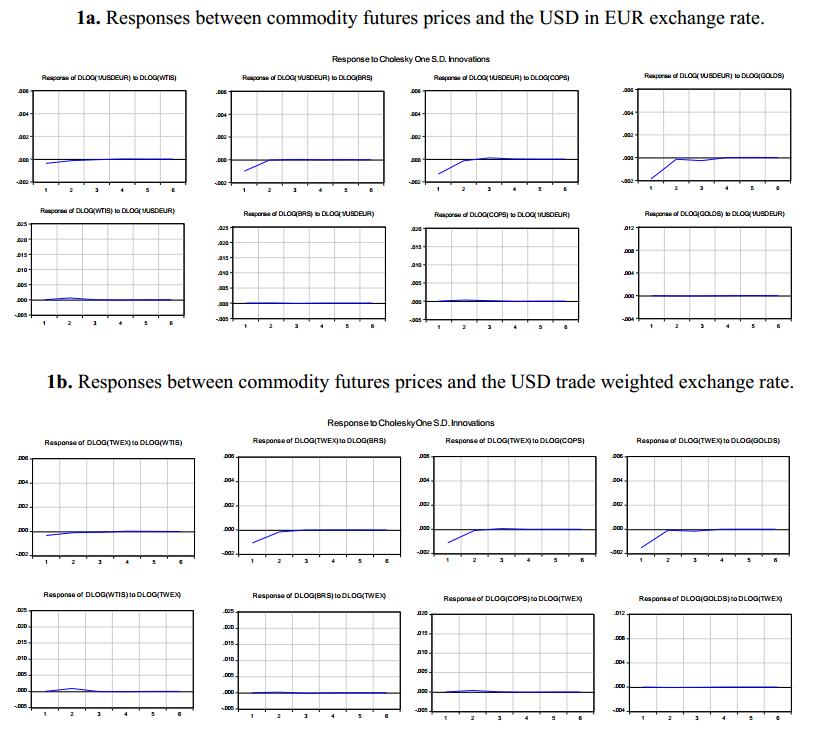
Citation: Lucjan T. Orlowski, Monika Sywak. Wavering interactions between commodity futures prices and us dollar exchange rates[J]. Quantitative Finance and Economics, 2019, 3(2): 221-243. doi: 10.3934/QFE.2019.2.221
[1] | Albert A. Agyemang-Badu, Fernando Gallardo Olmedo, José María Mella Márquez . Conditional macroeconomic and stock market volatility under regime switching: Empirical evidence from Africa. Quantitative Finance and Economics, 2024, 8(2): 255-285. doi: 10.3934/QFE.2024010 |
[2] | Yonghong Zhong, Richard I.D. Harris, Shuhong Deng . The spillover effects among offshore and onshore RMB exchange rate markets, RMB Hibor market. Quantitative Finance and Economics, 2020, 4(2): 294-309. doi: 10.3934/QFE.2020014 |
[3] | Takashi Kanamura . Diversification effect of commodity futures on financial markets. Quantitative Finance and Economics, 2018, 2(4): 821-836. doi: 10.3934/QFE.2018.4.821 |
[4] | Joseph Atta-Mensah . Commodity-linked bonds as an innovative financing instrument for African countries to build back better. Quantitative Finance and Economics, 2021, 5(3): 516-541. doi: 10.3934/QFE.2021023 |
[5] | Tolga Tuzcuoğlu . The impact of financial fragility on firm performance: an analysis of BIST companies. Quantitative Finance and Economics, 2020, 4(2): 310-342. doi: 10.3934/QFE.2020015 |
[6] | Kim Hiang Liow, Jeongseop Song, Xiaoxia Zhou . Volatility connectedness and market dependence across major financial markets in China economy. Quantitative Finance and Economics, 2021, 5(3): 397-420. doi: 10.3934/QFE.2021018 |
[7] | Melike E. Bildirici, Mesut M. Badur . The effects of oil prices on confidence and stock return in China, India and Russia. Quantitative Finance and Economics, 2018, 2(4): 884-903. doi: 10.3934/QFE.2018.4.884 |
[8] | Isaac O. Ajao, Hammed A. Olayinka, Moruf A. Olugbode, OlaOluwa S. Yaya, Olanrewaju I. Shittu . Long memory cointegration and dynamic connectedness of volatility in US dollar exchange rates, with FOREX portfolio investment strategy. Quantitative Finance and Economics, 2023, 7(4): 646-664. doi: 10.3934/QFE.2023031 |
[9] | Lukáš Pichl, Taisei Kaizoji . Volatility Analysis of Bitcoin Price Time Series. Quantitative Finance and Economics, 2017, 1(4): 474-485. doi: 10.3934/QFE.2017.4.474 |
[10] | Zheng Nan, Taisei Kaizoji . Bitcoin-based triangular arbitrage with the Euro/U.S. dollar as a foreign futures hedge: modeling with a bivariate GARCH model. Quantitative Finance and Economics, 2019, 3(2): 347-365. doi: 10.3934/QFE.2019.2.347 |
Our study examines the interplay between returns on selected commodities (changes in logs of commodity futures prices) and the US Dollar exchange rates. The underlying hypothesis is that shocks in commodity futures settlement prices inversely affect US Dollar (USD) values in foreign currencies. In other words, rising commodity futures prices result in USD depreciation and declining prices in USD appreciation. Based on key findings in the prior literature, we assume that the impact of commodity futures prices on the exchange rate varies significantly in time. This causal impact is particularly sensitive to financial market risk conditions. Specifically, at normal market periods USD depreciation is associated with rising commodity prices, while at times of financial distress, i.e. under high market risk conditions, USD appreciation corresponds with higher commodity prices.
We focus our analysis on two crude oil and two metals one-month futures settlement prices that have been widely discussed in the literature as more or less significantly related to exchange rate movements. Specifically, the commodities included in our exercise are: West Texas Intermediate (WTI) and Brent crude oil, copper and gold. We use the USD value in EUR and USD Trade Weighted (TWEX) exchange rates. We test causal interactions and impulse responses between commodity futures prices and exchange rates. We employ linear multiple breakpoint regression to examine their changes over time. We assume that returns on crude oil futures and the exchange rates are very sensitive to market risk conditions. Our underlying assumption is that at normal, low market risk periods the two pairs of returns display an inverse relationship, while at turbulent times their relationship becomes positive. As suggested by several recent studies (Lizardo and Mollick, 2010; Ding and Vo, 2012; Reboredo, 2012), these relationships hold well for all examined commodity futures prices in relation to USD in EUR and TWEX, albeit mainly in the aftermath of the recent financial crisis, i.e. in the presence of massive liquidity injections to financial markets.
Our analytical assumption is based on key findings in several prior studies. The literature examining relationships between commodity spot and futures prices and exchange rates is extensive. We assume that there is a prevalent causal impact of changes in commodity futures prices on the USD exchange rate, which is consistent with a similar directional inference discussed by Lizardo and Mollick (2010), Ding and Vo (2012), and Fratzscher, et al. (2014).
We begin with a brief survey of pertinent literature in Section 2. Section 3 presents the data description and the analysis of bi-variate causal relationships between changes in logs (returns) to commodity prices and USD exchange rates by testing them with Bayesian vector autoregression (BVAR) with impulse response functions. The prevalent causal impact of changes in commodity prices on the USD exchange rates allows us to devise an underlying analytical model presented in Section 4. The model is empirically tested with Bai-Perron multiple breakpoint (MBP) regressions in Section 5. MBP enables us to identify discernible phases in the changeable relationships between commodity futures and the exchange rates. We gain insights on their time varying patterns by estimating Two-State Markov Switching Models (MSM) shown and discussed in Section 6. The concluding Section 7 summarizes key findings of our study and presents some policy recommendations.
The literature examining the relationships between commodity spot as well as futures prices and USD exchange rates is extensive and it seems to follow two research streams. The first of them is consistent with our analytical assumptions and empirical findings assuming a causal impact of changes in commodity prices on the exchange rate. The second stream follows reversed causal effects, assuming a prevalent impact of changes in the USD exchange rate on commodity prices.
In essence, our model and empirical tests are based on the first causal reaction, i.e. on the transmission of shocks in commodity futures prices on the exchange rate, as implied by the BVAR tests and impulse response functions discussed below. The causal effects of changes in commodity prices on exchange rates are evidenced among others by Lizardo and Mollick (2010). They show that crude oil prices significantly and continuously explain changes in the USD exchange rate. Reboredo (2012), Ding and Vo (2012) and Chiang, et al. (2014) expand this analysis by demonstrating that such causal impact became stronger during the recent financial crisis. We add to this debate by showing reversals in such inference. While at normal periods increasing commodity futures prices entail the USD depreciation, they result in the USD appreciation at times of financial distress. In our analysis, this direct relationship is transmitted via higher market risk during turbulent market conditions that lead to the USD appreciation.
There are several studies in the literature that assume a reverse causal relationship between commodity futures prices and exchange rates, i.e. a transmission of changes in the exchange rate onto commodity prices. Among others, Sadorsky (2000) shows that futures prices of crude oil, heating oil and gasoline are strongly co-integrated with the trade-weighted exchange rate. Based on a structural VAR analysis of 1990–2007 quarterly data, Akram (2009) argues that a weaker dollar leads significantly to higher commodity prices. Chen et al. (2010) show that exchange rates have a strong predictive power in forecasting changes in global commodity prices. They argue that the opposite causal reaction is notably less robust. Coudert and Mignon (2016) argue that there is a negative transmission of changes in the dollar exchange rate into real oil price, except for the mid-2000s when this transmission becomes positive.
There is a notable distinction between short-run and long-run effects of changes in commodity futures prices on the exchange rate. Among others, Bénassy-Quéré et al. (2005) argue that oil prices significantly and inversely affect the USD exchange rate in the short-run, but their relationship becomes direct in the long-run. Yet, their analysis is based on monthly data ending in 2004 and may not hold for the more recent period much affected by the recent global financial crisis and its resolution policies. In newer studies, Allegret et al.(2015, 2017) show that real currency appreciation following demand-driven rise in oil prices affects only selected countries and their exchange rates and they argue that the proportional role of individual macroeconomic and institutional factors affecting oil prices has changed over time.
There are several studies supporting the second stream of the literature that assumes prevalence of a causal impact of changes in the USD exchange rate on commodity prices. Based on historical evidence of co-movements between oil prices and exchange rates, Zhang et al. (2008) as well as Zhang (2013) show that changes in the USD exchange rate inversely affect changes in oil prices. However, they also show that sudden surges in exchange rate volatility have no impact on fluctuations in oil prices. Similarly, Wu et al. (2012) as well as Beckmann and Czudaj (2013) provide some evidence that the USD depreciation against major currencies results in a corresponding increase in oil prices, although this functional relationship is subject to right-skewness, i.e. prevalence of positive over negative shocks, as well as leptokurtosis (tail risks)1. In a similar vein, Sari et al. (2010) provide evidence of short run responses of changes in metal future prices and weaker response of oil prices to fluctuations in USD exchange rates.
1 For the analysis of tail risks in various financial markets during the 2008–2010 financial crisis see Orlowski (2012).
As a compromise to the discussion about prevalence of causal effects in the relationships between commodity (spot and futures) prices and USD exchange rate, Fratzscher et al. (2014) argue that there is a pronounced causality between oil prices and USD exchange rate in both directions. Nevertheless, they concur that the USD depreciation is brought about by positive shocks in oil prices and this directional effect has been prevalent. They further prove that the negative correlation between oil prices and USD exchange rates has become recently become stronger due to higher market risk triggered by the recent financial crisis. As a result, crude oil and its derivatives have gained importance as global financial assets. In an earlier study, Breitenfellner and Cuaresma (2008) also demonstrated an increasing association between oil prices and exchange rates, attributing it to improved accuracy of forecasts of both commodity prices and exchange rate. The strengthening impact of commodity prices on exchange rates and on other macroeconomic variables stemming from greater stability of their changes and improved forecast accuracy is also proven by Joëts, et al. (2015).
In sum, the literature seems to imply increasing inference of changes in commodity futures prices on the USD exchange rate. Under normal, low risk market conditions, this relationship is inverse, i.e. increasing commodity prices entail USD depreciation, and decreasing prices are associated with the USD appreciation. This functional relationship becomes direct during turbulent, high market risk times. We contribute to the literature by testing the causal effects, varied intensity and stability of these functional interactions between commodity futures prices and USD exchange rate in subsequent sections. While the majority of published empirical studies on this subject are based on low (quarterly or monthly) data frequency, we conduct our empirical tests on daily data. Moreover, we employ the multiple breakpoint regression and the Markov switching process to identify precise daily breakpoints and switching episodes in the tested series.
Before devising a model examining association between commodity futures prices and the USD exchange rates, we intend to analyze causal directions and transmission of shocks between these variables. For this purpose, we employ Bayesian vector autoregression (BVAR) analysis and the corresponding impulse reaction functions on our two crude oil and metal prices and separately, USD in EUR and trade weighted USD exchange rates.
As a basis for BVAR and subsequent tests in our study, we use daily data on futures settlement prices and average exchange rates for a sample period January 5, 1999–August 12, 2016 (4401 observations). The beginning of our sample period is determined by the inception of EUR in January 1999. The data are obtained from Bloomberg and Federal Reserve Bank of St. Louis—Federal Reserve Economic Data (FRED). All variables in our empirical exercises are stationary, as they are entered in changes in logs, i.e. captured as percent returns. The order of our BVAR tests is optimized for the number of response lags by minimizing the Akaike information criterion (AIC) at different lag specifications. AIC results suggest a BVAR optimization with 2 lagged terms in each of the examined cases. Our BVAR(2) tests assume Monte Carlo distribution of error terms. From BVAR(2) tests, we derive un-accumulated impulse responses that are shown in Figures 1a and 1b.
The results shown in Figure 1a indicate that the change in logs of USD value in EUR responds inversely to one-standard deviation shocks in commodity futures prices, as displayed in the upper-row diagrams. The opposite causal reactions of commodity prices to the exchange rate are indiscernible (the lower-row diagrams). Brent prices' response is stronger than that of WTI prices. The responses of metal futures, i.e. copper and gold, are stronger than those for crude oil futures. As shown by impulse response functions in Figure 1b, the causal reactions of futures prices to the USD trade weighted exchange rate are almost identical to the responses to USD in EUR.
We note that our BVAR tests and impulse response functions are consistent with the directional inference suggested by Lizardo and Mollick (2010), Ding and Vo (2012) and Reboredo (2012). We assume that there is a discernible transmission of market-implied inflation expectations onto commodity futures prices and ultimately to the exchange rate. The first "leg" of this transmission, i.e. a strong causal impact of changes in the 5-year and the 10-year breakeven inflation to changes in commodity futures prices, is proven empirically by Orlowski (2017)2. Moreover, our causal interactions are reversed to those implied by Zhang et al. (2008), Wu et al. (2012) as well as Beckmann and Czekaj (2013), all of whom showing prevalence of a casual inference from nominal USD exchange rates to oil prices. They also demonstrate that these responses are sensitive to sample periods, market risk conditions and testing (data generating) specifications.
2 Breakeven inflation reflects inflation expectations of government bond markets participants, as it is derived from the spread between yield on government bonds and the rates on the corresponding maturity TIPS (Treasury InflationProtected Securities).
In sum, we detect pronounced and rather instantaneous inverse responses of exchange rates to changes in commodity futures prices. Specifically, positive shocks in all four commodity prices entail a USD depreciation, with a one-day lag. Recognizing the prevalence of such causal reactions, we devise an underlying analytical function for further, more specific empirical tests.
Taking into consideration the transmission of shocks from commodity futures prices to the USD exchange rates, we devise the following functional relationship that is a basis for the remainder of our analysis:
Δloget=β0+β1Δlog(CPt)+εt | (1) |
with
We fundamentally agree that the relationships between commodity futures prices and USD exchange rates are not uniform over time. They are particularly sensitive to market risk and market liquidity conditions, among other influential factors which in-depth examination is beyond the scope of our analysis3. In order to account for different patterns in the relationship prescribed by Equation 1 at tranquil vs. turbulent markets, we introduce the Chicago Board Options Exchange VIX market volatility variable into the examined functional relationship in the following form. We augment Equation 1 with a dummy variable DVIX that assumes the value of 1 at turbulent market periods when VIX exceeds the threshold of 24 and 0 for the tranquil market days of VIX remaining below the threshold. We have identified the VIX threshold of 24 by running the Bai-Perron Threshold estimation of the stochastic VIX series for the entire sample period, permitting just one structural break. The threshold test has identified 3350 tranquil market days, i.e. VIX oscillating below the obtained threshold, and 1050 days of turbulent markets.
3 A number of studies examine a broad range of macroeconomic and institutional factors affecting commodity prices and exchange rates. Worth noting studies addressing these issues include Blanchard and Gali (2007), Fraetzscher et al. (2014), and Joëts et al. (2017).
The modified functional relationship that accounts for market turbulence by adding the
Δloget=β0+β1Δlog(CPt)+β2DVIX+β3Δlog(CPt)∗DVIX+ε′t | (2) |
The interactive term
We have conducted a number of empirical tests of the functional relationships represented by Equation 2. The results of the linear MBP estimations as well as non-parametric MSM tests are shown and discussed in the subsequent sections. We choose only the most robust estimations that are optimized by minimizing the Akaike information criterion.
We tests the functional relationships between the exchange rates and futures price with the Bai-Perron multiple breakpoints (MBP) regressions in order to identify possible discernible phases in individual functional relationships. The estimation results for the tests of the USD in EUR as a function of commodity futures prices based on Equation 2 are shown in Tables 1a and 1b.
Phases based on break points | Changes in the USD in EUR exchange rate as a function of changes in log of WTI price | Phases based on break points | Changes in USD in EUR exchange rate as a function of changes in log of Brent price | ||||||
|
|
|
|
|
|
|
|
||
Phase Ⅰ 1/05/1999 – 1/23/2003 (998 obs) |
0.001 (1.41) |
0.015 (1.29) |
-0.001 (-1.56) |
-0.001 (-0.07) |
Phase Ⅰ 1/05/1999 – 1/22/2003 (986 obs) |
0.001 (1.42) |
0.010 (0.84) |
-0.001 (-1.45) |
0.016 (0.94) |
Phase Ⅱ 1/24/2003 – 3/18/2009 (1535) |
-0.001 (-0.78) |
-0.042*** (-4.88) |
0.001 (0.22) |
-0.010 (-0.84) |
Phase Ⅱ 1/23/2003 – 3/18/2009 (1547) |
-0.001 (-0.71) |
-0.051*** (-5.64) |
-0.001 (-0.21) |
-0.019 (-1.46) |
Phase Ⅲ 3/19/2009 – 3/22/2013 (1012) |
0.001 (0.52) |
-1.151*** (-10.82) |
-0.001 (-0.10) |
-0.006 (-0.32) |
Phase Ⅲ 3/19/2009 – 3/22/2013 (1012) |
0.002 (0.74) |
-0.149*** (-9.56) |
-0.001 (-0.36) |
-0.017 (-0.91) |
Phase Ⅳ 3/25/2013 – 8/12/2016 (855) |
0.002 (0.88) |
-0.035*** (-3.89) |
0.001 (0.09) |
0.108*** (5.14) |
Phase Ⅳ 3/25/2013 – 8/12/2016 (855) |
0.002 (0.90) |
-0.030*** (-3.16) |
0.001 (0.49) |
0.115*** (5.06) |
Diagnostic statistics: | |||||||||
F-statistics Log likelh. AIC DW |
23.529 16166 -7.341 1.981 |
22.495 16159 -7.338 1.984 |
|||||||
Notes: t-statistics in parentheses; *** denotes significance at 1%, ** at 5%, * at 10%. Daily data for a sample period January 5, 1999–August 12, 2016 (4401 observations). Source: Authors' own estimation based on Bloomberg and the Federal Reserve Bank of St. Louis FRED daily data. |
Phases based on break points | Changes in USD in EUR exchange rate a function of changes in log of copper prices | Phases based on break points | Changes in USD in EUR exchange rate as a function of gold prices | ||||||
|
|
|
|
|
|
|
|
||
Phase Ⅰ 1/05/1999 - 5/24/2002 (835 obs) |
0.001 (1.49) |
0.018 (0.75) |
-0.001 (-0.95) |
-0.056 (-1.51) |
Phase Ⅰ 1/05/1999 - 4/16/2002 (808 obs) |
0.001 * (1.87) |
-0.083** (-2.49) |
-0.001 (-1.01) |
-0.064 (-1.36) |
Phase Ⅱ 5/28/2002 - 5/19/2005 (736) |
-0.001 (0.58) |
-0.121*** (-7.81) |
-0.001 (-0.97) |
0.267*** (6.82) |
Phase Ⅱ 4/17/2002 - 9/02/2005 (836) |
-0.001 (-0.33) |
-0.441*** (-21.48) |
-0.006 (-1.54) |
0.114*** (2.89) |
Phase Ⅲ 5/20/2005 - 3/18/2008 (710) |
-0.001 (-1.40) |
-0.023*** (-2.58) |
-0.002 (-0.37) |
-0.015 (-0.66) |
Phase Ⅲ 9/06/2005 - 11/02/2009 (1048) |
-0.001 (-0.02) |
-0.178*** (-9.74) |
0.001 (0.01) |
-0.033 (-1.29) |
Phase Ⅳ 3/19/2008 – 3/23/2013 (1266) |
0.001 (0.78) |
-0.187*** (-11.68) |
-0.001 (-0.17) |
0.048** (2.55) |
Phase Ⅳ 11/03/2009 - 8/12/2016 (1708) |
0.001 (0.74) |
-0.208*** (-14.45) |
0.001 (1.17) |
0.164*** (5.72) |
Phase Ⅴ 3/27/2013 - 8/12/2016 (853) |
0.001 (0.69) |
-0.093*** (-5.81) |
-0.001 (-0.09) |
0.265*** (5.58) |
|||||
Diagnostic statistics: | |||||||||
F-statistics Log likelh. AIC DW |
27.893 16246 -7.375 1.969 |
59.471 16403 -7.449 2.011 |
|||||||
Note: Source as in Table 1a. |
The results shown in Table 1a reflect the MBP estimation of the USD in EUR exchange rate as a function of crude oil prices. There are four discernible periods, separated by three breakpoints for both WTI and Brent prices. Incidentally, the timing of these breakpoints is almost identical in both cases and the results are quite similar. There is no significant relationship between crude oil prices and USD in EUR exchange rate in the early period, i.e. in Phase 1 that begins January 5, 1999 and end January 22 (for Brent) and January 23 (for WTI) of 2003. In Phase 2 capturing the period between late January 2003 and mid-March 2009, there is an inverse, statistically significant relationship between both crude oil prices and the USD in EUR exchange rate. Specifically, an increase in oil futures prices is associated with the USD depreciation, although the estimated values of
The interactive term is significant only during the most recent period, i.e. in Phase Ⅳ. Its estimated
Similar results are obtained in the MBP estimation of the relationship between the USD in EUR and metals prices shown in Table 1B. The MBP estimation identifies five discernible periods for the copper series and four for the gold series. The timing of breakpoints is different in this case. Nevertheless, the absolute values of the estimated
In order to insulate the factors specific to the euro and the euro-denominated assets from our analytical framework, we examine the relationship between commodity prices and the USD trade weighted exchange rate. The results of the MBP regression tests for changes in logs of USD trade weighted exchange rate as a function of WTI and Brent crude oil prices are shown in Table 2A. The tests identify three breakpoints (four distinctive phases) for WTI series and just one breakpoint (two phases) for Brent. Phase Ⅰ for the WTI series, capturing a January 5, 1999–January 23, 2003 subperiod, shown no relationship of crude oil prices and the USD exchange rate. In Phase Ⅱ and Ⅲ, we observe an inverse relationship between the tested variables. This relationship is stronger in Phase Ⅲ than in the preceding period, suggesting a strong association between decreasing WTI prices (from their peak in early July 2008) and USD appreciation. During the most recent period of October 5, 2012–August 12, 2016 (Phase Ⅳ), this inverse relationship becomes somewhat weaker, as implied by the lower estimated absolute value of
Phases based on break points | Changes in the USD trade weighted exchange rate as a function of changes in log of WTI price | Phases based on break points | Changes in USD trade weighted exchange rate as a function of changes in log of Brent price | ||||||
|
|
|
|
|
|
|
|
||
Phase Ⅰ 1/05/1999 - 1/23/2003 (998 obs) |
0.001 (1.41) |
0.001 (0.07) |
-0.001 (-0.44) |
0.001 (0.08) |
Phase Ⅰ 1/05/1999 - 8/16/2007 (2134 obs) |
-0.001 (-0.77) |
-0.025*** (0-4.61) |
0.001 (0.44) |
0.021** (2.28) |
Phase Ⅱ 1/24/2003 - 3/18/2009 (1535) |
-0.001 (-0.99) |
-0.042*** (-6.14) |
0.001 (0.79) |
-0.005 (-0.53) |
Phase Ⅱ 8/17/2007 - 3/18/2009 (2266) |
0.001 (0.88) |
-0.073*** (-12.47) |
-0.001 (-0.90) |
-0.002 (-0.26) |
Phase Ⅲ 3/19/2009 - 10/04/2012 (896) |
-0.001 (-0.33) |
-0.119*** (-11.00) |
-0.001 (-0.16) |
-0.016 (-1.11) |
|||||
Phase Ⅳ 10/05/2012 - 8/12/2016 (971) |
0.001 (1.54) |
-0.051*** (-8.11) |
0.001 (0.96) |
0.074*** (5.01) |
|||||
Diagnostic statistics: | |||||||||
F-statistics Log likelh. AIC DW |
33.829 17664 -8.022 2.035 |
50.526 17594 -7.994 2.047 |
|||||||
Note: Source: as in Table 1a. |
It is worth noting that the
The results of the MBP estimations of Equation 2 for USD trade weighted exchange rate as a function of copper and gold futures settlement prices are shown in Table 2B. The relationship between copper prices and the exchange rate is not significant during the earliest sub-period, i.e. in Phase Ⅰ. It is statistically significant with a negative sign during the remaining sub-periods, indicating a pronounced inverse relationships in Phase Ⅱ (August 6, 2002–May 18, 2005) and Ⅳ (March 19, 2008–November 23, 2012) and a weaker association in Phase Ⅲ and Ⅴ. Turbulent market conditions have a significant positive effect on the relationship between copper and USD trade weighted exchange rate in Phase Ⅱ and Ⅴ and these results are fully consistent with the MBP estimation of the USD in EUR exchange rate series in Table 1B. A similar consistency takes place in estimation of gold prices and exchange rates. However this time, in the case of the USD trade weighted exchange rate, there is a statistically significant reversal in the impact of turbulent markets on the examined relationship between Phases Ⅰ and Ⅱ. During the episodes of high market risk, higher gold prices were associated with the USD depreciation in Phase Ⅰ, while they became linked with the USD appreciation in Phase Ⅱ and again in Phase Ⅳ, but not during the eve and the peak of the financial crisis captured by Phase Ⅲ.
Phases | Changes in USD trade weighted ex. rate as a function of copper prices | Phases | Changes in USD trade weighted ex. rate as a function of gold prices | ||||||
|
|
|
|
|
|
|
|
||
Phase Ⅰ 1/05/1999 - 8/05/2002 (881 obs) |
0.001 (0.45) |
0.010 (0.78) |
0.001 (0.05) |
-0.037* (-1.89) |
Phase Ⅰ 1/05/1999 - 4/16/2002 (808 obs) |
0.001 (1.11) |
-0.048*** (-2.65) |
0.001 (0.20) |
-0.065*** (-2.56) |
Phase Ⅱ 8/06/2002 - 5/18/2005 (690) |
-0.001 (-0.64) |
-0.111*** (-9.39) |
-0.001 (-0.76) |
0.221*** (7.00) |
Phase Ⅱ 4/17/2002 - 9/02/2005 (836) |
-0.001 (-0.69) |
-0.357*** (-22.90) |
-0.001 (-0.99) |
0.126*** (4.31) |
Phase Ⅲ 5/19/2005 - 3/18/2008 (710) |
-0.001 (-1.69) |
-0.020*** (-2.94) |
0.001 (0.09) |
-0.007 (-0.39) |
Phase Ⅲ 9/06/2005 - 11/02/2009 (1048) |
0.001 (0.02) |
-0.150*** (-10.27) |
0.001 (0.07) |
-0.024 (-1.19) |
Phase Ⅳ 3/19/2008 – 11/23/2012 (1183) |
0.001 (0.22) |
-0.150*** (-12.46) |
0.001 (0.06) |
0.026* (1.84) |
Phase Ⅳ 11/03/2009 - 8/12/2016 (1708) |
0.001 (0.73) |
-0.192*** (-19.27) |
0.001 (1.48) |
0.132*** (6.69) |
Phase Ⅴ 11/26/2012 - 8/12/2016 (936) |
0.001 (1.32) |
-0.089*** (-7.95) |
0.001 (0.01) |
0.163*** (4.73) |
|||||
Diagnostic statistics: | |||||||||
F-statistics Log likelh. AIC DW |
40.691 17781 -8.073 2.021 |
84.596 17983 -8.167 2.078 |
|||||||
Note: Source as in Table 1a. |
In sum, our tests show prevalence of an inverse relationship between commodity prices and USD exchange rates. However, during the most recent period, i.e. in the aftermath of global financial crisis, their relationships switches from negative to significantly positive during episodes of high market risk, i.e. when VIX exceeds the obtained threshold of 24. The normal inverse relationship between increasing (decreasing) commodity prices and USD depreciation (appreciation) switches to their positive co-movement at times of financial distress, with a reversed interaction between gold price and USD trade weighted exchange rate during the early sample period of 1999–2002. We recognize that it is not possible to consider and find a unified phase division in the tested relationships, since we include diversified (energy and metal) commodity futures that react differently to changes in economic fundamentals and exchange rates. Therefore, the breakpoints remain intrinsically heterogeneous.
In order to examine stability and the exact time pattern of the multiple breakpoint regression estimation for the USD exchange rates as a function of commodity prices, we employ a Two-State Markov Switching Model. Its estimation also enables us to show directional changes and stability of either direct or inverse relationships between both pairs of variables during the entire examined sample period.
A two-state Markov switching process to simulate is specified as follows:
The process in State 1 is specified as:
Δloget|St=1=c1+γ1ΔlogCPt+ε1tε1t→N(0,1) | (3) |
We expect the process estimated for State (or "Regime") 1 to follow a seemingly different relationship between the returns to the exchange rate and commodity futures prices during the examined sample period to that obtained for State ("Regime") 2. The process reflecting State or Regime 2 is prescribed by
Δloget|St=2=c2+γ2ΔlogCPt+ε2tε2t→N(0,1) | (4) |
The corresponding transition probability matrix is specified as:
P=[p11p21p12p22] | (5) |
The results of the Markov switching estimation for change in log of the USD in EUR exchange rate as a function of changes in log of WTI and Brent futures prices are shown in Table 3. The estimations are augmented with a log sigma as a common term.
Changes in USD in EUR ex. rate as a function of changes in WTI price | Changes in USD in EUR ex. rate as a function of changes in Brent price | |
Regime Ⅰ | |
|
Regime Ⅱ | |
|
Common terms: Log Sigma | ||
-5.144*** (-419.9) | -5.102*** (-457.0) | |
Diagnostic tests: | Log likelihood = 16140 Akaike Info. Criterion = -7.333 Durbin Watson stats. = 1.977 |
Log likelihood = 16142 Akaike Info. Criterion = -7.334 Durbin Watson stats. = 1.994 |
Constant transition probabilities, | ||
Probability of staying (switching): | ||
Regime Ⅰ Regime Ⅱ |
0.78 (0.22) 0.49 (0.51) |
0.99 (0.01) 0.01 (0.99) |
Constant expected durations: | ||
Regime Ⅰ Regime Ⅱ |
4.6 days 2.0 days |
205 days 105 days |
Notes: as in Table 1, z-statistics in parentheses. Source: as in Table 1. |
The obtained States or Regimes from the Markov switching estimations for the WTI and Brent series are somewhat different. In the case of WTI futures prices, Regime Ⅰ indicates a rather weak, positive relationship (a low
The regimes for Brent futures are more divergent. Regime Ⅰ is prescribed by an inverse, albeit statistically insignificant co-movement. Regime Ⅱ reflects a strong, positive relationship. However, the more ambiguous relationship prescribed by Regime Ⅰ overwhelmingly dominates the process with its 99 percent probability of remaining in it on any given day and its expected duration of 205 days. Evidently, the co-movement between Brent futures and USD in EUR exchange rate is normally not robust, although it becomes stronger and significant at less prevalent times prescribed by Regime Ⅱ.
Estimations of Markov switching processes for changes in (logs of) USD in EUR exchange rate as a function of changes in (logs of) copper and gold futures prices are shown in Table 4. The relationship between copper futures prices and the exchange rate is mainly positive. Regime Ⅰ depicts a weaker and Regime Ⅱ considerably stronger positive interactions. Both
Changes in USD in EUR ex. rate as a function of changes in copper price | Changes in USD in EUR ex. rate as a function of changes in gold price | |
Regime Ⅰ | |
|
Regime Ⅱ | |
|
Common terms: | -5.140*** (-424.2) | -5.102*** (-457.0) |
Log Sigma | ||
Diagnostic tests: | Log likelihood = 16185 Akaike Info. Criterion = -7.354 Durbin Watson stats. = 1.989 |
Log likelihood = 16142 Akaike Info. Criterion = -7.334 Durbin Watson stats. = 1.994 |
Constant transition probabilities, | ||
Probability of staying (switching): | ||
Regime Ⅰ | 0.72 (0.23) | 0.97 (0.03) |
Regime Ⅱ | 0.16 (0.83) | 0.98 (0.02) |
Constant expected durations: | ||
Regime Ⅰ | 3.6 days | 32 days |
Regime Ⅱ | 1.2 days | 49 days |
Notes: as in Table 1, z-statistics in parentheses. Source: as in Table 1. |
One of the key, rather unexpected findings of our study are observed in Table 5 that shows relationships between changes in (logs of) USD trade weighted exchange rate and changes in (logs of) crude oil futures prices. Somewhat contrary to the results in Table 3, this relationship is mainly inverse for both WTI and Brent. In both cases Regime Ⅰ reflects a milder inverse co-movement, while Regime Ⅱ shows considerably stronger inverse relationships. All regime trajectories are statistically significant. The dispersion of results found in Tables 3 and 5 implies a significant negative impact of crude oil futures prices on USD values transmitted via other currencies included in the USD trade weighted basket, primarily via the British Pound. In both WTI and Brent cases, Regimes Ⅰ, i.e. those reflecting somewhat milder inverse interactions, dominate the process with their longer expected duration and lower switching probability.
Changes in USD trade weighted ex. rate as a function of changes in WTI price | Changes in USD trade weighted ex. rate as a function of changes in Brent price | |
Regime Ⅰ | |
|
Regime Ⅱ | |
|
Common terms: | ||
AR(1) Log Sigma | -0.021 (-1.32) -5.469*** (-413.0) |
NA -5.483*** (-447.4) |
Diagnostic tests: | Log likelihood = 17665 Akaike Info. Criterion = -8.029 Durbin Watson stats. = 1.980 |
Log likelihood = 17641 Akaike Info. Criterion = -8.015 Durbin Watson stats. = 2.040 |
Constant transition probabilities, | ||
Probability of staying (switching): | ||
Regime Ⅰ Regime Ⅱ |
0.98 (0.02) 0.95 (0.05) |
0.85 (0.15) 0.31 (0.69) |
Constant expected durations: | ||
Regime Ⅰ Regime Ⅱ |
54 days 21 days |
6.5 days 1.5 days |
Notes: as in Table 1, z-statistics in parentheses. Source: as in Table 1. |
A similar reversal from positive to inverse interactions is observed in estimations of changes in USD trade weighted exchange rate as a function of copper and gold futures prices shown in Table 6. Both regimes in the case of copper futures prices in relation to the USD trade weighted exchange rate indicate prevalence of an inverse relationship, in contrast to the direct relationship for the USD in EUR series. Regime Ⅱ implies a milder inverse co-movement and Regime Ⅰ a considerably stronger inverse relationship. However, Regime Ⅱ is dominant with its longer expected duration and a bit higher probability of remaining in it. The Markov switching relationship for gold futures prices is dominated by Regime Ⅰ reflecting a milder inverse relationship. All obtained estimated
Changes in USD trade weighted ex. rate as a function of changes in copper price | Changes in USD trade weighted ex. rate as a function of changes in gold price | |
Regime Ⅰ | |
|
Regime Ⅱ | |
|
Common terms: Log Sigma | -5.507*** (-448.2) | -5.579*** (-452.8) |
Diagnostic tests: | Log likelihood = 17733 Akaike Info. Criterion = -8.057 Durbin Watson stats. = 2.036 |
Log likelihood = 18051 Akaike Info. Criterion = -8.202 Durbin Watson stats. = 2.077 |
Constant transition probabilities, | ||
Probability of staying (switching): | ||
Regime Ⅰ Regime Ⅱ |
0.75 (0.25) 0.79 (0.21) |
0.95 (0.05) 0.92 (0.08) |
Constant expected durations: | ||
Regime Ⅰ Regime Ⅱ |
1.3 days 4.8 days |
19 days 12 days |
Notes: as in Table 1, z-statistics in parentheses. Source: as in Table 1. |
Further insights in stability and reliability of the obtained Markov switching regimes can be derived from Figures 2a-d and 3a-d showing one-step ahead regime probabilities for the USD in EUR and USD trade weighted exchange rates respectively. The regime probabilities path shows in Figure 2a implies an orderly pattern of both regimes in the relationship between WTI futures and USD in EUR exchange rate. There are only minor discernible switching episodes around the peak of the financial crisis at the end of 2008 and the instability of the euro stemming from the sovereign debt crisis in the euro area in 2012–2013. The switching pattern for Brent futures prices vis-à-vis USD in EUR exchange rate two regimes is rather disorderly, as shown in Figure 2b. The regimes were rather stable only at the early stage of the sample period in 1999–2002. Time distribution of predicted regime probabilities for crude oil futures prices as a function of the USD trade weighted exchange rate shown in Figures 3a and 3b is exactly reverse for WTI and Brent. Both identified regimes for WTI series in relation to USD trade weighted exchange rate are very unstable (Figure 3a), while the regimes for Brent show a remarkable stability (Figure 3b).
The patterns of predicted regime switching probabilities for copper and gold futures prices in relation to USD in EUR exchange rate (Figures 2c and 2d) and USD trade weighted exchange rate (Figures 3c and 3d) are very similar. The switching patterns in the case of copper are rather orderly. There are minor switching episodes only in the case of the USD and EUR exchange rate series (Figure 2c) around the peak of the recent crisis and the timing of the euro area sovereign debt crisis. No discernible switching episodes are observed for the copper series as a function of the USD trade weighted exchange rate. In contrast, the patterns for gold series are very unstable in relation to both exchange rates. There are several regime reversals in the case of gold and USD in EUR exchange rate series, particularly during the first half of the entire sample period, i.e. between 1999 and 2007. The pattern for gold in relation to USD trade weighted exchange rate (Figure 3d) is very unsettled through the entire sample period.
In sum, the Markov switching estimations indicate rather unstable interactions between crude oil as well as gold futures prices and USD exchange rates. Stability of the identified regimes for copper futures prices and both USD exchange rates is considerably better. Both WTI and Brent crude oil futures prices are positively related with USD in EUR values. They are inversely related to the USD values on the basis of the trade weighted exchange rate, being presumably strongly affected by fluctuations in other exchange rates.
We examine the impact of changes in commodity one-month futures prices on USD exchange rates. Changes in WTI and Brent crude oil futures prices are inversely related with the value of USD in EUR and the USD trade weighted exchange rate. The impact of changes in WTI on USD exchange rates becomes positive under turbulent market conditions that we define as the days of CBOE VIX exceeding the value of 24. However, this positive effect holds only during the most recent sample period of October 5, 2012–August 12, 2016. Changes in copper and gold prices are also inversely related to changes in USD values in EUR and the trade weighted USD exchange rate. We also observe a positive interaction between changes in the two examined metal futures prices and the USD exchange rates during turbulent market conditions, with the exception of the 1999–2002 and 2005–2009 sub-periods.
In essence, market interactions between returns on commodity futures and the exchange rates are not uniform for the examined two crude oils and two metals and exchange rates. There is a correlation between the price of commodity futures and the exchange rates but the relationship is subject to structural breaks over time. The interplay between these returns is very sensitive to the market risk conditions. At normal market periods, i.e. low market risk conditions, there is a significant inverse relationship between commodity futures and exchange rate returns.
These key findings of our study are derived from Bayesian VAR and Bai-Perron multiple breakpoint tests. We further test for dynamic properties of the examined series by employing a stationary Two-State Markov switching process. We find unstable interactions between crude oil as well as gold futures prices and USD exchange rates. Stability of the identified regimes for copper futures prices and both USD exchange rates is considerably better.
In hindsight, we find that USD exchange rates are driven by changes in commodity prices, albeit to varied degrees. Such interaction can be viewed as a special case of the purchasing power parity theory of exchange rate movements where exchange rate changes are affected by the expected price movement in commodity prices.
Although our empirical exercise is focused only on the relationships between four selected commodity futures prices and USD exchange rates, we recognize importance of other factors affecting broader commodity futures prices and exchange rates. Their intricate relationships deserve thorough examination in future research.
We are indebted to Michael J. Gorman, David M. Kemme, Bluford N. Putnam, Carolyne C. Soper and the anonymous referees for their useful comments and suggestions to earlier versions of our paper. All possible errors and omissions are ours only.
The authors declare no conflict of interest in this paper.
[1] |
Akram QF (2009) Commodity prices, interest rates and the dollar. Energy Econ 31: 838–851. doi: 10.1016/j.eneco.2009.05.016
![]() |
[2] |
Allegret JP, Mignon V, Sallenave A (2015) Oil price shocks: Lessons from a model with trade and financial interdependencies. Econ Model 49: 232–247. doi: 10.1016/j.econmod.2015.04.009
![]() |
[3] |
Allegret JP, Couharde C, Mignon V, et al. (2017) Oil currencies in the face of oil shocks: What can be learned from time-varying specifications? Appl Econ 49: 1774–1793. doi: 10.1080/00036846.2016.1226490
![]() |
[4] |
Beckmann J, Czudaj R (2013) Oil prices and effective dollar exchange rates. Int Rev Econ Financ 27: 621–636. doi: 10.1016/j.iref.2012.12.002
![]() |
[5] | Bénassy-Quéré A, Mignon V, Penot A (2005) China and the relationship between the oil price and the dollar. CEPII Working Paper No. 2005–16. |
[6] | Blanchard OJ, Gali J (2007) The macroeconomic effects of oil shocks: Why are the 2000s so different from the 1970s?. NBER Working Paper No. 13368. |
[7] | Breitenfellner A, Crespo-Cuaresma J (2008) Crude oil prices and the USD/EUR exchange rate. Monetary Policy Econ Q 4. |
[8] | Chen YC, Rogoff KS, Rossi B (2010) Can exchange rates forecast commodity prices? Q J Econ 125: 1145–1194. |
[9] |
Chiang I-HE, Hughen WK, Sagi JS (2015) Estimating oil risk factors using information from equity and derivatives markets. J Financ 70: 769–804. doi: 10.1111/jofi.12222
![]() |
[10] |
Coudert V, Mignon V (2016) Reassessing the empirical relationship between the oil price and the dollar. Energy Policy 95: 147–157. doi: 10.1016/j.enpol.2016.05.002
![]() |
[11] |
Ding L, Vo M (2012) Exchange rates and oil prices: A multivariate volatility analysis. Q Rev Econ Financ 52: 15–37. doi: 10.1016/j.qref.2012.01.003
![]() |
[12] | Fratzscher M, Schneider D, van Robays I (2014) Oil prices, exchange rates and asset prices. European Central Bank Working Paper No. 1689. |
[13] |
Joëts M, Mignon V, Razafindrabe T (2017) Does the volatility of commodity prices reflect macroeconomic uncertainty? Energy Econ 68: 313–326. doi: 10.1016/j.eneco.2017.09.017
![]() |
[14] |
Lizardo RA, Mollick AV (2010) Oil price fluctuations and US dollar exchange rates. Energy Econ 32: 399–408. doi: 10.1016/j.eneco.2009.10.005
![]() |
[15] |
Orlowski LT (2012) Financial crisis and extreme market risks: Evidence from Europe. Rev Financ Econ 21: 120–130. doi: 10.1016/j.rfe.2012.06.006
![]() |
[16] |
Orlowski LT (2015) Monetary expansion and bank credit: A lack of spark. J Policy Model 37: 510–520. doi: 10.1016/j.jpolmod.2015.03.013
![]() |
[17] | Orlowski LT (2017) Volatility of commodity futures prices and market-implied inflation expectations. J Int Financ Mark Inst Money 51: 133–141. |
[18] |
Reboredo JC (2012) Modeling oil price and exchange rate co-movements. J Policy Model 34: 419–440. doi: 10.1016/j.jpolmod.2011.10.005
![]() |
[19] |
Sadorsky P (2000) The empirical relationship between energy futures prices and exchange rates. Energy Econ 22: 253–266. doi: 10.1016/S0140-9883(99)00027-4
![]() |
[20] |
Sari R, Hammoudeh S, Soytas U (2010) Dynamics of oil price, precious metal prices and exchange rate. Energy Econ 32: 351–362. doi: 10.1016/j.eneco.2009.08.010
![]() |
[21] |
Wu CC, Chung H, Chang YH (2012) The economic value of co-movement between oil price and exchange rate using copula-based GARCH models. Energy Econ 34: 270–282. doi: 10.1016/j.eneco.2011.07.007
![]() |
[22] | Zhang YJ (2013) The links between the price of oil and the value of US Dollar. Int J Energy Econ Policy 3: 341–351. |
[23] |
Zhang YJ, Fan Y, Tsai HT, et al. (2008) Spillover effect of US dollar exchange rate on oil prices. J Policy Model 30: 973–991. doi: 10.1016/j.jpolmod.2008.02.002
![]() |
1. | Zhifeng Dai, Huiting Zhou, Fenghua Wen, Shaoyi He, Efficient predictability of stock return volatility: The role of stock market implied volatility, 2020, 52, 10629408, 101174, 10.1016/j.najef.2020.101174 | |
2. | Xian Chen, Yang Li, Jihong Xiao, Fenghua Wen, Oil shocks, competition, and corporate investment: Evidence from China, 2020, 89, 01409883, 104819, 10.1016/j.eneco.2020.104819 | |
3. | Liming Chen, Ziqing Du, Yong Tan, Sustainable exchange rates in China: Is there the heterogeneous effect of economic policy uncertainty?, 2019, 1, 2643-1092, 346, 10.3934/GF.2019.4.346 | |
4. | Tangyong Liu, Xu Gong, Boqiang Lin, Analyzing the frequency dynamics of volatility spillovers across precious and industrial metal markets, 2021, 41, 0270-7314, 1375, 10.1002/fut.22217 | |
5. | Chi Wei Su, Xin Yue Song, Meng Qin, Oana-Ramona Lobonţ, Is copper a safe haven for oil?, 2024, 91, 03014207, 104897, 10.1016/j.resourpol.2024.104897 |
Phases based on break points | Changes in the USD in EUR exchange rate as a function of changes in log of WTI price | Phases based on break points | Changes in USD in EUR exchange rate as a function of changes in log of Brent price | ||||||
|
|
|
|
|
|
|
|
||
Phase Ⅰ 1/05/1999 – 1/23/2003 (998 obs) |
0.001 (1.41) |
0.015 (1.29) |
-0.001 (-1.56) |
-0.001 (-0.07) |
Phase Ⅰ 1/05/1999 – 1/22/2003 (986 obs) |
0.001 (1.42) |
0.010 (0.84) |
-0.001 (-1.45) |
0.016 (0.94) |
Phase Ⅱ 1/24/2003 – 3/18/2009 (1535) |
-0.001 (-0.78) |
-0.042*** (-4.88) |
0.001 (0.22) |
-0.010 (-0.84) |
Phase Ⅱ 1/23/2003 – 3/18/2009 (1547) |
-0.001 (-0.71) |
-0.051*** (-5.64) |
-0.001 (-0.21) |
-0.019 (-1.46) |
Phase Ⅲ 3/19/2009 – 3/22/2013 (1012) |
0.001 (0.52) |
-1.151*** (-10.82) |
-0.001 (-0.10) |
-0.006 (-0.32) |
Phase Ⅲ 3/19/2009 – 3/22/2013 (1012) |
0.002 (0.74) |
-0.149*** (-9.56) |
-0.001 (-0.36) |
-0.017 (-0.91) |
Phase Ⅳ 3/25/2013 – 8/12/2016 (855) |
0.002 (0.88) |
-0.035*** (-3.89) |
0.001 (0.09) |
0.108*** (5.14) |
Phase Ⅳ 3/25/2013 – 8/12/2016 (855) |
0.002 (0.90) |
-0.030*** (-3.16) |
0.001 (0.49) |
0.115*** (5.06) |
Diagnostic statistics: | |||||||||
F-statistics Log likelh. AIC DW |
23.529 16166 -7.341 1.981 |
22.495 16159 -7.338 1.984 |
|||||||
Notes: t-statistics in parentheses; *** denotes significance at 1%, ** at 5%, * at 10%. Daily data for a sample period January 5, 1999–August 12, 2016 (4401 observations). Source: Authors' own estimation based on Bloomberg and the Federal Reserve Bank of St. Louis FRED daily data. |
Phases based on break points | Changes in USD in EUR exchange rate a function of changes in log of copper prices | Phases based on break points | Changes in USD in EUR exchange rate as a function of gold prices | ||||||
|
|
|
|
|
|
|
|
||
Phase Ⅰ 1/05/1999 - 5/24/2002 (835 obs) |
0.001 (1.49) |
0.018 (0.75) |
-0.001 (-0.95) |
-0.056 (-1.51) |
Phase Ⅰ 1/05/1999 - 4/16/2002 (808 obs) |
0.001 * (1.87) |
-0.083** (-2.49) |
-0.001 (-1.01) |
-0.064 (-1.36) |
Phase Ⅱ 5/28/2002 - 5/19/2005 (736) |
-0.001 (0.58) |
-0.121*** (-7.81) |
-0.001 (-0.97) |
0.267*** (6.82) |
Phase Ⅱ 4/17/2002 - 9/02/2005 (836) |
-0.001 (-0.33) |
-0.441*** (-21.48) |
-0.006 (-1.54) |
0.114*** (2.89) |
Phase Ⅲ 5/20/2005 - 3/18/2008 (710) |
-0.001 (-1.40) |
-0.023*** (-2.58) |
-0.002 (-0.37) |
-0.015 (-0.66) |
Phase Ⅲ 9/06/2005 - 11/02/2009 (1048) |
-0.001 (-0.02) |
-0.178*** (-9.74) |
0.001 (0.01) |
-0.033 (-1.29) |
Phase Ⅳ 3/19/2008 – 3/23/2013 (1266) |
0.001 (0.78) |
-0.187*** (-11.68) |
-0.001 (-0.17) |
0.048** (2.55) |
Phase Ⅳ 11/03/2009 - 8/12/2016 (1708) |
0.001 (0.74) |
-0.208*** (-14.45) |
0.001 (1.17) |
0.164*** (5.72) |
Phase Ⅴ 3/27/2013 - 8/12/2016 (853) |
0.001 (0.69) |
-0.093*** (-5.81) |
-0.001 (-0.09) |
0.265*** (5.58) |
|||||
Diagnostic statistics: | |||||||||
F-statistics Log likelh. AIC DW |
27.893 16246 -7.375 1.969 |
59.471 16403 -7.449 2.011 |
|||||||
Note: Source as in Table 1a. |
Phases based on break points | Changes in the USD trade weighted exchange rate as a function of changes in log of WTI price | Phases based on break points | Changes in USD trade weighted exchange rate as a function of changes in log of Brent price | ||||||
|
|
|
|
|
|
|
|
||
Phase Ⅰ 1/05/1999 - 1/23/2003 (998 obs) |
0.001 (1.41) |
0.001 (0.07) |
-0.001 (-0.44) |
0.001 (0.08) |
Phase Ⅰ 1/05/1999 - 8/16/2007 (2134 obs) |
-0.001 (-0.77) |
-0.025*** (0-4.61) |
0.001 (0.44) |
0.021** (2.28) |
Phase Ⅱ 1/24/2003 - 3/18/2009 (1535) |
-0.001 (-0.99) |
-0.042*** (-6.14) |
0.001 (0.79) |
-0.005 (-0.53) |
Phase Ⅱ 8/17/2007 - 3/18/2009 (2266) |
0.001 (0.88) |
-0.073*** (-12.47) |
-0.001 (-0.90) |
-0.002 (-0.26) |
Phase Ⅲ 3/19/2009 - 10/04/2012 (896) |
-0.001 (-0.33) |
-0.119*** (-11.00) |
-0.001 (-0.16) |
-0.016 (-1.11) |
|||||
Phase Ⅳ 10/05/2012 - 8/12/2016 (971) |
0.001 (1.54) |
-0.051*** (-8.11) |
0.001 (0.96) |
0.074*** (5.01) |
|||||
Diagnostic statistics: | |||||||||
F-statistics Log likelh. AIC DW |
33.829 17664 -8.022 2.035 |
50.526 17594 -7.994 2.047 |
|||||||
Note: Source: as in Table 1a. |
Phases | Changes in USD trade weighted ex. rate as a function of copper prices | Phases | Changes in USD trade weighted ex. rate as a function of gold prices | ||||||
|
|
|
|
|
|
|
|
||
Phase Ⅰ 1/05/1999 - 8/05/2002 (881 obs) |
0.001 (0.45) |
0.010 (0.78) |
0.001 (0.05) |
-0.037* (-1.89) |
Phase Ⅰ 1/05/1999 - 4/16/2002 (808 obs) |
0.001 (1.11) |
-0.048*** (-2.65) |
0.001 (0.20) |
-0.065*** (-2.56) |
Phase Ⅱ 8/06/2002 - 5/18/2005 (690) |
-0.001 (-0.64) |
-0.111*** (-9.39) |
-0.001 (-0.76) |
0.221*** (7.00) |
Phase Ⅱ 4/17/2002 - 9/02/2005 (836) |
-0.001 (-0.69) |
-0.357*** (-22.90) |
-0.001 (-0.99) |
0.126*** (4.31) |
Phase Ⅲ 5/19/2005 - 3/18/2008 (710) |
-0.001 (-1.69) |
-0.020*** (-2.94) |
0.001 (0.09) |
-0.007 (-0.39) |
Phase Ⅲ 9/06/2005 - 11/02/2009 (1048) |
0.001 (0.02) |
-0.150*** (-10.27) |
0.001 (0.07) |
-0.024 (-1.19) |
Phase Ⅳ 3/19/2008 – 11/23/2012 (1183) |
0.001 (0.22) |
-0.150*** (-12.46) |
0.001 (0.06) |
0.026* (1.84) |
Phase Ⅳ 11/03/2009 - 8/12/2016 (1708) |
0.001 (0.73) |
-0.192*** (-19.27) |
0.001 (1.48) |
0.132*** (6.69) |
Phase Ⅴ 11/26/2012 - 8/12/2016 (936) |
0.001 (1.32) |
-0.089*** (-7.95) |
0.001 (0.01) |
0.163*** (4.73) |
|||||
Diagnostic statistics: | |||||||||
F-statistics Log likelh. AIC DW |
40.691 17781 -8.073 2.021 |
84.596 17983 -8.167 2.078 |
|||||||
Note: Source as in Table 1a. |
Changes in USD in EUR ex. rate as a function of changes in WTI price | Changes in USD in EUR ex. rate as a function of changes in Brent price | |
Regime Ⅰ | |
|
Regime Ⅱ | |
|
Common terms: Log Sigma | ||
-5.144*** (-419.9) | -5.102*** (-457.0) | |
Diagnostic tests: | Log likelihood = 16140 Akaike Info. Criterion = -7.333 Durbin Watson stats. = 1.977 |
Log likelihood = 16142 Akaike Info. Criterion = -7.334 Durbin Watson stats. = 1.994 |
Constant transition probabilities, | ||
Probability of staying (switching): | ||
Regime Ⅰ Regime Ⅱ |
0.78 (0.22) 0.49 (0.51) |
0.99 (0.01) 0.01 (0.99) |
Constant expected durations: | ||
Regime Ⅰ Regime Ⅱ |
4.6 days 2.0 days |
205 days 105 days |
Notes: as in Table 1, z-statistics in parentheses. Source: as in Table 1. |
Changes in USD in EUR ex. rate as a function of changes in copper price | Changes in USD in EUR ex. rate as a function of changes in gold price | |
Regime Ⅰ | |
|
Regime Ⅱ | |
|
Common terms: | -5.140*** (-424.2) | -5.102*** (-457.0) |
Log Sigma | ||
Diagnostic tests: | Log likelihood = 16185 Akaike Info. Criterion = -7.354 Durbin Watson stats. = 1.989 |
Log likelihood = 16142 Akaike Info. Criterion = -7.334 Durbin Watson stats. = 1.994 |
Constant transition probabilities, | ||
Probability of staying (switching): | ||
Regime Ⅰ | 0.72 (0.23) | 0.97 (0.03) |
Regime Ⅱ | 0.16 (0.83) | 0.98 (0.02) |
Constant expected durations: | ||
Regime Ⅰ | 3.6 days | 32 days |
Regime Ⅱ | 1.2 days | 49 days |
Notes: as in Table 1, z-statistics in parentheses. Source: as in Table 1. |
Changes in USD trade weighted ex. rate as a function of changes in WTI price | Changes in USD trade weighted ex. rate as a function of changes in Brent price | |
Regime Ⅰ | |
|
Regime Ⅱ | |
|
Common terms: | ||
AR(1) Log Sigma | -0.021 (-1.32) -5.469*** (-413.0) |
NA -5.483*** (-447.4) |
Diagnostic tests: | Log likelihood = 17665 Akaike Info. Criterion = -8.029 Durbin Watson stats. = 1.980 |
Log likelihood = 17641 Akaike Info. Criterion = -8.015 Durbin Watson stats. = 2.040 |
Constant transition probabilities, | ||
Probability of staying (switching): | ||
Regime Ⅰ Regime Ⅱ |
0.98 (0.02) 0.95 (0.05) |
0.85 (0.15) 0.31 (0.69) |
Constant expected durations: | ||
Regime Ⅰ Regime Ⅱ |
54 days 21 days |
6.5 days 1.5 days |
Notes: as in Table 1, z-statistics in parentheses. Source: as in Table 1. |
Changes in USD trade weighted ex. rate as a function of changes in copper price | Changes in USD trade weighted ex. rate as a function of changes in gold price | |
Regime Ⅰ | |
|
Regime Ⅱ | |
|
Common terms: Log Sigma | -5.507*** (-448.2) | -5.579*** (-452.8) |
Diagnostic tests: | Log likelihood = 17733 Akaike Info. Criterion = -8.057 Durbin Watson stats. = 2.036 |
Log likelihood = 18051 Akaike Info. Criterion = -8.202 Durbin Watson stats. = 2.077 |
Constant transition probabilities, | ||
Probability of staying (switching): | ||
Regime Ⅰ Regime Ⅱ |
0.75 (0.25) 0.79 (0.21) |
0.95 (0.05) 0.92 (0.08) |
Constant expected durations: | ||
Regime Ⅰ Regime Ⅱ |
1.3 days 4.8 days |
19 days 12 days |
Notes: as in Table 1, z-statistics in parentheses. Source: as in Table 1. |
Phases based on break points | Changes in the USD in EUR exchange rate as a function of changes in log of WTI price | Phases based on break points | Changes in USD in EUR exchange rate as a function of changes in log of Brent price | ||||||
|
|
|
|
|
|
|
|
||
Phase Ⅰ 1/05/1999 – 1/23/2003 (998 obs) |
0.001 (1.41) |
0.015 (1.29) |
-0.001 (-1.56) |
-0.001 (-0.07) |
Phase Ⅰ 1/05/1999 – 1/22/2003 (986 obs) |
0.001 (1.42) |
0.010 (0.84) |
-0.001 (-1.45) |
0.016 (0.94) |
Phase Ⅱ 1/24/2003 – 3/18/2009 (1535) |
-0.001 (-0.78) |
-0.042*** (-4.88) |
0.001 (0.22) |
-0.010 (-0.84) |
Phase Ⅱ 1/23/2003 – 3/18/2009 (1547) |
-0.001 (-0.71) |
-0.051*** (-5.64) |
-0.001 (-0.21) |
-0.019 (-1.46) |
Phase Ⅲ 3/19/2009 – 3/22/2013 (1012) |
0.001 (0.52) |
-1.151*** (-10.82) |
-0.001 (-0.10) |
-0.006 (-0.32) |
Phase Ⅲ 3/19/2009 – 3/22/2013 (1012) |
0.002 (0.74) |
-0.149*** (-9.56) |
-0.001 (-0.36) |
-0.017 (-0.91) |
Phase Ⅳ 3/25/2013 – 8/12/2016 (855) |
0.002 (0.88) |
-0.035*** (-3.89) |
0.001 (0.09) |
0.108*** (5.14) |
Phase Ⅳ 3/25/2013 – 8/12/2016 (855) |
0.002 (0.90) |
-0.030*** (-3.16) |
0.001 (0.49) |
0.115*** (5.06) |
Diagnostic statistics: | |||||||||
F-statistics Log likelh. AIC DW |
23.529 16166 -7.341 1.981 |
22.495 16159 -7.338 1.984 |
|||||||
Notes: t-statistics in parentheses; *** denotes significance at 1%, ** at 5%, * at 10%. Daily data for a sample period January 5, 1999–August 12, 2016 (4401 observations). Source: Authors' own estimation based on Bloomberg and the Federal Reserve Bank of St. Louis FRED daily data. |
Phases based on break points | Changes in USD in EUR exchange rate a function of changes in log of copper prices | Phases based on break points | Changes in USD in EUR exchange rate as a function of gold prices | ||||||
|
|
|
|
|
|
|
|
||
Phase Ⅰ 1/05/1999 - 5/24/2002 (835 obs) |
0.001 (1.49) |
0.018 (0.75) |
-0.001 (-0.95) |
-0.056 (-1.51) |
Phase Ⅰ 1/05/1999 - 4/16/2002 (808 obs) |
0.001 * (1.87) |
-0.083** (-2.49) |
-0.001 (-1.01) |
-0.064 (-1.36) |
Phase Ⅱ 5/28/2002 - 5/19/2005 (736) |
-0.001 (0.58) |
-0.121*** (-7.81) |
-0.001 (-0.97) |
0.267*** (6.82) |
Phase Ⅱ 4/17/2002 - 9/02/2005 (836) |
-0.001 (-0.33) |
-0.441*** (-21.48) |
-0.006 (-1.54) |
0.114*** (2.89) |
Phase Ⅲ 5/20/2005 - 3/18/2008 (710) |
-0.001 (-1.40) |
-0.023*** (-2.58) |
-0.002 (-0.37) |
-0.015 (-0.66) |
Phase Ⅲ 9/06/2005 - 11/02/2009 (1048) |
-0.001 (-0.02) |
-0.178*** (-9.74) |
0.001 (0.01) |
-0.033 (-1.29) |
Phase Ⅳ 3/19/2008 – 3/23/2013 (1266) |
0.001 (0.78) |
-0.187*** (-11.68) |
-0.001 (-0.17) |
0.048** (2.55) |
Phase Ⅳ 11/03/2009 - 8/12/2016 (1708) |
0.001 (0.74) |
-0.208*** (-14.45) |
0.001 (1.17) |
0.164*** (5.72) |
Phase Ⅴ 3/27/2013 - 8/12/2016 (853) |
0.001 (0.69) |
-0.093*** (-5.81) |
-0.001 (-0.09) |
0.265*** (5.58) |
|||||
Diagnostic statistics: | |||||||||
F-statistics Log likelh. AIC DW |
27.893 16246 -7.375 1.969 |
59.471 16403 -7.449 2.011 |
|||||||
Note: Source as in Table 1a. |
Phases based on break points | Changes in the USD trade weighted exchange rate as a function of changes in log of WTI price | Phases based on break points | Changes in USD trade weighted exchange rate as a function of changes in log of Brent price | ||||||
|
|
|
|
|
|
|
|
||
Phase Ⅰ 1/05/1999 - 1/23/2003 (998 obs) |
0.001 (1.41) |
0.001 (0.07) |
-0.001 (-0.44) |
0.001 (0.08) |
Phase Ⅰ 1/05/1999 - 8/16/2007 (2134 obs) |
-0.001 (-0.77) |
-0.025*** (0-4.61) |
0.001 (0.44) |
0.021** (2.28) |
Phase Ⅱ 1/24/2003 - 3/18/2009 (1535) |
-0.001 (-0.99) |
-0.042*** (-6.14) |
0.001 (0.79) |
-0.005 (-0.53) |
Phase Ⅱ 8/17/2007 - 3/18/2009 (2266) |
0.001 (0.88) |
-0.073*** (-12.47) |
-0.001 (-0.90) |
-0.002 (-0.26) |
Phase Ⅲ 3/19/2009 - 10/04/2012 (896) |
-0.001 (-0.33) |
-0.119*** (-11.00) |
-0.001 (-0.16) |
-0.016 (-1.11) |
|||||
Phase Ⅳ 10/05/2012 - 8/12/2016 (971) |
0.001 (1.54) |
-0.051*** (-8.11) |
0.001 (0.96) |
0.074*** (5.01) |
|||||
Diagnostic statistics: | |||||||||
F-statistics Log likelh. AIC DW |
33.829 17664 -8.022 2.035 |
50.526 17594 -7.994 2.047 |
|||||||
Note: Source: as in Table 1a. |
Phases | Changes in USD trade weighted ex. rate as a function of copper prices | Phases | Changes in USD trade weighted ex. rate as a function of gold prices | ||||||
|
|
|
|
|
|
|
|
||
Phase Ⅰ 1/05/1999 - 8/05/2002 (881 obs) |
0.001 (0.45) |
0.010 (0.78) |
0.001 (0.05) |
-0.037* (-1.89) |
Phase Ⅰ 1/05/1999 - 4/16/2002 (808 obs) |
0.001 (1.11) |
-0.048*** (-2.65) |
0.001 (0.20) |
-0.065*** (-2.56) |
Phase Ⅱ 8/06/2002 - 5/18/2005 (690) |
-0.001 (-0.64) |
-0.111*** (-9.39) |
-0.001 (-0.76) |
0.221*** (7.00) |
Phase Ⅱ 4/17/2002 - 9/02/2005 (836) |
-0.001 (-0.69) |
-0.357*** (-22.90) |
-0.001 (-0.99) |
0.126*** (4.31) |
Phase Ⅲ 5/19/2005 - 3/18/2008 (710) |
-0.001 (-1.69) |
-0.020*** (-2.94) |
0.001 (0.09) |
-0.007 (-0.39) |
Phase Ⅲ 9/06/2005 - 11/02/2009 (1048) |
0.001 (0.02) |
-0.150*** (-10.27) |
0.001 (0.07) |
-0.024 (-1.19) |
Phase Ⅳ 3/19/2008 – 11/23/2012 (1183) |
0.001 (0.22) |
-0.150*** (-12.46) |
0.001 (0.06) |
0.026* (1.84) |
Phase Ⅳ 11/03/2009 - 8/12/2016 (1708) |
0.001 (0.73) |
-0.192*** (-19.27) |
0.001 (1.48) |
0.132*** (6.69) |
Phase Ⅴ 11/26/2012 - 8/12/2016 (936) |
0.001 (1.32) |
-0.089*** (-7.95) |
0.001 (0.01) |
0.163*** (4.73) |
|||||
Diagnostic statistics: | |||||||||
F-statistics Log likelh. AIC DW |
40.691 17781 -8.073 2.021 |
84.596 17983 -8.167 2.078 |
|||||||
Note: Source as in Table 1a. |
Changes in USD in EUR ex. rate as a function of changes in WTI price | Changes in USD in EUR ex. rate as a function of changes in Brent price | |
Regime Ⅰ | |
|
Regime Ⅱ | |
|
Common terms: Log Sigma | ||
-5.144*** (-419.9) | -5.102*** (-457.0) | |
Diagnostic tests: | Log likelihood = 16140 Akaike Info. Criterion = -7.333 Durbin Watson stats. = 1.977 |
Log likelihood = 16142 Akaike Info. Criterion = -7.334 Durbin Watson stats. = 1.994 |
Constant transition probabilities, | ||
Probability of staying (switching): | ||
Regime Ⅰ Regime Ⅱ |
0.78 (0.22) 0.49 (0.51) |
0.99 (0.01) 0.01 (0.99) |
Constant expected durations: | ||
Regime Ⅰ Regime Ⅱ |
4.6 days 2.0 days |
205 days 105 days |
Notes: as in Table 1, z-statistics in parentheses. Source: as in Table 1. |
Changes in USD in EUR ex. rate as a function of changes in copper price | Changes in USD in EUR ex. rate as a function of changes in gold price | |
Regime Ⅰ | |
|
Regime Ⅱ | |
|
Common terms: | -5.140*** (-424.2) | -5.102*** (-457.0) |
Log Sigma | ||
Diagnostic tests: | Log likelihood = 16185 Akaike Info. Criterion = -7.354 Durbin Watson stats. = 1.989 |
Log likelihood = 16142 Akaike Info. Criterion = -7.334 Durbin Watson stats. = 1.994 |
Constant transition probabilities, | ||
Probability of staying (switching): | ||
Regime Ⅰ | 0.72 (0.23) | 0.97 (0.03) |
Regime Ⅱ | 0.16 (0.83) | 0.98 (0.02) |
Constant expected durations: | ||
Regime Ⅰ | 3.6 days | 32 days |
Regime Ⅱ | 1.2 days | 49 days |
Notes: as in Table 1, z-statistics in parentheses. Source: as in Table 1. |
Changes in USD trade weighted ex. rate as a function of changes in WTI price | Changes in USD trade weighted ex. rate as a function of changes in Brent price | |
Regime Ⅰ | |
|
Regime Ⅱ | |
|
Common terms: | ||
AR(1) Log Sigma | -0.021 (-1.32) -5.469*** (-413.0) |
NA -5.483*** (-447.4) |
Diagnostic tests: | Log likelihood = 17665 Akaike Info. Criterion = -8.029 Durbin Watson stats. = 1.980 |
Log likelihood = 17641 Akaike Info. Criterion = -8.015 Durbin Watson stats. = 2.040 |
Constant transition probabilities, | ||
Probability of staying (switching): | ||
Regime Ⅰ Regime Ⅱ |
0.98 (0.02) 0.95 (0.05) |
0.85 (0.15) 0.31 (0.69) |
Constant expected durations: | ||
Regime Ⅰ Regime Ⅱ |
54 days 21 days |
6.5 days 1.5 days |
Notes: as in Table 1, z-statistics in parentheses. Source: as in Table 1. |
Changes in USD trade weighted ex. rate as a function of changes in copper price | Changes in USD trade weighted ex. rate as a function of changes in gold price | |
Regime Ⅰ | |
|
Regime Ⅱ | |
|
Common terms: Log Sigma | -5.507*** (-448.2) | -5.579*** (-452.8) |
Diagnostic tests: | Log likelihood = 17733 Akaike Info. Criterion = -8.057 Durbin Watson stats. = 2.036 |
Log likelihood = 18051 Akaike Info. Criterion = -8.202 Durbin Watson stats. = 2.077 |
Constant transition probabilities, | ||
Probability of staying (switching): | ||
Regime Ⅰ Regime Ⅱ |
0.75 (0.25) 0.79 (0.21) |
0.95 (0.05) 0.92 (0.08) |
Constant expected durations: | ||
Regime Ⅰ Regime Ⅱ |
1.3 days 4.8 days |
19 days 12 days |
Notes: as in Table 1, z-statistics in parentheses. Source: as in Table 1. |