi: 10.1093/biosci/biw025
![]() |
[30] | Samreen I, Majeed M (2020) Spatial econometric model of the spillover effects of financial development on carbon emissions: A global analysis. Pak J Commer Soc Sci 14: 569–202. |
[31] | Sepehrdoust H, Zamani S (2017) The Challenge of Economic Growth and Environmental Protection in Developing Economies. Iran Econ Rev 21: 865–883. |
[32] | Samargandi N, Fidrmuc J, Ghosh S (2013) Is the relationship between financial development and economic growth monotonic for middle income countries? Is there relationship between financial development and economic. Economics and Finance Working Paper Series 13-2. Available from: http://www.brunel.ac.uk/economics |
[33] |
Tsani SZ (2010) Energy consumption and economic growth: A causality analysis for Greece. Energ Econ 32: 582–590. https://doi.org/10.1016/j.eneco.2009.09.007 doi: 10.1016/j.eneco.2009.09.007
![]() |
[34] |
Wang S, Li G, Fang C (2018) Urbanization, economic growth, energy consumption, and CO2 emissions: Empirical evidence from countries with different income levels. Renew Sust Energ Rev 81: 2144–2159. https://doi.org/10.1016/j.rser.2017.06.025 doi: 10.1016/j.rser.2017.06.025
![]() |
[35] |
Xu B, Lin B (2015) How Industrialization and Urbanization Process Impacts on CO2 Emissions in China: Evidence from Non-parametric Additive Regression Models. Energ Econ 48: 188–202. https://doi.org/10.1016/j.eneco.2015.01.005. doi: 10.1016/j.eneco.2015.01.005
![]() |
[36] |
Xiaoqing Z, Jianlan R (2011) The Relationship between Co2 Emissions and Industrial Structure Adjustment for Shandong Province. Energ Proc 5: 1121–1125. https://doi.org/10.1016/j.egypro.2011.03.197 doi: 10.1016/j.egypro.2011.03.197
![]() |
[37] |
Zhou X, Zhang J, Li J (2013) Industrial structural transformation and carbon dioxide emissions in China. Energ Policy 57: 43–51. https://doi.org/10.1016/j.egypro.2011.03.197 doi: 10.1016/j.egypro.2011.03.197
![]() |
[38] |
Zhu Z, Liu Y, Tian X, et al. (2017) CO2 emissions from the industrialization and urbanization processes in the manufacturing center Tianjin in China. J Clean Prod 168: 867–875. https://doi.org/10.1016/j.jclepro.2017.08.245 doi: 10.1016/j.jclepro.2017.08.245
![]() |
1. | Sarangam Majumdar, Sukla Pal, Information transmission in microbial and fungal communication: from classical to quantum, 2018, 12, 1873-9601, 491, 10.1007/s12079-018-0462-6 | |
2. | H.J. Eberl, E.M. Jalbert, A. Dumitrache, G.M. Wolfaardt, A spatially explicit model of inverse colony formation of cellulolytic biofilms, 2017, 122, 1369703X, 141, 10.1016/j.bej.2017.03.007 | |
3. | B. D’Acunto, L. Frunzo, I. Klapper, M.R. Mattei, P. Stoodley, Mathematical modeling of dispersal phenomenon in biofilms, 2019, 307, 00255564, 70, 10.1016/j.mbs.2018.07.009 | |
4. | M. R. Mattei, L. Frunzo, B. D’Acunto, Y. Pechaud, F. Pirozzi, G. Esposito, Continuum and discrete approach in modeling biofilm development and structure: a review, 2018, 76, 0303-6812, 945, 10.1007/s00285-017-1165-y | |
5. | Liu Feng, Dai Xiangjuan, Mei Qicheng, Ling Guang, Wang Xinmei, 2018, Bifurcation Analysis and Control of Fractional-Order Quorum Sensing Network, 978-988-15639-5-8, 10197, 10.23919/ChiCC.2018.8483057 | |
6. | Jia Zhao, Qi Wang, Three-Dimensional Numerical Simulations of Biofilm Dynamics with Quorum Sensing in a Flow Cell, 2017, 79, 0092-8240, 884, 10.1007/s11538-017-0259-4 | |
7. | Hana Ueda, Kristina Stephens, Konstantina Trivisa, William E. Bentley, Sang Yup Lee, Bacteria Floc, but Do They Flock? Insights from Population Interaction Models of Quorum Sensing, 2019, 10, 2150-7511, 10.1128/mBio.00972-19 | |
8. | Pavel Zarva, Hermann J. Eberl, 2020, Chapter 17, 978-3-030-50435-9, 228, 10.1007/978-3-030-50436-6_17 | |
9. | Estefanía Garibay‐Valdez, Luis Rafael Martínez‐Córdova, Francisco Vargas‐Albores, Maurício G. C. Emerenciano, Anselmo Miranda‐Baeza, Edilmar Cortés‐Jacinto, Ángel M. Ortiz‐Estrada, Francesco Cicala, Marcel Martínez‐Porchas, The biofouling process: The science behind a valuable phenomenon for aquaculture, 2022, 1753-5123, 10.1111/raq.12770 | |
10. | Christoph Helmer, Ansgar Jüngel, Antoine Zurek, Analysis of a finite-volume scheme for a single-species biofilm model, 2023, 185, 01689274, 386, 10.1016/j.apnum.2022.12.002 | |
11. | Blessing O. Emerenini, Hermann J. Eberl, Reactor scale modeling of quorum sensing induced biofilm dispersal, 2022, 418, 00963003, 126792, 10.1016/j.amc.2021.126792 | |
12. | Christoph Helmer, Ansgar Jüngel, Existence analysis for a reaction-diffusion Cahn–Hilliard-type system with degenerate mobility and singular potential modeling biofilm growth, 2023, 0, 1078-0947, 0, 10.3934/dcds.2023069 |
DATASETS (TISSUES) | METHOD | |||
IDHS-Deep | LangMoDHS | IDHS-Deep | LangMoDHS | |
Training datasets | Independent datasets | |||
Forebrain | 0.934 | 0.938 | 0.939 | 0.939 |
Midbrain | 0.931 | 0.931 | 0.920 | 0.932 |
Hindbrain | 0.911 | 0.915 | 0.914 | 0.926 |
Liver | 0.927 | 0.932 | 0.924 | 0.935 |
Lung | 0.906 | 0.920 | 0.885 | 0.919 |
Heart | 0.955 | 0.957 | 0.949 | 0.960 |
Kidney | 0.934 | 0.934 | 0.923 | 0.938 |
Limb | 0.909 | 0.907 | 0.908 | 0.918 |
Stomach | 0.877 | 0.848 | 0.931 | 0.836 |
Thymus | 0.921 | 0.738 | 0.896 | 0.770 |
Craniofacial | 0.908 | 0.871 | 0.894 | 0.901 |
Retina | 0.902 | 0.900 | 0.894 | 0.911 |
Muller retina | 0.904 | 0.882 | 0.901 | 0.901 |
Neural tube | 0.896 | 0.763 | 0.900 | 0.804 |
DATASETS (STAGES) | METHOD | |||
IDHS-Deep | LangMoDHS | IDHS-Deep | LangMoDHS | |
Training datasets | Independent datasets | |||
ESC | 0.923 | 0.920 | 0.899 | 0.921 |
Early-Fetal | 0.949 | 0.950 | 0.940 | 0.952 |
Late-Fetal | 0.923 | 0.907 | 0.901 | 0.910 |
Adult | 0.916 | 0.925 | 0.905 | 0.930 |
Training datasets | Forebr-ain | Midbr-ain | Hindb-rain | Liver | Lung | Heart | Kidn-ey | Limb | Stom-ach | Thym-us | Cranio-facial | Retina | Muller retina | Neural tube |
Independent datasets | ||||||||||||||
Forebrain | 0.939 | 0.939 | 0.922 | 0.923 | 0.926 | 0.944 | 0.904 | 0.930 | 0.789 | 0.721 | 0.796 | 0.914 | 0.708 | 0.791 |
Midbrain | 0.923 | 0.932 | 0.918 | 0.917 | 0.918 | 0.929 | 0.901 | 0.922 | 0.759 | 0.725 | 0.786 | 0.908 | 0.730 | 0.764 |
Hindbrain | 0.913 | 0.918 | 0.926 | 0.910 | 0.910 | 0.919 | 0.895 | 0.914 | 0.723 | 0.698 | 0.765 | 0.908 | 0.734 | 0.732 |
Liver | 0.901 | 0.907 | 0.907 | 0.935 | 0.924 | 0.907 | 0.922 | 0.910 | 0.671 | 0.658 | 0.728 | 0.901 | 0.747 | 0.672 |
Lung | 0.885 | 0.884 | 0.885 | 0.896 | 0.919 | 0.884 | 0.892 | 0.885 | 0.662 | 0.655 | 0.722 | 0.882 | 0.732 | 0.659 |
Heart | 0.950 | 0.953 | 0.938 | 0.938 | 0.939 | 0.960 | 0.920 | 0.947 | 0.797 | 0.710 | 0.789 | 0.934 | 0.700 | 0.792 |
Kidney | 0.889 | 0.896 | 0.901 | 0.919 | 0.913 | 0.884 | 0.938 | 0.894 | 0.594 | 0.608 | 0.673 | 0.903 | 0.755 | 0.624 |
Limb | 0.911 | 0.916 | 0.910 | 0.910 | 0.906 | 0.920 | 0.890 | 0.918 | 0.744 | 0.704 | 0.780 | 0.898 | 0.739 | 0.742 |
Stomach | 0.935 | 0.945 | 0.912 | 0.920 | 0.920 | 0.950 | 0.893 | 0.917 | 0.836 | 0.735 | 0.812 | 0.895 | 0.689 | 0.801 |
Thymus | 0.899 | 0.909 | 0.895 | 0.902 | 0.907 | 0.900 | 0.883 | 0.899 | 0.719 | 0.770 | 0.774 | 0.882 | 0.743 | 0.724 |
Craniofacial | 0.892 | 0.903 | 0.904 | 0.897 | 0.904 | 0.895 | 0.890 | 0.903 | 0.710 | 0.712 | 0.901 | 0.888 | 0.754 | 0.715 |
Retina | 0.888 | 0.895 | 0.897 | 0.899 | 0.892 | 0.887 | 0.894 | 0.893 | 0.673 | 0.674 | 0.742 | 0.911 | 0.747 | 0.680 |
Muller retina | 0.843 | 0.855 | 0.861 | 0.875 | 0.866 | 0.824 | 0.886 | 0.855 | 0.571 | 0.627 | 0.680 | 0.860 | 0.901 | 0.613 |
Neural tube | 0.931 | 0.933 | 0.913 | 0.915 | 0.918 | 0.933 | 0.895 | 0.926 | 0.750 | 0.711 | 0.788 | 0.910 | 0.747 | 0.804 |
Training datasets | ESC | Early-Fetal | Late-Fetal | Adult |
Independent datasets | ||||
ESC | 0.921 | 0.919 | 0.908 | 0.911 |
Early-Fetal | 0.940 | 0.952 | 0.937 | 0.934 |
Late-Fetal | 0.890 | 0.891 | 0.910 | 0.903 |
Adult | 0.905 | 0.902 | 0.908 | 0.930 |
DATASETS (TISSUES) | Information entropy | |||||||||||
A | T | C | G | |||||||||
POS | NEG | ALL | POS | NEG | ALL | POS | NEG | ALL | POS | NEG | ALL | |
Forebrain | 7.782 | 7.997 | 7.922 | 7.793 | 8.001 | 7.914 | 7.782 | 7.999 | 7.909 | 7.789 | 7.997 | 7.925 |
Midbrain | 7.729 | 7.998 | 7.912 | 7.736 | 8.001 | 7.901 | 7.728 | 7.997 | 7.895 | 7.732 | 8.000 | 7.913 |
Hindbrain | 7.796 | 7.997 | 7.927 | 7.804 | 7.994 | 7.918 | 7.794 | 7.997 | 7.917 | 7.795 | 7.994 | 7.923 |
Liver | 7.775 | 8.001 | 7.918 | 7.769 | 7.998 | 7.908 | 7.758 | 7.998 | 7.904 | 7.768 | 8.000 | 7.915 |
Lung | 7.770 | 7.993 | 7.910 | 7.761 | 7.993 | 7.902 | 7.767 | 7.993 | 7.904 | 7.763 | 7.990 | 7.908 |
Heart | 7.842 | 7.992 | 7.935 | 7.837 | 7.994 | 7.924 | 7.831 | 7.992 | 7.919 | 7.844 | 7.993 | 7.936 |
Kidney | 7.732 | 7.990 | 7.895 | 7.724 | 7.994 | 7.894 | 7.725 | 7.988 | 7.890 | 7.727 | 7.988 | 7.891 |
Limb | 7.781 | 8.002 | 7.926 | 7.787 | 8.001 | 7.916 | 7.777 | 7.996 | 7.911 | 7.786 | 7.999 | 7.925 |
Stomach | 7.788 | 7.999 | 7.957 | 7.767 | 7.992 | 7.934 | 7.765 | 7.996 | 7.937 | 7.772 | 8.000 | 7.956 |
Thymus | 7.592 | 7.979 | 7.867 | 7.613 | 7.980 | 7.857 | 7.602 | 7.984 | 7.859 | 7.612 | 7.987 | 7.881 |
Craniofacial | 7.620 | 7.994 | 7.880 | 7.634 | 7.992 | 7.870 | 7.632 | 7.994 | 7.871 | 7.626 | 7.991 | 7.878 |
Retina | 7.714 | 7.984 | 7.891 | 7.705 | 7.986 | 7.882 | 7.718 | 7.986 | 7.886 | 7.717 | 7.982 | 7.891 |
Muller retina | 7.571 | 7.990 | 7.862 | 7.560 | 7.995 | 7.873 | 7.581 | 7.994 | 7.876 | 7.555 | 7.988 | 7.858 |
Neural tube | 7.770 | 8.001 | 7.908 | 7.695 | 7.997 | 7.890 | 7.694 | 7.994 | 7.888 | 7.690 | 7.994 | 7.901 |
DATASETS (STAGES) | Information Entropy | |||||||||||
A | T | C | G | |||||||||
POS | NEG | ALL | POS | NEG | ALL | POS | NEG | ALL | POS | NEG | ALL | |
ESC | 7.717 | 7.992 | 7.904 | 7.708 | 8.000 | 7.888 | 7.710 | 7.993 | 7.883 | 7.720 | 7.997 | 7.908 |
Early-Fetal | 7.830 | 7.995 | 7.934 | 7.828 | 7.998 | 7.924 | 7.821 | 7.996 | 7.920 | 7.832 | 7.996 | 7.935 |
Late-Fetal | 7.650 | 7.998 | 7.888 | 7.656 | 8.000 | 7.885 | 7.652 | 7.998 | 7.881 | 7.659 | 7.998 | 7.890 |
Adult | 7.763 | 7.992 | 7.908 | 7.754 | 7.994 | 7.900 | 7.757 | 7.993 | 7.900 | 7.755 | 7.992 | 7.905 |
DATASETS (TISSUES) | METHOD | |||
IDHS-Deep | LangMoDHS | IDHS-Deep | LangMoDHS | |
Training datasets | Independent datasets | |||
Forebrain | 0.934 | 0.938 | 0.939 | 0.939 |
Midbrain | 0.931 | 0.931 | 0.920 | 0.932 |
Hindbrain | 0.911 | 0.915 | 0.914 | 0.926 |
Liver | 0.927 | 0.932 | 0.924 | 0.935 |
Lung | 0.906 | 0.920 | 0.885 | 0.919 |
Heart | 0.955 | 0.957 | 0.949 | 0.960 |
Kidney | 0.934 | 0.934 | 0.923 | 0.938 |
Limb | 0.909 | 0.907 | 0.908 | 0.918 |
Stomach | 0.877 | 0.848 | 0.931 | 0.836 |
Thymus | 0.921 | 0.738 | 0.896 | 0.770 |
Craniofacial | 0.908 | 0.871 | 0.894 | 0.901 |
Retina | 0.902 | 0.900 | 0.894 | 0.911 |
Muller retina | 0.904 | 0.882 | 0.901 | 0.901 |
Neural tube | 0.896 | 0.763 | 0.900 | 0.804 |
DATASETS (STAGES) | METHOD | |||
IDHS-Deep | LangMoDHS | IDHS-Deep | LangMoDHS | |
Training datasets | Independent datasets | |||
ESC | 0.923 | 0.920 | 0.899 | 0.921 |
Early-Fetal | 0.949 | 0.950 | 0.940 | 0.952 |
Late-Fetal | 0.923 | 0.907 | 0.901 | 0.910 |
Adult | 0.916 | 0.925 | 0.905 | 0.930 |
Training datasets | Forebr-ain | Midbr-ain | Hindb-rain | Liver | Lung | Heart | Kidn-ey | Limb | Stom-ach | Thym-us | Cranio-facial | Retina | Muller retina | Neural tube |
Independent datasets | ||||||||||||||
Forebrain | 0.939 | 0.939 | 0.922 | 0.923 | 0.926 | 0.944 | 0.904 | 0.930 | 0.789 | 0.721 | 0.796 | 0.914 | 0.708 | 0.791 |
Midbrain | 0.923 | 0.932 | 0.918 | 0.917 | 0.918 | 0.929 | 0.901 | 0.922 | 0.759 | 0.725 | 0.786 | 0.908 | 0.730 | 0.764 |
Hindbrain | 0.913 | 0.918 | 0.926 | 0.910 | 0.910 | 0.919 | 0.895 | 0.914 | 0.723 | 0.698 | 0.765 | 0.908 | 0.734 | 0.732 |
Liver | 0.901 | 0.907 | 0.907 | 0.935 | 0.924 | 0.907 | 0.922 | 0.910 | 0.671 | 0.658 | 0.728 | 0.901 | 0.747 | 0.672 |
Lung | 0.885 | 0.884 | 0.885 | 0.896 | 0.919 | 0.884 | 0.892 | 0.885 | 0.662 | 0.655 | 0.722 | 0.882 | 0.732 | 0.659 |
Heart | 0.950 | 0.953 | 0.938 | 0.938 | 0.939 | 0.960 | 0.920 | 0.947 | 0.797 | 0.710 | 0.789 | 0.934 | 0.700 | 0.792 |
Kidney | 0.889 | 0.896 | 0.901 | 0.919 | 0.913 | 0.884 | 0.938 | 0.894 | 0.594 | 0.608 | 0.673 | 0.903 | 0.755 | 0.624 |
Limb | 0.911 | 0.916 | 0.910 | 0.910 | 0.906 | 0.920 | 0.890 | 0.918 | 0.744 | 0.704 | 0.780 | 0.898 | 0.739 | 0.742 |
Stomach | 0.935 | 0.945 | 0.912 | 0.920 | 0.920 | 0.950 | 0.893 | 0.917 | 0.836 | 0.735 | 0.812 | 0.895 | 0.689 | 0.801 |
Thymus | 0.899 | 0.909 | 0.895 | 0.902 | 0.907 | 0.900 | 0.883 | 0.899 | 0.719 | 0.770 | 0.774 | 0.882 | 0.743 | 0.724 |
Craniofacial | 0.892 | 0.903 | 0.904 | 0.897 | 0.904 | 0.895 | 0.890 | 0.903 | 0.710 | 0.712 | 0.901 | 0.888 | 0.754 | 0.715 |
Retina | 0.888 | 0.895 | 0.897 | 0.899 | 0.892 | 0.887 | 0.894 | 0.893 | 0.673 | 0.674 | 0.742 | 0.911 | 0.747 | 0.680 |
Muller retina | 0.843 | 0.855 | 0.861 | 0.875 | 0.866 | 0.824 | 0.886 | 0.855 | 0.571 | 0.627 | 0.680 | 0.860 | 0.901 | 0.613 |
Neural tube | 0.931 | 0.933 | 0.913 | 0.915 | 0.918 | 0.933 | 0.895 | 0.926 | 0.750 | 0.711 | 0.788 | 0.910 | 0.747 | 0.804 |
Training datasets | ESC | Early-Fetal | Late-Fetal | Adult |
Independent datasets | ||||
ESC | 0.921 | 0.919 | 0.908 | 0.911 |
Early-Fetal | 0.940 | 0.952 | 0.937 | 0.934 |
Late-Fetal | 0.890 | 0.891 | 0.910 | 0.903 |
Adult | 0.905 | 0.902 | 0.908 | 0.930 |
DATASETS (TISSUES) | Information entropy | |||||||||||
A | T | C | G | |||||||||
POS | NEG | ALL | POS | NEG | ALL | POS | NEG | ALL | POS | NEG | ALL | |
Forebrain | 7.782 | 7.997 | 7.922 | 7.793 | 8.001 | 7.914 | 7.782 | 7.999 | 7.909 | 7.789 | 7.997 | 7.925 |
Midbrain | 7.729 | 7.998 | 7.912 | 7.736 | 8.001 | 7.901 | 7.728 | 7.997 | 7.895 | 7.732 | 8.000 | 7.913 |
Hindbrain | 7.796 | 7.997 | 7.927 | 7.804 | 7.994 | 7.918 | 7.794 | 7.997 | 7.917 | 7.795 | 7.994 | 7.923 |
Liver | 7.775 | 8.001 | 7.918 | 7.769 | 7.998 | 7.908 | 7.758 | 7.998 | 7.904 | 7.768 | 8.000 | 7.915 |
Lung | 7.770 | 7.993 | 7.910 | 7.761 | 7.993 | 7.902 | 7.767 | 7.993 | 7.904 | 7.763 | 7.990 | 7.908 |
Heart | 7.842 | 7.992 | 7.935 | 7.837 | 7.994 | 7.924 | 7.831 | 7.992 | 7.919 | 7.844 | 7.993 | 7.936 |
Kidney | 7.732 | 7.990 | 7.895 | 7.724 | 7.994 | 7.894 | 7.725 | 7.988 | 7.890 | 7.727 | 7.988 | 7.891 |
Limb | 7.781 | 8.002 | 7.926 | 7.787 | 8.001 | 7.916 | 7.777 | 7.996 | 7.911 | 7.786 | 7.999 | 7.925 |
Stomach | 7.788 | 7.999 | 7.957 | 7.767 | 7.992 | 7.934 | 7.765 | 7.996 | 7.937 | 7.772 | 8.000 | 7.956 |
Thymus | 7.592 | 7.979 | 7.867 | 7.613 | 7.980 | 7.857 | 7.602 | 7.984 | 7.859 | 7.612 | 7.987 | 7.881 |
Craniofacial | 7.620 | 7.994 | 7.880 | 7.634 | 7.992 | 7.870 | 7.632 | 7.994 | 7.871 | 7.626 | 7.991 | 7.878 |
Retina | 7.714 | 7.984 | 7.891 | 7.705 | 7.986 | 7.882 | 7.718 | 7.986 | 7.886 | 7.717 | 7.982 | 7.891 |
Muller retina | 7.571 | 7.990 | 7.862 | 7.560 | 7.995 | 7.873 | 7.581 | 7.994 | 7.876 | 7.555 | 7.988 | 7.858 |
Neural tube | 7.770 | 8.001 | 7.908 | 7.695 | 7.997 | 7.890 | 7.694 | 7.994 | 7.888 | 7.690 | 7.994 | 7.901 |
DATASETS (STAGES) | Information Entropy | |||||||||||
A | T | C | G | |||||||||
POS | NEG | ALL | POS | NEG | ALL | POS | NEG | ALL | POS | NEG | ALL | |
ESC | 7.717 | 7.992 | 7.904 | 7.708 | 8.000 | 7.888 | 7.710 | 7.993 | 7.883 | 7.720 | 7.997 | 7.908 |
Early-Fetal | 7.830 | 7.995 | 7.934 | 7.828 | 7.998 | 7.924 | 7.821 | 7.996 | 7.920 | 7.832 | 7.996 | 7.935 |
Late-Fetal | 7.650 | 7.998 | 7.888 | 7.656 | 8.000 | 7.885 | 7.652 | 7.998 | 7.881 | 7.659 | 7.998 | 7.890 |
Adult | 7.763 | 7.992 | 7.908 | 7.754 | 7.994 | 7.900 | 7.757 | 7.993 | 7.900 | 7.755 | 7.992 | 7.905 |