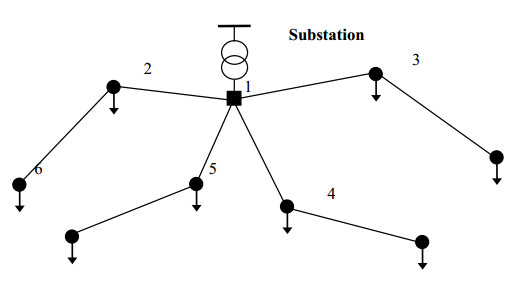
Citation: V. V. Thang, Thanhtung Ha. Optimal siting and sizing of renewable sources in distribution system planning based on life cycle cost and considering uncertainties[J]. AIMS Energy, 2019, 7(2): 211-226. doi: 10.3934/energy.2019.2.211
[1] | Li Bin, Muhammad Shahzad, Qi Bing, Muhammad Ahsan, Muhammad U Shoukat, Hafiz MA Khan, Nabeel AM Fahal . The probabilistic load flow analysis by considering uncertainty with correlated loads and photovoltaic generation using Copula theory. AIMS Energy, 2018, 6(3): 414-435. doi: 10.3934/energy.2018.3.414 |
[2] | Sonia Longo, Maurizio Cellura, Francesco Guarino, Vincenzo La Rocca, Giuseppe Maniscalco, Massimo Morale . Embodied energy and environmental impacts of a biomass boiler: a life cycle approach. AIMS Energy, 2015, 3(2): 214-226. doi: 10.3934/energy.2015.2.214 |
[3] | Abdulrahman Th. Mohammad, Amer Ibrahim Ismael . An equivalent photovoltaic solar system to solve the problems of electricity in Iraqi houses. AIMS Energy, 2019, 7(5): 660-670. doi: 10.3934/energy.2019.5.660 |
[4] | Ryuto Shigenobu, Oludamilare Bode Adewuyi, Atsushi Yona, Tomonobu Senjyu . Demand response strategy management with active and reactive power incentive in the smart grid: a two-level optimization approach. AIMS Energy, 2017, 5(3): 482-505. doi: 10.3934/energy.2017.3.482 |
[5] | Mowffaq M. Oreijah, Mohammed Yunus . A parametric analysis to evaluate the performance metrics of power generation system involving Trilateral Flash Cycle using three different working fluids for low grade waste heat. AIMS Energy, 2019, 7(4): 483-492. doi: 10.3934/energy.2019.4.483 |
[6] | Albert K. Awopone, Ahmed F. Zobaa . Analyses of optimum generation scenarios for sustainable power generation in Ghana. AIMS Energy, 2017, 5(2): 193-208. doi: 10.3934/energy.2017.2.193 |
[7] | Abshir Ashour, Taib Iskandar Mohamad, Kamaruzzaman Sopian, Norasikin Ahmad Ludin, Khaled Alzahrani, Adnan Ibrahim . Performance optimization of a photovoltaic-diesel hybrid power system for Yanbu, Saudi Arabia. AIMS Energy, 2021, 9(6): 1260-1273. doi: 10.3934/energy.2021058 |
[8] | Jinhua Tian, Yueyuan Zhang, Yanan Gao, Yu Qin, Bihan Fan, Cheng Zhang, Qiqi Zang . Distributed optimization and scheduling strategy for source load storage distribution grid based on alliance chain. AIMS Energy, 2024, 12(5): 946-967. doi: 10.3934/energy.2024044 |
[9] | Khuthadzo Kgopana, Olawale Popoola . Improved utilization of hybrid energy for low-income houses based on energy consumption pattern. AIMS Energy, 2023, 11(1): 79-109. doi: 10.3934/energy.2023005 |
[10] | Matteo Moncecchi, Davide Falabretti, Marco Merlo . Regional energy planning based on distribution grid hosting capacity. AIMS Energy, 2019, 7(3): 264-284. doi: 10.3934/energy.2019.3.264 |
The application of renewable sources (RS) contributes to the economic benefits and technical advances in the distribution system (DS). The RS are conducive when reducing the losses of power and voltage, improving flexibility and reliability, extending equipment life of the system as well as lessening the costs of purchasing energy from the market [1,2,3,4,5,6]. Additionally, the RS integrated in energy systems can reduce the pollutant emissions including CO, CO2, SO2 and NOx from traditional energy sources [7,8,9]. Therefore, the many researches have considered the application of RS in the planning and operating of DS with different approaches used for the optimization of RS generation and integration subproblems [10]. The long-term planning model is proposed to maximize the benefit of a project integrating the RS [11]. Similarly, the model planning the DS considering the distributed generators is also introduced with the objective is minimizing the investment and operation cost of distributed generators, cost to upgrade feeder and substations during the planning period, energy expenses purchased from markets and emission costs [12]. The planning models to simultaneously select optimal allocation of RS and capacitor banks in DS are also introduced in researches [13,14]. The objective function is minimizing the total cost of investment and operation of DS, pollutants emission cost of resources. The results showed that the RS have great effect in DS planning by reducing for power and energy loss, energy cost and emissions.
A new methodology is proposed to optimize the hybrid renewable energy system with various system configurations based on reliability and economic constraints [15]. The objective function is utilized to minimize the net present cost of project computed by HOMER software. Similarly, HOMER is also applied to optimize the configuration for grid-connected hybrid power systems considering RS such as PV and wind turbine [16]. The results is for computing the optimum system architecture and size of equipment, at the same time, the hybrid system reduces the significant amount of greenhouse gas emissions leading to greater environmental impact compared to the existing system. However, the above studies only compute with average load, electrical price, solar radiation and wind speed while they always change and are stochastic values. In addition, the lifetime and uptime of equipment on the planning period are not the same, the planning time is usually shorter than the equipment life cycle and the impact of reactive power is ignored in optimal problems.
Hence, life cycle cost (LCC) during computation time is introduced to analyze planning and operating of RS in energy systems [17,18,19,20]. Beside, the output power of wind turbine (WT) and photovoltaic (PV) as well as the load and electrical price are stochastic parameters that are usually expressed by the probability density function (pdf) in which the normal probability density function is chosen for modeling the uncertainties of the load and electrical price [21]. Similarly, the wind speed is modeled by the weibull probability density function and the beta probability density function is selected to present the irradiance data [22,23,24,25,26,27]. The output power of WT and PV depends on wind speeds and radiations, respectively, and thus those are stochastic parameters. The stochastic parameters are divided into levels (states) by clustering technique with specific values and different probabilities. In this context, a combined model are utilized to integrate the output power of WT and PV, the load and energy price [25,28,29]. The planning of the DS considering uncertainties is presented at several researches with stochastic parameters including the load, electrical, output power of the RS. The siting and sizing of the RS, as well as upgrading capacity and time of feeders, are concurrently chosen with the cost of the object at the present value [22,23,24,25,26,27,28,29,30,31] or power loss [32]. Similarly, the stochastic optimization approaches are also utilized to examine uncertainties in optimizing the configuration of energy systems. The objective is minimizing the cost of energy of over a particular time horizon [33] or the LCC which constitutes the capital cost, the replacement cost, the resale cost, and the operations and maintenance costs of the system [34]. However, the lifetime and uptime of the equipment have not been considered and so the error of the planning problem is large. Additionally, the optimal site selection of electric vehicle charging stations based on a bayesian network model is proposed, which simultaneously consider the uncertainty and the economic, environmental, and social criteria [35]. The optimal integration of electric vehicles and RS in a smart residential district is also introduced. The results showed that the amounts of power from RS reduce the annual energy cost of district [36].
Therefore, this research proposes a planning framework to analyze the optimal siting, sizing and technology of the RS with the objective function being minimum LCC during the planning period and considers uncertainties of the DS. The lifetime and uptime of equipment examined as well as the LCC objective function increase the accuracy of planning results. The LCC includes the energy cost, emission taxes, the investment and operation cost as well as the residual value of RS at the end of the calculation period. The technical parameters and economic effectiveness of the DS are determined and the normal operation of the DS is guaranteed by constraints. A combination model is utilized to integrate the uncertain parameters as demand, electrical price and output power of RS. The loss and operation parameters of the DS are analyzed at the all of states with the alternating current (AC) constraints contributing to the reduction of errors. The planning problem with uncertainties is very complex and thus GAMS/SCENRED is employed to quickly reduce the scenario number of the problem while guaranteeing the small error of calculation results as shown in researches [37,38,39,40].
Next section of the paper represents the modeling of uncertain parameters, the modeling problem with objective function and constraints presents in section 3. Section 4 shows results analyzed for the test system and the conclusion demonstrates in section 5.
The output power of RS changes random depending on natural intermittent of primary sources. Similarly, the energy price and electrical demand also are stochastic parameters. Hence, the pdf is often utilized to model uncertainties of parameters in DS as introduced in researchers [31,41]. Because the pdf is the continuous function, the clustering technique is utilized to divide stochastic parameters into different states. In each state, there is a specific value with the related probability.
The load at buses randomly change according to demand of consumers and is modeled by the normal probability density function as present at Eq 1 in which the µ is mean of the distribution, σ is the standard deviation and σ2 is variance [28,41,22,26]. The electricity price is also a stochastic parameter at the competitive market and expressed by the normal probability density function as Eq 1 [22,26].
P(X=x|μ,σ2)=f(x)=1√2πσexp[−(x−μ)22σ2] | (1) |
A value related with probability at each state of solar irradiance is expressed by beta probability density function as Eq 2 [29,44] in which the Iir is the solar irradiance, µ is mean and σ is the standard deviation of the stochastic variable.
fb(Iir)={Γ(α+β)Γ(α)Γ(β).Iir(α−1).(1−Iir)(β−1)if0≤Iir≤1,α,β0elseβ=(1−μ).(μ.(1+μ)σ2−1);α=μ.β1−μ | (2) |
In each state, the output power is analyzed as expression (3) in which the kF.s is the fill factor and the n is the number of modules. The Is, Us are the generated current and voltage of the PV module at state s. Besides, the ISC and UOC are short circuit current and open circuit voltage, the UMPP and IMPP are current and the voltage at the maximum power point, respectively. The kU, kI are the coefficients depending on temperature, voltage and current of PV. The θA, θN are the ambient and nominal operating temperature of module PV.
Similarly, the wind speed also changes random represented under rayleigh probability density function which is a special case of weibull probability density function as expression (4) with the shape index k = 2 and the scale index c = 1.128vm [28,29,23].
PPVs(Iir)=n.kF.s.Us(Iir).Is(Iir)kF.s=UMPP.s.IMPP.sUOC.ISCUs(Iir)=UOC−kU.(θA+Iir.s.θN−200.8)Is(Iir)=Iir.s.[ISC+kI.(θA+Iir.s.θN−200.8−25)] | (3) |
fr(v)=(2vc2)exp[−(vc)2] | (4) |
The value and probability of wind speed in each state are determined when the clustering technique is utilized to divide the pdf into the states. In this context, the output power of the WT is calculated as Eq 5.
PWTs(v)={0 vs≤vci or vco≤vsPWTr.vs−vcivr−vci vci≤vs≤vrPWTr vr≤vs≤vco | (5) |
where, the PWTs and PWTr are output and rated power of WT. The vci, vcr, and vco are the cut-in speed, rated speed and cut-off speed of WT, respectively.
The multi-state parameters of the DS are examined by utilizing a combinable model to integrate all possible states of parameters [28,29]. Hence, the matrix M is represented as Eq 6 to enumerate all combination of states of the load, electricity price and output power of RS. In which, the Cs is the matrix that enumerates the possible values of parameters, the λs{ Cs} is one-column matrix that represents probability corresponding with matrix Cs and the Ns is the sum of individual states of the matrix M which is computed by product the possible states of the parameters.
M= { Cs, λs { Cs } } λs { Cs } = λρs { ρs } .λLs { PLs } .λWTs { PWTs } .λPVs { PPVs } Ns=nρs.nLs.nWTs.nPVs∀s∈Ns | (6) |
where, the ρs, PLs are the electricity price and load corresponding with probabilities λρs and λLs. The PWTs, PPVs are the output power of WT and PV corresponding with probabilities λWTs and λPVs, respectively. The nλs, nLs are the numbers of states assumed of the electricity price and load while thenWTs,nPVsare the numbers of states of the output power of RS, respectively.
The numbers of scenarios in matrix M are very big depending on choosing state number in each pdf. Therefore, the GAMS/SCENRED tool is employed to quickly reduce the scenario number of problem lead to decrease the computable volume and time while the results are guaranteed with the small error [42]. Beside, the choosing state numbers are very important because of its affect to the accuracy of results and the ability to calculate. The higher numbers of states selected, the higher accuracy is and increasing complexity of computation and vice versa.
When the renewable sources participate in the DS, the economic benefits and the electrical quality of this system are considerably enhanced. However, the invested cost of the RS is often high and siting, sizing as well as technique of the RS significant effect to efficiency of planning problem and thus a planning framework should be created to optimize the invested parameters of the RS in the DS planning. In this study, the mathematic model with the LCC objective function can examine the different lifetime and uptime of the RS in any planning period. Moreover, the binary variables and uncertainty of parameters in the DS added in objective and constrains greatly enhance the accuracy and appropriateness of investment decisions in practice. The mathematic model of the problem, which determines siting, sizing and locating of RS in DS, includes the objective and constrains detailed as follows.
The objective function of the model is the LCC of the project during the planning period as presented in Eq 7.
LCC=T∑t=11(1+r)t(NRS∑i=1NK∑k=1CRSP.k.PRSk,i,t+8760NK∑k=1NRS∑i=1NS∑s=1λs.ρRSOM.k.PRSk,i,t,s+8760NT∑i=1NS∑s=1kρ.λs(ρPs.PTi,t,s+ρQs.QTi,t,s)+8760NRS∑i=1NK∑k=1NS∑s=1β.(ξT−ξRS).λs.PRSk,i,t,s−TRSC.k−tRSinv.kTRSC.kNRS∑i=1NK∑k=1CRSP.k.PRSk,i,t) | (7) |
In Eq 7, the first and second parts are the investment and operation cost of RS, the third part is cost to purchase energy from the grid, the fourth part is emission taxes cost reduced due to low emissions of RS and the last part is residual value of equipment at the end of planning period. All of them are calculated at the base year by discount rate r.
Where, CRSP.k is the invested cost of the RS with technology k; PRSk,i,t,s is the out power variables of the RS; ρRSOM.k is operation and management (O & M) of the RS; PTi,t,s,QTi,t,s are the active and reactive powers received from main grid; ρPs,ρQs are electrical energies price with rise factor in each year kρ; ξT,ξRS are CO2 emission coefficient of the traditional sources and RS with emission tax of CO2 is β; NT is total buses connected to main grid; NRS is total buses for installing the RS; k, NK are technology type and technologies set of the RS considered for selection; t, T are year and planning period; TRSC.k,tRSinv.k are lifetime and invested time of the RS with technology k.
The nonlinear power flow model AC is selected to calculate both active and reactive power loss and thus the calculating operation cost of the DS is more accurate. They are reformed to integrate the RS as represented in Eq 8 with state index s of uncertainty parameters. The load at buses and the output power depending on technology k of RS are computed according to factors and rated powers present in Eqs 9 and 10. Similarly, the electricity prices are computed as Eq 11 with the peak price of active power is ρP and reactive power isρQ.
PTi,t,s−PLi,t,s+NK∑k=1PRSk,i,t,s=N∑j=1|Yij,t|.|Ui,t,s|.|Uj,t,s|.cos(θij,t−δj,t,s−δi,t,s)QTi,t,s−QLi,t,s+NK∑k=1QRSk,i,t,s=−N∑j=1|Yij,t|.|Ui,t,s|.|Uj,t,s|.sin(θij,t−δj,t,s−δi,t,s)∀i∈N,t∈T,s∈NS | (8) |
PLi,t,s=kLs.PLi,tQLi,t,s=kLs.QLi,t∀i∈N,t∈T,s∈NS | (9) |
PRSk,i,t,s=kRSk,s.PRSk,i,t;QRSk,i,t,s=PRSk,i,t,s.tanφk ∀k∈NK,i∈N,t∈T,s∈NS | (10) |
ρPs=kρs.ρPρQs=kρs.ρQ∀s∈NS | (11) |
where PLi,t,s,QLi,t,s are the active and reactive powers of the loads with demand factor at state s kLs; |Ui,t,s|,δi,t,sare module and angle of bus voltages; |Yij,t|,θij,t are magnitude and angle of admittance matrix element formulated by the feeder impedances in year t; The out power of the RS at state s is reflected by coefficient kRSk,h; ρP,ρQ are maximum price of electrical energies at hour h and kρs is coefficient analyzing at state s.
The binary variable γk, i, t is utilized to limit sizing and power of RS that those can be chosen as shown in Eq 12. Each type of RS should only be invested one time at each bus during the planning period.
PRSk,i,t≥0;PRSk,i,t−PRSk.max.γk, i, t≤0;T∑t=1γi, k, t≤1∀k∈NK,i∈N,t∈T | (12) |
Beside, the each feeder or connectable substation has a capacity limit for total power flow through it in all states. Because of that, the constraints (13) are proposed to guarantee that the equipment of the DS is not overloaded with the limited capacity of feeders SFij.maxand connectable substationSTi.max. Similarly, the voltage profile at buses must be guaranteed in a limit allowing the ordinary operation of the system as shown in Eq 14. At the substation bus connected to the grid, the voltage is often stability so it is assumed the constant. The voltage at load buses is usually varied under change of load. Hence, the voltage is limited as constrains (14) with minimum voltage profile Umin and maximum voltage profile Umax.
SFij,t,s≤SFij.max; STi,t,s≤STi.max ∀ij∈N,t∈T,s∈S | (13) |
|Ui,t,s|=constant ∀i∈NT,t∈T,s∈NSUmin≤|Ui,t,s|≤Umax ∀i∈N,t∈T,s∈NS | (14) |
The proposed model is Mixed-Integer Nonlinear Programming (MINLP) and thus the GAMS/BONMIN solver is utilized to optimize the planning problem [40].
The radial diagram 9-bus with a connectable substation introduced by researches [13,15] as represented in Figure 1 is selected to investigate the feasibility and efficiency of the proposed model. The data of the system is modified to match the problem in which the normal voltage is 22 kV, the load at buses presents in the appendix and grows 5% annually. The peak demand total at the base year is 17.95 MW and 14.08 MVAr, respectively. A substation is connected to the main grid at bus 1 to feed energy for loads with maximum capacity 25 MVA.
Because of quick installation and small space, the candidate site chose to install the RS are the load buses. The power limit at each bus assumes 5 MW and the discrete step selected to calculate is 0.1 MW [28,29]. The cost of investment, average O & M and the analyzed parameter of RS are presented on table 1 [43,44]. Besides, the emission coefficient and tax of resources are also introduced on table 2 [12,31].
Type of RS | Parameters | Value | Parameters | Value |
PV | Peak power, Pr (kW) | 100 | kU (mV/℃); kI (mA/℃) | 14.4; 1.22 |
UOC; UMPP (V) | 22; 17.32 | ISC; IMPP (A) | 5.3; 4.76 | |
Life time (year) | 25 | θN; θA (℃) | 25; 43 | |
Invested cost (M$/MW) | 1.5 | O & M cost ($/MWh) | 20 | |
WT | Rate power, Pr (kW) | 100 | vr (m/s) | 14 |
vci (m/s) | 4 | vco (m/s) | 24 | |
Invested cost (M$/MW) | 1.8 | O & M cost ($/MWh) | 40 | |
Life time (year) | 25 |
Parameters | Value |
Emission tax of CO2, β | 10 $/ton |
Emission coefficient of traditional sources, ξT | 0.68 kg/kWh |
Emission coefficient of RS, ξRS | 0.02 kg/kWh |
Discount rate, r | 10% |
The voltage at the substation bus is assumed the constant equaling 1.05 pu while the voltages at load buses allow change from 0.9 pu to 1.1 pu.
The stochastic parameters, which are conceptualized under the pdf, are divided by clustering technique into states under the annual data as shown in Table 3. The 15 levels (statuses) with the specific value and related probability to guarantee a reasonable balance between accuracy and computed speed are selected to analyze in this research [28,29,22,23,24,25,26].
State | PV | WT | Load | Electrical price | ||||
kRSs | λPVs | kRSs | λWTs | kLs | λLs | kρs | λρs | |
1 | 0 | 0.450 | 0 | 0.199 | 0.30 | 0.001 | 0.30 | 0.012 |
2 | 0.06 | 0.002 | 0.1 | 0.112 | 0.35 | 0.003 | 0.35 | 0.023 |
3 | 0.12 | 0.005 | 0.2 | 0.113 | 0.40 | 0.010 | 0.40 | 0.041 |
4 | 0.18 | 0.012 | 0.3 | 0.106 | 0.45 | 0.028 | 0.45 | 0.065 |
5 | 0.23 | 0.023 | 0.4 | 0.096 | 0.50 | 0.062 | 0.50 | 0.092 |
6 | 0.29 | 0.039 | 0.5 | 0.085 | 0.55 | 0.111 | 0.55 | 0.116 |
7 | 0.35 | 0.057 | 0.6 | 0.072 | 0.60 | 0.159 | 0.60 | 0.131 |
8 | 0.40 | 0.073 | 0.7 | 0.059 | 0.65 | 0.184 | 0.65 | 0.132 |
9 | 0.45 | 0.083 | 0.8 | 0.047 | 0.70 | 0.172 | 0.70 | 0.119 |
10 | 0.50 | 0.084 | 0.9 | 0.036 | 0.75 | 0.130 | 0.75 | 0.096 |
11 | 0.55 | 0.072 | 1.0 | 0.027 | 0.80 | 0.079 | 0.80 | 0.070 |
12 | 0.60 | 0.053 | 1.0 | 0.020 | 0.85 | 0.039 | 0.85 | 0.045 |
13 | 0.65 | 0.031 | 1.0 | 0.014 | 0.90 | 0.015 | 0.90 | 0.026 |
14 | 0.70 | 0.013 | 1.0 | 0.009 | 0.95 | 0.005 | 0.95 | 0.014 |
15 | 0.75 | 0.003 | 1.0 | 0.006 | 1.00 | 0.001 | 1.00 | 0.006 |
The agammaegation of uncertainty parameters is made by matrix M with 154 (50625) scenarios, the GAMS/SCENRED tool utilized to reduce number of scenarios to 15 [42].
The GAMS/BONMIN solver is applied to optimize the planning problem. The simulation result determines the siting, sizing and time invested of each RS as table 4. The WT and PV are chosen to install at buses, which are far connectable substation as buses 7, 8 and 9 because they decrease the loss and energy cost. The total power of PV chose is 7.0MW in the first year to salvage the low operation cost of this source. Similarly, the 8.1MW is the rated power of WT installed in DS at the 6th year.
Type of RS | Siting (bus) | Rated power (MW) | Planning year |
PV | 7 | 2.3 | 1 |
8 | 2.3 | 1 | |
9 | 2.4 | 1 | |
WT | 7 | 2.7 | 6 |
8 | 2.6 | 6 | |
9 | 2.8 | 6 |
The voltage profile at buses is improved because of loss in the reduction of DS as shown in Figure 2. The lowest voltage profile when without RS is 0.88 pu at bus 7 of the 10th planning year. Therefore, the electrical quality does not guarantee supply to consumers. Conversely, the voltage profiles at all buses are guaranteed during planning period with value being greater than 0.92 pu in case with RS.
The power and energy loss also decreased due to a decrease in power flow in the DS as presented in Figure 3. The PV installed at first year resulted in the decrease of maximal power loss at beginning of planning time by 2%, and increase by 3.97% at the 10th year when the WT was applied at the 6th year. Besides, the RS reduces energy loss of the DS as well as energy cost from the market. The electrical energy obtained from the market decreased from 1,098,100.0 MWh to 858,310.0 MWh in correspondence with 239,790.0 MWh or 21.84%. The CO2 emission of the RS equals only 2.9% of the traditional sources or the reduction is about 0.66 tons/MWh and thus the CO2 emission drops 158,261.4 tons at the case with RS as shown in table 5. In this case, the emission from the traditional sources is 583,650.8 tons while from the RS is only 4,795.8 tons.
Case | Total life cycle cost, M$ | Emission taxes cost, M$ | CO2 emission, ton |
Without RS | 82.92 | 5.34 | 746,708.0 |
With RS | 76.58 | 4.22 | 588,446.6 |
Comparison of two cases | 6.34 | 1.12 | 158,261.4 |
As presented in table 5, the effect of investment project considering the RS is verified along with the reduction of LCC. The LCC decreased by 7.65% (6.34 M$) when the RS is involved. Similarly, the emission taxes in the case of RS is only 4.22 M$, 1.12 M$ less compared to the situation without RS. The simulation result shows the feasibility and effectiveness of the proposed model as well as the benefit brought by RS to the DS when the stochastic parameters are included in the problem.
This research introduced a model to optimize the invested siting, sizing, setting and time of the RS in the DS considering the uncertain parameters. The developed model features a number of important aspects:
● The planning framework proposed with the objective function that minimizes the LCC of investment project including the investment and operation cost of the RS, the cost for purchasing the energy from market, the emission taxes cost and the residual value of equipment at the end of the analysis period. Therefore, the different lifetime and uptime of the RS are examined which improves the accuracy and suitability for the practical planning problem.
● A combinable model with the clustering technique is utilized to integrate the multi-state uncertain parameters of planning problem as the electrical price, load, out power of the PV and WT.
● The binary variables are integrated in MINLP model to perform the invested decision of the RS with limited power and selected power, which agree with the actual parameters.
● The simulation results demonstrate how the application of the RS in DS could promote the reduction of LCC, and minimize power losses and emission cost during the planning stage. The voltage profile at buses and the flexibility of system operation could also be improved when the RS is considered when investing in DS planning problem.
The cases study has illustrated the feasibility and effectiveness of the proposed model with uncertainty parameters of the DS, which indicates that the optimization method could be brought into practice. However, upgrading the feeders and substations during planning period can be deferred when RS are selected to invest because it reduces the power transmitted from the utility grid. The simultaneous selection the siting, sizing of the RS and upgrading the feeders and substations of the DS would also be an interesting work for future research.
Bus i-j | SFij.max(MVA) | Lij (km) | Rij (Ω) | Xij (Ω) |
1-2 | 6.67 | 5.3 | 4.10 | 2.27 |
1-3 | 6.67 | 9.2 | 7.11 | 3.95 |
1-4 | 6.67 | 10.3 | 7.96 | 4.42 |
1-5 | 6.67 | 10.5 | 8.12 | 4.50 |
2-6 | 6.67 | 11.7 | 9.04 | 5.02 |
3-7 | 6.67 | 18.2 | 14.07 | 7.81 |
4-8 | 6.67 | 17.2 | 13.30 | 7.38 |
5-9 | 6.67 | 15.2 | 11.75 | 6.52 |
Bus | PLi(MW) | QLi(MVAr) | Bus | PLi(MW) | QLi(MVAr) |
1 | - | - | 6 | 1.58 | 1.37 |
2 | 2.83 | 2.29 | 7 | 1.31 | 1.14 |
3 | 2.78 | 2.38 | 8 | 1.16 | 0.98 |
4 | 2.95 | 1.99 | 9 | 1.22 | 1.05 |
5 | 2.86 | 1.90 | Total | 16.69 | 13.10 |
The research is supported by Thai Nguyen University of Technology (TNUT), Thai Nguyen, Viet Nam.
The author declares no conflict of interest in this paper.
[1] | Sambaiah KS (2018) A review on optimal allocation and sizing techniques for DG in distributionsystems. Int J Renew Energy Res 8: 1236–1256. |
[2] |
Taner G (2019) Renewable energy, non-renewable energy and sustainable development. Int J Sustainable Dev World Ecology 26: 1–10. doi: 10.1080/13504509.2018.1471012
![]() |
[3] |
Georgilaki PS, Hatziargyriou ND (2015) A review of power distribution planning in the modern power systems era: Models, methods and future research. Electr Power Syst Res 121: 89–100. doi: 10.1016/j.epsr.2014.12.010
![]() |
[4] | Bhadoria VS, Singh N, Shrivastava V (2013) A review on distributed generation definitions and DG impacts on distribution system. International Conference on Advanced Computing and Communication Technologies 1–7. |
[5] |
Theo WL, Limb JS, Ho WS, et al. (2017) Review of distributed generation (DG) system planning and optimisation techniques: Comparison of numerical and mathematical modelling methods. Renew Sust Energy Rev 67: 531–573. doi: 10.1016/j.rser.2016.09.063
![]() |
[6] | Mohandes B, Moursi MSE, Hatziargyriou ND, et al. (2019) A review of power system flexibility with high penetration of renewables. IEEE Trans Power Syst (Early Access) 2019: 1–13. |
[7] | Thang VV, Trung NH (2019) Evaluating efficiency of renewable energy sources in planning micro-grids considering uncertainties. J Energy Syst 3: 14–25. |
[8] |
Bayod-Rújula AA, Yuan Y, Martínez-Gracia A, et al. (2018) Modelling and simulation of a building energy hub. Proceedings 2: 1–6. doi: 10.3390/proceedings2010001
![]() |
[9] | Deng S, Wu Q, Jing Z, et al. (2017) Optimal capacity configuration for energy hubs considering part-load characteristics of generation units. Energies 10: 2–19. |
[10] | Acharya PS, Wagh MM, Kulkarni VV (2019) Intelligent algorithmic multi-objective optimization for renewable energy system generation and integration problems: A review. Int J Renew Energy Res 9: 271–280. |
[11] |
Arriag M, Cañizares CA, Kazerani M (2016) Long-term renewable energy planning model for remote communities. IEEE Trans Sust Energy 7: 221–231. doi: 10.1109/TSTE.2015.2483489
![]() |
[12] |
Wong S, Bhattacharya K, Fuller JD (2009) Electric power distribution system design and planning in a deregulated environment. IET Gener Transm. Distrib 3: 1061–1078. doi: 10.1049/iet-gtd.2008.0553
![]() |
[13] | Abapour S, Khosroshahi AE, Frakho A, et al. (2015) Optimal integration of wind power resources in distribution networks considering demand response programs. 9th International Conference on Electrical and Electronics Engineering 26–28. |
[14] | Sambaiah KS, Jayabarathi T (2019) Optimal allocation of renewable distributed generation and capacitor banks in distribution systems using Salp Swarm algorithm. Int J Renew Energy Res 9: 96–107. |
[15] | Singh R, Bansal RC, Singh AR, et al. (2019) Multi-objective optimization of hybrid renewable energy system using reformed electric system cascade analysis for islanding and grid connected modes of operation. IEEE Access 6: 1–26. |
[16] | Nurunnab M, Roy NK, Pota HR (2019) Optimal sizing of grid-tied hybrid renewable energy systems considering inverter to PV ratio - A case study. J Renew Sust Energy 11: 1–14. |
[17] | Su H, Zhang J, Liang Z, et al. (2010) Power distribution network planning optimization based on life cycle cost. China International Conference on Electricity Distribution 1–8. |
[18] | Liu L, Cheng H, Ma Z, et al. (2010) Life cycle cost estimate of power system planning. International Conference on Power System Technology 1–8. |
[19] | Yi-Song JR, Long-Li JQ (2012) Comparative study of 10kV distribution network structure based on Life Cycle Cost analysis. China International Conference on Electricity Distribution 1–5. |
[20] |
Ristimäki M, Säynäjoki A, Heinonen J, et al. (2013) Combining life cycle costing and life cycle assessment for an analysis of a new residential district energy system design. Energy 63: 168–179. doi: 10.1016/j.energy.2013.10.030
![]() |
[21] | Zhang T, Song X, Meng X, et al. (2013) A probabilistic load model base on chi-square method for distribution network. 2nd IET Renewable Power Generation Conference 1–4. |
[22] | Liu Z, Wen F, Ledwich G (2010) Optimal siting and sizing of distributed generators in distribution systems considering uncertainties. IEEE Trans Power Delivery 26: 2541–2551. |
[23] |
Montoya-Bueno S, Muñoz JI, Contreras J (2015) A stochastic investment model for renewable generation in distribution systems. IEEE Trans Sust Energy 6: 1466–1474. doi: 10.1109/TSTE.2015.2444438
![]() |
[24] | Sadeghi M, Kalantar M (2014) Probabilistic analysis of wind turbine planning in distribution systems. 19th Conference on Electrical Power Distribution Networks 1–6. |
[25] |
Shaaban MF, El-Saadany EF (2014) Accommodating high penetrations of PEVs and renewable DG considering uncertainties in distribution systems. IEEE Trans Power Syst 29: 259–270. doi: 10.1109/TPWRS.2013.2278847
![]() |
[26] | Soroudi A, Mohammadi-Ivatloo B, Rabiee A (2014) Energy hub management with intermittent wind power, In: Large Scale Renewable Power Generation, Green Energy and Technology, Springer, 413–438. |
[27] |
Fan M, Vittal V, Heydt GT, et al. (2012) Probabilistic power flow studies for transmission systems with photovoltaic generation using cumulants. IEEE Trans Power Syst 27: 2251–2261. doi: 10.1109/TPWRS.2012.2190533
![]() |
[28] |
Atwa YM, El-Saadany EF (2011) Probabilistic approach for optimal allocation of wind based distributed generation in distribution systems. IET Renew Power Gener. 5: 79–88. doi: 10.1049/iet-rpg.2009.0011
![]() |
[29] | Atwa YM, El-Saadany EF, Salama MMA, et al. (2010) Optimal Renewable Resources Mix for Distribution System Energy Loss Minimization. IEEE Trans Power Syst 25: 360–370. |
[30] | Yu Y, Wen X, Zhao J, et al. (2018) Co-Planning of Demand response and distributed generators in an active distribution network. Energies 11: 2–18. |
[31] |
Santos SF, Fitiwi DZ, Bizuayehu AW, et al. (2017) Impacts of operational variability and uncertainty on distributed generation investment planning: A comprehensive sensitivity analysis. IEEE Trans Sust Energy 8: 855–869. doi: 10.1109/TSTE.2016.2624506
![]() |
[32] | Gkaidatzis PA, Bouhouras AS, Sgouras KI, et al. (2019) Efficient RES penetration under optimal distributed generation placement approach. Energies 12: 1–32. |
[33] |
Zheng Y, Jenkins BM, Kornbluth K, et al. (2018) Optimization under uncertainty of a biomass-integrated renewable energy microgrid with energy storage. Renew Energ 123: 204–217. doi: 10.1016/j.renene.2018.01.120
![]() |
[34] | Konneh DA, Howlader HOR, Shigenobu R, et al. (2019) A multi-criteria decision maker for grid-connected hybrid renewable energy systems selection using multi-objective particle swarm optimization. Sustainability 11: 1–36. |
[35] |
Hosseini S, Sarder MD (2019) Development of a Bayesian network model for optimal site selection of electric vehicle charging station. Electr Power Energy Syst 105: 110–122. doi: 10.1016/j.ijepes.2018.08.011
![]() |
[36] | Longo M, Foiadelli F, Yaïci W (2019) Simulation and optimisation study of the integration of distributed generation and electric vehicles in smart residential district. Int J Energy Environ Eng 8: 1–15. |
[37] |
Pazouki S, Haghifamb MR, Moser A (2014) Uncertainty modeling in optimal operation of energy hub in presence of wind, storage and demand response. Electr Power Energy Syst 61: 335–345. doi: 10.1016/j.ijepes.2014.03.038
![]() |
[38] |
Chen ZP, Yan Z (2018) Scenario tree reduction methods through clustering nodes. Comput Chem Eng 109: 96–111. doi: 10.1016/j.compchemeng.2017.10.017
![]() |
[39] | Shi Z, Wang Z, Jin Y, et al. (2018) Optimal allocation of intermittent distributed generation under active management. Energies 11: 2–19. |
[40] | Rosenthal RE (2008) A user's guide. GAMS Dev Corp 1–262. |
[41] | Abdelkader B, Linda S, Tarek B (2014) Analysis of radial distribution system load flow under uncertainties with fuzzy arithmetic algorithm. 3rd International Conference on Information Processing and Electrical Engineering 1–6. |
[42] |
Khodayar ME, Barati M, Shahidehpour M (2012) Integration of high reliability distribution system in microgrid operation. IEEE Trans Smart Grid 3: 1997–2006. doi: 10.1109/TSG.2012.2213348
![]() |
[43] | IRENA 2018, Renewable power generation costs in 2017, In: International Renewable Energy Agency, Abu Dhabi, 1–158. |
[44] |
Muneer W, Bhattacharya K, Cañizares C (2011) Large-scale solar PV investment models, tools and analysis: The Ontario case. IEEE Trans Power Syst 26: 2547–2555. doi: 10.1109/TPWRS.2011.2146796
![]() |
1. | Nooriya A. Mohammed, Ammar Al-Bazi, Management of renewable energy production and distribution planning using agent-based modelling, 2021, 164, 09601481, 509, 10.1016/j.renene.2020.08.159 | |
2. | Abdul Matin Ibrahimi, Mohammad Masih Sediqi, Harun Or Rashid Howlader, Mir Sayed Shah Danish, Shantanu Chakraborty, Tomonobu Senjyu, Generation expansion planning considering renewable energy integration and optimal unit commitment: A case study of Afghanistan, 2019, 7, 2333-8334, 441, 10.3934/energy.2019.4.441 | |
3. | Merlin Sajini M.L., Suja S., Merlin Gilbert Raj S., Impact analysis of time‐varying voltage‐dependent load models on hybrid DG planning in a radial distribution system using analytical approach, 2021, 15, 1752-1416, 153, 10.1049/rpg2.12013 | |
4. | Angelo Raciti, Santi Agatino Rizzo, Giovanni Susinni, Circuit model of LED light bulb suitable for typical voltage THD on LV distribution networks, 2020, 212, 03787788, 109665, 10.1016/j.enbuild.2019.109665 | |
5. | 2020, Bi-Level Distribution Network Planning Integrated with Energy Storage to PV-Connected Network, 978-1-7281-5635-4, 1325, 10.1109/ISIE45063.2020.9152508 | |
6. | Vu Van Thang, Nguyen Hien Trung, 2020, Chapter 61, 978-3-030-37496-9, 534, 10.1007/978-3-030-37497-6_61 | |
7. | V. V. Thang, N. H. Trung, 2021, Chapter 45, 978-3-030-64718-6, 399, 10.1007/978-3-030-64719-3_45 | |
8. | Mahmoud M. Gamil, Mohammed Elsayed Lotfy, Ashraf M. Hemeida, Paras Mandal, Hiroshi Takahashi, Tomonobu Senjyu, Optimal sizing of a residential microgrid in Egypt under deterministic and stochastic conditions with PV/WG/Biomass Energy integration, 2021, 9, 2333-8334, 483, 10.3934/energy.2021024 | |
9. | V. V. Thang, N. H. Trung, Probabilistic optimization of planning and operation of networked microgrids with renewable energy resources considering demand response programs, 2022, 1868-3967, 10.1007/s12667-022-00559-8 | |
10. | Abdolmajid Erfani, Mehdi Tavakolan, Ali Hassandokht Mashhadi, Pouria Mohammadi, Heterogeneous or homogeneous? A modified decision-making approach in renewable energy investment projects, 2021, 9, 2333-8334, 558, 10.3934/energy.2021027 | |
11. | Zahir Barahmand, Marianne S. Eikeland, Techno-Economic and Life Cycle Cost Analysis through the Lens of Uncertainty: A Scoping Review, 2022, 14, 2071-1050, 12191, 10.3390/su141912191 | |
12. | Aprajay Verma, K Shanti Swarup, An analysis of distribution planning under a regulatory regime: An integrated framework, 2023, 4, 2634-1581, 179, 10.1049/enc2.12088 | |
13. | Mohammad Ahmad A. Al-Ja’Afreh, Bilal Amjad, Kirkland Rowe, Geev Mokryani, Jorge L. Angarita Marquez, Optimal planning and forecasting of active distribution networks using a multi-stage deep learning based technique, 2023, 10, 23524847, 686, 10.1016/j.egyr.2023.07.014 | |
14. | Alejandro Valencia-Díaz, Sebastián García H., Ricardo A. Hincapie I., Stochastic optimization for siting and sizing of renewable distributed generation and D-STATCOMs, 2025, 27726711, 101026, 10.1016/j.prime.2025.101026 |
Type of RS | Parameters | Value | Parameters | Value |
PV | Peak power, Pr (kW) | 100 | kU (mV/℃); kI (mA/℃) | 14.4; 1.22 |
UOC; UMPP (V) | 22; 17.32 | ISC; IMPP (A) | 5.3; 4.76 | |
Life time (year) | 25 | θN; θA (℃) | 25; 43 | |
Invested cost (M$/MW) | 1.5 | O & M cost ($/MWh) | 20 | |
WT | Rate power, Pr (kW) | 100 | vr (m/s) | 14 |
vci (m/s) | 4 | vco (m/s) | 24 | |
Invested cost (M$/MW) | 1.8 | O & M cost ($/MWh) | 40 | |
Life time (year) | 25 |
Parameters | Value |
Emission tax of CO2, β | 10 $/ton |
Emission coefficient of traditional sources, ξT | 0.68 kg/kWh |
Emission coefficient of RS, ξRS | 0.02 kg/kWh |
Discount rate, r | 10% |
State | PV | WT | Load | Electrical price | ||||
kRSs | λPVs | kRSs | λWTs | kLs | λLs | kρs | λρs | |
1 | 0 | 0.450 | 0 | 0.199 | 0.30 | 0.001 | 0.30 | 0.012 |
2 | 0.06 | 0.002 | 0.1 | 0.112 | 0.35 | 0.003 | 0.35 | 0.023 |
3 | 0.12 | 0.005 | 0.2 | 0.113 | 0.40 | 0.010 | 0.40 | 0.041 |
4 | 0.18 | 0.012 | 0.3 | 0.106 | 0.45 | 0.028 | 0.45 | 0.065 |
5 | 0.23 | 0.023 | 0.4 | 0.096 | 0.50 | 0.062 | 0.50 | 0.092 |
6 | 0.29 | 0.039 | 0.5 | 0.085 | 0.55 | 0.111 | 0.55 | 0.116 |
7 | 0.35 | 0.057 | 0.6 | 0.072 | 0.60 | 0.159 | 0.60 | 0.131 |
8 | 0.40 | 0.073 | 0.7 | 0.059 | 0.65 | 0.184 | 0.65 | 0.132 |
9 | 0.45 | 0.083 | 0.8 | 0.047 | 0.70 | 0.172 | 0.70 | 0.119 |
10 | 0.50 | 0.084 | 0.9 | 0.036 | 0.75 | 0.130 | 0.75 | 0.096 |
11 | 0.55 | 0.072 | 1.0 | 0.027 | 0.80 | 0.079 | 0.80 | 0.070 |
12 | 0.60 | 0.053 | 1.0 | 0.020 | 0.85 | 0.039 | 0.85 | 0.045 |
13 | 0.65 | 0.031 | 1.0 | 0.014 | 0.90 | 0.015 | 0.90 | 0.026 |
14 | 0.70 | 0.013 | 1.0 | 0.009 | 0.95 | 0.005 | 0.95 | 0.014 |
15 | 0.75 | 0.003 | 1.0 | 0.006 | 1.00 | 0.001 | 1.00 | 0.006 |
Type of RS | Siting (bus) | Rated power (MW) | Planning year |
PV | 7 | 2.3 | 1 |
8 | 2.3 | 1 | |
9 | 2.4 | 1 | |
WT | 7 | 2.7 | 6 |
8 | 2.6 | 6 | |
9 | 2.8 | 6 |
Case | Total life cycle cost, M$ | Emission taxes cost, M$ | CO2 emission, ton |
Without RS | 82.92 | 5.34 | 746,708.0 |
With RS | 76.58 | 4.22 | 588,446.6 |
Comparison of two cases | 6.34 | 1.12 | 158,261.4 |
Bus i-j | SFij.max(MVA) | Lij (km) | Rij (Ω) | Xij (Ω) |
1-2 | 6.67 | 5.3 | 4.10 | 2.27 |
1-3 | 6.67 | 9.2 | 7.11 | 3.95 |
1-4 | 6.67 | 10.3 | 7.96 | 4.42 |
1-5 | 6.67 | 10.5 | 8.12 | 4.50 |
2-6 | 6.67 | 11.7 | 9.04 | 5.02 |
3-7 | 6.67 | 18.2 | 14.07 | 7.81 |
4-8 | 6.67 | 17.2 | 13.30 | 7.38 |
5-9 | 6.67 | 15.2 | 11.75 | 6.52 |
Bus | PLi(MW) | QLi(MVAr) | Bus | PLi(MW) | QLi(MVAr) |
1 | - | - | 6 | 1.58 | 1.37 |
2 | 2.83 | 2.29 | 7 | 1.31 | 1.14 |
3 | 2.78 | 2.38 | 8 | 1.16 | 0.98 |
4 | 2.95 | 1.99 | 9 | 1.22 | 1.05 |
5 | 2.86 | 1.90 | Total | 16.69 | 13.10 |
Type of RS | Parameters | Value | Parameters | Value |
PV | Peak power, Pr (kW) | 100 | kU (mV/℃); kI (mA/℃) | 14.4; 1.22 |
UOC; UMPP (V) | 22; 17.32 | ISC; IMPP (A) | 5.3; 4.76 | |
Life time (year) | 25 | θN; θA (℃) | 25; 43 | |
Invested cost (M$/MW) | 1.5 | O & M cost ($/MWh) | 20 | |
WT | Rate power, Pr (kW) | 100 | vr (m/s) | 14 |
vci (m/s) | 4 | vco (m/s) | 24 | |
Invested cost (M$/MW) | 1.8 | O & M cost ($/MWh) | 40 | |
Life time (year) | 25 |
Parameters | Value |
Emission tax of CO2, β | 10 $/ton |
Emission coefficient of traditional sources, ξT | 0.68 kg/kWh |
Emission coefficient of RS, ξRS | 0.02 kg/kWh |
Discount rate, r | 10% |
State | PV | WT | Load | Electrical price | ||||
kRSs | λPVs | kRSs | λWTs | kLs | λLs | kρs | λρs | |
1 | 0 | 0.450 | 0 | 0.199 | 0.30 | 0.001 | 0.30 | 0.012 |
2 | 0.06 | 0.002 | 0.1 | 0.112 | 0.35 | 0.003 | 0.35 | 0.023 |
3 | 0.12 | 0.005 | 0.2 | 0.113 | 0.40 | 0.010 | 0.40 | 0.041 |
4 | 0.18 | 0.012 | 0.3 | 0.106 | 0.45 | 0.028 | 0.45 | 0.065 |
5 | 0.23 | 0.023 | 0.4 | 0.096 | 0.50 | 0.062 | 0.50 | 0.092 |
6 | 0.29 | 0.039 | 0.5 | 0.085 | 0.55 | 0.111 | 0.55 | 0.116 |
7 | 0.35 | 0.057 | 0.6 | 0.072 | 0.60 | 0.159 | 0.60 | 0.131 |
8 | 0.40 | 0.073 | 0.7 | 0.059 | 0.65 | 0.184 | 0.65 | 0.132 |
9 | 0.45 | 0.083 | 0.8 | 0.047 | 0.70 | 0.172 | 0.70 | 0.119 |
10 | 0.50 | 0.084 | 0.9 | 0.036 | 0.75 | 0.130 | 0.75 | 0.096 |
11 | 0.55 | 0.072 | 1.0 | 0.027 | 0.80 | 0.079 | 0.80 | 0.070 |
12 | 0.60 | 0.053 | 1.0 | 0.020 | 0.85 | 0.039 | 0.85 | 0.045 |
13 | 0.65 | 0.031 | 1.0 | 0.014 | 0.90 | 0.015 | 0.90 | 0.026 |
14 | 0.70 | 0.013 | 1.0 | 0.009 | 0.95 | 0.005 | 0.95 | 0.014 |
15 | 0.75 | 0.003 | 1.0 | 0.006 | 1.00 | 0.001 | 1.00 | 0.006 |
Type of RS | Siting (bus) | Rated power (MW) | Planning year |
PV | 7 | 2.3 | 1 |
8 | 2.3 | 1 | |
9 | 2.4 | 1 | |
WT | 7 | 2.7 | 6 |
8 | 2.6 | 6 | |
9 | 2.8 | 6 |
Case | Total life cycle cost, M$ | Emission taxes cost, M$ | CO2 emission, ton |
Without RS | 82.92 | 5.34 | 746,708.0 |
With RS | 76.58 | 4.22 | 588,446.6 |
Comparison of two cases | 6.34 | 1.12 | 158,261.4 |
Bus i-j | SFij.max(MVA) | Lij (km) | Rij (Ω) | Xij (Ω) |
1-2 | 6.67 | 5.3 | 4.10 | 2.27 |
1-3 | 6.67 | 9.2 | 7.11 | 3.95 |
1-4 | 6.67 | 10.3 | 7.96 | 4.42 |
1-5 | 6.67 | 10.5 | 8.12 | 4.50 |
2-6 | 6.67 | 11.7 | 9.04 | 5.02 |
3-7 | 6.67 | 18.2 | 14.07 | 7.81 |
4-8 | 6.67 | 17.2 | 13.30 | 7.38 |
5-9 | 6.67 | 15.2 | 11.75 | 6.52 |
Bus | PLi(MW) | QLi(MVAr) | Bus | PLi(MW) | QLi(MVAr) |
1 | - | - | 6 | 1.58 | 1.37 |
2 | 2.83 | 2.29 | 7 | 1.31 | 1.14 |
3 | 2.78 | 2.38 | 8 | 1.16 | 0.98 |
4 | 2.95 | 1.99 | 9 | 1.22 | 1.05 |
5 | 2.86 | 1.90 | Total | 16.69 | 13.10 |