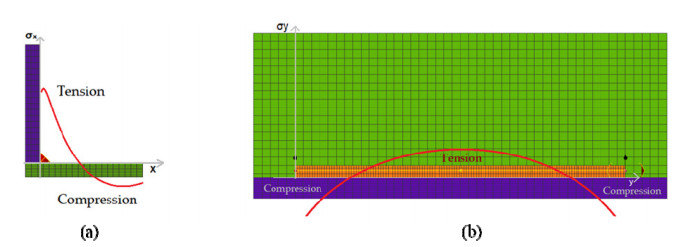
We propose an epidemiological model with distributed recovery and death rates. It represents an integrodifferential system of equations for susceptible, exposed, infectious, recovered and dead compartments. This model can be reduced to the conventional ODE model under the assumption that recovery and death rates are uniformly distributed in time during disease duration. Another limiting case, where recovery and death rates are given by the delta-function, leads to a new point-wise delay model with two time delays corresponding to the infectivity period and disease duration. Existence and positiveness of solutions for the distributed delay model and point-wise delay model are proved. The basic reproduction number and the final size of the epidemic are determined. Both, the ODE model and the delay models are used to describe COVID-19 epidemic progression. The delay model gives a better approximation of the Omicron data than the conventional ODE model from the point of view of parameter estimation.
Citation: Masoud Saade, Samiran Ghosh, Malay Banerjee, Vitaly Volpert. An epidemic model with time delays determined by the infectivity and disease durations[J]. Mathematical Biosciences and Engineering, 2023, 20(7): 12864-12888. doi: 10.3934/mbe.2023574
[1] | Harish Mooli, Srinivasa Rao Seeram, Satyanarayana Goteti, Nageswara Rao Boggarapu . Optimal weld bead profiles in the conduction mode LBW of thin Ti–6Al–4V alloy sheets. AIMS Materials Science, 2021, 8(5): 698-715. doi: 10.3934/matersci.2021042 |
[2] | Ehsan Harati, Paul Kah . Laser welding of aluminum battery tab to variable Al/Cu busbars in Li-ion battery joint. AIMS Materials Science, 2022, 9(6): 884-918. doi: 10.3934/matersci.2022053 |
[3] | N. S. Biradar . Effect of transverse mechanical arc oscillation on hot cracking (solidification & liquation) and weld metal properties of AA2014 T6 TIG welds. AIMS Materials Science, 2016, 3(4): 1544-1560. doi: 10.3934/matersci.2016.4.1544 |
[4] | Paul Kah, Pavel Layus, Benoit Ndiwe . Submerged arc welding process peculiarities in application for Arctic structures. AIMS Materials Science, 2022, 9(3): 498-511. doi: 10.3934/matersci.2022029 |
[5] | Utpal K. Dhar, Md. Farabi Rahman, Mustafa Oguzhan Ayanoglu, Ahammad Abdullah . Welding on C67 steel grade sheet: Influence of the parameters and post welding heat treatment. AIMS Materials Science, 2023, 10(3): 453-464. doi: 10.3934/matersci.2023025 |
[6] | Muhammad Awwaluddin, Sri Hastuty, Djoko Hadi Prajitno, Makmuri, Budi Prasetiyo, Yudi Irawadi, Jekki Hendrawan, Harry Purnama, Eko Agus Nugroho . Effect of Yttrium on corrosion resistance of Zr-based alloys in Ringer's lactate solution for biomaterial applications. AIMS Materials Science, 2024, 11(3): 565-584. doi: 10.3934/matersci.2024028 |
[7] | Francois Njock Bayock, Paul Kah, Kibong Marius Tony . Heat input effects on mechanical constraints and microstructural constituents of MAG and laser 316L austenitic stainless-steel welded joints. AIMS Materials Science, 2022, 9(2): 236-254. doi: 10.3934/matersci.2022014 |
[8] | Shashi Bahl, Tarunpreet Singh, Virinder Kumar, Shankar Sehgal, Ashok Kumar Bagha . A systematic review on recent progress in advanced joining techniques of the lightweight materials. AIMS Materials Science, 2021, 8(1): 62-81. doi: 10.3934/matersci.2021005 |
[9] | Rafael R. Lucas, Rita C.M. Sales-Contini, Francisco J.G. da Silva, Edson C. Botelho, Rogério P. Mota . Plasma electrolytic oxidation (PEO): An alternative to conventional anodization process. AIMS Materials Science, 2024, 11(4): 684-711. doi: 10.3934/matersci.2024035 |
[10] | Pawan Kumar, B B Verma . Propagation of corrosion induced fatigue crack in aluminum alloy. AIMS Materials Science, 2022, 9(3): 512-521. doi: 10.3934/matersci.2022030 |
We propose an epidemiological model with distributed recovery and death rates. It represents an integrodifferential system of equations for susceptible, exposed, infectious, recovered and dead compartments. This model can be reduced to the conventional ODE model under the assumption that recovery and death rates are uniformly distributed in time during disease duration. Another limiting case, where recovery and death rates are given by the delta-function, leads to a new point-wise delay model with two time delays corresponding to the infectivity period and disease duration. Existence and positiveness of solutions for the distributed delay model and point-wise delay model are proved. The basic reproduction number and the final size of the epidemic are determined. Both, the ODE model and the delay models are used to describe COVID-19 epidemic progression. The delay model gives a better approximation of the Omicron data than the conventional ODE model from the point of view of parameter estimation.
Aluminum alloys gathers wide range of acceptance and potential application in automobile, aerospace, ship building industries, off shore structures and construction of bridges due to the low weight, superior strength to weight ratio and other anti-corrosive properties of this material [1]. Compared to steel, the main advantages of aluminum alloys are the high strength-to-weight ratio, the excellent corrosion resistance and reduced maintenance, which compensate their higher price compared to steel [2,3,4]. For example, 6xxx series Al-alloys are widely used due to the excellent physical and chemical properties, such as corrosion resistance, good formability and weldability [5]. The aluminum alloy studied in this work is 6082-T6, which is an extruded medium to high strength Al–Mg–Si alloy that contains manganese to increase ductility and toughness. The T6 condition is obtained through artificial ageing at a temperature of approximately 180 ℃ [6]. The most important advantages of 6082 aluminum alloys are low specific weight, high corrosion resistance and superior thermal conductivity [7,8].
One of the most common welding techniques for aluminum alloys is gas-metal arc welding (GMAW), which offers advantages such as deep penetration, smooth weld bead, high welding speed, large metal deposition rate, lower spatter, lower distortion and shrinkage, and lesser probability of porosity and fusion defects and the possibility to be robotized [9,10,11,12]. This process, due to the high temperatures involved, causes thermomechanical distortion and generation of residual stresses [13], which can lead to a premature collapse of the welded structure. In this way the mechanical properties of welding joints may determine the performance and service life of a welded structural part. Thus, the welding quality of aluminum alloy should be carefully considered [14].
In recent years, extensive studies [15] have been reported to investigate the influence of shielding gas on metal transfer [16], the influence of different wire-electrodes on metallurgical characterization [17], and effects of processing parameters (such as gas mixtures [18] and preheating [19]) on mechanical properties of GMAW process [20]. However, the basic parameters in robot welding, such as electric current, torch angle and welding speed, also need attention. Despite of many advantages in the GMAW process, there are still some shortcomings needing to be resolved, such as the welded distortion [21] and the generation of residual stresses [13].
To understand these behaviors in welds, three types of studies can be used: analytical, experimental and numerical. Among the analytical solutions, Rosenthal [22] proposed, for the first time, an analytical solution in 1946, of a semi-infinite body for thin and thick plates based on constant properties of the material. In 1999, Nguyen [23] showed an analytical solution based on Green's vector function using a Gaussian double-ellipsoidal thermal distribution. Goldak et al. [24] presented a numerical solution in the form of a semi-ellipsoidal and double-ellipsoidal heat source (applied methodology), more recently, Nasiri and Enzinger [25] proposed an analytical solution for heating flux in welding called adaptive function. These authors measured temperature values close to the melting line and determined the relative error less than 5% concerning the experimental method.
In this work, a set of numerical simulations based on experimental validation were implemented. It is important to notice that experimental analysis is extremely important to predict the reduction of mechanical properties induced by welding [26]. This prediction can be made by computational methods, due to the advancement of technology, through the Finite Element Method (FEM). It is possible to simulate a weld bead and approximate what the result will be, such as residual stresses and distortions [27,28,29,30].
Despite the capabilities of finite element software, it is important to perceive the discrepancy in computational results in relation to the experimental results. For this, we conducted a study of a corner welding of two aluminum bars 6082-T6 and validate the numerical results with the experimental ones. This study intends to observe the influence of certain welding parameters like electric current, torch angle and welding speed with three different levels each in the values of residual stresses. To perform the combinations of levels for the different parameters resorted to an orthogonal array of Taguchi L27 resulting 27 different combinations. The 27 combinations were simulated using the Software Simufacwelding® 6.0, from which it was possible to recall the values of the maximum principal stresses (MaxPS).
The residual stress plays an important role in crack initiating and propagating in the metal parts [31]. Residual stresses are those stresses that remain after deformation with all external forces removed [32], occurs in materials and mechanical components during manufacture from many processes like plastic deformation or forming, machining or welding processes, among others [33,34]. Both material and deposition process may produce a residual stress, which may be particularly critical in applications where a large mismatch exists between thermal, structural and mechanical properties of the layers and substrates [32]. That is, the state of a residual stress depends both, on the previous processes it underwent and also on the properties of the material that relate to the mechanical process.
For particular case of welding process, residual stresses are caused by the application of intense heat or thermal loading at the weld joint, which causes plasticity of the material underneath and immediately surrounding the weld arc, but the nearby cooler material remains elastic and acts to constrain the heated material. The thermal cycle imposed on any welded object causes thermal expansions and contractions to occur which vary with time and location. Because this expansion is not uniform, stresses appear when hot regions near the weld are restrained by cooler regions further away. Plastic deformations, occurring as a result of these stresses lead to residual stresses in the object after the temperatures have returned to ambient levels [35]. In the welding process, tensile and compressive stresses may arise along the sheet and along the weld bead. The typical distribution of both longitudinal (a) and transverse (b) residual stresses in welding joints can be shown in Figure 1 [36].
In the present work, the influence of the main welding parameters on the residual stress amplitude in the welding corner joint is analyzed. For this, an orthogonal array of tests was developed by Taguchi, L27, where it is possible to combine different levels for the defined parameters. Table 1 shows the levels used for each parameter.
Nº | Symbol | Parameters | Units | Levels | ||||
1 | 2 | 3 | ||||||
1 | A | Welding current | (A) | 163 | 181 | 202 | ||
2 | B | Welding speed | (mm/s) | 10 | 13 | 16 | ||
3 | C | Torch angle | (o) | 30º | 45º | 60º |
In this case, a L27 array was used to define the combination of parameters. The numerical simulations were carried out using SymufactWelding® software version 6.0 using a double ellipsoid model for energy distribution transferred from the heat source to the component [24].
The double ellipsoid model (Figure 2) and transversal section cord (Figure 3) were previously calibrated with experimental welding (Figure 4) which, in this, case was used a robot. The measurements are obtained from metallographic samples of welding cord as shown in Figure 5.
Having the dimensions of the experimental cords for all combinations, these values were used in the simulations. The numerical model shown in Figure 6 corresponds to the model projected in the SymufactWelding® software, where the plates to be welded, the weld bead, and the fixing devices were inserted, in the same orientation and position of the experimental tests.
Figure 6 shows the studied process model. The model configuration is specified as: two components (plates), 1 bearing, 2 clamps, 1 robot, and the arc welding process. Other specifications related to the material, welding parameters, trajectories and assembly have also been specified. As input conditions in the SymufactWelding® software, the values of voltage (V), current (A), speed (mm/s) and torch angle (º) were used, as shown in Table 2. The mesh pattern used in the simulations is shown in Figure 7.
Simulation | Current (A) | Speed (mm/s) | Torch angle (º) | Voltage (V) | MaxPS (MPa) |
1 | 163 | 10 | 30 | 22.6 | 75.4138 |
2 | 163 | 10 | 45 | 22.6 | 72.7757 |
3 | 163 | 10 | 60 | 22.6 | 62.2036 |
4 | 163 | 13 | 30 | 22.6 | 78.6806 |
5 | 163 | 13 | 45 | 22.6 | 82.6118 |
6 | 163 | 13 | 60 | 22.6 | 76.7528 |
7 | 163 | 16 | 30 | 22.6 | 94.3697 |
8 | 163 | 16 | 45 | 22.6 | 85.5548 |
9 | 163 | 16 | 60 | 22.6 | 84.5229 |
10 | 181 | 10 | 30 | 23.4 | 70.9412 |
11 | 181 | 10 | 45 | 23.4 | 73.7608 |
12 | 181 | 10 | 60 | 23.4 | 61.0019 |
13 | 181 | 13 | 30 | 23.4 | 73.8837 |
14 | 181 | 13 | 45 | 23.4 | 74.4494 |
15 | 181 | 13 | 60 | 23.4 | 71.9547 |
16 | 181 | 16 | 30 | 23.4 | 78.6379 |
17 | 181 | 16 | 45 | 23.4 | 85.487 |
18 | 181 | 16 | 60 | 23.4 | 85.7779 |
19 | 202 | 10 | 30 | 23.8 | 61.0982 |
20 | 202 | 10 | 45 | 23.8 | 62.5565 |
21 | 202 | 10 | 60 | 23.8 | 54.5618 |
22 | 202 | 13 | 30 | 23.8 | 80.4348 |
23 | 202 | 13 | 45 | 23.8 | 76.0197 |
24 | 202 | 13 | 60 | 23.8 | 73.9517 |
25 | 202 | 16 | 30 | 23.8 | 79.484 |
26 | 202 | 16 | 45 | 23.8 | 77.2585 |
27 | 202 | 16 | 60 | 23.8 | 79.3789 |
After all simulations completed, the values of the maximum principal stress (MaxPS) were withdrawn at various points along the x-axis, Figure 7.
The maximum values of the residual stresses for each simulation are presented in Table 2.
The simulation required 8 h on a virtual machine cluster (HPC) with 64 GB of RAM. The average value of the residual stresses (MaxPS) for each parameter is presented in Table 3. Based on the results is possible to determine the combination that minimizes the residual stresses. In this case, the combination that minimizes the residual stress is A3B1C1 (202 A, 10 mm/s and 30º). On the other hand, the combination that maximizes the residual stress is A1B3C3 (163 A, 16 mm/s and 60º).
Parameters | Level | MaxPS |
A | 1 | 79.21 |
2 | 75.10 | |
3 | 71.64 | |
B | 1 | 66.03 |
2 | 76.53 | |
3 | 83.39 | |
C | 1 | 72.23 |
2 | 76.72 | |
3 | 76.99 |
The goal of the analysis of variance (ANOVA) is to determine which design welding parameters affect meaningfully the residual stresses. In Table 4 it is possible to observe the ANOVA analysis for MaxPS residual stresses. Thus, for MaxPS residual stresses the most influent parameter is welding speed (65%) followed by the welding current with a contribution of 12%, and the torch angle with 6%. The possible reason for such an observation could be that with the increase in welding speed, the rate of heat input is higher than the rate of heat dissipation. This effect results in greater heating located in the melting zone and thermal incompatibility, which causes the formation of residual stresses. That is, if the heat source moves faster, there will be less time for the heat transfer to occur with the surrounding material. This effect has already been proven analytically by Zasa et al. [37].
Source | DF | SQ | MS | F-value | P-value | Contribution (%) |
Current (A) | 2 | 258.6 | 129.3 | 7.14 | 0.005 | 12% |
Welding speed (mm/s) | 2 | 1374.5 | 687.27 | 37.93 | 0 | 65% |
Torch angle (º) | 2 | 128.5 | 64.27 | 3.55 | 0.048 | 6% |
Error | 20 | 362.4 | 18.12 | |||
Total | 26 | 2124 |
Where DF is the degree of freedom, SQ is the sum of squares and MS the mean squares.
In order to validate the numerical simulations, was implemented an experimental procedure to welding a specimen using a robot (Yaskawa MA2010 with a DX200 controller) and the welding machine (Fronius TransPuls 4000 CMT R). After the welding of each specimen the residual stress was measured. As the main objective of this work is to validation the combination to minimize (simulation 19) and maximize (simulation 9) the level of residual stresses. The residual stresses were measured with the hole-drilling technique (Figure 8) using the RS-200 milling guide that is a high-precision instrument for analyzing residual stresses (Figure 9). To measure the strain relaxation resorted to a special rosette of strain gages, that was located immediately ahead the weld bead, at x axis direction, and 50 millimeters in y direction at half cord.
The residual stresses values were determined using H-Drill software. The residual stresses are determined from the strain values measured in the strain gauges (Table 5).
MaxPS (MPa) | Error | |||
Numerical | Experimental | |||
S_09 | 85 | 106 | 20% | |
S_19 | 61 | 67 | 9% |
The numerical simulations of GMAW welding process with SymufactWelding® software proved to be a useful and powerful tool for the calculation of residual stresses in the weld joints with 6082-T6 aluminum alloy.
The Taguchi method allows the determination of the combination of welding parameters to minimize and maximize the value of residual stresses. The optimal combination that minimizes the MaxPS residual stresses is A3B1C1, i.e., a welding current of 202 A, a welding speed of 10 mm/s and 30° for the torch angle. On the other hand, A1B3C3 combination was the one that maximizes MaxPS, i.e., 163 A, 16 mm/s and 60° for the welding current, welding speed and torch angle, respectively.
The ANOVA analysis was successful in identifying the influence of each parameter on the variation of residual stresses. In the case of MaxPS, the welding speed was the most influent parameter (65%) while the angle torch was the least important parameter (6%).
The numerical simulations were validated with experimental tests and were proved that the numerical results are relatively close to the experimental data, but not on the security side. The relative error in the measurements is 20% in combination 19 and 9% in combination 9.
This work has been supported by FCT-Fundação para a Ciência e Tecnologia within the R & D Units Project Scope: CIMO (UIDB/00690/2020).
The authors declare that there are no conflicts of interest.
[1] |
M. Barthélemy, A. Barrat, R. Pastor-Satorras, A. Vespignani, Dynamical patterns of epidemic outbreaks in complex heterogeneous networks, J. Theor. Biol., 235 (2005), 275–288. https://doi.org/10.1016/j.jtbi.2005.01.011 doi: 10.1016/j.jtbi.2005.01.011
![]() |
[2] |
S. Fisher-Hoch, L. Hutwagner, Opportunistic candidiasis: an epidemic of the 1980s, Clin. Infect. Dis., 21 (1995), 897–904. https://doi.org/10.1093/clinids/21.4.897 doi: 10.1093/clinids/21.4.897
![]() |
[3] |
C. Chintu, U. H. Athale, P. Patil, Childhood cancers in zambia before and after the hiv epidemic, Arch. Dis. Child., 73 (1995), 100–105. https://doi.org/10.1136/adc.73.2.100 doi: 10.1136/adc.73.2.100
![]() |
[4] |
R. M. Anderson, C. Fraser, A. C. Ghani, C. A. Donnelly, S. Riley, N. M. Ferguson, et al., Epidemiology, transmission dynamics and control of sars: the 2002–2003 epidemic, Philos. Trans. R. Soc. Lond. B. Biol. Sci., 359 (2004), 1091–1105. https://doi.org/10.1098/rstb.2004.1490 doi: 10.1098/rstb.2004.1490
![]() |
[5] |
W. Lam, N. Zhong, W. Tan, Overview on sars in asia and the world, Respirology, 8 (2003), S2–S5. https://doi.org/10.1046/j.1440-1843.2003.00516.x doi: 10.1046/j.1440-1843.2003.00516.x
![]() |
[6] |
H. Chen, G. Smith, K. Li, J. Wang, X. Fan, J. Rayner, et al., Establishment of multiple sublineages of h5n1 influenza virus in asia: implications for pandemic control, Proc. Natl. Acad. Sci., 103 (2006), 2845–2850. https://doi.org/10.1073/pnas.0511120103 doi: 10.1073/pnas.0511120103
![]() |
[7] |
A. M. Kilpatrick, A. A. Chmura, D. W. Gibbons, R. C. Fleischer, P. P. Marra, P. Daszak, Predicting the global spread of h5n1 avian influenza, Proc. Natl. Acad. Sci., 103 (2006), 19368–19373. https://doi.org/10.1073/pnas.0609227103 doi: 10.1073/pnas.0609227103
![]() |
[8] |
S. Jain, L. Kamimoto, A. M. Bramley, A. M. Schmitz, S. R. Benoit, J. Louie, et al., Hospitalized patients with 2009 h1n1 influenza in the united states, april–june 2009, N. Engl. J. Med., 361 (2009), 1935–1944. https://doi.org/10.1056/NEJMoa0906695 doi: 10.1056/NEJMoa0906695
![]() |
[9] |
M. P. Girard, J. S. Tam, O. M. Assossou, M. P. Kieny, The 2009 a (h1n1) influenza virus pandemic: A review, Vaccine, 28 (2010), 4895–4902. https://doi.org/10.1016/j.vaccine.2010.05.031 doi: 10.1016/j.vaccine.2010.05.031
![]() |
[10] |
T. R. Frieden, I. Damon, B. P. Bell, T. Kenyon, S. Nichol, Ebola 2014—new challenges, new global response and responsibility, N. Engl. J. Med., 371 (2014), 1177–1180. https://doi.org/10.1056/NEJMp1409903 doi: 10.1056/NEJMp1409903
![]() |
[11] |
W. E. R. Team, Ebola virus disease in west africa—the first 9 months of the epidemic and forward projections, N. Engl. J. Med., 371 (2014), 1481–1495. https://doi.org/10.1056/NEJMoa1411100 doi: 10.1056/NEJMoa1411100
![]() |
[12] |
W. O. Kermack, A. G. McKendrick, A contribution to the mathematical theory of epidemics, Proc. R. Soc. Lond., 115 (1927), 700–721. https://doi.org/10.1098/rspa.1927.0118 doi: 10.1098/rspa.1927.0118
![]() |
[13] |
R. Almeida, S. Qureshi, A fractional measles model having monotonic real statistical data for constant transmission rate of the disease, Fractal Fract., 3 (2019), 53. https://doi.org/10.3390/fractalfract3040053 doi: 10.3390/fractalfract3040053
![]() |
[14] |
S. Sharma, V. Volpert, M. Banerjee, Extended seiqr type model for covid-19 epidemic and data analysis, Math. Biosci. Eng., 2 (2020), 7562-7604. https://doi.org/10.3934/mbe.2020386 doi: 10.3934/mbe.2020386
![]() |
[15] | F. Brauer, P. Van den Driessche, J. Wu, L. J. Allen, Mathematical epidemiology, Springer, 2008. |
[16] |
A. d'Onofrio, M. Banerjee, P. Manfredi, Spatial behavioural responses to the spread of an infectious disease can suppress turing and turing–hopf patterning of the disease, Phys. A Stat. Mech. Appl., 545 (2020), 123773. https://doi.org/10.1016/j.physa.2019.123773 doi: 10.1016/j.physa.2019.123773
![]() |
[17] |
G. Q. Sun, Z. Jin, Q. X. Liu, L. Li, Chaos induced by breakup of waves in a spatial epidemic model with nonlinear incidence rate, J. Stat. Mech. Theory Exp., 2008 (2008), P08011. https://doi.org/10.1088/1742-5468/2008/08/P08011 doi: 10.1088/1742-5468/2008/08/P08011
![]() |
[18] |
D. Bichara, A. Iggidr, Multi-patch and multi-group epidemic models: a new framework, J. Math. Biol., 77 (2018), 107–134. https://doi.org/10.1007/s00285-017-1191-9 doi: 10.1007/s00285-017-1191-9
![]() |
[19] |
R. K. McCormack, L. J. Allen, Multi-patch deterministic and stochastic models for wildlife diseases, J. Biol. Dyn., 1 (2007), 63–85. https://doi.org/10.1080/17513750601032711 doi: 10.1080/17513750601032711
![]() |
[20] |
E. H. Elbasha, A. B. Gumel, Vaccination and herd immunity thresholds in heterogeneous populations, J. Math. Biol., 83 (2021), 73. https://doi.org/10.1007/s00285-021-01686-z doi: 10.1007/s00285-021-01686-z
![]() |
[21] |
S. Aniţa, M. Banerjee, S. Ghosh, V. Volpert, Vaccination in a two-group epidemic model, Appl. Math. Lett., 119 (2021), 107197. https://doi.org/10.1016/j.aml.2021.107197 doi: 10.1016/j.aml.2021.107197
![]() |
[22] |
T. S. Faniran, A. Ali, N. E. Al-Hazmi, J. K. K. Asamoah, T. A. Nofal, M. O. Adewole, New variant of sars-cov-2 dynamics with imperfect vaccine, Complexity, 2022 (2022). https://doi.org/10.1155/2022/1062180 doi: 10.1155/2022/1062180
![]() |
[23] |
N. Ahmed, Z. Wei, D. Baleanu, M. Rafiq, M. Rehman, Spatio-temporal numerical modeling of reaction-diffusion measles epidemic system, Chaos Interdiscip. J. Nonlinear Sci., 29 (2019), 103101. https://doi.org/10.1063/1.5116807 doi: 10.1063/1.5116807
![]() |
[24] |
J. Filipe, M. Maule, Effects of dispersal mechanisms on spatio-temporal development of epidemics, J. Theor. Biol., 226 (2004), 125–141. https://doi.org/10.1016/s0022-5193(03)00278-9 doi: 10.1016/s0022-5193(03)00278-9
![]() |
[25] | M. Martcheva, An introduction to mathematical epidemiology, Springer, 2015. |
[26] | F. Brauer, C. Castillo-Chavez, Z. Feng, Mathematical models in epidemiology, Springer, 2019. |
[27] |
H. W. Hethcote, The mathematics of infectious diseases, SIAM Review, 42 (2000), 599–653. https://doi.org/10.1137/S0036144500371907 doi: 10.1137/S0036144500371907
![]() |
[28] |
H. S. Hurd, J. B. Kaneene, The application of simulation models and systems analysis in epidemiology: A review, Prev. Vet. Med., 15 (1993), 81–99. https://doi.org/10.1016/0167-5877(93)90105-3 doi: 10.1016/0167-5877(93)90105-3
![]() |
[29] |
S. Ghosh, V. Volpert, M. Banerjee, An epidemic model with time-distributed recovery and death rates, Bull. Math. Biol., 84 (2022), 78. https://doi.org/10.1007/s11538-022-01028-0 doi: 10.1007/s11538-022-01028-0
![]() |
[30] | V. Volpert, M. Banerjee, S. Petrovskii, On a quarantine model of coronavirus infection and data analysis, preprint, arXiv: 2003.09444. |
[31] |
S. Ghosh, V. Volpert, M. Banerjee, An epidemic model with time delay determined by the disease duration, Mathematics, 10 (2022), 2561. https://doi.org/10.3390/math10152561 doi: 10.3390/math10152561
![]() |
[32] |
Q. Zheng, J. Shen, V. Pandey, L. Guan, Y. Guo, Turing instability in a network-organized epidemic model with delay, Chaos Solitons Fractals, 168 (2023), 113205. https://doi.org/10.1016/j.chaos.2023.113205 doi: 10.1016/j.chaos.2023.113205
![]() |
[33] |
K. Ciesielski, On stefan banach and some of his results, Banach J. Math. Anal., 1 (2007), 1–10. https://doi.org/10.15352/bjma/1240321550 doi: 10.15352/bjma/1240321550
![]() |
[34] |
J. Quarleri, V. Galvan, M. V. Delpino, Omicron variant of the sars-cov-2: a quest to define the consequences of its high mutational load, Geroscience, (2022), 1–4. https://doi.org/10.1007/s11357-021-00500-4 doi: 10.1007/s11357-021-00500-4
![]() |
[35] |
A. Gowrisankar, T. Priyanka, S. Banerjee, Omicron: a mysterious variant of concern, Eur. Phys. J. Plus., 137 (2022), 1–8. https://doi.org/10.1140/epjp/s13360-021-02321-y doi: 10.1140/epjp/s13360-021-02321-y
![]() |
[36] | S. Collins, E. Starkman, Coronavirus incubation period, 2022. Available from: https://www.webmd.com/covid/coronavirus-incubation-period. |
[37] | J. Ries, Omicron infection timeline: When symptoms start and how long they last, 2022. Available from: https://www.health.com/news/omicron-timeline. |
[38] | COVID-19 Coronavirus Pandemic, 2023. Available from: https://www.worldometers.info/coronavirus/. |
[39] |
S. Ghosh, M. Banerjee, V. Volpert, Immuno-epidemiological model-based prediction of further covid-19 epidemic outbreaks due to immunity waning, Math. Model. Nat. Phenom., 17 (2022), 9. https://doi.org/10.1051/mmnp/2022017 doi: 10.1051/mmnp/2022017
![]() |
1. | Glauco Nobrega, Maria Sabrina Souza, Manuel Rodríguez-Martín, Pablo Rodríguez-Gonzálvez, João Ribeiro, Parametric Optimization of the GMAW Welding Process in Thin Thickness of Austenitic Stainless Steel by Taguchi Method, 2021, 11, 2076-3417, 8742, 10.3390/app11188742 | |
2. | Rebai Billel, Contribution to study the effect of (Reuss, LRVE, Tamura) models on the axial and shear stress of sandwich FGM plate (Ti–6A1–4V/ZrO2) subjected on linear and nonlinear thermal loads, 2023, 10, 2372-0484, 26, 10.3934/matersci.2023002 | |
3. | Lucas Martins Garcia, Verônica Teixeira Noronha, João Ribeiro, Effect of Welding Orientation in Angular Distortion in Multipass GMAW, 2021, 5, 2504-4494, 63, 10.3390/jmmp5020063 | |
4. | Qiang Wang, Zhandong Wan, Tianyi Zhao, Yue Zhao, Dongyang Yan, Guoqing Wang, Aiping Wu, Tensile properties of TIG welded 2219-T8 aluminum alloy joints in consideration of residual stress releasing and specimen size, 2022, 18, 22387854, 1502, 10.1016/j.jmrt.2022.03.059 | |
5. | Inês S. Afonso, Manuel Rodríguez Martín, João E. Ribeiro, Effect of Welding Sequence in Angular Distortion on Butt Joint GMAW Process, 2022, 12, 2076-3417, 10402, 10.3390/app122010402 | |
6. | V. V. Kvasnytskyi, A. O. Perepichai, O. V. Prokhorenko, O. A. Slyvinskyi, I. M. Lagodzinskyi, Numerical Simulation of the Stress-Strain State of a PAW-MIG-Welded 1561 Aluminum Alloy Butt Joint Produced Under Different Process Modes, 2024, 56, 0039-2316, 258, 10.1007/s11223-024-00645-5 | |
7. | Yang Hu, Weichi Pei, Hongchao Ji, Rongdi Yu, Shengqiang Liu, Tungsten Inert Gas Welding of 6061-T6 Aluminum Alloy Frame: Finite Element Simulation and Experiment, 2024, 17, 1996-1944, 1039, 10.3390/ma17051039 | |
8. | JiaYuan Luo, Chao Tan, Xin Li, Yuhang Tang, Influence of welding residual stress and welding method on fracture properties of welded joints, 2024, 238, 0954-4062, 1629, 10.1177/09544062231187208 |
Nº | Symbol | Parameters | Units | Levels | ||||
1 | 2 | 3 | ||||||
1 | A | Welding current | (A) | 163 | 181 | 202 | ||
2 | B | Welding speed | (mm/s) | 10 | 13 | 16 | ||
3 | C | Torch angle | (o) | 30º | 45º | 60º |
Simulation | Current (A) | Speed (mm/s) | Torch angle (º) | Voltage (V) | MaxPS (MPa) |
1 | 163 | 10 | 30 | 22.6 | 75.4138 |
2 | 163 | 10 | 45 | 22.6 | 72.7757 |
3 | 163 | 10 | 60 | 22.6 | 62.2036 |
4 | 163 | 13 | 30 | 22.6 | 78.6806 |
5 | 163 | 13 | 45 | 22.6 | 82.6118 |
6 | 163 | 13 | 60 | 22.6 | 76.7528 |
7 | 163 | 16 | 30 | 22.6 | 94.3697 |
8 | 163 | 16 | 45 | 22.6 | 85.5548 |
9 | 163 | 16 | 60 | 22.6 | 84.5229 |
10 | 181 | 10 | 30 | 23.4 | 70.9412 |
11 | 181 | 10 | 45 | 23.4 | 73.7608 |
12 | 181 | 10 | 60 | 23.4 | 61.0019 |
13 | 181 | 13 | 30 | 23.4 | 73.8837 |
14 | 181 | 13 | 45 | 23.4 | 74.4494 |
15 | 181 | 13 | 60 | 23.4 | 71.9547 |
16 | 181 | 16 | 30 | 23.4 | 78.6379 |
17 | 181 | 16 | 45 | 23.4 | 85.487 |
18 | 181 | 16 | 60 | 23.4 | 85.7779 |
19 | 202 | 10 | 30 | 23.8 | 61.0982 |
20 | 202 | 10 | 45 | 23.8 | 62.5565 |
21 | 202 | 10 | 60 | 23.8 | 54.5618 |
22 | 202 | 13 | 30 | 23.8 | 80.4348 |
23 | 202 | 13 | 45 | 23.8 | 76.0197 |
24 | 202 | 13 | 60 | 23.8 | 73.9517 |
25 | 202 | 16 | 30 | 23.8 | 79.484 |
26 | 202 | 16 | 45 | 23.8 | 77.2585 |
27 | 202 | 16 | 60 | 23.8 | 79.3789 |
Parameters | Level | MaxPS |
A | 1 | 79.21 |
2 | 75.10 | |
3 | 71.64 | |
B | 1 | 66.03 |
2 | 76.53 | |
3 | 83.39 | |
C | 1 | 72.23 |
2 | 76.72 | |
3 | 76.99 |
Source | DF | SQ | MS | F-value | P-value | Contribution (%) |
Current (A) | 2 | 258.6 | 129.3 | 7.14 | 0.005 | 12% |
Welding speed (mm/s) | 2 | 1374.5 | 687.27 | 37.93 | 0 | 65% |
Torch angle (º) | 2 | 128.5 | 64.27 | 3.55 | 0.048 | 6% |
Error | 20 | 362.4 | 18.12 | |||
Total | 26 | 2124 |
MaxPS (MPa) | Error | |||
Numerical | Experimental | |||
S_09 | 85 | 106 | 20% | |
S_19 | 61 | 67 | 9% |
Nº | Symbol | Parameters | Units | Levels | ||||
1 | 2 | 3 | ||||||
1 | A | Welding current | (A) | 163 | 181 | 202 | ||
2 | B | Welding speed | (mm/s) | 10 | 13 | 16 | ||
3 | C | Torch angle | (o) | 30º | 45º | 60º |
Simulation | Current (A) | Speed (mm/s) | Torch angle (º) | Voltage (V) | MaxPS (MPa) |
1 | 163 | 10 | 30 | 22.6 | 75.4138 |
2 | 163 | 10 | 45 | 22.6 | 72.7757 |
3 | 163 | 10 | 60 | 22.6 | 62.2036 |
4 | 163 | 13 | 30 | 22.6 | 78.6806 |
5 | 163 | 13 | 45 | 22.6 | 82.6118 |
6 | 163 | 13 | 60 | 22.6 | 76.7528 |
7 | 163 | 16 | 30 | 22.6 | 94.3697 |
8 | 163 | 16 | 45 | 22.6 | 85.5548 |
9 | 163 | 16 | 60 | 22.6 | 84.5229 |
10 | 181 | 10 | 30 | 23.4 | 70.9412 |
11 | 181 | 10 | 45 | 23.4 | 73.7608 |
12 | 181 | 10 | 60 | 23.4 | 61.0019 |
13 | 181 | 13 | 30 | 23.4 | 73.8837 |
14 | 181 | 13 | 45 | 23.4 | 74.4494 |
15 | 181 | 13 | 60 | 23.4 | 71.9547 |
16 | 181 | 16 | 30 | 23.4 | 78.6379 |
17 | 181 | 16 | 45 | 23.4 | 85.487 |
18 | 181 | 16 | 60 | 23.4 | 85.7779 |
19 | 202 | 10 | 30 | 23.8 | 61.0982 |
20 | 202 | 10 | 45 | 23.8 | 62.5565 |
21 | 202 | 10 | 60 | 23.8 | 54.5618 |
22 | 202 | 13 | 30 | 23.8 | 80.4348 |
23 | 202 | 13 | 45 | 23.8 | 76.0197 |
24 | 202 | 13 | 60 | 23.8 | 73.9517 |
25 | 202 | 16 | 30 | 23.8 | 79.484 |
26 | 202 | 16 | 45 | 23.8 | 77.2585 |
27 | 202 | 16 | 60 | 23.8 | 79.3789 |
Parameters | Level | MaxPS |
A | 1 | 79.21 |
2 | 75.10 | |
3 | 71.64 | |
B | 1 | 66.03 |
2 | 76.53 | |
3 | 83.39 | |
C | 1 | 72.23 |
2 | 76.72 | |
3 | 76.99 |
Source | DF | SQ | MS | F-value | P-value | Contribution (%) |
Current (A) | 2 | 258.6 | 129.3 | 7.14 | 0.005 | 12% |
Welding speed (mm/s) | 2 | 1374.5 | 687.27 | 37.93 | 0 | 65% |
Torch angle (º) | 2 | 128.5 | 64.27 | 3.55 | 0.048 | 6% |
Error | 20 | 362.4 | 18.12 | |||
Total | 26 | 2124 |
MaxPS (MPa) | Error | |||
Numerical | Experimental | |||
S_09 | 85 | 106 | 20% | |
S_19 | 61 | 67 | 9% |