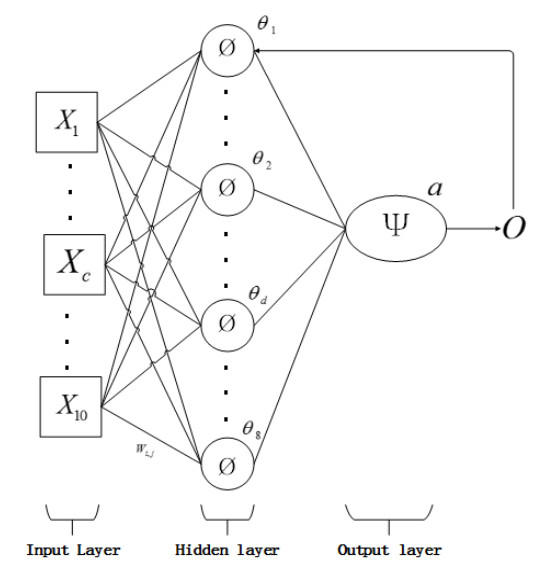
Citation: Wanchun Fan, Yan Jiang, Songyang Huang, Weiguo Liu. Research and prediction of opioid crisis based on BP neural network and Markov chain[J]. AIMS Mathematics, 2019, 4(5): 1357-1368. doi: 10.3934/math.2019.5.1357
[1] | Luigi Accardi, Amenallah Andolsi, Farrukh Mukhamedov, Mohamed Rhaima, Abdessatar Souissi . Clustering quantum Markov chains on trees associated with open quantum random walks. AIMS Mathematics, 2023, 8(10): 23003-23015. doi: 10.3934/math.20231170 |
[2] | Abdessatar Souissi, El Gheteb Soueidy, Mohamed Rhaima . Clustering property for quantum Markov chains on the comb graph. AIMS Mathematics, 2023, 8(4): 7865-7880. doi: 10.3934/math.2023396 |
[3] | Luigi Accardi, El Gheted Soueidi, Abdessatar Souissi, Mohamed Rhaima, Farrukh Mukhamedov, Farzona Mukhamedova . Structure of backward quantum Markov chains. AIMS Mathematics, 2024, 9(10): 28044-28057. doi: 10.3934/math.20241360 |
[4] | Xiaolong Chen, Hongfeng Zhang, Cora Un In Wong . Optimization study of tourism total revenue prediction model based on the Grey Markov chain: a case study of Macau. AIMS Mathematics, 2024, 9(6): 16187-16202. doi: 10.3934/math.2024783 |
[5] | Ciro D'Apice, Alexander Dudin, Sergei Dudin, Rosanna Manzo . Study of a semi-open queueing network with hysteresis control of service regimes. AIMS Mathematics, 2025, 10(2): 3095-3123. doi: 10.3934/math.2025144 |
[6] | Lin Xu, Linlin Wang, Hao Wang, Liming Zhang . Optimal investment game for two regulated players with regime switching. AIMS Mathematics, 2024, 9(12): 34674-34704. doi: 10.3934/math.20241651 |
[7] | Dewang Li, Meilan Qiu, Zhongliang Luo . Huizhou resident population, Guangdong resident population and elderly population forecast based on the NAR neural network Markov model. AIMS Mathematics, 2024, 9(2): 3235-3252. doi: 10.3934/math.2024157 |
[8] | Ilyos Abdullayev, Elvir Akhmetshin, Irina Kosorukova, Elena Klochko, Woong Cho, Gyanendra Prasad Joshi . Modeling of extended osprey optimization algorithm with Bayesian neural network: An application on Fintech to predict financial crisis. AIMS Mathematics, 2024, 9(7): 17555-17577. doi: 10.3934/math.2024853 |
[9] | Spyridon D. Mourtas, Emmanouil Drakonakis, Zacharias Bragoudakis . Forecasting the gross domestic product using a weight direct determination neural network. AIMS Mathematics, 2023, 8(10): 24254-24273. doi: 10.3934/math.20231237 |
[10] | Thanasak Mouktonglang, Zulqurnain Sabir, Muhammad Asif Zahoor Raja, Saira Bhatti, Thongchai Botmart, Wajaree Weera, Chantapish Zamart . Designing Meyer wavelet neural networks for the three-species food chain model. AIMS Mathematics, 2023, 8(1): 61-75. doi: 10.3934/math.2023003 |
The United States is experiencing a national crisis regarding the use of synthetic and non-synthetic opioids, either for the treatment and management of pain (legal, prescription use) or for recreational purposes (illegal, non-prescription use). It is said that opioid abuse is one of the greatest public health challenges of the 21st century. In the United States, this problem can date back to the 1980s. Some scholars think of opioid addiction is not high, and the major producers of opioid drugs also agammaessively marketing its products in pledge, opioid drugs are not addictive, which makes the dosage of opioid present surge trend year by year. The National Forensic Laboratory Information System (NFLIS), as part of the Drug Enforcement Administration's (DEA) Office of Diversion Control, publishes a data-heavy annual report addressing "drug identification results and associated information from drug cases analyzed by federal, state, and local forensic laboratories". The database within NFLIS includes data from crime laboratories that handle over 88% of the nation's estimated 1.2 million annual state and local drug cases. For this problem, we focus on the individual counties located in five U.S. states: Ohio, Kentucky, West Virginia, Virginia, and Tennessee. According to statistics, the number of people taking oxycodone for non-medical use rose rapidly from $400,000 in 1999 to more than $2.8 million in 2003. It may be a huge opioid market that makes the motivation for many pharmaceutical companies to take risks. In recent years, opioids have accounted for more than half of drug overdose deaths in the United States. In terms of health insurance costs, post-addiction treatment, and criminal justice investigations, the United States is losing about $80 billion a year to opioid abuse.
As for the abuse of opioids, the literature at home and abroad mainly focuses on the mechanism of addiction and the qualitative study of socio-economic factors, but there is still no corresponding data research and quantitative analysis, and the prediction analysis and research of the abuse of opioids. But for data prediction, there are many kinds of forecasting methods, including the grey system forecasting method, BP neural network forecasting method, and mathematical statistics forecasting method. At the same time, when studying the situation of drug abuse in the region, it is often affected by local social and economic factors, so BP neural network can adapt to this situation well. It is found that the prediction accuracy can be improved by using the Markov chain to correct the residual value.
Therefore, based on the work done by predecessors and considering the opioid crisis, we take solutions to analyze the drug incidents that happened in Ohio, Pennsylvania, Kentucky, Virginia, and West Virginia. Then propose strategies to deal with the crisis. At first, we use the Sequential Cluster Method to divide the trendless sequence into 6 intervals, determine the drug identification threshold level and introduce State Response Level (SRL) to reflect hazard levels. After that, we develop a Markov Model to forecast the state of future drug incidents. Then, we take the Grey Correlation Analysis to test whether there are correlations between opioid and heroin cases and socio-economic factors. Taking the factors that we select according to the correlation coefficients into account, we build the BP Neural Network and write programs to simulate it. Though BP Neural Network has a certain degree of fitting in the prediction, there is still some error in the results. Hence, Markov Chain Method is adopted to modify them. Finally, we identify strategies for countering the opioid crisis and verify the effectiveness of the strategies.
The Sequential clustering method, a kind of clustering analysis, was first proposed by Fisher in 1958 [1]. It has the characteristics of objective and optimal, which can help minimize the difference between samples within the sample segment and maximize the difference between segment and segment. In this paper, this method is used to segment the occurrence times of synthetic opioid and heroin incidents in each county of the six states. The segmented interval is called state Ej(j=1,2,3,…). Furthermore, we also used this method to introduce State Response Level (SRL), which can reflect hazard levels of opioid events.
The clustering process is as follows:
Step1. Calculate class diameter
In Sequential cluster method, "class diameter" is used to represent the length of each interval, that is, the difference degree of each state. The smaller the diameter, the smaller the difference within the interval will be.The diameters of the classes xi,xi+1,…,xj,(1≤i≤j≤n) are denoted by D(i,j), and the diameter is determined by the sum of squared deviations, which is: D(i,j)=∑jl=i(Xl−ˉXij)2.
Step2. Calculate the error function
To determine how to segment the interval optimally, the error function is introduced to indicate whether the segmentation is good or bad:
E[P(n,k)]=k∑l=1D(il,il+1−1) | (2.1) |
where:
● E[P(n,k)] is defined as the sum of the diameters of the intervals. When n and k fixed, the segmentation that minimize the value of E[P(n,k)] is defined as the optimal segmentation.
Step3. Determine the optimal number of sections We evaluated the segmentation effect by using β the ratio of the sum of squared deviations within the segment No further dividing is necessary when the value approaches 1.The specific formula is as follows:
β=E[P(n,k)]E[P(n,k+1)] | (2.2) |
Model Preparation Based on the closeness of the trend of each factor, Grey correlation analysis is to quantitatively describe the close relationship between each reference sequence and the comparison sequence. As a measure of the degree of association between factors, it is very suitable for dynamic process analysis. The correlation coefficient formula:
ξ(k)=mini(△i(min))+ρmaxi(△i(max))|x0(k)−xi(k)|+ρmaxi(△i(max)) | (2.3) |
where
● ξ(k) means correlation coefficient between data from indicators k and 38 and reported opioid and heroin incidents;
● ρ is the resolution coefficient, 0⩽ρ⩽1.
Among which:
mini(△i(min))=min(mink|x0(k)−xi(k)|) | (2.4) |
maxi(△i(max))=max(maxk|x0(k)−xi(k)|) | (2.5) |
The general expression of the degree of association is:
ri=1/NN∑k=1ξi(k) | (2.6) |
The composition and working mechanism of BP network can be described by the three-layer neural network structure in Figure 1.
where:
● X represents the input from the outside world, the subscript represents the serial number of the node.
● W represents the weight of each layer in the network, and the subscript represents the order of nodes.
● I is hidden layer node.
● J is the input layer node.
● K is the output layer node.
● Θ is the thresholds of the hidden layer.
● α is the thresholds o the output layer, respectively.
● ϕ is the incentive function of the hidden layer.
● ψ is the excitation function of the output layer.
● O is the output portion, the subscripts are used to indicate the order of nodes.
The data is serialized into different states through sequential cluster method, denoted by Em(m=1,2,3,…).
State transition probability Pij:
P(k)ij=m(k)ijMi | (2.7) |
where
● Mi represents Ei the number of occurrences each.
● m(k)ij means from Ei to Ej requires k steps to complete.
● m is the number of states divided.
The state transition matrix is:
P=[P11P12⋯ P1mP21P22⋯ P2m⋮⋮⋱⋮Pn1Pn2⋯ Pnm] | (2.8) |
F=Fg1−q | (2.9) |
where
● Fg represents the predicted value of the BP neural network.
● q represents the boundary value of the Markov chain state interval.
Based on the NFLIS drug identification count for synthetic opioids and heroin from each county in five states between 2010 and 2017 (Table 1), and data on socio-economic factors provided by the U.S. census bureau, our research ideas are shown in the following flow chart (Figure 2):
Philadelphia, PA | Jefferson, KY | Hamilton, OH | |
2010 | 33513 | 5426 | 13780 |
2011 | 26969 | 5953 | 10837 |
2012 | 25279 | 5494 | 10453 |
2013 | 21761 | 5184 | 11063 |
2014 | 17597 | 4512 | 12208 |
2015 | 21387 | 3836 | 14406 |
2016 | 21194 | 3825 | 17376 |
2017 | 19614 | 4635 | 22074 |
According to the sociological theory of drug abuse [2,4], we screened 38 indicators from the 605 indicators given and used MATLAB to calculate correlation coefficients between the 38 indicators and the number of reported opioids and heroin events in Philadelphia County, Jefferson County, and Hamilton County. The top 7 indicators for each county's relevance to opioid and heroin incidents are as follows (Table 2):
Philadelphia, PA | Jefferson, KY | Hamilton, OH | |
I1 | 0.859333 | 0.830618 | 0.836953 |
I2 | 0.839719 | 0.785214 | 0.822447 |
I3 | 0.835311 | 0.798635 | 0.785641 |
I4 | 0.834103 | 0.77384 | 0.802659 |
I5 | 0.795508 | 0.763527 | 0.816289 |
I6 | 0.700659 | 0.774867 | 0.812202 |
I7 | 0.781134 | 0.76568 | 0.798186 |
where:
● I1 represents the proportion of low education level people.
● I2 represents female bad marriage rate.
● I3 represents the proportion of the elderly living alone.
● I4 is male bad marriage rate.
● I5 is the percentage of persons with disabilities.
● I6 is the proportion of veteran.
● I7 is the number of new pregnant women.
The above chart shows the results of the grey relational analysis clearly. The correlation coefficient return by MATLAB indicates that the use of opioids and heroin is somewhat related to the socio-economic data provided by the US Census. We can find that the top 7 indicators of the three counties are the same, but the order is slightly different. In Philadelphia County and Jefferson County, the top three indicators of association with opioid and heroin incidents are: The proportion of low education level people, Female Bad Marriage Rate and the Proportion of the elderly living alone. And in Hamilton County, the top three indicators of association with opioid and heroin incidents are:the proportion of low education level people, Female Bad Marriage Rate and the Percentage of persons with disability.
According to the ranking result of the correlation coefficient, we finally choose the top 7 indicators with high correlation degrees as the input node of the BP network. The reported number of opioids and heroin events is a node of the output layer. After repeated tests, it is found that there are 8 hidden layer nodes and the network structure is 10-8-1. Then we need to determine the optimal parameter setting. The processed data samples are divided into a training set, a verification set, and a test set. By means of cross-validation, corresponding parameters are constantly modified to minimize the error of the verification set. So we set the learning rate as 0.1, the training momentum coefficient as 0.8, the maximum convergence time as 10000, and the minimum error allowed by the training target as 0.00001. The number of training samples is 4 (from 2010 to 2014), and the number of predicted samples is 2. At last, the predicted results are shown in Tables 3, 4 and 5. The Absolute Error and Relative Error formula:
AbsoluteError=PredictedValue−ActualValue | (3.1) |
RelativeError=(PredictedValue−ActualValue)/ActualValue | (3.2) |
Year | Actual Value | Predicted Value | Absolute Error | Relative Error |
2015 | 21387 | 18623 | -2764 | -0.129237 |
2016 | 21194 | 25927 | 4733 | 0.223318 |
Year | Actual Value | Predicted Value | Absolute Error | Relative Error |
2015 | 14406 | 12490 | -1916 | -0.133000 |
2016 | 17376 | 15363.9 | -2012.1 | -0.115798 |
Year | Actual Value | Predicted Value | Absolute Error | Relative Error |
2015 | 4512 | 3894 | -1916 | -0.115798 |
2016 | 5184 | 4656.26 | -527.74 | -0.101802 |
As can be seen from the tables, the BP network has a certain degree of fitting in the prediction. However, there is still some error in the results. Then, the Markov chain method is adopted to modify the results, so as to obtain a better prediction.
In order to get the result closer to the real value, we use Markov method to correct the residual error of the neural network. Since the interval of relative error in 2014 is located in the interval of E3, it can be predicted that the relative error of the prediction sequence is located in the interval of E1 and E2 respectively. Then we take Markov method to modify, and the modifying results are shown in Table 6.
BP Neural Network | Markov Modification | ||||
Year | Actual Value | Predicted Value | Relative Error | Corrected Value | Relative Error |
2015 | 21387 | 18623 | -0.129237 | 21061.34 | -0.01523 |
2016 | 21194 | 25927 | 0.223318 | 21788.54 | 0.028052 |
Obviously, after using Markov correction, the prediction error is reduced from 0.03437 and 0.049446 to 0.01523 and 0.028052, and the prediction accuracy is further improved, which verifies the feasibility of this method. Similarly, the results of other states and counties can be obtained. Some of the results show as Table 7 and Table 8.
BP Neural Network | Markov Modification | ||||
Year | Actual Value | Predicted Value | Relative Error | Corrected Value | Relative Error |
2015 | 14406 | 12490 | -0.133000 | 13974.66 | -0.02994 |
2016 | 17376 | 15363.9 | -0.115798 | 16181.26 | -0.06876 |
BP Neural Network | Markov Modification | ||||
Year | Actual Value | Predicted Value | Relative Error | Corrected Value | Relative Error |
2015 | 4512 | 3894 | -0.115798 | 4281.214 | -0.05115 |
2016 | 5184 | 4656.261 | -0.101802 | 4754.166 | -0.08292 |
In conclusion, the method of using Markov to modify the neural network has great accuracy in the prediction of opioid events and the analysis of fluctuation characteristics, which can be applied to the prediction of a large number of addictive drug events.
Using the methods above, the future state of opioid abuse in each county of the five states was obtained, and the six states correspond to six colors, which were presented by the heat map as follows (Figure 3):
Obviously, opioid abuse in Pennsylvania, Ohio and Kentucky is more serious. Therefore, in order to effectively control the situation of opioid abuse in the region, we proposed corresponding strategies and tested their effectiveness below.
Based on the results given in part 2, we can find that the drug use status of each county is related to the socio-economic data provided by the US Census. For the sake of identifying strategies to address the opioids crisis, we should take measures to control indicators with a high correlation to achieve the effect of controlling drug abuse [3]. In most counties, the educational level and the proportion of elderly people living alone over 65 years of age are highly correlated with drug abuse. On the basis of the (sociology literature), policies should take up with improving the educational level of the region, strengthening the popularization of drug science, improving the level of social welfare to reduce the proportion of elderly living alone, and improving the well-being of the elderly living alone, which can help reduce the number of opioids abuse and addictions to achieving the goal of controlling the regional opioids crisis.
To test the effectiveness of this strategy, we will take Philadelphia as an example and use our model to simulate the prediction of drug identification counts after the policy takes effect from 2013 to 2017 and compare with the actual values. In the test, to effectively test the effect of the policy and eliminate the influence of interfering factors, we will adopt the control variable method, assuming that all the other indicators remain unchanged except the factors tested from 2013 to 2017.
Raising the level of education in a region means that the proportion of low education in a region decreases. It is assumed that after the policy measures were adopted in 2012, the level of low education in the region steadily declined year by year. Add the adjusted index data from 2013 to 2017 to the trained neural network for prediction. Figure 4 shows the effect of the annual percentage decline on Philadelphia's drug identification count: As can be seen from the figure, improving the education level in the region can help reduce the drug use and addiction in the region, thus alleviating the crisis of opioids use. However, it should be noted that when the educational level rises by 5%, the marginal utility of the policy reaches the maximum value, which can be considered as the limit of the success of the policy.
By raising the level of welfare in the region and increasing the attention to the elderly living alone, it will help reduce the excessive use of opioids by elderly living alone due to emptiness, pain and other reasons. assuming that policy measures are taken in 2012, the proportion of elderly people living alone declines, add the adjusted 2013–2017 indicator data to the trained neural network for prediction. The adjusted index data from 2013 to 2017 are added into the trained neural network for prediction. Figure 5 shows the effect of the annual percentage decline (referring to the effectiveness of the policy) on Philadelphia's drug identification count:
As can be seen from the figure, although reducing the proportion of elderly people living alone can reduce the Philadelphia's drug identification count, the effect is not deep and has a certain time lag. To have a significant impact, the annual percentage reduction must be more than 1%, which is difficult to achieve in practice. At the same time, since policies are indirectly applied to the main body through society, there is no direct impact on reducing the proportion of elderly people living alone, and the effectiveness of policies is further weakened. As a result, the policy has tested poorly to contain the opioids crisis.
This paper manages to analyze the use of synthetic and non-synthetic opioids. We use many theories and methods such as Sequential Clustering, Heat Map, Grey Correlation Analysis, Markov Chain. Our model exhibits great potential in concluding below:
● We visualize the data and visually demonstrate the temporal and spatial characteristics of the reported dose of drug events.
● We derive the relation between the socio-economic factors and the drug incidents reported from Grey Relational Analysis.
● We make predictions about future drug incident reports and point out the concerns of the US government.
● Finally, we offer measures for the factors that are highly correlated with the reported amount of drug accidents, and verified the effectiveness of the measures.
The authors declare that there is no conflicts of interest in this paper.
[1] | X. Xianhai, Fisher ordered clustering method and its application in extraction of temperature anomalies in furnace tubes, Guangxi Dianli, (2005), 15-17. |
[2] | H. Dan, A review of sociological theories on drug abuse abroad-a case study of relevant research results in the United States, Realism, (2010), 86-89. |
[3] |
C. S. Davis, A. J. Lieberman, H. Hernandez-Delgado, et al. Laws limiting the prescribing or dispensing of opioids for acute pain in the United States:A national systematic legal review., Drug Alcohol Depen., 194 (2019), 166-172. doi: 10.1016/j.drugalcdep.2018.09.022
![]() |
[4] | W. Ke, P. Hemin, G. Jiatai, Research on the spread of opioid abuse and its trend prediction, Journal of Technology Wind, (2019), 244. |
1. | Haroon Barakat, Osama Khaled, Hadeer Ghonem, Predicting future order statistics with random sample size, 2021, 6, 2473-6988, 5133, 10.3934/math.2021304 | |
2. | Shiho Kino, Yu-Tien Hsu, Koichiro Shiba, Yung-Shin Chien, Carol Mita, Ichiro Kawachi, Adel Daoud, A scoping review on the use of machine learning in research on social determinants of health: Trends and research prospects, 2021, 15, 23528273, 100836, 10.1016/j.ssmph.2021.100836 | |
3. | H. M. Barakat, Haidy A. Newer, Exact prediction intervals for future exponential and Pareto lifetimes based on ordered ranked set sampling of non-random and random size, 2022, 63, 0932-5026, 1801, 10.1007/s00362-022-01295-y | |
4. | Dewang Li, Daming Xu, Meilan Qiu, Shuiping Yang, Forecasting the public financial budget expenditure in Dongguan with an optimal weighted combination Markov model, 2023, 8, 2473-6988, 15600, 10.3934/math.2023796 | |
5. | Dewang Li, Meilan Qiu, Shuiping Yang, Chao Wang, Zhongliang Luo, An optimal fractional-order accumulative Grey Markov model with variable parameters and its application in total energy consumption, 2023, 8, 2473-6988, 26425, 10.3934/math.20231349 | |
6. | Dewang Li, Meilan Qiu, Zhongliang Luo, Huizhou resident population, Guangdong resident population and elderly population forecast based on the NAR neural network Markov model, 2024, 9, 2473-6988, 3235, 10.3934/math.2024157 | |
7. | Mesliha Gezen Ucar, Integrated entropy-based MCDM methods for investigating the effectiveness of Turkey’s energy policies, 2024, 1868-3967, 10.1007/s12667-024-00688-2 | |
8. | Dewang Li, Chingfei Luo, Meilan Qiu, Optimal Weighted Markov Model and Markov Optimal Weighted Combination Model with Their Application in Hunan’s Gross Domestic Product, 2025, 13, 2227-7390, 533, 10.3390/math13030533 | |
9. | Xiong Li, Yande Liu, Yunjuan Yan, Guantian Wang, Discrimination of Geographical Origin for Purple Sweet Potato Using Hyperspectral Imaging Combined with Multivariate Analysis, 2025, 1939-1900, 10.56530/spectroscopy.mk6084y8 |
Philadelphia, PA | Jefferson, KY | Hamilton, OH | |
2010 | 33513 | 5426 | 13780 |
2011 | 26969 | 5953 | 10837 |
2012 | 25279 | 5494 | 10453 |
2013 | 21761 | 5184 | 11063 |
2014 | 17597 | 4512 | 12208 |
2015 | 21387 | 3836 | 14406 |
2016 | 21194 | 3825 | 17376 |
2017 | 19614 | 4635 | 22074 |
Philadelphia, PA | Jefferson, KY | Hamilton, OH | |
I1 | 0.859333 | 0.830618 | 0.836953 |
I2 | 0.839719 | 0.785214 | 0.822447 |
I3 | 0.835311 | 0.798635 | 0.785641 |
I4 | 0.834103 | 0.77384 | 0.802659 |
I5 | 0.795508 | 0.763527 | 0.816289 |
I6 | 0.700659 | 0.774867 | 0.812202 |
I7 | 0.781134 | 0.76568 | 0.798186 |
Year | Actual Value | Predicted Value | Absolute Error | Relative Error |
2015 | 21387 | 18623 | -2764 | -0.129237 |
2016 | 21194 | 25927 | 4733 | 0.223318 |
Year | Actual Value | Predicted Value | Absolute Error | Relative Error |
2015 | 14406 | 12490 | -1916 | -0.133000 |
2016 | 17376 | 15363.9 | -2012.1 | -0.115798 |
Year | Actual Value | Predicted Value | Absolute Error | Relative Error |
2015 | 4512 | 3894 | -1916 | -0.115798 |
2016 | 5184 | 4656.26 | -527.74 | -0.101802 |
BP Neural Network | Markov Modification | ||||
Year | Actual Value | Predicted Value | Relative Error | Corrected Value | Relative Error |
2015 | 21387 | 18623 | -0.129237 | 21061.34 | -0.01523 |
2016 | 21194 | 25927 | 0.223318 | 21788.54 | 0.028052 |
BP Neural Network | Markov Modification | ||||
Year | Actual Value | Predicted Value | Relative Error | Corrected Value | Relative Error |
2015 | 14406 | 12490 | -0.133000 | 13974.66 | -0.02994 |
2016 | 17376 | 15363.9 | -0.115798 | 16181.26 | -0.06876 |
BP Neural Network | Markov Modification | ||||
Year | Actual Value | Predicted Value | Relative Error | Corrected Value | Relative Error |
2015 | 4512 | 3894 | -0.115798 | 4281.214 | -0.05115 |
2016 | 5184 | 4656.261 | -0.101802 | 4754.166 | -0.08292 |
Philadelphia, PA | Jefferson, KY | Hamilton, OH | |
2010 | 33513 | 5426 | 13780 |
2011 | 26969 | 5953 | 10837 |
2012 | 25279 | 5494 | 10453 |
2013 | 21761 | 5184 | 11063 |
2014 | 17597 | 4512 | 12208 |
2015 | 21387 | 3836 | 14406 |
2016 | 21194 | 3825 | 17376 |
2017 | 19614 | 4635 | 22074 |
Philadelphia, PA | Jefferson, KY | Hamilton, OH | |
I1 | 0.859333 | 0.830618 | 0.836953 |
I2 | 0.839719 | 0.785214 | 0.822447 |
I3 | 0.835311 | 0.798635 | 0.785641 |
I4 | 0.834103 | 0.77384 | 0.802659 |
I5 | 0.795508 | 0.763527 | 0.816289 |
I6 | 0.700659 | 0.774867 | 0.812202 |
I7 | 0.781134 | 0.76568 | 0.798186 |
Year | Actual Value | Predicted Value | Absolute Error | Relative Error |
2015 | 21387 | 18623 | -2764 | -0.129237 |
2016 | 21194 | 25927 | 4733 | 0.223318 |
Year | Actual Value | Predicted Value | Absolute Error | Relative Error |
2015 | 14406 | 12490 | -1916 | -0.133000 |
2016 | 17376 | 15363.9 | -2012.1 | -0.115798 |
Year | Actual Value | Predicted Value | Absolute Error | Relative Error |
2015 | 4512 | 3894 | -1916 | -0.115798 |
2016 | 5184 | 4656.26 | -527.74 | -0.101802 |
BP Neural Network | Markov Modification | ||||
Year | Actual Value | Predicted Value | Relative Error | Corrected Value | Relative Error |
2015 | 21387 | 18623 | -0.129237 | 21061.34 | -0.01523 |
2016 | 21194 | 25927 | 0.223318 | 21788.54 | 0.028052 |
BP Neural Network | Markov Modification | ||||
Year | Actual Value | Predicted Value | Relative Error | Corrected Value | Relative Error |
2015 | 14406 | 12490 | -0.133000 | 13974.66 | -0.02994 |
2016 | 17376 | 15363.9 | -0.115798 | 16181.26 | -0.06876 |
BP Neural Network | Markov Modification | ||||
Year | Actual Value | Predicted Value | Relative Error | Corrected Value | Relative Error |
2015 | 4512 | 3894 | -0.115798 | 4281.214 | -0.05115 |
2016 | 5184 | 4656.261 | -0.101802 | 4754.166 | -0.08292 |