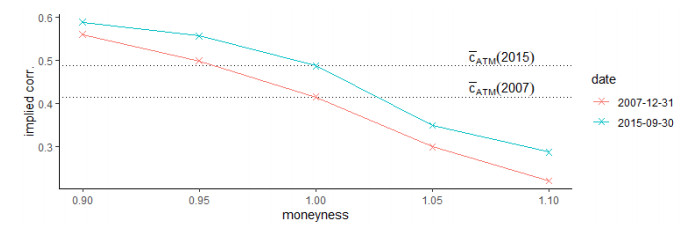
Citation: Guillermo Peña. A new trading algorithm with financial applications[J]. Quantitative Finance and Economics, 2020, 4(4): 596-607. doi: 10.3934/QFE.2020027
[1] | María Anguiano, Renata Bunoiu . Homogenization of Bingham flow in thin porous media. Networks and Heterogeneous Media, 2020, 15(1): 87-110. doi: 10.3934/nhm.2020004 |
[2] | Leda Bucciantini, Angiolo Farina, Antonio Fasano . Flows in porous media with erosion of the solid matrix. Networks and Heterogeneous Media, 2010, 5(1): 63-95. doi: 10.3934/nhm.2010.5.63 |
[3] | Verónica Anaya, Mostafa Bendahmane, David Mora, Ricardo Ruiz Baier . On a vorticity-based formulation for reaction-diffusion-Brinkman systems. Networks and Heterogeneous Media, 2018, 13(1): 69-94. doi: 10.3934/nhm.2018004 |
[4] | Edoardo Mainini . On the signed porous medium flow. Networks and Heterogeneous Media, 2012, 7(3): 525-541. doi: 10.3934/nhm.2012.7.525 |
[5] | Steinar Evje, Kenneth H. Karlsen . Hyperbolic-elliptic models for well-reservoir flow. Networks and Heterogeneous Media, 2006, 1(4): 639-673. doi: 10.3934/nhm.2006.1.639 |
[6] | Andro Mikelić, Giovanna Guidoboni, Sunčica Čanić . Fluid-structure interaction in a pre-stressed tube with thick elastic walls I: the stationary Stokes problem. Networks and Heterogeneous Media, 2007, 2(3): 397-423. doi: 10.3934/nhm.2007.2.397 |
[7] | Laura Cattaneo, Paolo Zunino . Computational models for fluid exchange between microcirculation and tissue interstitium. Networks and Heterogeneous Media, 2014, 9(1): 135-159. doi: 10.3934/nhm.2014.9.135 |
[8] | Michael Eden, Michael Böhm . Homogenization of a poro-elasticity model coupled with diffusive transport and a first order reaction for concrete. Networks and Heterogeneous Media, 2014, 9(4): 599-615. doi: 10.3934/nhm.2014.9.599 |
[9] | Catherine Choquet, Ali Sili . Homogenization of a model of displacement with unbounded viscosity. Networks and Heterogeneous Media, 2009, 4(4): 649-666. doi: 10.3934/nhm.2009.4.649 |
[10] | Jean-Marc Hérard, Olivier Hurisse . Some attempts to couple distinct fluid models. Networks and Heterogeneous Media, 2010, 5(3): 649-660. doi: 10.3934/nhm.2010.5.649 |
Equity investment practice frequently distinguishes between up- and down correlations as stock markets typically face such asymmetries: during bullish times, average correlation among stocks is low to moderate, but remarkably increases if markets turn into bearish. This effect is not only thoroughly documented within realized return patterns (e.g. Erb et al. (1994) or Ang & Chen (2002)), but also highly affects portfolio diversification. Given so, this paper investigates whether such correlation duality also exists at investor expectations and how it can be quantified from forward looking data. Hence, aim of the presented paper is to develop an econometric model which is able to fulfill this task. The core of the model builds on option implied volatilities, which reflect per construction investor expectations. Upon those risk believes it is demonstrated that asymmetric up-/down correlations will cause skewness in the joint distribution of the market portfolio. Let the correlation spread be understood as down-correlations minus up ones. If this spread is positive, then marginal return distributions of constituents will aggregate superior for the negative side of the joint distribution (i.e., market portfolio) than for the positive side. As a logic consequent, the joint distribution will be negatively skewed, even though if single stocks would be normally distributed. Thus, the model argues that expected skewness of the market portfolio in excess of marginal skews can be directly linked to correlation duality, which in turn allows to estimate such. Besides the theoretical discourse, robustness of the model is verified upon simulated data to analyze its precision and to verify that it is free of statistical biases. From empirical data of the S & P500 I demonstrate that it is straightforward to implement and highlight its practical usage for applications in corporate finance (e.g., equity valuation) or portfolio management.
While the body of finance literature on option implied volatilities is already very broad (like Schmalensee & Trippi (1978), Bakshi et al. (2003) or Schneider et al. (2020)), research on equity implied correlations is scarce (e.g., Skintzi & Refenes (2005)). Further, although correlation duality is profoundly documented within historical return data (Longin & Solnik (1995), Cappiello et al. (2006) or Ang & Bekaert (2015)), to the best of my knowledge no study yet analyzed up- and down correlations within forward looking data. Therefore, this paper contributes to existing literature by providing a model which is able to quantify expected correlation asymmetries and delivers initial insights into their empirical behavior with some remarkable findings.
The reading starts with a brief literature overview to trace the outline of the relevant field. Section 3 sketches the model from a theoretical perspective, whose quality is evaluated on simulated data in Section 4. Within the fifth section the model is applied upon real world data which allows to analyze empirical characteristics of the expected correlation spread. Final conclusion are drawn in Section 6.
Generally speaking, this paper relates to two areas of finance research. First, to empirical studies analyzing equity correlations during different market phases. Second, to the measurement of investor expectations out of option data.
The field of correlation's time varying behavior enjoys a broad body of academic literature. For example, there are earlier studies like King & Wadhwani (1990) or Bertero & Mayer (1990) which found that correlations across international financial markets significantly increased during the market crash of 1987, which gives indication in favor of down correlations being higher than up correlations. On a long time analysis (1960–1990), Longin & Solnik (1995) conclude that the level of correlation is greater during phases of high volatility, which can also be interpreted in favor of the market mood dependent correlation duality. However, there are also early studies that do not coerce with this statement, like Kaplanis (1988) or Ratner (1992) who claim that correlations remain constant over time, or Kizys & Pierdzioch (2006) who find on the period of 1970–2004 that correlation's dependence on the business cycle is not significant. Erb et al. (1994) explicitly analyze correlation structures among countries in dependence of the economic cycle with the conclusion, that during recessions they are substantially greater than during market recoveries. While there are conflicting results especially at earlier studies, by now the majority of evidence consent to correlation asymmetries, for example Ramchand & Susmel (1998), Longin & Solnik (2001), Ang & Chen (2002), Sancetta & Satchell (2007), Cappiello et al. (2006) or Ang & Bekaert (2015). Besides macroeconomic analyzes of equity correlation structures, other works focused on implementing such asymmetries. A frequent example in that context are dual factor pricing models (especially CAPM, see Pettengill et al. (1995)), which are not only used at investment practice, but also find broad support in academic research like Howton & Peterson (1999), Faff (2001), Pettengill et al. (2002) or Chong et al. (2011), Chong & Phillips (2013).
What those mentioned studies have in common is that they analyze correlations of realized returns. When quantifying expectations, however, the use of such historical return data can be problematic as there is no guarantee that realizations in the past will repeat themselves in the future. (cp. Bali et al. (2019) or Schneider et al. (2020)) This is why the study presented here focuses on the use of forward looking data. Or, to be more precisely, the model builds on the availability of option implied volatilities. Literature in this discipline was initialized by Black & Scholes (1973), the use of implied volatilities as investor expectations goes back to Schmalensee & Trippi (1978). Guo et al. (2016) point out that working with implied volatilities rather than option prices directly became common practice in quantitative finance. Recent examples of option implied information are (among many others) Bakshi et al. (2003) who provide a model for ex-ante higher moments, Schneider et al. (2020) analyzing asset pricing anomalies or Bali et al. (2019) with an expectation-based empirical asset pricing study - just to name a few. Besides the field of asset pricing, implied volatilities are also used in an economic context, for example by Fassas, Kenourgios & Papadamou (2020) to quantify the effect of monetary policy announcements upon expected currency risk. Or, by Fassas, Papadamou & Philippas (2020) where implied volatilities serve to measure international spillover effects in investor risk-aversion with respect to quantitative easing. Also pointing into the behavioral direction, Äijö (2008) and Schadner (2020a) find empirical support that the implied volatility term structure indicates investor sentiment. On the econometric side, Jeon et al. (2020) demonstrate that implied volatilities can be used to enhance the predictive power of existing models.
As there exist many studies on implied volatilities as well as implied correlations for the FX market (e.g., Walter & Lopez (2000)), literature on implied equity correlations is rather scarce. The difficulty of computing implied correlations (and the respective matrix) arises from the fact that in a stock market there are no direct derivatives for pairs of stocks, thus no unique solution to this problem currently exists. However, one mathematically feasible solution to the ex-ante correlation matrix is the CBOE Implied Correlation Index (cp. Skintzi & Refenes (2005)), which solves the puzzle under the equi-correlation assumption (i.e., all pairs within the implied correlation matrix share the same value). This purely ex-ante methodology was mixed with ex-post computations by researchers like Buss & Vilkov(2009, 2012), Numpacharoen & Numpacharoen (2013) or Driessen et al. (2013). Building on Skintzi & Refenes (2005)'s equicorrelation method, Linders & Schoutens (2014) provide an empirical analysis of the implied correlation surface with options of fixed maturities and different degrees of moneyness. They find that, just like for the implied volatility smile (cp. Mixon (2010)), the ex-ante correlation curve over degrees of moneyness is typically downward sloping. Without further tests nor discussion, they mention that "...the decreasing implied correlation curve may imply that the market expects stocks to go down simultaneously, while they are expected to go up more independently" (Linders & Schoutens (2014)). This statement is key cause for this study to not only develop a model that captures implied correlation duality, but also to investigate whether there is a link between the implied correlation surface and ex-ante correlation spreads.
Assume a financial market of n traded stocks i∈I={1,…,n} that are weighted according to the n×1 vector w to form the market portfolio m. There are financial options upon each single firm as well as the market index per se traded, such that respective prices can be used to express implied volatilities of the underlying.1 As implied volatilities are per definition forward looking, they serve as proxies for expected risk under the risk neutral measure.2 Given this framework, m's first two central moments are identical to its cumulants κ and computed as
κ1;m=μm=w′μand | (1) |
κ2;m=σ2m+κ21;mwithσ2m=w′DΓDw | (2) |
1For example, by Black-Scholes option pricing formula an observed call price can be iteratively solved to observe the implied volatility the derivative is priced under, see e.g. Schmalensee & Trippi (1978).
2Known in finance literature as Q-measure. Note that the study presented here makes all computations for Q dynamics. The reader interested in P expectations can apply the very same model, but has to subtract a variance risk premium from Q implied volatilities, see e.g. in Carr & Wu (2006).
where μ corresponds to the n×1 vector of expected stock returns, Γ to the n×n correlation matrix and D being a n×n diagonal matrix of implied volatilities, ∀i:Dii=σi. Since w is directly observable and σ2m as well as D can be easily estimated from option prices, CBOE's equicorrelation approach now sets all off-diagonal elements within Γ equal to an average level ˉc that resembles Equation 2, meaning that w′DΓDw≡w′DˉΓDw. CBOE's respective white paper (Skintzi & Refenes (2005)) suggests approximating ˉc by iteratively solving this equilibrium. However, different to that, Schadner (2020b) suggests a closed form solution that is computational more efficient. Let us introduce the n×n identity matrix E as well as J=1−E where 1 is a n×n unity matrix (consisting only of 1's). Thus, the equicorrelation matrix can be split up as
ˉΓ=(1ˉc⋯ˉc1⋯⋮⋮⋱)=ˉc(01⋯10⋯⋮⋮⋱)+(10⋯01⋯⋮⋮⋱)=ˉcJ+E∀i≠j:ˉcij=ˉc | (3) |
such that the implied correlation puzzle under the equicorrelation model is solved by
ˉc=σ2m−v0v1withv0:=w′D2w,v1:=w′DJDw | (4) |
which is basically one plausible solution to this ex-ante correlation puzzle. Nonetheless, as only n+1 implied volatilities are observable but there exist (n2−1)/n unknown correlation pairs, several other theoretically possible solutions exist. Say we fix option's time to maturity to some fixed target level τ (e.g., one month), and apply Equation 4 over different levels of moneyness d=ln(PstrikeP0).3 In the Black-Scholes market model, where higher than 2nd order moments are zero and correlations are symmetric, all implied volatilities would form a flat surface over all levels of τ and d. This assumption is clearly an unrealistic scenario, but still a good starting point hence it became common practice to compute Black-Scholes implied volatilities and fit regression splines to estimate higher moments (cp. Zhang & Xiang (2008)). With Equation 3, ˉc can be easily computed for different levels of d, which by plotting ˉc against d forms the implied correlation surface. Figure 1 is such an example of the S & P500 for the dates Dec. 31, 2007 and Sept. 30, 2015.
3P0 is the current price of the underlying, Pstrike the strike price of the option.
While in the Black-Scholes framework this curve should be flat, Linders & Schoutens (2014) find that empirically this curve is typically of negative slope. Without any proof, they mention that this smirk may be attributable to the fact that realized correlations during market downturns are on average higher than for up markets, and that the slope may be directly interpreted as investor expect correlation asymmetries. However, to the best of the author's knowledge, no study yet has explicitly analyzed whether this statement holds or not.
For the subsequent reading please consider the following notation: when used as syntax, + refers to positive returns (i.e., interval over [0,∞)); vice versa, − refers to negative returns (interval of (−∞,0)). Further, note that generally for any pdf of x it holds that
σ2=E[x2]−E[x]2 | (5) |
σ2+μ2=∫∞−∞x2f(x)dx | (6) |
=∫0−∞x2f(x)dx+∫∞0x2f(x)dx | (7) |
=PM−+PM+ | (8) |
with PM− as the 2nd order lower partial moment and PM+ as the 2nd order upper partial moment, both with a reference point equal to 0 such that PM− corresponds to negative returns and vice versa PM+ to positive ones.
Lemma 1. Correlation-duality causes skewness in the joint distribution of the portfolio.
Proof Consider a continuous multivariate normal random variable ˜X={˜x1,˜x2,...} which describes a set of correlated stocks I. The return vector of the market portfolio rm is thus given as the value weighted sum of ˜X, rm=w′˜X. If joint densities of ˜X are elliptical, then it is straight forward that the joint distribution rm will be normally distributed and the link between stocks can be efficiently expressed by their linear dependence, i.e. Pearson's correlation. In such a setting, PM−m can be (statistically unbiased) approximated by the value weighted aggregate of individual PMi's:3
PM−m≈w′L−ΓL−w≡w′L−ˉΓL−wL−n×n:{L−ij=PM−i∀i=j0∀i≠j | (9) |
^PM−m=ˉcl−1+l−0 | (10) |
4Like portfolio value-at-risk from the RiskMetrics model, see Longerstaey & Spencer (1996).
with ^PM−m as the unbiased approximate of PM−m and
l−1=w′L−JL−wandl−0=w′L−L−w | (11) |
Note that likewise D is a diagonal matrix of volatilities, L is a diagonal matrix of individual partial moments. Equations 9 to 11 are equivalent for the construction of PM+m, hence
^PM+m=ˉcl+1+l+0 | (12) |
What happens now if correlations are on average higher during market downturns (rm<0) than during market recovery (rm>0), say ˉc−>ˉc>ˉc+? To analyze this, we define the correlation-duality regime as follows:
corr{˜X}:={Γ+≡ˉc+J+Eifrm>0Γ−≡ˉc−J+Eifrm<0 | (13) |
Meaning that positive market returns are generated under Γ+ and vice versa with Γ−. Given so, the PMm's will now aggregate under different correlation parameter, that is,
^PM−m=ˉc−l−1+l−0and^PM+m=ˉc+l+1+l+0 | (14) |
Let us define Δˉc:=ˉc−−ˉc+ and note that l1's and l0's (Equation 11) are drawn from individual distributions, hence (mathematically) independent of Δˉc. Henceforth, by Equation 14 it follows that
iffΔˉc>0:^PM−m(ˉc−)>^PM−m(ˉc)and^PM+m(ˉc+)<^PM+m(ˉc) | (15) |
such that with an increasing correlation spread, probability mass within the joint distribution will be shifted from the positive to the negative tail. Henceforth, the joint distribution becomes asymmetric, which is in contradiction to the joint distribution being normal. In this sense, the larger the discrepancy between ˉc+ and ˉc−, the more will be one side of the mean be weighted against the other one. From this follows that correlation duality causes skewness in the return distribution of the market portfolio, even though if single constituents would be normally distributed. By Equation 15, if ˉc−>ˉc+ then median(rm)>μm which implies that rm will be negatively skewed; vice versa for ˉc−<ˉc+. Hence,
∂^PM−m∂Δˉc>0and∂^PM+m∂Δˉc<0⟹∂γm∂Δˉc<0a.s. | (16) |
means that the larger the correlation spread, the more negative the expected skew of the market portfolio becomes. This law should still hold if individual stock returns are not normally distributed, even if other aggregation procedures apply. Thus, X may be described by any realistic multivariate stock return distribution, it generally holds that ˉc+≠ˉc− creates skewness in the market portfolio.
Lemma 2. Correlation-duality causes portfolio skewness as it causes co-skewness among stocks.
Proof Consider the way Pearson's skewness is defined,
γ:=sσ3=E[(x−μσ)3]=E[x3]−3E[x2]μ+2μ3σ3=κ3−3κ2κ1+2κ31κ3/22 | (17) |
with s as the distribution's third (non-standardized) moment. The first two moments were already defined in Equation 2. For our market portfolio the observable term that remains is the third cumulant κ3;m which is computed from
κ3;m=w′κ3;X(w⊗w)≡∑i∑j∑kwiwjwksijk | (18) |
where κ3;X is the super-symmetric (non-standardized) skew-co-skewness matrix of size n×n2. This means that κ3;X is a combination of n many symmetric n×n matrices,
κ3;Xn×n2={κ3;1n×nκ3;2n×n⋯κ3;nn×n} | (19) |
κ3;m is thus equivalent to the value weighted sum of each possible skew/co-skewness term sijk. Like the co-variance matrix has entries for single firm variances, κ3;X also has entries that are linked to individual distribution's skewness. Specifically, firm i's skewness is captured at the ith position on the diagonal of the κ3;i sub-matrix,
individualskews:diag(κ3;i)i=si=γiσ3i∀i∈I | (20) |
All other elements within κ3;X that are not on such special positions are co-skewness terms. In the case of normally distributed stocks we faced individual skews equal zero, γi=0,∀i. Under non-normal distributions, individual skews may be different from zero, but still computable (from option data) on an individual level and thus independent of other firms. Therefore, one recognizes that correlation-duality can only drive cross-sectional co-skewness terms in order to cause skew in the aggregated portfolio. Being aware of that, one can distinguish γm generated from individual skew and γm caused by co-skewness. To get there, I develop an approach to compute the equi-co-skew from option data which is similar to the equicorrelation procedure before.
Let K be a n×n2 matrix with 0's on the positions indicated by Equation 20 and 1's otherwise. Respectively, 1n×n2−K indicates positions affected by individual skews. Likewise D contained firm volatilities, we capture individual skew loadings on the n×n diagonal matrix S. Hence the feasible solution to κ3;X with individual skews but equi-co-skews is given by
κ3;X=ˉsK⏟co−skews+S(1−K)⏟skews | (21) |
which implies
κ3;m=w′[ˉsK+S(1−K)](w⊗w) | (22) |
=ˉsw′K(w⊗w)⏟u1+w′S(1−K)(w⊗w)⏟u0 | (23) |
=ˉsu1+u0 | (24) |
With this simplification, portfolio skewness composes as
γm=1σ3m[ˉsu1+u0−3μmσ2m−μ3m] | (25) |
through which the equi-co-skew can be computed out of option data,
ˉs=1u1[γmσ3m−u0+3μmσ2m+μ3m] | (26) |
As far as the author is aware of this approach is first in finance literature to estimate expected co-skewness completely from ex-ante data and is perhaps interesting for several other applications. Back to our task, since all individual skew contributions are captured within u0 and co-skew effects within ˉsu1, by Equation 25 one can distinguish portfolio skewness generated from firm skews and co-skews as 5
γm=γm;co+u0σ3mwithγm;co:=ˉsu1σ3m−3μmσm−μ3mσ3m | (27) |
5Note that in the case of multivariate normal it holds that ˉs≠0. This is easily illustrated by ˜X∼N(⋅):s˜X=E[˜X3]−3E[˜X2]E[˜X]+2E[˜X]3 and since in this case it holds that u0=0andE[˜X3]=ˉsu1+u0≡3E[˜X2]E[˜X]−2E[˜X]3⟹s˜X=0iffˉs≠0⟹γ˜X=0.
Therefore, since
∂γm∂Δˉc<0andu0σ3m⊥Δˉc⟹∂γm;co∂Δˉc<0 | (28) |
it is shown that correlation-duality causes skewness as it generates co-skewness among stocks.
To quantify the magnitude of Δˉc on γm, one has to approximate the shape of market distribution. I do so by Gram-Charlier expansion using the first three moments, 6 whose cdf is generally given as
F(x)=∫∞−∞f(x)dx | (29) |
=∫∞−∞ϕ(x)[1+γ6He3(z)]dxHe3=z3−3z,z=x−μσ | (30) |
=∫∞−∞ϕ(x)dx+γ6∫∞−∞ϕ(x)He3(z)dx | (31) |
6As demonstrated above, correlation duality is a generator of asymmetry, thus I am not interested into excess kurtosis and assume even higher moments are neglectable small.
where ϕ(x) denotes the pdf of a normal distribution and He3 is the third Hermite polynomial. Taking Equation 5, for the market portfolio one writes
σ2m+μ2m⏟PM=∫∞−∞x2ϕ(x)dx⏟PMnorm+γm6∫∞−∞x2ϕ(x)He3(z)dx⏟PMcorr | (32) |
which can be interpreted as the total 2nd partial moment of the skewed joint distribution PM composes of a normally distributed part PMnorm and a correction term PMcorr. The split is convenient because for PMnorm we know that the aggregation formula of Equation 14 applies unbiased, such that it holds
PM−PMcorr=∫0−∞x2ϕ(x)dx+∫∞0x2ϕ(x)dx | (33) |
^PM−^PMcorr=ˉc−l−1+l−0+ˉc+l+1+l+0 | (34) |
Note that from option implied data, the parameters σ,γ,μ can be estimated, such that the integrals can be computed. In order to correct for individual skews, PMcorr is computed under γm;co (Equation 27) rather than total γm which should remove potential statistical biases related to non-symmetric marginal distributions. With this in mind, we can do the split
^PM−−^PM−corr=ˉc−l−1+l−0and^PM+−^PM+corr=ˉc+l+1+l+0 | (35) |
such that by rearranging terms, the average expected up and down correlation can be computed by
ˉc−=1l−1[^PM−−^PM−corr−l−0]andˉc+=1l+1[^PM+−^PM+corr−l+0] | (36) |
Lemma 3. The option implied correlation surface contains information about asymmetric expectations for up-/down correlations.
Proof It is generally known that a smile within the Black-Scholes implied volatility surface over different strikes refers to non-zero higher moments believes (cp. for example Bakshi et al. (2003), Mixon (2010)). Respectively, the slope within is (positively) connected to ex-ante skewness for which finance literature developed several methods to approximate. On the example of Gram-Charlier expansion, the volatility surface σ(d) over different levels of moneyness d can be interpolated as
σ(d)=σATM+γd6√τd:=ln(PstrikeP0) | (37) |
(cp. Jarrow & Rudd (1982)) with Pstrike as the option's strike level, P0 as the current price of the underlying and σATM as the at-the-money implied volatility (i.e., σ(Pstrike=P0)). In awareness of Equation 4, one is now able to write the implied volatility surface in terms of equi-correlations,
σm(d)=√ˉc(d)v1+v0 | (38) |
so combining these two equations, the implied correlation surface can be directly linked to market skewness, 7
(√ˉc(d)v1+v0−σm)6√τd=γm⟺ˉc(d)=1v1(σm+γmd6√τ)2−v0v1 | (39) |
7Note that for simplicity σm≡σm;ATM in my notation.
Therefore, substituting back in what we already know,
ˉc(d)=1v1[σm+(γm;co(ˉc+,ˉc−)+u0σ3m)d6√τ]2−v0v1 | (40) |
models the link between asymmetric expected correlations and the implied correlation surface. Since
∀d:∂γm;co∂Δˉc<0⟹∂ˉc(d)∂Δˉc<0a.s. | (41) |
it is shown that the implied correlation surface reflects that investors may have different believes for up- than for down correlations. Further, this will also confirm the statement of Linders & Schoutens (2014) that if ∂ˉc(d)∂d<0, then Δˉc>0
Besides the demonstrated Gram-Charlier case, within every risk-neutral approximation method available in literature, 8 γm is positively proportional to ∂σ(d)∂d such that the result of Equation 41 generally holds almost surely.
8It is common that a negative slope within the implied volatility surface corresponds to negative skewness believes (see e.g. Jarrow & Rudd (1982), Bakshi et al. (2003), Mixon (2010) or Bali et al. (2019))
Quality of the dual-correlation model is evaluated upon simulated data, where one can explicitly control for true up-/down correlations and backtest the estimation precision as if they were hidden. For this purpose, the simulation is broken down into two parts. I start by analyzing the joint distributional asymmetry caused through a correlation spread. Specifically, the impact of Δˉc on ΔPM and on portfolio skewness are quantified. Then I continue by evaluating the model precision itself, that is the error of true up-/down correlations versus those estimated through the Gram-Charlier approach. All computations executed within this section and in the empirical part were made using the statistical software R.
The simulation data is generated as follows. The number of stocks is set to n=100 and the time-series length to 1000, where one time step represents one trading day. For all stocks the mean return is defined to equal the risk free rate, which is set to 1% per year, thus ∀i:μi=rf=1%/252.9 Differently, stock volatilities are drawn from a random uniform distribution ranging between 10%/√252 and 50%/√252. Since real stock markets typically show a very unequally distribution of market values, w is created as
w=w0∑w0withw0(i)=1ln(i+1)∀i∈[1,n] | (42) |
9Same mean return for all stocks is required to meet Q dynamics. 1% p.a. divided by 252 trading days converts annual quotations to daily returns.
to better meet real world scenarios.10 With this setting, two correlated multivariate normal return matrices are generated, one under the true up correlation, r+∼N(μ,σ;ˉc+), and one under the true down correlation, r−∼N(μ,σ;ˉc−). ˉc+ is drawn from a uniform random distribution within [0.2,0.6] and respectively ˉc−∈[0.3,0.7]. Now, two portfolios are formed, r+p=w′r+ and r−p=w′r−, which serve as reference. The cross-sectional return matrix r with distinct up-/down correlations is now generated by combining the subsamples r+|r+p>0 and r−|r−p<0,
r:=[r+|r+p>0r−|r−p<0] | (43) |
10Visualization of input volatilities and weights can be found in the Appendix.
where rows are randomly re-sampled afterwards in order to remove systematic auto-correlation. Once r is generated, the return vector of the market portfolio simply evolves as rm=w′r. This procedure is then repeated a 1000 times to get a basket of simulated return data under different correlation spreads.
As discussed in Section 3, ceteris paribus, a difference in up- and down correlations is expected to cause asymmetric 2nd order partial moments in the joint distribution of the market portfolio. By the simulated data one is now able to explicitly verify this argument. For each of the 1000 simulated r matrices I compute PM−, PM+ and skewness of the respective market portfolio. Figure 2 visualizes the spread in partial moments as well as γm plotted against Δˉc, both plots come with a linearly fitted line to further emphasize the relation. Although every single firm's r is drawn from a normal distribution, both plots confirm that the joint distribution becomes asymmetric around its mean as soon as down-correlations are different from up-correlations. Note that Δˉc=0 leads to γm being on average 0, which is straight forward as it describes the mono-correlation regime-otherwise, if this picture would not evolve, then there would be an error in the generated data. The proper fit of the OLS lines indicate that there is no systematic statistical bias with respect to the approximation of the lower partial moments by the suggested Gram-Charlier expansion. By Equation 35 this linearity is an important feature.11 Goodness of fit of the regression lines are 0.91 and 0.86 with highly significant coefficients (t-statistics of 103.5, −78.0). Importantly, there does not seem to be any significant misestimation with respect to the level of the correlation spread, neither in ΔPM nor in γm. With the patterns found in Figure 2 Hypothesis 1 can be confirmed. Since all individual skews are equal to 0, the right plot of Figure 2 together with Equation 27 confirm Hypothesis 2.
11I confirm that Gram-Charlier expansion is suitable as both plots seem to result in (ⅰ) elliptical estimates, (ⅱ) do not show patterns of non-linearity and (ⅲ) residuals look to fulfill homoscedasticity. In the case of simulated data being drawn from a different distribution one may check whether the correction term of Equation 36 is sufficient to remove statistical biases, otherwise further specifications are required.
Having underpin Hypothesis 1 and 2, the question arises of how precise the proposed approximation method is. For this purpose I plot ex-ante specified up-/down correlations against estimated ones of Equation 36 and investigate whether there are systematic misestimations. Figure 3 does this plot. In addition, the displayed lines are of intercept 0 and slope of 1. Hence the lines serve as reference: the closer the estimates are on the line the higher the accuracy. Therefore, by Figure 3 one observes that estimation accuracy seems proper and there is no significant heteroscedasticity with respect to the level of true up-/down correlations. This conclusion is important in an econometric sense, as by such one can presume the model to be statistically unbiased. The estimation error for up-correlations ranged between −0.055 and 0.061 at a mean of 0.002, respective values for the down-scenario are −0.081, 0.071 and −0.0005.
Going further into details, one may be also interested whether there is a statistical bias related to the spread of correlations. Herein Figure 4 grasps the corresponding results. From visual inspection I am not able to identify any systematic misestimation, as residuals for both cases seem to be homoscedastic in Δˉc.
Concluding the Simulation, I find support in favor of Hypothesis 1 and 2. By inspecting residuals of estimated up-/down correlations, I presume that the proposed method delivers accurate and statistically unbiased estimates for ˉc+ and ˉc−. Hence I suggest the model to be robust and appropriate for econometric usage.
Within this section the discussed framework is applied upon empirical data. First, I start by computing time-series of expected up-, down- and mid- correlations and compare them to each other. Mid correlation is simply defined as the one used in Equation 3, that is CBOE's equi-correlation. Second, I analyze whether the implied correlation smirk as mentioned in Section 3 or Linders & Schoutens (2014) is indeed empirically linked to differences in expected up-/down correlations. Within the third part the model is used to demonstrate practical relevance upon an example of computing expected up- and down CAPM betas, which are frequently used by portfolio managers (but typically estimated ex-post).
All data are derived from Bloomberg L.P. and Thomson Reuter's Datastream. Computations are made upon the S & P 500 cross-section using option implied volatilities with a target maturity of 1 month for both the single stocks and the index itself. The observation horizon is Dec. 2007 to Sept. 2018 and the computations are rolled over on an end-of-monthly basis. Note that the input data reflect investor expectations under risk-neutrality (Q-measure), which can be transformed into P dynamics by explicitly modeling expected variance as well as correlation risk premia. However, this is out of the scope of this study and I would like to refer the reader interested into such a conversion to Carr & Wu (2009) or Fassas & Papadamou (2018).
Herein the described model of Section 3 is applied upon empirical data to compute time series of up and down correlations. Computations are made under the correction of firms' individual ex-ante skews, 12 see Equation 27. The results are visualized in Figure 5 and summarized in Table 1. Overall I find that for the entire observation horizon the correlation spread was positive with a slightly increasing trend. This means that the aggregate of investor expectations thoroughly presumed equity returns to be higher correlated for market downturns (rm<0) than for recoveries (rm>0). As can be seen in Figure 5, the correlation spread shows time-varying behavior and ranges between 0.074 and 0.303 with a mean of 0.167. Thus, non-surprisingly, a t-test of the spread results in Δˉc being significantly positive at a t-statistic of 41.18. When compared to CBOE's equicorrelation, the ˉc+ was on average 24.7% lower than ˉc, while ˉc− was 25% higher, hence diversification opportunities diminish in bearish markets. Concluding those observations, patterns found in empirical ex-post analyses (discussed in Section 2) are also reflected ex-ante within investor expectations, such that assuming a mono-correlation regime may cause statistical biases in financial analyses. This significant spread also underlines the importance of using a dual-correlation regime within practical applications.
Corr. | Min. | 1st Qu. | Median | Mean | 3rd Qu. | Max. |
up | 0.049 | 0.196 | 0.293 | 0.300 | 0.392 | 0.626 |
mid | 0.104 | 0.290 | 0.372 | 0.384 | 0.477 | 0.737 |
down | 0.161 | 0.367 | 0.453 | 0.468 | 0.555 | 0.854 |
Δˉc | 0.074 | 0.133 | 0.165 | 0.167 | 0.197 | 0.303 |
up/mid | -54.5% | -31.3% | -21.8% | -24.7% | -16.7% | -8.1% |
down/mid | 8.3% | 17.3% | 22.4% | 25.0% | 31.6% | 55.1% |
12Ex-ante skewness are estimated according to Jarrow & Rudd (1982), i.e. regression fit of Equation 37
In this context I believe it is further interesting to analyze correlation's and spread's dependency on market mood. With economic intuition one would argue that an risk averse investor trades a higher spread for bearish times (i.e., greater likelihood for downturns) than for confident times. For this purpose I use the sentiment index iH of Schadner (2020a)13 which is a measure of the magnitude and the direction of the market mood (optimistic/pessimistic). iH as originally presented is transformed in the following way, ~iH=2(iH−0.5) such that ~iH∈(−1,1), from which follows that ~iH is specified over the same interval as correlations are. ~iH is interpreted as the more positive ~iH becomes, the better the observed market mood; vice versa, if ~iH turns negative, then investors are pessimistic. Respective results of the conducted analysis can be found in Figure 6 and Table 2. Since it is documented that the level of average implied correlation seems to be dependent on the economic cycle (see Linders & Schoutens (2014)), I start by quantifying the link between ˉc and ~iH. From plotting empirical ~iH against ˉc and fitting a simple OLS regression, I find that there is a significant negative relation between those two variables with a coefficient of −0.717 (t-statistic of -18.56). Hence the finding coerces with the statement of Linders & Schoutens (2014). I further investigate how Δˉc changes with respect to changes in ~iH. If market participants are risk averse, then economic intuition would tell that correlation spreads increase if market fear rises and vice versa. Let Δt(Δˉc):=Δˉct−Δˉct−1 and Δt(iH):=~iHt−~iHt−1 express the time changes in correlation spreads and market mood, by plotting corresponding observations against each other (Figure 6, right plot) one can inspect that the suggested relation indeed holds in empirical data. Also for this setting, a simple OLS regression delivers a proper fit and indicates the regression's coefficients to be highly significant at a level of −0.225 and t-statistic of −7.828.
(1) levels | (2) time increments | ||
average corr. | Δt(Δˉc) | ||
~iH | −0.717∗∗∗ | Δt~iH | −0.225∗∗∗ |
(0.039) | (0.029) | ||
Intercept | 0.518∗∗∗ | Intercept | 0.001 |
(0.010) | (0.003) | ||
R2 | 0.729 | 0.325 | |
Note: *p < 0.1; **p < 0.05; ***p < 0.01 |
13Option implied Hurst exponent which is computed from the term-structure of S & P500 ATM implied volatilities.
An additional empirical analysis has been carried out with respect to the S & P 500 constituent turnover. The turnover is defined as the number of firms entering or exiting the index, respective decisions for the index are made on a quarterly basis. Hence, the data is split into quarters and for each sub-sample the turnover as well as quarter-average and end-of-quarter ˉc, Δˉc are computed. In a next step, Spearman dependencies between turnover and ˉc, Δˉc are estimated.14 The results indicate no monotonic relationship between the turnover and ˉc, estimates are −0.023 (q.-average) and −0.010 (end-of-q.). The relationships between turnover and implied spreads Δˉc are slightly negative at −0.161 (q.-average) and −0.212 (end-of-q.), but still in-significant. Therefore I conclude that there is no substantial dependence between turnover rates and implied correlations.
14Spearman instead of Pearson measure is used due to the smaller sample size.
Within the subsequent analysis it is evaluated whether Hypothesis 3 is met in empirical data or not. Specifically, time series of equicorrelations (Equation 3) are computed for moneyness levels of 90%, 95%, 100%, 105% and 110%; Figure 7 shows the respective output. Hypothesis 3 basically states that the duality spread (ˉc−−ˉc+; cp. Figure 5) has to increases when the lines in Figure 7 diverge, and diminish when the lines within the plot overlap. The test of Hypothesis 3 is broken down into two set-ups, a 'two-sided' one (Figure 8, upper plots) and a 'one-sided' one (Figure 8, lower plots). For the two-sided set-up both options of the OTM spread have a moneyness level different from 100%, while for the one-sided set-up one of the option's moneyness level is fixed to at-the-money and the other one is non-ATM. The reason to do so is the following, while with the two-sided test I evaluate whether there is a general connection between moneyness spreads and correlation duality, the one-sided test also evaluates whether the direction is correct. Let excess correlations be defined as estimates in excess of ˉc (i.e., mid-correlation computed under ATM level). By the economic argument of Linders & Schoutens (2014) as well as the sketched proof before, the excess down correlation has to be positively related to excess correlations of moneyness levels lower than 100%. Vice versa, excess correlations of moneyness > 100% should be positively related to the excess up-correlation. The two-sided spreads are chosen to be symmetric around ATM, thus I analyze the 95%–105% spread and the 90%–110% one. For the one-sided test implied estimates are compared for ±5% in excess of ATM. Results for the one-sided as well as two-sided set-up can be found in Figure 8 and Table 3. Generally, I find thorough empirical support for Hypothesis 3. As initially suggested, all four analyzes realize a significant positive relation between spreads from the correlation smile and spreads from estimated up-/down correlations. This does not only confirm the model, but also motivates to use (excess) correlations of the implied smile as proxies for expected up- and down correlations.
moneyness spread: | ||||
95% - 105% | 90% - 110% | 5% - 100% | 100% - 110% | |
Up-/Down Spread | 1.003∗∗∗ | 0.947∗∗∗ | ||
(ˉc−−ˉc+) | (0.137) | (0.174) | ||
Excess Down | 1.037∗∗∗ | |||
(ˉc−−ˉc) | (0.232) | |||
Excess Up | 1.003∗∗∗ | |||
(ˉc−ˉ2+) | (0.202) | |||
Intercept | 0.065∗∗∗ | 0.118∗∗∗ | 0.064∗∗∗ | −0.001 |
(0.024) | (0.030) | (0.020) | (0.017) | |
R2 | 0.294 | 0.188 | 0.135 | 0.162 |
Note: *p < 0.1; **p < 0.05; ***p < 0.01 |
From those observations I conclude the statement of Linders & Schoutens (2014) and thus also Hypothesis 3 to hold. Hence the implied correlation surface may be directly used for approximating expected up-/down correlations.
Within this section I highlight the use of the dual correlation regime upon the example of up- and down betas of the CAPM model (Sharpe (1964)), which are frequently used by investment practitioners in the context of corporate valuation, portfolio or risk management purposes. (cp. Chong & Phillips (2013)) Worth to mention, the beta-duality within this study is addressed via the channel of asymmetric correlation believes, an alternative (economic) approach is given by Campbell & Vuolteenaho (2004) who break CAPM beta into two components: a discount-rate beta and a cash-flow beta. Within their work, the former one is the "good" beta, the latter one the "bad" beta. At the dual-correlation model, up-betas are "good" (preferred by investors) and down-betas are "bad" (disfavored).15
15 A link between the two models exists if it can be proven that discount-rate and cash-flow news are drivers of asymmetric correlation believes. This proof requires an in-depth theoretical and empirical analysis and is out of scope of this study.
Under standard CAPM, a stock's beta βi is defined as
βi=cov(i,m)σ2m=ρi,mσiσmσ2m | (44) |
Thus, the extension for correlation duality is very simple; given volatilities as well as correlations are computed ex-ante, at time t, the vector of expected up betas β+ and down betas β− evolve from Equations 36 and 44 as
β+=1σ2mDΓ+Dw and β−=1σ2mDΓ−Dw | (45) |
which are computed on a monthly basis. Note that the use of implied data here has two main advantages. First, beta estimates are per construction time conditional as implied volatilities are. Second, since all input data are ex-ante, estimates reflect per definition investor expectations which is a key requirement of CAPM.
For the empirical analysis the correlation estimates from before are used to compute β+t and β−t for each t. Respectively, mid-betas (βmid) are calculated under the equicorrelation regime using ˉc. Therefore, I receive time-series for each of the three different beta vectors. Table 4 summarizes the resulting estimates over time and stocks. Since by Figure 5 the down correlation is mainly larger than its counterpart, it is not surprising that β+it's are on average below 1 and β−it's above 1. By expressing the estimates relative to βmid the picture is very similar to Table 3 from before. That is, the mono-correlation model would underestimate market risk exposure on average by 24.8%, while it overestimates upside potential by 24.5%. This conclusion fosters the relevance of such a dual-correlation regime for efficient portfolio risk management. Densities of β+ and β− are visualized in Figure 10. At Figure 9 time-series of the cross-sectional beta vectors are summarized, showing the 5% and 95% quantile as well as the mean of β+ and β−. As Δˉc is increasing over the observation horizon, it follows that average β+ decreases while β− increases over t. Interesting at this point, the discrepancy between up- and down betas is less during the time period of the financial crisis (2008).
Min. | 1st Qu. | Median | Mean | 3rd Qu. | Max. | |
β+ | 0.017 | 0.617 | 0.781 | 0.836 | 0.999 | 5.142 |
β− | 0.029 | 1.052 | 1.294 | 1.379 | 1.592 | 10.324 |
β+/βmid | -54.5% | -31.3% | -21.7% | -24.5% | -16.5% | -7.8% |
β−/βmid | 8.0% | 17.2% | 22.2% | 24.8% | 31.6% | 55.1% |
Henceforth it gets obvious that there is a clear difference between expected up- and down betas. To put it in a nutshell, the empirical application demonstrates that the proposed model of correlation duality is straight forward to implement and gives some interesting insights to financial market's behavior.
It is commonly known that equity correlations are typically higher during market down turns than for recovery times, which is not only documented in academic literature, but also frequently incorporated by investment practitioners for risk- and portfolio management purposes. Given this fact, this paper develops a model which allows to estimate expected up- and down correlations while relying purely on forward looking data (i.e., option implied volatilities). To the best of my knowledge, the method is first to be completely specified ex-ante and thus not affected by a backward looking bias, which allows to react faster to changes in the the current market situation. When validated on simulated data, the approach evolves to deliver reliable and unbiased estimates. Applied upon empirical data (S & P500, 2007–2018), several conclusions are derived. For example, expected down correlations are mainly higher than up correlations; the spread is on average 0.167. In greater detail, it is observed that this spread is time-varying and significantly dependent on current market mood: the worse, the greater the correlation spread. From this follows that stock diversification is expected to be more difficult when it is needed the most. By comparing estimated ex-ante up-/down correlations with the implied correlation surface I am able to confirm presumptions made in other studies, demonstrating that there is a theoretical as well as empirical link between them. To further highlight the practical relevance of a dual-correlation regime, empirical insights are provided that there is a substantial difference between expected up- and down CAPM betas. Additional potential practical implications of the implied correlation regime are for example at portfolio management, where one wants to control for down-side risk (e.g., value-at-risk) using such forward looking information. Another example could be in the context of policy research: implied volatility indices are highly affected by policy announcements (e.g., Rehman (2017), Shaikh (2019)), analyzing respective responses of implied up- and down-correlations is then likely to indicate how policy announcements affect changes in investor risk perceptions.
Most of the research for this work has been conducted during the author's former affiliation with the University of Innsbruck, Austria.
The author declares no conflicts of interest in this paper.
[1] | Anderson JE (1979) A theoretical foundation for the gravity equation. Am Econ Rev 69: 106-116. |
[2] |
Cartea Á, Jaimungal S, Ricci J (2014) Buy low, sell high: A high frequency trading perspective. SIAM J Financ Math 5: 415-444. doi: 10.1137/130911196
![]() |
[3] | Deardorff A (1998) Determinants of bilateral trade: does gravity work in a neoclassical world? In: The regionalization of the world economy, University of Chicago Press, 7-32. |
[4] | Dunis CL, Laws J, Naïm P (2004) Applied quantitative methods for trading and investment, John Wiley & Sons. |
[5] |
Evenett SJ, Keller W (2002) On theories explaining the success of the gravity equation. J Polit Econ 110: 281-316. doi: 10.1086/338746
![]() |
[6] | Feenstra RC (2015) Advanced international trade: theory and evidence, Princeton university press. |
[7] | Feenstra RC, Markusen JR, Rose AK (2001) Using the gravity equation to differentiate among alternative theories of trade. Can J Econ/Revue canadienne d'économique 34: 430-447. |
[8] |
Feuerriegel S, Prendinger H (2016) News-Based Trading Strategies. Decis Support Syst 90: 65-74. doi: 10.1016/j.dss.2016.06.020
![]() |
[9] |
Hafezi R, Shahrabi J, Hadavandi E (2015) A Bat-Neural Network Multiagent System (BNNMAS) for Stock Price Prediction: Case Study of DAX Stock Price. Appl Soft Comput 29: 196-210. doi: 10.1016/j.asoc.2014.12.028
![]() |
[10] |
Huang B, Huan Y, Xu LD, et al. (2019) Automated trading systems statistical and machine learning methods and hardware implementation: a survey. Enterp Inf Syst 13: 132-144. doi: 10.1080/17517575.2018.1493145
![]() |
[11] |
Isard W (1954) Location theory and trade theory: short-run analysis. Q J Econ, 305-320. doi: 10.2307/1884452
![]() |
[12] | Kaufman PJ (2005) The New Trading Systems and Methods, Wiley Trading. |
[13] | Krugman P (1983) New theories of trade among industrial countries. Am Econ Rev 73: 343-347. |
[14] |
Lee CM, Ready MJ (1991) Inferring trade direction from intraday data. J Financ 46: 733-746. doi: 10.1111/j.1540-6261.1991.tb02683.x
![]() |
[15] |
Loewenstein G, Thaler RH (1989) Anomalies: intertemporal choice. J Econ Perspect 3: 181-193. doi: 10.1257/jep.3.4.181
![]() |
[16] |
López-Laborda J, Peña G (2018) A New Method for Applying VAT to Financial Services. Natl Tax J 71: 155-182. doi: 10.17310/ntj.2018.1.05
![]() |
[17] |
Treleaven P, Galas M, Lalchand V (2013) Algorithmic trading review. Commun ACM 56: 76-85. doi: 10.1145/2500117
![]() |
Corr. | Min. | 1st Qu. | Median | Mean | 3rd Qu. | Max. |
up | 0.049 | 0.196 | 0.293 | 0.300 | 0.392 | 0.626 |
mid | 0.104 | 0.290 | 0.372 | 0.384 | 0.477 | 0.737 |
down | 0.161 | 0.367 | 0.453 | 0.468 | 0.555 | 0.854 |
Δˉc | 0.074 | 0.133 | 0.165 | 0.167 | 0.197 | 0.303 |
up/mid | -54.5% | -31.3% | -21.8% | -24.7% | -16.7% | -8.1% |
down/mid | 8.3% | 17.3% | 22.4% | 25.0% | 31.6% | 55.1% |
(1) levels | (2) time increments | ||
average corr. | Δt(Δˉc) | ||
~iH | −0.717∗∗∗ | Δt~iH | −0.225∗∗∗ |
(0.039) | (0.029) | ||
Intercept | 0.518∗∗∗ | Intercept | 0.001 |
(0.010) | (0.003) | ||
R2 | 0.729 | 0.325 | |
Note: *p < 0.1; **p < 0.05; ***p < 0.01 |
moneyness spread: | ||||
95% - 105% | 90% - 110% | 5% - 100% | 100% - 110% | |
Up-/Down Spread | 1.003∗∗∗ | 0.947∗∗∗ | ||
(ˉc−−ˉc+) | (0.137) | (0.174) | ||
Excess Down | 1.037∗∗∗ | |||
(ˉc−−ˉc) | (0.232) | |||
Excess Up | 1.003∗∗∗ | |||
(ˉc−ˉ2+) | (0.202) | |||
Intercept | 0.065∗∗∗ | 0.118∗∗∗ | 0.064∗∗∗ | −0.001 |
(0.024) | (0.030) | (0.020) | (0.017) | |
R2 | 0.294 | 0.188 | 0.135 | 0.162 |
Note: *p < 0.1; **p < 0.05; ***p < 0.01 |
Min. | 1st Qu. | Median | Mean | 3rd Qu. | Max. | |
β+ | 0.017 | 0.617 | 0.781 | 0.836 | 0.999 | 5.142 |
β− | 0.029 | 1.052 | 1.294 | 1.379 | 1.592 | 10.324 |
β+/βmid | -54.5% | -31.3% | -21.7% | -24.5% | -16.5% | -7.8% |
β−/βmid | 8.0% | 17.2% | 22.2% | 24.8% | 31.6% | 55.1% |
Corr. | Min. | 1st Qu. | Median | Mean | 3rd Qu. | Max. |
up | 0.049 | 0.196 | 0.293 | 0.300 | 0.392 | 0.626 |
mid | 0.104 | 0.290 | 0.372 | 0.384 | 0.477 | 0.737 |
down | 0.161 | 0.367 | 0.453 | 0.468 | 0.555 | 0.854 |
Δˉc | 0.074 | 0.133 | 0.165 | 0.167 | 0.197 | 0.303 |
up/mid | -54.5% | -31.3% | -21.8% | -24.7% | -16.7% | -8.1% |
down/mid | 8.3% | 17.3% | 22.4% | 25.0% | 31.6% | 55.1% |
(1) levels | (2) time increments | ||
average corr. | Δt(Δˉc) | ||
~iH | −0.717∗∗∗ | Δt~iH | −0.225∗∗∗ |
(0.039) | (0.029) | ||
Intercept | 0.518∗∗∗ | Intercept | 0.001 |
(0.010) | (0.003) | ||
R2 | 0.729 | 0.325 | |
Note: *p < 0.1; **p < 0.05; ***p < 0.01 |
moneyness spread: | ||||
95% - 105% | 90% - 110% | 5% - 100% | 100% - 110% | |
Up-/Down Spread | 1.003∗∗∗ | 0.947∗∗∗ | ||
(ˉc−−ˉc+) | (0.137) | (0.174) | ||
Excess Down | 1.037∗∗∗ | |||
(ˉc−−ˉc) | (0.232) | |||
Excess Up | 1.003∗∗∗ | |||
(ˉc−ˉ2+) | (0.202) | |||
Intercept | 0.065∗∗∗ | 0.118∗∗∗ | 0.064∗∗∗ | −0.001 |
(0.024) | (0.030) | (0.020) | (0.017) | |
R2 | 0.294 | 0.188 | 0.135 | 0.162 |
Note: *p < 0.1; **p < 0.05; ***p < 0.01 |
Min. | 1st Qu. | Median | Mean | 3rd Qu. | Max. | |
β+ | 0.017 | 0.617 | 0.781 | 0.836 | 0.999 | 5.142 |
β− | 0.029 | 1.052 | 1.294 | 1.379 | 1.592 | 10.324 |
β+/βmid | -54.5% | -31.3% | -21.7% | -24.5% | -16.5% | -7.8% |
β−/βmid | 8.0% | 17.2% | 22.2% | 24.8% | 31.6% | 55.1% |