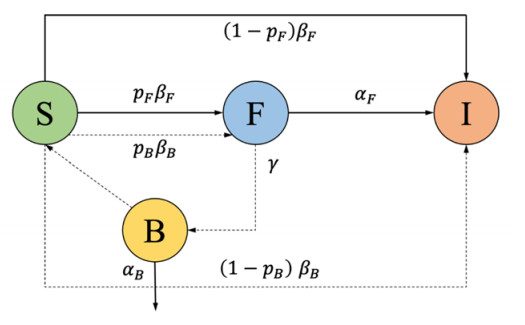
Citation: Luciana Porto de Souza Vandenberghe, Lina Marcela Blandon Garcia, Cristine Rodrigues, Marcela Cândido Camara, Gilberto Vinícius de Melo Pereira, Juliana de Oliveira, Carlos Ricardo Soccol. Potential applications of plant probiotic microorganisms in agriculture and forestry[J]. AIMS Microbiology, 2017, 3(3): 629-648. doi: 10.3934/microbiol.2017.3.629
[1] | Sarafa A. Iyaniwura, Musa Rabiu, Jummy F. David, Jude D. Kong . Assessing the impact of adherence to Non-pharmaceutical interventions and indirect transmission on the dynamics of COVID-19: a mathematical modelling study. Mathematical Biosciences and Engineering, 2021, 18(6): 8905-8932. doi: 10.3934/mbe.2021439 |
[2] | Jing Liao, Jinling Wang, Jiarong Li, Xin Jiang . The dynamics and control of a multi-lingual rumor propagation model with non-smooth inhibition mechanism. Mathematical Biosciences and Engineering, 2024, 21(4): 5068-5091. doi: 10.3934/mbe.2024224 |
[3] | Fulian Yin, Xueying Shao, Jianhong Wu . Nearcasting forwarding behaviors and information propagation in Chinese Sina-Microblog. Mathematical Biosciences and Engineering, 2019, 16(5): 5380-5394. doi: 10.3934/mbe.2019268 |
[4] | Yi Wang, Shicheng Zhong, Guo Wang . Dynamic selection of clarification channels in rumor propagation containment. Mathematical Biosciences and Engineering, 2023, 20(8): 14995-15017. doi: 10.3934/mbe.2023672 |
[5] | Fulian Yin, Hongyu Pang, Lingyao Zhu, Peiqi Liu, Xueying Shao, Qingyu Liu, Jianhong Wu . The role of proactive behavior on COVID-19 infordemic in the Chinese Sina-Microblog: a modeling study. Mathematical Biosciences and Engineering, 2021, 18(6): 7389-7401. doi: 10.3934/mbe.2021365 |
[6] | Xiaonan Chen, Suxia Zhang . An SEIR model for information propagation with a hot search effect in complex networks. Mathematical Biosciences and Engineering, 2023, 20(1): 1251-1273. doi: 10.3934/mbe.2023057 |
[7] | Junli Liu . Threshold dynamics of a time-delayed hantavirus infection model in periodic environments. Mathematical Biosciences and Engineering, 2019, 16(5): 4758-4776. doi: 10.3934/mbe.2019239 |
[8] | Qiao Xiang, Tianhong Huang, Qin Zhang, Yufeng Li, Amr Tolba, Isack Bulugu . A novel sentiment analysis method based on multi-scale deep learning. Mathematical Biosciences and Engineering, 2023, 20(5): 8766-8781. doi: 10.3934/mbe.2023385 |
[9] | Curtis L. Wesley, Linda J. S. Allen, Michel Langlais . Models for the spread and persistence of hantavirus infection in rodents with direct and indirect transmission. Mathematical Biosciences and Engineering, 2010, 7(1): 195-211. doi: 10.3934/mbe.2010.7.195 |
[10] | Jun Zhai, Bilin Xu . Research on meme transmission based on individual heterogeneity. Mathematical Biosciences and Engineering, 2021, 18(5): 5176-5193. doi: 10.3934/mbe.2021263 |
The coronavirus disease 2019 (COVID-19) pandemic not only has social effects on the healthcare system, but also public opinion guidance. Under the life-disease dual pressure, people are more likely to be affected by fake news [1]. We need to conduct more in-depth research on information propagation mechanisms to propose more effective intervention strategies.
It is significant to get deep insight into the information ecology on social media platforms. The concept of "information ecology" was first proposed by Davenport [2], who stated that the information environment consists of other people, information technology and information regulations, which remarkably affect the formation of public opinion and sentiment. Nowadays, it is mainly reflected as "topic communities" constructed by Internet technology, such as the "hotspots" on the Chinese Sina microblogging platform and the "trending" topics on Twitter and Facebook. Such algorithm mechanisms realize the comprehensive ordering of information presented to reflect public opinion related to a particular emergency, and they represent a new paradigm for the "pseudo-environment" proposed by Lippman and Curtis [3]. Pieces of information subject to hot topics construct a "pseudo-environment" for netizens to improve their cognition, and for simplicity. Therefore, in the omni-media era, the two approaches to accessing information are divided into direct and indirect, where the former way means that netizens receive information from connected friends, and the latter means that they receive information from hot topics suggestions.
This paper discusses a data-model dual-drive research approach that has been applied to develop intervention strategies in response to public emergencies. First, we regard the "forwarding behavior" as an essential way for netizens to spread information, and the data form to describe its propagation effect is temporal forwarding quantities. Based on the characteristics of this kind of data form, we propose a susceptible-forwarding-immune pseudo-environment (SFI-PE) dynamic model with direct and indirect behaviors. Second, we introduce external interventions into the SFI-PE model to simulate their effects on information propagation, termed the macro-controlled SFI-PE (M-SFI-PE) model. To guide public communication, we implement qualitative and quantitative analysis to help design optimal intervention strategies.
The subsequent materials are organized as follows. In Section 2, we review relevant literature from past years. In Section 3, we establish the SFI-PE model based on the coexistence of direct and indirect propagation modes and subsequently detail the construction of an M-SFI-PE dynamic model that considers external interventions. In Section 4, we present the numerical analysis based on real data from the Chinese Sina microblogging platform to validate our SFI-PE model, as well as parameter sensitivity analysis. Section 5 draws some conclusions and discusses the optional strategy for public opinion guidance.
Since the outbreak of COVID-19, more and more scholars have begun focusing on its social impact and conducting further research, such as research on health analysis and prediction [4], sentiment analysis for social media platforms [5] and public opinion guidance [6,7,8,9,10,11]. Recently, Bibi et al. [5] proposed a model that detected COVID-19-related fake news with an accuracy of 86.12% and outperformed standard machine learning algorithms. Yin et al. [12] analyzed public opinion toward COVID-19 vaccination and proposed several recommendations to remind governments to build confidence in a targeted way.
The epidemiological model is the most widely used method to analyze information propagation qualitatively and quantitatively. In infectious disease epidemiology, pathogen transmission can involve direct and indirect pathways. For the direct pathways, the susceptible-infected (SI) model [13,14], the susceptible-exposed-infected-recovered (SEIR) model [15], the susceptible-infected-recovered (SIR) model [16,17], the susceptible-infected-susceptible (SIS) model [18] and the susceptible-infected-recovered-susceptible (SIRS) model [19] are classical and fundamental in the area of research on infection between populations. Researchers have further studied pathogen transmission through the environment for the indirect pathways [20,21,22,23]. For instance, Cortez et al. [24] proposed an approach with direct versus environmentally mediated indirect transmission pathways, consequently contributing to identifying how differences in the transmission pathways could result in quantitatively different epidemiological dynamics, and how those differences could be used to identify the transmission pathway from population-level time-series data. Vasilyeva et al. [25] studied the relative influence of the different etiological and behavioral aspects on these pathways to support the intervention.
Due to similarities between information propagation and the spread of infectious diseases in populations, Yin et al. [26] inherited and developed the traditional SIR model to propose a susceptible-forwarding-immune (SFI) model for the purpose of studying the dynamics of populations by modeling the propagation process. Similarly, Yu et al. [27] considered two pathways of information propagation, namely, those among friends and marketing accounts, to establish a rumor propagation model. These previous studies paid attention to the dynamics and attributes of people without the information environment. Therefore, our work focuses on the synergistic effect of humans and the environment as based on direct and indirect propagation behaviors to fill in the research gap in the field of information propagation.
To construct the research framework for information propagation, we analyzed the operation mechanisms on typical social media platforms. We found that direct and indirect modes are different but common ways to promote information propagation in the real world. Specifically, the direct mode based on social relations, such as relations with fans, refers to the situation in which users can directly see and forward messages from their friends. And, the indirect mode emphasizes the impact of the environment, such as the trending topics sorted by an algorithmic mechanism, wherein users are required to enter the topic details page to contact relevant messages from strangers. Therefore, we developed a novel SFI-PE dynamic model to consider the environmental impact and constructed an information propagation system with the direct and indirect propagation behaviors, as shown in Figure 1.
Assuming the total number of users (N) remains unchanged in a closed environment, we divided them into three states: the susceptible state S in which users are unaware of the information but have opportunities; the forwarding state F in which users have forwarded the information to influence susceptible users; the immune state I in which users have already forwarded the information but are now inactive, or they are subjectively not interested in the information. We define S(t), F(t), and I(t) as the numbers of people in the respective states at time t and each user is in a unique state. Therefore, S(t)+F(t)+I(t)=N. Specially, we denote B as the environmental impact, and it involves the relevant information in topic communities. Table 1 shows the parameter interpretations of the SFI-PE model.
Parameter | Interpretation |
βF | The average direct exposure rate, i.e., the average rate at which susceptible users can access information through social relations. |
βB | The average indirect exposure rate, i.e., the average rate at which susceptible users can access information through the topic communities. |
pF | The average direct forwarding probability, i.e., the average probability that susceptible users see and forward the information through social relations. |
pB | The average indirect forwarding probability, i.e., the average probability that susceptible users see and forward the information from the topic communities. |
αF | The average immune rate, i.e., the average rate at which forwarding users become inactive, is related to the behavioral law of users. |
αB | The average metabolic rate, i.e., the average rate at which information in topic communities gradually becomes inactive, is related to the algorithmic law of social media platforms. |
γ | The average transfer rate, i.e., the average rate at which the information is filtered and presented in topic communities generated by social media algorithms. |
The form of differential equations related to the SFI-PE model is described as follows:
{dS(t)dt=−βFS(t)F(t)−βBS(t)B(t)dF(t)dt=pFβFS(t)F(t)+pBβBS(t)B(t)−αFF(t)dI(t)dt=(1−pF)βFS(t)F(t)+(1−pB)βBS(t)B(t)+αFF(t)dB(t)dt=γF(t)−αBB(t). | (3.1) |
For the direct information transmission relying on social relations, an active forwarding user will contact an average number of βFN users per unit time, and the probability that the contacted user is susceptible is S(t)/N; so, an active forwarding user will contact βFS(t) susceptible users, among whom pFβFS(t) will choose to forward the information. For the indirect pathway of relying on topic communities, a piece of information will reach βBS(t) susceptible users, among whom pBβBS(t) will choose to forward the information.
Traditionally, βFS(t)F(t) susceptible users are directly affected by forwarding users, among whom pFβFS(t)F(t) will choose to forward the information and transfer from the susceptible state (S) to the forwarding state (F). In our dynamic system, βBS(t)B(t) susceptible users are indirectly affected by information in the topic communities; among them, pBβBS(t)B(t) susceptible users will transfer to the forwarding state (F). However, (1−pF)βFS(t)F(t)+(1−pB)βBS(t)B(t) susceptible users are not interested in the information, so they transfer to the immune state (I). Considering that the information in the topic communities and the users in the forwarding state are no longer to influence others after the active exposure period, there are αBB(t) pieces of information that reach the end of the information life cycle, and αFF(t) forwarding users transfer to the immune state (I). Under the Internet algorithm, γF(t) pieces of information will be screened and presented in topic communities per unit time.
We introduced external interventions into the SFI-PE model to create an M-SFI-PE dynamic model, as shown in Figure 2. This model was developed in consideration of the facts that external interventions take place in every link in the dynamic process and that the research on them is of great importance to guide public opinion. For negative emergencies such as rumors, effective external interventions can reverse the information propagation to reduce social panic and reconcile social contradictions. For positive events and official rumor-refuting information, effective external interventions can promote information propagation to help create a positive social atmosphere and guarantee social order.
We denote M as external interventions. First, external interventions take place in the direct transitions from the susceptible state (S) to the forwarding state (F), and from the susceptible state (S) to the immune state (I). Similarly, external interventions also take place in the indirect transitions wherein the susceptible users (S) transfer to the forwarding state (F) or the immune state (I) after coming into contact with information in the environment (B). Third, external interventions can regulate the information transition, as indicated by the dotted line from the forwarding state (F) to the environment module (B) in Figure 2. However, the average immune rate αF and the average metabolic rate αB are mainly related to the behavior rules and the algorithm settings, which are hard to manipulate using external measures.
Table 2 shows the new parameter interpretations for the M-SFI-PE model. The definitions of parameters βF, βB, pF, pB and γ are shown in Table 1.
Parameter | Interpretation |
βMF | The coefficient for the effects of external interventions on βF, i.e., the effects of external interventions on the direct access to information relying on social relations. |
βMB | The coefficient for the effects of external interventions on βB, i.e., the effects of external interventions on the indirect access to information relying on topic communities. |
pMF | The coefficient for the effects of external interventions on pF, i.e., the effects of external interventions on the direct forwarding probability relying on social relations. |
pMB | The coefficient for the effects of external interventions on pB, i.e., the effects of external interventions on the indirect forwarding probability relying on topic communities. |
γM | The coefficient for the effects of external interventions on γ, i.e., the effects of external interventions on the process of filtering and presenting information in topic communities. |
The form of differential equations related to the M-SFI-PE model is described as follows:
{dS(t)dt=−(βF−βMF)S(t)F(t)−(βB−βMB)S(t)B(t)dF(t)dt=(pF−pMF)(βF−βMF)S(t)F(t)+(pB−pMB)(βB−βMB)S(t)B(t)−αFF(t)dI(t)dt=(1−pF+pMF)(βF−βMF)S(t)F(t)+(1−pB+pMB)(βB−βMB)S(t)B(t)+αFF(t)dB(t)dt=(γ−γM)F(t)−αBB(t). | (3.2) |
Here, external interventions can reverse the information propagation or positively promote it. For the direct mode, βMF, i.e., the coefficient for the effects of external interventions on βF, changes the average direct exposure rate to βF−βMF; pMF, i.e., the coefficient for the effects of external interventions on pF, changes the average direct forwarding probability to pF−pMF. For the indirect one, γM, i.e., the coefficient for the effects of external interventions on γ, changes the average transfer rate to γ−γM; βMB, i.e., the coefficient for the effects of external interventions on βB, changes the average indirect exposure rate to βB−βMB; pMB, i.e., the coefficient for the effects of external interventions on pB, changes the average indirect forwarding probability to pB−pMB.
In the information propagation process, the cumulative forwarding population characterizes the scale of the propagation community. Further deduced from the master equations of the SFI-PE model, the equation of the forwarding cumulant without external interventions can be given by
CSFI−PE(t)=∫t0pFβFS(t)F(t)dt+∫t0pBβBS(t)B(t)dt. | (3.3) |
Similarly, the equation of the forwarding cumulant with external interventions, as deduced from the master equations of the M-SFI-PE model, can be given by
CM−SFI−PE(t)=∫t0(pF−pMF)(βF−βMF)S(t)F(t)dt+∫t0(pB−pMB)(βB−βMB)S(t)B(t)dt. | (3.4) |
With all of this in place, we construct the key indices to develop the evaluation criteria for the intervention methods for public communication. First, the outbreak peak of forwarding Fmax represents the intensity of information propagation, and it refers to the maximum number of current forwarding users F(t). Second, the final forwarding scale Cs represents the breadth of information propagation, and it refers to the maximum number of the cumulative forwarding users CSFI−PE(t), or CM−SFI−PE(t) when information propagation ends. Third, the peak time of forwarding tFmax represents the speed with which public opinion explodes to its zenith, and it refers to the time to reach Fmax. Finally, the public communication reproduction number R0 measures whether public opinion is likely to break out, and it refers to the average number of secondary spreaders caused by each forwarding user when external interventions are excluded and all users are susceptible.
Especially, the public communication reproduction number R0 originates from the epidemiology field to measure whether an infectious disease is likely to break out. Thus, we refer to the calculation of the primary reproduction ratio to obtain its expression [28], and we rewrite it as follows. If the number of forwarding users per unit time decreases, public opinion will not break out. That is, public opinion is on the wane when S(t)=S0. Because F(t)≥B(t)>0, we deduce that
R0=pFβFS0+pBβBS0αF. | (3.5) |
The size of R0 determines the speed of the outbreak without external interventions. When R0<1, the number of forwarding users decreases rapidly; hence, public opinion will never break out. However, when R0>1, the forwarding population grows exponentially and public opinion is bound to explode. That is, for the positive events with R0<1, the external interventions can help to promote the outbreak of public opinion, while, for the negative emergencies with R0>1, the external interventions can help to suppress them.
To analyze the information propagation dynamics, we selected the Chinese Sina microblogging platform, i.e., the most popular social media platform in China, as the typical case for numerical analysis. We screened two specific events related to the regular epidemic prevention and social security measures on the Chinese Sina microblogging platform and collected the real and accurate forwarding time and the corresponding copywriting to implement data fitting. To fit our model with the propagation data, we used the Least Squares method (LS) to estimate the parameters and the initial susceptible population S0. The parameter vector can be set as Θ = (βF,βB,pF,pB,αF,αB,γ,S0), and the corresponding numerical calculation based on the parameter vector for Ck is denoted by fC(k,Θ). Then, we obtain the LS error function
LS=∑Tk=0|fC(k,Θ)−Ck|2, | (4.1) |
where Ck denotes the actual cumulative forwarding quantity corresponding to the sampling point k, where k=0,1,2,…,T; additionally, we set the beginning time to 0 and the sampling interval to 1 hour.
Furthermore, we introduced the mean absolute percentage error (MAPE) [29] to measure the goodness of data fitting and validate the efficiency of our model, which has a benchmark of 0. The principle is that the closer the result of the MAPE is to 0, the better the performance. We adopted the MAPE as an indicator because it has a very intuitive interpretation in terms of relative error; due to its definition, its use is recommended for tasks in which sensitivity to relative variations is more important than sensitivity to absolute variations, which is in line with our purpose. The MAPE is defined as follows:
MAPE=100%mn∑mk=1∑ni=1|ˆyki−ykiyki| | (4.2) |
where m is the number of data groups, n is the number of sampling points in the data group, yki is the ith value of the kth group of empirical data and ˆyki is the predicted value of yki.
According to Figure 3, the fitting curves approximately coincide with the corresponding data points. For the positive event, MAPE=0.0024%, and for the negative event, MAPE=0.0035%, which indicates that our model can perfectly depict the information propagation. By comparing the estimated values, we can form some conclusions. First, γ is small, indicating that only a tiny amount of forwarded information would be screened and presented in topic communities. Second, βF and βB are determined by the density of the propagation network, and βF>βB, which explains that it is easier for users to access information directly on the homepages than indirectly by entering the public pages. Third, pF is smaller than pB, so the susceptible users are more proactive and willing to forward information when coming into contact with the relevant information via topic communities. Finally, αF, which is related to the behavior rules, is larger than αB, indicating that the exposure period in topic communities is longer.
We further conducted numerical experiments to compare the data fitting performance of the traditional SFI model and our SFI-PE model. In detail, we set the values of parameters with the same definition in these two models to be the same. According to Figure 4, the fitting results for the SFI model largely deviate from the real points. By calculation, the MAPE for the positive event was found to equal 0.1085%, and that for the negative event was found to equal 0.4845%. Obviously, the performance of our model is better than that of the traditional one.
Table 3 shows the values of indices. For the positive and negative events, the public communication reproduction number R0>1; so, the forwarding populations grew exponentially in our case studies, indicating that public opinion is bound to break out. Moreover, the outbreak peak of forwarding for the negative event Fmax=3938.7000 was found to be larger than that for the positive event Fmax=822.6215, indicating that the information intensity for the negative event is larger than that for the positive one. In addition, the time to reach the outbreak peak of forwarding for the positive event tFmax=42 was found to be earlier than that for the negative event tFmax=71. Finally, we calculated the final forwarding scale for the negative event Cs=23,188, which was found to be larger than that for the positive event Cs=3853.3000. This explains that the negative event has a broader propagation scale than the positive one.
Fmax | Cs | tFmax | R0 | |
For the positive event | 822.6215 | 3853.3000 | 42 | 4.1351 |
For the negative event | 3938.7000 | 23,188 | 71 | 6.9910 |
In order to analyze how the parameters (βB, βF, pB, pF, γ, αB, αF, S0) affect information propagation and thus help to optimize design intervention strategies, we applied partial rank correlation coefficients (PRCCs) [30] and a single-parameter change method for further study. We took the positive event as an example to analyze its effects on the outbreak peak of forwarding Fmax, the final forwarding scale Cs and the public communication reproduction number R0. The experimental results of the relevant sensitivity analyses are shown in Figure 5.
The results indicate that, regarding the intensity of information propagation, increasing pF, S0, βF and pB while decreasing αF facilitates its improvement. The opposite conditions would cause it to decrease. Regarding the breadth of information propagation, increasing pF, S0 and pB while decreasing αB and αF helps to extend it. Otherwise, it would shrink. If public opinion erupts, increasing pF, S0, βB, βF and pB while decreasing αF will further promote it or restrain the explosion of public opinion.
Specifically, the average direct exposure rate βF, average direct exposure rate βB, average direct forwarding probability pF and average indirect forwarding probability pB are essential parameters affecting information propagation. Thus, we conducted further research on their respective impacts, as shown in Figure 6.
We compared the results in Figure 6 and drew some conclusions. First, the parameters related to the environment (βB,pB) were found to have a minimal effect on the outbreak peak of forwarding Fmax and a moderate effect on the final forwarding scale Cs. The parameters related to social relations (βF,pF) were found to have a more significant effect on the outbreak peak of forwarding Fmax. Especially, the parameter βF mainly affects the forwarding scale in the early stage and has an inverse impact on the final forwarding scale Cs, while the significant influence of pF on the forwarding scale is the whole process. Therefore, we can formulate intervention strategies according to the results of parameter sensitivity analysis.
We took the above cases as the base scenario and assumed the new parameters of the M-SFI-PE model appropriately. Below, we compare five new scenarios with the base one to simulate and investigate the influence of different intervention measures on our dynamic system.
According to the numerical results for the SFI-PE model, we set the default values as the control group. New parameter values for the M-SFI-PE model were set as one-tenth of the default parameters. The negative sign reflects the promotion effect of external interventions on information propagation. For instance, we set βMF=−9.4000×10−5 as Experimental Group (a), βMB=−4.1900×10−5 as Experimental Group (b), pMF=−0.0461 as Experimental Group (c), pMB=−0.0850 as Experimental Group (d) and γM=−0.0100 as Experimental Group (e). We used Matlab to conduct simulation analysis for the M-SFI-PE model; the results are shown in Figure 6.
It can be seen in Figure 7(a), (b) that, in the transition from the susceptible state (S) to the forwarding state (F), only Experimental Group (c) was able to accelerate the decline of S(t). Experimental Groups (a) and (c) were able to increase the maximum value of F(t) and reach the outbreak peak of information propagation faster. The other intervention methods were found to have relatively weak effects. As for Figure 7(b), (c), in the transition from the forwarding state (F) to the immune state (I), the number of current immune users I(t) increased simultaneously under the influence of pMF. Nevertheless, the final scale of immune users did not significantly expand, so the proportion of influential spreaders can be expected to increase correspondingly. As for Figure 7(d), all five of the intervention methods were found to contribute to increasing B(t); among them, adjusting pMF and γM was found to have a better effect.
Table 4 shows the values of the post-intervention indices for the positive event.
Fmax | Cs | tFmax | |
Control Group | 822.6215 | 3853.3000 | 42 |
Experimental Group (a) | 934.6235 | 3822.2000 | 37 |
Experimental Group (b) | 830.9647 | 3886.1000 | 41 |
Experimental Group (c) | 1027.2000 | 4175.7000 | 37 |
Experimental Group (d) | 833.0052 | 3920.3000 | 41 |
Experimental Group (e) | 830.9647 | 3886.1000 | 41 |
We can draw the following conclusions by comparing the results in Table 4. First, Experimental Group (c) had the best intervention effect on Fmax in terms of increasing the intensity of information propagation. The order of intervention methods is pMF>βMF>pMB>βMB≈γM. Second, in terms of expanding the breadth of information propagation, Experimental Group (c) had the best intervention effect on Cs when the parameter pMF was adjusted. The order of intervention methods is pMF>pMB>βMB≈γM. Third, in terms of accelerating the peak of information propagation by decreasing the value of tFmax, the order of intervention methods is pMF≈βMF>βMB≈pMB≈γM.
Similarly, we set βMF=1.3400×10−5 as Experimental Group (a), βMB=1.200×10−5 as Experimental Group (b), pMF=0.0220 as Experimental Group (c), pMB=0.0729 as Experimental Group (d) and γM=0.0104 as Experimental Group (e). The positive sign reflects the suppression effect on information propagation. Figure 8 shows the simulation results.
It can be seen in Figure 8(a), (b) that, in the transition from the susceptible state (S) to the forwarding state (F), Experimental Groups (a) and (c) were able to decelerate the decline of S(t), decrease the maximum value of F(t) and postpone the time to reach the outbreak peak of information propagation. The significance of intervention effects is pMF>βMF. As for Figure 8(b), (c), in the transition from the forwarding state (F) to the immune state (I), I(t) decreased simultaneously under the influence of pMF and βMF. Nevertheless, the final scale of immune users did not significantly shrink, so the proportion of influential spreaders can be expected to decrease correspondingly. As for Figure 8(d), all five of the intervention methods were able to decrease B(t). Among them, γM adjustment was found to have the best effect, followed by pMF.
Table 5 shows the values of the post-intervention indices for the negative event.
Fmax | Cs | tFmax | |
Control Group | 3938.7000 | 23,188 | 71 |
Experimental Group (a) | 3628.2000 | 24,135 | 80 |
Experimental Group (b) | 3773.4000 | 22,778 | 72 |
Experimental Group (c) | 3292.4000 | 22,149 | 80 |
Experimental Group (d) | 3747.0000 | 22,397 | 72 |
Experimental Group (e) | 3773.4000 | 22,778 | 72 |
We can draw the following conclusions by comparing the results in Table 5. First, Experimental Group (c) had the best intervention effect on Fmax in terms of decreasing the intensity of information propagation. The order of intervention methods is pMF>βMF>pMB>βMB≈γM. Second, in terms of narrowing the breadth of information propagation, Experimental Group (c) had the best intervention effect on Cs when the parameter pMF was adjusted. The order of intervention methods is pMF>pMB>βMB≈γM. Third, in terms of decelerating to the peak of public communication by increasing the value of tFmax, the order of intervention methods is pMF≈βMF>βMB≈pMB≈γM.
In the era of the post-COVID-19 waves, guiding public opinion has become the most urgent and long-term important issue due to the contradiction between epidemic prevention and production recovery. Our SFI-PE dynamic model introduces environmental factors into the traditional SFI model to explore the law of information propagation with the direct and indirect propagation behaviors on social platforms. The M-SFI-PE model was developed as an extension of the SFI-PE model to further analyze the effects of external interventions on information dissemination and help to design optimal intervention strategies. We validated our models by selecting two quintessential public health emergency messages and numerically fitting the results to the actual propagation data. The results of further simulations indicate that adjusting pMF, the coefficient for the effects of external interventions on pF, is the best intervention approach for public communication, and that pF is the most crucial parameter among them.
The practical method based on our experimental results for promoting information propagation is to improve the forwarding willingness of susceptible users by increasing the average direct forwarding probability pF. Media, for example, many platforms of which have photos and documentary descriptions that can show the specific implementation scenario of social security measures, can thus enhance the content of emotional resonance and sense of communication. Moreover, we can simultaneously adjust the average direct exposure rate βF, average indirect exposure rate βB, average indirect forwarding probability pB and average transfer rate γ to integrate their influences on public communication. For instance, in the early stage, the media can cooperate with opinion leaders with many followers and a larger γ to obtain a larger βF and accelerate the expansion of the public communication scale. Later, the public concerns can be extracted to form a general entry that is put at the top of the hot search list to make it more eye-catching, thus increasing βB and pB. Regarding rumor suppression, to decrease pF, pop-up windows can be set as a warning to inform the users of the distortion of such information. In addition, seditious opinion leaders should be prevented from entering information propagation in the early stage to obtain a smaller βF. Later, it is also necessary to monitor relevant terms in real time to prevent secondary outbreaks caused by related derivative events appearing on public pages, such as "trending" and "hotspots, " thus decreasing βB and pB.
Our research promotes network self-regulation and can be used to develop effective intervention strategies for promoting or suppressing events. However, in the discussed scenario, the repeated propagation behaviors of users is an essential factor contributing to the secondary outbreak of events, which affects the efficiency of external interventions and thus needs to be considered. Therefore, our future research will focus on the dynamics driven by repeated propagation behaviors and the corresponding intervention means.
This work was supported by the National Key Research and Development Program (Nos. 2021YFF0901705, 2021YFF0901700); the State Key Laboratory of Media Convergence and Communication, Communication University of China; the Fundamental Research Funds for the Central Universities and the High-Quality and Cutting-Edge Disciplines Construction Project for Universities in Beijing (Internet Information, Communication University of China).
The authors declare that there is no conflict of interest.
[1] |
Picard C, Baruffa E, Bosco M (2008) Enrichment and diversity of plant-probiotic microorganisms in the rhizosphere of hybrid maize during four growth cycles. Soil Biol Biochem 40: 106–115. doi: 10.1016/j.soilbio.2007.07.011
![]() |
[2] |
Benrebah F, Prevost D, Yezza A, et al. (2007) Agro-industrial waste materials and wastewater sludge for rhizobial inoculant production: A review. Bioresour Technol 98: 3535–3546. doi: 10.1016/j.biortech.2006.11.066
![]() |
[3] |
Mayak S, Tirosh T, Glick BR (2004) Plant growth-promoting bacteria confer resistance in tomato plants to salt stress. Plant Physiol Biochem 42: 565–572. doi: 10.1016/j.plaphy.2004.05.009
![]() |
[4] |
Singh JS, Pandey VC, Singh DP (2011) Efficient soil microorganisms: A new dimension for sustainable agriculture and environmental development. Agric Ecosyst Environ 140: 339–353. doi: 10.1016/j.agee.2011.01.017
![]() |
[5] | Gomez CG, Valero NV, Brigard RC (2012) Halotolerant/alkalophilic bacteria associated with the cyanobacterium Arthrospira platensis (Nordstedt) Gomont that promote early growth in Sorghum bicolor (L.). Moench Agron Colomb 30: 111–115. |
[6] |
Yang J, Kloepper JW, Ryu CM (2009) Rhizosphere bacteria help plants tolerate abiotic stress. Trends Plant Sci 14: 1–4. doi: 10.1016/j.tplants.2008.10.004
![]() |
[7] |
Bhattacharyya D, Yu SM, Lee YH (2015) Volatile compounds from Alcaligenes faecalis JBCS1294 confer salt tolerance in Arabidopsis thaliana through the auxin and gibberellin pathways and differential modulation of gene expression in root and shoot tissues. Plant Growth Regul 75: 297–306. doi: 10.1007/s10725-014-9953-5
![]() |
[8] | Zhang Z, Lin W, Yang Y, et al. (2011) Effects of consecutively monocultured Rehmannia glutinosa L. on diversity of fungal community in rhizospheric soil. Agric Sci China 10: 1374–1384. |
[9] | Zhang H, Kim MS, Sun Y, et al. (2008) Soil bacteria confer plant salt tolerance by tissue-specific regulation of the sodium transporter HKT1. Mol Plant Microbe In 21: 737–744. |
[10] |
Porcel R, Zamarreño Á, García-Mina J, et al. (2014) Involvement of plant endogenous ABA in Bacillus megaterium PGPR activity in tomato plants. BMC Plant Biol 14: 36. doi: 10.1186/1471-2229-14-36
![]() |
[11] |
Tsuda K, Tsuji G, Higashiyama M, et al. (2016) Biological control of bacterial soft rot in Chinese cabbage by Lactobacillus plantarum strain BY under field conditions. Biol Control 100: 63–69. doi: 10.1016/j.biocontrol.2016.05.010
![]() |
[12] |
Waqas M, Khan AL, Kamran M, et al. (2012) Endophytic fungi produce gibberellins and indoleacetic acid and promotes host-plant growth during stress. Molecules 17: 10754–10773. doi: 10.3390/molecules170910754
![]() |
[13] | Khan AL, Hamayun M, Ahmad N, et al. (2011) Exophiala sp. LHL08 reprograms Cucumis sativus to higher growth under abiotic stresses. Physiol Plant 143: 329–343. |
[14] |
Khan AL, Hamayun M, Ahmad N, et al. (2011) Salinity stress resistance offered by endophytic fungal interaction between Penicillium minioluteum LHL09 and glycine max. L. J Microbiol Biotechnol 21: 893–902. doi: 10.4014/jmb.1103.03012
![]() |
[15] | De Palma M, D'Agostino N, Proietti S, et al. (2016) Suppression Subtractive Hybridization analysis provides new insights into the tomato (Solanum lycopersicum L.) response to the plant probiotic microorganism Trichoderma longibrachiatum MK1. J Plant Physiol 190: 79–94. |
[16] |
Qin Y, Druzhinina IS, Pan X, et al. (2016) Microbially mediated plant salt tolerance and microbiome-based solutions for saline agriculture. Biotechnol Adv 34: 1245–1259. doi: 10.1016/j.biotechadv.2016.08.005
![]() |
[17] |
Upadhyay SK, Singh JS, Saxena AK, et al. (2012) Impact of PGPR inoculation on growth and antioxidant status of wheat under saline conditions. Plant Biol 14: 605–611. doi: 10.1111/j.1438-8677.2011.00533.x
![]() |
[18] |
Redman RS, Kim YO, Woodward CJDA, et al. (2011) Increased fitness of rice plants to abiotic stress via habitat adapted symbiosis: A strategy for mitigating impacts of climate change. PLoS One 6: e14823. doi: 10.1371/journal.pone.0014823
![]() |
[19] |
Belimov AA, Dodd IC, Safronova VI, et al. (2014) Abscisic acid metabolizing rhizobacteria decrease ABA concentrations in planta and alter plant growth. Plant Physiol Biochem 74: 84–91. doi: 10.1016/j.plaphy.2013.10.032
![]() |
[20] |
Banaei-Asl F, Bandehagh A, Uliaei ED, et al. (2015) Proteomic analysis of canola root inoculated with bacteria under salt stress. J Proteomics 124: 88–111. doi: 10.1016/j.jprot.2015.04.009
![]() |
[21] | Melnick RL, Zidack NK, Bailey BA, et al. (2008) Bacterial endophytes: Bacillus spp. from annual crops as potential biological control agents of black pod rot of cacao. Biological Control 46: 46–56. |
[22] |
Strobel GA (2002) Rainforest endophytes and bioactive products. Crit Rev Biotechnol 22: 315–333. doi: 10.1080/07388550290789531
![]() |
[23] |
Shoresh M, Yedidia I, Chet I (2005) Involvement of jasmonic acid/ethylenesignaling pathway in the systemic resistance induced in cucumber by Trichoderma asperellum T203. Phytopathology 95: 76–84. doi: 10.1094/PHYTO-95-0076
![]() |
[24] | Glick BR (2015) Beneficial Plant-Bacterial Interactions, Heidelberg: Springer, 243. |
[25] |
Santoyo G, Moreno-Hagelsieb G, Del COM, et al. (2016) Plant growth-promoting bacterial endophytes. Microbiol Res 183: 92–99. doi: 10.1016/j.micres.2015.11.008
![]() |
[26] | Cerozi BDS, Fitzsimmons K (2016) Use of Bacillus spp. to enhance phosphorus vailability and serve as a plant growth promoter in aquaponics systems. Sci Hortic -Amsterdam 211: 277–282. |
[27] |
Vinale F, Sivasithamparam K, Ghisalberti EL, et al. (2008) A novel role for Trichoderma secondary metabolites in the interactions with plants. Physiol Mol Plant P 72: 80–86. doi: 10.1016/j.pmpp.2008.05.005
![]() |
[28] |
Larsen J, Pineda-Sánchez H, Delgado-Arellano I, et al. (2017) Interactions between microbial plant growth promoters and their effects on maize growth performance in different mineral and organic fertilization scenarios. Rhizosphere 3: 75–81. doi: 10.1016/j.rhisph.2017.01.003
![]() |
[29] |
Kuklinsky-Sobral J, Araújo WL, Mendes R, et al. (2004) Isolation and characterization of soybean-associated bacteria and their potential for plant growth promotion. Environ Microbiol 6: 1244–1251. doi: 10.1111/j.1462-2920.2004.00658.x
![]() |
[30] |
Meng Q, Jiang H, Hao JJ (2016) Effects of Bacillus velezensis strain BAC03 in promoting plant growth. Biol Control 98: 18–26. doi: 10.1016/j.biocontrol.2016.03.010
![]() |
[31] |
Cohen AC, Travaglia CN, Bottini R, et al. (2009) Participation of abscisic acid and gibberellins produced by endophytic Azospirillum in the alleviation of drought effects in maize. Botany 87: 455–462. doi: 10.1139/B09-023
![]() |
[32] |
Silva HSA, Tozzi JPL, Terrasan CRF, et al. (2012) Endophytic microorganisms from coffee tissues as plant growth promoters and biocontrol agents of coffee leaf rust. Biol Control 63: 62–67. doi: 10.1016/j.biocontrol.2012.06.005
![]() |
[33] | Miransari M (2010) Current research, technology and education topics in applied microbiology and microbial biotechnology, In: Microbiology Book Series-2010 Edition, Spain. |
[34] |
Vessey JK (2003) Plant growth promoting rhizobacteria as biofertilizers. Plant Soil 255: 571–586. doi: 10.1023/A:1026037216893
![]() |
[35] |
Richardson AE, Barea JM, Mcneill AM, et al. (2009) Acquisition of phosphorus and nitrogen in the rhizosphere and plant growth promotion by microorganisms. Plant Soil 321: 305–339. doi: 10.1007/s11104-009-9895-2
![]() |
[36] |
Rodriguez H, Fraga R (1999) Phosphate solubilizing bacteria and their role in plant growth promotion. Biotechnol Adv 17: 319–339. doi: 10.1016/S0734-9750(99)00014-2
![]() |
[37] |
Sturz AV, Nowak J (2000) Endophytic communities of rhizobacteria and the strategies required to create yield enhancing associations with crops. Appl Soil Ecol 15: 183–190. doi: 10.1016/S0929-1393(00)00094-9
![]() |
[38] |
Sudhakar P, Chattopadhyay GN, Gangwar SK, et al. (2000) Effect of foliar application of Azotobacter, Azospirillum and Beijerinckia on leaf yield and quality of mulberry (Morus alba). J Agric Sci 134: 227–234. doi: 10.1017/S0021859699007376
![]() |
[39] |
Karlidag H, Esitken A, Turan M, et al. (2007) Effects of root inoculation of plant growth promoting rhizobacteria (PGPR) on yield, growth and nutrient element contents of leaves of apple. Sci Hortic-Amsterdam 114: 16–20. doi: 10.1016/j.scienta.2007.04.013
![]() |
[40] | Ilyas N, Bano A (2012) Potencial use of soil microbial community in agriculture, In: Bacteria in Agrobiology: Plant Probiotics, 1 Eds., Berlin: Springer Berlin Heidelberg, 45–64. |
[41] | Glick BR (2012) Plant growth-promoting bacteria: mechanisms and applications. Scientifica. |
[42] |
Glick BR (2014) Bacteria with ACC deaminase can promote plant growth and help to feed the world. Microbiol Res 169: 30–39. doi: 10.1016/j.micres.2013.09.009
![]() |
[43] |
Brígido C, Glick BB, Oliveira S (2017) Survey of plant growth-promoting mechanisms in native Portuguese Chickpea Mesorhizobium isolates. Microbial Ecol 73: 900–915. doi: 10.1007/s00248-016-0891-9
![]() |
[44] |
Bhattacharyya PN, Jha DK (2012) Plant growth-promoting rhizobacteria (PGPR): emergence in agriculture. World J Microb Biotechnol 28: 1327–1350. doi: 10.1007/s11274-011-0979-9
![]() |
[45] |
Gray EJ, Smith DL (2005) Intracellular and extracellular PGPR: commonalities and distinctions in the plant-bacterium signaling processes. Soil Biol Biochem 37: 395–412. doi: 10.1016/j.soilbio.2004.08.030
![]() |
[46] | Figueiredo MVB, Martinez CR, Burity HA, et al. (2008) Plant growth-promoting rhizobacteria for improving nodulation and nitrogen fixation in the common bean (Phaseolus vulgaris L.). World J Microb Biotechnol 24: 1187e93. |
[47] | Ahemad M, Kibret M (2014) Mechanisms and applications of plant growth promoting rhizobacteria: Current perspective. J King Saud Univ-Sci 26: 1–20. |
[48] | Antoun H, Prévost D (2006) Ecology of Plant Growth Promoting Rhizobacteria, In: Siddiqui ZA, Editor, PGPR: Biocontrol and Biofertilization, Dordrecht: Springer Netherlands, 1–38. |
[49] |
Brunner SM, Goos RJ, Swenson SJ, et al. (2015) Impact of nitrogen fixing and plant growth-promoting bacteria on a phloem-feeding soybean herbivore. Appl Soil Ecol 86: 71–81. doi: 10.1016/j.apsoil.2014.10.007
![]() |
[50] |
Smith SE, Manjarrez M, Stonor R, et al. (2015) Indigenous arbuscular mycorrhizal (AM) fungi contribute to wheat phosphate uptake in a semi-arid field environment, shown by tracking with radioactive phosphorus. Appl Soil Ecol 96: 68–74. doi: 10.1016/j.apsoil.2015.07.002
![]() |
[51] |
Mensah JA, Koch AM, Antunes PM, et al. (2015) High functional diversity within species of arbuscular mycorrhizal fungi is associated with differences in phosphate and nitrogen uptake and fungal phosphate metabolism. Mycorrhiza 25: 533–546. doi: 10.1007/s00572-015-0631-x
![]() |
[52] |
Augé RM, Toler HD, Saxton AM (2015) Arbuscular mycorrhizal symbiosis alters stomatal conductance of host plants more under drought than under amply watered conditions: a meta-analysis. Mycorrhiza 25: 13–24. doi: 10.1007/s00572-014-0585-4
![]() |
[53] |
Schübler A, Schwarzott D, Walker C (2001) A new fungal phylum, the Glomeromycota: phylogeny and evolution. Mycol Res 105: 1413–1421. doi: 10.1017/S0953756201005196
![]() |
[54] |
Dodd JC, Boddington CL, Rodriguez A, et al. (2000) Mycelium of Arbuscular Mycorrhizal fungi (AMF) from different genera: form, function and detection. Plant Soil 226: 131–151. doi: 10.1023/A:1026574828169
![]() |
[55] |
Artursson V, Finlay RD, Jansson JK (2006) Interactions between arbuscular mycorrhizal fungi and bacteria and their potential for stimulating plant growth. Environ Microbiol 8: 1–10. doi: 10.1111/j.1462-2920.2005.00942.x
![]() |
[56] |
Miransari M (2011) Interactions between arbuscular mycorrhizal fungi and soil bacteria. Appl Microbiol Biotechnol 89: 917–930. doi: 10.1007/s00253-010-3004-6
![]() |
[57] |
Zhang L, Xu M, Liu Y, et al. (2016) Carbon and phosphorus exchange may enable cooperation between an arbuscular mycorrhizal fungus and a phosphate-solubilizing bacterium. New Phytologist 210: 1022–1032. doi: 10.1111/nph.13838
![]() |
[58] |
Chaiyasen A, Young JPW, Teaumroong N, et al. (2014) Characterization of arbuscular mycorrhizal fungus communities of Aquilaria crassna and Tectona grandis roots and soils in Thailand plantations. Plos One 9: e112591. doi: 10.1371/journal.pone.0112591
![]() |
[59] | Chaiyasen A, Douds DD, Gavinlertvatan P, et al. (2017) Diversity of arbuscular mycorrhizal fungi in Tectona grandis Linn.f. plantations and their effects on growth of micropropagated plantlets. New Forest 48: 547–562. |
[60] | Brundrett MC, Bougher N, Dell B, et al. (1996) Working with mycorrhizas in forestry and agriculture. |
[61] | Smith S, Read D (2008) Mycorrhizal Symbiosis, New York: Academic Press. |
[62] |
Porcel R, Ruiz-Lozano JM (2004) Arbuscular mycorrhizal influence on leaf water potential, solute accumulation, and oxidative stress in soybean plants subjected to drought stress. J Exp Bot 55: 1743–1750. doi: 10.1093/jxb/erh188
![]() |
[63] |
Doubková P, Vlasáková E, Sudová R (2013) Arbuscular mycorrhizal symbiosis alleviates drought stress imposed on Knautia arvensis plants in serpentine soil. Plant Soil 370: 149–161. doi: 10.1007/s11104-013-1610-7
![]() |
[64] | Azcón-Aguilar C, Barea JM (1996) Arbuscular mycorrhizas and biological control of soil-borne plant pathogens-an overview of the mechanisms involved. Mycorrhiza 6: 457–464. |
[65] |
Wright SF, Upadhyaya A (1998) A survey of soils for aggregate stability and glomalin, a glycoprotein produced by hyphae of arbuscular mycorrhizal fungi. Plant Soil 198: 97–107. doi: 10.1023/A:1004347701584
![]() |
[66] |
Rillig MC (2004) Arbuscular mycorrhizae, glomalin, and soil aggregation. Can J Soil Sci 84: 355–363. doi: 10.4141/S04-003
![]() |
[67] |
Rillig MC, Ramsey PW, Morris S, et al. (2003) Glomalin, an arbuscularmycorrhizal fungal soil protein, responds to land-use change. Plant Soil 253: 293–299. doi: 10.1023/A:1024807820579
![]() |
[68] |
Bhardwaj D, Ansari MW, Sahoo K, et al. (2014) Biofertilizers function as key player in sustainable agriculture by improving soil fertility, plant tolerance and crop productivity. Microb Cell Fact 13: 1–10. doi: 10.1186/1475-2859-13-1
![]() |
[69] | Goswami D, Thakker JN, Dhandhukia PC (2016) Portraying mechanics of plant growth promoting rhizobacteria (PGPR): A review. Cogent Food Agr 2: 1–19. |
[70] |
Kang SM, Khan AL, Waqas M, et al. (2014) Plant growth-promoting rhizobacteria reduce adverse effects of salinity and osmotic stress by regulating phytohormones and antioxidants in Cucumis sativus. J Plant Interact 9: 673–682. doi: 10.1080/17429145.2014.894587
![]() |
[71] |
Bartels D, Sunkar R (2005) Drought and salt tolerance in plants. Crit Rev Plant Sci 24: 23–58. doi: 10.1080/07352680590910410
![]() |
[72] | Hussain TM, Chandrasekhar T, Hazara M, et al. (2008) Recent advances in salt stress biology-A review. Biotechnol Mol Biol Rev 3: 8–13. |
[73] |
Saleem M, Arshad M, Hussain S, et al. (2007) Perspective of plant growth promoting rhizobacteria (PGPR) containing ACC deaminase in stress agriculture. J Ind Microbiol Biotechnol 34: 635–648. doi: 10.1007/s10295-007-0240-6
![]() |
[74] | Shaharoona B, Arshad M, Zahir ZA (2006) Performance of Pseudomonas spp. containing ACC-deaminase for improving growth and yield of maize (Zea mays L.) in the presence of nitrogenous fertilizer. Soil Biol Biochem 38: 2971–2975. |
[75] |
Egamberdiyeva D (2007) The effect of plant growth promoting bacteria on growth and nutrient uptake of maize in two different soils. Appl Soil Ecol 36: 184–189. doi: 10.1016/j.apsoil.2007.02.005
![]() |
[76] | Barriuso J, Solano BR, Gutiérrez MFJ (2008) Protection against pathogen and salt stress by four plant growth-promoting Rhizobacteria isolated from Pinus sp. on Arabidopsis thaliana. Biol Control 98: 666–672. |
[77] |
Kohler J, Hernández JA, Caravaca F, et al. (2009) Induction of antioxidant enzymes is involved in the greater effectiveness of a PGPR versus AM fungi with respect to increasing the tolerance of lettuce to severe salt stress. Environ Exp Botany 65: 245–252. doi: 10.1016/j.envexpbot.2008.09.008
![]() |
[78] | Sandhya V, Ali SZ, Grover M, et al. (2010) Effect of plant growth promoting Pseudomonas spp. on compatible solutes, antioxidant status and plant growth of maize under drought stress. Plant Growth Regul 62: 21–30. |
[79] |
Seneviratne G, Jayasekara APDA, De Silva MSDL, et al. (2011) Developed microbial biofilms can restore deteriorated convencional agricultural soils. Soil Biol Biochem 43: 1059–1062. doi: 10.1016/j.soilbio.2011.01.026
![]() |
[80] | Shukla PS, Agarwal PK, Jha B (2012) Improved salinity tolerance of Arachis hypogaea (L.) by the interaction of halotolerant plant-growth-promoting-rhizobacteria. J Plant Growth Regul 31: 195–206. |
[81] |
Swarnalakshmi K, Prasanna R, Kumar A, et al. (2013) Evaluating the influence of novel cyanobacterial biofilmed biofertilizers on soil fertility and plant nutrition in wheat. Eur J Soil Biol 55: 107–116. doi: 10.1016/j.ejsobi.2012.12.008
![]() |
[82] |
Sarma RK, Saikia R (2014) Alleviation of drought stress in mug bean by strain Pseudomonas aeruginosa GGRJ21. Plant Soil 377: 111–126. doi: 10.1007/s11104-013-1981-9
![]() |
[83] | Cardinale M, Ratering S, Suarez C, et al. (2015) Paradox of plant growth promotion potencial of rhizobacteria and their actual promotion effect on growth of barley (Hordeum vulgare L.) under salt stress. Microbiol Res 181: 22–32. |
[84] |
Islam F, Yasmeen T, Arif MS, et al. (2016) Plant growth promotion bacteria confer salt tolerance in Vigna radiata by up-regulating antioxidant defense and biological soil fertility. Plant Growth Regul 80: 23–36. doi: 10.1007/s10725-015-0142-y
![]() |
[85] |
Shahzad R, Khan AL, Bilal S, et al. (2017) Inoculation of abscisic acid-producing endophytic bacteria enhances salinity stress tolerance in Oryza sativa. Environ Exp Botany 136: 68–77. doi: 10.1016/j.envexpbot.2017.01.010
![]() |
[86] |
Rajkumar M, Sandhya S, Prasad MNV, et al. (2012) Perspectives of plant-associated microbes in heavy metal phytoremediation. Biotechnol Adv 30: 1562–1574. doi: 10.1016/j.biotechadv.2012.04.011
![]() |
[87] | Saleh S, Huang XD, Greenberg BM, et al. (2004) Phytoremediation of persistent organic contaminants in the environment, In: Singh A, Ward O, Editors, Appl ied Bioremediation Phytoremediation, Berlin: Springer-Verlag, 115–134. |
[88] |
Zhuang X, Chen J, Shim H, et al. (2007) New advances in plant growth-promotion rhizobacteria for bioremediation. Environ Int 33: 406–413. doi: 10.1016/j.envint.2006.12.005
![]() |
[89] | Ma Y, Prasad MNV, Rajkumar M, et al. (2010) Plant growth promoting rhizobacteria and endophytes accelerate phytoremediation of metalliferous soils. Biotechnol Adv 29: 248–258. |
[90] |
Pinter IF, Salomon MV, Berli F, et al. (2017) Characterization of the As(III) tolerance conferred by plant growth promoting rhizobacteria to in vitro-grown grapevine. Appl Soil Ecol 109: 60–68. doi: 10.1016/j.apsoil.2016.10.003
![]() |
[91] | Babu AG, Kim JD, Oh BT (2013) Enhancement of heavy metal phytoremediation by Alnus firma with endophytic Bacillus thuringiensis GDB-1. J Hazard Mat 250: 477–483. |
[92] | Kuklinsky-Sobral J, Araújo WL, Mendes R, et al. (2004) Isolation and characterization of soybean-associated bacteria and their potential for plant growth promotion. Environ Microbiol 12: 1244–1251. |
[93] |
Chelius MK, Triplett EW (2001) The diversity of archaea and bacteria in association with the roots of Zea mays L. Microb Ecol 41: 252–263. doi: 10.1007/s002480000087
![]() |
[94] |
Garbeva P, van Overbeek LS, van Vuurde JEL, et al. (2001) Analysis of endophytic bacterial communities of potato by plating and denaturing gradient gel electrophoresis (DGGE) of 16S rDNA based PCR fragments. Microb Ecol 41: 369–383. doi: 10.1007/s002480000096
![]() |
[95] |
Araújo WL, Marcon J, Maccheroni WJ, et al. (2002) Diversity of endophytic bacterial populations and their interaction with Xylella fastidiosa in citrus plants. Appl Environ Microbiol 68: 4906–4914. doi: 10.1128/AEM.68.10.4906-4914.2002
![]() |
[96] |
Zinniel DK, Lambrecht P, Harris B, et al. (2002) Isolation and characterization of endophytic colonizing bacteria from agronomic crops and prairie plants. Appl Environ Microbiol 68: 2198–2208. doi: 10.1128/AEM.68.5.2198-2208.2002
![]() |
[97] |
Idris R, Trifonova R, Puschenreiter M, et al. (2004) Bacterial communities associated with flowering plants of the Ni Hyperaccumulator Thlaspi goesingense. Appl Environ Microbiol 70: 2667–2677. doi: 10.1128/AEM.70.5.2667-2677.2004
![]() |
[98] |
Andreote FD, Carneiro RT, Salles JF, et al. (2009) Culture-independent assessment of Alphaproteobacteria related to order Rhizobiales and the diversity of cultivated Methylobacterium in the rhizosphere and rhizoplane of transgenic eucalyptus. Microb Ecol 57: 82–93. doi: 10.1007/s00248-008-9405-8
![]() |
[99] |
Sagaram US, DeAngelis KM, Trivedi P, et al. (2009) Bacterial diversity analysis of Huanglongbing pathogen-infected citrus, using phylochip arrays and 16S rRNA gene clone library sequencing. Appl Environ Microbiol 75: 1566–1574. doi: 10.1128/AEM.02404-08
![]() |
[100] |
Gv DMP, Magalhães KT, Lorenzetii ER, et al. (2012) A multiphasic approach for the identification of endophytic bacterial in strawberry fruit and their potential for plant growth promotion. Microb Ecol 63: 405–417. doi: 10.1007/s00248-011-9919-3
![]() |
[101] |
Xia X, Lie TK, Qian X (2011) Species diversity, distribution, and genetic structure of endophytic and epiphytic Trichoderma associated with banana roots. Microb Ecol 61: 619–625. doi: 10.1007/s00248-010-9770-y
![]() |
[102] |
Ikeda AC, Bassani LL, Adamoski D, et al. (2013) Morphological and genetic characterization of endophytic bacteria isolated from roots of different maize genotypes. Microb Ecol 65: 154–160. doi: 10.1007/s00248-012-0104-0
![]() |
[103] | Verma VC, Gond SK, Kumar A, et al. (2009) Endophytic actinomycetes from Azadirachta indica A. Juss.: isolation, diversity, and anti-microbial activity. Microb Ecol 57: 749–756. |
[104] |
Manter DK, Delgado JA, Holm DG, et al. (2010) Pyrosequencing reveals a highly diverse and cultivar-specific bacterial endophyte community in potato roots. Microb Ecol 60: 157–166. doi: 10.1007/s00248-010-9658-x
![]() |
[105] | Cruz LM, Souza EM, Weber OB, et al. (2001) 16S Ribosomal DNA characterization of nitrogen-fixing bacteria isolated from banana (Musa spp.) and pineapple (Ananas comosus (L.) Merril). Appl Environ Microbiol 67: 2375–2379. |
[106] |
Marques ASA, Marchaison A, Gardan L, et al. (2008) BOXPCR-based identification of bacterial species belonging to Pseudomonas syringae: P. viridiflava group. Gen Mol Biol 31: 106–115. doi: 10.1590/S1415-47572008000100019
![]() |
[107] |
Menna P, Barcellos FG, Hungria M (2009) Phylogeny and taxonomy of a diverse collection of Bradyrhizobium strains based on multilocus sequence analysis of 16S rRNA, ITS, glnII, recA, atpD and dnaK genes. Int J Syst Evol Microbiol 59: 2934–2950. doi: 10.1099/ijs.0.009779-0
![]() |
[108] |
Zhang XX, Gao JS, Cao YH (2013) Long-term rice and green manure rotation alters the endophytic bacterial communities of the rice root. Microb Ecol 66: 917–926. doi: 10.1007/s00248-013-0293-1
![]() |
[109] |
Onstott TC, Phelps TJ, Colwell FS, et al. (1998) Observations pertaining to the origin and ecology of microorganisms recovered from the deep subsurface of Taylorsville Basin, Virginia. Geomicrobiol J 15: 353–385. doi: 10.1080/01490459809378088
![]() |
[110] |
Nadkarni MA, Martin FE, Hunter N, et al. (2009) Methods for optimizing DNA extraction before quantifying oral bacterial numbers by real-time PCR. FEMS Microbiol Lett 296: 45–51. doi: 10.1111/j.1574-6968.2009.01629.x
![]() |
[111] | Muyzer G, De WEC, Uitterlinden AG (1993) Profiling of complex microbial populations by denaturing gradient gel electroforesis analysis of polymerase chain reaction-amplified genes coding for 16S rRNA. Appl Environ Microbiol 59: 695–700. |
[112] |
Orphan VJ, Taylor LT, Hafenbradl D, et al. (2000) Culture-dependent and culture-independent characterization of microbial assemblages associated with high-temperature petroleum reservoirs. Appl Environ Microbiol 66: 700–711. doi: 10.1128/AEM.66.2.700-711.2000
![]() |
[113] | Monteiro JM, Vollú RE, Coelho MRR, et al. (2009) Comparison of the bacterial community and characterization of plant growth-promoting rhizobacteria from different genotypes of Chrysopogon zizanioides (L.) Roberty (Vetiver) rhizospheres. J Microbiol 4: 363–370. |
[114] | Sun L, Qiu F, Zhang X, et al. (2008) Endophytic bacterial diversity in rice (Oryza sativa L.) roots estimated by 16S rDNA sequence analysis. Microb Ecol 55: 415–424. |
[115] | Snyder LAS, Loman N, Pallen MJ, et al. (2009) Next-generation sequencing-the promise and perils of charting the great microbial unknown. Microb Ecol 57: 1–3. |
[116] |
Studholme DJ, Glover RH, Boonham N (2011) Application of high-throughput DNA sequencing in phytopathology. Annu Rev Phytopathol 49: 87–105. doi: 10.1146/annurev-phyto-072910-095408
![]() |
[117] |
Lundberg DS, Lebeis SL, Paredes SH (2012) Defining the core Arabidopsis thaliana root microbiome. Nature 488: 86–90. doi: 10.1038/nature11237
![]() |
[118] | Akinsanya MA, Goh JK, Lim SP, et al. (2015) Metagenomics study of endophytic bacteria in Aloe vera using next-generation technology: Genom Data 6: 159–163. |
Parameter | Interpretation |
βF | The average direct exposure rate, i.e., the average rate at which susceptible users can access information through social relations. |
βB | The average indirect exposure rate, i.e., the average rate at which susceptible users can access information through the topic communities. |
pF | The average direct forwarding probability, i.e., the average probability that susceptible users see and forward the information through social relations. |
pB | The average indirect forwarding probability, i.e., the average probability that susceptible users see and forward the information from the topic communities. |
αF | The average immune rate, i.e., the average rate at which forwarding users become inactive, is related to the behavioral law of users. |
αB | The average metabolic rate, i.e., the average rate at which information in topic communities gradually becomes inactive, is related to the algorithmic law of social media platforms. |
γ | The average transfer rate, i.e., the average rate at which the information is filtered and presented in topic communities generated by social media algorithms. |
Parameter | Interpretation |
βMF | The coefficient for the effects of external interventions on βF, i.e., the effects of external interventions on the direct access to information relying on social relations. |
βMB | The coefficient for the effects of external interventions on βB, i.e., the effects of external interventions on the indirect access to information relying on topic communities. |
pMF | The coefficient for the effects of external interventions on pF, i.e., the effects of external interventions on the direct forwarding probability relying on social relations. |
pMB | The coefficient for the effects of external interventions on pB, i.e., the effects of external interventions on the indirect forwarding probability relying on topic communities. |
γM | The coefficient for the effects of external interventions on γ, i.e., the effects of external interventions on the process of filtering and presenting information in topic communities. |
Fmax | Cs | tFmax | R0 | |
For the positive event | 822.6215 | 3853.3000 | 42 | 4.1351 |
For the negative event | 3938.7000 | 23,188 | 71 | 6.9910 |
Fmax | Cs | tFmax | |
Control Group | 822.6215 | 3853.3000 | 42 |
Experimental Group (a) | 934.6235 | 3822.2000 | 37 |
Experimental Group (b) | 830.9647 | 3886.1000 | 41 |
Experimental Group (c) | 1027.2000 | 4175.7000 | 37 |
Experimental Group (d) | 833.0052 | 3920.3000 | 41 |
Experimental Group (e) | 830.9647 | 3886.1000 | 41 |
Fmax | Cs | tFmax | |
Control Group | 3938.7000 | 23,188 | 71 |
Experimental Group (a) | 3628.2000 | 24,135 | 80 |
Experimental Group (b) | 3773.4000 | 22,778 | 72 |
Experimental Group (c) | 3292.4000 | 22,149 | 80 |
Experimental Group (d) | 3747.0000 | 22,397 | 72 |
Experimental Group (e) | 3773.4000 | 22,778 | 72 |
Parameter | Interpretation |
βF | The average direct exposure rate, i.e., the average rate at which susceptible users can access information through social relations. |
βB | The average indirect exposure rate, i.e., the average rate at which susceptible users can access information through the topic communities. |
pF | The average direct forwarding probability, i.e., the average probability that susceptible users see and forward the information through social relations. |
pB | The average indirect forwarding probability, i.e., the average probability that susceptible users see and forward the information from the topic communities. |
αF | The average immune rate, i.e., the average rate at which forwarding users become inactive, is related to the behavioral law of users. |
αB | The average metabolic rate, i.e., the average rate at which information in topic communities gradually becomes inactive, is related to the algorithmic law of social media platforms. |
γ | The average transfer rate, i.e., the average rate at which the information is filtered and presented in topic communities generated by social media algorithms. |
Parameter | Interpretation |
βMF | The coefficient for the effects of external interventions on βF, i.e., the effects of external interventions on the direct access to information relying on social relations. |
βMB | The coefficient for the effects of external interventions on βB, i.e., the effects of external interventions on the indirect access to information relying on topic communities. |
pMF | The coefficient for the effects of external interventions on pF, i.e., the effects of external interventions on the direct forwarding probability relying on social relations. |
pMB | The coefficient for the effects of external interventions on pB, i.e., the effects of external interventions on the indirect forwarding probability relying on topic communities. |
γM | The coefficient for the effects of external interventions on γ, i.e., the effects of external interventions on the process of filtering and presenting information in topic communities. |
Fmax | Cs | tFmax | R0 | |
For the positive event | 822.6215 | 3853.3000 | 42 | 4.1351 |
For the negative event | 3938.7000 | 23,188 | 71 | 6.9910 |
Fmax | Cs | tFmax | |
Control Group | 822.6215 | 3853.3000 | 42 |
Experimental Group (a) | 934.6235 | 3822.2000 | 37 |
Experimental Group (b) | 830.9647 | 3886.1000 | 41 |
Experimental Group (c) | 1027.2000 | 4175.7000 | 37 |
Experimental Group (d) | 833.0052 | 3920.3000 | 41 |
Experimental Group (e) | 830.9647 | 3886.1000 | 41 |
Fmax | Cs | tFmax | |
Control Group | 3938.7000 | 23,188 | 71 |
Experimental Group (a) | 3628.2000 | 24,135 | 80 |
Experimental Group (b) | 3773.4000 | 22,778 | 72 |
Experimental Group (c) | 3292.4000 | 22,149 | 80 |
Experimental Group (d) | 3747.0000 | 22,397 | 72 |
Experimental Group (e) | 3773.4000 | 22,778 | 72 |