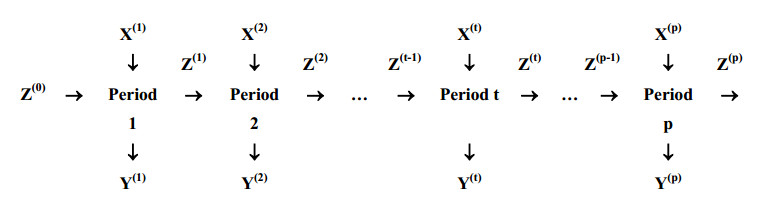
Citation: Yonca Erdem Demirtaş, Neslihan Fidan Keçeci. The efficiency of private pension companies using dynamic data envelopment analysis[J]. Quantitative Finance and Economics, 2020, 4(2): 204-219. doi: 10.3934/QFE.2020009
[1] | Rita Shakouri, Maziar Salahi, Sohrab Kordrostami . Stochastic p-robust approach to two-stage network DEA model. Quantitative Finance and Economics, 2019, 3(2): 315-346. doi: 10.3934/QFE.2019.2.315 |
[2] | Fisnik Morina, Simon Grima . The impact of pension fund assets on economic growth in transition countries, emerging economies, and developed countries. Quantitative Finance and Economics, 2022, 6(3): 459-504. doi: 10.3934/QFE.2022020 |
[3] | Miquel Carreras-Simó, Germà Coenders . The relationship between asset and capital structure: a compositional approach with panel vector autoregressive models. Quantitative Finance and Economics, 2021, 5(4): 571-590. doi: 10.3934/QFE.2021025 |
[4] | Salvatore Scognamiglio . Longevity risk analysis: applications to the Italian regional data. Quantitative Finance and Economics, 2022, 6(1): 138-157. doi: 10.3934/QFE.2022006 |
[5] | Tolga Tuzcuoğlu . The impact of financial fragility on firm performance: an analysis of BIST companies. Quantitative Finance and Economics, 2020, 4(2): 310-342. doi: 10.3934/QFE.2020015 |
[6] | Yonghong Zhong, Junhao Zhong . The spread of debt risk from real estate companies to banks: Evidence from China. Quantitative Finance and Economics, 2023, 7(3): 371-390. doi: 10.3934/QFE.2023018 |
[7] | Ana Sousa, Ana Braga, Jorge Cunha . Impact of macroeconomic indicators on bankruptcy prediction models: Case of the Portuguese construction sector. Quantitative Finance and Economics, 2022, 6(3): 405-432. doi: 10.3934/QFE.2022018 |
[8] | Jinyu Chen, Junqi Liu, Meng He . The impact of stock liquidity on corporate environmental information disclosure: Does climate risk matter?. Quantitative Finance and Economics, 2024, 8(4): 678-704. doi: 10.3934/QFE.2024026 |
[9] | Elżbieta Rychłowska-Musiał . Real options bargaining games. Quantitative Finance and Economics, 2019, 3(4): 624-644. doi: 10.3934/QFE.2019.4.624 |
[10] | Dimitra Loukia Kolia, Simeon Papadopoulos . The levels of bank capital, risk and efficiency in the Eurozone and the U.S. in the aftermath of the financial crisis. Quantitative Finance and Economics, 2020, 4(1): 66-90. doi: 10.3934/QFE.2020004 |
An investor wants to enlarge his capital and earnings with several investment tools. Pension funds are one of the most used investment tools for individual investors. PPCs manage the funds in a range of financial assets according to the individual’s contribution margin. Each individual can intervene in the portfolio selection or choose the portfolios that are already generated by the fund company.
The return or loss of funds varies depending on the securities in the portfolio. Well-managed portfolios allow investors to gain profit, on the other hand, low return funding causes the loss of money. As a matter of course, an investor prefers effectively managed portfolios. In this study, the performances of fund companies are handled just because of fund management importance. PPS contributes not only to the individual’s saving but also to the nations’ economy according to the size of the investment. PPCs provide both quantitative and qualitative benefits to financial markets. It can be considered that pension fund companies contribute to the development of financial markets, which accelerates economic growth in the market phase (Davis, 2005). Therefore, PPS has an important place in developed and developing countries' economies and is preferred due to being easily accessible by individuals. Also, it makes them have a foresight of their retirement term. Besides creating a second retirement opportunity for participants, PPS causes dynamism in the country's economy in terms of transferring resources to long-term investments. With PPS, which is crucial to the creation of savings consciousness, long-term funds have increased and thus the effects of fluctuations in financial markets can be softened. Moreover, PPS strengthens the relationship between savings and investment. From this point of view, it is important for PPCs, to carry out its activities effectively (Karakaya et al., 2014).
DEA is a nonparametric technique to measure the relative efficiencies of firms or DMUs that use the same kind of inputs to produce the same kind of outputs in a specific time period. DEA does not require any data on the input and output prices and it has been widely applied to not only non-profit organizations but also private sector firms. Thus, DEA is frequently used to measure the relative efficiency of profit based financial instruments such as banks, insurance companies, and PPCs. After the essential work of Charnes et al. (1978), several studies have been published including the theory and application of DEA methodology in the literature (Emrouznejad and Yang, 2018).
Most of the studies related to DEA are said to be static that measure the performance of DMUs for a specific time period by using cross-sectional data. Some other studies are interested in efficiency that changes over time, in these studies, the agammaegate efficiency, window analysis or the Malmquist index are used to measure the efficiency scores of DMUs. The agammaegate efficiency is the convex combination of the efficiency scores of the separate terms. The relative efficiency scores of DMUs are calculated for every time period separately via window DEA. However, the aforementioned three kinds of techniques do not incorporate quasi-fixed variables (or carry-overs) that span across several consecutive terms, these methods neglect the inter-relations between time periods. Thus, the importance of Dynamic DEA becomes evident.
We measure the relative efficiency scores of PPCs by using Dynamic DEA. Inputs of the model are considered as the number of workers, total assets; and the outputs of the model are the number of contracts, total contribution and the market share of each PPC. To link the consecutive periods for a time interval Shareholders’ Equity is used as a quasi-fixed input data. This study has an important role just because of being the first Dynamic DEA application to PPCs in between other dynamic studies.
The rest of the paper is organized as follows; in Section 2 the relevant literature on the finance applications of DEA are represented. The Dynamic DEA model, which was derived by Fare and Grosskopf (1997) and formulated by Kao (2013) is clearly introduced in Section 3. Section 4 represents the data and the inputs and outputs in the Dynamic DEA model used in the implementation of the study. Then in the next section, efficiency scores of 16 Turkish PPCs during the 2014–2016 years are given in the tabular form. Efficiency scores calculated from the dynamic model and the conventional DEA model are compared. Finally, some conclusions are presented based on future studies.
In the literature, different multi criteria decision making techniques are recently used to measure the performance DMUs in the finance sector. Gurol and Imam (2018) used TOPSIS to measure the performance of the private pension sector in Turkey between 2006 and 2016. In their study commonly used financial indicators such as shareholder’s equity, number of contracts, total assets are included in the performance measurement. Bulbul and Baykal (2017) used VIKOR, which is one of the most used multi criteria decision making techniques, to analyze the performance of the insurance companies’ in Turkey.
In order to examine long-term productions, businesses, companies or, etc. the overall performance of DMUs can be defined by considering the relationships between successive periods. Additionally, not only the inter-relations of time periods but also the inter-relations of the processes for a DMU can be examined. The general structure is introduced in Fare, Grosskopf, and Whittaker (2007) called as Network DEA. Multi-process organizations can be given as examples of network structure. This kind of system can have sub-processes, and the component processes may interact with each other. Some outputs of one process can be used as inputs by another process. The performance of the component processes is analyzed easily by Network DEA. Network systems have various types of processes, such that; serial, parallel, mixed, hierarchical or dynamic. Which DEA methodology will be selected is basically related to the type of the production system or DMUs that are under consideration. Due to the differences in the structure of the models, network DEA does not have a standard form. Dynamic DEA model is a special type of network model; it includes the serial connection of periods that have the same production structure.
The first introductory study of the dynamic properties of production systems with multi-outputs was proposed by Fare and Grosskopf (1997). Therefore, this study is important to become a basis for many later studies on Dynamic DEA in the literature. Fare and Grosskopf (1997) proposed several kinds of formulations that represent the intertemporal relations of inputs, outputs and/or quasi-fixed inputs according to network theory. Nemoto and Goto (2003) extended the DEA to a dynamic form so that the behavior of the production system can be modeled with the efficient production frontier. Based on the work of Nemoto and Goto (1999), they show how the efficiencies of quasi-fixed inputs and their adjustment processes are evaluated. Kao and Hwang (2010) emphasize the importance of measuring the efficiency of DMUs in complex system form, such as including sub-processes. Because the conventional DEA models can not be able to explain the performance of component processes, the authors propose a relational Network DEA approach that handles the interactions between component processes when calculating the system efficiency. The model is applied to investigate the effect of information technology on firm performance in the banking industry (Kao and Hwang, 2010). Tunca and Deliktas (2015) applied the dynamic model that is proposed in Nemoto and Goto (2003) to measure agricultural efficiency levels of OECD countries over the 1966–2007 period. Nourani et al. (2019) point out that the efficiency scores can be improved with the Dynamic DEA model. In their literature review, Mariz et al. (2018) compare to the areas and the rank positions of DEA applications. They show that after energy and environment, manufacturing and agriculture areas, banking and insurance are the most published areas. Despite recently developed DEA models have been used in the finance industries there are insufficient studies in the literature on Dynamic DEA approaches to examine the efficiency of insurance firms (Kaffash et al., 2019).
Some application studies have measured the effectiveness of insurance companies from Turkey with traditional DEA (Gokgoz, 2010; Ruzgar and Akkaya, 2009; Altan 2010; Karakaya et al, 2014; Dalkilic and Ada, 2014; Ali, 2016; Erdemir and Tatlidil, 2017). There are also implementation studies where the effectiveness of banks and financial companies are measured by DEA, as similar field to insurance companies (Grigorian and Manole, 2006; Luo et al., 2012; Kaffash and Marra, 2017; Kolia and Papadopoulos, 2020). Specifically, Repkova (2013) applied to Dynamic DEA to measure the efficiency of banks. Kaffach et al. (2019) survey the studies with DEA application in the insurance area. The first review study of dynamic models was conducted by Fallah-Fini, Triantis and Johnson (2014). Then, Mariz et al. (2018), also review the studies focusing on Dynamic DEA applications considering the distinction of industry. Therefore, they have also reviewed the studies conducted in the field of banking and finance (Mariz et al., 2018). In their review, Mariz et al. (2018) mentioned the studies with Dynamic DEA on life insurance.
In some Data Envelopment models “dynamism” represents the changes in productivity in terms of a capacity variable due to technological progress or regress. In Sengupta (1999) time-varying capital input due to the changes in input prices over time is analyzed. The model can be used when unit prices of inputs and outputs are available but changes over time due to capacity-based inputs. The author proposed to compute an efficient level of various inputs and thus the optimal cost for any DMU by using the given input prices or their expected changes over-time. This structure is different from the network topology but important work in terms of points of attention.
Figure 1 represents the general form of a dynamic system of a DMU that is under consideration for a time interval. More detailed information on Network DEA can be found in Kao (2014).
The selection of inputs and outputs is particularly difficult for financial firms and important for the validity of the analysis. In the manufacturing sector, firms use physical resources to produce physical products as a transformation of resources. The idea under the DEA methodology is to identify the optimal inputs that enable the quantities of decision units to be desired outputs. Due to the outputs are mostly intangible for financial firms, the issue is here to determine what they produce. Their outputs consist primarily of services (Cummins et al., 2004). When trying to find a criterion to select a particular variable is an input or an output, generally, all other things being kept constant, it can be considered whether an increase in the quantity is desirable or undesirable. But more criteria are needed for the selection of inputs and outputs to evaluate financial firm performances. The first of these is the availability of the data. Second, other analyzes in the literature are a way of defining an alternative criterion that ensures the validity of the analysis. The final criterion for the selection of measurements is the professional views of the individual’s concerned (Brockett et al., 2005). Traditional literature has used the so-called “production approach” for analysis on the efficiency of financial firms (Ferrier and Lovell, 1990; Berger and Humphrey, 1997; Cummins et al., 1999 and Cummins et al., 2004). Pension fund managers use capital equipment and also employ labor and marketing services (Barrientos and Boussofiane 2005). Though criticized by the uncertainty that would result in efficiency enhancement of the respective company, the production approach is preferable. Also, pension fund companies can be viewed as open systems that use inputs (resources) to obtain outputs (converted resources) and distribute these outputs to shareholders (Ali, 2016).
Given the little guidance of the literature on the selection of the inputs of outputs in the model, Barros et al. (2005) and Garcia (2010) rely on microeconomics with Varian (1987). There are the number of the studies on DEA implementing on PPCs (Barrientos and Boussofiane, 2005; Barros and Garcia, 2006; Garcia, 2010; Eling, 2017 and Anandarao et al., 2019). According to the survey of DEA applications in the insurance industry by Kaffash et al. (2019), they conclude that mostly labor and capital variables were used as inputs. On the other hand, mostly premiums, personal lines, and losses variables are used outputs.
In Altan (2010), inputs and outputs are determined by taking into consideration the production approach and the structure and financial ratios of Turkish companies. All items in the balance sheet may be an input and output.
The basic idea under the dynamic model is a decision in a time period impacts later time periods. For instance, if a company makes stocks of any products, then the stocked products may be used in production in future periods. Moreover, in the financial business, a portion of profit can be added to capital, and it is an example of using one period’s outputs as an input in the next time periods (Fare et al., 2007).
To measure the relative efficiencies of DMUs that use m inputs to produce s outputs during the length of p time periods, total quantities of all periods are generally used in the traditional DEA model that is proposed by Charnes et al. (1978). Therefore, the model is referred to author’s initials CCR (Charnes, Cooper and Rhodes) and based on the assumption of constant returns to scale (CRS), which occurs the relative change in production should be equal to the proportionate change in the factor variables. In Banker, Charnes and Cooper (1984), they have present BCC (with author’s initials) model based on the assumption of variable returns to scale (VRS). Accordingly, CCR and BCC models are also called as CRS (constant return to scale) and VRS (variable returns to scale) models, in some other papers related to DEA literature, respectively. BCC model differs from the CCR model only adding a constraint of the summation of the weights of equal to 1 (the convexity constraint). The calculated efficient frontier is a line without the VRS constraint. Thus, theoretically, the CCR-efficiency (or CRS score) does not exceed the BCC-efficiency (or VRS score) for the corresponding DMUs. There is a simple rule that if the DMUs are homogenous and it is fair to compare them with the same number of input usage and output produce the CCR model can be used. (Seiford and Zhu, 1999).
There is an aim of the DEA model (input minimization or output maximization orientation) that is based on the market conditions of the DMU. Generally, DMUs are expected to be output-oriented in competitive markets. Because a DMU aims to maximize the output, which is dependent to demand, by keeping its inputs under control (Barros and Garcia, 2006; Wanke and Barros, 2016). In this context, we prefer to use the output-oriented CCR DEA model in our analysis and make the following explanations on it accordingly.
Let Xtij and Ytrj denote the quantities of the i−th input and r−th output of DMU j in period t, respectively. The summation of these quantities such as Xij=∑pt=1Xtij and Yrj=∑pt=1Ytrjp∑t=1 are used in the following output-oriented DEA model (1) under the CRS assumption by considering all p periods as a whole static system for measuring the relative efficiency of DMU k, Ek :
1/Ek=maxΘ |
subject to
n∑j=1λjXij≤Xik,i=1,…,mn∑j=1λjYrj≥ΘYrk,r=1,…,sλj≥0,j=1,…,nΘunrestrictedinsign. | (1) |
The production process can be classified in three ways, single period, and multi periods without interaction between periods, and multi-periods with the interaction between periods. If the problem is checking efficiencies of DMUs for a single period production process, then the Static DEA model can be enough. Additionally, for multi-period production systems without input-output dependency between periods, the static model can be run for each period separately, or the Malmquist Index can be used. In the multi-period production systems with input-output dependence, the relationship between periods emerges with capital stock, lagged output and capital output according to Emrouznejad and Thanassoulis (2005). Therefore, activities carried out between two periods and transferred from one period to another should be considered while analyzing the effectiveness of the production process.
In dynamic systems, two consecutive periods are connected with flows. Flows represent the intermediate products that are outputs of period t−1 and also inputs of period t. Intermediate products are called “quasi-fixed inputs”. The role of quasi-fixed inputs is found in two ways; they are in the today’s production system as “inputs” and also yesterday’s “outputs”, which connects the serial periods (Nemoto and Goto, 1999). There is a repetition of single-period structure in the dynamic system since the inputs, outputs and quasi-fixed inputs are the same kinds of for every period. Instead of measuring the efficiency score for a single-period, the overall efficiency is measured in the multi-period systems. Inter-relations between the consecutive periods can be described by a Dynamic DEA model and relative efficiency of a set of multi-period DMUs can be measured.
This study considers a dynamic system includes a sequence of periods that are linked by quasi-fixed inputs represented by Ztij The basic idea under quasi-fixed inputs is a portion of the output produced in the preceding period is stocked to use in the next period as input. Sometimes an intermediate product can be used to link the periods, whether all or a portion of the intermediate product is used for production in the next period the structure of the mathematical model is the same. Several examples of this structure exist in the literature. There is various type of formulations proposed for Dynamic DEA in the literature, these models can also be discussed from a theoretical point of view. Also, the structure of the production system plays a basic role to determine and select the dynamic model which is used.
Therefore, in this study, the model that is derived from the idea of network theory of Fare and Grosskopf (1997) is handled. The mathematical model used in their study is formulated in Kao (2013). The technical efficiency EFGk, is measured with the following output-oriented Dynamic DEA model (2). Because it is thought that the inefficient PPC can be efficient through the increase of its outputs, while the inputs remain unchanged.
1/Ek=maxΘ |
subject to
n∑j=1λtjXtij≤Xtik,i=1,…,m;t=1,…,pn∑j=1λtjYtrj≥ΘYtrk,r=1,…,s;t=1,…,pn∑j=1λtjZt−1fj≤Zt−1fk,f=1,…,g;t=1,…,pn∑j=1λtjZtfj≥Ztfk,f=1,…,g;t=1,…,p−1 | (2) |
n∑j=1λpjZpfj≥ΘZpfk,f=1,…,gλtj≥0,j=1,…,n;t=1,…,pΘunrestrictedinsign. |
In model (2), the consecutive periods are linked via quasi-fixed inputs (Zt). The output of the previous period is used as input for the next period in every successive period. For every period t; Zt-1 variable act as input and Zt act as output. The constraints on the quasi-fixed variables are added with the same logic of the model (1). The output-oriented model is used and for the final period the quasi-fixed variable is considered as final output, then the last constraint is written with Θ.
The legal framework of the Turkish PPS was completed in 2002. In Turkey, voluntary basis individual PPS came into operation in 2003 with the involvement of six pension companies. The system is supervised and surveillance by the Undersecretariat of Treasury under the Prime Ministry of Turkey, Capital Markets Board and Pension Monitoring Center. PPS plays a complementary role in the Social Security System. By this system, it is aimed to make individuals saving during their active working period to have extra income in the retirement term, and also contribute to the nation’s economy with their investments. By January 1, 2013, Government Contribution is started in Turkey then PPS became more advantageous for participants. The government contribution is the amount paid by the state to the participant’s pension account at 25% of the contributions paid by the participant. On January 1, 2017, with the beginning of the automatic enrolment of the public and private employees to the PPS, PPCs that have more than 1000 workers were included. After the April 2017, public employees under the age of 45 and companies with 250 to 1000 employees were included in the PPS. The automatic enrolment will be finalized gradually by the year 2019 (Pension Monitoring Center).
According to the annual report of Undersecretariat of Treasury of Turkey; by the end of 2016, a total of 61 insurances, reinsurance, and pension companies are operating in Turkey. They are 37 of which are non-life, 18 are life and retirement, 4 are life and 2 are reinsurance companies. As of 31/12/2016, the number of participants in the system approached 6.6 million people and the number of contracts concluded approached 7.8 million people. These numbers show that in 2016 the number of participants increased by 9.7% and the number of contracts increased by 10.7%. At the end of 2016, a total contribution of 51.6 billion TL has been paid for the private pension contracts that have been implemented since the beginning of 2013 (7.0 billion TL), and the value of the participant funds has reached TL 60.8 billion TL. The amounts disclosed do not include the amounts of the funds repaid to the persons referred to by the contributions paid by the participants leaving the system. In 2016, the contributions contributed and the contributions contributed including the state contribution increased by 27% and 18%, respectively (Republic of Turkey Prime Ministry Undersecretariat of Treasury).
The comparative efficiency analysis of these PPCs relative to each other is the key point of this work. Using the end-year data for 2013–2016, the relative efficiency scores of companies in the PPS, which is an important point in today’s markets, were examined by Dynamic DEA. In this section, the data is introduced and then the selection of the variables are explained.
Data is taken from the website of the Undersecretariat of Treasury (www.hazine.gov.tr) as an MS Excel Sheet form. Under secretariat of Treasury generally publish an annual report of the previous year in July of next year and for our analysis. We consider the values from the annual reports and take into account between the 2013–2016 years when automatic enrolment has not yet begun. Therefore, the effect of automatic enrolment on the efficiency of PPC is out of the scope of this study.
Due to the quasi-fixed inputs, the data includes the indicators published during the 2013–2016 years. Accordingly, efficiency scores of PPCs that are in operation continuously during the time period 2014–2016 are measured in this study. There are 19 PPCs with data published in this sector during the 2014–2016 years. BANK ASIA has not been taken into consideration since it did not operate after 2016. Moreover, if a company buys another company without changing its own name, disproportionately changes in the financial indicators of the receiver company affect the relative efficiency of that company. Since FIBA A.Ş. bought the ERGO A.Ş. in September 2016, both of the receiver company and the received company are ignored in the study accordingly. Thus, only 16 efficiency scores of Turkish PPCs are investigated with Dynamic DEA in this application.
In the dynamic model, it is necessary to use a variable that links the periods, called as quasi-fixed inputs. While using the quasi-fixed inputs, the output of time t−1 is used as input of time t. Since NN-Hayat A.Ş. was not in operation in 2013, the Zt−1j value for 2014 (i.e. Z2013i) is not available. In order to prevent missing one-year from the data, we prefer which is usual to use, the same value with Zt−1j and Ztj for 2013 on that company (Kao, 2013).
According to the application studies with DEA, we have used balance sheet items as inputs and outputs in our study. Despite the different assumptions about measuring efficiency, there is often a reasonable agreement to identify important inputs and outputs for financial institutions. Consistent with traditional efficiency literature and considering the availability of the data, we define the number of workers and total assets as inputs for PPCs. The number of contracts, total contribution and market share of each PPC are used as outputs. We also see from literature; deposits are specified having input characteristics just because of the interest payments. However, because of providing a substantial amount of liquidity, safekeeping, and payment services to depositors, the deposit can be considered as output. In the same manner, Shareholders’ equity represents the difference between the assets and the liabilities of the firm. In principle, it is defined as the amount of money that would have shareholders after the firm discharged its obligations. Retained earnings is part of shareholder equity and can be considered as savings for future use (Ross et al., 2005). Thanks to its definition, the shareholders’ equity is used to link consecutive periods in the time interval, it is called as quasi-fixed input for the dynamic model.
Figure 2 visualizes the concept of the dynamic structure of the PPCs with their variables.
Table 1 represents the descriptive statistics of used input, output and quasi-fixed variables of the model. The units of the variables are explained under the Table 1.
Mean | Standard Dev. | Min. | Max. | ||
Inputs | Number of Workers | 516.90 | 406.35 | 56 | 1617 |
Total Assets | 3831676.98 | 3900716.68 | 66971.95 | 14391682.20 | |
Outputs | Number of Contracts | 415130.77 | 384873.81 | 20292.00 | 1290319.00 |
Total Contribution | 888764.92 | 908988.15 | 21154.56 | 3231199.12 | |
Market Share | 0.80 | 0.57 | 0.01 | 2.68 | |
Quasi-fixed input | Shareholders’ Equity | 272119.01 | 272343.00 | 8774.96 | 1313412.90 |
Following the general choice of the variables as input and output in DEA; correlations among the inputs or the outputs affect the efficiency scores of DMUs. Low correlations among the inputs (or outputs) variables are suitable. Additionally, the correlation between the input and the output is essential. The decision of the DEA attributes is considered with this scope in addition to the literature survey.
If the number of DMUs is less than the total number of input and output a large portion of DMUs will be identified as efficient. In the envelopment model, since its orientation to relative efficiency; the number of degrees of freedom will increase with the number of DMUs and decrease with the number of input and output. Cooper, Seiford and Tone (2007) suggest that the number of DMUs has to be equal or greater than max {m∗s,3∗(m+s)}, being m and s the number of input and output criteria, respectively. In this study, we have 2 inputs, 3 outputs, and 1 quasi-fixed input. The number of PPCs is equal to 16, so the number of DMUs rule given in Cooper et al. (2007) is satisfied.
In this part of the paper, we represent the effects of the quasi-fixed input are obtained in the same way for both of the approaches, overall period (Dynamic and Static DEA models) or separately for each year (Static with or without quasi-fixed input). In Figure 3, the comparison of the efficiency scores that are obtained from the Dynamic and Static DEA models are given. We see that the efficiency scores differ according to consider quasi-fixed input.
In Table 2 comparison of the efficiency scores that are obtained from the Dynamic and Static DEA models is given. The mathematical model (1) which is a conventional DEA model is used to calculate the relative static efficiency scores of the PPCs. In this model total values of the variables are used as input and output and the quasi-fixed input is ignored because of the structure of the static model. Since the effects of the inter-relations of the consecutive periods are a new idea of the dynamic model, when doing a comparison of these two models it shouldn’t be added to the static model. Then the overall period is considered both two models have labeled seven companies are efficient at the same time, they are AEGON E/H, ALLIANZ H/E, ANADOLU H/E, CIGNA FINANS E/H, HALK H/E, KATILIM E/H, and ZIRAAT H/E. The dynamic model has found 11 companies as efficient among 16 companies, the static model has found only 8 companies are efficient. When we recalculate with the dynamic model, efficiency scores of 9 out of 16 companies are changed.
Dynamic Model | Static Model | |
AEGON E/H | 1.00 | 1.00 |
ALLIANZ H/E | 1.00 | 1.00 |
ALLIANZ Y/E | 0.99 | 0.96 |
ANADOLU H/E | 1.00 | 1.00 |
AVIVASA E/H | 0.89 | 1.00 |
AXA H/E | 1.00 | 0.47 |
BNP PARIBAS CARDIF EMEKLILIK | 0.78 | 0.74 |
CIGNA FINANS E/H | 1.00 | 1.00 |
GARANTI E/H | 1.00 | 0.82 |
GROUPAMA EMEKLILIK | 0.84 | 0.57 |
HALK H/E | 1.00 | 1.00 |
KATILIM E/H | 1.00 | 1.00 |
METLIFE E/H | 1.00 | 0.67 |
NN H/E | 1.00 | 0.75 |
VAKIF EMEKLILIK | 0.81 | 0.97 |
ZIRAAT H/E | 1.00 | 1.00 |
Specifically, ALLIANZ Y/E’s efficiency score in this model is equal to 0.99. Therefore, 68.75% of the companies are efficient regarding Dynamic DEA and 50% for the conventional model. According to the results, the dynamic model has given greater scores than the conventional one for seven companies (ALLIANZ Y/E, AXA H/E, BNP PARIBAS CARDIF E, GARANTI H/E, GROUPAMA E, METLIFE E/H and NN H/E) whereas only two of the companies (AVIVASA E/H and VAKIF E) have the smaller score in the dynamic model according to the conventional one. The lowest efficiency scores of inefficient companies are 0.78 for the dynamic model and 0.47 for the static model. Moreover, especially for AXA H/E, the efficiency score of the company dramatically change (from 0.47 to 1) and the company has reached efficient status via dynamic analysis. Similarly, METLIFE E/H is an efficient company with Dynamic DEA whereas it is inefficient with 0.67 score via static model. These results show that the efficiency score can be improved by considering the effects of the inter-relations of the consecutive periods. Obviously, these differences in the results by two approaches also have the effect of the Shareholders’ equity variable chosen as quasi-fixed input data.
Furthermore, to know the role of the quasi-fixed input data on the decision of labeling efficient in the used models, we compare the static DEA is modeled with and without quasi-fixed input for each year separately. In terms of the structure of the conventional DEA model, the quasi-fixed input is added to the model as follows; the output of the previous year is used as input for the investigated year in the time period. Figure 4a and Figure 4b represent quasi-fixed input effect on the static model which is run for each year 2014, 2015 and 2016, separately.
The efficiency scores of the companies are given in Table 3 for each year in the time period 2014–2016 with the assumption of CRS. According to Table 3, the results are consistent with the results given in Table 2 for the overall period.
Static with quasi-fixed input | Static without quasi-fixed input | ||||||
2014 | 2015 | 2016 | 2014 | 2015 | 2016 | ||
AEGON E/H | 1.00 | 1.00 | 1.00 | 1.00 | 1.00 | 1.00 | |
ALLIANZ H/E | 1.00 | 1.00 | 1.00 | 1.00 | 1.00 | 1.00 | |
ALLIANZ Y/E | 0.99 | 0.84 | 0.76 | 0.98 | 0.70 | 0.63 | |
ANADOLU H/E | 1.00 | 0.96 | 0.77 | 1.00 | 1.00 | 0.63 | |
AVIVASA E/H | 0.89 | 0.77 | 0.77 | 1.00 | 1.00 | 0.98 | |
AXA H/E | 1.00 | 1.00 | 0.92 | 0.46 | 0.45 | 0.46 | |
BNP PARIBAS CARDIF EMEKLILIK | 0.87 | 0.84 | 0.77 | 0.72 | 0.61 | 0.89 | |
CIGNA FINANS E/H | 1.00 | 1.00 | 1.00 | 1.00 | 1.00 | 1.00 | |
GARANTI E/H | 1.00 | 1.00 | 0.92 | 1.00 | 1.00 | 0.66 | |
GROUPAMA EMEKLILIK | 0.89 | 0.99 | 0.79 | 0.83 | 0.60 | 0.44 | |
HALK H/E | 1.00 | 1.00 | 1.00 | 1.00 | 0.86 | 0.79 | |
KATILIM E/H | 1.00 | 1.00 | 1.00 | 1.00 | 1.00 | 1.00 | |
METLIFE E/H | 1.00 | 1.00 | 1.00 | 0.64 | 0.69 | 0.70 | |
NN H/E | 1.00 | 1.00 | 0.62 | 1.00 | 0.63 | 0.59 | |
VAKIF EMEKLILIK | 0.82 | 0.67 | 0.79 | 0.93 | 0.96 | 0.90 | |
ZIRAAT H/E | 1.00 | 1.00 | 1.00 | 1.00 | 1.00 | 1.00 |
The effects of the quasi-fixed input data are obtained in the same way for both of the approaches, overall period or separately for each year. Therefore, to measure efficiency decision maker may take into consideration both or one of the approaches depending on the situation. We do not prefer to say that Dynamic DEA is better than the traditional one. Instead, we can use the dynamic approach when we think that there are variables that should be taken into account when measuring the efficiency and that we should consider the interaction between time periods.
When measuring the relative efficiency of a set of DMU in a period of time the traditional DEA methodology assumes the whole system static. If the time period is divided into sub-periods and the interrelations between the sub-periods can be established, then the system is called dynamic. In this study, the Dynamic DEA model is used to investigate to relative efficiencies of PPCs in Turkey, between 2014 and 2016 years.
This study focuses on the static and dynamic analysis which can be preferred one to each other based on the situation, rather than the relations between the analysis results. In this study, the Shareholders Equity is used as quasi-fixed variable. Our results clearly demonstrate that the efficiency score can be improved by considering the effects of the inter-relations of the consecutive periods. The differences in the results by two approaches have the effect of the Shareholders’ equity data chosen as quasi-fixed input. When the analyses in this area are surveyed, it is seen that the results obtained vary according to the type of companies, working time, country and method.
According to the literature search, this analysis has an important role as being the first application of Dynamic DEA on PPCs. Thanks to the dynamic feature of the analysis, the study can be viewed as an example of usage Panel Data. Even though the analysis based on a specific period of time and the data, the implications arising from the results of the study are important for the companies' policies for the next time periods. More applications can be done with other financial indicators to show interrelations between the periods. Moreover, the dynamic version of DEA is a relatively new tool and it can be applied and decision-making units to be investigated.
All authors declare no conflicts of interest in this paper.
[1] | Ali AS (2016) Efficiency of Private Pension Companies in Turkey Using Data Envelopment Analysis (DEA), In: Chaos, Complexity and Leadership 2014, Springer, 495-505. |
[2] | Altan MS (2010) Türk Sigortacilik Sektöründe Etkinlik: Veri Zarflama Analizi Yöntemi ile Bir Uygulama. Gazi Üniversitesi Iktisadi ve Idari Bilimler Fakültesi Dergisi 12: 185-204. |
[3] |
Anandarao S, Durai SRS, Goyari P (2019) Efficiency decomposition in two-stage data envelopment analysis: an application to life insurance companies in India. J Quant Econ 17: 271-285. doi: 10.1007/s40953-018-0148-1
![]() |
[4] |
Banker RD, Charnes A, Cooper WW (1984) Some models for estimating technical and scale inefficiencies in data envelopment analysis. Manage Sci 30: 1078-1092. doi: 10.1287/mnsc.30.9.1078
![]() |
[5] | Barrientos A, Boussofiane A (2005) How efficient are pension fund managers in Chile. Revista de Economia Contemporânea 9: 289-311. |
[6] |
Barros CP, Barroso N, Borges MR (2005) Evaluating the efficiency and productivity of insurance companies with a Malmquist index: A case study for Portugal. Geneva Pap Risk Insurance-Issues Pract 30: 244-267. doi: 10.1057/palgrave.gpp.2510029
![]() |
[7] |
Barros CP, Garcia MTM (2006) Performance evaluation of pension funds management companies with data envelopment analysis. Risk Manage Insur Rev 9: 165-188. doi: 10.1111/j.1540-6296.2006.00092.x
![]() |
[8] |
Berger AN, Humphrey DB (1997) Efficiency of financial institutions: International survey and directions for future research. Eur J Oper Res 98: 175-212. doi: 10.1016/S0377-2217(96)00342-6
![]() |
[9] |
Brockett PL, Cooper WW, Golden LL, et al. (2005) Financial intermediary versus production approach to efficiency of marketing distribution systems and organizational structure of insurance companies. J Risk Insur 72: 393-412. doi: 10.1111/j.1539-6975.2005.00130.x
![]() |
[10] | Bulbul SE, Baykal KB (2017) Hayat dışı branşlarda faaliyet gösteren Türk sigorta şirketlerinin finansal performans analizi: Vikoryönetimi. 3rd National Insurance and Actuarial Congress, Karabük, 1-9. |
[11] |
Charnes A, Cooper WW, Rhodes E (1978) Measuring the efficiency of decision making units. Eur J Oper Res 2: 429-444. doi: 10.1016/0377-2217(78)90138-8
![]() |
[12] | Cooper W, Seiford LM, Tone K (2007) A Comprehensive Text with Models, Applications, References and DEA-Solver Software. Data Envelopment Anal. |
[13] |
Cummins JD, Weiss MA, Zi H (1999) Organizational form and efficiency: The coexistence of stock and mutual property-liability insurers. Manage Sci 45: 1254-1269. doi: 10.1287/mnsc.45.9.1254
![]() |
[14] |
Cummins JD, Rubio-Misas M, Zi H (2004) The effect of organizational structure on efficiency: Evidence from the Spanish insurance industry. J Bank Financ 28: 3113-3150. doi: 10.1016/j.jbankfin.2004.05.004
![]() |
[15] | Davis EP (2005) The role of pension funds as institutional investors in emerging markets. Brunel University Research Archive. Economics and Finance Discussion Papers, Brunel University, 05-18, London. |
[16] |
Eling M, Schaper P (2017) Under pressure: how the business environment affects productivity and efficiency of European life insurance companies. Eur J Oper Res 258: 1082-1094. doi: 10.1016/j.ejor.2016.08.070
![]() |
[17] |
Emrouznejad A, Thanassoulis E (2005) A mathematical model for dynamic efficiency using data envelopment analysis. Appl Math Comput 160: 363-378. doi: 10.1016/j.amc.2003.09.026
![]() |
[18] |
Emrouznejad A, Yang GL (2018) A survey and analysis of the first 40 years of scholarly literature in DEA: 1978-2016. Socio-Econ Plann Sci 61: 4-8. doi: 10.1016/j.seps.2017.01.008
![]() |
[19] | Erdemir OK, Tatlidil H (2017) Veri Zarflama Analizinde Veri İndirgeme: Sigorta Şirketlerinin Etkinliği Üzerine Bir Araştırma. J Current Res Bus Econ 7: 65-78. |
[20] |
Fallah-Fini S, Triantis K, Johnson AL (2014) Reviewing the literature on non-parametric dynamic efficiency measurement: state-of-the-art. J Prod Anal 41: 51-67. doi: 10.1007/s11123-013-0349-8
![]() |
[21] |
Fare R, Grosskopf S (1997) Intertemporal production frontiers: with Dynamic DEA. J Oper Res Society 48: 656-656. doi: 10.1057/palgrave.jors.2600780
![]() |
[22] | Fare R, Grosskopf S, Whittaker G (2007) Network DEA, In: Modeling data irregularities and structural complexities in data envelopment analysis, Springer, 209-240. |
[23] |
Ferrier GD, Lovell CK (1990) Measuring cost efficiency in banking: Econometric and linear programming evidence. J Econometrics 46: 229-245. doi: 10.1016/0304-4076(90)90057-Z
![]() |
[24] |
Garcia MTM (2010) Efficiency evaluation of the Portuguese pension funds management companies. J Int Financ Markets Inst Money 20: 259-266. doi: 10.1016/j.intfin.2010.03.003
![]() |
[25] |
Grigorian DA, Manole V (2006) Determinants of commercial bank performance in transition: an application of data envelopment analysis. Comp Econ Stud 48: 497-522. doi: 10.1057/palgrave.ces.8100129
![]() |
[26] |
Gokgoz F (2010) Measuring the financial efficiencies and performances of Turkish funds. Acta Oeconomica 60: 295-320. doi: 10.1556/AOecon.60.2010.3.4
![]() |
[27] | Gurol B, Imam M (2018) Measuring The Performance Of Private Pension Sector By Topsis Multi Criteria Decision-Making Method. J Econ Financ Accounting 5: 288-295. |
[28] |
Kaffash S, Marra M (2017) Data envelopment analysis in financial services: a citations network analysis of banks, insurance companies and money market funds. Annals Oper Res 253: 307-344. doi: 10.1007/s10479-016-2294-1
![]() |
[29] | Kaffash S, Azizi R, Huang Y, et al. (2019) A survey of data envelopment analysis applications in the insurance industry 1993-2018. Eur J Oper Res. |
[30] |
Kao C (2013) Dynamic data envelopment analysis: A relational analysis. Eur J Oper Res 227: 325-330. doi: 10.1016/j.ejor.2012.12.012
![]() |
[31] |
Kao C (2014) Network data envelopment analysis: A review. Eur J Oper Res 239: 1-16. doi: 10.1016/j.ejor.2014.02.039
![]() |
[32] |
Kao C, Hwang SN (2010) Efficiency measurement for network systems: IT impact on firm performance. Decis Support Syst 48: 437-446. doi: 10.1016/j.dss.2009.06.002
![]() |
[33] | Karakaya A, Kurtaran A, Dagli H (2014) Bireysel emeklilik sirketlerinin veri zarflama analizi ile etkinlik ölçümü: Türkiye örnegi. Yönetim ve Ekonomi Arastırmaları Dergisi 12: 1-23. |
[34] |
Kolia DL, Papadopoulos S (2020) The levels of bank capital, risk and efficiency in the Eurozone and the US in the aftermath of the financial crisis. Quant Financ Econ 4: 66-90. doi: 10.3934/QFE.2020004
![]() |
[35] |
Luo Y, Bi G, Liang L (2012) Input/output indicator selection for DEA efficiency evaluation: An empirical study of Chinese commercial banks. Expert Syst Appl 39: 1118-1123. doi: 10.1016/j.eswa.2011.07.111
![]() |
[36] |
Mariz FB, Almeida MR, Aloise D (2018) A review of Dynamic Data Envelopment Analysis: state of the art and applications. Int Tran Oper Res 25: 469-505. doi: 10.1111/itor.12468
![]() |
[37] |
Nemoto J, Goto M (1999) Dynamic data envelopment analysis: modeling intertemporal behavior of a firm in the presence of productive inefficiencies. Econ Lett 64: 51-56. doi: 10.1016/S0165-1765(99)00070-1
![]() |
[38] |
Nemoto J, Goto M (2003) Measurement of dynamic efficiency in production: an application of data envelopment analysis to Japanese electric utilities. J Prod Anal 19: 191-210. doi: 10.1023/A:1022805500570
![]() |
[39] |
Nourani M, Ting IWK, Lu WM, et al. (2019) Capital Structure And Dynamic Performance: Evidence From Asean-5 Banks. Singapore Econ Rev 64: 495-516. doi: 10.1142/S021759081650034X
![]() |
[40] | Pension Monitoring Center. Available from: https://www.egm.org.tr/data-center/statistics/ips-statistics/summary-ips-data/. |
[41] | Republic of Turkey Prime Ministry Undersecretariat of Treasury. Available from: https://www.treasury.gov.tr/insurance-reports. |
[42] | Ross SA, Westerfield R, Jaffe JF (2005) Corporate Finance, International Edition. McGraw-Hill/Irwin. |
[43] | Ruzgar B, Akkaya A (2009) Examining efficiencies of private pension companies in Turkey with data envelopment analysis, In IWW2009-proceeding of the IVth international workshop on applications of wavelets to real world problems, At Kocaeli, Turkey. |
[44] |
Seiford LM, Zhu J (1999) An investigation of returns to scale in data envelopment analysis. Omega 27: 1-11. doi: 10.1016/S0305-0483(98)00025-5
![]() |
[45] |
Sengupta JK (1999) A dynamic efficiency model using data envelopment analysis. Int J Prod Econ 62: 209-218. doi: 10.1016/S0925-5273(98)00244-8
![]() |
[46] | Tunca H, Deliktas E (2015) OECD Ülkelerinde Tarımsal Etkinlik Ölçümü: Dinamik Veri Zarflama Analizi. Ege Acad Rev 15. |
[47] | Ulucan A (2002) İSO 500 Şirketlerinin Etkinliklerinin Ölçülmesinde Veri Zarflama Analizi Yaklaşımı: Farklı Girdi Çıktı Bileşenleri ve Ölçeğe Göre Getiri Yaklaşımları ile Değerlendirmeler. Ankara üniversitesi SBF dergisi 57: 185-202. |
[48] | Varian HR (1987) Intermediate economics: A modern approach, W. W. Norton & Company, NewYork. |
[49] |
Wanke P, Barros CP (2016) Efficiency drivers in Brazilian insurance: A two-stage DEA meta frontier-data mining approach. Econ Model 53: 8-22. doi: 10.1016/j.econmod.2015.11.005
![]() |
1. | Yue Liu, Pierre Failler, Liming Chen, Can Mandatory Disclosure Policies Promote Corporate Environmental Responsibility?—Quasi-Natural Experimental Research on China, 2021, 18, 1660-4601, 6033, 10.3390/ijerph18116033 | |
2. | Yongrong Xin, Xiyin Chang, Jianing Zhu, How does the digital economy affect energy efficiency? Empirical research on Chinese cities, 2022, 0958-305X, 0958305X2211434, 10.1177/0958305X221143411 | |
3. | Shuanglian Chen, Cunyi Yang, Khaldoon Albitar, Is there any heterogeneous impact of mandatory disclosure on corporate social responsibility dimensions? Evidence from a quasi-natural experiment in China, 2021, 1, 2769-2140, 272, 10.3934/DSFE.2021015 | |
4. | Shuai Wang, Cunyi Yang, Zhenghui Li, Green Total Factor Productivity Growth: Policy-Guided or Market-Driven?, 2022, 19, 1660-4601, 10471, 10.3390/ijerph191710471 | |
5. | Zhenghui Li, Fanqi Zou, Bin Mo, Does mandatory CSR disclosure affect enterprise total factor productivity?, 2022, 35, 1331-677X, 4902, 10.1080/1331677X.2021.2019596 | |
6. | Shuaishuai Jia, Yushan Qiu, Cunyi Yang, Sustainable Development Goals, Financial Inclusion, and Grain Security Efficiency, 2021, 11, 2073-4395, 2542, 10.3390/agronomy11122542 | |
7. | Dimitra Loukia Kolia, Simeon Papadopoulos, Integration in banking efficiency: a comparative analysis of the European Union, the Eurozone, and the United States banks, 2022, 6, 2514-4774, 48, 10.1108/JCMS-08-2021-0026 | |
8. | Zeynep KÜÇÜKKIRALI, Üzeyir AYDIN, TÜRKİYE’DE FAALİYET GÖSTEREN BİREYSEL EMEKLİLİK ŞİRKETLERİNİN ETKİNLİĞİ, 2021, 1013-1388, 10.51551/verimlilik.940655 | |
9. | Fanqi Zou, Tinghui Li, Feite Zhou, Does the Level of Financial Cognition Affect the Income of Rural Households? Based on the Moderating Effect of the Digital Financial Inclusion Index, 2021, 11, 2073-4395, 1813, 10.3390/agronomy11091813 | |
10. | Qingqing Hu, Xue Li, Yanhong Feng, Do Green Credit Affect Green Total Factor Productivity? Empirical Evidence from China, 2022, 9, 2296-598X, 10.3389/fenrg.2021.821242 | |
11. | Wenqiang Fu, Rongwu Zhang, Can Digitalization Levels Affect Agricultural Total Factor Productivity? Evidence From China, 2022, 6, 2571-581X, 10.3389/fsufs.2022.860780 | |
12. | Bruvine Orchidée Mazonga Mfoutou, Richard Danquah, Assessing the Efficiency of Defined Benefit Private and Public Pension Plans using Data Envelopment Analysis Adjusted Estimator, 2022, 2278-5337, 227853372211326, 10.1177/22785337221132617 | |
13. | Shuai Wang, Cunyi Yang, Zhenghui Li, Spatio-Temporal Evolution Characteristics and Spatial Interaction Spillover Effects of New-Urbanization and Green Land Utilization Efficiency, 2021, 10, 2073-445X, 1105, 10.3390/land10101105 | |
14. | Fisnik Morina, Simon Grima, The impact of pension fund assets on economic growth in transition countries, emerging economies, and developed countries, 2022, 6, 2573-0134, 459, 10.3934/QFE.2022020 | |
15. | Qingqing Hu, Xiaobing Wang, Mark Xu, Are there heterogeneous impacts of social support on subjective well-being?, 2021, 3, 2689-3010, 360, 10.3934/NAR.2021019 | |
16. | Qingqing Hu, Yanhong Feng, Mark Xu, Are There Heterogeneous Impacts of Air Pollution on Mental Health?, 2021, 9, 2296-2565, 10.3389/fpubh.2021.780022 | |
17. | Haihua Liu, Peng Wang, Zejun Li, Is There Any Difference in the Impact of Digital Transformation on the Quantity and Efficiency of Enterprise Technological Innovation? Taking China’s Agricultural Listed Companies as an Example, 2021, 13, 2071-1050, 12972, 10.3390/su132312972 | |
18. | Pengzhen Liu, Yanmin Zhao, Jianing Zhu, Cunyi Yang, Technological industry agglomeration, green innovation efficiency, and development quality of city cluster, 2022, 4, 2643-1092, 411, 10.3934/GF.2022020 | |
19. | Güven GÜNEY, Özlem TOPÇUOĞLU, TÜRKİYE’DE DEVLET KATKISI SONRASI BİREYSEL EMEKLİLİK SİSTEMİNİN ETKİNLİĞİ, 2023, 25, 2564-7164, 419, 10.31460/mbdd.1251665 | |
20. | Emmanuel Owusu Oppong, Bruvine Orchidée Mazonga Mfoutou, Assessing the Efficiency and Persistence of African Stock Markets through DEA Score: Evidence from COVID-19 Pandemic, 2023, 0972-2629, 10.1177/09722629231185251 | |
21. | Maryam Badrizadeh, Joseph C. Paradi, Mohammadreza Alirezaee, Improving pension funds’ performance using data envelopment analysis considering government regulations, 2023, 7, 2519898X, 38, 10.22495/cgsrv7i2p4 | |
22. | Nitin Arora, Dilpreet Sharma, Shubhendra Jit Talwar, A sustainable measure of efficiency performance and validity of Environmental Kuznets Curve – an intercountry Data Envelopment Analysis approach, 2025, 2054-6238, 10.1108/JEAS-07-2024-0256 |
Mean | Standard Dev. | Min. | Max. | ||
Inputs | Number of Workers | 516.90 | 406.35 | 56 | 1617 |
Total Assets | 3831676.98 | 3900716.68 | 66971.95 | 14391682.20 | |
Outputs | Number of Contracts | 415130.77 | 384873.81 | 20292.00 | 1290319.00 |
Total Contribution | 888764.92 | 908988.15 | 21154.56 | 3231199.12 | |
Market Share | 0.80 | 0.57 | 0.01 | 2.68 | |
Quasi-fixed input | Shareholders’ Equity | 272119.01 | 272343.00 | 8774.96 | 1313412.90 |
Dynamic Model | Static Model | |
AEGON E/H | 1.00 | 1.00 |
ALLIANZ H/E | 1.00 | 1.00 |
ALLIANZ Y/E | 0.99 | 0.96 |
ANADOLU H/E | 1.00 | 1.00 |
AVIVASA E/H | 0.89 | 1.00 |
AXA H/E | 1.00 | 0.47 |
BNP PARIBAS CARDIF EMEKLILIK | 0.78 | 0.74 |
CIGNA FINANS E/H | 1.00 | 1.00 |
GARANTI E/H | 1.00 | 0.82 |
GROUPAMA EMEKLILIK | 0.84 | 0.57 |
HALK H/E | 1.00 | 1.00 |
KATILIM E/H | 1.00 | 1.00 |
METLIFE E/H | 1.00 | 0.67 |
NN H/E | 1.00 | 0.75 |
VAKIF EMEKLILIK | 0.81 | 0.97 |
ZIRAAT H/E | 1.00 | 1.00 |
Static with quasi-fixed input | Static without quasi-fixed input | ||||||
2014 | 2015 | 2016 | 2014 | 2015 | 2016 | ||
AEGON E/H | 1.00 | 1.00 | 1.00 | 1.00 | 1.00 | 1.00 | |
ALLIANZ H/E | 1.00 | 1.00 | 1.00 | 1.00 | 1.00 | 1.00 | |
ALLIANZ Y/E | 0.99 | 0.84 | 0.76 | 0.98 | 0.70 | 0.63 | |
ANADOLU H/E | 1.00 | 0.96 | 0.77 | 1.00 | 1.00 | 0.63 | |
AVIVASA E/H | 0.89 | 0.77 | 0.77 | 1.00 | 1.00 | 0.98 | |
AXA H/E | 1.00 | 1.00 | 0.92 | 0.46 | 0.45 | 0.46 | |
BNP PARIBAS CARDIF EMEKLILIK | 0.87 | 0.84 | 0.77 | 0.72 | 0.61 | 0.89 | |
CIGNA FINANS E/H | 1.00 | 1.00 | 1.00 | 1.00 | 1.00 | 1.00 | |
GARANTI E/H | 1.00 | 1.00 | 0.92 | 1.00 | 1.00 | 0.66 | |
GROUPAMA EMEKLILIK | 0.89 | 0.99 | 0.79 | 0.83 | 0.60 | 0.44 | |
HALK H/E | 1.00 | 1.00 | 1.00 | 1.00 | 0.86 | 0.79 | |
KATILIM E/H | 1.00 | 1.00 | 1.00 | 1.00 | 1.00 | 1.00 | |
METLIFE E/H | 1.00 | 1.00 | 1.00 | 0.64 | 0.69 | 0.70 | |
NN H/E | 1.00 | 1.00 | 0.62 | 1.00 | 0.63 | 0.59 | |
VAKIF EMEKLILIK | 0.82 | 0.67 | 0.79 | 0.93 | 0.96 | 0.90 | |
ZIRAAT H/E | 1.00 | 1.00 | 1.00 | 1.00 | 1.00 | 1.00 |
Mean | Standard Dev. | Min. | Max. | ||
Inputs | Number of Workers | 516.90 | 406.35 | 56 | 1617 |
Total Assets | 3831676.98 | 3900716.68 | 66971.95 | 14391682.20 | |
Outputs | Number of Contracts | 415130.77 | 384873.81 | 20292.00 | 1290319.00 |
Total Contribution | 888764.92 | 908988.15 | 21154.56 | 3231199.12 | |
Market Share | 0.80 | 0.57 | 0.01 | 2.68 | |
Quasi-fixed input | Shareholders’ Equity | 272119.01 | 272343.00 | 8774.96 | 1313412.90 |
Dynamic Model | Static Model | |
AEGON E/H | 1.00 | 1.00 |
ALLIANZ H/E | 1.00 | 1.00 |
ALLIANZ Y/E | 0.99 | 0.96 |
ANADOLU H/E | 1.00 | 1.00 |
AVIVASA E/H | 0.89 | 1.00 |
AXA H/E | 1.00 | 0.47 |
BNP PARIBAS CARDIF EMEKLILIK | 0.78 | 0.74 |
CIGNA FINANS E/H | 1.00 | 1.00 |
GARANTI E/H | 1.00 | 0.82 |
GROUPAMA EMEKLILIK | 0.84 | 0.57 |
HALK H/E | 1.00 | 1.00 |
KATILIM E/H | 1.00 | 1.00 |
METLIFE E/H | 1.00 | 0.67 |
NN H/E | 1.00 | 0.75 |
VAKIF EMEKLILIK | 0.81 | 0.97 |
ZIRAAT H/E | 1.00 | 1.00 |
Static with quasi-fixed input | Static without quasi-fixed input | ||||||
2014 | 2015 | 2016 | 2014 | 2015 | 2016 | ||
AEGON E/H | 1.00 | 1.00 | 1.00 | 1.00 | 1.00 | 1.00 | |
ALLIANZ H/E | 1.00 | 1.00 | 1.00 | 1.00 | 1.00 | 1.00 | |
ALLIANZ Y/E | 0.99 | 0.84 | 0.76 | 0.98 | 0.70 | 0.63 | |
ANADOLU H/E | 1.00 | 0.96 | 0.77 | 1.00 | 1.00 | 0.63 | |
AVIVASA E/H | 0.89 | 0.77 | 0.77 | 1.00 | 1.00 | 0.98 | |
AXA H/E | 1.00 | 1.00 | 0.92 | 0.46 | 0.45 | 0.46 | |
BNP PARIBAS CARDIF EMEKLILIK | 0.87 | 0.84 | 0.77 | 0.72 | 0.61 | 0.89 | |
CIGNA FINANS E/H | 1.00 | 1.00 | 1.00 | 1.00 | 1.00 | 1.00 | |
GARANTI E/H | 1.00 | 1.00 | 0.92 | 1.00 | 1.00 | 0.66 | |
GROUPAMA EMEKLILIK | 0.89 | 0.99 | 0.79 | 0.83 | 0.60 | 0.44 | |
HALK H/E | 1.00 | 1.00 | 1.00 | 1.00 | 0.86 | 0.79 | |
KATILIM E/H | 1.00 | 1.00 | 1.00 | 1.00 | 1.00 | 1.00 | |
METLIFE E/H | 1.00 | 1.00 | 1.00 | 0.64 | 0.69 | 0.70 | |
NN H/E | 1.00 | 1.00 | 0.62 | 1.00 | 0.63 | 0.59 | |
VAKIF EMEKLILIK | 0.82 | 0.67 | 0.79 | 0.93 | 0.96 | 0.90 | |
ZIRAAT H/E | 1.00 | 1.00 | 1.00 | 1.00 | 1.00 | 1.00 |