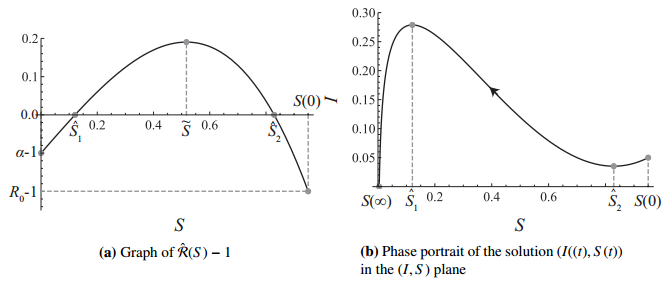
Citation: Sima Fallah Arzpeyma, Parisa Hajir, Pooya Bahari Khorram. The relationship between facet tropism and lumbar disc herniation in patients with low back pain[J]. AIMS Medical Science, 2019, 6(3): 181-190. doi: 10.3934/medsci.2019.3.181
[1] | Yukun Tan, Durward Cator III, Martial Ndeffo-Mbah, Ulisses Braga-Neto . A stochastic metapopulation state-space approach to modeling and estimating COVID-19 spread. Mathematical Biosciences and Engineering, 2021, 18(6): 7685-7710. doi: 10.3934/mbe.2021381 |
[2] | Damilola Olabode, Jordan Culp, Allison Fisher, Angela Tower, Dylan Hull-Nye, Xueying Wang . Deterministic and stochastic models for the epidemic dynamics of COVID-19 in Wuhan, China. Mathematical Biosciences and Engineering, 2021, 18(1): 950-967. doi: 10.3934/mbe.2021050 |
[3] | Jiying Ma, Wei Lin . Dynamics of a stochastic COVID-19 epidemic model considering asymptomatic and isolated infected individuals. Mathematical Biosciences and Engineering, 2022, 19(5): 5169-5189. doi: 10.3934/mbe.2022242 |
[4] | Jiangbo Hao, Lirong Huang, Maoxing Liu, Yangjun Ma . Analysis of the COVID-19 model with self-protection and isolation measures affected by the environment. Mathematical Biosciences and Engineering, 2024, 21(4): 4835-4852. doi: 10.3934/mbe.2024213 |
[5] | Haiyan Wang, Nao Yamamoto . Using a partial differential equation with Google Mobility data to predict COVID-19 in Arizona. Mathematical Biosciences and Engineering, 2020, 17(5): 4891-4904. doi: 10.3934/mbe.2020266 |
[6] | Fang Wang, Lianying Cao, Xiaoji Song . Mathematical modeling of mutated COVID-19 transmission with quarantine, isolation and vaccination. Mathematical Biosciences and Engineering, 2022, 19(8): 8035-8056. doi: 10.3934/mbe.2022376 |
[7] | Akhil Kumar Srivastav, Pankaj Kumar Tiwari, Prashant K Srivastava, Mini Ghosh, Yun Kang . A mathematical model for the impacts of face mask, hospitalization and quarantine on the dynamics of COVID-19 in India: deterministic vs. stochastic. Mathematical Biosciences and Engineering, 2021, 18(1): 182-213. doi: 10.3934/mbe.2021010 |
[8] | Tianfang Hou, Guijie Lan, Sanling Yuan, Tonghua Zhang . Threshold dynamics of a stochastic SIHR epidemic model of COVID-19 with general population-size dependent contact rate. Mathematical Biosciences and Engineering, 2022, 19(4): 4217-4236. doi: 10.3934/mbe.2022195 |
[9] | Pannathon Kreabkhontho, Watchara Teparos, Thitiya Theparod . Potential for eliminating COVID-19 in Thailand through third-dose vaccination: A modeling approach. Mathematical Biosciences and Engineering, 2024, 21(8): 6807-6828. doi: 10.3934/mbe.2024298 |
[10] | Weike Zhou, Aili Wang, Fan Xia, Yanni Xiao, Sanyi Tang . Effects of media reporting on mitigating spread of COVID-19 in the early phase of the outbreak. Mathematical Biosciences and Engineering, 2020, 17(3): 2693-2707. doi: 10.3934/mbe.2020147 |
Modelling is often used to understand the transmission dynamics of infectious diseases. The susceptible-infective-removed (SIR) model is known to be the simplest model to describe these transmission dynamics[1,10]. The SIR model describes the transmission of pathogens from infected to susceptible individuals and the removal of infected individuals from the targeted host population due to the establishment of immunity or the host's death or immigration. Due to the wide variation in the natural history of pathogens, many extensions of the basic SIR model have been proposed so far.
An important extension is the inclusion of the evolution of susceptibility to an infection along with a pathogen. The basic SIR model assumes that the host immunity perfectly protects the host from reinfection over time and reinfection can never occur. However, reinfection events are frequently observed for many infectious diseases such as tuberculosis[31] and those caused by coronavirus[20], respiratory syncytial virus[15] and hepatitis C virus[30]. One of the important mechanisms of reinfection is waning immunity. Decreased herd immunity by waning immunity of individuals induces re-emergence of an epidemic, and boosting immunity by re-vaccination is required to control the epidemic[4]. Another mechanism is imperfection of immunity by an infection event. A booster dose of a vaccine is required to establish a high enough immunity level to protect hosts from reinfection[29], which implies that multiple exposures to the pathogen is required to establish the high enough immunity level. Moreover, the enhancement of susceptibility to reinfection is also observed for several infectious diseases[32,24].
An epidemic model incorporating variable susceptibility of recovered individuals was formulated by Kermack and McKendrick[22,23]. However, the authors did not obtain a clear biological conclusion[8,18]. In[17,18] the author performed stability analysis for the Kermack and McKendrick's reinfection model formulated as a system of partial differential equations. The existence and bifurcation of the endemic equilibrium was analyzed in detail. Destabilization of the endemic equilibrium was shown to be possible for epidemic models with waning immunity[9,16,28]. Previous modeling studies showed that waning and natural-boosting immunity by exposing to pathogens can trigger a counter-intuitive effect of vaccination[27]. It is suggested that waning immunity in vaccinated hosts can trigger backward bifurcation of the endemic equilibrium[2,6,26]. Estimating vaccine effectiveness is essential to control epidemics. However, vaccine effectiveness reflects the complicated epidemiological dynamics which is scaled by waning and natural-boosting immunity, e.g., waning and boosting of immunity can induce periodic epidemic outbreaks as part of the long-term behavior of the epidemic[3].
Unlike the long-term behavior of an epidemic, its short-term behavior with waning and boosting of immunity is not well understood despite many field data of short-term epidemics have been analyzed using models that do not consider waning and boosting of immunity. For the short-term behavior of epidemics, the dynamics with the constant immune protection rate against reinfection has been studied so far. However, waning and boosting of immunity change the immune protection rate. In[21] the author analyzed transient dynamics of a reinfection epidemic model, while ignoring the demographic process in the model. In the model, reinfection of recovered individuals occurs assuming that these individuals have suitable susceptibility to the disease. It was shown that the disease transmission dynamics changes qualitatively when the basic reproduction number crosses the reinfection threshold. See also[13] for the model with demographic process.
This paper presents a mathematical model taking into account waning and enhancing susceptibility following an infection. We suppose that reinfection can reduce the immune protection level, e.g., as it is the case in antibody-dependent enhancement[5].
The timescale of natural-waning immunity, which is not due to reinfection, is relatively longer than that of the transmission dynamics e.g., the minimum annual waning rate of immunity is $ -2.9\% $ for rubella and $ -1.6\% $ for measles[25], compared to the infectious periods of $ 11 $ days for rubella[11] and $ 14 $ days for measles[1]. Hence the proposed model focuses only on waning and enhancing susceptibility triggered following an infection.
Since the waning and boosting of immunity can induce periodic infection outbreak on the long-term disease transmission dynamics ([3]), a complicated epidemic curve can be observed in the model for the short-term disease transmission dynamics as well. In this paper, an explicit solution for a number of infective individuals in terms of a number of susceptible individuals is obtained. Consequently, the impact of waning and enhancing susceptibility following an infection on the short-term behavior of the transmission dynamics is explored. The shape of the short-term epidemic curve is analyzed in detail.
The paper is organized as follows. In Section 2 an epidemic model taking into account waning and enhancing susceptibility following an infection is formulated as a nonlinear system of differential equations. The model includes the standard SIR epidemic model and the reinfection epidemic model studied in[21] as special cases. Section 3 studies the disease transmission dynamics when the basic reproduction number $ \mathcal{R}_{0} $ exceeds one. The epidemic peak is shown to be one, as is the case for the standard SIR epidemic model. Section 4 considers the disease transmission dynamics when $ \mathcal{R}_{0}\leq1 $. It demonstrates that the epidemic occurs even if $ \mathcal{R}_{0}\leq1 $ due to the enhancement of susceptibility of recovered individuals. The shape of the epidemic curve is analyzed in detail. In Section 5, the relatiton of final epidemic size with the model parameters is derived from the obtained explicit solutions. Plans for future work are discussed in Section 6.
First of all let us introduce the epidemic model studied in[21]. In the model it is assumed that the infectious disease induces partial immunity. Denote by $ S(t), \ I(t) $ and $ R(t) $ the proportions of susceptible population, infective population and recovered population at time $ t $, respectively. The partial immunity model is formulated as
$ S′(t)=−βS(t)I(t), $
|
(2.1a) |
$ I′(t)=βS(t)I(t)+βσR(t)I(t)−γI(t), $
|
(2.1b) |
$ R′(t)=γI(t)−βσR(t)I(t). $
|
(2.1c) |
The positive parameters $ \beta $ and $ \gamma $ are the transmission coefficient and the recovery rate, respectively. The parameter $ \sigma $ is the relative susceptibility of recovered individuals, who have been infected at least once and have recovered from the infection. We obtain the standard SIR epidemic model, if $ \sigma = 0 $, i.e., recovered individuals are completely protected from the infection.
In this paper the partial immunity model (2.1) is modified as follows. When a recovered individual is exposed to the force of infection, immunity is boosted with probability $ 1-\alpha $ so that one obtains permanent immunity to the disease, while one contracts the disease again with probability $ \alpha $. The partial immunity model (2.1) is modified as
$ S′(t)=−βS(t)I(t), $
|
(2.2a) |
$ I′(t)=βS(t)I(t)−γI(t)+βσαI(t)R(t), $
|
(2.2b) |
$ R′(t)=γI(t)−βσI(t)R(t), $
|
(2.2c) |
$ B′(t)=βσ(1−α)I(t)R(t) $
|
(2.2d) |
with the following initial conditions
$ S(0)=S0>0, I(0)=I0>0, R(0)=R0≥0, B(0)=B0≥0,S0+I0+R0+B0=1. $
|
Here $ B(t) $ denotes the proportion of population with permanent immunity at time $ t $. We obtain the model (2.1) by $ \alpha = 1 $ and the SIR model by $ \alpha = 0 $. Throughout the paper, we assume the following two conditions
$ 0<σ, $
|
(2.3) |
$ 0<α<1. $
|
(2.4) |
We first prove a basic result for asymptotic behavior of the solution.
Lemma 1. There exist $ \lim_{t\to\infty}S(t), \lim_{t\to\infty}I(t), \lim_{t\to\infty}R(t) $ and $ \lim_{t\to\infty}B(t) $. It holds that
$ limt→∞I(t)=0. $
|
(2.5) |
Proof. One easily sees that $ S, \ R $ and $ B $ are respectively monotone bounded functions. Specifically $ S $ is a monotone decreasing function, while $ B $ is a monotone increasing function. Therefore, $ S, \ R $ and $ B $ tend to some constants. Since $ S(t)+I(t)+R(t)+B(t) = 1 $ holds for $ t\geq0 $, $ \lim_{t\to\infty}I(t) $ also exists. We now claim that (2.5) holds. From (2.2a), (2.2b) and (2.2c) one has
$ S^{\prime}(t)+I^{\prime}(t)+\alpha R^{\prime}(t) = -\gamma\left(1-\alpha\right)I(t). $ |
Suppose that $ \lim_{t\to\infty}I(t) > 0 $. Integrating the above equation, we derive a contradiction. Hence (2.5) holds.
We define the basic reproduction number by
$ \mathcal{R}_{0}: = b\left(S_{0}+\alpha\sigma R_{0}\right), $ |
where $ b = \frac{\beta}{\gamma} $. The basic reproduction number is the expected number of secondary cases produced by one infective individual in the expected one infectious period, $ \frac{1}{\gamma} $ in the initial phase of epidemic. Noting that both susceptible and recovered populations, which compose the initial host population, have susceptibility to the disease, we may call $ \mathcal{R}_{0} $ the basic reproduction number, although $ \mathcal{R}_{0} $ is conventionally called the effective reproduction number[19].
From (2.2a) and (2.2b) one obtains the following
$ dIdS=−1bS(R(S,R)−1) $
|
(3.1) |
and
$ dI(t)dt=γI(t)(R(S(t),R(t))−1), $
|
(3.2) |
where
$ \mathcal{R}(S,R): = b\left(S+\alpha\sigma R\right),\ S\geq0,\ R\geq0. $ |
Noting that $ \mathcal{R}\left(S_{0}, R_{0}\right) = \mathcal{R}_{0} $, it is easy to see that
$ R0>1⇔I′(0)>0,R0=1⇔I′(0)=0,R0<1⇔I′(0)<0, $
|
i.e., if $ \mathcal{R}_{0} > 1 $ then the epidemic curve initially grows, while if $ \mathcal{R}_{0} < 1 $ then the epidemic curve initially decays.
First we show that $ R(t) $ can be expressed in terms of $ S(t) $.
Proposition 1. It holds that
$ R(t)=1σb(1−(1−σbR0)(S(t)S0)σ),t≥0. $
|
(3.3) |
Proof. Assume that $ 1-\sigma bR_{0}\not = 0 $ holds. From the equations (2.2a) and (2.2c) we have
$ dRdS=−1−σbRbS. $
|
(3.4) |
Using the separation of variables, we obtain
$ (S(t)S0)σ=1−σbR(t)1−σbR0, $
|
(3.5) |
thus (3.3) follows. It is easy to see that the equality in (3.3) also holds, if $ 1-\sigma bR_{0} = 0 $. From Lemma 1 we have
$ R(t)=r(S(t)), $
|
(3.6) |
where
$ r(S):=1σb(1−(1−σbR0)(SS0)σ), 0≤S≤S0. $
|
(3.7) |
Then we can show that $ I(t) $ and $ B(t) $ are explicitly expressed in terms of $ S(t) $ and $ R(t) $.
Proposition 2. It holds that
$ I(t)=I0+(S0−S(t))+(1−α)1bln(S(t)S0)+α(R0−R(t)), $
|
(3.8) |
$ B(t)=B0+(1−α){−1bln(S(t)S0)+(R0−R(t))} $
|
(3.9) |
for $ t\geq0 $.
Proof. From (2.2a) and (2.2b) we have
$ dIdS=−1+1bS−ασRS. $
|
(3.10) |
One can compute, using (3.6) and (3.7),
$ ∫σRSdS=σ∫1S[1σb(1−(1−σbR0)(SS0)σ)]dS=1b[∫1SdS−(1−σbR0)∫(SS0)σ1SdS]. $
|
From (3.5) and (3.4) in the proof of Lemma 1 we get
$ ∫S(t)S0(SS0)σ1SdS=−b∫R(t)R0(1−σbR1−σbR0)11−σbRdR=−b11−σbR0∫R(t)R0dR.=−b11−σbR0(R(t)−R0). $
|
Therefore, we get
$ \int_{S_{0}}^{S(t)}\frac{\sigma R}{S}dS = \frac{1}{b}\ln\left(\frac{S(t)}{S_{0}}\right)+\left(R(t)-R_{0}\right). $ |
Thus we obtain (3.8).
From (2.2a) and (2.2b) we have
$ dBdS=−(1−α)σRS. $
|
(3.11) |
Similarly, one obtains (3.9).
To analyze the epidemic curve, we study the function $ \mathcal{R}(S, R) $ with $ R = r\left(S\right) $. Let
$ \hat{\mathcal{R}}(S): = \mathcal{R}(S,r\left(S\right)). $ |
We then compute the first and second derivatives of $ \hat{\mathcal{R}} $:
$ ˆR′(S)=b(1+ασr′(S)), $
|
(3.12) |
$ ˆR′′(S)=bασr′′(S). $
|
(3.13) |
From the equation (3.4) in Lemma 1, it is easy to obtain the following result.
Lemma 2. One has
$ r′(S)=−1b(1−σbR(0))Sσ−1Sσ0, $
|
(3.14) |
$ r′′(S)=(σ−1)1Sr′(S). $
|
(3.15) |
Note that, for $ {\sigma = 1} $ or $ 1-\sigma bR_{0} = 0 $, $ r $ is a constant function. For $ {\sigma\not = 1} $ and $ 1-\sigma bR_{0}\not = 0 $, , $ r $is either monotone increasing or decreasing function, thus $ \hat{\mathcal{R}} $ has at most one extremum.
We now show the standard epidemic case if $ \mathcal{R}_{0} > 1 $ holds.
Proposition 3. Let us assume that $ \mathcal{R}_{0} > 1 $ holds. Then
$ ˆR(0)=α<1<ˆR(S0)=R0 $
|
(3.16) |
holds and there exists a unique root of
$ \hat{\mathcal{R}}(S) = 1, 0 \lt S \lt S_{0}. $ |
Proof. It is easy to see that (3.16) holds. First assume that $ r^{\prime}(S)\geq0 $ for $ 0\leq S\leq S_{0} $. Then, from (3.12), one can see that $ \hat{\mathcal{R}} $ is an increasing function. Thus we obtain the conclusion. Next assume that $ r^{\prime}(S) < 0 $ for $ 0\leq S\leq S_{0} $. By (3.13) and Lemma 2, one sees that $ \hat{\mathcal{R}} $ has at most one minimum for $ 0\leq S\leq S_{0} $. Therefore, from (3.16), we obtain the conclusion.
Then, from Proposition 3, Lemma 1 and (3.2), we obtain the following result.
Theorem 1. Let us assume that $ \mathcal{R}_{0} > 1 $ holds. Then there is a $ t_{p} > 0 $ such that $ I $ is monotonically increasing for $ t\in\left(0, t_{p}\right) $ and monotonically decreasing for $ t > t_{p} $. It holds $ \lim_{t\to\infty}I(t) = 0 $.
In the standard SIR model, when $ \mathcal{R}_{0}\leq1 $ holds, then the epidemic curve monotonically decreases and infective population tends to $ 0 $ eventually as time goes to infinity. The situation changes in the model with waning and enhancing susceptibility following infection(2.2), due to the susceptibility of the recovered individuals. In particular, if $ \sigma > 1 $ then there is a possible delayed outbreak as the recovered population increases which will induce the epidemic later even if $ \mathcal{R}_{0}\leq1 $. The basic reproduction number, which characterizes the initial dynamics, is not a sufficient criterion to determine the outbreak due to the recovered population.
First let us consider a simple case that $ \sigma\leq1 $ holds. We have the standard scenario: if $ \mathcal{R}_{0}\leq1 $ then the epidemic does not occur. Subsequently we study the disease transmission dynamics when $ \sigma > 1 $. We show that enhancement of susceptibility after the infection can induce an epidemic later.
We show that the infective population is monotonically decreasing for $ t\geq0 $, similar to the SIR model, when $ \mathcal{R}_{0}\leq1 $.
Proposition 4. Let us assume that $ \mathcal{R}_{0}\leq1 $ and $ \sigma\leq1 $ holds. Then
$ \mathcal{\hat{R}}(S)\leq1, 0\leq S\leq S_{0}. $ |
Proof. Note that
$ ˆR(0)=α<1, ˆR(S0)=R0≤1 $
|
(4.1) |
holds. First let us consider the case that $ \sigma = 1 $ or $ 1-\sigma bR_{0} = 0 $. In these cases $ \mathcal{R}(S) $ is a linear function of $ S $, thus we obtain the conclusion from (4.1). Let us assume that $ \sigma < 1 $ and $ 1-\sigma bR_{0}\not = 0 $ hold. If $ 1-\sigma bR_{0} < 0 $, then one has $ r^{\prime}(S)\geq0 $ for $ 0 < S < S_{0} $, thus $ \hat{\mathcal{R}} $ is an increasing function. We then obtain the conclusion from (4.1). Next assume that $ 1-\sigma bR_{0} > 0 $. One sees that
$ \lim\limits_{S\downarrow0}r^{\prime}(S) = -\infty\implies\lim\limits_{S\downarrow0}\hat{\mathcal{R}}^{\prime}(S) = -\infty. $ |
Then $ r^{\prime}(S) < 0 $ holds for $ 0 < S < S_{0} $. By Lemma 2, one sees that $ \hat{\mathcal{R}} $ has at most one minimum for $ 0\leq S\leq S_{0} $. Therefore we obtain the conclusion.
Then, from Propositions 4, Lemma 1 and (3.2), we obtain the following result.
Theorem 2. Let us assume that $ \mathcal{R}_{0}\leq1 $ and $ \sigma\leq1 $ hold. Then $ I $ is monotonically decreasing for $ t\geq0 $. It holds $ \lim_{t\to\infty}I(t) = 0 $.
In this subsection we consider the case that
$ R0≤1, σ>1 $
|
(4.2) |
hold. We show the following results for the graph of $ \hat{\mathcal{R}} $.
Proposition 5. Let us assume that $ \mathcal{R}_{0}\leq1 $ and $ \sigma > 1 $ hold.
1. If
$ b(S0+ασ2R0)≥ασ $
|
(4.3) |
then $ \hat{\mathcal{R}}(S)\leq1 $ for $ 0\leq S\leq S(0) $.
2. If
$ b(S0+ασ2R0)<ασ $
|
(4.4) |
then there is a unique maximum for $ 0 < S < S_{0} $ at $ S = \tilde{S} $, where
$ ˜S:=(bS0σα(1−σbR0))1σ−1S0<S0. $
|
(4.5) |
Then
(a) If $ \hat{\mathcal{R}}(\tilde{S}) > 1 $ then there are two roots for $ \hat{\mathcal{R}}(S) = 1 $ for $ 0 < S < S_{0} $. Denote the roots by $ \hat{S}_{1} $ and $ \hat{S}_{2} $ such that
$ 0 \lt \hat{S}_{1} \lt \tilde{S} \lt \hat{S}_{2} \lt S_{0}, $ |
then
$ \hat{\mathcal{R}}(S){<1,ˆS2<S≤S0,>1,ˆS1<S<ˆS2,<1,0<S<ˆS1. $
|
(b) If $ \mathit{{{\hat{\mathcal{R}}}}} (\tilde{S})\leq1 $ then $ \hat{\mathcal{R}}(S)\leq1 $ for $ 0\leq S\leq S_{0} $.
Proof. For $ \sigma > 1 $ one sees that
$ \lim\limits_{S\downarrow0}r^{\prime}(S) = 0\implies\lim\limits_{S\downarrow0}\hat{\mathcal{R}}^{\prime}(S) = b \gt 0. $ |
From the monotonicity of $ \hat{\mathcal{R}}^{\prime} $, if $ \lim_{S\uparrow S_{0}}\hat{\mathcal{R}}^{\prime}(S) > 0 $ then $ \hat{\mathcal{R}}(S)\leq1 $ for $ 0\leq S\leq S_{0} $ follows. Computing
$ limS↑S0ˆR′(S)=b(1+ασr′(S0))=1S(0)(b(S0+ασ2R0)−ασ), $
|
one can see that (4.3) is equivalent to that $ \lim_{S\uparrow S_{0}}\hat{\mathcal{R}}^{\prime}(S) > 0 $ holds.
Next assume that (4.4) holds. Then
$ \lim\limits_{S\uparrow S_{0}}\hat{\mathcal{R}}^{\prime}(S) \lt 0 \lt \lim\limits_{S\downarrow0}\hat{\mathcal{R}}^{\prime}(S). $ |
From the monotonicity of $ \hat{\mathcal{R}}^{\prime}, $ there is a unique maximum for $ 0 < S < S_{0} $. Solving $ \hat{\mathcal{R}}^{\prime}(S) = 0 $, we obtain $ \tilde{S} $ given as in (4.5). It is now straightforward to obtain the statements (a) and (b).
In Figure 1, we plot the graph of the function $ \hat{\mathcal{R}}(S)-1 $ for $ 0\leq S\leq S_{0} $, where $ \mathcal{R}_{0}\leq1 $ and $ \sigma > 1 $. Parameters are fixed so that (4.4) and $ \hat{\mathcal{R}}(\tilde{S}) > 1 $ hold.
From Proposition 5, Lemma 1 and 3.2, we first obtain the result for the extinction of the disease.
Theorem 3. Let us assume that $ \mathcal{R}_{0}\leq1 $ and $ \sigma > 1 $ holds. If either
1. (4.3) holds, or
2. (4.4) and $ \hat{\mathcal{R}}(\tilde{S})\leq1 $ hold,
then $ I $ is monotonically decreasing for $ t\geq0 $. It follows that $ \lim_{t\to\infty}I(t) = 0 $.
Now it is assumed that (4.2) holds. If
$ \hat{\mathcal{R}}(\tilde{S}) \gt 1 $ |
holds, where $ \tilde{S} $ is given by (4.5), then $ I(t) $ may attain a minimum and a maximum (see Figure 1). This implies that even if $ \mathcal{R}_{0}\le1 $, the epidemic curve may grow for a certain time interval, which we call delayed epidemic.
To determine if the delayed epidemic indeed occurs, we evaluate the minimum of $ I(t) $ using (3.8) in Proposition 2. If $ S(t) = \hat{S}_{2} $ for some $ t $, $ I(t) $ is given by $ \underline{I} $, where
$ I_:=I0+(S0−ˆS2)+(1−α)1bln(ˆS2S0)+α(R0−r(ˆS2)). $
|
(4.6) |
See also Figure 1(B) for the phase portrait in the $ (I, S) $-plane.
Theorem 4. Let us assume that $ \mathcal{R}_{0}\leq1 $ and $ \sigma > 1 $ holds. Furthermore, assume that (4.4) and
$ \hat{\mathcal{R}}(\tilde{S}) \gt 1 $ |
hold.
1. If $ \underline{I}\leq0 $, then $ I $ is monotonically decreasing for $ t\geq0 $.
2. If $ \underline{I} > 0 $, then there is an interval $ \left[t_{1}, t_{2}\right] $ such that $ I $ increases for $ t_{1}\leq t\leq t_{2} $ and decreases for $ 0\le t\leq t_{1} $ and $ t_{2}\leq t $.
It follows that $ \lim_{t\to\infty}I(t) = 0 $.
Proof. One sees that $ I $ has a local maximum and minimum with respect to $ t $ and $ S $, where $ \hat{\mathcal{R}}(S) = 1 $ holds (see (3.1) and (3.2)). $ I $ has a local minimum at $ S = \hat{S}_{2}\in\left(\tilde{S}, S_{0}\right) $ and $ I $ is increasing for $ \hat{S}_{2}\leq S\leq S_{0} $ (see Figure 1(B)). Noting that $ I(t) > 0 $ for $ t\geq0 $ and that $ S $ is a decreasing function with respect to $ t $, $ \underline{I}\leq0 $ implies that $ I $ is monotonically decreasing for $ t\geq0 $. On the other hand, if $ \underline{I} > 0 $ then $ I $ is monotonically increasing for $ S < \hat{S}_{1} $, decreasing for $ \hat{S}_{1} < S < \hat{S}_{2} $ and then increasing for $ \hat{S}_{2} < S $. There exist $ t_{1} $ and $ t_{2} $ such that $ S(t_{1}) = \hat{S}_{2} $ and $ S(t_{2}) = \hat{S}_{1} $. Thus we obtain the conclusion. From Lemma 1, it follows that $ \lim_{t\to\infty}I(t) = 0 $.
Thus the model has three different transmission dynamics: no epidemic, normal epidemic and delayed epidemic as illustrated in Figure 2. Figure 3 shows parameter regions for the three different disease transmission dynamics. The region for the delayed epidemic become larger with respect to the initial condition of $ I $ and the susceptibility $ \sigma $. Since the initial condition of $ I $ is involved in the condition of Theorem 4.3, the initial condition qualitatively changes the epidemic curve, see Figure 4: delayed epidemic is induced by a large initial condition.
Due to the nonlinearity, it may not be possible to explicitly express the condition under which a delayed epidemic occurs in terms of the the initial conditions. We here consider a special case $ \sigma = 2 $ in order to illustrate that a certain amount of the initial infectives is required for a delayed epidemic. We assume that $ R_{0} = B_{0} = 0 $ to keep the presentation simple, although one may follow the approach for $ R_{0} > 0 $ or $ B_{0} > 0 $. We let
$ x(t) = \frac{S(t)}{S_{0}},\ y(t) = \frac{1-\sigma bR(t)}{1-\sigma bR_{0}}. $ |
Then, from (3.5) in the proof of Lemma 1, we get $ y(t) = x(t)^{\sigma} $. Assume that $ \sigma = 2 $ and $ R_{0} = B_{0} = 0 $ hold. One easily sees that the condition $ \mathcal{R}_{0}\leq1 $ is equivalent to
$ bS0≤1. $
|
(4.7) |
It can be seen that $ \mathcal{\hat{R}}(S) = 1 $ has two roots if and only the following equation has two roots
$ f(x)=0, x∈[0,1], $
|
(4.8) |
where
$ f(x): = bS_{0}x-\alpha x^{2}-(1-\alpha) = 0. $ |
Note $ f(0) = -(1-\alpha) < 0 $ and $ f(1) = bS_{0}-1\leq0 $. If $ \left(bS_{0}\right)^{2} > 4\alpha\left(1-\alpha\right) $ then the equation (4.8) has two roots. Together with the condition (4.7) we now assume that
$ 2√α(1−α)<bS0≤1 $
|
(4.9) |
holds. If (4.8) holds, we have
$ bS0=αx+1−αx, $
|
(4.10) |
where we regard $ x $ as a free parameter. Under the condition (4.9), equation (4.10) has two roots in $ \left[0, 1\right] $ for $ \frac{1}{2} < \alpha < 1 $ and the larger root exists in the interval $ \left(\sqrt{\left(1-\alpha\right)/\alpha}, 1\right] $, thus we vary $ x $ in $ \left(\sqrt{\left(1-\alpha\right)/\alpha}, 1\right] $.
From (3.8) one can express $ I(t) $ in terms of $ x(t) $:
$ I(t)=1b(b(1−S0x(t))+αx(t)2−12+(1−α)lnx(t)). $
|
(4.11) |
Assume $ \underline{I} = 0 $, which is the threshold condition for a delayed epidemic. Using (4.11) and (4.10), $ b $ and $ S_{0} $ can be expressed in terms of $ x $ as
$ b=(1−α2)+12αx2−(1−α)lnx,S0=αx+1−αx(1−α2)+12αx2−(1−α)lnx $
|
for $ x\in\left(\sqrt{\left(1-\alpha\right)/\alpha}, 1\right] $. Then we can plot a parametrized curve in $ (I_{0}, b) $ plane, using $ I_{0} = 1-S_{0} $, which shows the threshold condition for a delayed epidemic, see Figure 5. In the Figure 5, we also plot two curves $ bS_{0} = b\left(1-I_{0}\right) = 1 $ (corresponding to the condition $ \mathcal{R}_{0} = 1 $) and $ bS_{0} = b\left(1-I_{0}\right) = 2\sqrt{\alpha\left(1-\alpha\right)} $. A delayed epidemic occurs in the region enclosed by the three curves, showing that delayed epidemic requires sufficiently large initial infectives. Figure 5 also shows that if $ I_{0} $ decreases, then either the condition $ \mathcal{R}_{0} > 1 $ holds (i.e., normal epidemic) or $ \underline{I}\leq0 $ holds (i.e., no epidemic), depending on the value of $ b $.
Consider a special case that $ R_{0}\to0 $. The basic reproduction number is given as $ \mathcal{R}_{0} = bS_{0} $. The conditions (4.4) becomes
$ \mathcal{R}_{0} \lt \sigma\alpha $ |
and $ \tilde{S} = \left(\frac{\mathcal{R}_{0}}{\sigma\alpha}\right)^{\frac{1}{\sigma-1}}S_{0} $. If $ \mathcal{\hat{R}}(\tilde{S}) > 1 $ holds, then the delayed epidemic may occur.
Let
$ \left(S(\infty),I(\infty),R(\infty),B(\infty)\right) = \lim\limits_{t\to\infty}\left(S(t),I(t),R(t),B(t)\right). $ |
It follows that $ I(\infty) = 0 $. From the relations (3.8), (3.3) and (3.9), one sees that $ (S(\infty), R(\infty), B(\infty)) $ satisfy the following equations
$ 0=I0+(S0−S(∞))+(1−α)1bln(S(∞)S0)+α(R0−R(∞)), $
|
(5.1) |
$ R(∞)=r(S(∞)), $
|
(5.2) |
$ B(∞)=B0+(1−α){−1bln(S(∞)S0)+(R0−R(∞))}. $
|
(5.3) |
The final epidemic size is given by $ R(\infty)+B(\infty) $, the number of individuals who are infected at least once. From (5.1) and (5.2) we get the following equation
$ 0=I0+(S0−S(∞))+(1−α)1bln(S(∞)S0)+α(R0−rS((∞))). $
|
(5.4) |
In Figure 6, we plot $ R(\infty)+B(\infty) = 1-S(\infty), \ R(\infty) $ and $ B(\infty) $ with respect to $ \mathcal{R}_{0} $.
Numerically we observe that $ R(\infty) $ is not monotone with respect to $ \mathcal{R}_{0} $. Small $ \mathcal{R}_{0} $ allows the increase of $ R(\infty) $, on the other hand, does not contribute to the increase of $ B(t) $, the outbreak ends before the transition from $ R(t) $ to $ B(t) $ via $ I(t) $ occurs among most $ R(t) $. Increase of $ \mathcal{R}_{0} $ contributes the transition from $ R(t) $ to $ B(t) $, consequently, $ R(\infty) $ decreases. Despite of non-monotnic relation of $ R(\infty) $ with respect to $ \mathcal{R}_{0} $, $ R(\infty)+B(\infty) $ is likely to increase monotonically with the increase of $ \mathcal{R}_{0} $ as shown in Figure 6.
When $ \alpha = 0 $ we obtain the standard SIR setting. Letting $ I(0)\to0 $ and $ S(0)\to1 $, the basic reproduction number is given as $ \mathcal{R}_{0} = b $. In this case, from (5.1), we obtain the well known final size relation
$ 0 = \left(1-S\left(\infty\right)\right)+\frac{1}{\mathcal{R}_{0}}\ln\left(S\left(\infty\right)\right), $ |
In this paper, we study a disease transmission dynamics model after incorporating changes in immune protection level following an infection. The proposed modelling approach describing waning and enhancement of susceptibility following an infection captures the delayed epidemics in addition to the standard transmission dynamics. A delayed epidemic shows a negative slope in the initial phase of the epidemic. Therefore, the estimation of $ \mathcal{R}_{0} $ using the initial slope of the epidemic makes it difficult to capture the actual epidemic coming later. The condition for a delayed epidemic is derived using the analytic transient solution of $ I(t) $.
A delayed epidemic, illustrated in Figures 2 and 4, occurs due to the enhancement of susceptibility of the recovered population (i.e., $ \sigma > 1 $). For example, antibody dependent enhancement can boost the viral replication within the host body. Consequently, the host susceptibility can be enhanced at the time of reinfection[33]. Theorem 4.3 formulates a condition for the delayed epidemic. One of the necessary condition for a delayed epidemic is (4.4) in Proposition 5. The condition (4.4) is necessary for the epidemic curve increase; it relates the effective susceptible population increase, which is defined as
$ L(t): = S(t)+\alpha\sigma R(t). $ |
Since it holds that
$ L′(0)=S′(0)+ασR′(0)=γI0(−bS0+ασ(1−bσR0)), $
|
one can see that
$ L′(0)>0⇔bS0<σα(1−bσR0). $
|
Therefore, the effective susceptible population increase at the initial time is necessary for a delayed epidemic and may induce the delayed epidemic even if $ \mathcal{R}_{0}\leq1 $.
We remark that $ \mathcal{R}_{0} $ cannot measure the outbreak potential of a delayed epidemic. In principle, $ \mathcal{R}_{0} $ is derived based on the linearized system of the the initial disease transmission dynamics. The linearized system at the initial phase does not provide enough information to predict a delayed epidemic. Similar phenomena can be observed in epidemic models that show backward bifurcation of the endemic equilibrium[2,6,14,19,26]. These studies show that there is a stable endemic equilibrium even if the basic reproduction number is less than unity. Unlike these models, the short-term disease transmission dynamics model has many equilibria, which are associated with the zero eigenvalue. Our study illustrates that the outbreak potential should be carefully examined in the short-term disease transmission dynamics using the transient solution.
$ \mathcal{R}_{0} $ can be estimated from the final epidemic size. It should be noted that $ \mathcal{R}_{0} $ can be overestimated if the model neglects the enhancement of susceptibility following an infection. Figure 7 shows the estimated $ \mathcal{R}_{0} $ using a fixed final epidemic size of $ 0.5 $ with varied $ \alpha $ and $ \sigma $. In this case, our model is equivalent to the standard SIR model when $ \alpha = 0 $ or $ \sigma = 0 $. If waning and boosting of immunity are introduced, i.e., $ \alpha > 0 $ or $ \sigma > 0 $, the estimated $ \mathcal{R}_{0} $ is always lower than the estimate obtained using the standard epidemic model with $ \alpha = 0 $ or $ \sigma = 0 $. To estimate the precise $ \mathcal{R}_{0} $ from the final epidemic size, an appropriate modelling with respect to waning and boosting of immunity is required.
$ \mathcal{R}_{0} $ can also be estimated from the time-series of $ I(t) $. Since $ I(t) $ in the proposed model can be expressed as the closed-form function of $ S(t) $, if $ I(t)+S(t) $ is given, e.g., $ B(t)+R(t) $ is estimated from a serological survey, $ I(t) $ can be obtained as a function of time, which can be used to estimate $ \mathcal{R}_{0} $.
The proposed mathematical model describing waning and enhancing susceptibility following an infection has a limitation. In particular, it describes a step-wise level of boosting immunity i.e., $ R $ has susceptibility $ \sigma $ to an infectious disease while $ B $ has a complete protection against reinfection. This setting is suitable for infectious diseases where multiple infections can establish drastic increase of the immunity level. On the other hand, several classes of $ R $ with varied immune protection level are required to describe gradual change of the immunity level resulted from waning and boosting of immunity.
The authors thank the reviewer for the careful reading of the manuscript. The original manuscript has been improved by the reviewer's helpful comments. The first author was supported by JSPS Grant-in-Aid for Young Scientists (B) 16K20976 of Japan Society for the Promotion of Science. The second author was supported by CREST, Japan Science and Technology Agency.
The author declares no conflicts of interest in this paper.
[1] | Katz JN (2006) Lumbar disc disorders and low-back pain: socioeconomic factors and consequences. J Bone Joint Surg Am 88: 21–24. |
[2] |
Adams MA, Roughley PJ (2006) What is intervertebral disc degeneration, and what causes it? Spine 31: 2151–2161. doi: 10.1097/01.brs.0000231761.73859.2c
![]() |
[3] | Nachemson A (1960) Lumbar intradiscal pressure: experimental studies on post-mortem material. Acta Orthop Scand 31: 1–104. |
[4] |
Atlas SJ, Chang Y, Kammann E, et al. (2000) Long-term disability and return to work among patients who have a herniated lumbar disc: the effect of disability compensation. J Bone Joint Surg Am 82: 4–15. doi: 10.2106/00004623-200001000-00002
![]() |
[5] |
Vialle LR, Vialle EN, Henao JES, et al. (2010) Hérnia discal lombar Lumbar disc herniation. Rev Bras Ortop 45: 17–22. doi: 10.1590/S0102-36162010000100004
![]() |
[6] |
Jacobsen S, Sonne-Holm S, Rovsing H, et al. (2007) Degenerative lumbar spondylolisthesis: an epidemiological perspective: the Copenhagen Osteoarthritis Study. Spine 32: 120–125. doi: 10.1097/01.brs.0000250979.12398.96
![]() |
[7] |
Tulsi RS, Hermanis GM (1993) A study of the angle of inclination and facet curvature of superior lumbar zygapophyseal facets. Spine 18: 1311–1317. doi: 10.1097/00007632-199308000-00010
![]() |
[8] |
Lee DY, Lee SH (2009) Effects of facet tropism and disk degeneration on far lateral lumbar disk herniation: comparison with posterolateral lumbar disk herniation. Neurol Med Chir (Tokyo) 49: 57–61. doi: 10.2176/nmc.49.57
![]() |
[9] |
Brailsford JF (1929) Deformities of the lumbosacral region of the spine. Br J Surger 16: 562–627. doi: 10.1002/bjs.1800166405
![]() |
[10] | Farfan H, Sullivan J (1967) The relation of facet orientation to intervertebral disc failure. Can J surg J can de chir 10: 179. |
[11] |
Kim HJ, Chun HJ, Lee HM, et al. (2013) The biomechanical influence of the facet joint orientation and the facet tropism in the lumbar spine. Spine J 13: 1301–1308. doi: 10.1016/j.spinee.2013.06.025
![]() |
[12] |
Cyron B, Hutton W (1980) Articular tropism and stability of the lumbar spine. Spine 5: 168–172. doi: 10.1097/00007632-198003000-00011
![]() |
[13] | Chadha M, Sharma G, Arora SS, et al. (2013)Association of facet tropism with lumbar disc herniation. Eur Spine J 22: 1045–1052. |
[14] |
Farfan H, Cossette J, Robertson G, et al. (1970) The effects of torsion on the lumbar intervertebral joints: the role of torsion in the production of disc degeneration. J Bone Joint Surg Am 52: 468–497. doi: 10.2106/00004623-197052030-00006
![]() |
[15] |
Noren R, Trafimow J, Andersson G, et al. (1991) The role of facet joint tropism and facet angle in disc degeneration. Spine 16: 530–532. doi: 10.1097/00007632-199105000-00008
![]() |
[16] |
Ko HY, Park BK (1997) Facet tropism in lumbar motion segments and its significance in disc herniation. Arch Phys Medicin Rehabil 78: 1211–1214. doi: 10.1016/S0003-9993(97)90334-8
![]() |
[17] |
Linov L, Klindukhov A, Li L, et al. (2013) Lumbar facet joint orientation and osteoarthritis: a cross-sectional study. J Back Musculoskeletal Rehabil 26: 421–426. doi: 10.3233/BMR-130401
![]() |
[18] |
Van JS, Verbiest H, Van FS (1985) The orientation of laminae and facet joints in the lower lumbar spine. Spine 10: 59–63. doi: 10.1097/00007632-198501000-00009
![]() |
[19] |
Ahmed AM, Duncan NA, Burke DL (1990) The effect of facet geometry on the axial torque-rotation response of lumbar motion segments. Spine 15: 391–401. doi: 10.1097/00007632-199005000-00010
![]() |
[20] |
Vanharanta H, Floyd T, Ohnmeiss DD, et al. (1993) The relationship of facet tropism to degenerative disc disease. Spine 18: 1000–1005. doi: 10.1097/00007632-199306150-00008
![]() |
[21] | Cassidy JD, Loback D, Yong-Hing K, et al. (1992) Lumbar facet joint asymmetry. Intervertebral disc herniation. Spine 17: 570–574. |
[22] |
Adams M, Hutton W (1981) The relevance of torsion to the mechanical derangement of the lumbar spine. Spine 6: 241–248. doi: 10.1097/00007632-198105000-00006
![]() |
[23] | Yu H, Hou S, Wu W, et al. (1998) The relationship of facet orientation to intervertebral disc protrusion and lateral recess stenosis in lower lumbar spine. Zhonghua wai ke za zhi 36: 176–8, 31. |
[24] | Grogan J, Nowicki BH, Schmidt TA, et al. (1997) Lumbar facet joint tropism does not accelerate degeneration of the facet joints. Am J Neuror 18: 1325–1329. |
[25] |
Gao T, Lai Q, Zhou S, et al. (2017) Correlation between facet tropism and lumbar degenerative disease: a retrospective analysis. BMC Musculoskeletal Disord 18: 483. doi: 10.1186/s12891-017-1849-x
![]() |
[26] | Long DM, BenDebba M, Torgerson WS, et al. (1996) Persistent back pain and sciatica in the United States: patient characteristics. J Spinal Disord 9: 40–58. |
[27] | Putti V (1927) New conceptions in the pathogenesis of sciatic pain. Lancet 2: 53–60. |
[28] |
Tisot RA, Vieira JDS, Collares DDS, et al. (2018) Influence of facet tropism on the location of lumbar disc herniation. Coluna/Columna 17: 23–26. doi: 10.1590/s1808-185120181701179264
![]() |
[29] | Lumbar DDD (2013) Associação entre tropismo facetário e doença degenerativa de disco lombar. |
[30] |
Liu Z, Duan Y, Rong X, et al. (2017) Variation of facet joint orientation and tropism in lumbar degenerative spondylolisthesis and disc herniation at L4–L5: A systematic review and meta-analysis. Clin Neurol Neurosurgery 161: 41–47. doi: 10.1016/j.clineuro.2017.08.005
![]() |
[31] |
Mohanty SP, Kanhangad MP, Kamath S, et al. (2018) Zygapophyseal joint orientation and facet tropism and their association with lumbar disc prolapse. Asian Spine J 12: 902–909. doi: 10.31616/asj.2018.12.5.902
![]() |
[32] |
Wang H, Zhou Y (2016) Facet tropism: possible role in the pathology of lumbar disc herniation in adolescents. J Neurosurgery Pediatr 18: 111–115. doi: 10.3171/2015.7.PEDS15175
![]() |
[33] | Pichaisak W, Chotiyarnwong C, Chotiyarnwong P (2015) Facet joint orientation and tropism in lumbar degenerative disc disease and spondylolisthesis. J Med Assoc Thai 98: 373–379. |
[34] |
Ghandhari H, Ameri E, Hasani H, et al. (2018) Is facet tropism associated with increased risk of disc herniation in the lumbar spine? Asian Spine J 12: 428. doi: 10.4184/asj.2018.12.3.428
![]() |
[35] | Kunakornsawat S, Ngamlamaidt K, Tungsiripat R, et al. (2007) The relationship of facet tropism to lumbar disc herniation. J M ed Assoc Thai 90: 1337–1341. |
[36] |
Karacan I, Aydin T, Sahin Z, et al. (2004) Facet angles in lumbar disc herniation: their relation to anthropometric features. Spine 29: 1132–1136. doi: 10.1097/00007632-200405150-00016
![]() |
[37] |
Kong MH, He W, Tsai Y-D, et al. (2009) Relationship of facet tropism with degeneration and stability of functional spinal unit. Yonsei Med J 50: 624–629. doi: 10.3349/ymj.2009.50.5.624
![]() |
[38] |
Eun SS, Lee H-Y, Lee S-H, et al. (2012) MRI versus CT for the diagnosis of lumbar spinal stenosis. J Neuroradiology 39: 104–109. doi: 10.1016/j.neurad.2011.02.008
![]() |
[39] |
Videman T, Leppävuori J, Kaprio J, et al. (1998) Volvo award winner in basic science studies: Intragenic polymorphisms of the vitamin D receptor gene associated with intervertebral disc degeneration. Spine 23: 2477–2485. doi: 10.1097/00007632-199812010-00002
![]() |
[40] |
Battié MC, Videman T, Parent E (2004) Lumbar disc degeneration: epidemiology and genetic influences. Spine 29: 2679–2690. doi: 10.1097/01.brs.0000146457.83240.eb
![]() |
[41] |
Battié MC, Videman T, Gibbons LE, et al. (1995) Determinants of lumbar disc degeneration: a study relating lifetime exposures and magnetic resonance imaging findings in identical twins. Spine 20: 2601–2612. doi: 10.1097/00007632-199512150-00001
![]() |
[42] |
Cohen SP, Raja SN (2007) Pathogenesis, diagnosis, and treatment of lumbar zygapophysial (facet) joint pain. Anesthesiology 106: 591–615. doi: 10.1097/00000542-200703000-00024
![]() |
1. | Pei-Yu Liu, Sha He, Li-Bin Rong, San-Yi Tang, The effect of control measures on COVID-19 transmission in Italy: Comparison with Guangdong province in China, 2020, 9, 2049-9957, 10.1186/s40249-020-00730-2 | |
2. | Debadatta Adak, Abhijit Majumder, Nandadulal Bairagi, Mathematical perspective of Covid-19 pandemic: Disease extinction criteria in deterministic and stochastic models, 2021, 142, 09600779, 110381, 10.1016/j.chaos.2020.110381 | |
3. | Nao Yamamoto, Bohan Jiang, Haiyan Wang, Quantifying compliance with COVID-19 mitigation policies in the US: A mathematical modeling study, 2021, 6, 24680427, 503, 10.1016/j.idm.2021.02.004 | |
4. | Yongmei Su, Sinuo Liu, Shurui Song, Xiaoke Li, Yongan Ye, Stability Analysis and Clinic Phenomenon Simulation of a Fractional-Order HBV Infection Model, 2020, 2020, 1076-2787, 1, 10.1155/2020/8864403 | |
5. | Virender Singh Panwar, P.S. Sheik Uduman, J.F. Gómez-Aguilar, Mathematical modeling of coronavirus disease COVID-19 dynamics using CF and ABC non-singular fractional derivatives, 2021, 145, 09600779, 110757, 10.1016/j.chaos.2021.110757 | |
6. | Abdelhafid Zeroual, Fouzi Harrou, Abdelkader Dairi, Ying Sun, Deep learning methods for forecasting COVID-19 time-Series data: A Comparative study, 2020, 140, 09600779, 110121, 10.1016/j.chaos.2020.110121 | |
7. | Prasantha Bharathi Dhandapani, Dumitru Baleanu, Jayakumar Thippan, Vinoth Sivakumar, On stiff, fuzzy IRD-14 day average transmission model of COVID-19 pandemic disease, 2020, 7, 2375-1495, 208, 10.3934/bioeng.2020018 | |
8. | Zishuo Yan, Yueheng Lan, Modeling COVID-19 infection in a confined space, 2020, 101, 0924-090X, 1643, 10.1007/s11071-020-05802-4 | |
9. | Ugochinyere Ihuoma Nwosu, Chukwudi Paul Obite, Modeling Ivory Coast COVID-19 cases: Identification of a high-performance model for utilization, 2021, 20, 22113797, 103763, 10.1016/j.rinp.2020.103763 | |
10. | Costas A. Varotsos, Vladimir F. Krapivin, Yong Xue, Diagnostic model for the society safety under COVID-19 pandemic conditions, 2021, 136, 09257535, 105164, 10.1016/j.ssci.2021.105164 | |
11. | Marouane Mahrouf, Adnane Boukhouima, Houssine Zine, El Mehdi Lotfi, Delfim F. M. Torres, Noura Yousfi, Modeling and Forecasting of COVID-19 Spreading by Delayed Stochastic Differential Equations, 2021, 10, 2075-1680, 18, 10.3390/axioms10010018 | |
12. | Shuyun Jiao, Mingzhan Huang, An SIHR epidemic model of the COVID-19 with general population-size dependent contact rate, 2020, 5, 2473-6988, 6714, 10.3934/math.2020431 | |
13. | Sarbaz H. A. Khoshnaw, Rizgar H. Salih, Sadegh Sulaimany, E. Augeraud, M. Banerjee, J.-S. Dhersin, A. d'Onofrio, T. Lipniacki, S. Petrovskii, Chi Tran, A. Veber-Delattre, E. Vergu, V. Volpert, Mathematical modelling for coronavirus disease (COVID-19) in predicting future behaviours and sensitivity analysis, 2020, 15, 0973-5348, 33, 10.1051/mmnp/2020020 | |
14. | Guo-Rong Xing, Ming-Tao Li, Li Li, Gui-Quan Sun, The Impact of Population Migration on the Spread of COVID-19: A Case Study of Guangdong Province and Hunan Province in China, 2020, 8, 2296-424X, 10.3389/fphy.2020.587483 | |
15. | Md. Kamrujjaman, Md. Shahriar Mahmud, Shakil Ahmed, Md. Omar Qayum, Mohammad Morshad Alam, Md Nazmul Hassan, Md Rafiul Islam, Kaniz Fatema Nipa, Ummugul Bulut, SARS-CoV-2 and Rohingya Refugee Camp, Bangladesh: Uncertainty and How the Government Took Over the Situation, 2021, 10, 2079-7737, 124, 10.3390/biology10020124 | |
16. | Chinwendu E. Madubueze, Sambo Dachollom, Isaac Obiajulu Onwubuya, Controlling the Spread of COVID-19: Optimal Control Analysis, 2020, 2020, 1748-670X, 1, 10.1155/2020/6862516 | |
17. | Ayub Ahmed, Bashdar Salam, Mahmud Mohammad, Ali Akgül, Sarbaz H. A. Khoshnaw, Analysis coronavirus disease (COVID-19) model using numerical approaches and logistic model, 2020, 7, 2375-1495, 130, 10.3934/bioeng.2020013 | |
18. | Islam Abdalla Mohamed, Anis Ben Aissa, Loay F. Hussein, Ahmed I. Taloba, Tarak kallel, A new model for epidemic prediction: COVID-19 in kingdom saudi arabia case study, 2021, 22147853, 10.1016/j.matpr.2021.01.088 | |
19. | Nikolaos Yiannakoulias, Catherine E. Slavik, Shelby L. Sturrock, J. Connor Darlington, Open government data, uncertainty and coronavirus: An infodemiological case study, 2020, 265, 02779536, 113549, 10.1016/j.socscimed.2020.113549 | |
20. | I. Neri, L. Gammaitoni, Role of fluctuations in epidemic resurgence after a lockdown, 2021, 11, 2045-2322, 10.1038/s41598-021-85808-z | |
21. | Elba Raimúndez, Erika Dudkin, Jakob Vanhoefer, Emad Alamoudi, Simon Merkt, Lara Fuhrmann, Fan Bai, Jan Hasenauer, COVID-19 outbreak in Wuhan demonstrates the limitations of publicly available case numbers for epidemiological modeling, 2021, 34, 17554365, 100439, 10.1016/j.epidem.2021.100439 | |
22. | Sarbaz H.A. Khoshnaw, Muhammad Shahzad, Mehboob Ali, Faisal Sultan, A quantitative and qualitative analysis of the COVID–19 pandemic model, 2020, 138, 09600779, 109932, 10.1016/j.chaos.2020.109932 | |
23. | Agrim Subedi, Madhav Raj Pandey, Sahadev Bistha, Catalino Dotollo Jr., Feliciano Jonh Matibag Jr., Lok Raj Poudel, Krishna Prasad Subedi, Impact of Solar Planet on Coronavirus (COVID-19) Outbreak, 2020, 2395-602X, 425, 10.32628/IJSRST207646 | |
24. | J.E. Macías-Díaz, Ali Raza, Nauman Ahmed, Muhammad Rafiq, Analysis of a nonstandard computer method to simulate a nonlinear stochastic epidemiological model of coronavirus-like diseases, 2021, 01692607, 106054, 10.1016/j.cmpb.2021.106054 | |
25. | James Francis Oehmke, Charles B Moss, Lauren Nadya Singh, Theresa Bristol Oehmke, Lori Ann Post, Dynamic Panel Surveillance of COVID-19 Transmission in the United States to Inform Health Policy: Observational Statistical Study, 2020, 22, 1438-8871, e21955, 10.2196/21955 | |
26. | Csaba Farkas, David Iclanzan, Boróka Olteán-Péter, Géza Vekov, Estimation of parameters for a humidity-dependent compartmental model of the COVID-19 outbreak, 2021, 9, 2167-8359, e10790, 10.7717/peerj.10790 | |
27. | Benjamin Wacker, Jan Schlüter, Time-continuous and time-discrete SIR models revisited: theory and applications, 2020, 2020, 1687-1847, 10.1186/s13662-020-02995-1 | |
28. | Dipo Aldila, Analyzing the impact of the media campaign and rapid testing for COVID-19 as an optimal control problem in East Java, Indonesia, 2020, 141, 09600779, 110364, 10.1016/j.chaos.2020.110364 | |
29. | Abhijit Majumder, Debadatta Adak, Nandadulal Bairagi, Persistence and extinction criteria of Covid-19 pandemic: India as a case study, 2021, 0736-2994, 1, 10.1080/07362994.2021.1894172 | |
30. | Costas A. Varotsos, Vladimir F. Krapivin, A new model for the spread of COVID-19 and the improvement of safety, 2020, 132, 09257535, 104962, 10.1016/j.ssci.2020.104962 | |
31. | Saad Awadh Alanazi, M. M. Kamruzzaman, Madallah Alruwaili, Nasser Alshammari, Salman Ali Alqahtani, Ali Karime, Dilbag Singh, Measuring and Preventing COVID-19 Using the SIR Model and Machine Learning in Smart Health Care, 2020, 2020, 2040-2309, 1, 10.1155/2020/8857346 | |
32. | Michał Wieczorek, Jakub Siłka, Marcin Woźniak, Neural network powered COVID-19 spread forecasting model, 2020, 140, 09600779, 110203, 10.1016/j.chaos.2020.110203 | |
33. | N.H. Sweilam, S.M. AL - Mekhlafi, D. Baleanu, A hybrid stochastic fractional order Coronavirus (2019-nCov) mathematical model, 2021, 145, 09600779, 110762, 10.1016/j.chaos.2021.110762 | |
34. | Nitin K Kamboj, Sangeeta Sharma, Sandeep Sharma, 2021, Chapter 16, 978-981-33-6263-5, 309, 10.1007/978-981-33-6264-2_16 | |
35. | S. Ibrahim-Tiamiyu, O. V. Oni, E. O. Adeleke, Markovian Analysis of Covid-19 Dynamics, 2021, 4, 2689-5323, 94, 10.52589/ajmss_fzzq3wga | |
36. | Hanna Wulkow, Tim O. F. Conrad, Nataša Djurdjevac Conrad, Sebastian A. Müller, Kai Nagel, Christof Schütte, Seyedali Mirjalili, Prediction of Covid-19 spreading and optimal coordination of counter-measures: From microscopic to macroscopic models to Pareto fronts, 2021, 16, 1932-6203, e0249676, 10.1371/journal.pone.0249676 | |
37. | J. Đorđević, I. Papić, N. Šuvak, A two diffusion stochastic model for the spread of the new corona virus SARS-CoV-2, 2021, 09600779, 110991, 10.1016/j.chaos.2021.110991 | |
38. | Ruqi Li, Yurong Song, Haiyan Wang, Guo-Ping Jiang, Min Xiao, Reactive–diffusion epidemic model on human mobility networks: Analysis and applications to COVID-19 in China, 2023, 609, 03784371, 128337, 10.1016/j.physa.2022.128337 | |
39. | Omaji Samuel, Akogwu Blessing Omojo, Abdulkarim Musa Onuja, Yunisa Sunday, Prayag Tiwari, Deepak Gupta, Ghulam Hafeez, Adamu Sani Yahaya, Oluwaseun Jumoke Fatoba, Shahab Shamshirband, IoMT: A COVID-19 Healthcare System Driven by Federated Learning and Blockchain, 2023, 27, 2168-2194, 823, 10.1109/JBHI.2022.3143576 | |
40. | Abdon Atangana, Seda İgret Araz, 2022, Chapter 6, 978-981-19-0728-9, 131, 10.1007/978-981-19-0729-6_6 | |
41. | M. Aykut Attar, Ayça Tekin-Koru, Latent social distancing: Identification, causes and consequences, 2022, 46, 09393625, 100944, 10.1016/j.ecosys.2022.100944 | |
42. | Tiri Chinyoka, Stochastic modelling of the dynamics of infections caused by the SARS-CoV-2 and COVID-19 under various conditions of lockdown, quarantine, and testing, 2021, 28, 22113797, 104573, 10.1016/j.rinp.2021.104573 | |
43. | Vanshika Aggarwal, Geeta Arora, Homan Emadifar, Faraidun K. Hamasalh, Masoumeh Khademi, Sania Qureshi, Numerical Simulation to Predict COVID-19 Cases in Punjab, 2022, 2022, 1748-6718, 1, 10.1155/2022/7546393 | |
44. | Noureddine Ouerfelli, Narcisa Vrinceanu, Diana Coman, Adriana Lavinia Cioca, Empirical Modeling of COVID-19 Evolution with High/Direct Impact on Public Health and Risk Assessment, 2022, 19, 1660-4601, 3707, 10.3390/ijerph19063707 | |
45. | Huili Wei, Wenhe Li, Dynamical behaviors of a Lotka-Volterra competition system with the Ornstein-Uhlenbeck process, 2023, 20, 1551-0018, 7882, 10.3934/mbe.2023341 | |
46. | Zhenfeng Shi, Daqing Jiang, Xinhong Zhang, Ahmed Alsaedi, A stochastic SEIRS rabies model with population dispersal: Stationary distribution and probability density function, 2022, 427, 00963003, 127189, 10.1016/j.amc.2022.127189 | |
47. | W. Ahmad, M. Abbas, M. Rafiq, D. Baleanu, Mathematical analysis for the effect of voluntary vaccination on the propagation of Corona virus pandemic, 2021, 31, 22113797, 104917, 10.1016/j.rinp.2021.104917 | |
48. | Ajay Kumar, Tsan-Ming Choi, Samuel Fosso Wamba, Shivam Gupta, Kim Hua Tan, Infection vulnerability stratification risk modelling of COVID-19 data: a deterministic SEIR epidemic model analysis, 2021, 0254-5330, 10.1007/s10479-021-04091-3 | |
49. | Abdon Atangana, Seda İgret Araz, 2022, Chapter 8, 978-981-19-0728-9, 237, 10.1007/978-981-19-0729-6_8 | |
50. | Shehzad Afzal, Sohaib Ghani, Hank C. Jenkins-Smith, David S. Ebert, Markus Hadwiger, Ibrahim Hoteit, 2020, A Visual Analytics Based Decision Making Environment for COVID-19 Modeling and Visualization, 978-1-7281-8014-4, 86, 10.1109/VIS47514.2020.00024 | |
51. | Abdon Atangana, Seda İgret Araz, 2022, Chapter 11, 978-981-19-0728-9, 423, 10.1007/978-981-19-0729-6_11 | |
52. | Xueying Wang, Sunpeng Wang, Jin Wang, Libin Rong, A Multiscale Model of COVID-19 Dynamics, 2022, 84, 0092-8240, 10.1007/s11538-022-01058-8 | |
53. | André Vinícius Gonçalves, Ione Jayce Ceola Schneider, Fernanda Vargas Amaral, Leandro Pereira Garcia, Gustavo Medeiros de Araújo, 2021, Chapter 20, 978-3-030-77416-5, 272, 10.1007/978-3-030-77417-2_20 | |
54. | Bo Yang, Zhenhua Yu, Yuanli Cai, A spread model of COVID-19 with some strict anti-epidemic measures, 2022, 109, 0924-090X, 265, 10.1007/s11071-022-07244-6 | |
55. | Wojciech Wróblewski, Norbert Tuśnio, Paweł Wolny, Dorota Siuta, Janusz Trzebicki, Teresa Bączkowska, Olga Dzikowska-Diduch, Piotr Pruszczyk, Fire Safety of Healthcare Units in Conditions of Oxygen Therapy in COVID-19: Empirical Establishing of Effects of Elevated Oxygen Concentrations, 2022, 14, 2071-1050, 4315, 10.3390/su14074315 | |
56. | Tailei Zhang, Zhimin Li, Analysis of COVID-19 epidemic transmission trend based on a time-delayed dynamic model, 2023, 22, 1534-0392, 1, 10.3934/cpaa.2021088 | |
57. | Leonardo S. Lima, Dynamics based on analysis of public data for spreading of disease, 2021, 11, 2045-2322, 10.1038/s41598-021-91024-6 | |
58. | Haoran Fan, 2021, Research on Clinical Treatment of Biomedicine in Preventing Epidemic Model, 9781450385077, 94, 10.1145/3502871.3502887 | |
59. | Sha He, Jie Yang, Mengqi He, Dingding Yan, Sanyi Tang, Libin Rong, The risk of future waves of COVID-19: modeling and data analysis, 2021, 18, 1551-0018, 5409, 10.3934/mbe.2021274 | |
60. | Eiman Ijaz, Johar Ali, Abbas Khan, Muhammad Shafiq, Taj Munir, 2023, Chapter 9, 978-1-80356-566-8, 10.5772/intechopen.108450 | |
61. | Yuansan Liu, Saransh Srivastava, Zuo Huang, Felisa J. Vázquez-Abad, Pandemic model with data-driven phase detection, a study using COVID-19 data, 2021, 0160-5682, 1, 10.1080/01605682.2021.1982652 | |
62. | Roman Zúñiga Macías, Humberto Gutiérrez-Pulido, Edgar Alejandro Guerrero Arroyo, Abel Palafox González, Geographical network model for COVID-19 spread among dynamic epidemic regions, 2022, 19, 1551-0018, 4237, 10.3934/mbe.2022196 | |
63. | Abdon Atangana, Seda İgret Araz, 2022, Chapter 10, 978-981-19-0728-9, 353, 10.1007/978-981-19-0729-6_10 | |
64. | Supriya Vanahalli, Preethi N, 2021, An Intelligent System to Forecast COVID-19 Pandemic using Hybrid Neural Network, 978-1-6654-2306-9, 543, 10.1109/CSNT51715.2021.9509622 | |
65. | Tarun Kumar Martheswaran, Hamida Hamdi, Amal Al-Barty, Abeer Abu Zaid, Biswadeep Das, Prediction of dengue fever outbreaks using climate variability and Markov chain Monte Carlo techniques in a stochastic susceptible-infected-removed model, 2022, 12, 2045-2322, 10.1038/s41598-022-09489-y | |
66. | Abdulaziz Attaallah, Sabita Khatri, Mohd Nadeem, Syed Anas Ansar, Abhishek Kumar Pandey, Alka Agrawal, Prediction of COVID-19 Pandemic Spread in Kingdom of Saudi Arabia, 2021, 37, 0267-6192, 313, 10.32604/csse.2021.014933 | |
67. | Mihaela Cornelia Dan, Simona Irina Goia, Vlad I. Roşca, 2022, Chapter 11, 978-3-030-82754-0, 189, 10.1007/978-3-030-82755-7_11 | |
68. | Martijn H. H. Schoot Uiterkamp, Martijn Gösgens, Hans Heesterbeek, Remco van der Hofstad, Nelly Litvak, The role of inter-regional mobility in forecasting SARS-CoV-2 transmission, 2022, 19, 1742-5662, 10.1098/rsif.2022.0486 | |
69. | Yansong Pei, Bing Liu, Haokun Qi, Extinction and stationary distribution of stochastic predator-prey model with group defense behavior, 2022, 19, 1551-0018, 13062, 10.3934/mbe.2022610 | |
70. | Feres A. Salem, Ubirajara F. Moreno, A Multi-Agent-Based Simulation Model for the Spreading of Diseases Through Social Interactions During Pandemics, 2022, 33, 2195-3880, 1161, 10.1007/s40313-022-00920-3 | |
71. | Abdon Atangana, Seda İgret Araz, 2022, Chapter 9, 978-981-19-0728-9, 275, 10.1007/978-981-19-0729-6_9 | |
72. | Carla Marcelino Trassante, Victor dos Santos Barboza, Liziane dos Santos Rocha, Paulo Maximiliano Correa, Cristiane Luchese, Ethel Antunes Wilhelm, Claudio Martin Pereira de Pereira, Matheus Dellaméa Baldissera, Virginia Cielo Rech, Janice Luehring Giongo, Rodrigo de Almeida Vaucher, Detection of SARS-CoV-2 virus using an alternative molecular method and evaluation of biochemical, hematological, inflammatory, and oxidative stress in healthcare professionals, 2021, 158, 08824010, 104975, 10.1016/j.micpath.2021.104975 | |
73. | Manal El Mouhib, Kamal Azghiou, Abdelhamid Benali, 2022, Connected and Autonomous Vehicles against a Malware Spread : A Stochastic Modeling Approach, 978-1-6654-8684-2, 1, 10.1109/IEMTRONICS55184.2022.9795713 | |
74. | Seung-Nam Park, Hyong-Ha Kim, Kyoung Beom Lee, Inherently high uncertainty in predicting the time evolution of epidemics, 2021, 43, 2092-7193, e2021014, 10.4178/epih.e2021014 | |
75. | Shahriar Seddighi Chaharborj, Sarkhosh Seddighi Chaharborj, Jalal Hassanzadeh Asl, Pei See Phang, Controlling of pandemic COVID-19 using optimal control theory, 2021, 26, 22113797, 104311, 10.1016/j.rinp.2021.104311 | |
76. | Xiao-Li Ding, Iván Area, Juan J. Nieto, Controlled singular evolution equations and Pontryagin type maximum principle with applications, 2022, 11, 2163-2480, 1655, 10.3934/eect.2021059 | |
77. | Amir Veisi, Hadi Delavari, A novel fractional-order feedback management of COVID-19 prevalence, 2022, 25, 0972-0510, 1345, 10.1080/09720510.2021.1970951 | |
78. | Junaid Iqbal Khan, Jebran Khan, Furqan Ali, Farman Ullah, Jamshid Bacha, Sungchang Lee, Artificial Intelligence and Internet of Things (AI-IoT) Technologies in Response to COVID-19 Pandemic: A Systematic Review, 2022, 10, 2169-3536, 62613, 10.1109/ACCESS.2022.3181605 | |
79. | Divine Wanduku, The multilevel hierarchical data EM-algorithm. Applications to discrete-time Markov chain epidemic models, 2022, 8, 24058440, e12622, 10.1016/j.heliyon.2022.e12622 | |
80. | Xiaoying Wang, Qing Han, Jude Dzevela Kong, Studying the mixed transmission in a community with age heterogeneity: COVID-19 as a case study, 2022, 7, 24680427, 250, 10.1016/j.idm.2022.05.006 | |
81. | 2023, 9780443186790, 229, 10.1016/B978-0-44-318679-0.00013-2 | |
82. | Nursanti Anggriani, Lazarus Kalvein Beay, Modeling of COVID-19 spread with self-isolation at home and hospitalized classes, 2022, 36, 22113797, 105378, 10.1016/j.rinp.2022.105378 | |
83. | Leonardo dos Santos Lima, Fractional Stochastic Differential Equation Approach for Spreading of Diseases, 2022, 24, 1099-4300, 719, 10.3390/e24050719 | |
84. | Sahil Ahuja, Nitin Arvind Shelke, Pawan Kumar Singh, A deep learning framework using CNN and stacked Bi-GRU for COVID-19 predictions in India, 2022, 16, 1863-1703, 579, 10.1007/s11760-021-01988-1 | |
85. | Bimal Kumar Mishra, Stochastic models on the transmission of novel COVID-19, 2022, 13, 0975-6809, 599, 10.1007/s13198-021-01312-7 | |
86. | C. Gokila, M. Sambath, K. Balachandran, Yong-Ki Ma, Stationary distribution and long-time behavior of COVID-19 model with stochastic effect, 2023, 16, 1793-5245, 10.1142/S1793524522500747 | |
87. | Roozbeh Abolpour, Sara Siamak, Mohsen Mohammadi, Parisa Moradi, Maryam Dehghani, Linear parameter varying model of COVID-19 pandemic exploiting basis functions, 2021, 70, 17468094, 102999, 10.1016/j.bspc.2021.102999 | |
88. | Kexin Chen, Chi Seng Pun, Hoi Ying Wong, Efficient social distancing during the COVID-19 pandemic: Integrating economic and public health considerations, 2023, 304, 03772217, 84, 10.1016/j.ejor.2021.11.012 | |
89. | Sultanah M. Alshammari, Waleed K. Almutiry, Harsha Gwalani, Saeed M. Algarni, Kawther Saeedi, Measuring the impact of suspending Umrah, a global mass gathering in Saudi Arabia on the COVID-19 pandemic, 2021, 1381-298X, 10.1007/s10588-021-09343-y | |
90. | Sewanou H. Honfo, Hemaho B. Taboe, Romain Glèlè Kakaï, Modeling COVID-19 dynamics in the sixteen West African countries, 2022, 18, 24682276, e01408, 10.1016/j.sciaf.2022.e01408 | |
91. | Natasha Sharma, Atul Kumar Verma, Arvind Kumar Gupta, Spatial network based model forecasting transmission and control of COVID-19, 2021, 581, 03784371, 126223, 10.1016/j.physa.2021.126223 | |
92. | Enes Eryarsoy, Masoud Shahmanzari, Fehmi Tanrisever, Models for government intervention during a pandemic, 2023, 304, 03772217, 69, 10.1016/j.ejor.2021.12.036 | |
93. | Aaqib Inam, Ayesha Sarwar, Ayesha Atta, Iftikhar Naaseer, Shahan Yamin Siddiqui, Muhammad Adnan Khan, Detection of COVID-19 Enhanced by a Deep Extreme Learning Machine, 2021, 27, 1079-8587, 701, 10.32604/iasc.2021.014235 | |
94. | A. Mhlanga, T. V. Mupedza, A patchy theoretical model for the transmission dynamics of SARS-Cov-2 with optimal control, 2022, 12, 2045-2322, 10.1038/s41598-022-21553-1 | |
95. | P. Renukadevi, A. Rajiv Kannan, Covid-19 Forecasting with Deep Learning-based Half-binomial Distribution Cat Swarm Optimization, 2023, 44, 0267-6192, 629, 10.32604/csse.2023.024217 | |
96. | Sanmitra Ghosh, Saptarshi Chatterjee, Shatarupa Biswas, Megha Dutta, 2022, 9780323858441, 97, 10.1016/B978-0-323-85844-1.00010-6 | |
97. | Demián García-Violini, Ricardo Sánchez-Peña, Marcela Moscoso-Vásquez, Fabricio Garelli, Non-pharmaceutical intervention to reduce COVID-19 impact in Argentina, 2022, 124, 00190578, 225, 10.1016/j.isatra.2021.06.024 | |
98. | Ayman Mourad, Fatima Mroue, Zahraa Taha, Stochastic mathematical models for the spread of COVID-19: a novel epidemiological approach, 2022, 39, 1477-8599, 49, 10.1093/imammb/dqab019 | |
99. | Cheng-Cheng Zhu, Jiang Zhu, Spread trend of COVID-19 epidemic outbreak in China: using exponential attractor method in a spatial heterogeneous SEIQR model, 2020, 17, 1551-0018, 3062, 10.3934/mbe.2020174 | |
100. | Aysan Vaez, Amin Izadi, Majid Mirmohammadkhani, Mohammad Memarian, Maral Hemati, Parviz Kokhaei, Infectivity Period After Symptom Relief in SARS-CoV-2 Patients: An Experimental Trial, 2022, 9, 2423-4451, 10.5812/mejrh.115349 | |
101. | William Qian, Sanjukta Bhowmick, Marty O’Neill, Suhasini Ramisetty-Mikler, Armin R. Mikler, Applying a Probabilistic Infection Model for studying contagion processes in contact networks, 2021, 54, 18777503, 101419, 10.1016/j.jocs.2021.101419 | |
102. | Jingli Ren, Haiyan Wang, 2023, 9780443186790, 173, 10.1016/B978-0-44-318679-0.00012-0 | |
103. | Adesoye Idowu Abioye, Olumuyiwa James Peter, Hammed Abiodun Ogunseye, Festus Abiodun Oguntolu, Kayode Oshinubi, Abdullahi Adinoyi Ibrahim, Ilyas Khan, Mathematical model of COVID-19 in Nigeria with optimal control, 2021, 28, 22113797, 104598, 10.1016/j.rinp.2021.104598 | |
104. | Wenhui Zhu, Jie Feng, Cheng Li, Huimin Wang, Yang Zhong, Lijun Zhou, Xingyu Zhang, Tao Zhang, COVID-19 Risk Assessment for the Tokyo Olympic Games, 2021, 9, 2296-2565, 10.3389/fpubh.2021.730611 | |
105. | Jin Guo, Aili Wang, Weike Zhou, Yinjiao Gong, Stacey R. Smith?, Discrete epidemic modelling of COVID-19 transmission in Shaanxi Province with media reporting and imported cases, 2021, 19, 1551-0018, 1388, 10.3934/mbe.2022064 | |
106. | A.I.K. Butt, W. Ahmad, M. Rafiq, D. Baleanu, Numerical analysis of Atangana-Baleanu fractional model to understand the propagation of a novel corona virus pandemic, 2022, 61, 11100168, 7007, 10.1016/j.aej.2021.12.042 | |
107. | Abdon Atangana, Seda İgret Araz, 2022, Chapter 12, 978-981-19-0728-9, 481, 10.1007/978-981-19-0729-6_12 | |
108. | Mathilde Massard, Raluca Eftimie, Antoine Perasso, Bruno Saussereau, A multi-strain epidemic model for COVID-19 with infected and asymptomatic cases: Application to French data, 2022, 545, 00225193, 111117, 10.1016/j.jtbi.2022.111117 | |
109. | Kazem Nouri, Milad Fahimi, Leila Torkzadeh, Dumitru Baleanu, Stochastic Epidemic Model of Covid-19 via the Reservoir-People Transmission Network, 2022, 72, 1546-2226, 1495, 10.32604/cmc.2022.024406 | |
110. | Fran Sérgio Lobato, Gustavo Barbosa Libotte, Gustavo Mendes Platt, Mathematical modelling of the second wave of COVID-19 infections using deterministic and stochastic SIDR models, 2021, 106, 0924-090X, 1359, 10.1007/s11071-021-06680-0 | |
111. | D. Nagarajan, R. Sujatha, G. Kuppuswami, J. Kavikumar, Real-time forecasting of the COVID 19 using fuzzy grey Markov: a different approach in decision-making, 2022, 41, 2238-3603, 10.1007/s40314-022-01949-5 | |
112. | Chuang Xu, Yongzhen Pei, Shengqiang Liu, Jinzhi Lei, Effectiveness of non-pharmaceutical interventions against local transmission of COVID-19: An individual-based modelling study, 2021, 6, 24680427, 848, 10.1016/j.idm.2021.06.005 | |
113. | Wenjing Zhao, Lin Zhang, Junling Wang, Lili Wang, How has academia responded to the urgent needs created by COVID-19? A multi-level global, regional and national analysis, 2022, 0165-5515, 016555152210846, 10.1177/01655515221084646 | |
114. | Christiana Tsiligianni, Aristeides Tsiligiannis, Christos Tsiliyannis, A stochastic inventory model of COVID-19 and robust, real-time identification of carriers at large and infection rate via asymptotic laws, 2023, 304, 03772217, 42, 10.1016/j.ejor.2021.12.037 | |
115. | Xinyu Bai, Shaojuan Ma, Stochastic dynamical behavior of COVID-19 model based on secondary vaccination, 2022, 20, 1551-0018, 2980, 10.3934/mbe.2023141 | |
116. | S Zenian, The SIR Model for COVID-19 in Malaysia, 2022, 2314, 1742-6588, 012007, 10.1088/1742-6596/2314/1/012007 | |
117. | Ru-Qi Li, Yu-Rong Song, Guo-Ping Jiang, Prediction of epidemics dynamics on networks with partial differential equations: A case study for COVID-19 in China* , 2021, 30, 1674-1056, 120202, 10.1088/1674-1056/ac2b16 | |
118. | Cunjuan Dong, Changcheng Xiang, Wenjin Qin, Yi Yang, Global dynamics for a Filippov system with media effects, 2022, 19, 1551-0018, 2835, 10.3934/mbe.2022130 | |
119. | André Vinícius Gonçalves, Gustavo Medeiros de Araujo, Leandro Pereira Garcia, Fernanda Vargas Amaral, Ione Jayce Ceola Schneider, Feature Importance Analysis by Nowcasting Perspective to Predict COVID-19, 2022, 27, 1383-469X, 1967, 10.1007/s11036-022-01966-y | |
120. | Farid A. Badria, 2022, Chapter 2, 978-1-80355-330-6, 10.5772/intechopen.103153 | |
121. | A. M. Elshehawey, Zhengming Qian, A novel high-order multivariate Markov model for spatiotemporal analysis with application to COVID-19 outbreak, 2023, 52, 1226-3192, 495, 10.1007/s42952-023-00210-x | |
122. | Wenxuan Li, Suli Liu, Dynamic analysis of a stochastic epidemic model incorporating the double epidemic hypothesis and Crowley-Martin incidence term, 2023, 31, 2688-1594, 6134, 10.3934/era.2023312 | |
123. | Costas A. Varotsos, Vladimir F. Krapivin, Ferdenant A. Mkrtchyan, Yong Xue, 2023, Chapter 2, 978-3-031-28876-0, 119, 10.1007/978-3-031-28877-7_2 | |
124. | Mirna Patricia Ponce-Flores, Jesús David Terán-Villanueva, Salvador Ibarra-Martínez, José Antonio Castán-Rocha, Generalized Pandemic Model with COVID-19 for Early-Stage Infection Forecasting, 2023, 11, 2227-7390, 3924, 10.3390/math11183924 | |
125. | Costas A. Varotsos, Vladimir F. Krapivin, Ferdenant A. Mkrtchyan, Yong Xue, 2023, Chapter 3, 978-3-031-28876-0, 163, 10.1007/978-3-031-28877-7_3 | |
126. | Mohammed Salman, Prativa Sahoo, Anushaya Mohapatra, Sanjay Kumar Mohanty, Libin Rong, An infectious disease epidemic model with migration and stochastic transmission in deterministic and stochastic environments, 2024, 5, 27724425, 100337, 10.1016/j.health.2024.100337 | |
127. | Yuxiao Zhao, Hui Wang, Dongxu Wang, The Dynamic Behavior of a Stochastic SEIRM Model of COVID-19 with Standard Incidence Rate, 2024, 12, 2227-7390, 2966, 10.3390/math12192966 | |
128. | Niketa Ukaj, Christian Hellmich, Stefan Scheiner, Aging Epidemiology: A Hereditary Mechanics–Inspired Approach to COVID-19 Fatality Rates, 2024, 150, 0733-9399, 10.1061/JENMDT.EMENG-7640 | |
129. | Xiaojie Mu, Daqing Jiang, A stochastic SIHR epidemic model with general population-size dependent contact rate and Ornstein–Uhlenbeck process: dynamics analysis, 2024, 112, 0924-090X, 10703, 10.1007/s11071-024-09586-9 | |
130. | A. Lahrouz, T. Caraballo, I. Bouzalmat, A. Settati, Randomness suppress backward bifurcation in an epidemic model with limited medical resources, 2025, 140, 10075704, 108380, 10.1016/j.cnsns.2024.108380 | |
131. | Denis Ndanguza, Jean Pierre Ngendahayo, Annie Uwimana, Jean de Dieu Niyigena, Isambi S. Mbalawata, Innocent Ngaruye, Joseph Nzabanita, Emmanuel Masabo, Marcel Gahamanyi, Bosco Nyandwi, Justine Dushimirimana, Lydie Mpinganzima, Celestin Kurujyibwami, Leon Fidele Uwimbabazi Ruganzu, Venuste Nyagahakwa, Wellars Banzi, Solange Mukeshimana, Jean Pierre Muhirwa, Jean Paul Nsabimana, Jeanne Uwonkunda, Betty Kivumbi Nannyonga, Japhet Niyobuhungiro, Eric Rutaganda, Jean Marie Ntaganda, Analysis of the COVID-19 pandemic in Rwanda using a stochastic model, 2024, 03, 2811-0072, 10.1142/S281100722350013X | |
132. | Sida Kang, Tianhao Liu, Hongyu Liu, Yuhan Hu, Xilin Hou, Sanket Kaushik, Dynamic analysis and optimal control of stochastic information cross-dissemination and variation model with random parametric perturbations, 2024, 19, 1932-6203, e0303300, 10.1371/journal.pone.0303300 | |
133. | Ge Zhang, Zhiming Li, Anwarud Din, Tao Chen, Dynamic analysis and optimal control of a stochastic COVID-19 model, 2024, 215, 03784754, 498, 10.1016/j.matcom.2023.08.005 | |
134. | Qianqian Sun, Dejun Tan, Shuwen Zhang, Epidemic threshold of a COVID-19 model with gaussian white noise and semi-Markov switching, 2023, 360, 00160032, 6846, 10.1016/j.jfranklin.2023.04.035 | |
135. | Costas A. Varotsos, Vladimir F. Krapivin, Ferdenant A. Mkrtchyan, Yong Xue, 2023, Chapter 6, 978-3-031-28876-0, 293, 10.1007/978-3-031-28877-7_6 | |
136. | Daniele Baccega, Paolo Castagno, Antonio Fernández Anta, Matteo Sereno, Enhancing COVID-19 forecasting precision through the integration of compartmental models, machine learning and variants, 2024, 14, 2045-2322, 10.1038/s41598-024-69660-5 | |
137. | Ugo Avila-Ponce de León, Angel G. C. Pérez, Eric Avila-Vales, Modeling the SARS-CoV-2 Omicron variant dynamics in the United States with booster dose vaccination and waning immunity, 2023, 20, 1551-0018, 10909, 10.3934/mbe.2023484 | |
138. | Jie Yang, Sanyi Tang, Jie Lou, A stochastic discrete-time co-evolution model and its application in simulating the impacts of behavior changes on the secondary and multiple outbreaks of the COVID-19 pandemic, 2023, 138, 2190-5444, 10.1140/epjp/s13360-023-04702-x | |
139. | Xinhong Zhang, Qing Yang, Tan Su, Dynamical behavior and numerical simulation of a stochastic eco-epidemiological model with Ornstein–Uhlenbeck process, 2023, 123, 10075704, 107284, 10.1016/j.cnsns.2023.107284 | |
140. | Christos Tsiliyannis, Beyond SIRD models: a novel dynamic model for epidemics, relating infected with entries to health care units and application for identification and restraining policy, 2024, 41, 1477-8599, 192, 10.1093/imammb/dqae008 | |
141. | Costas A. Varotsos, Vladimir F. Krapivin, Ferdenant A. Mkrtchyan, Yong Xue, 2023, Chapter 1, 978-3-031-28876-0, 1, 10.1007/978-3-031-28877-7_1 | |
142. | Yue Yu, Yuanshun Tan, Sanyi Tang, Stability analysis of the COVID-19 model with age structure under media effect, 2023, 42, 2238-3603, 10.1007/s40314-023-02330-w | |
143. | Costas A. Varotsos, Vladimir F. Krapivin, Ferdenant A. Mkrtchyan, Yong Xue, 2023, Chapter 7, 978-3-031-28876-0, 399, 10.1007/978-3-031-28877-7_7 | |
144. | Khristo Tarnev, Nikolay K. Vitanov, 2023, 2939, 0094-243X, 090009, 10.1063/5.0178872 | |
145. | Henry Egbezien Inegbedion, A Time Series Forecast of COVID-19 Infections, Recoveries and Fatalities in Nigeria, 2023, 15, 2071-1050, 7324, 10.3390/su15097324 | |
146. | Berik Koichubekov, Aliya Takuadina, Ilya Korshukov, Marina Sorokina, Anar Turmukhambetova, The Epidemiological and Economic Impact of COVID-19 in Kazakhstan: An Agent-Based Modeling, 2023, 11, 2227-9032, 2968, 10.3390/healthcare11222968 | |
147. | Ayman Mourad, Fatima Mroue, Discrete spread model for COVID‐19: the case of Lebanon, 2022, 10, 2095-4689, 157, 10.15302/J-QB-022-0292 | |
148. | Ashley N. Micuda, Mark R. Anderson, Irina Babayan, Erin Bolger, Logan Cantin, Gillian Groth, Ry Pressman-Cyna, Charlotte Z. Reed, Noah J. Rowe, Mehdi Shafiee, Benjamin Tam, Marie C. Vidal, Tianai Ye, Ryan D. Martin, Exploring a targeted approach for public health capacity restrictions during COVID-19 using a new computational model, 2024, 9, 24680427, 234, 10.1016/j.idm.2024.01.001 | |
149. | Smita R Pidurkar, Seema Raut, 2024, 3139, 0094-243X, 120001, 10.1063/5.0224961 | |
150. | Costas A. Varotsos, Vladimir F. Krapivin, Ferdenant A. Mkrtchyan, Yong Xue, 2023, Chapter 4, 978-3-031-28876-0, 233, 10.1007/978-3-031-28877-7_4 | |
151. | Jyy-I Hong, Limiting Behaviors of Stochastic Spread Models Using Branching Processes, 2023, 12, 2075-1680, 652, 10.3390/axioms12070652 | |
152. | Arindam Brahma, Samir Chatterjee, Kala Seal, Ben Fitzpatrick, Youyou Tao, Development of a Cohort Analytics Tool for Monitoring Progression Patterns in Cardiovascular Diseases: Advanced Stochastic Modeling Approach, 2024, 12, 2291-9694, e59392, 10.2196/59392 | |
153. | Frederico José Ribeiro Pelogia, Henrique Mohallem Paiva, Roberson Saraiva Polli, Multi-wave modelling and short-term prediction of ICU bed occupancy by patients with Covid-19 in regions of Italy, 2024, 19, 0973-5348, 13, 10.1051/mmnp/2024012 | |
154. | S. Swetha, S. Sindu Devi, K. Kannan, Optimal Control Strategies for COVID-19 Using SEIQR Mathematical Model, 2024, 0369-8203, 10.1007/s40010-024-00898-4 | |
155. | Costas A. Varotsos, Vladimir F. Krapivin, Ferdenant A. Mkrtchyan, Yong Xue, 2023, Chapter 5, 978-3-031-28876-0, 247, 10.1007/978-3-031-28877-7_5 | |
156. | Ikpe Justice Akpan, Murali Shanker, Onyebuchi Felix Offodile, Discrete‐event simulation is still alive and strong: evidence from bibliometric performance evaluation of research during COVID‐19 global health pandemic, 2024, 31, 0969-6016, 2069, 10.1111/itor.13418 | |
157. | Yung-Tsan Jou, Ming-Chang Lin, Riana Magdalena Silitonga, Shao-Yang Lu, Ni-Ying Hsu, A Systematic Model to Improve Productivity in a Transformer Manufacturing Company: A Simulation Case Study, 2024, 14, 2076-3417, 519, 10.3390/app14020519 | |
158. | P. Sasikala, L. Mary Immaculate Sheela, An Efficient COVID-19 Disease Outbreak Prediction Using BI-SSOA-TMLPNN and ARIMA, 2024, 24, 0219-4678, 10.1142/S0219467823400119 | |
159. | Xinhe Zhu, Yuanyou Shi, Yongmin Zhong, An EKF prediction of COVID-19 propagation under vaccinations and viral variants, 2025, 231, 03784754, 221, 10.1016/j.matcom.2024.12.012 | |
160. | Yuping Chen, Lijun Pei, Youming Lei, A review of mathematical researches on the COVID-19 pandemic, 2025, 13, 2195-268X, 10.1007/s40435-024-01519-z | |
161. | PRITAM SAHA, KALYAN KUMAR PAL, UTTAM GHOSH, PANKAJ KUMAR TIWARI, EFFECTS OF VACCINATION AND SATURATED TREATMENT ON COVID-19 TRANSMISSION IN INDIA: DETERMINISTIC AND STOCHASTIC APPROACHES, 2025, 33, 0218-3390, 53, 10.1142/S021833902450044X | |
162. | Yuanyou Shi, Xinhang Zhu, Xinhe Zhu, Baiqi Cheng, Yongmin Zhong, Kalman Filter-Based Epidemiological Model for Post-COVID-19 Era Surveillance and Prediction, 2025, 25, 1424-8220, 2507, 10.3390/s25082507 | |
163. | Guo Jiang, Zhicen Hu, Yuanqin Chen, An epidemic model of HIV transmission with two stochastic perturbations, 2025, 2025, 2731-4235, 10.1186/s13662-025-03952-6 | |
164. | Chang Zhou, Zhiming Li, Parameter estimation of stochastic SEIR epidemic model using particle MCMC, 2025, 35, 1054-1500, 10.1063/5.0264087 |