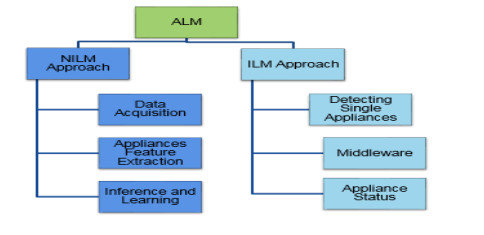
Citation: Maria Jose Miguez, Wenyaw Chan, Luis Espinoza, Ralph Tarter, Caroline Perez. Marijuana use among adolescents is associated with deleterious alterations in mature BDNF[J]. AIMS Public Health, 2019, 6(1): 4-14. doi: 10.3934/publichealth.2019.1.4
[1] | Zhen Yu, Sheng-Huang Lin, Chia-Ching Cho, Changping Chen . Performance management algorithm of financial shared service center based on Internet of Things public cloud privacy protection. Mathematical Biosciences and Engineering, 2023, 20(7): 12510-12528. doi: 10.3934/mbe.2023557 |
[2] | Lei Zhang, Lina Ge . A clustering-based differential privacy protection algorithm for weighted social networks. Mathematical Biosciences and Engineering, 2024, 21(3): 3755-3773. doi: 10.3934/mbe.2024166 |
[3] | Jia Ouyang, Yinyin Xiao, Shaopeng Liu, Zhenghong Xiao, Xiuxiu Liao . Set-valued data collection with local differential privacy based on category hierarchy. Mathematical Biosciences and Engineering, 2021, 18(3): 2733-2763. doi: 10.3934/mbe.2021139 |
[4] | Muhammad Ahmad Nawaz Ul Ghani, Kun She, Muhammad Arslan Rauf, Shumaila Khan, Masoud Alajmi, Yazeed Yasin Ghadi, Hend Khalid Alkahtani . Toward robust and privacy-enhanced facial recognition: A decentralized blockchain-based approach with GANs and deep learning. Mathematical Biosciences and Engineering, 2024, 21(3): 4165-4186. doi: 10.3934/mbe.2024184 |
[5] | Songfeng Liu, Jinyan Wang, Wenliang Zhang . Federated personalized random forest for human activity recognition. Mathematical Biosciences and Engineering, 2022, 19(1): 953-971. doi: 10.3934/mbe.2022044 |
[6] | Kwabena Owusu-Agyemang, Zhen Qin, Appiah Benjamin, Hu Xiong, Zhiguang Qin . Insuring against the perils in distributed learning: privacy-preserving empirical risk minimization. Mathematical Biosciences and Engineering, 2021, 18(4): 3006-3033. doi: 10.3934/mbe.2021151 |
[7] | Chunkai Zhang, Ao Yin, Wei Zuo, Yingyang Chen . Privacy preserving anomaly detection based on local density estimation. Mathematical Biosciences and Engineering, 2020, 17(4): 3478-3497. doi: 10.3934/mbe.2020196 |
[8] | Lihong Guo, Jian Wang, Haitao Wu, Najla Al-Nabhan . XML security protection scheme based on Kerberos authentication and polynomials authorization. Mathematical Biosciences and Engineering, 2020, 17(5): 4609-4630. doi: 10.3934/mbe.2020254 |
[9] | Yuan Tian, Biao Song, Mznah Al Rodhaan, Chen Rong Huang, Mohammed A. Al-Dhelaan, Abdullah Al-Dhelaan, Najla Al-Nabhan . A stochastic location privacy protection scheme for edge computing. Mathematical Biosciences and Engineering, 2020, 17(3): 2636-2649. doi: 10.3934/mbe.2020144 |
[10] | Qinwang Niu, Haoyue Li, Yu Liu, Zhibo Qin, Li-bo Zhang, Junxin Chen, Zhihan Lyu . Toward the Internet of Medical Things: Architecture, trends and challenges. Mathematical Biosciences and Engineering, 2024, 21(1): 650-678. doi: 10.3934/mbe.2024028 |
In todays and future technology trends, there is a lot of interest to expand smart devices that are compatible with Internet of Things (IoT) [1] based system design. Research work within this area aims to connect devices with most appropriate way towards the development of smart and safe living and smart city technologies [1,2]. In addition, innovative technological systems, as smart homes and smart grids, have enhanced automation limited duties to develop rapidly with the adaptation of new technologies to their applications [2]. The communication between home appliances of consumers is improved by installing advanced technology such as smart plug and smart meter [3,4]. The solution enables real-time monitoring and remote controlling capability of appliances on the consumer premises.
IoT enabled smart grid is offering a reliable, high quality, flexible, and cost-effective energy supply. Advancement of information technology applications on electrical grid have been empowered real-time data acquisition and data analysis. These application are supported by a new kind of sensor technology for monitoring of the transmission control system [1,2,3] from distribution network to individual homes. Furthermore, combination of smart grid and smart meter allows proliferation of advanced demand-side management applications. Load profiling with smart meter indirectly can be also used for supporting services for senior citizens and their growing demand for living self-independently. However, the existing systems for health status monitoring based on profiling appliance’s power consumption is still immature. So far, health monitoring system is accomplished by either wearables or sensor-based systems [4,5,6,7]. There is a limited number of proposal from the research associated with smart meter data analyzing towards assisting personal health care system [8,9,10]. Smart meters and advance metering infrastructure, unlike IoT wearables, have capacity of analyzing a huge amount of data in real-time [4,5] for a number of houses and supporting a number of services and applications for homes. The goal of this work is to select an identification method for identity detection of appliances with the target to be cost-effective and accurate. This is based with the implementation of the classification of the energy load patterns. The collected energy measurements using smart meter and associated analysis can provide an abnormality detection scheme and to be usable for future eHealth monitoring application. The method is promising because it permits a cost-effective improvement that can empower an extensive modification on the eHealth system [9,10].
This particular work targets on NIALM system for the appliances level of energy consumption that is based on a single-phase smart meter or socket connected for total agammaegated measurements. Utilization of NIALM has a competitive advantage because of its easy installation on the existing electrical system without performing structural modification. The identification appliances and their load consumption can be detected from central system and can be also controlled for minimizing energy costs and maximizing user's monitoring system at lower cost. The main contributions of this work are:
● Comparison of different classification algorithms and demonstration of suitability of k-NN as most suitable classifier for most of the appliances used in the household.
● Improvement of NIALM techniques to distinguish the signature of different appliances and their practical behavioral pattern problems based on the agammaegated measurement of the house hold.
● Employment of k-NN algorithm to evaluate and to show the performance for accuracy.
NIALM approach delivers a cost effective solution to monitor appliances with a single metering unit. It also can provide an important information on the appliances during on their on/off status. The information can be beneficial for an activity monitoring system for elderly people, our main research that is not be discuss much in this paper. However, appliances identification is to be used for following up the real-time health status and for serving as a tool for elderly living independently without caregivers by staying in their own homes. This paper further focuses on the approach of using machine learning for NIALM classification, in order to detect or classify the appliances from the agammaegate power consumption with a high-level accuracy performance.
The rest of the paper is organized as follows. Section 2 presents the background and related work of the appliances identification techniques and describes the k-NN algorithm. Section 3 discusses the NIALM system and the k-NN algorithm for NIALM classification. Experimental results and implementation analysis are presented in Section 4. Finally, Section 5 summarizes the key result and further steps for research in this work.
ALM system has two categories: 1) Intrusive Load Monitoring (ILM), 2) Non-Intrusive Load Monitoring (NILM). In the next paragraphs, we described these two methods in brief:
ILM method require a group of sensors attached to each appliance to show information about the appliance load measurement used for functions of appliances load monitoring and classification. The connections of sensors and each appliance through smart plugs [11] provide more accurate measurement than NILM system. The drawback of ILM method is its cost, which is considered to be very high due to the installation complicity of due to the multiple sensor configurations. In the research [12], ILM approach is used as middleware on Atlas and OSGi [12] platform which was implemented to detect a single appliance solution for reduction of costs, as shown in Figure 1. This method can identify real-time controlling and monitoring of household status by detecting each appliance connected to their own smart plug. The proposed framework of two ALM system types is as shown in the Figure 1.
NILM approach identifies energy consumption of each appliance through a connection of a single meter that is attached to center of the house node of electrical system network. NILM concept was originally developed by George Hart during the 80s [13] and have been deployed for expansion of a non-intrusive system to monitor the power consumption of a specific appliance from the power load of the central control system of the electrical network. The method needs very high frequency (for PLAID dataset, 30 kHz) for data communication than ILM methods. It also requires computing a more complex algorithm for identification of the appliances. The process for appliance identification has following phases as described below:
● Data Acquisition and processing phase: It starts from the measurements of current, voltage and power consumption and de-noising the obtained measurements. These measurements are used for detection of the appliances by feature extraction. The electrical load measurement is taken at a certain frequency for best result of load pattern identification. Nowadays, smart meters are the more common tools for measuring load consumption. With smart meter, one can obtain pattern and even to distinguish features, starting from data acquisition phase of data collection.
● Appliances Feature Extraction phase. The feature is extracted from the signals associated with the voltage and current measurements of appliance and their corresponding current and voltage waveforms in order to compute reactive and active power states. Further, appliance signature for event detection can be extracted from on / off state change of appliances on the power consumption measurements. The identification of initial appliance state changes, and t power level analysis detects the state of the appliances from on to off or vice-versa. The load features can be extracted based on load signatures using various methods [14,15,16,17], in order to provide appliances signature information. Basically, the method [15] based on a numerical perspective meaning using statistical analysis methods in order to distinguish different appliances that are typically expressed in form of vectors and dimensions to determine the amount of features. Then, the selected features can increase the NILAM system classification capability. The real power (P) and reactive power (Q) of the appliances are developed by analyzing physical load characteristics of the turn-on operation of the transient energy and the traditional steady-state power features [16]. The feature extraction can be processed with different method as we described in previous section, in order to gain an accurate appliance dataset that can be used for the process of the next phase.
● Load recognition phase: The load recognition process matches the appliances load consumption features and with the features in the database that is obtained from the appliance operating event. The initial appliance state is important for understanding about appliance current-voltage pattern in order to choose an appropriate classification method from either supervised or unsupervised algorithms. The identification procedure is done through classification that is grouped with electrical appliances based on pattern of the current or Ⅴ-Ⅰ trajectory features. On this paper after comparing different machine learning methods, the classification we used is based a training data model. The identification / classification of the appliances is performed by using the k-nearest neighbor’s classifier (k- NN) algorithm.
K-NN is one of ML algorithms that is used for solving classification and regression problems. The algorithm uses a supervised and instance-based learning algorithm that has been presented by [18], in the early 1950s. k-NN classifier usually used for pattern recognition, image recognition, data mining, text categorization, and predictive analysis. Researchers [18] have proposed k-NN classifier method that uses a memory area for training stage, and its classification space is at least the same as the training area. In that case, the processing time needed for classification steps of k-NN method can be greater than that of the training steps. The main computation of k-NN classifier algorithm is based by the distance calculation between the training dataset and the testing dataset of each element, and by concurrently categorizing instance of measurements. Through years, many researchers have developed and presented k-NN [13], on the NIALM analysis [18,19,20]. The k-NN classifier described on [19] followed the procedures for labeling of testing instances from a majority vote of nearest neighbors of each point and by recommending items of neighboring of unknown values. The labeling of the features followed the nearest neighbors in order to label the value of predictable features. On another research a Probabilistic k-NN (Pk-NN) [20] algorithm was used to compare temperature and humidity sensor datasets. The Pk-NN classifier performance was high and achieved accuracy was acceptable. The algorithm is considered as the easiest and the most common algorithm for processing and allocation of the stored data in the memory. It is also used with a comparison of a new database to perform accurate classification. Even from 19th, distance- weighted k-NN rule in [20] has been shown to motivate selection of the size of k-NN for improving the classification performance. Nowadays, k-NN have been demonstrated [21,22] to be an accurate, relevant and competent classifier method for text categorization, pattern recognition, image recognition.
We propose that NIALM classification method can be used for monitoring household’s appliance power consumption. We used the PLAID [23] dataset as benchmark, and processing scheme of appliances classification as shown in Figure 2 below.
PLAID dataset [23] is an open publically available dataset that contains voltage and current measurements from 60 houses in the USA located at Pittsburgh, Pennsylvania and are collected at sampling frequency 30 kHz. The data contains the energy consumption of 11 kinds of appliances from 55 houses. The data was collected during the summer of 2013 and winter of 2014 and has 1074 sample datasets. The load consumption measurement of each appliance indicates the different instances and variable model of collection can be able to characterize each appliance type. On average 5 instances or measurements have performed for each appliance by considering each of the corresponding state of transition. Table 1, shows the summary of datasets [24] for each appliance. The measurement data are processed and extracted with an interval of a second to cover both the steady-state action and for some appliances with the available startup transient [25]. The PLAID dataset is used here as benchmark dataset for evaluating various approach to classification of various appliances in household. The identification of appliance power usage and pattern have been executed in diverse systems as explained in the paper [26] and based on event-based methodology for NIALM [27]. The collected data considered the measurements for the steady and transient states of the appliance. However, the transient event state is sampled at high frequency whereas the steady state is extracted from low frequency sampled of power measurements. The collected data preprocessed with different stages such as de-noising, filtering, harmonic component separation, signal synchronicity, etc. to be able to indicate an accurate and sensible information.
Appliance type | Number ofInstance | Appliance type | Number ofInstance |
Compact fluorescent lamp | 175 | Fridge | 38 |
Vacuum cleaner | 38 | Incandescent light bulb | 114 |
Hair dryer | 156 | Fan | 115 |
Microwave | 139 | Washing Machine | 26 |
Air conditioner | 66 | Heater | 35 |
Laptop | 172 | Total | 1074 |
NIALM is a methodology used to disagammaegate total power consumption into individual electrical appliance load. To measure the agammaegated signal, the appliances are activated one after another by monitoring the deactivation [26]. The features for the electrical signature is used from the publicly available PLAID data set are extracted by the combination of identifying the on or off state of and events of appliances and by event detection. The feature extraction can be processed from signature of appliances that are measured separately while each appliance is at on/off event state, i.e. the parameters are taken by measuring the load consumption of each appliances one at a time. The feature extraction is based on the Ⅴ-Ⅰ trajectory (voltage/current), and steady state measurements of devices before and after the event. It is also a crucial step to find a recognition mechanism for appliances during the transient threshold crossing point from on/off state or vise verse state. Overall, the data processing stage is responsible for delivering the filtered data to the event detection stage with the electrical parameters such as harmonic components, reactive power, and transient and active power waveforms. The recognition feature of the appliances is different upon event change of appliances state. For this work, as we stated earlier the experiments are done from NIALM, publicly available smart meter PLAID [24] dataset. Our aim is to perform analysis of the correlation between NIALM and the activity detection of consumers with accurate results. The process of identification is done according to the characteristics of the appliance operating states [26,27]. (1) two operating states as on/off; (lamp), (2) multistate (finite state machines) with multistate and a repeated operation; (washing machine), and (3) the infinite state that operates for longer time, day, week, operations; (mobile phones). The type of appliances according to their load consumption pattern is shown in Figure 3.
In all types of states, the signature of the load indicates a specific information for recognizing each appliance separately. In summary, appliance’s signature is categorized according to steady-state signatures and transient signatures of the appliances.
The signature shows mode of the event from agammaegate power measurements. The measurements from the energy load of appliance are processed and extracted for analyzing appliance load behavior. We obtained the plot of Figure 4, from the given PLAID [24] current and voltage measurements for each appliance.
The signature of appliances is depending on the nonlinear variation state of operation of appliance state. It means that when the appliance is turned on, an instant flow of current appears, as is shown in Figure 5b, which describes transient event of the current pattern. In that case the amplitude of current will be much more than the level of steady-state current [20]. In addition, the onset happens with the change of patterns during the on switching of the selected appliances on transition of operating transition [27,28,29,30]. Such events for the appliances appears when the appliance change its power state, e.g. during transients of charging, boiling, rotating, etc. Normally, offset is caused by appliances switch off with a quick step to down to zero power or other similar way for cutting of power. As an example if the power is off immediately but washing machine motor is operating generator until mechanical stop. The parameters of these states indicate over or under shoots of power amplitude when turned on, and rise/fall time and settling time. For low power appliances, the power change will be visible at real power (P), reactive Power (Q) of the feature as shown as over lapping space as in Figure 5(b). The real power is the amount of energy consumed during operation and for the reactive load there is no any real power load. However, devices that have an electric motor when they turned on, produce a transient event and they have a non-sinusoidal current during operation as shown in Figure 5(a).
Applying feature extraction on the appliances signature of load consumption, can help to categorizes loads by distinguishing similar Ⅴ-Ⅰ trajectories of appliances. This is depending on the type of the appliance state and signature, as described in the section above on the appliance signature and features. Mainly, the appliance features are categorized according to steady state and transient state features including the non-traditional signatures. The steady state variation can also depend on power change between real and reactive power. The non-traditional signatures are often obtained from the mixture of traditional appliance features based on routine of the overall power consumption measurements. The recognition module spots on/off state change of the devices by distinguishing the load variations as described above. Additionally, it shows actions on the change of state (steady state or transient state) that is described as in subsections above. Appliance feature extraction and their state translations discussed in the previous literature [25,26,27] and we summarize this in Figure 6.
The feature extraction thus is based on signature of appliances, as shown in Figure 6, which are classified by appliance steady and transient signatures. The transient describes the edge, trend, duration, and phase of the signature, while the steady state shown with current, the voltage and frequency components of appliances. However, as we described earlier our feature extraction method is based on the voltage and current readings obtained from the PLAID dataset which is used for appliances signature. The feature set includes the parameters such as peak power and current, and RMS current by considering steady state signatures of the appliances [26,27]. Figure 4 shows the voltage and current trajectory (Ⅴ-Ⅰ) of 11 appliances that are classified by steady and transient signatures of the appliances. The Ⅴ-Ⅰ trajectory can create a visible signature or pattern or appliance. We use the plot of the visual features for classification of the appliance. Equation 2, states the resultant wave of the period for voltage (ⅴ) and current (ⅰ) that were developed over time at a sampling frequency (fs) and grid frequency (fg) [28].
$ \Phi {\rm{p}} = {\left[ {{\rm{ \mathsf{ φ} }}\left( {\rm{p}} \right), {\rm{ \mathsf{ φ} }}\left( {{\rm{p}} + 1} \right), \ldots , {\rm{ \mathsf{ φ} }}\left( {{\rm{p}} + {\rm{d}} - 1} \right){\rm{ }}} \right]^{\rm{T}}} € {{\rm{R}}^{\rm{d}}}, \;{\rm{ \mathsf{ φ} }} € \left\{ {{\rm{i}}, {\rm{v}}} \right\} $ | (1) |
where d is the number of samples per period fs/fg), p is point at a time, φp, is the resultant wave.
The PLAID dataset is obtained from readings of one smart meter per household for load consumption of the appliances. The feature has extracted and a combination of features to be used for NIALM and classification of appliances on daily bases. The recognized patterns of features and the classification can help to recognize each appliance based on the operation signature of specific state of the appliances during a definite period operation.
The results of the appliances classification are depending on the valuable and effective processing method and by identifying features from the available PLAID smart meter dataset. The pattern can be recognized by the operating and estimated energy consumption of each type of the appliances. We verified the accuracy of classification by matching with the available set of features. Here the quantity of the input data is not a factor for the accuracy but it depends rather on the quality of the available information in terms of the feature extraction of the appliances [24,31]. For the load classification mainly machine learning algorithms and optimization and machine learning algorithms used the supervised and unsupervised methods for the classifications. In the study, as also shown below in Figure 7, we have investigated various classification algorithms that are used for features of the smart meter load measurement in order to find out suitable classification algorithms for the dataset. Among them [18] k-Nearest Neighbors (k-NN), Random Forest (RForest), Decision Tree (DTree), Gaussian naive Bayes (GNB) and logistic regression classifier (LGC), have been checked [18]. These multiple classifiers are applied to the dataset on the previous study [18]. The steps of presentation of these classifiers have been proved with various sets of the extracted input features from the measurements taken using smart meters. Some of the features that are used for identifying the appliances, for the classification purposes have both transient and steady state features, as we already mentioned in the previous sections. The binary Ⅵ-trajectory is used as a feature because of being the most capable feature extracted from the previous study achieved on PLAID [18]. This feature is created by plotting voltage compared to current of an instance by giving a binary a 0 or 1 value according the existing model of the trajectory.
Figure 7, shows a plot on comparison of the different ML algorithm, for the purpose of identifying the accurate and suitable algorithm and the classification accuracy is changes with the sampling frequency. The result is based on the PLAID dataset as input and application software based with Python3 code representation and other necessary packages of R studio as illustrated in GitHub [24]. The k-NN and RF showed best performance among the other classifier method. However, and k-NN classifier suits the classification of active or reactive power input features. Even RF seems to be better in result at Figure 7, but we do not use it according to the analysis done by the previous work [32]. It was demonstrated that the k-NN achieved higher accuracy both for imbalanced and balanced datasets, similar to PLAID high frequency dataset, than RF. In addition, we choose for the appliance classification method based on the k-NN procedure since it shows the best result and the algorithm is broadly used as the classification algorithm with accurate implementation [33]. k-NN is simple and effective classification algorithms and we show on the confusion matrix [34] its performance evaluation. It shows that k-NN matrix has higher recognition accuracy for the NIALM methods. For the unbalanced dataset in Table 1, k-NN gives better performance by automatically detecting either linear or non-linear distributed data set. Hence, it tends to perform very well with many data points. In addition, the algorithm is used as a data-mining algorithm for feature extraction and pattern recognition including its classification of the features can be easily implement with low error rate.
Figure 7, indicates the different algorithms classifications accuracy vs the sampling frequency (kHz) plot that are processed on the PLAID dataset measurement for classifying the household appliances.
The dataset, using NILAM has classified the total power consumption of household into individual appliance consumption (fridge, air conditioner, etc.).
Our method using k-NN for classification of events can be summarize as follows:
● Detection of events: Events are variations of total consumption, which caused by the change of state of an appliance. Different signal processing algorithms can detect events.
● Clustering of events: Events detected are further clustered, e.g. we try to group events that are likely to come from the same change of state of the appliances. The unsupervised machine learning in particular the k-NN algorithms is used at this stage.
● Modeling of appliances: With the clusters obtained above and time series analysis, appliance models are built. An appliance model regroups clusters to representing a change of state of the appliance. The supervised machine learning in particular k-Means algorithms is used at this stage.
● Tracking of appliances consumption: Once appliance models built, it is possible to track the behavior of each appliance from the load consumption of this appliance at each time.
Neighbors-based classification is a type of the instance-based learning, which does not construct a general internal model, but simply stores instances of the training data [37]. Classification is computed from a majority vote of the nearest neighbors of each point. A query point is assigned to the data class, which has the most of representatives within the nearest neighbors of the particular point [37]. k-NN algorithm is the most common technique to implement the nearest neighbor classification in where k is an integer value. However, the optimal choice of the value of k is highly data-dependent [35]. Various matrices have been evaluated to determine the distance for categorizing query points in a training dataset [36,37,38].
However, the k-NN computation of the algorithms is developed by using the distance measure between training dataset and the testing dataset of each element or means identify the k nearest neighbors of a test point. There are many ways to measure the distance between attributes in testing set and training set. The most significant ones is Euclidean equations is shown in equation (1) [39].
$ \text { Euclidean: } \mathrm{D}(\mathrm{x}, \mathrm{y}) = \left(\left(\mathrm{x}_{1}-\mathrm{y}_{1}\right)^{2}+\left(\mathrm{x}_{2}-\mathrm{y}_{2}\right)^{2}+\ldots . .+\left(\mathrm{x}_{2}-\mathrm{y}_{2}\right)^{2}\right)^{1 / 2} $ | (2) |
From the formulas, for a definite of nearest number of k, and the unknown dataset x and a distance metric D, and k-NN checks all over the dataset and compute D among x and every training assignment. The new instance is classified by a common selecting its neighbors and by labeling and allocating to the same the class, the instants with maximum shared between their k nearest neighbors [39]. With k = 1, for instance, the case is just allocated to the class of its nearest neighbor. The nearest neighbor search is a challenging task in multidimensional data. The important factors in the nearest neighbor search (k-NN) are adapting the runtime with accuracy for practical and feasible huge datasets
To summarize shortly the procedure for k-NN classification; it starts by processing the PLAID dataset and extracting its features based in the state of the appliances. The extracted dataset will be categorized into training and testing sets. The similarity will be calculated according to the closest test samples in the training set and by labeling of the new data point with a result of the nearest K data points in the training dataset. Later, the outcome of neighbors and closest neighbors are labeled for unknown data. In that case, the appliance classification based on the k-NN input by knowing the samples on the dataset output and the result, or the output will be on the majority label and we use the same common k-NN algorithm or characterize the NIALM methods [40]. The next sections will discuss the evaluation metrics of the classification of k-NN algorithm for the implementation.
The PLAID dataset that is composed of 11 appliances (vacuum cleaner, microwave, compact fluorescent lamp, fan, laptop, fridge, hairdryer, heater, incandescent lightbulb, air conditioner, and washing machine) on them on-off state are considered for this model. We discard the washing machine from our evaluation because of the lack of data and due to the appliance has a variable current waveform which not constant during the process. The other issue was from the PLAID data was not possible to separate the testing dataset from the whole training dataset. However, for the purpose of evaluating the training and the testing was performed on the whole dataset and a higher tanning accuracy was obtained. Using our program, we divided the training and testing data proportion that is kept as 90%: 10% throughout the entire of this procedure.
The VI-binary image is used as input to confirm the classification accuracy. The accuracy evaluation metrics is extensively applied for assessing the classification routine. It mainly helps for analyzing of misleading assumption mostly for unnecessary datasets [23]. The parameters for the confusion matrix [34] are obtained from True Positive (TP) and True Negative (TN) for calculating performance evaluation described on Table 2. The TP & FN are considered when the appliances are on and off respectively.
Predicted Positive | Predicted Negative | |
Actual Positive | True Positive (TP) | False negative (FN) |
Actual Negative | False Positive (FP) | True Negative (TN) |
The performance outcome which is calculated from true positive rate measurements are defined as sensitivity or recall parameters and the precision is the positive predicted value. Formula (7) shows the calculation for precision and recall value [34]. The evaluation criteria are distinguishing by performance evaluation indicator to consider the classification accuracy with the pointers of accuracy [34], error rate, G-mean and F-measure that are calculated as shown below:
$ Accuracy = \frac{TP+TN}{TP+FP+TN+FN} $ | (4) |
$ F-measure = \frac{2xPrecisionxRecall}{Precision+Recall} $ | (5) |
$ G-mean = \sqrt{Precision+Recall} $ | (6) |
The precision and the recall can be evaluated from the matrix as:
$ Precision = \frac{TP}{TP+FN}, \;\;\;Recall = \frac{TP}{TP+ +FN} $ | (7) |
The confusion matrix that displays the classification of each appliance for the k-NN algorithm is shown in Figure 8. For evaluation of k-NN the data set is divides as testing and training dataset. The 90% of the dataset are taken for training purpose and the rest 10% for testing. The k-NN algorithm has identified, or classified, the appliances from the NIALM dataset very well. However, it is not reliable for some of the appliances because of different reasons: the sample data used are insufficient, and lack of the algorithm ability in identifying the multi-state appliances and the appliances with similar power consumption profiles. However, the accuracies of the identification taken by our experiments are acceptable in case of almost all the appliances. For the appliances (vacuum cleaner, fluorescent lamp, AC, bulb, fan, microwave, hairdryer) the identification number of are mostly dispersed on the central sloping of the matrix. This is indicating when the rate of true positive is more, and the accuracy achieved with the k-NN algorithm is as shown high. From the confusion matrix, for some of the energy consumption of the appliances (laptop, fridge, heater), there is a false positive detection rate, which is high. This case happened for the reasons that are mentioned above and may be -also depend on selection of k values. The F-measure and the G-mean rate based on the performance of K-NN should be considered. However, the algorithm proposed in this paper has a high F1 value and an accuracy from 93% up to 100% is achieved in most cases with the implementation of k-NN algorithm. The confusion matrix as shown in Figure 8, gives more details on the appliances accuracy for the 1-NN configuration and Table 3 shows appliance performance evaluation by the overall F-measure and G-mean value of k-NN classifier.
Appliance | F-Measure | G-mean |
Compact FL Laptop Microwave Hairdryer Vacuum Cleaner Fridge Heater Il bulb Fan Air-conditioned Total |
0.99 0.72 1 1 1 0.68 0.23 0.97 1 1 0.9 |
1 0.75 1 1 1 0.73 0.21 0.97 1 1 0.94 |
Table 4 and Figure 9 present the comparisons of our F-measure matrix result, with the previous result of Convolutional Neural Network (CNN) [41] that was based on the PLAID dataset on NIALM and classification of the appliances. The CNN classifies is better for recognizing of appliances from transient power state signal than k-NN.
Appliances | K-NN (our result) | CNN [41] |
CFL Laptop Microwave Hairdryer Vacuum Fridge Heater I bulb Fan AC |
99 % 72% 100% 100% 100% 88% 24% 97% 100% 100% |
96% 98% 93% 79% 98% 97% 82% 81% 60% 47% |
On the other hand, F-measure and G-mean are the performance evaluation metrics for appliance classification. In Table 3 comparison of F- measure of our k-NN classifier and CNN shows that the k-NN algorithm have more accuracy than CNN for the PLAID dataset appliances classification. Figure 9 below shows how for most of the appliances identification (CFL, microwave, hair dryer, vacuum, fridge, Incandescent light bulb(Ibulb), fan and AC), the performance of classifier was extremely good with 100% classification accuracy for each appliances The CNN classifier performance for NIALM detection of appliances for transient power signal data was higher than KNN. However, for the majority of classification of the appliance k-NN was higher.
NIALM based open dataset PLAID [23,24] shows a promising result for analyzing variations between current and voltage and detecting the energy consumption of each appliance from a single point agammaegated measurements. The extracted features from the voltage-current trajectory are implemented with the k-NN classifier in order to recognize the appliances. The accuracy matrix F-score average equals to 90%. The supervised learner based k-NN implementation has a better performance of accuracy than the rest of the compared machine learning algorithm to classify and to detect each appliance. The k-NN classifier shows that accuracy of the classification is depend on the size of the appliances training datasets. The paper shows the on/off state and event detection of the appliances with the deployment of k-NN classifier has acceptable accuracy for the identification of the appliances. The result of the appliances identification can show that NIALM, besides being an effective technique for advanced demand side management with appliances identification, can be also operated for ambient assisted living by providing indirectly information about the activity and health status for each household from the detected appliance usage. We can show this assumption further as the load consumption and the monitoring system we proposed can recognize the associated activities (cleaning, cooking, sleeping, etc.) of the user on daily basis. Therefore, the method can rely better on the information of the event of the activities and the duration of the appliance usage. In addition, we discovered the appliance energy consumption can be used to consumers monitoring systems with the implementation of machine learning. NIALM can identify the energy consumption of specific appliances with low cost power meter plug that are connected from the central control system.
In this paper we presented an approach to classify appliances with NIALM techniques by distinguishing signature of different appliances and their behavioral pattern based on disagammaegated total energy consumption data to individual appliance specific energy load. The comparison of different classification algorithms have demonstrated that k-NN is most suitable classifier for most of appliances used in the household. Our analysis summarizes the identification performance can be done based on the operational states of the appliances. Finally, the implementation of the k-NN algorithm for PLAID dataset appliance classification has a potential scalability for detecting activity of the occupant and for monitoring their daily routines.
For the future, there will be more discussion about the further steps on facilitating effective behavioral detection using NIALM. Further studies are needed to demonstrate the processing and identification events on the effective hardware implementation of NIALM with k-NN that can be more suitable for detecting transient state appliances based a smart meter dataset. Thus providing low cost scalability for system development.
We would like to thank the Swedish Funding Agency, SIDA for funding and supported through iGrid Project and STINT through IoT4Health Projects at School of Electrical Engineering and Computer Science, Division of Electronics and Embedded system, Royal Institute of Technology (KTH).
The authors declare that there is no conflict of interest in this paper.
[1] | World Health Organization. Substance Abuse: Facts and Figures, 2015. Available from: http://www.who.int/substance_abuse/facts/cannabis/en/. |
[2] |
Jacobus J, Tapert SF (2014) Effects of Cannabis on the Adolescent Brain. Curr Pharm Des 20: 2186–2193. doi: 10.2174/13816128113199990426
![]() |
[3] | World Drug Report 2014. United Nations publication, Sales No. E.14.XI.7. Available from: http://www.unodc.org/documents/wdr2014/World_Drug_Report_2014_web.pdf |
[4] |
Sevigny EL (2013) Is today's marijuana more potent simply because it's fresher? Drug Test Anal 5: 62–67. doi: 10.1002/dta.1430
![]() |
[5] |
D'Souza DC, Pittman B, Perry E (2009) Simen A Preliminary evidence of cannabinoid effects on brain-derived neurotrophic factor (BDNF) levels in humans. Psychopharmacology 202: 569. doi: 10.1007/s00213-008-1333-2
![]() |
[6] |
Jockers-Scherübl MC, Danker-Hopfe H, Mahlberg R, et al. (2004) Brain-derived neurotrophic factor serum concentrations are increased in drug-naive schizophrenic patients with chronic cannabis abuse and multiple substance abuse. Neurosci Lett 371: 79–83. doi: 10.1016/j.neulet.2004.08.045
![]() |
[7] | Arseneault L, Cannon M, Poulton R, et al. (2002) Cannabis use in adolescence and risk for adult psychosis: longitudinal prospective study. BMJ 7374: 1212–1213. |
[8] |
Karege F, Perret G, Bondolfi G, et al. (2002) Decreased serum brain-derived neurotrophic factor levels in major depressed patients. Psychiatry Res 109: 143–148. doi: 10.1016/S0165-1781(02)00005-7
![]() |
[9] | NIDA Marijuana National Institute on Drug Abuse, 2017. Available from: https://www.drugabuse.gov/publications/research-reports/marijuana. |
[10] |
Freedland CS, Whitlow CT, Miller MD, et al. (2002) Dose‐dependent effects of Δ9‐tetrahydrocannabinol on rates of local cerebral glucose utilization in rat. Synapse 45: 134–142. doi: 10.1002/syn.10089
![]() |
[11] |
Atakan Z (2012) Cannabis, a complex plant: different compounds and different effects on individuals. Ther Adv Psychopharmacol 2: 241–254. doi: 10.1177/2045125312457586
![]() |
[12] |
Berghuis P, Dobszay MB, Wang X, et al. (2005) Endocannabinoids regulate interneuron migration and morphogenesis by transactivating the TrkB receptor. Proc Natl Acad Sci U S A 102: 19115–19120. doi: 10.1073/pnas.0509494102
![]() |
[13] |
Angelucci F, Ricci V, Spalletta G, et al. (2008) Reduced serum concentrations of nerve growth factor, but not brain-derived neurotrophic factor, in chronic cannabis abusers. Eur Neuropsychopharmacol 18: 882–887. doi: 10.1016/j.euroneuro.2008.07.008
![]() |
[14] | Klug M, Maarten VDB (2013) An investigation into "two hit" effects of BDNF deficiency and young-adult cannabinoid receptor stimulation on prepulse inhibition regulation and memory in mice. Front Behav Neurosci 7: 149. |
[15] |
Boulle F, Van Den Hove DL, Jakob SB et al. (2012) Epigenetic regulation of the BDNF gene: implications for psychiatric disorders. Mol Psychiatry 17: 584. doi: 10.1038/mp.2011.107
![]() |
[16] |
Jones S, Bonci A (2005) Synaptic plasticity and drug addiction. Curr Opin Pharmacol 5: 20–25. doi: 10.1016/j.coph.2004.08.011
![]() |
[17] |
Jacobus J, Tapert SF (2014) Effects of Cannabis on the Adolescent Brain. Curr Pharm Des 20: 2186–2193. doi: 10.2174/13816128113199990426
![]() |
[18] | Weiland BJ, Thayer RE, Depue BE, et al. (2015) Daily marijuana use is not associated with brain morphometric measures in adolescents or adults. J Neurosci 35: 1505–1512. |
[19] |
Gilman JM, Kuster JK, Lee S, et al. (2014) Cannabis Use Is Quantitatively Associated with Nucleus Accumbens and Amygdala Abnormalities in Young Adult Recreational Users. J Neurosci 34: 5529–5538. doi: 10.1523/JNEUROSCI.4745-13.2014
![]() |
[20] | National Institute on Drug Abuse. The NIDA Quick Screen. Screening for Drug Use in General Medical Settings: Resource Guide, March, 2012. Available from: https://www.drugabuse.gov/drugs-abuse/opioids |
[21] |
Levy S, Weiss R, Sherritt L, et al. (2014) An Electronic Screen for Triaging Adolescent Substance Use by Risk Levels. JAMA Pediatr 168: 822–828. doi: 10.1001/jamapediatrics.2014.774
![]() |
[22] |
Lu B (2003) Pro-region of neurotrophins: role in synaptic modulation. Neuron 39: 735–738. doi: 10.1016/S0896-6273(03)00538-5
![]() |
[23] |
Klein AB, Williamson R, Santini MA, et al. (2011) Blood BDNF concentrations reflect brain-tissue BDNF levels across species. Int J Neuropsychopharmacol 14: 347–353. doi: 10.1017/S1461145710000738
![]() |
[24] |
Richardson MT, Ainsworth BE, Jacobs DR, et al. (2001) Validation of the Stanford 7-day recall to assess habitual physical activity. Ann Epidemiol 11: 145–153. doi: 10.1016/S1047-2797(00)00190-3
![]() |
[25] |
Koh HK, Blakey CR, Roper AY (2014) Healthy People 2020: a report card on the health of the nation. JAMA 311: 2475–2476. doi: 10.1001/jama.2014.6446
![]() |
[26] |
Konings M, Henquet C, Maharajh HD, et al. (2008) Early exposure to cannabis and risk for psychosis in young adolescents in Trinidad. Acta Psychiatr Scand 118: 209–213. doi: 10.1111/j.1600-0447.2008.01202.x
![]() |
[27] | Jessor R, Chase JA, Donovan JE (1980) Psychosocial correlates of marijuana use and problem drinking in a national sample of adolescents. Am J Public Health 6: 604–613. |
[28] |
Stefanis NC, Delespaul P, Henquet C, et al. (2004) Early adolescent cannabis exposure and positive and negative dimensions of psychosis. Addiction 99: 1333–1341. doi: 10.1111/j.1360-0443.2004.00806.x
![]() |
[29] |
Decoster J, van Os J, Kenis G, et al. (2011) Age at onset of psychotic disorder: Cannabis, BDNF Val66Met, and sex‐specific models of gene–environment interaction. Am J Med Genet B Neuropsychiatr Genet 156: 363–369. doi: 10.1002/ajmg.b.31174
![]() |
[30] | Hill AB (1965) The Environment and Disease: Association or Causation? Proc R Soc Med 58: 295–300. |
[31] |
Yücel M, Lorenzetti V, Suo C, et al. (2016) Hippocampal harms, protection and recovery following regular cannabis use. Transl Psychiatry 6: e710. doi: 10.1038/tp.2015.201
![]() |
[32] | Shieh PB, Ghosh A (1999) Molecular mechanisms underlying activity-dependent regulation of BDNF expression. J Neurobiol 1: 127–134. |
[33] |
Butovsky E, Juknat A, Goncharov I, et al. (2005) In vivo up‐regulation of brain‐derived neurotrophic factor in specific brain areas by chronic exposure to Δ9‐tetrahydrocannabinol. J neurochemistry 93: 802–811. doi: 10.1111/j.1471-4159.2005.03074.x
![]() |
[34] | Murray PS, Holmes PV (2011) An Overview of Brain-Derived Neurotrophic Factor and Implications for Excitotoxic Vulnerability in the Hippocampus. Int J Pept 654085. |
[35] |
Monteggia LM, Barrot M, Powell CM, et al. (2004) Essential role of brain-derived neurotrophic factor in adult hippocampal function. Proc Natl Acad Sci U S A 101: 10827–10832. doi: 10.1073/pnas.0402141101
![]() |
[36] |
Kleim JA, Chan S, Pringle E, et al. (2006) BDNF val66met polymorphism is associated with modified experience-dependent plasticity in human motor cortex. Nat Neurosci 9: 735–737. doi: 10.1038/nn1699
![]() |
[37] |
Beste C, Kolev V, Yordanova J, et al. (2010) The role of the BDNF Val66Met polymorphism for the synchronization of error-specific neural networks. J Neurosci 30: 10727–10733. doi: 10.1523/JNEUROSCI.2493-10.2010
![]() |
[38] |
Li X, Wolf ME (2015) Multiple faces of BDNF in cocaine addiction. Behav Brain Res 279: 240–254. doi: 10.1016/j.bbr.2014.11.018
![]() |
[39] |
Vargas-Perez H, Ting-A Kee R, Walton CH, et al. (2009) Ventral tegmental area BDNF induces an opiate-dependent-like reward state in naïve rats. Science 324:1732–1734. doi: 10.1126/science.1168501
![]() |
[40] |
Verheij MM, Vendruscolo LF, Caffino L, et al. (2016) Systemic delivery of a brain-penetrant TrkB antagonist reduces cocaine self-administration and normalizes TrkB signaling in the nucleus accumbens and prefrontal cortex. J Neurosci 36: 8149–8159. doi: 10.1523/JNEUROSCI.2711-14.2016
![]() |
[41] | McGinty JF, Whitfield TW, Berglind WJ (2010) Brain-derived neurotrophic factor and cocaine addiction. Brain Res 1314C: 183. |
[42] |
Fujimura H, Altar CA, Chen R, et al. (2002) Brain-derived neurotrophic factor is stored in human platelets and released by agonist stimulation. Thromb Haemost 87: 728–734. doi: 10.1055/s-0037-1613072
![]() |
[43] |
Pan W, Banks WA, Fasold MB, et al. (1998) Transport of brain-derived neurotrophic factor across the blood-brain barrier. Neuropharmacology 37: 1553–1561. doi: 10.1016/S0028-3908(98)00141-5
![]() |
[44] |
Radka SF, Holst PA, Fritsche M, et al. (1996) Presence of brain-derived neurotrophic factor in brain and human and rat but not mouse serum detected by a sensitive and specific immunoassay. Brain Res 709: 122–301. doi: 10.1016/0006-8993(95)01321-0
![]() |
1. | Gregor Jenuš, Žiga Koncilija, Tatjana Hajtnik, Avtomatizirano prekrivanje z arhivskim zakonom varovanih osebni podatkov - anonimizacija, 2021, 2021, 25910884, 149, 10.54356/MA/2021/LSBO6521 | |
2. | Drashti Desai , Pravin Shende, Integration of Internet of Things with Quantum Dots: A State-of-the-art of Medicine, 2021, 27, 13816128, 2068, 10.2174/1381612827666210222113740 | |
3. | Shivani Choudhary, Neeru Sidana, Richa Goel, 2022, chapter 11, 9781668434482, 202, 10.4018/978-1-6684-3448-2.ch011 | |
4. | Abubakar Mohammed, Sanjeev Kumar, Hammandikko Gaya Mu'Azu, Rajiv Kumar, Praveen Shah, Minakshi Memoria, Amarjeet Rawat, Ashulekha Gupta, 2022, Data Security And Protection: A Mechanism For Managing Data Theft and Cybercrime in Online Platforms Of Educational Institutions, 978-1-6654-9602-5, 758, 10.1109/COM-IT-CON54601.2022.9850702 | |
5. | Radi Romansky, Mathematical Model Investigation of a Technological Structure for Personal Data Protection, 2023, 12, 2075-1680, 102, 10.3390/axioms12020102 | |
6. | Radi Petrov Romansky, Irina Stancheva Noninska, Deterministic Model Investigation of Processes in a Heterogeneous E-Learning Environment, 2022, 13, 1947-3478, 1, 10.4018/IJHCITP.293228 | |
7. | Yawen Mi, Enduo Gao, 2022, Information Sharing Security Protection System Based on Artificial Intelligence, 978-1-6654-9111-2, 1, 10.1109/ICMNWC56175.2022.10031986 | |
8. | He-Jun Lu, Juan Liu, 2021, Design and Research of Cloud Virtual Simulation Experiment Teaching Case in the Period of COVID-19, 9781450385800, 1, 10.1145/3510309.3510310 | |
9. | Hongli Yu, Juan He, Keqiang Li, Wen Qi, Jiahui Lin, Anna Szumilewicz, Quality assessment of pre- and postnatal nutrition and exercise mobile applications in the United States and China, 2023, 9, 2296-861X, 10.3389/fnut.2022.942331 | |
10. | Muhammad Shoaib Farooq, Rabia Tehseen, Junaid Nasir Qureshi, Uzma Omer, Rimsha Yaqoob, Hafiz Abdullah Tanweer, Zabihullah Atal, FFM: Flood Forecasting Model Using Federated Learning, 2023, 11, 2169-3536, 24472, 10.1109/ACCESS.2023.3252896 | |
11. | Hasan Fehmi Demirci, Elif Dikmetaş Yardan, Data management in the digital health environment scale development study*, 2023, 23, 1472-6963, 10.1186/s12913-023-10205-3 | |
12. | Yashpal Azad, Amit Kumar, 2024, chapter 12, 9798369317624, 228, 10.4018/979-8-3693-1762-4.ch012 | |
13. | Xingyu Chen, Zhiyi Chen, Lin Lin, Hongyan Yan, Zhiyong Huang, Zhi Huang, A Deep Learning-Based National Digital Literacy Assessment Framework Utilizing Mobile Big Data and Survey Data, 2023, 11, 2169-3536, 108658, 10.1109/ACCESS.2023.3321831 | |
14. | Liangqing Li, University social responsibility, the level of digital ethics and knowledge about data security: The case of first-year and fifth-year students, 2024, 1360-2357, 10.1007/s10639-023-12443-8 | |
15. | Salah A.M. Ali, Abdelmoneim B.M. Metwally, Abdelnasser T.I. Mohamed, 2024, Enhancing AIS Reliability: Suggested Framework for the Role of Trust Service and Artificial Intelligence, 979-8-3503-7222-9, 520, 10.1109/ICETSIS61505.2024.10459587 | |
16. | Ayenew Sisay Gebeyew, Zegeye Regasa Wordofa, Ayana Alebachew Muluneh, Adamu Ambachew Shibabaw, Agmasie Damtew Walle, Sefefe Birhanu Tizie, Muluken Belachew Mengistie, Mitiku Kassaw Takillo, Bayou Tilahun Assaye, Adualem Fentahun Senishaw, Gizaw Hailye, Aynadis Worku Shimie, Fikadu Wake Butta, Attitudes of Health Professionals Toward Digital Health Data Security in Northwest Ethiopia: Cross-Sectional Study, 2024, 16, 1947-2579, e57764, 10.2196/57764 | |
17. | Sunil Kumar Nahak, Sanjit Kumar Acharya, Dushmant Padhy, 2024, Chapter 39, 978-981-97-1322-6, 457, 10.1007/978-981-97-1323-3_39 | |
18. | Mohamed Abomhara, Livinus Obiora Nweke, Sule Yildirim Yayilgan, Debora Comparin, Kristel Teyras, Stéphanie de Labriolle, Enhancing privacy protections in national identification systems: an examination of stakeholders’ knowledge, attitudes, and practices of privacy by design, 2024, 23, 1615-5262, 3665, 10.1007/s10207-024-00905-0 | |
19. | Jenny Guber, Iris Reinhartz-Berger, Privacy-Compliant Software Reuse in Early Development Phases: A Systematic Literature Review, 2024, 167, 09505849, 107351, 10.1016/j.infsof.2023.107351 | |
20. | Petra Vujković, 2024, Measuring Smart Public Governance Maturity in Public Administration Institutions: A Multi-Attribute Approach, 9789612868710, 799, 10.18690/um.fov.4.2024.49 | |
21. | Si-Jung Kim, Do-Eun Cho, Smart City Security Model for Privacy Protection, 2024, 25, 1598-2009, 1281, 10.9728/dcs.2024.25.5.1281 | |
22. | Yuriy Pronyuk, THE TRANSFORMATION OF MANAGERIAL APPROACHES IN THE IMPLEMENTATION OF THE STATE DIGITAL POLICY OF COMMUNICATION AND INFORMATION TECHNOLOGY AT THE CURRENT STAGE, 2023, 2786-5533, 10.52363/passa-2023.1-9 | |
23. | Dasol Choi, Soora Choi, Eunsun Lee, Jinwoo Seo, Dongbin Na, 2024, Towards Efficient Machine Unlearning with Data Augmentation: Guided Loss-Increasing (GLI) to Prevent the Catastrophic Model Utility Drop, 979-8-3503-6547-4, 93, 10.1109/CVPRW63382.2024.00014 | |
24. | Durgasuany Maniraja, Asmaa Mahfoud Al-Hakimi, 2024, Hero Cyber Quest: Cybersecurity Awareness Training Mobile Application, 979-8-3503-8655-4, 114, 10.1109/ICSGRC62081.2024.10691048 | |
25. | Fatma Çiftçi Kıraç, Dijital Sağlık Ortamında Veri Yönetiminin Hasta Memnuniyetine Etkisi, 2024, 21, 1304-8120, 849, 10.33437/ksusbd.1445155 | |
26. | Alanawd Alshehri, A Review of Online Information Privacy Theories Advanced in Eight AIS Journals Over the Last Decade, 2024, 37, 1040-1628, 1, 10.4018/IRMJ.349977 | |
27. | N. Siva, B. Venkata Sivaiah, K. Dharani, K. V. Srilekha, E. Yaswanth, G. Venkateshwarlu, 2024, Federated Learning-Based Flood Forecasting Model Enhancing Predictive Accuracy and Privacy in Flood Prediction, 979-8-3503-6115-5, 1, 10.1109/INCET61516.2024.10593560 | |
28. | Asma Mahfoudh Hezam Al-Hakimi, Muhammad Hassan, 2024, Anti-Social Engineering: The Importance of Social Engineering Awareness Training Web Platform, 979-8-3503-8655-4, 35, 10.1109/ICSGRC62081.2024.10691315 | |
29. | Mahshid Eltemasi, Exploring the intersection of information literacy and rights in the information age: presentation of the conceptual model, 2024, 2514-9342, 10.1108/GKMC-02-2024-0064 | |
30. | Frendika suda utama, Didik Endro Purwoleksono, Taufik Rachman, Data Leakage of the Indonesian Elections Commission in Legal Aspects of Personal Data Protection, 2024, 7, 2621-5225, 479, 10.20473/mi.v7i3.55931 | |
31. | Atul Grover, 2024, chapter 11, 9798369317624, 201, 10.4018/979-8-3693-1762-4.ch011 | |
32. | Shalaka S. Mahadik, Pranav M. Pawar, Raja Muthalagu, Neeli Rashmi Prasad, Sin-Kuen Hawkins, Dimitris Stripelis, Sreedhar Rao, Peter Ejim, Bruce Hecht, Digital Privacy in Healthcare: State-of-the-Art and Future Vision, 2024, 12, 2169-3536, 84273, 10.1109/ACCESS.2024.3410035 | |
33. | Ogechi Adeola, Olaniyi Evans, 2023, Chapter 5, 978-3-031-17862-7, 105, 10.1007/978-3-031-17863-4_5 | |
34. | Munir Ahmad, Asmat Ali, 2023, chapter 13, 9781668499191, 248, 10.4018/978-1-6684-9919-1.ch013 | |
35. | Radi Romansky, 2023, Internet of Things and User Privacy Protection, 979-8-3503-3805-8, 1, 10.1109/InfoTech58664.2023.10266883 | |
36. | Amit Gadekar, S. B. Goyal, Mohd Muqeem, Anand Singh Rajawat, Yao Peiyu, Weiming Chee, 2024, Chapter 36, 978-3-031-50886-8, 359, 10.1007/978-3-031-50887-5_36 | |
37. | D. V. Bakhteev, A. M. Sosnovikova, E. V. Kazenas, Overcoming Illegal Cross-border Transfer of Personal Data, 2024, 2, 2949-2483, 943, 10.21202/jdtl.2024.45 | |
38. | Javeria Aseer, Ansarullah Tantry, Sayed Abdul Majid Gilani, Firas Armosh, Tausif Mulla, Firoz Khan, Richard Peel, 2024, Chapter 37, 978-3-031-75959-8, 387, 10.1007/978-3-031-75960-4_37 | |
39. | Manvi Gupta, Ayush Gupta, Cyber Security Legal Framework in India – Overlaps, Problems and Challenges, 2025, 12, 2394-3130, 11, 10.48001/jbmis.1201002 | |
40. | Daysi Alexandra Tayupanta-Guangatal , María Cristina Mafla-Sánchez , Naomy Hurtado-Acosta , Iruma Alfonso-Gonzáles, Protección de datos personales en era digital [Personal data protection in the digital age], 2024, 3, 3028-872X, 357, 10.62574/6ta6bg70 | |
41. | Jovana Banović, Ivana Radisavljević, Privacy, personal data protection and the digital age: A (criminal) law "omnibus", 2025, 59, 0085-6320, 36, 10.5937/socpreg59-56730 | |
42. | Sarah E. Polk, Fredrik Öhman, Jason Hassenstab, Alexandra König, Kathryn V. Papp, Michael Schöll, David Berron, A scoping review of remote and unsupervised digital cognitive assessments in preclinical Alzheimer’s disease, 2025, 8, 2398-6352, 10.1038/s41746-025-01583-5 |
Appliance type | Number ofInstance | Appliance type | Number ofInstance |
Compact fluorescent lamp | 175 | Fridge | 38 |
Vacuum cleaner | 38 | Incandescent light bulb | 114 |
Hair dryer | 156 | Fan | 115 |
Microwave | 139 | Washing Machine | 26 |
Air conditioner | 66 | Heater | 35 |
Laptop | 172 | Total | 1074 |
Predicted Positive | Predicted Negative | |
Actual Positive | True Positive (TP) | False negative (FN) |
Actual Negative | False Positive (FP) | True Negative (TN) |
Appliance | F-Measure | G-mean |
Compact FL Laptop Microwave Hairdryer Vacuum Cleaner Fridge Heater Il bulb Fan Air-conditioned Total |
0.99 0.72 1 1 1 0.68 0.23 0.97 1 1 0.9 |
1 0.75 1 1 1 0.73 0.21 0.97 1 1 0.94 |
Appliances | K-NN (our result) | CNN [41] |
CFL Laptop Microwave Hairdryer Vacuum Fridge Heater I bulb Fan AC |
99 % 72% 100% 100% 100% 88% 24% 97% 100% 100% |
96% 98% 93% 79% 98% 97% 82% 81% 60% 47% |
Appliance type | Number ofInstance | Appliance type | Number ofInstance |
Compact fluorescent lamp | 175 | Fridge | 38 |
Vacuum cleaner | 38 | Incandescent light bulb | 114 |
Hair dryer | 156 | Fan | 115 |
Microwave | 139 | Washing Machine | 26 |
Air conditioner | 66 | Heater | 35 |
Laptop | 172 | Total | 1074 |
Predicted Positive | Predicted Negative | |
Actual Positive | True Positive (TP) | False negative (FN) |
Actual Negative | False Positive (FP) | True Negative (TN) |
Appliance | F-Measure | G-mean |
Compact FL Laptop Microwave Hairdryer Vacuum Cleaner Fridge Heater Il bulb Fan Air-conditioned Total |
0.99 0.72 1 1 1 0.68 0.23 0.97 1 1 0.9 |
1 0.75 1 1 1 0.73 0.21 0.97 1 1 0.94 |
Appliances | K-NN (our result) | CNN [41] |
CFL Laptop Microwave Hairdryer Vacuum Fridge Heater I bulb Fan AC |
99 % 72% 100% 100% 100% 88% 24% 97% 100% 100% |
96% 98% 93% 79% 98% 97% 82% 81% 60% 47% |