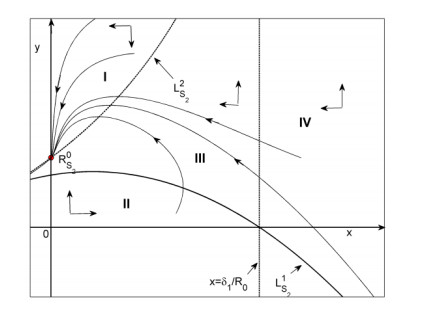
Citation: Vincent Carré, Sébastien Schramm, Frédéric Aubriet. Study of a complex environmental mixture by electrospray ionization and laser desorption ionization high resolution mass spectrometry: the cigarette smoke aerosol[J]. AIMS Environmental Science, 2015, 2(3): 547-564. doi: 10.3934/environsci.2015.3.547
[1] | Baolin Kang, Xiang Hou, Bing Liu . Threshold control strategy for a Filippov model with group defense of pests and a constant-rate release of natural enemies. Mathematical Biosciences and Engineering, 2023, 20(7): 12076-12092. doi: 10.3934/mbe.2023537 |
[2] | Yi Yang, Lirong Liu, Changcheng Xiang, Wenjie Qin . Switching dynamics analysis of forest-pest model describing effects of external periodic disturbance. Mathematical Biosciences and Engineering, 2020, 17(4): 4328-4347. doi: 10.3934/mbe.2020239 |
[3] | Yuan Tian, Sanyi Tang . Dynamics of a density-dependent predator-prey biological system with nonlinear impulsive control. Mathematical Biosciences and Engineering, 2021, 18(6): 7318-7343. doi: 10.3934/mbe.2021362 |
[4] | Bing Liu, Gang Hu, Baolin Kang, Xin Huang . Analysis of a hybrid pest management model incorporating pest resistance and different control strategies. Mathematical Biosciences and Engineering, 2020, 17(5): 4364-4383. doi: 10.3934/mbe.2020241 |
[5] | Liping Wu, Zhongyi Xiang . A study of integrated pest management models with instantaneous and non-instantaneous impulse effects. Mathematical Biosciences and Engineering, 2024, 21(2): 3063-3094. doi: 10.3934/mbe.2024136 |
[6] | Bruno Buonomo , Francesco Giannino , Stéphanie Saussure , Ezio Venturino . Effects of limited volatiles release by plants in tritrophic interactions. Mathematical Biosciences and Engineering, 2019, 16(5): 3331-3344. doi: 10.3934/mbe.2019166 |
[7] | Wenjie Qin, Yue Xia, Yi Yang . An eco-epidemic model for assessing the application of integrated pest management strategies. Mathematical Biosciences and Engineering, 2023, 20(9): 16506-16527. doi: 10.3934/mbe.2023736 |
[8] | Luis F. Gordillo . Optimal sterile insect release for area-wide integrated pest management in a density regulated pest population. Mathematical Biosciences and Engineering, 2014, 11(3): 511-521. doi: 10.3934/mbe.2014.11.511 |
[9] | Aili Wang, Yanni Xiao, Huaiping Zhu . Dynamics of a Filippov epidemic model with limited hospital beds. Mathematical Biosciences and Engineering, 2018, 15(3): 739-764. doi: 10.3934/mbe.2018033 |
[10] | Guodong Li, Wenjie Li, Ying Zhang, Yajuan Guan . Sliding dynamics and bifurcations of a human influenza system under logistic source and broken line control strategy. Mathematical Biosciences and Engineering, 2023, 20(4): 6800-6837. doi: 10.3934/mbe.2023293 |
Various modelling and analytical techniques have been widely employed to address the effectiveness of integrated pest management (IPM), which is a threshold control strategy [1,2,3] and it can be defined as follows: if the number of pests is below the economic threshold (ET), then the integrated control strategy is not applied at all; above the ET then the integrated control measures including biological control and chemical control are applied with the aim of maintaining the number of pests below the economic injury level (EIL). In order to reveal the effectiveness of IPM, various mathematical models have been developed and studied based on the action mechanism of pesticides and the implementation of control strategies [4,5,6,7,8,9,10]. In particular, Filippov systems which are widely employed to depict intermittent control strategies and to describe many practical problems [7,11,12,13,14,15,16,17,18], have been received much attention and investigated [7,16,17,18]. However, most of the previous models strategy were designed to simplify the dynamic behavior of two subsystems without considering the constant releasing rate commonly used in IPM [17,18]. In practical biological control methods, a constant releasing strategy is often used to facilitate operation and avoid monitoring the number of natural enemies, which has been proved to play an important role in the pest control [19,20,21,22].
In the present paper, a planar Filippov system formulated by two subsystems is proposed to reveal the effect of the constant releasing rate of natural enemy on the pest control, in which the discontinuity boundary or switching line is determined by the ET [23,24,25,26,27]. Therefore, the IPM strategy including spraying pesticide which the killing rate assumes to be proportional to the number of pests and releasing a constant number of natural enemies is applied once the number of the pest population exceeds the ET, otherwise the pest-natural enemy system is free from any control effects.
Based on the above facts, the pest-natural enemy interaction system without control measurements can be modelled by the classical Holling-Ⅱ predator-prey model [28,29] as follows:
$ {dx(t)dt=rx−rKx2−βxy1+ωx,dy(t)dt=ηβxy1+ωx−δy, $ | (1.1) |
where $ x(t) $ and $ y(t) $ represent the number of prey (pest) and predator (natural enemy), $ r $ is the intrinsic growth rate of the pest population and $ K $ represents its carrying capacity, $ \dfrac{\beta x}{1+\omega x} $ denotes the Holling-Ⅱ functional response function, which is a saturating function of the numbers of pests present, $ \delta $ denotes the death rate of the natural enemy population, and $ \eta $ ($ \eta\in (0, 1] $) denotes the conversion rate of the pest. The dynamics of this subsystem (also called an untreated subsystem) is well known and has been well studied [28], and will be summarized in the coming section.
The other subsystem (also called a treated subsystem) must be employed to model the artificial control strategies (spraying pesticides and releasing natural enemies), which can be represented as follows:
$ {dx(t)dt=rx−rKx2−βxy1+ωx−αx,dy(t)dt=ηβxy1+ωx−δ1y+τ, $ | (1.2) |
where $ \tau $ represents the releasing constant of natural enemies, $ \alpha $ denotes the pest killing rate due to the spraying of pesticides, and $ \delta_{1}\geq \delta $ implies that the pesticide has side effects on the natural enemy. From a mathematical point of view, the essential difference between model (1.1) and model (1.2) is the constant release rate $ \tau $, which will result in rich dynamical behaviors and will be investigated in more detail in Section 3.
Combining the above two subsystems, we get the following non-smooth Filippov pest-natural enemy system with constant releasing rate as follows:
$ {dx(t)dt=rx−rKx2−βxy1+ωx−ϵαx,dy(t)dt=ηβxy1+ωx−(δ+ϵh)y+ϵτ $ | (1.3) |
with
$ {ϵ=0,x(t)<ET,ϵ=1,x(t)>ET, $ |
where for convenience we denote $ \delta_{1} = \delta+h $ with $ h\geq 0 $. Note that if $ \tau = 0 $, then two subsystems (i.e., models (1.1) and (1.2) of Filippov system (1.3) have exactly the same dynamics, which have been widely used and investigated [24].
The objective of this paper is to systematically study the dynamical properties of non-smooth Filippov pest-natural enemy system with constant releasing rate and discuss bifurcations of model (1.3) depending on important parameters. This paper is organized as follows. In Section 2, we introduce the main definitions and terminologies of the generic second-order Filippov system. In Section 3, we systematically study the dynamical behavior of system (1.2) (i.e., subsystem $ S_{2} $) and address how the constant releasing strategy affects the qualitative behaviors, which is crucial for analyzing Filippov system (1.3). The results show that system (1.2) exists rich dynamical behaviors including transcritical, saddle-node, Hopf and Bogdanov-Takens bifurcations [30,31]. In Section 4, we study the existence and stability of the regular/virtual equilibria, pseudo-equilibria, sliding segments and sliding bifurcations of system (1.3) by employing the methods for non-smooth dynamical systems including the Filippov convex method and sliding bifurcation techniques [23,26,27,32]. Particular attention is paid to the effect of the threshold level ET and releasing constant $ \tau $ on the dynamical behavior of Filippov system (1.3) and successful pest control. The paper ends with brief conclusions and discussions.
The generic second-order (i.e., $ X(t)\in R^{2}_{+} $) Filippov system can be defined as follows [12,17,23]:
$ ˙X(t)={FS1(X),X∈S1,FS2(X),X∈S2, $ | (2.1) |
where $ X(t) = \{x(t), y(t)\}^{T} $ and $ {R^{2}_{+}} = \{(x, y)|x \geq 0, y \geq 0\} $, and the regions $ S_{1} $ and $ S_{2} $ are separated by the discontinuity boundary $ \Sigma $ described by $ H(X) = 0 $, where $ H(X) $ is a smooth scalar function with non-vanishing gradient $ H_{X}(X) $ on $ \Sigma $. For convenience, we call Filippov system (2.1) defined in region $ S_{1} $ as subsystem $ S_{1} $ and defined in region $ S_{2} $ as subsystem $ S_{2} $. Denote
$ \sigma(X) = \langle H_{X}(X), F_{S_{1}}(X)\rangle \cdot \langle H_{X}(X), F_{S_{2}}(X)\rangle, $ |
where $ \langle, \rangle $ is a Cartesian product in $ R^{2} $ and $ H_{X}(X) $ pointing to the region $ S_{2} $ is called the positive direction. Based on the above concepts, we provide the necessary definitions of Filippov system (2.1) as follows:
Definition 1. If all these points $ (x, y)\in \Sigma $ satisfy the condition $ \sigma(X)\leq 0 $, then we say that $ (x, y) $ belongs to the sliding segment, denoted by $ \Sigma_{s} $. While the complement to $ \Sigma_{s} $ in $ \Sigma $ is called a crossing set, denoted by $ \Sigma_{c} $. For the stability of the sliding segment, if
$ \langle H_{X}(X), F_{S_{1}}(X)\rangle \gt 0, \, \, \langle H_{X}(X), F_{S_{2}}(X)\rangle \lt 0, $ |
then the sliding segment is stable. If
$ \langle H_{X}(X), F_{S_{1}}(X)\rangle \lt 0, \, \, \langle H_{X}(X), F_{S_{2}}(X)\rangle \gt 0, $ |
then it is unstable.
Definition 2. If the point $ R(x^{*}, y^{*}) $ of Filippov system (2.1) satisfies the following conditions
$ F_{S_{1}}(x^{*}, y^{*}) = 0\ \mathrm{for}\ (x^{*}, y^{*})\in S_{1}\quad \mathrm{or} \quad F_{S_{2}}(x^{*}, y^{*}) = 0 \ \mathrm{for}\ (x^{*}, y^{*})\in S_{2}, $ |
then it is called a regular equilibrium of Filippov system (2.1). Similarly, if it satisfies
$ F_{S_{1}}(x^{*}, y^{*}) = 0\ \mathrm{for}\ (x^{*}, y^{*})\in S_{2}\quad \mathrm{or} \quad F_{S_{2}}(x^{*}, y^{*}) = 0\ \mathrm{for}\ (x^{*}, y^{*})\in S_{1}, $ |
then it is called a virtual equilibrium of Filippov system (2.1).
Definition 3. If the point $ T(x^{*}, y^{*}) $ of Filippov system (2.1) satisfies
$ \langle H_{X}(x^{*}, y^{*}), F_{S_{1}}(x^{*}, y^{*})\rangle = 0\ \mathrm{for}\ (x^{*}, y^{*})\in \Sigma_{s}\quad \mathrm{or} \quad \langle H_{X}(x^{*}, y^{*}), F_{S_{2}}(x^{*}, y^{*})\rangle = 0\ \mathrm{for}\ (x^{*}, y^{*})\in \Sigma_{s}, $ |
then it is called a tangent point of Filippov system (2.1).
Definition 4. If the point $ P(x^{*}, y^{*}) $ of Filippov system (2.1) satisfies
$ F_{S_{1}}(x^{*}, y^{*}) = 0\ \mathrm{for}\ H(x^{*}, y^{*}) = 0\quad \mathrm{or} \quad F_{S_{2}}(x^{*}, y^{*}) = 0\ \mathrm{for}\ H(x^{*}, y^{*}) = 0, $ |
then it is called a boundary equilibrium of Filippov system (2.1).
Note that the three points $ P(x^{*}, y^{*}) $, $ T(x^{*}, y^{*}) $ and $ R(x^{*}, y^{*}) $ may collide together for some critical cases, and for more definitions and properties of Filippov system (2.1) please see [12,17,23]. Now let us turn to non-smooth Filippov system (1.3), which can be represented as:
$ ˙X(t)={f1(x,y),(x,y)∈S1,f2(x,y),(x,y)∈S2, $ | (2.2) |
where the discontinuity boundary is $ \Sigma = \{(x, y)\in {R^{2}_{+}}|H(x, y) = 0\} $ and $ H(x, y) = x-ET $, which separates the two regions $ S_{1} = \{(x, y) \in {R^{2}_{+}}|H(x, y) < 0\} $ and $ S_{2} = \{(x, y) \in {R^{2}_{+}}|H(x, y) > 0\} $. The vector field for subsystem $ S_{1} $ is
$ f_{1}(x, y) = \left\{r x-\frac{r}{K} x^{2}-\frac{\beta x y}{1+\omega x}, \quad \frac{\eta\beta x y}{1+\omega x}-\delta y\right\}^{T} $ |
and the vector field for subsystem $ S_{2} $ is
$ f_{2}(x, y) = \left\{r x-\frac{r}{K} x^{2}-\frac{\beta x y}{1+\omega x}-\alpha x, \quad \frac{\eta\beta x y}{1+\omega x}-\delta_{1} y+\tau\right\}^{T}. $ |
The dynamics of two subsystems $ S_{1} $ and $ S_{2} $ are crucial for analyzing the global dynamics of Filippov system (2.2), and the complete dynamical behavior of subsystem $ S_{1} $ has been discussed in more detail in the literature [28]. That is to say, subsystem $ S_{1} $ always exists with a trivial equilibrium $ R^{0}_{S_{1}}(0, 0) $ which is unstable, and a boundary equilibrium $ R^{1}_{S_{1}}(K, 0) $ which is globally stable if $ \frac{\delta}{\eta \beta-\delta \omega}\geq K $ or $ \frac{\delta}{\eta \beta-\delta \omega} < 0 $holds true. Meanwhile, subsystem $ S_{1} $ exists with a positive equilibrium $ R^{2}_{S_{1}}\left(\frac{\delta}{\eta \beta-\delta \omega}, \frac{r\eta(K\eta \beta-K \delta \omega-\delta)}{K(\eta \beta-\delta \omega)^{2}}\right) $ if and only if $ 0 < \frac{\delta}{\eta \beta-\delta \omega} < K $, which is a globally stable node or focus provided that $ \omega K \leq \frac{\eta \beta+\delta \omega}{\eta \beta-\delta \omega} $. Otherwise, it is an unstable node or focus and there exists a unique and stable limit cycle.
However, the dynamical behavior of subsystem $ S_2 $ has not been addressed so far because the constant releasing rate will bring some challenges for qualitative analysis. Although a similar model was investigated in reference [31], we realize that the sign of the constant $ \tau $ (minus $ \tau $ was used in [31]) could result in different dynamics including the existence and stability of equilibria and various bifurcations. Thus, we first carry out qualitative analyses for subsystem $ S_2 $ in the following.
In this section, we will investigate the dynamical behavior of subsystem $ S_{2} $ and focus on the effect of parameter $ \tau $ on the dynamics, i.e., the effect of the control strategy (releasing natural enemies) in the pest control. For convenience, we rewrite the subsystem $ S_{2} $ as follows:
$ {dx(t)dt=rx−rKx2−βxy1+ωx−αx≐P1(x,y),dy(t)dt=ηβxy1+ωx−δ1y+τ≐Q1(x,y), $ | (3.1) |
here we assume that $ r > \alpha $.
Firstly, let us begin to determine the location and number of the equilibria of system (3.1) in $ R^{2}_{+} $. It is easy to check that the point $ R^{0}_{S_{2}}(0, \frac{\tau}{\delta_{1}}) $ is always the boundary equilibrium of system (3.1). In the following, we mainly focus on the existence of the positive equilibria of system (3.1).
It is clear that equations
$ {P1(x,y)=0,Q1(x,y)=0, $ | (3.2) |
have at most two positive real solutions:
$ x_{1}\doteq\frac{KR_{2}-K\sqrt{\Delta}}{2rR_{0}}, \ y_{1}\doteq(r_{1}-\frac{r}{K}x_{1})\frac{1+\omega x_{1}}{\beta} $ |
and
$ x_{2}\doteq\frac{KR_{2}+K\sqrt{\Delta}}{2rR_{0}}, \ y_{2}\doteq(r_{1}-\frac{r}{K}x_{2})\frac{1+\omega x_{2}}{\beta}, $ |
where
$ r_{1}\doteq r-\alpha, \ \ R_{0} \doteq \eta \beta-\delta_{1} \omega, \ \ R_{1} \doteq \beta \tau-r_{1} \delta_{1}, \ \ R_{2}\doteq r_{1}(\eta \beta-\delta_{1} \omega)+\frac{r \delta_{1}}{K} \ \ \mathrm{and} \ \ \Delta \doteq R^{2}_{2}+\frac{4rR_{0}R_{1}}{K}. $ |
That is to say, system (3.1) has at most two positive equilibria in $ R^{2}_{+} $, denoted by $ R^{1}_{S_{2}}(x_{1}, y_{1}) $ and $ R^{2}_{S_{2}}(x_{2}, y_{2}) $. And we have the following simple theorem which describes the number and location of equilibria of system (3.1). The proof is omitted.
Lemma 3.1. For system (3.1), with $ R_{0} $, $ R_{1} $, $ R_{2} $ and $ \Delta $ defined as above, we have:
(ⅰ) when $ R_{0} > 0 $, system (3.1) has at most two equilibria in $ R^{2}_{+} $ and it has the following possibilities:
(a) if $ R_{1} > 0 $ holds, then system (3.1) has a unique equilibrium $ R^{0}_{S_{2}} $;
(b) if $ R_{1} < 0 $ holds, then system (3.1) has two equilibria $ R^{0}_{S_{2}} $ and $ R^{1}_{S_{2}} $;
(c) if $ R_{1} = 0 $ holds, then $ R^{0}_{S_{2}} $ and $ R^{1}_{S_{2}} $ coalesce at a boundary equilibrium of multiplicity 2.
(ⅱ) when $ R_{0} < 0 $, system (3.1) has at most three equilibria in $ R^{2}_{+} $ and it has the following possibilities:
(a) if $ R_{1} > 0 $, $ R_{2} < 0 $ and $ \Delta > 0 $ hold, then system (3.1) has three equilibria $ R^{0}_{S_{2}} $, $ R^{1}_{S_{2}} $ and $ R^{2}_{S_{2}} $;
(b) if $ R_{1} > 0 $, $ R_{2} < 0 $ and $ \Delta = 0 $ hold, then $ R^{1}_{S_{2}} $ and $ R^{2}_{S_{2}} $ coalesce at a positive equilibrium of multiplicity 2, which coexists with $ R^{0}_{S_{2}} $;
(c) if $ R_{1} > 0 $, $ R_{2} < 0 $ and $ \Delta < 0 $ hold, then system (3.1) has a unique equilibrium $ R^{0}_{S_{2}} $;
(d) if $ R_{1} < 0 $ holds, then system (3.1) has two equilibria $ R^{0}_{S_{2}} $ and $ R^{1}_{S_{2}} $;
(e) if $ R_{1} = 0 $ and $ R_{2} < 0 $ hold, then $ R^{0}_{S_{2}} $ and $ R^{2}_{S_{2}} $ coalesce at a boundary equilibrium of multiplicity 2, which coexists with $ R^{1}_{S_{2}} $;
(f) if $ R_{1} = 0 $ and $ R_{2} > 0 $ hold, then $ R^{0}_{S_{2}} $ and $ R^{1}_{S_{2}} $ coalesce at a boundary equilibrium of multiplicity 2, and $ R^{2}_{S_{2}} $ disappears in this case;
(g) if $ R_{1} = 0 $ and $ R_{2} = 0 $ hold, then $ R^{0}_{S_{2}} $, $ R^{1}_{S_{2}} $ and $ R^{2}_{S_{2}} $ coalesce at a boundary equilibrium of multiplicity 3.
Next we discuss the possible phase portraits of system (3.1) and analyze the stability of equilibria according to Lemma 3.1.
In this case, system (3.1) has at most two equilibria $ R^{1}_{S_{2}}(x_{1}, y_{1}) $ and $ R^{0}_{S_{2}}(0, \frac{\tau}{\delta_{1}}) $ in $ R^{2}_{+} $, and we can get the following results.
Theorem 3.1. If $ R_{0} > 0 $ and $ R_{1} > 0 $, then system (3.1) exists with a boundary equilibrium $ R^{0}_{S_{2}} $ in $ R^{2}_{+} $, which is a globally asymptotically stable node.
Proof. In this case, system (3.1) only has a boundary equilibrium $ R^{0}_{S_{2}}(0, \frac{\tau}{\delta_{1}}) $ in $ R^{2}_{+} $. And the Jacobian matrix at $ R^{0}_{S_{2}} $ is given by
$ \textbf{A}_{R^{0}_{S_{2}}} = (r1−2rxK−βy(1+ωx)2−βx1+ωxηβy(1+ωx)2ηβx1+ωx−δ1)\Bigg|_{R^{0}_{S_{2}}} = (r1−βτδ10ηβτδ1−δ1). $ |
The corresponding characteristic equation is
$ |\textbf{A}_{R^{0}_{S_{2}}}-\lambda \textbf{E}| = \lambda^{2}+p_{R^{0}_{S_{2}}}\lambda +q_{R^{0}_{S_{2}}} = 0, $ |
where $ q_{R^{0}_{S_{2}}}\doteq \beta \tau-r_{1} \delta_{1} = R_{1} > 0, \, \, p_{R^{0}_{S_{2}}} \doteq \delta_{1}+\frac{\beta \tau}{\delta_{1}}-r_{1} > 0 $ and $ p^{2}_{R^{0}_{S_{2}}}-4q_{R^{0}_{S_{2}}} = (\delta_{1}-\frac{\beta \tau}{\delta_{1}}+r_{1})^{2} > 0 $. This means that $ R^{0}_{S_{2}} $ is a locally asymptotically stable node. Especially, when $ \tau = 0 $, we have $ q_{R^{0}_{S_{2}}} = -r_{1} \delta_{1} < 0 $ which indicates that $ R^{0}_{S_{2}} $ is an unstable saddle.
Now we begin to discuss the global stability of $ R^{0}_{S_{2}} $. In the first quadrant, the two non-zero isoclines $ L^{1}_{S_{2}} $ and $ L^{2}_{S_{2}} $ of system (3.1) divide $ {R^{2}_{+}} $ into four regions:
$ I = \{(x, y)|P_1(x, y) \lt 0, \, Q_1(x, y) \lt 0\};\ II = \{(x, y)|P_1(x, y) \gt 0, \, Q_1(x, y) \gt 0\}; $ |
$ III = \left\{(x, y)|P_1(x, y) \lt 0, \, Q_1(x, y) \gt 0, \, x \leq \frac{\delta_{1}}{R_{0}}\right\}; $ |
$ IV = \left\{(x, y)|P_1(x, y) \lt 0, \, Q_1(x, y) \gt 0, \, x \gt \frac{\delta_{1}}{R_{0}}\right\}, $ |
at which the signs of two functions $ P_{1}(x, y) $ and $ Q_{1}(x, y) $ are clear.
It is known that system (3.1) does not exist with any other equilibria in $ {R^{2}_{+}} $ except $ R^{0}_{S_{2}} $. From Figure 1, we can see that the trajectory $ \{x(t, x_{0}, y_{0}), y(t, x_{0}, y_{0})\} $ of system (3.1) will approach the boundary equilibrium $ R^{0}_{S_{2}} $ directly, or intersects with the non-zero isoclines $ L^{1}_{S_{2}} $ or $ L^{2}_{S_{2}} $ firstly, and then tends to the boundary equilibrium $ R^{0}_{S_{2}} $ as $ t\rightarrow+\infty $ for any initial value $ (x_{0}, y_{0})\in I\bigcup II\bigcup III $.
By a simple calculation, we have
$ \left|\frac{dy}{dx}\right| = \left|\frac{Q_{1}(x, y)}{P_{1}(x, y)}\right| = \left|\frac{\eta \beta-\delta_{1} \omega}{\beta}\right|\cdot\left|\frac{1-\frac{\frac{\delta_{1}}{\eta \beta-\delta_{1} \omega}}{x}+\frac{\frac{\delta_{1}}{\eta \beta-\delta_{1} \omega}(\frac{\tau}{\beta}+\frac{\tau \omega}{\beta}x)}{xy}}{1+\frac{\frac{r \omega}{\beta K}x^{2}-\frac{r_{1} \omega-\frac{r}{K}}{\beta}-\frac{r_{1}}{\beta}}{xy}}\right| \lt \frac{R_{0}}{\beta} $ |
for all $ (x, y)\in IV $ and $ y\gg1 $. That is to say, the trajectories of system (3.1) can not always remain to the right of the line $ x = \frac{\delta_{1}}{R_{0}} $, which indicates that the trajectories starting from the region $ IV $ must cross the line $ x = \frac{\delta_{1}}{R_{0}} $ and enter into the region $ III $, and then approach the boundary equilibrium $ R^{0}_{S_{2}} $ as $ t\rightarrow+\infty $. Thus, the boundary equilibrium $ R^{0}_{S_{2}} $ is a globally stable node. This completes the proof.
Theorem 3.2. If $ R_{0} > 0 $ and $ R_{1} = 0 $, then system (3.1) does not exist with a positive equilibrium, and the boundary equilibrium $ R^{0}_{S_{2}} $ is a saddle-node of codimension 1.
Proof. Note that if $ R_{1} = 0 $, then the boundary equilibrium $ R^{0}_{S_{2}} $ collides with the positive equilibrium $ R^{1}_{S_{2}} $ in $ R^{2}_{+} $, which is a high order singular point. Translating the boundary equilibrium $ R^{0}_{S_{2}}(0, \frac{\tau}{\delta_{1}}) $ to the origin by making the change of variables $ u = x $ and $ v = y-\frac{\tau}{\delta_{1}} $, renaming $ (u, v) $ as $ (x, y) $ and expanding the right-hand side of system (3.1) in a Taylor series about the origin, then we can obtain
$ {dxdt=(r1ω−rK)x2−βxy−r1ω2x3+βωx2y+M1(x,y),dydt=r1ηx−δ1y−r1ηωx2+ηβxy+r1ηω2x3−ηβωx2y+M2(x,y), $ | (3.3) |
where $ M_{1}(x, y) $ and $ M_{2}(x, y) $ are $ C^{\infty} $ functions in $ (x, y) $ at least of the fourth order.
Notice that $ \delta_{1} > 0 $, making the following change of variables
$ u = x, \ v = r_{1} \eta x - \delta_{1} y $ |
and renaming $ (u, v) $ as $ (x, y) $, then system (3.3) becomes
$ {dxdt=−R2δ1x2+βδ1xy+r1ωR0δ1x3−βωδ1x2y+M3(x,y)≐P2(x,y),dydt=−δ1y−r1ηδ1(R2+δ1R0)x2+(1+r1δ1)ηβxy+r1ηωR0(1+r1δ1)x3−ηβω(1+r1δ1)x2y+M4(x,y)≐Q2(x,y)−δ1y, $ | (3.4) |
where $ R_{2} > 0 $, and $ M_{3}(x, y) $, $ M_{4}(x, y) $ are $ C^{\infty} $ functions in $ (x, y) $ at least of the fourth order. Solving the equation $ -\delta_{1} y+Q_{2}(x, y) = 0 $, we can obtain the implicit solution
$ ϕ(x)≐−r1ηδ21(R2+δ1R0)x2+O(x3). $ | (3.5) |
where $ R_{2}+\delta_{1} R_{0} > 0 $. And substituting $ \phi(x) $ into $ P_{2}(x, y) $, which can be written in the form
$ ψ(x)≐P2(x,ϕ(x))=−R2δ1x2+O(x3). $ | (3.6) |
According to Theorems 7.1–7.3 in [30], it is easy to obtain that $ R^{0}_{S_{2}} $ is a saddle-node of codimension 1 if $ R_{0} > 0 $ and $ R_{1} = 0 $. The proof is completed.
In the following, we discuss the stability of the positive equilibria of system (3.1). For convenience, we first denote
$ R_{3}\doteq \frac{K(\eta \beta-\delta_{1} \omega-\frac{r}{K}+r_{1} \omega)x_{1}}{K\delta_{1}+2r\omega x^{2}_{1}}\ \ \ \mathrm{and} \ \ \ R_{4}\doteq \frac{(\eta \beta-\delta_{1} \omega-\frac{r}{K}+r_{1} \omega)\sqrt{2r \delta_{1} \omega K}}{4r \delta_{1} \omega}. $ |
Moreover, we have the following main results.
Theorem 3.3. If $ R_{0} > 0 $ and $ R_{1} < 0 $, then system (3.1) has an unstable boundary equilibrium $ R^{0}_{S_{2}} $ and a positive equilibrium $ R^{1}_{S_{2}} $ in $ R^{2}_{+} $. Further, if $ R_{3} < 1 $, then $ R^{1}_{S_{2}} $ is a locally stable node (or a focus), and it is globally stable provided that $ R_{4} < 1 $.
Proof. For the boundary equilibrium $ R^{0}_{S_{2}}(0, \frac{\tau}{\delta_{1}}) $, we have
$ |\textbf{A}_{R^{0}_{S_{2}}}-\lambda \textbf{E}| = \lambda^{2}+p_{R^{0}_{S_{2}}}\lambda +q_{R^{0}_{S_{2}}} = 0 $ |
with $ q_{R^{0}_{S_{2}}} = \beta \tau-r_{1} \delta_{1} = R_{1} < 0 $, which indicates that $ R^{0}_{S_{2}} $ is an unstable saddle.
For the positive equilibrium $ R^{1}_{S_{2}}(x_{1}, y_{1}) $, the characteristic equation is as follows:
$ |AR1S2−λE|=λ2+pR1S2λ+qR1S2=0, $ | (3.7) |
where
$ pR1S2=11+ωx1[2rωKx21−(ηβ−δ1ω−rK+r1ω)x1+δ1] $ | (3.8) |
and
$ qR1S2=2rR0x1K(1+ωx1)[KR22rR0−x1]. $ | (3.9) |
Obviously, $ q_{R^{1}_{S_{2}}} > 0 $. It follows from (3.8) that if
$ \eta \beta-\delta_{1} \omega -\frac{r}{K}+r_{1} \omega \lt \frac{K \delta_{1}+2r\omega x^{2}_{1}}{K x_{1}} $ |
holds true, which is equivalent to the following inequality
$ \frac{K(\eta \beta-\delta_{1} \omega-\frac{r}{K}+r_{1} \omega)x_{1}}{K \delta_{1} +2r\omega x^{2}_{1}} \lt 1, \ \mathrm{i.e.}, \ R_{3} \lt 1, $ |
then $ p_{R^{1}_{S_{2}}} > 0 $ and consequently $ R^{1}_{S_{2}} $ is a locally asymptotically stable node (or focus).
In order to show the global stability of $ R^{1}_{S_{2}} $, for simplifying the calculations, we consider a polynomial system, which has the same equilibria $ R^{0}_{S_{2}} $, $ R^{1}_{S_{2}} $, $ R^{2}_{S_{2}} $ and dynamics as system (3.1) for $ x > -\frac{1}{\omega} $. To do this, multiplying both sides of system (3.1) by the function $ \frac{1+\omega x}{\beta} $ and introducing a new time variable $ \tau $ by $ dt = \frac{1+\omega x}{\beta}d\tau $ yield the following polynomial system:
$ {dx(t)dτ=x(a1+a2x−a3x2−y)≐P2(x,y),dy(t)dτ=b1xy−b2y+b3+b4x≐Q2(x,y), $ | (3.10) |
where $ a_{1} = \frac{r_{1}}{\beta}, a_{2} = \frac{r_{1} \omega-\frac{r}{K}}{\beta}, a_{3} = \frac{r \omega}{\beta K}, b_{1} = \frac{\eta \beta-\delta_{1} \omega}{\beta}, b_{2} = \frac{\delta_{1}}{\beta}, b_{3} = \frac{\tau}{\beta}, b_{4} = \frac{\tau \omega}{\beta} $, and $ a_{1}, a_{3}, b_{1}, b_{2}, b_{3}, b_{4} $ are all positive constants, the signs of $ a_{2} $ could vary.
Let $ B(x, y) = x^{-1} $ be the Dulac function [30], by a simple calculation, we can obtain that
$ \frac{\partial BP_{2}(x, y)}{\partial x} = \frac{\partial(a_{1}+a_{2}x-a_{3}x^{2}-y)}{\partial x} = a_{2}-2a_{3}x, $ |
$ \frac{\partial BQ_{2}(x, y)}{\partial y} = \frac{\partial(b_{1}y-b_{2}x^{-1}y+b_{3}x^{-1}+b_{4})}{\partial y} = b_{1}-b_{2}x^{-1}, $ |
$ D≐∂BP2∂x+∂BQ2∂y=−x−1[2a3x2−(a2+b1)x+b2]≐−x−1g(x). $ | (3.11) |
It follows from (3.11) that if
$ \Delta_{2}\doteq(a_{2}+b_{1})^{2}-8a_{3}b_{2} = (\frac{\eta \beta-\delta_{1} \omega-\frac{r}{K}+r_{1} \omega}{\beta})^{2}-\frac{8r \delta_{1} \omega}{\beta^{2} K} \lt 0, $ |
i.e.,
$ -2\sqrt{\frac{2r \delta_{1} \omega}{K}} \lt \eta \beta-\delta_{1} \omega-\frac{r}{K}+r_{1}\omega \lt 2\sqrt{\frac{2r \delta_{1} \omega}{K}}, $ |
then the function $ g(x) = 2a_{3}x^{2}-(a_{2}+b_{1})x+b_{2} > 0 $ for all $ x\in R $. That is to say, $ D < 0 $ for all $ (x, y)\in {R^{2}_{+}} $. Note that for the function $ g(x) $, it is easy to see that when $ a_{2}+b_{1} < 0 $, i.e., $ \eta \beta-\delta_{1} \omega-\frac{r}{K}+r_{1}\omega < 0, $ we have $ g(x) > 0 $ for all $ x\geq 0 $ and consequently $ D < 0 $ for all $ (x, y)\in {R^{2}_{+}} $.
Therefore, according to the above analyses, we can conclude that if
$ \eta \beta-\delta_{1} \omega-\dfrac{r}{K}+r_{1}\omega \lt 2\sqrt{\dfrac{2r \delta_{1} \omega}{K}}, $ |
which is equivalent to the inequality
$ \frac{(\eta \beta-\delta_{1} \omega-\frac{r}{K}+r_{1}\omega)\sqrt{2r \delta_{1} \omega K}}{4r \delta_{1} \omega} \lt 1, \ \mathrm{i.e.}, \ R_{4} \lt 1, $ |
then $ D < 0 $, and consequently system (3.10) does not exist with any periodic solutions lying in the interior of $ {R^{2}_{+}} $, which indicates that $ R^{1}_{S_{2}} $ is globally stable, as shown in Figure 2A. This completes the proof.
Remark It is easy to see that the condition $ R_4 < 1 $ is stronger than the inequality $ R_3 < 1 $, which reveals that the local stability of the equilibrium $ R^{1}_{S_{2}} $ does not indicate the global stability. However, extensive numerical investigations show that $ R^{1}_{S_{2}} $ is a globally stale equilibrium provided that $ R_3 < 1 $, but unfortunately we have no way to prove it at present.
Theorem 3.4. If $ R_{3} > 1 $ holds true, then system (3.10) has at least one limit cycle in the interior of $ {R^{2}_{+}} $.
Proof. Based on the analyses in Theorem 3.3, we know that if $ R_{3} > 1 $, then the positive equilibrium $ R^{1}_{S_{2}} $ is an unstable focus (or node). To show the existence of a limit cycle, we define the following four lines:
$ L_1: x-\frac{r_{1}K}{r} = 0; \ L_2: x = 0; \ L_3: y = 0 \ \mathrm{and}\ L_4: x+\frac{1}{b_{1}}y+P = 0, $ |
which form a closed region $ G $, where $ P $ is a constant and the positive equilibrium $ R^{1}_{S_{2}} $ belongs to $ G $. For all $ x\in \left [0, \frac{r_{1}K}{r}\right] $ and $ y > 0 $, we have
$ \frac{dL_1}{dt}\Big|_{x = \frac{r_{1}K}{r}} = -\frac{r_{1}K}{r}y \lt 0, $ |
which indicates that if the trajectories of system (3.10) intersect with the line $ L_1 $, it will pass from the right side of the line $ L_1 $ to the left, entering into the region $ G $. Moreover, it follows from
$ \frac{dL_3}{dt}\Big|_{y = 0} = b_{3}+b_{4}x \gt 0 $ |
that if the trajectories of system (3.10) intersect with the line $ L_3 $, it will pass from the below of the line $ L_{3} $ to the top, entering into the region $ G $. Further, by a simple calculation, we have
$ \frac{dL_4}{dt}\Big|_{y = -b_{1}(x+P)} = x\left(a_{1}+a_{2}x-a_{3}x^{2}+\frac{b_{4}}{b_{1}}\right)+\left(1+b_{2}\right)P+\frac{b_{3}}{b_{1}}. $ |
Thus, if we choose the constant $ P $ such that $ P < -\max\left\{\frac{1}{1+b_{2}}[x(a_{1}+a_{2}x-a_{3}x^{2}+\frac{b_{4}}{b_{1}})+\frac{b_{3}}{b_{1}}]\right\} $, then $ \frac{dL_4}{dt}\Big|_{y = -b_{1}(x+P)} < 0 $. That is to say, if the trajectories of system (3.10) intersect with the line $ L_4 $, it will pass from the top of the line $ L_4 $ to below it, entering into the region $ G $.
Note that $ L_2 $ is one of the trajectories of system (3.10), which approaches the boundary equilibrium $ R^{0}_{S_{2}} $ and the trajectories initiating from region $ G $ will remain in it, i.e., $ L_1 $, $ L_2 $, $ L_3 $ and $ L_4 $ form a Bendixson curve. Thus, according to the Poincaré-Bendixson Theorem [30], system (3.10) has at least one limit cycle around the positive equilibrium $ R^{1}_{S_{2}} $, as shown in Figure 2B. This completes the proof.
Unfortunately, we can not employ the main results shown in literature [30] to address the uniqueness of the limit cycle. Therefore, in the following, we further consider the uniqueness and stability of the limit cycle through bifurcation analyses in subsection 3.2.
In this case, system (3.1) has at most three equilibria in $ R^{2}_{+} $ including two positive equilibria $ R^{1}_{S_{2}}(x_{1}, y_{1}) $ and $ R^{2}_{S_{2}}(x_{2}, y_{2}) $, and a boundary equilibrium $ R^{0}_{S_{2}}(0, \frac{\tau}{\delta_{1}}) $ which is a stable node if $ R_{1} > 0 $ and it is an unstable saddle if $ R_{1} < 0 $. Based on the characteristic equation (3.7), for $ R^{1}_{S_{2}} $ and $ R^{2}_{S_{2}} $, we have
$ p_{R^{1}_{S_{2}}} = \frac{1}{1+\omega x_{1}}\left[\frac{2rw}{K}x^{2}_{1}-(\eta \beta-\delta_{1} \omega-\frac{r}{K}+r_{1}\omega)x_{1}+\delta_{1}\right], q_{R^{1}_{S_{2}}} = \frac{x_{1}\sqrt{\Delta}}{1+\omega x_{1}} $ |
and
$ p_{R^{1}_{S_{2}}} = \frac{1}{1+\omega x_{2}}\left[\frac{2rw}{K}x^{2}_{2}-(\eta \beta-\delta_{1} \omega-\frac{r}{K}+r_{1}\omega)x_{2}+\delta_{1}\right], q_{R^{1}_{S_{2}}} = -\frac{x_{2}\sqrt{\Delta}}{1+\omega x_{2}}. $ |
Obviously, $ q_{R^{1}_{S_{2}}} > 0 $ and $ q_{R^{2}_{S_{2}}} < 0 $. That is to say, $ R^{2}_{S_{2}} $ is an unstable saddle and $ R^{1}_{S_{2}} $ is an elementary and not saddle-type equilibrium. Further, we have the following results:
Theorem 3.5. If $ R_{0} < 0 $, $ R_{1} > 0 $, $ R_{2} < 0 $ and $ \Delta > 0 $, then system (3.1) exists with three equilibria: $ R^{0}_{S_{2}} $, $ R^{1}_{S_{2}} $ and $ R^{2}_{S_{2}} $. Further, $ R^{0}_{S_{2}} $ is a locally stable node, $ R^{2}_{S_{2}} $ is an unstable saddle and $ R^{1}_{S_{2}} $ is a node (or a focus) which is locally stable provided $ R_{3} < 1 $.
Theorem 3.6. If $ R_{0} < 0 $, $ R_{1} > 0 $, $ R_{2} < 0 $ and $ \Delta = 0 $, then system (3.1) exists with a locally stable boundary equilibrium $ R^{0}_{S_{2}} $. Moreover, $ R^{1}_{S_{2}} $ collides with $ R^{2}_{S_{2}} $, which is a saddle-node of codimension 1 when $ R_{3} \neq 1 $.
Theorem 3.7. If $ R_{0} < 0 $, $ R_{1} > 0 $, $ R_{2} < 0 $ and $ \Delta < 0 $, then system (3.1) exists with a boundary equilibrium $ R^{0}_{S_{2}} $, which is a globally asymptotically stable node.
Theorem 3.8. If $ R_{0} < 0 $ and $ R_{1} < 0 $, then system (3.1) has an unstable boundary equilibrium $ R^{0}_{S_{2}} $ and a positive equilibrium $ R^{1}_{S_{2}} $. Further, if $ R_{3} < 1 $, then $ R^{1}_{S_{2}} $ is a locally stable node (or a focus), and it is globally stable provided that $ R_{4} < 1 $.
Theorem 3.9. If $ R_{0} < 0 $ and $ R_{1} = 0 $, then the boundary equilibrium $ R^{0}_{S_{2}} $ is a high order singular point. More precisely,
(ⅰ) $ R^{0}_{S_{2}} $ is a saddle-node of codimension 1 if $ R_{2}\neq 0 $;
(ⅱ) $ R^{0}_{S_{2}} $ is an unstable saddle of codimension 2 if $ R_{2} = 0 $.
Proof. The proof of the conclusion (ⅰ) is similar to that for Theorem 2, we discuss the conclusion (ⅱ) in the following.
For system (3.4), when $ R_{2} = 0 $, then it can be represented as
$ {dxdt=βδ1xy+r1ωR0δ1x3−βωδ1x2y+M3(x,y)≐P2(x,y),dydt=−δ1y−r1ηR0x2+(1+r1δ1)ηβxy+r1ηωR0(1+r1δ1)x3−ηβω(1+r1δ1)x2y+M4(x,y)≐Q2(x,y)−δ1y, $ | (3.12) |
Solving the equation $ -\delta_{1} y+Q_{2}(x, y) = 0 $, we obtain the implicit solution
$ ϕ(x)≐−r1ηR0δ1x2+O(x3). $ | (3.13) |
Then substituting $ \phi(x) $ into $ P_{2}(x, y) $, which can be written in the form
$ ψ(x)≐P2(x,ϕ(x))=−r1R20δ21x3+O(x4). $ | (3.14) |
According to Theorems 7.1–7.3 in [30], it is easy to obtain that $ R^{0}_{S_{2}} $ is an unstable saddle of codimension 2 if $ R_{0} < 0 $, $ R_{1} = 0 $ and $ R_{2} = 0 $. The proof is completed.
In this section, we will discuss various possible bifurcations of system (3.1) including transcritical, saddle-node, Hopf and Bogdanov-Takens bifurcations.
It follows from Lemma 3.1 and Theorems 3.1–3.3, that the positive equilibrium $ R^{1}_{S_{2}} $ collides with the boundary equilibrium $ R^{0}_{S_{2}} $ when $ R_{1} = 0 $, and solving $ R_1 = 0 $ with respect to $ \tau $, we have $ \tau^{*}_{0} = \frac{r_{1} \delta_{1} }{\beta} $. Moreover, if $ \tau < \tau^{*}_{0} $, then system (3.1) has two equilibria: $ R^{1}_{S_{2}} $ which is a stable node (or focus) provided that $ R_{3} < 1 $, and $ R^{0}_{S_{2}} $ which is an unstable saddle. If $ \tau > \tau^{*}_{0} $, then $ R^{1}_{S_{2}} $ becomes negative which is unstable, and $ R^{0}_{S_{2}} $ becomes a stable node, which indicates that the transcritical bifurcation occur at $ \tau^{*}_{0} $, as shown in Figure 3. Further, we can obtain that
$ TB = \left\{(r, \alpha, \beta, \eta, \omega, \tau, \delta_{1})| \ R_{0} \gt 0, \tau = \tau^{*}_{0} \right\} $ |
is a transcritical bifurcation surface. This indicates that there exists a critical releasing rate $ \tau^{*}_{0} $ such that the pests and natural enemies coexist in the form of a positive equilibrium or a periodic orbit with a finite period when the releasing rate $ \tau < \tau^{*}_{0} $, and the pest population goes extinct when the releasing rate $ \tau > \tau^{*}_{0} $.
From Lemma 3.1 and Theorems 3.5–3.7, we can obtain that
$ SN = \left\{(r, \alpha, \beta, \eta, \omega, \tau, \delta_{1}, K)| \ R_{0} \lt 0, R_{1} \gt 0, R_{2} \lt 0 \ \text{and} \ \Delta = 0 \right\} $ |
is a saddle-node bifurcation surface. When the parameters pass from the one side of the surface to the other, the number of positive equilibria of system (3.1) changes from zero to two, and the two positive equilibria are saddle and node, as shown in Figure 4. Especially, if we choose $ \tau $ as bifurcation parameter and solving $ \Delta = 0 $ with respect to $ \tau $, we have $ \tau^{*}_{1} = \frac{K[\frac{r \delta_{1}}{K}-r_{1}R_{0}]^{2}}{4 \beta r R_{0}} $ and the above saddle-node bifurcation surface can be represented as
$ SN = \left\{(r, \alpha, \beta, \eta, \omega, \tau, \delta_{1}, K)| \ R_{0} \lt 0, R_{1} \gt 0, R_{2} \lt 0 \ \text{and} \ \tau = \tau^{*}_{1} \right\}. $ |
The saddle-node bifurcation reveals that if the releasing rate $ \tau < \tau^{*}_{1} $, then there exist some parameter spaces such that the pests and natural enemies may coexist in the form of a positive equilibrium or a periodic orbit with a finite period for different initial values, and if the releasing rate $ \tau > \tau^{*}_{1} $, then the pest population will be driven to extinction, as shown in Figure 4B, C.
For the characteristic equation related to the positive equilibrium $ R^{1}_{S_{2}}(x_{1}, y_{1}) $ of system (3.1), we have
$ λ2+pR1S2λ+qR1S2=0, $ | (3.15) |
where
$ qR1S2=2rR0x1K(1+ωx1)[KR22rR0−x1]>0. $ | (3.16) |
and
$ pR1S2=11+ωx1[2rωKx21−(ηβ−δ1ω−rK+r1ω)x1+δ1]. $ | (3.17) |
Obviously, when $ p_{R^{1}_{S_{2}}} = 0 $, solving the characteristic equation (3.15), we can get two complex eigenvalues $ \lambda_{1, 2} = \pm ib_{0} $ with $ b_{0} = \sqrt{q_{R^{1}_{S_{2}}}} $. That is to say, the positive equilibrium $ R^{1}_{S_{2}} $ is a center-type equilibrium of the linear system of system (3.1), which indicates that system (3.1) may undergo a Hopf bifurcation if the bifurcation parameters are chosen suitably.
We choose the releasing constant $ \tau $ as the bifurcation parameter and fix all other parameters. To determine the critical bifurcation value $ \tau^{*} $, we consider the equation $ p_{R^{1}_{S_{2}}}(\tau^{*}) = 0 $, i.e.,
$ m2(τ∗)2+m1τ∗+m0=0, $ | (3.18) |
where $ m_{2} = \frac{16r^{2}\beta^{2}a^{2}_{4}R^{2}_{0}}{K^{2}} $, $ m_{1} = \frac{4r \beta R_{0}(2a_{4}a_{6}-a^{2}_{5}-2r_{1}R_{0}+\frac{2r \delta_{1}}{K})}{K} $, $ m_{0} = a^{2}_{4}[\frac{r \delta_{1}}{K}-r_{1}R_{0}]^{4}+(2a_{4}a_{6}-a^{2}_{5})[\frac{r \delta_{1}}{K}-r_{1}R_{0}]^{2} $, $ a_{4} = \frac{K\omega}{2r\beta R^{2}_{0}} $, $ a_{5} = \frac{K}{2rR_{0}}(\frac{r_{1}\omega-\frac{r}{K}+\eta \beta-\delta_{1}\omega}{\beta}-\frac{r_{1}K}{r}-\frac{\delta_{1}}{R_{0}}) $ and $ a_{6} = \frac{r\omega}{2\beta K}(\frac{r_{1}K}{r}+\frac{\delta_{1}}{R_{0}})^{2}+ \left[\frac{\delta_{1}}{\beta}-\frac{r_{1}\omega-\frac{r}{K}+\eta \beta-\delta_{1}\omega}{\beta}(\frac{r_{1}K}{2r}+\frac{\delta_{1}}{2R_{0}})\right]. $ Denote $ \Delta_{3}\doteq m^{2}_{1}-4m_{0}m_{2} $, then for the existence of critical value $ \tau^* $ we have the following results:
Lemma 3.2. The existence of parameter $ \tau^{*} $ can be described as follows:
(ⅰ) if $ \Delta_{3} < 0 $, then $ \tau^{*} $ does not exist.
(ⅱ) if $ \Delta_{3} = 0 $ and $ m_{1} < 0 $, then there exists a unique $ \tau^{*} $.
(ⅲ) if $ \Delta_{3} > 0 $, $ m_{0} = 0 $ and $ m_{1} < 0 $, then there exists a unique $ \tau^{*} $.
(Ⅴ) if $ \Delta_{3} > 0 $, $ m_{0} < 0 $, then there exists a unique $ \tau^{*} $.
(Ⅳ) if $ \Delta_{3} > 0 $, $ m_{0} > 0 $ and $ m_{1} < 0 $, then there exist two critical values of $ \tau^{*} $.
Based on Lemma 3.2, we assume that there exists at least a critical value $ \tau^* $ such that $ p_{R^{1}_{S_{2}}}(\tau^{*}) = 0 $, and then address the Hopf bifurcation, i.e., we have the following main results:
Theorem 3.10. For system (3.1), assume that there exists a critical value $ \tau^{*} > 0 $ such that $ p_{R^{1}_{S_{2}}}(\tau^{*}) = 0 $, then system (3.1) undergoes a Hopf bifurcation and there is a unique limit cycle in the neighborhood of $ R^{1}_{S_{2}} $ provided $ R_{4} > 1 $.
Proof. Let $ \lambda = a(\tau)+ib(\tau) $ be a complex root of the characteristic equation (3.15), where $ a(\tau^{*}) = 0 $ and $ b(\tau^{*}) = b_{0} $. Substituting it into the characteristic equation (3.15), it becomes
$ \left[a(\tau)+i b(\tau)\right]^{2}+p_{R^{1}_{S_{2}}}\left[a(\tau)+i b(\tau)\right]+q_{R^{1}_{S_{2}}} = 0, $ |
which can be represented as
$ {a(τ)2−b(τ)2+pR1S2(τ)a(τ)+qR1S2(τ)=0,2a(τ)b(τ)+pR1S2(τ)b(τ)=0. $ | (3.19) |
Taking the partial derivative of (3.19) with respect to $ \tau $, we can obtain
$ {2[a(τ)a′(τ)−b(τ)b′(τ)]+pR1S2(τ)a′(τ)+p′R1S2(τ)a(τ)+q′R1S2(τ)=0,2[a′(τ)b(τ)+a(τ)b′(τ)]+p′R1S2(τ)b(τ)+pR1S2(τ)b′(τ)=0. $ |
It follows from $ a(\tau^{*}) = 0 $, $ p_{R^{1}_{S_{2}}}(\tau^{*}) = 0 $ and $ b(\tau^{*}) = b_{0} $ that we have
$ a′(τ)|τ=τ∗=−12p′R1S2(τ)|τ=τ∗, $ | (3.20) |
which is called the transversality condition [33,34].
If $ a'(\tau)|_{\tau = \tau^{*}} \neq 0 $, then the transversality condition (3.20) is satisfied, which indicates that the Hopf bifurcation takes place and there exists a unique limit cycle in the neighborhood of the positive equilibrium $ R^{1}_{S_{2}} $. By a simple calculation, we can obtain that
$ a′(τ)|τ=τ∗=−12(1+ωx1)[4rwKx1−(ηβ−δ1ω−rK+r1ω)]dx1dτ|τ=τ∗=−2rωK(1+ωx1)[(x1−K(ηβ−δ1ω−rK+r1ω)4rω]dx1dτ|τ=τ∗, $ | (3.21) |
where $ \frac{dx_{1}}{d \tau}\big|_{\tau = \tau^{*}} = -\frac{\beta}{\sqrt{R^{2}_{2}+\frac{4rR_{0}R_{1}}{K}}} < 0 $.
It follows from $ p_{R^{1}_{S_{2}}} = \frac{1}{1+\omega x_{1}}\left[\frac{2r \omega}{K}x_{1}^{2}-(\eta \beta-\delta_{1} \omega-\frac{r}{K}+r_{1} \omega)x_{1}+\delta_{1} \right] $ that $ x_{1} $ is a positive real root of the following equation:
$ 2rωKx21−(ηβ−δ1ω−rK+r1ω)x1+δ1=0 $ | (3.22) |
at $ \tau = \tau^{*} $, which indicates that
$ \Delta_{4} \doteq (\eta \beta-\delta_{1} \omega-\frac{r}{K}+r_{1} \omega)^{2}-\frac{8r \omega \delta_{1}}{K} \geq 0 \ \mathrm{and} \ \eta \beta-\delta_{1} \omega-\frac{r}{K}+r_{1} \omega \gt 0 $ |
in the above equation. Solving equation (3.22) yields two roots, denoted by
$ x_{11} = \frac{K(\eta \beta -\delta_{1} \omega-\frac{r}{K}+r_{1} \omega)}{4 r \omega}+ \frac{K \sqrt{\Delta_{4}}}{4 r \omega}\ \mathrm{and} \ x_{12} = \frac{K(\eta \beta -\delta_{1} \omega-\frac{r}{K}+r_{1} \omega)}{4 r \omega}- \frac{K \sqrt{\Delta_{4}}}{4 r \omega}. $ |
If $ x_{1} = x_{11} $, then we have
$ a′(τ)|τ=τ∗=−K√Δ44rωdx1dτ|τ=τ∗. $ | (3.23) |
If $ x_{1} = x_{12} $, then we have
$ a′(τ)|τ=τ∗=K√Δ44rωdx1dτ|τ=τ∗. $ | (3.24) |
Therefore, it follows from (3.23) and (3.24) that if $ \Delta_{4} > 0 $, which is equivalent to the inequality
$ \frac{(\eta \beta-\delta_{1} \omega-\frac{r}{K}+r_{1}\omega)\sqrt{2r \delta_{1} \omega K}}{4r \delta_{1}\omega} \gt 1, \ \mathrm{i.e.}, \ R_{4} \gt 1, $ |
then $ a'(\tau)|_{\tau = \tau^{*}}\neq 0 $, and consequently the Hopf bifurcation takes place at $ \tau = \tau^{*} $.
To determine the stability of the limit cycle and the direction of the Hopf bifurcation in this case, we need to compute the first Liapunov coefficient $ l_{1}(\tau^{*}) $ [34,35] related to the positive equilibrium $ R^{1}_{S_{2}} $. To do this, we translate the origin of the coordinates to the positive equilibrium $ R^1_{S_{2}} $ of system (3.1) by the change of variables
$ \bar{x} = x-x_{1}, \quad \bar{y} = y-y_{1}, $ |
rewrite ($ \bar{x}, \bar{y} $) as (x, y) and expand the right-hand side of system (3.1) in a Taylor series about the origin, then we can obtain
$ {dxdt=ax+by+a20x2+2a11xy+a30x3+a21x2y+R1(x,y),dydτ=cx+dy+b20x2+2b11xy+b30x3+b21x2y+R2(x,y), $ | (3.25) |
where $ a \doteq r_{1}-\frac{2r}{K}x_{1}-\frac{\beta y_{1}}{(1+\omega x_{1})^{2}} $, $ b \doteq -\frac{\beta x_{1}}{1+\omega x_{1}} $, $ c \doteq \frac{\eta \beta y_{1}}{(1+\omega x_{1})^{2}} $, $ d \doteq -\delta_{1}+\frac{\eta \beta x_{1}}{1+\omega x_{1}} $, $ a_{20} \doteq -\frac{r}{K}+\frac{\beta \omega y_{1}}{(1+\omega x_{1})^{3}} $, $ a_{11} \doteq -\frac{\beta}{2(1+\omega x_{1})^{2}} $, $ b_{20} \doteq -\frac{\eta \beta \omega y_{1}}{(1+\omega x_{1})^{3}} $, $ b_{11} \doteq \frac{\eta \beta}{2(1+\omega x_{1})^{2}} $, $ a_{30} \doteq -\frac{\beta \omega^{2} y_{1}}{(1+\omega x_{1})^{4}} $, $ a_{21} \doteq \frac{\beta \omega}{(1+\omega x_{1})^{3}} $, $ b_{30} \doteq \frac{\eta \beta \omega^{2} y_{1}}{(1+\omega x_{1})^{4}} $, $ b_{21} \doteq -\frac{\eta \beta \omega}{(1+\omega x_{1})^{3}} $, and $ R_{1}(x, y) $ and $ R_{2}(x, y) $ are $ C^{\infty} $ functions in $ (x, y) $ at least of the fourth order.
Therefore, by employing the formula of the first Liapunov number $ l_{1}(\tau^{*}) $ at the origin of (3.25) in [34,35], we have
$ l1(τ∗)=−rωx21Kβb30[(r1δ1β2−τβ)+rω(ηβ−δ1ω)β2Kx31+βK2rω(δ1β−ηβ−δ1ωβx1)(δ1(ηβ−δ1ω)β2x1+rδ1ωβ2K)]=−rωx21Kβ3b30[−R1+rωR0Kx31+Kδ1R02rω(δ1R0−x1)(R0x1+rωK)]. $ |
Moreover, if $ l_{1}(\tau^{*}) < 0 $, then system (3.1) undergoes a supercritical Hopf bifurcation at $ \tau = \tau^{*} $ and there is a unique and stable limit cycle [35]. If $ l_{1}(\tau^{*}) > 0 $, then system (3.1) undergoes a subcritical Hopf bifurcation at $ \tau = \tau^{*} $ and there is a unique and unstable limit cycle [35]. This completes the proof.
To confirm the main results obtained here, we choose $ \tau $ as the bifurcation parameter and fix the others as those shown in Figure 5. Bifurcation diagrams shown in Figure 5A, B indicate there exist Hopf bifurcation points $ \tau^{*}_{i} \ (i = 2, 3, 4) $ marked as HB. Moreover, by a simple calculation, we have $ \tau^{*}_{4} = 0.27 $, $ p_{R^{1}_{S_{2}}}(\tau^{*}_{4}) = 0 $, $ a^{'}_{R^{1}_{S_{2}}}(\tau)|_{\tau = \tau^{*}_{4}}\neq 0 $ and $ l_{1}(\tau^{*}_{4}) < 0 $, i.e., system (3.1) undergoes a supercritical Hopf bifurcation at $ \tau^{*}_{4} $ and it has a stable limit cycle in the neighborhood of $ R^{1}_{S_{2}} $, as shown in Figure 5B, C.
From Lemma 3.1 and Theorem 3.6, we can obtain that if $ R_{0} < 0 $, $ R_{1} > 0 $, $ R_{2} < 0 $ and $ \Delta = 0 $, then $ R^{1}_{S_{2}} $ collides with $ R^{2}_{S_{2}} $ as shown in Figure 4-A, and for convenience we still denote it by $ R^{1}_{S_{2}} $. According to the characteristic equation (3.7), by a simple calculation, we have
$ {pR1S2=11+ωx1[2rwKx21−(ηβ−δ1ω−rK+r1ω)x1+δ1],qR1S2=2rR0x1K(1+ωx1)[KR22rR0−x1] $ | (3.26) |
with $ x_{1} = \frac{r_{1}K}{2r}+\frac{\delta_{1}}{2(\eta \beta-\delta_{1}\omega)} = \frac{KR_{2}}{2rR_{0}} $. Note that we have $ q_{R^{1}_{S_{2}}} = 0 $ in this case. And if $ p_{R^{1}_{S_{2}}} = 0 $ (i.e., $ R_{3} = 1 $), then the characteristic equation (3.7) related to the positive equilibrium $ R^{1}_{S_{2}} $ has two zero eigenvalues. This suggests that system (3.1) may admit a Bogdanov-Takens bifurcation [30,31,34] under a small parameter perturbation if the bifurcation parameters are chosen suitably and we confirm this by giving the following theorem.
Theorem 3.11. Suppose $ p_{R^{1}_{S_{2}}} = 0 $ and $ q_{R^{1}_{S_{2}}} = 0 $, then the positive equilibrium $ R^{1}_{S_{2}}(x_{1}, y_{1}) $ of system (3.1) is a cusp of codimension 2 if $ ef \neq 0 $, where $ e = 2b_{11}+2a_{20}-\frac{2a a_{11}}{b} $ and $ f = a a_{20}-\frac{2a^{2} a_{11}}{b}+b b_{20}-2a b_{11} $.
Proof. Assume that $ p_{R^{1}_{S_{2}}} = 0 $ and $ q_{R^{1}_{S_{2}}} = 0 $, translating the positive equilibrium $ R^{1}_{S_{2}}(x_{1}, y_{1}) $ to the origin by the change of variables $ u = x-x_{1} $ and $ v = y-y_{1} $, renaming $ (u, v) $ as $ (x, y) $ and expanding the right-hand side of system (3.1) in a Taylor series about the origin, then we can obtain
$ {dxdt=ax+by+a20x2+2a11xy+R3(x,y),dydt=cx+dy+b20x2+2b11xy+R4(x,y), $ | (3.27) |
where $ R_{3}(x, y) $ and $ R_{4}(x, y) $ are $ C^{\infty} $ functions in $ (x, y) $ at least of the third order.
Notice that $ b\neq 0 $, making the following change of variables
$ u = x, \, \, \, \, v = ax+by $ |
and renaming $ (u, v) $ as $ (x, y) $, then system (3.27) becomes
$ {dxdt=y+(a20−2aa11b)x2+2a11bxy+R5(x,y),dydt=(aa20−2a2a11b+bb20−2ab11)x2+(2aa11b+2b11)xy+R6(x,y), $ | (3.28) |
where $ R_{5}(x, y) $ and $ R_{6}(x, y) $ are $ C^{\infty} $ functions in $ (x, y) $ at least of the third order. Let
$ u = x-\frac{a_{11}}{b}x^{2}, \, \, \, \, v = y+(a_{20}-\frac{2 a a_{11}}{b})x^{2} $ |
and rename $ (u, v) $ as $ (x, y) $, then system (3.28) can be written as
$ {dxdt=y+R7(x,y),dydt=(aa20−2a2a11b+bb20−2ab11)x2+(2b11+2a20−2aa11b)xy+R8(x,y), $ | (3.29) |
which can be represented as
$ {dxdt=y+R7(x,y),dydt=exy+fx2+R8(x,y), $ |
where $ e = 2 b_{11}+2 a_{20}-\frac{2 a a_{11}}{b} $ and $ f = a a_{20}-\frac{2 a^{2} a_{11}}{b}+b b_{20}-2a b_{11} $, and $ R_{7}(x, y) $ and $ R_{8}(x, y) $ are $ C^{\infty} $ functions in $ (x, y) $ at least of the third order.
Therefore, if $ ef\neq 0 $, then the positive equilibrium $ R^{1}_{S_{2}} $ of system (3.1) is a cusp of codimension 2 by the qualitative theory of ordinary differential equations and the theory of differential manifolds [30,34]. This completes the proof.
Further, we choose $ \tau $ and $ \delta_{1} $ as bifurcation parameters, and suppose that there exist parameters $ \tau^{*} > 0 $ and $ \delta^{*}_{1} > 0 $ such that $ p_{R^{1}_{S_{2}}} = 0 $ and $ q_{R^{1}_{S_{2}}} = 0 $, i.e.,
$ {2rωK[rδ∗1+Kr1(ηβ−δ∗1ω)2r(ηβ−δ∗1ω)]2−(ηβ−δ∗1ω−rK+r1ω)[rδ∗1+Kr1(ηβ−δ∗1ω)2r(ηβ−δ∗1ω)]+δ∗1=0,4rK(βτ∗−r1δ∗1)(ηβ−δ∗1ω)+[r1(ηβ−δ∗1ω)+rδ∗1K]2=0. $ |
Note that the existence of $ (\tau^{*}, \delta_{1}^{*}) $ can be easily confirmed by numerical investigations. We study the dynamical behavior of system (3.1) when parameters $ \tau $ and $ \delta_{1} $ vary in a small neighborhood of $ (\tau^{*}, \delta^{*}_{1}) $, and analyze the local representations of the bifurcation curves in a small neighborhood of the positive equilibrium $ R^{1}_{S_{2}} $.
Theorem 3.12. Suppose $ ef\neq 0 $ at the positive equilibrium $ R^{1}_{S_{2}} $, then system (3.1) undergoes a Bogdanov-Takens bifurcation in a small neighborhood of $ R^{1}_{S_{2}} $ as $ (\tau, \delta_{1}) $ varies near $ (\tau^{*}, \delta^{*}_{1}) $ provided that $ ae+2f\neq 0 $, and system (3.1) has the following bifurcation behaviors in a small neighborhood of $ R^{1}_{S_{2}} $:
(ⅰ) the saddle-node bifurcation curve: $ SN = \{(\varepsilon_{1}, \varepsilon_{2})|\ \frac{ge^{4}}{f^{3}_{1}} = 0\} $;
(ⅱ) the Hopf bifurcation curve: $ HB = \{(\varepsilon_{1}, \varepsilon_{2})|\ \frac{ge^{4}}{f^{3}_{1}}+\frac{h^{2}e^{4}}{f^{4}_{1}} = 0, \frac{he^{2}}{f^{2}_{1}} > 0\} $;
(ⅲ) the homoclinic bifurcation curve: $ HL = \{(\varepsilon_{1}, \varepsilon_{2})|\ \frac{ge^{4}}{f^{3}_{1}}+\frac{49}{25}\frac{h^{2}e^{4}}{f^{4}_{1}} = 0, \frac{he^{2}}{f^{2}_{1}} > 0\} $.
Proof. First of all, let $ \delta_{1} = \delta^{*}_{1}-\varepsilon_{1} $ and $ \tau = \tau^{*}-\varepsilon_{2} $, then system (3.1) can be represented as
$ {dxdt=r1x−rKx2−βxy1+ωx,dydt=ηβxy1+ωx−(δ∗1−ε1)y+(τ∗−ε2). $ | (3.30) |
Translating the positive equilibrium $ R^{1}_{S_{2}}(x_{1}, y_{1}) $ to the origin by the change of variables $ \bar{x} = x-x_{1} $ and $ \bar{y} = y-y_{1} $, renaming $ (\bar{x}, \bar{y}) $ as $ (x, y) $ and expanding the right-hand side of system (3.30) in a Taylor series about the origin, then we can obtain
$ {dxdt=ax+by+a20x2+2a11xy+R9(x,y,ε1,ε2),dydt=(ε1y1−ε2)+cx+d1y+b20x2+2b11xy+R10(x,y,ε1,ε2), $ | (3.31) |
where $ d_{1} = (-\delta^{*}_{1} +\varepsilon_{1})+\frac{\eta \beta x_{1}}{1+\omega x_{1}} $, and $ R_{9}(x, y, \varepsilon_{1}, \varepsilon_{2}) $ and $ R_{10}(x, y, \varepsilon_{1}, \varepsilon_{2}) $ are $ C^{\infty} $ functions in $ (x, y) $ at least of the third order, whose coefficients depend smoothly on $ \varepsilon_{1} $ and $ \varepsilon_{2} $. Let
$ u = x, \, \, \, \, v = ax+by $ |
and rename $ (u, v) $ as $ (x, y) $, then system (3.31) can be written as
$ {dxdt=y+(a20−2aa11b)x2+2a11bxy+R11(x,y,ε1,ε2),dydt=b(ε1y1−ε2)−aε1x+ε1y+(aa20−2a2a11b+bb20−2ab11)x2+(2aa11b+2b11)xy+R12(x,y,ε1,ε2), $ | (3.32) |
where $ R_{11}(x, y, \varepsilon_{1}, \varepsilon_{2}) $ and $ R_{12}(x, y, \varepsilon_{1}, \varepsilon_{2}) $ are $ C^{\infty} $ functions in $ (x, y) $ at least of the third order, whose coefficients depend smoothly on $ \varepsilon_{1} $ and $ \varepsilon_{2} $. Making the following change of variables
$ u = x-\frac{a_{11}}{b}x^{2}, \, \, \, \, v = y+(a_{20}-\frac{2a a_{11}}{b})x^{2} $ |
and renaming $ (u, v) $ as $ (x, y) $, then system (3.32) becomes
$ {dxdt=y+R13(x,y,ε1,ε2),dydt=b(ε1y1−ε2)−aε1x+ε1y+exy+f1x2+R14(x,y,ε1,ε2), $ | (3.33) |
where $ f_{1} = a a_{20}-\frac{2a^{2} a_{11}}{b}+b b_{20}-2a b_{11}-\frac{a a_{11}}{b}\varepsilon_{1}-\varepsilon_{1}(a_{20}-\frac{2a a_{11}}{b}) $, and $ R_{13}(x, y, \varepsilon_{1}, \varepsilon_{2}) $ and $ R_{14}(x, y, \varepsilon_{1}, \varepsilon_{2}) $ are $ C^{\infty} $ functions in $ (x, y) $ at least of the third order, whose coefficients depend smoothly on $ \varepsilon_{1} $ and $ \varepsilon_{2} $.
Next, we make the following change of variables
$ u = x, \, \, \, \, y = y+R_{13}(x, y, \varepsilon_{1}, \varepsilon_{2}) $ |
and rename $ (u, v) $ as $ (x, y) $, then system (3.33) becomes
$ {dxdt=y,dydt=b(ε1y1−ε2)−aε1x+ε1y+exy+f1x2+R15(x,y,ε1,ε2), $ | (3.34) |
where $ R_{15}(x, y, \varepsilon_{1}, \varepsilon_{2}) $ is a $ C^{\infty} $ function in $ (x, y) $ at least of the third order, whose coefficients depend smoothly on $ \varepsilon_{1} $ and $ \varepsilon_{2} $. By setting $ u = x+\frac{\varepsilon_{1}}{e} $ and $ v = y $, renaming $ (u, v) $ as $ (x, y) $, then system (3.34) becomes
$ {dxdt=y,dydt=g+hx+exy+f1x2+R16(x,y,ε1,ε2), $ | (3.35) |
where $ g = b(\varepsilon_{1} y_{1}-\varepsilon_{2})+\frac{f_{1}\varepsilon^{2}_{1}}{e^{2}}+\frac{a\varepsilon^{2}_{1}}{e} $ and $ h = -a\varepsilon_{1}-\frac{2f_{1}\varepsilon_{1}}{e} $, and $ R_{14}(x, y, \varepsilon_{1}, \varepsilon_{2}) $ is a $ C^{\infty} $ function in $ (x, y) $ at least of the third order, whose coefficients depend smoothly on $ \varepsilon_{1} $ and $ \varepsilon_{2} $. Moreover,
$ \lim\limits_{\varepsilon_{j}\to 0}f_{1} = a a_{20}-\frac{2a^{2} a_{11}}{b}+b b_{20}-2a b_{11} = f, $ |
where $ j = 1, 2 $.
Notice that $ f_{1}\neq 0 $ when $ \varepsilon_{j} $ are small. Making the change of variables one more time by setting
$ u = \frac{e^{2} }{f_{1}}x, \, \, v = \frac{e^{3} }{f_{1}^{2}}y, \, \, \tau = \frac{e }{f_{1}}t $ |
when $ \varepsilon_{j} $ are small, and renaming $ (u, v, \tau) $ as $ (x, y, t) $, then system (3.35) can be represented as
$ {dxdt=y,dydt=μ1(ε1,ε2)+μ2(ε1,ε2)x+xy+x2+R17(x,y,ε1,ε2), $ | (3.36) |
where $ \mu_{1}(\varepsilon_{1}, \varepsilon_{2}) = \frac{ge^{4}}{f^{3}_{1}} $ and $ \mu_{2}(\varepsilon_{1}, \varepsilon_{2}) = \frac{he^{2}}{f^{2}_{1}} $, and $ R_{15}(x, y, \varepsilon_{1}, \varepsilon_{2}) $ is a $ C^{\infty} $ function in $ (x, y) $ at least of the third order, whose coefficients depend smoothly on $ \varepsilon_{1} $ and $ \varepsilon_{2} $. Moreover, by a simple calculation, we can obtain that
$ \Bigg | \frac{\partial(\mu_{1}(\varepsilon_{1}, \varepsilon_{2}), \mu_{2}(\varepsilon_{1}, \varepsilon_{2}))}{\partial(\varepsilon_{1}, \varepsilon_{2})}\Bigg |_{(0, 0)} = (-1)\frac{be^{5}}{f^{5}}(ae+2f), $ |
when $ ae+2f\neq 0 $, the above parameter transformation is a homeomorphism in a small neighborhood of the origin [36], and $ \mu_{1} $ and $ \mu_{2} $ are independent parameters.
Based on the above analyses, according to the theorems in Bogdanov and Takens [30,31], we can obtain the local representations of the bifurcation curves of system (3.36) in a small neighborhood of the origin if $ ae+2f\neq 0 $, and it is equivalent to the bifurcation behavior of system (3.1) in a small neighborhood of $ R^{1}_{S_{2}} $, as shown in Theorem 3.12. This completes the proof.
In this section, we mainly analyze the complete behavior of non-smooth Filippov system (2.2) including the existence, stability of the regular/virtual equilibria, pseudo-equilibria, sliding segments, sliding bifurcations and sliding periodic solutions.
In order to analyze the dynamical behavior of non-smooth Filippov system (2.2) in $ R^{2}_{+} $, we need to analyze the existence and stability of the sliding segments and tangent points firstly.
The sliding segment: by a simple calculation, we can obtain
$ σ(x,y)=⟨HX(x,y),f1(x,y)⟩⟨HX(x,y),f2(x,y)⟩=⟨(1,0),(rx−rKx2−βxy1+ωx,ηβxy1+ωx−δy)⟩ ⟨(1,0),(rx−rKx2−βxy1+ωx−αx,ηβxy1+ωx−δ1y+τ)⟩=x2(r−rKx−βy1+ωx)(r1−rKx−βy1+ωx), $ |
where $ r_{1} = r-\alpha $ and $ 0 < ET < K $. It is easy to obtain that if $ x = ET $, then $ \sigma(x, y)\leq 0 $ is equivalent to the inequalities
$ \frac{r_{1}}{\beta}+(\frac{r_{1}\omega}{\beta}-\frac{r}{\beta K})ET-\frac{r \omega}{\beta K}ET^{2}\leq y \leq \frac{r}{\beta}+(\frac{r\omega}{\beta}-\frac{r}{\beta K})ET-\frac{r \omega}{\beta K}ET^{2}. $ |
For convenience, denote
$ y_{\min}\doteq \frac{r_{1}}{\beta}+(\frac{r_{1}\omega}{\beta}-\frac{r}{\beta K})ET-\frac{r \omega}{\beta K}ET^{2}, $ |
$ y_{\max}\doteq \frac{r}{\beta}+(\frac{r\omega}{\beta}-\frac{r}{\beta K})ET-\frac{r \omega}{\beta K}ET^{2}, $ |
where $ y_{\min}\geq0 $ for all $ ET\in(0, \frac{r_{1}K}{r}] $; $ y_{\max} > 0 $ for all $ ET\in(0, K) $. And if one of the following conditions
$ y \lt y_{\min}\ \ \ \mathrm{or}\ \ \ y \gt y_{\max} $ |
holds true, then $ \sigma(x, y) > 0 $.
Therefore, the sliding segment of non-smooth Filippov system (2.2) is defined as
$ \Sigma_{s} = \{(x, y)|x = ET, \max\{0, y_{\min} \}\leq y \leq y_{\max}\}, $ |
and the crossing set is given as
$ \Sigma_{c} = \{(x, y)|x = ET, 0\leq y \lt \max\{0, y_{\min}\}\ \ \ \mathrm{or}\ \ \ y \gt y_{\max}\}. $ |
Moreover, we have
$ ⟨HX(x,y),f1(x,y)⟩=⟨(1,0),(rx−rKx2−βxy1+ωx,ηβxy1+ωx−δy)⟩=rx−rKx2−βxy1+ωx>0 $ |
and
$ ⟨HX(x,y),f2(x,y)⟩=⟨(1,0),(rx−rKx2−βxy1+ωx−αx,ηβxy1+ωx−δ1y+τ)⟩=r1x−rKx2−βxy1+ωx<0 $ |
for all points $ (x, y)\in \Sigma_{s} $, which indicates that the sliding segment $ \Sigma_{s} $ is stable.
According to the Filippov convex method [32], we can obtain the following sliding dynamical equation defined in $ (x, y)\in \Sigma_{s} $
$ dydt=λ(ηβx1+ωxy−δy)+(1−λ)(ηβx1+ωxy−δ1y+τ)≐F(y), x(t)=ET, $ | (4.1) |
where
$ \lambda = \frac{\langle(1, 0), ((r_{1}-\frac{r x}{K}) x-\frac{\beta x}{1+\omega x}y, \frac{\eta\beta x}{1+\omega x}y-\delta_{1} y+\tau)\rangle}{\langle(1, 0), (-\alpha x, (\delta-\delta_{1})y+\tau)\rangle} = (1-\frac{r}{\alpha})+\frac{r ET}{\alpha K}+\frac{\beta y}{\alpha(1+\omega ET)}. $ |
The equilibrium $ R_{0}(x^{*}, y^{*})\in \Sigma_{s} $ of system (4.1) is called the pseudo-equilibrium of non-smooth Filippov system (2.2).
Tangent point: The tangent points $ T_{i}(x_{i}, y_{i}) (i = 1, 2) $ of non-smooth Filippov system (2.2) satisfy the following equations
$ {H(xi,yi)=0,⟨HX(xi,yi),fi(xi,yi)⟩=0. $ |
By a simple calculation, we have
$ {x1=ET,y1=ymax;x2=ET,y2=ymin. $ |
That is to say, the sliding segment $ \Sigma_{s} $ is delimited by tangent points $ T_{1}(x_1, y_1) $ and $ T_{2}(x_2, y_2) $, which lie between the horizontal non-zero isocline $ L^{1}_{S_{1}} $ of subsystem $ S_{1} $ and $ L^{1}_{S_{2}} $ of subsystem $ S_{2} $, as shown in Figure 6A. Note that the tangent point $ T_2 $ becomes an end point of sliding segment once $ y_{\min} $ is less than zero. Therefore, we call $ T_{2}(ET, y_{\min})\in R^{2}_{+} $ as a tangent point if $ ET\in(0, \frac{r_{1}K}{r}] $. Otherwise, if $ ET\in(\frac{r_{1}K}{r}, K) $, we call $ T_{2} $ as an end point of the sliding segment $ \Sigma_{s} $.
For the equilibria of non-smooth Filippov system (2.2), there are four types: regular equilibrium, virtual equilibrium, pseudo-equilibrium and boundary equilibrium, which are associated with the discontinuity boundary $ \Sigma $ and have been defined in Section 2. For convenience, the equilibria of subsystem $ S_{1} $ are denoted by $ R^{i}_{S_{1}}(x^{i}_{S_{1}}, y^{i}_{S_{1}}) $ and the equilibria of subsystem $ S_{2} $ are denoted by $ R^{i}_{S_{2}}(x^{i}_{S_{2}}, y^{i}_{S_{2}}) $, $ i = 0, 1, 2 $.
For the existence and stability of the pseudo-equilibrium $ R_{0}(x^{*}, y^{*}) $ of non-smooth Filippov system (2.2), we only need to consider the existence and stability of equilibrium of system (4.1). Thus, we consider the equation $ F(y) = 0 $, i.e.,
$ λ(ηβET1+ωETy−δy)+(1−λ)(ηβET1+ωETy−δ1y+τ)=0, $ | (4.2) |
which can be represented as
$ β(δ1−δ)α(1+ωET)y2+[−δ−(rα−rETαK)(δ1−δ)+ηβET1+ωET−βτα(1+ωET)]y+rτα(1−ETK)=0. $ | (4.3) |
If $ \delta_{1} = \delta $ (i.e., the pesticide does not affect the predator), solving the above equation with respect to $ y $, we can obtain
$ y^{*} = \frac{\frac{\tau r}{\alpha}(\frac{ET}{K}-1)}{\frac{\eta \beta ET}{1+\omega ET}-\frac{\beta \tau}{\alpha(1+\omega ET)}-\delta}, $ |
where $ y^{*}\in[max\{0, y_{\min}\}, y_{\max}] $ if and only if $ ET\in[x^{1}_{S_{2}}, x^{2}_{S_{1}}] $. Moreover, it is easy to obtain that
$ F'(y^{*}) = -\frac{\beta \tau}{\alpha(1+\omega ET)}+\frac{\eta \beta ET}{1+\omega ET}-\delta = -\frac{\tau r}{\alpha y^{*}}(1-\frac{ET}{K}) \lt 0, $ |
which indicates that the pseudo-equilibrium $ R_{0}(x^{*}, y^{*}) = R_{0}(ET, y^{*})\in \Sigma_{s} $ is locally stable. Further, we have the following result.
Lemma 4.1. Suppose $ \delta_{1} = \delta $, then non-smooth Filippov system (2.2) has a unique and stable pseudo-equilibrium $ R_{0}(ET, y^{*}) $ if and only if $ ET\in [x^{1}_{S_{2}}, x^{2}_{S_{1}}] $.
If $ \delta_{1} > \delta $, it is clear that Eq (4.3) have at most two positive real roots:
$ y^{*}_{1} = \frac{(r-\frac{r ET}{K})(1+\omega ET)}{2 \beta}+\frac{\alpha \delta+\beta \tau -\alpha(\eta \beta-\delta \omega)ET}{2 \beta (\delta_{1}-\delta)}+\frac{\alpha (1+\omega ET) \sqrt{\Delta_{5}}}{2 \beta (\delta_{1}-\delta)} $ |
and
$ y^{*}_{2} = \frac{(r-\frac{r ET}{K})(1+\omega ET)}{2 \beta}+\frac{\alpha \delta+\beta \tau -\alpha(\eta \beta-\delta \omega)ET}{2 \beta (\delta_{1}-\delta)}-\frac{\alpha (1+\omega ET) \sqrt{\Delta_{5}}}{2 \beta (\delta_{1}-\delta)}, $ |
where
$ Δ5≐[−δ−(rα−rETαK)(δ1−δ)+ηβET1+ωET−βτα(1+ωET)]2−4βrτ(δ1−δ)(1−ETK)α2(1+ωET)>0. $ | (4.4) |
This indicates that there may exist two pseudo-equilibria, denoted by $ R^{1}_{0}(ET, y^{*}_{1}) $ and $ R^{2}_{0}(ET, y^{*}_{2}) $, as shown in Figure 7. Further, by a simple calculation, we can obtain that if $ \max\{0, y_{\min}\}\leq y_2^* < y_1^*\leq y_{\max} $, system (2.2) can exactly exist two pseudo-equilibria, and
$ F'(y^{*}_{1}) = \sqrt{\Delta_{5}} \quad \mathrm{and} \quad F'(y^{*}_{2}) = -\sqrt{\Delta_{5}}, $ |
which indicate that $ R^{1}_{0}(ET, y^{*}_{1}) $ is unstable provided that it lies in the $ \Sigma_s $, and $ R^{2}_{0}(ET, y^{*}_{2}) $ is locally stable provided that it lies in the $ \Sigma_s $.
Note that to show how the threshold value $ ET $ affects the existence of pseudo-equilibria of system (2.2), we first consider the function $ F(y) $ defined in (4.1) as a function of $ x $, i.e., the curve $ L_1 $ or $ L_2 $ shown in Figure 7, which can intersect with isoclines $ L_{S_1}^1 $ and $ L_{S_2}^1 $ at two points $ R_{S_2}^1 $ and $ R_{S_1}^2 $. Therefore, the horizontal components of both points may confirm the ranges of $ ET $, within them system (2.2) could exist one or two pseudo-equilibria, as shown in Figure 7. In particular, if $ \delta_{1} = \delta $, then there exists a pseudo-equilibrium $ R_{0}(ET, y^{*})\in \Sigma_{s} $ with $ ET\in [x^{1}_{S_{2}}, x^{2}_{S_{1}}] $. If $ \delta_{1} > \delta $, then for $ ET \in [x^{1}_{S_{2}}, x^{2}_{S_{1}}) $, there exists a unique pseudo-equilibrium $ R^{1}_{0} $; for $ ET \in [x^{2}_{S_{1}}, ET_{1}) $ with $ \Delta_{5}(ET_{1}) = 0 $ (here $ \Delta_{5} $ is considered as a function of $ ET $ defined by (4.4)), there exist two pseudo-equilibria $ R^{1}_{0} $ and $ R^{2}_{0} $, shown in Figure 7B. Especially, if $ ET = ET_{1} $, then $ R^{1}_{0} $ collides with $ R^{2}_{0} $.
Based on the above analyses, we can now clarify the types of equilibria of non-smooth Filippov system (2.2). It follows from the conditions of existence and stability of equilibria of subsystem $ S_{1} $, as shown in Section 2, then we consider the following three cases:
$ (C_{1}): 0 \lt \frac{\delta}{\eta \beta-\delta \omega} \lt K; \ (C_{2}): \frac{\delta}{\eta \beta-\delta \omega} \lt 0; \ (C_{3}): \frac{\delta}{\eta \beta-\delta \omega}\geq K. $ |
For Case $ (C_{1}) $, according to the properties of subsystem $ S_{2} $, we obtain that if $ R_{0} > 0 $ and $ R_{1} < 0 $, then there exist two equilibria: a boundary equilibrium $ R^{0}_{S_{2}}(0, \frac{\tau}{\delta_{1}}) $ which is a unstable saddle, and a positive equilibrium $ R^{1}_{S_{2}}(x^{1}_{S_{2}}, y^{1}_{S_{2}}) $ with
$ x^{1}_{S_{2}} = \frac{KR_{2}-K\sqrt{R^{2}_{2}+\frac{4rR_{0}R_{1}}{K}}}{2rR_{0}}, \ y^{1}_{S_{2}} = (r_{1}-\frac{r}{K}x^{1}_{S_{2}})\frac{1+\omega x^{1}_{S_{2}}}{\beta}. $ |
While subsystem $ S_{1} $ has a unique positive equilibrium $ R^{2}_{S_{1}}(\frac{\delta}{\eta \beta-\delta \omega}, \frac{r \eta (K\eta \beta-K \delta \omega-\delta)}{K(\eta \beta-\delta \omega)^{2}}) $.
If $ \delta_{1} = \delta $, by a simple calculation, we obtain that $ x^{1}_{S_{2}} < \frac{\delta}{\eta \beta-\delta \omega} $, which indicates that $ R^{1}_{S_{2}} $ is on the left of $ R^{2}_{S_{1}} $, as shown in Figure 6B and Figure 7A. And from Figure 7A, it is easy to see that $ R^{0}_{S_{1}} $ is always a regular equilibrium for subsystem $ S_{1} $, $ R^{1}_{S_{1}} $ is always a virtual equilibrium for subsystem $ S_{1} $ and $ R^{0}_{S_{2}} $ is always a virtual equilibrium for subsystem $ S_{2} $. Further, the existence of all types of equilibria will be discussed briefly in the following:
(ⅰ) If $ 0 < ET < x^{1}_{S_{2}} $, we conclude that $ R^{2}_{S_{1}} $ is a virtual equilibrium for subsystem $ S_{1} $, $ R^{1}_{S_{2}} $ is a regular equilibrium for subsystem $ S_{2} $, and $ T_{1} $ is an invisible tangent point and $ T_{2} $ is a visible tangent point [23,24].
(ⅱ) If $ x^{1}_{S_{2}} < ET < x^{2}_{S_{1}} $, we have that $ R^{2}_{S_{1}} $ is a virtual equilibrium for subsystem $ S_{1} $, $ R^{1}_{S_{2}} $ becomes a virtual equilibrium for subsystem $ S_{2} $, and $ T_{1} $ is an invisible tangent point and $ T_{2} $ becomes an invisible tangent point. Moreover, there exists a pseudo-equilibrium $ R_{0} $.
(ⅲ) If $ x^{2}_{S_{1}} < ET < K $, then $ R_{0} $ disappears, $ R^{2}_{S_{1}} $ becomes a regular equilibrium for subsystem $ S_{1} $, $ R^{1}_{S_{2}} $ is a virtual equilibrium for subsystem $ S_{2} $, $ T_{1} $ becomes a visible tangent point and $ T_{2} $ is an invisible tangent point. Especially, when $ ET = x^{1}_{S_{2}} $ (or $ ET = x^{2}_{S_{1}} $), $ R^{1}_{S_{2}} $ (or $ R^{2}_{S_{1}} $) collides with $ \Sigma $, which is a boundary equilibrium. Moreover, three points $ T_{2} $, $ R^{1}_{S_{2}} $ and $ R_{0} $ collide together in this case.
If $ \delta_{1} > \delta $, the position relations of all possible equilibria of two subsystems are hard to determine analytically. However, similar results can be obtained numerically as those shown above, so we omit them here. For Cases $ (C_{2}) $ and $ (C_{3}) $, subsystem $ S_{1} $ only has steady state $ R^{0}_{S_{1}}(0, 0) $ which is unstable, and exists a boundary equilibrium $ R^{1}_{S_{1}}(K, 0) $ which is globally stable related to region $ S_1 $. The positive equilibria of subsystem $ S_{2} $ lie between $ R^{0}_{S_{1}}(0, 0) $ and $ R^{1}_{S_{1}}(K, 0) $. Therefore, we can discuss the types of equilibria of system (2.2) similarly.
In this subsection, we address the bifurcations related to equilibria and sliding cycles concerning sliding segment $ \Sigma_s $.
Note that one of the main purposes of the present paper is to address the integrated pest management strategies for pest control and propose the non-smooth Filippov system (2.2), which indicates that how the key parameters related to control effectiveness affect the dynamics of system (2.2) are quite important. Thus, in this section we choose the releasing constant $ \tau $ as a bifurcation parameter and fix all others to discuss the variations of the trajectories and equilibria of system (2.2).
For Case $ (C_{1}) $, if $ \delta_{1} = \delta $, there may exist a stable pseudo-equilibrium $ R_{0}(x^{*}, y^{*}) $. Moreover, the bifurcation diagrams with respect to $ \tau $ shown in Figure 8A reveals that the existence interval of pseudo-equilibrium is an increasing function of $ \tau $. In this case, the pseudo-equilibrium $ R_0 $ is stable with respect to the sliding segment $ \Sigma_{s} $. All these results confirm that the larger $ \tau $, the more easily does the system stabilize at the pseudo-equilibrium $ R_0 $, i.e., the number of pests finally stabilizes at the $ ET $, as shown in Figures 8D and 8E. Note that for the untreated subsystem $ S_1 $, the solution may exceed the $ EIL $ resulting in a pest outbreak. However, if we choose the appropriate $ ET $ such as $ ET_i (i = 1, 2, 3, 4) $ shown in Figures 8D and 8E such that there exists the pseudo-equilibrium $ R_{0}\in \Sigma_{s} $, we can see that the number of pests is not only less than $ EIL $, but also can be stabilized at the pseudo-equilibrium $ R_0 $. Moreover, the lower is $ ET $, the lower is the number of pests.
If $ \delta_{1} > \delta $, i.e., spraying pesticides could kill natural enemies, then the effect of releasing constant $ \tau $ on the existence interval of the pseudo-equilibrium is complex and there may exist two pseudo-equilibria, as shown in the bifurcation diagrams of Figure 9A, B, C. From these we can see that the existence interval of the pseudo-equilibrium is a non-monotonic function of $ \tau $, which indicates that if pesticides can kill natural enemies, then the dynamics (particular sliding dynamics) of system (2.2) could be significantly affected, such as the stabilization level as shown in Figures 9D and 9E. It is interesting to note that although the number of pests in untreated subsystem $ S_1 $ could increase and exceed the $ EIL $, as shown in Figure 9D, the final size is less than the treated Filippov system (2.2) due to side effects of the pesticide on natural enemies. And it is easy to obtain that the side effects can be effectively avoided by increasing the releasing constant $ \tau $, as shown in Figure 9E, which can maintain the number of pests always below the $ EIL $ and realizes the control purpose.
For Case $ (C_{2}) $, it is easy to obtain that $ R^{0}_{S_{1}} $ and $ R^{0}_{S_{2}} $ always keep in region $ S_1 $, and $ R^{1}_{S_{1}} $ always lies in region $ S_{2} $. Hence, $ R^{0}_{S_{1}} $ is a regular equilibrium for subsystem $ S_{1} $, $ R^{0}_{S_{2}} $ is a virtual equilibrium for subsystem $ S_{2} $ and $ R^{1}_{S_{1}} $ is a virtual equilibrium for subsystem $ S_{1} $. Moreover, in this case, $ R^{2}_{S_{1}} $ disappears. If we choose $ \tau $ as the bifurcation parameter, then system (2.2) may have multiple positive equilibria such as $ R^{1}_{S_{2}} $ and $ R^{2}_{S_{2}} $, as parameter $ \tau $ varies. And the boundary equilibrium (BE) and pseudo-equilibrium could also appear. For example, if $ R^{1}_{S_{2}} $ collides with the switching line $ \Sigma $ as parameter $ \tau $ increases, then a BE appears, as shown in Figure 10. In particular, we have:
(ⅰ) if $ \tau^{*}_{5} < \tau < \tau^{*}_{6} $, then two virtual equilibria $ R^{1}_{S_{2}} $ and $ R^{2}_{S_{2}} $ coexist, and $ R^{1}_{S_{2}} $ collides with $ R^{2}_{S_{2}} $ at $ \tau = \tau^{*}_{6} $.
(ⅱ) if $ \tau > \tau^{*}_{6} $, $ R^{1}_{S_{2}} $ and $ R^{2}_{S_{2}} $ disappear simultaneously, and system (2.2) does not exist with any positive equilibrium.
Note that the existence and types of equilibria for non-smooth system (2.2) can be discussed by using bifurcation analyses of two subsystems with respect to key parameters (Fig. 10), which bring convenience to the analysis of local bifurcations of the Filippov system, especially those bifurcations related to equilibria. Thus, the local equilibrium bifurcations for other cases can be similarly addressed by employing similar techniques.
In this subsection, we choose $ ET $ as the bifurcation parameter to investigate the codimension-1 local and global bifurcations to analyze the effect of $ ET $ on the dynamics of system (2.2). We realize that there may exist similar local and global sliding bifurcations for Cases $ (C_{1}) $, $ (C_{2}) $ and $ (C_{3}) $, so we will take $ (C_{1}) $ as an example to discuss the possible local and global sliding bifurcations in more detail in the following.
For Case $ (C_{1}) $, if $ R_{1} < 0 $, then system (2.2) could have five equilibria $ R^{0}_{S_{1}}(0, 0) $, $ R^{1}_{S_{1}}(K, 0) $, $ R^{2}_{S_{1}}(x^{2}_{S_{1}}, y^{2}_{S_{1}}) $, $ R^{0}_{S_{2}}(0, \frac{\tau}{\delta_{1}}) $, $ R^{1}_{S_{2}}(x^{1}_{S_{2}}, y^{1}_{S_{2}}) $. In particular, if $ \delta_{1} = \delta $, then $ x^{1}_{S_{2}} < x^{2}_{S_{1}} $, i.e., $ R^{1}_{S_{2}} $ is on the left of $ R^{2}_{S_{1}} $, as shown in Figure 7A, which indicates that $ R^{1}_{S_{2}} $ and $ R^{2}_{S_{1}} $ can not be the regular equilibria at the same time and it is impossible to have limit cycles on both sides of the $ \Sigma $. If $ \delta_{1}\neq \delta $, there may exist rich bifurcations which will be addressed numerically, as shown in Figure 11. It reveals how the $ ET $ affects the dynamics of system (2.2), it is easy to see that as parameter $ ET $ varies, system (2.2) exists with boundary equilibrium bifurcations and periodic solutions which lie in region $ S_{1} $ (or $ S_{2} $). Moreover, there exist other types of periodic solutions including sliding periodic solutions, which include a piece of the sliding segment or the entire sliding segment in $ \Sigma $; crossing periodic solutions, which only include a point of the sliding segment or without any points of the sliding segment in $ \Sigma $ [17,23].
In the following part, we begin to discuss boundary equilibrium bifurcations and other types of periodic solutions though the global sliding bifurcations as parameter $ ET $ varies, as shown in Figure 11.
Touching bifurcation: A standard piece of the cycle can collide with the discontinuity boundary as parameter $ ET $ varies, this bifurcation is called a touching bifurcation (or grazing or even a sliding-grazing bifurcation) [17,23]. From Figure 11A, B and C, it is easy to see that if $ 8.45 < ET < K $, there exists a unique and stable cycle (i.e., periodic solution) in region $ S_{1} $. As parameter $ ET $ decreases, the cycle closes to $ \Sigma $. Especially, when $ ET\approx8.45 $, a touching bifurcation occurs, as shown in Figure 11B, where the cycle is tangent to $ \Sigma_{s} $ at the visible tangent point $ T_{1} $, denoted by $ X_{0}(x(t), y(t)) $. As parameter $ ET $ continues to decrease, $ X_{0}(x(t), y(t)) $ will contain a piece of the sliding segment in $ \Sigma $, becoming a sliding cycle (i.e., sliding periodic solution), as shown in Figure 11C with $ ET = 8 $. The computation of the critical cycle $ X_{0}(x(t), y(t)) $, which ends at the visible tangent point $ T_{1} $, is equivalent to solving the following boundary-value problem, which can be solved by XPPAUT software [37].
$ {˙X0(x(t),y(t))=f1(x(t),y(t)),X0(T1)=0,x(T01)=x(0)=ET,y(T01)=y(0)=rβ+(rωβ−rβK)ET−rωβKET2,⟨HX(T1),f1(T1)⟩=0, $ |
where $ T_{01} $ represents the period of the cycle $ X_{0}(x(t), y(t)) $.
Buckling bifurcation: From Figure 11C, D, we can obtain that there exists a stable sliding cycle, which passes the visible tangent point $ T_{1} $ for $ 7.25 < ET < 8.45 $. As parameter $ ET $ decreases, especially $ ET\approx7.25 $, the sliding cycle passes the invisible tangent point $ T_{2} $, denoted by $ X_{1}(x(t), y(t)) $. Moreover, it contains the entire sliding segment $ \Sigma_{s} $, which means that a buckling bifurcation occurs. As parameter $ ET $ continues to decrease, $ X_{1}(x(t), y(t)) $ remains but enters into region $ S_{2} $ before returning back to the sliding segment for $ ET > 4.9 $, as shown in Figure 11E.
Crossing bifurcation: From Figure 11E, F and G, we can see that a stable sliding cycle becomes a stable crossing cycle as parameter $ ET $ decreases. Especially, when $ ET $ reaches around 4.9, the sliding cycle only passes one point of the sliding segment $ \Sigma_{s} $ (i.e., the visible tangent point $ T_{1} $), denoted by $ X_{2}(x(t), y(t)) $. As parameter $ ET $ continues to decrease, $ X_{2}(x(t), y(t)) $ becomes a stable crossing cycle without any points of the sliding segment $ \Sigma_{s} $, as shown in Figure 11G. For the above processes, we say that a crossing bifurcation (or sliding-crossing) has occurred.
Pseudo-homoclinic bifurcation: As parameter $ ET $ decreases from 4.3 to 1.9128, the crossing bifurcation and buckling bifurcation occur again, as shown in Figure 11H, I and J. If parameter $ ET $ continues to decrease, we can see that when $ ET = 1.8998 $ (i.e., $ ET = x^{1}_{S_{2}} $), a stable sliding cycle surrounds the unstable focus $ R^{2}_{S_{1}} $ and the tangent point $ T_{2} $ collides with $ R^{1}_{S_{2}} $ simultaneously. As parameter $ ET $ reaches around 1.899, the stable sliding cycle passes the pseudo-equilibrium $ R_{0} $, which is a pseudo-saddle, forming a homoclinic orbit which contains a piece of sliding segment $ \Sigma_{s} $. In this case, we say that a pseudo-homoclinic bifurcation [17,23] occurs and there are limit cycles on both sides of the $ \Sigma $, as shown in Figure 11L. In fact, when $ ET = x^{1}_{S_{2}} $, three points $ T_{2} $, $ R^{1}_{S_{2}} $ and pseudo-equilibrium $ R_{0} $ can collide together. As parameter $ ET $ continues to decrease, the three points $ T_{2} $, $ R^{1}_{S_{2}} $ and $ R_{0} $ coexist, and there exists a stable sliding cycle surrounding the unstable equilibrium $ R^{1}_{S_{2}} $, as shown in Figure 11M. It follows from Figure 11(J-M) that a boundary equilibrium bifurcation (or the pseudo-equilibrium bifurcation) occurs at $ ET = 1.8998 $ (i.e., $ ET = x^{1}_{S_{2}} $). This bifurcation entails the catastrophic disappearance of a stable sliding cycle and a unstable pseudo-equilibrium $ R_{0} $.
Based on the above discussion, we can conclude that when we choose $ ET $ as the bifurcation parameter and fix all other parameters of system (2.2), there exist rich local and global sliding bifurcations as follows: touching $ \rightarrow $ buckling $ \rightarrow $ crossing $ \rightarrow $ crossing $ \rightarrow $ buckling $ \rightarrow $ pseudo-homoclinic $ \rightarrow $ boundary equilibrium bifurcations. It is interesting to note that it will cause the change of the regular/virtual equilibria, pseudo-equilibria and trajectories of system (2.2) as $ ET $ varies, as shown in Figures 11 and 12. In particular, the results shown in Figure 12 reveal the importance of choosing the threshold level $ ET $. Comparing with the two trajectories of treated and untreated systems shown in Figure 12, we conclude that for a given ET, the pest population can be successfully controlled and maintained below the EIL if the IPM is properly designed.
Based on the implementation of biological control in real life, we propose a new non-smooth Filippov system (2.2) with constant releasing rate in this paper. The detailed qualitative analyses for subsystem $ S_{2} $ were carried out first, which will be crucial for analyzing Filippov system (2.2). Our main results reveal that the releasing constant $ \tau $ plays an important role in determining the dynamics and bifurcations of subsystem $ S_{2} $. For example, the threshold conditions for the existence and stability of equilibria and the type of bifurcations including Hopf bifurcation, transcritical bifurcation, saddle-node bifurcation and Bogdanov-Takens bifurcation can be significantly affected by the releasing constant. Moreover, from a pest control point of view there exists a critical releasing rate such that the pest population will be driven to extinction when the releasing rate is greater than the critical value. Meanwhile, compared with the main results obtained in literature [31], we conclude that the sign of the constant $ \tau $ can significantly affect the dynamical behaviour.
Combining the dynamics of two subsystems $ S_1 $ and $ S_2 $, and employing the techniques for a non-smooth Filippov system, we focus on some typical cases on system (2.2) with aims to address how the threshold level $ ET $ affects the sliding dynamics and pest control [17,24]. The existence and stability of the sliding mode and pseudo-equilibria have been discussed first, and our results indicate that the releasing constant and side effects of the pesticide on natural enemies could result in multiple pseudo-equilibria. Especially, the existence interval of pseudo-equilibria can be greatly influenced by the threshold level $ ET $ and releasing constant $ \tau $, as shown in Figures 8 and 9. It is interesting to note that although the number of pests in the untreated subsystem could increase and exceed the $ EIL $, the stabilization level could be less than $ ET $ and stabilizes at a lower level than the treated subsystem (i.e., one of stable states of Filippov system (2.2)) due to side effects of the pesticide on natural enemies. In this case, the paradoxical effects of the Volterra principle occur, i.e., spraying pesticide does not reduce the number of pests, but increases them. However, the side effects can be effectively avoided by increasing the releasing constant, which can maintain the number of pests always below the $ EIL $ and realizes the control purpose. Furthermore, by numerical bifurcation analyses, the sliding bifurcations including boundary equilibrium bifurcation, touching bifurcation, buckling bifurcation, crossing bifurcation, pseudo-homoclinic bifurcation have been discussed as the threshold level $ ET $ varies, which indicates that the pest population can be successfully controlled and maintained below the $ EIL $ if the IPM strategy is properly designed, as shown in Figure 12.
In summary, the new model presented in this paper not only has new dynamical behaviour, but also adopts and develops new qualitative techniques. Moreover, the new dynamical behaviour presented in the model has clear practical significance which can help to guide pest control. Some new non-smooth systems involving the development of pest resistance [38,39] and adaptive control strategy [40,41] will be developed in later research and will be studied in detail.
The authors thank Prof Robert A. Cheke for his kind help and comments, thank the anonymous referees and the editor for their helpful comments and valuable suggestions. This work is supported by the National Natural Science Foundation of China (NSFCs, 61772017, 11631012, 11601301), and by the Fundamental Research Funds For the Central Universities (2018CBLZ001, GK201901008, GK201803006).
The authors declare there is no conflict of interest.
[1] |
Stedman RL (1968) Chemical composition of tobacco and tobacco smoke. Chem Rev 68: 153-207 doi: 10.1021/cr60252a002
![]() |
[2] | Smith CJ, Perfetti TA, Garg R, et al. (2003) IARC carcinogens reported in cigarette mainstream smoke and their calculated log P values. Food Chem Toxicol 41: 807-817 |
[3] |
Dallüge J, van Stee LLP, Xu X, Williams J, et al. (2002) Unravelling the composition of very complex samples by comprehensive gas chromatography coupled to time-of-flight mass spectrometry: Cigarette smoke. J Chromatogr A 974: 169-184 doi: 10.1016/S0021-9673(02)01384-5
![]() |
[4] |
Takanami Y, Chida M, Hasebe H, et al. (2003) Analysis of Cigarette Smoke by an Online Thermal Desorption System and Multidimensional GC-MS. J Chromatogr Sci 41: 317-322 doi: 10.1093/chromsci/41.6.317
![]() |
[5] | Borgerding M, Klus H (2005) Analysis of complex mixtures -- Cigarette smoke. Exp Toxicol Pathol 57 Suppl 1: 43-73 |
[6] |
Charles SM, Batterman SA, Jia C (2007) Composition and emissions of VOCs in main- and side-stream smoke of research cigarettes. Atmos Environ 41: 5371-5384 doi: 10.1016/j.atmosenv.2007.02.020
![]() |
[7] | Adam T, Mitschke S, Baker RR (2009) Investigation of tobacco pyrolysis gases and puff-by-puff resolved cigarette smoke by single photon ionisation (SPI)-time-of-flight mass spectrometry (TOFMS). Beitr Zur Tab Tob Res 23: 203-226 |
[8] |
Wang J, Weng J-J, Jia L-Y, et al. (2012) Study on Gas Phase Components in Mainstream Cigarette Smoke by Synchrotron Radiation Photoionization Mass Spectrometry. Chin J Anal Chem 40: 1048-1052 doi: 10.1016/S1872-2040(11)60559-8
![]() |
[9] |
Counts ME, Hsu FS, Laffoon SW, et al. (2004) Mainstream smoke constituent yields and predicting relationships from a worldwide market sample of cigarette brands: ISO smoking conditions. Regul Toxicol Pharmacol 39: 111-134 doi: 10.1016/j.yrtph.2003.12.005
![]() |
[10] |
Counts ME, Morton MJ, Laffoon SW, et al. (2005) Smoke composition and predicting relationships for international commercial cigarettes smoked with three machine-smoking conditions. Regul Toxicol Pharmacol 41: 185-227 doi: 10.1016/j.yrtph.2004.12.002
![]() |
[11] |
Castro D, Slezakova K, Delerue-Matos C, et al. (2011) Polycyclic aromatic hydrocarbons in gas and particulate phases of indoor environments influenced by tobacco smoke: Levels, phase distributions, and health risks. Atmos Environ 45: 1799-1808 doi: 10.1016/j.atmosenv.2011.01.018
![]() |
[12] |
Li M, Dong J-G, Huang Z-X, et al. (2012) Analysis of Cigarette Smoke Aerosol by Single Particle Aerosol Mass Spectrometer. Chin J Anal Chem 40: 936-939 doi: 10.1016/S1872-2040(11)60555-0
![]() |
[13] |
Wright C (2015) Standardized methods for the regulation of cigarette-smoke constituents. Trends Anal Chem 66: 118-127 doi: 10.1016/j.trac.2014.11.011
![]() |
[14] |
Ding YS, Zhang L, Jain RB, et al. (2008) Levels of Tobacco-Specific Nitrosamines and Polycyclic Aromatic Hydrocarbons in Mainstream Smoke from Different Tobacco Varieties. Cancer Epidemiol Biomarkers Prev 17: 3366-3371 doi: 10.1158/1055-9965.EPI-08-0320
![]() |
[15] |
Ding YS, Trommel JS, Yan XJ, et al. (2005) Determination of 14 Polycyclic Aromatic Hydrocarbons in Mainstream Smoke from Domestic Cigarettes. Environ Sci Technol 39: 471-478 doi: 10.1021/es048690k
![]() |
[16] |
Lu X, Cai J, Kong H, et al. (2003) Analysis of Cigarette Smoke Condensates by Comprehensive Two-Dimensional Gas Chromatography/Time-of-Flight Mass Spectrometry I Acidic Fraction. Anal Chem 75: 4441-4451 doi: 10.1021/ac0264224
![]() |
[17] |
Lu X, Zhao M, Kong H, et al. (2004) Characterization of cigarette smoke condensates by comprehensive two-dimensional gas chromatography/time-of-flight mass spectrometry (GC×GC/TOFMS) Part 2: Basic fraction. J Sep Sci 27: 101-109 doi: 10.1002/jssc.200301659
![]() |
[18] |
Brokl M, Bishop L, Wright CG, et al. (2013) Analysis of mainstream tobacco smoke particulate phase using comprehensive two-dimensional gas chromatography time-of-flight mass spectrometry. J Sep Sci 36: 1037-1044 doi: 10.1002/jssc.201200812
![]() |
[19] |
Hughey CA, Rodgers RP, Marshall AG (2002) Resolution of 11000 Compositionally Distinct Components in a Single Electrospray Ionization Fourier Transform Ion Cyclotron Resonance Mass Spectrum of Crude Oil. Anal Chem 74: 4145-4149 doi: 10.1021/ac020146b
![]() |
[20] | Rodgers RP, Schaub TM, Marshall AG (2005) Petroleomics: MS Returns to Its Roots. Anal Chem 77: 20-A |
[21] |
Hertkorn N, Ruecker C, Meringer M, et al. (2007) High-precision frequency measurements: indispensable tools at the core of the molecular-level analysis of complex systems. Anal Bioanal Chem 389: 1311-1327 doi: 10.1007/s00216-007-1577-4
![]() |
[22] |
Kujawinski EB (2002) Electrospray Ionization Fourier Transform Ion Cyclotron Resonance Mass Spectrometry (ESI FT-ICR MS): Characterization of Complex Environmental Mixtures. Environ Forensics 3: 207-216 doi: 10.1080/713848382
![]() |
[23] |
Schmitt-Kopplin P, Gelencsér A, Dabek-Zlotorzynska E, et al. (2010) Analysis of the Unresolved Organic Fraction in Atmospheric Aerosols with Ultrahigh-Resolution Mass Spectrometry and Nuclear Magnetic Resonance Spectroscopy: Organosulfates As Photochemical Smog Constituents. Anal Chem 82: 8017-8026 doi: 10.1021/ac101444r
![]() |
[24] |
Gonsior M, Zwartjes M, Cooper WJ, et al. (2011) Molecular characterization of effluent organic matter identified by ultrahigh resolution mass spectrometry. Water Res 45: 2943-2953 doi: 10.1016/j.watres.2011.03.016
![]() |
[25] |
Cottrell BA, Gonsior M, Isabelle LM, et al. (2013) A regional study of the seasonal variation in the molecular composition of rainwater. Atmos Environ 77: 588-597 doi: 10.1016/j.atmosenv.2013.05.027
![]() |
[26] |
Cortés-Francisco N, Harir M, Lucio M, et al. (2014) High-field FT-ICR mass spectrometry and NMR spectroscopy to characterize DOM removal through a nanofiltration pilot plant. Water Res 67: 154-165 doi: 10.1016/j.watres.2014.08.046
![]() |
[27] |
Gonsior M, Schmitt-Kopplin P, Stavklint H, et al. (2014) Changes in Dissolved Organic Matter during the Treatment Processes of a Drinking Water Plant in Sweden and Formation of Previously Unknown Disinfection Byproducts. Environ Sci Technol 48: 12714-12722 doi: 10.1021/es504349p
![]() |
[28] |
Schramm S, Carré V, Scheffler J-L, et al. (2011) Analysis of Mainstream and Sidestream Cigarette Smoke Particulate Matter by Laser Desorption Mass Spectrometry. Anal Chem 83: 133-142 doi: 10.1021/ac1019842
![]() |
[29] |
Schramm S, Carré V, Scheffler J-L, et al. (2014) Active and passive smoking - New insights on the molecular composition of different cigarette smoke aerosols by LDI-FTICRMS. Atmos Environ 92: 411-420 doi: 10.1016/j.atmosenv.2014.04.052
![]() |
[30] |
Schäfer M, Drayß M, Springer A, et al. (2007) Radical Cations in Electrospray Mass Spectrometry: Formation of Open-Shell Species, Examination of the Fragmentation Behaviour in ESI-MSn and Reaction Mechanism Studies by Detection of Transient Radical Cations. Eur J Org Chem 2007: 5162-5174 doi: 10.1002/ejoc.200700199
![]() |
[31] |
Cole DP, Smith EA, Dalluge D, et al. (2013) Molecular characterization of nitrogen-containing species in switchgrass bio-oils at various harvest times. Fuel 111: 718-726 doi: 10.1016/j.fuel.2013.04.064
![]() |
[32] |
Sudasinghe N, Dungan B, Lammers P, et al. (2014) High resolution FT-ICR mass spectral analysis of bio-oil and residual water soluble organics produced by hydrothermal liquefaction of the marine microalga Nannochloropsis salina. Fuel 119: 47-56 doi: 10.1016/j.fuel.2013.11.019
![]() |
[33] |
Baker RR (1987) A review of pyrolysis studies to unravel reaction steps in burning tobacco. J Anal Appl Pyrolysis 11: 555-573 doi: 10.1016/0165-2370(87)85054-4
![]() |
[34] |
Wu Z, Rodgers RP, Marshall AG (2004) Two- and Three-Dimensional van Krevelen Diagrams: A Graphical Analysis Complementary to the Kendrick Mass Plot for Sorting Elemental Compositions of Complex Organic Mixtures Based on Ultrahigh-Resolution Broadband Fourier Transform Ion Cyclotron Resonance Mass Measurements. Anal Chem 76: 2511-2516 doi: 10.1021/ac0355449
![]() |
[35] |
Cho Y, Ahmed A, Islam A, et al. (2015) Developments in FT-ICR MS instrumentation, ionization techniques, and data interpretation methods for petroleomics. Mass Spectrom Rev 34: 248-263 doi: 10.1002/mas.21438
![]() |
[36] |
Baker RR, Bishop LJ (2004) The pyrolysis of tobacco ingredients. J Anal Appl Pyrolysis 71: 223-311 doi: 10.1016/S0165-2370(03)00090-1
![]() |
[37] |
Mullen CA, Boateng AA (2008) Chemical Composition of Bio-oils Produced by Fast Pyrolysis of Two Energy Crops†. Energy Fuels 22: 2104-2109 doi: 10.1021/ef700776w
![]() |
[38] |
Olcese R, Carré V, Aubriet F, et al. (2013) Selectivity of Bio-oils Catalytic Hydrotreatment Assessed by Petroleomic and GC*GC/MS-FID Analysis. Energy Fuels 27: 2135-2145 doi: 10.1021/ef302145g
![]() |
[39] |
Li S, Olegario RM, Banyasz JL, et al. (2003) Gas chromatography-mass spectrometry analysis of polycyclic aromatic hydrocarbons in single puff of cigarette smoke. J Anal Appl Pyrolysis 66: 155-163 doi: 10.1016/S0165-2370(02)00111-0
![]() |
[40] |
Carré V, Aubriet F, Muller J-F (2005) Analysis of cigarette smoke by laser desorption mass spectrometry. Anal Chim Acta 540: 257-268 doi: 10.1016/j.aca.2005.03.034
![]() |
[41] |
Aubriet F, Carré V (2010) Potential of laser mass spectrometry for the analysis of environmental dust particles—A review. Anal Chim Acta 659: 34-54 doi: 10.1016/j.aca.2009.11.047
![]() |
[42] |
Cho Y, Witt M, Kim YH, et al. (2012) Characterization of Crude Oils at the Molecular Level by Use of Laser Desorption Ionization Fourier-Transform Ion Cyclotron Resonance Mass Spectrometry. Anal Chem 84: 8587-8594 doi: 10.1021/ac301615m
![]() |
[43] |
Cho Y, Jin JM, Witt M, et al. (2013) Comparing Laser Desorption Ionization and Atmospheric Pressure Photoionization Coupled to Fourier Transform Ion Cyclotron Resonance Mass Spectrometry To Characterize Shale Oils at the Molecular Level. Energy Fuels 27: 1830-1837 doi: 10.1021/ef3015662
![]() |
[44] |
Le Brech Y, Delmotte L, Raya J, et al. (2015) High Resolution Solid State 2D NMR Analysis of Biomass and Biochar. Anal Chem 87: 843-847 doi: 10.1021/ac504237c
![]() |
1. | Ayman A. Arafa, Soliman A.A. Hamdallah, Sanyi Tang, Yong Xu, Gamal M. Mahmoud, Dynamics Analysis of a Filippov Pest Control Model with Time Delay, 2021, 10075704, 105865, 10.1016/j.cnsns.2021.105865 | |
2. | Hao Zhou, Biao Tang, Huaiping Zhu, Sanyi Tang, Bifurcation and Dynamic Analyses of Non-monotonic Predator–Prey System with Constant Releasing Rate of Predators, 2022, 21, 1575-5460, 10.1007/s12346-021-00539-w | |
3. | Christian Cortés García, Bifurcations in discontinuous mathematical models with control strategy for a species, 2021, 19, 1551-0018, 1536, 10.3934/mbe.2022071 | |
4. | Xiang Hou, Bing Liu, Yilin Wang, Zhong Zhao, Complex dynamics in a Filippov pest control model with group defense, 2022, 15, 1793-5245, 10.1142/S179352452250053X | |
5. | Yunxiang Lu, Min Xiao, Jinling Liang, Jie Ding, Ying Zhou, Youhong Wan, Chunxia Fan, Hybrid Control Synthesis for Turing Instability and Hopf Bifurcation of Marine Planktonic Ecosystems With Diffusion, 2021, 9, 2169-3536, 111326, 10.1109/ACCESS.2021.3103446 | |
6. | Christian Cortés García, Bifurcations on a discontinuous Leslie–Grower model with harvesting and alternative food for predators and Holling II functional response, 2023, 116, 10075704, 106800, 10.1016/j.cnsns.2022.106800 | |
7. | Igor Belykh, Rachel Kuske, Maurizio Porfiri, David J. W. Simpson, Beyond the Bristol book: Advances and perspectives in non-smooth dynamics and applications, 2023, 33, 1054-1500, 010402, 10.1063/5.0138169 | |
8. | Hui Wang, Youping Yang, Dynamics analysis of a non-smooth Filippov pest-natural enemy system with time delay, 2023, 0924-090X, 10.1007/s11071-023-08332-x | |
9. | Yi Yang, Lirong Liu, Changcheng Xiang, Wenjie Qin, Switching dynamics analysis of forest-pest model describing effects of external periodic disturbance, 2020, 17, 1551-0018, 4328, 10.3934/mbe.2020239 | |
10. | Hao Zhou, Sanyi Tang, Bifurcation dynamics on the sliding vector field of a Filippov ecological system, 2022, 424, 00963003, 127052, 10.1016/j.amc.2022.127052 | |
11. | Jingna Liu, Qi Qi, Bing Liu, Shujing Gao, Pest control switching models with instantaneous and non-instantaneous impulsive effects, 2023, 205, 03784754, 926, 10.1016/j.matcom.2022.10.027 | |
12. | Soliman A. A. Hamdallah, Ayman A. Arafa, Stability analysis of Filippov prey–predator model with fear effect and prey refuge, 2024, 70, 1598-5865, 73, 10.1007/s12190-023-01934-z | |
13. | Wenxiu Li, Bifurcation analysis of a Filippov predator–prey model with two thresholds, 2024, 112, 0924-090X, 9639, 10.1007/s11071-024-09527-6 |