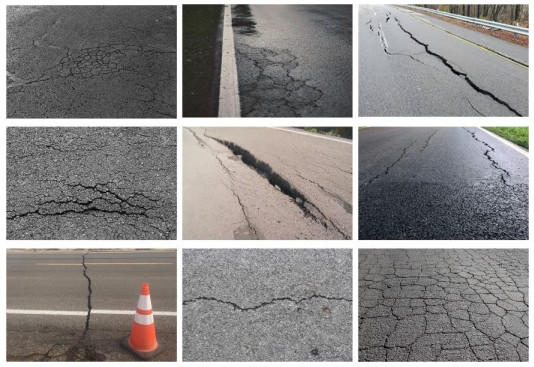
This paper proposed an end-to-end road crack segmentation model based on attention mechanism and deep FCN with generative adversarial learning. We create a segmentation network by introducing a visual attention mechanism and residual module to a fully convolutional network(FCN) to capture richer local features and more global semantic features and get a better segment result. Besides, we use an adversarial network consisting of convolutional layers as a discrimination network. The main contributions of this work are as follows: 1) We introduce a CNN model as a discriminate network to realize adversarial learning to guide the training of the segmentation network, which is trained in a min-max way: the discrimination network is trained by maximizing the loss function, while the segmentation network is trained with the only gradient passed by the discrimination network and aim at minimizing the loss function, and finally an optimal segmentation network is obtained; 2) We add the residual modular and the visual attention mechanism to U-Net, which makes the segmentation results more robust, refined and smooth; 3) Extensive experiments are conducted on three public road crack datasets to evaluate the performance of our proposed model. Qualitative and quantitative comparisons between the proposed method and the state-of-the-art methods show that the proposed method outperforms or is comparable to the state-of-the-art methods in both F1 score and precision. In particular, compared with U-Net, the mIoU of our proposed method is increased about 3%~17% compared with the three public datasets.
Citation: Xing Hu, Minghui Yao, Dawei Zhang. Road crack segmentation using an attention residual U-Net with generative adversarial learning[J]. Mathematical Biosciences and Engineering, 2021, 18(6): 9669-9684. doi: 10.3934/mbe.2021473
[1] | Dongwei Liu, Ning Sheng, Tao He, Wei Wang, Jianxia Zhang, Jianxin Zhang . SGEResU-Net for brain tumor segmentation. Mathematical Biosciences and Engineering, 2022, 19(6): 5576-5590. doi: 10.3934/mbe.2022261 |
[2] | Yuqing Zhang, Yutong Han, Jianxin Zhang . MAU-Net: Mixed attention U-Net for MRI brain tumor segmentation. Mathematical Biosciences and Engineering, 2023, 20(12): 20510-20527. doi: 10.3934/mbe.2023907 |
[3] | Hui Yao, Yuhan Wu, Shuo Liu, Yanhao Liu, Hua Xie . A pavement crack synthesis method based on conditional generative adversarial networks. Mathematical Biosciences and Engineering, 2024, 21(1): 903-923. doi: 10.3934/mbe.2024038 |
[4] | Jiajun Zhu, Rui Zhang, Haifei Zhang . An MRI brain tumor segmentation method based on improved U-Net. Mathematical Biosciences and Engineering, 2024, 21(1): 778-791. doi: 10.3934/mbe.2024033 |
[5] | Xiaoli Zhang, Kunmeng Liu, Kuixing Zhang, Xiang Li, Zhaocai Sun, Benzheng Wei . SAMS-Net: Fusion of attention mechanism and multi-scale features network for tumor infiltrating lymphocytes segmentation. Mathematical Biosciences and Engineering, 2023, 20(2): 2964-2979. doi: 10.3934/mbe.2023140 |
[6] | Tong Shan, Jiayong Yan, Xiaoyao Cui, Lijian Xie . DSCA-Net: A depthwise separable convolutional neural network with attention mechanism for medical image segmentation. Mathematical Biosciences and Engineering, 2023, 20(1): 365-382. doi: 10.3934/mbe.2023017 |
[7] | Shuaiyu Bu, Yuanyuan Li, Wenting Ren, Guoqiang Liu . ARU-DGAN: A dual generative adversarial network based on attention residual U-Net for magneto-acousto-electrical image denoising. Mathematical Biosciences and Engineering, 2023, 20(11): 19661-19685. doi: 10.3934/mbe.2023871 |
[8] | Jun Liu, Zhenhua Yan, Chaochao Zhou, Liren Shao, Yuanyuan Han, Yusheng Song . mfeeU-Net: A multi-scale feature extraction and enhancement U-Net for automatic liver segmentation from CT Images. Mathematical Biosciences and Engineering, 2023, 20(5): 7784-7801. doi: 10.3934/mbe.2023336 |
[9] | Xiaoyan Zhang, Mengmeng He, Hongan Li . DAU-Net: A medical image segmentation network combining the Hadamard product and dual scale attention gate. Mathematical Biosciences and Engineering, 2024, 21(2): 2753-2767. doi: 10.3934/mbe.2024122 |
[10] | Ning Sheng, Dongwei Liu, Jianxia Zhang, Chao Che, Jianxin Zhang . Second-order ResU-Net for automatic MRI brain tumor segmentation. Mathematical Biosciences and Engineering, 2021, 18(5): 4943-4960. doi: 10.3934/mbe.2021251 |
This paper proposed an end-to-end road crack segmentation model based on attention mechanism and deep FCN with generative adversarial learning. We create a segmentation network by introducing a visual attention mechanism and residual module to a fully convolutional network(FCN) to capture richer local features and more global semantic features and get a better segment result. Besides, we use an adversarial network consisting of convolutional layers as a discrimination network. The main contributions of this work are as follows: 1) We introduce a CNN model as a discriminate network to realize adversarial learning to guide the training of the segmentation network, which is trained in a min-max way: the discrimination network is trained by maximizing the loss function, while the segmentation network is trained with the only gradient passed by the discrimination network and aim at minimizing the loss function, and finally an optimal segmentation network is obtained; 2) We add the residual modular and the visual attention mechanism to U-Net, which makes the segmentation results more robust, refined and smooth; 3) Extensive experiments are conducted on three public road crack datasets to evaluate the performance of our proposed model. Qualitative and quantitative comparisons between the proposed method and the state-of-the-art methods show that the proposed method outperforms or is comparable to the state-of-the-art methods in both F1 score and precision. In particular, compared with U-Net, the mIoU of our proposed method is increased about 3%~17% compared with the three public datasets.
It is well known that the improvement of road facilities can help the economic growth and provide convenience for people's travel. However, the service life of the road is limited, and various road diseases will appear as time goes by due to the causes of nature and vehicle crushing. If the road diseases cannot be repaired in time, the degree of damage and the potential risk of traffic accidents will inevitably increase.
As one of the most common road diseases, road crack detection is essential for road maintenance. Figure 1 shows some examples of road crack. In the past, the task is mainly relied on maintenance workers to inspect the road surface. However, the manual detection is low efficiency, has a high labor cost, and tends to miss some non-obvious road cracks. With the rapid development of computer vision and artificial intelligence, the traditional manual way has been gradually alternated by automatic road crack detection. Compared with the rough location detection of road crack, pixel-level road crack segmentation can further evaluate the degree of road damage and help formulate an accurate maintenance plan.
Compared with the crack detection at the region level, segmenting road crack at the pixel level is more valuable to analyze the damage degree of road surface and help to make a more reasonable maintenance scheme. However, accurately segmenting the road crack at the pixel level is not trivial due to the complexity and diversity of road cracks, such as slender shapes, heavy noises, discontinuous edges, complex backgrounds, and various scales. This paper proposes a road crack segmentation method based on attention-based deep FCN with adversarial training. First, we use FCN as a segmentation network and add visual attention mechanism and residual structure to the segmentation network. Then we introduce a CNN as the discrimination network to guide the training of the segmentation network. The discrimination network is trained with two inputs: the original image masked by the predicted image generated from the segmentation network and the original image masked by the groundtruth. The segmentation network and the discrimination network are trained alternatively. The discrimination network is trained to maximize the loss resulting from the CNN feature differences between the segmented image and the groundtruth, while the segmentation network is trained with gradients passed by the discrimination network to minimize the loss function. The capability of both the segmentation network and discrimination network has improved alternatively after the adversarial training process. When the discrimination network fails to identify the inputted original image is masked by the predicted mask or groundtruth, i.e., the Nash equilibrium is achieved, the optimal segmentation network is obtained. The overall network structure of this article is shown in Figure 2.
The main contributions of this paper are summarized as follows:
1) Inspired by adversarial training, we use six layers of CNN as a discriminate network and use the same loss function to ensure that a discriminate network only passes the segmentation network's gradient. And finally, the model of segmentation can be most optimal and accurately segment the road crack in different scales and shapes and complex road conditions;
2) We add more convolutional layers to extract more features based on a fully convolutional neural network. Meanwhile, with the help of an attention mechanism, our model can capture richer features and get more refined, smooth, and accurate pixel-level segmentation results;
3) Our proposed model is trained on whole images with 128 × 128 image resolution and gets a satisfactory result in a relatively short training time. We analyze the experimental results on three public datasets qualitatively and quantitatively to demonstrate the effectiveness of the proposed method.
The rest of this paper is organized as follows: In the second section, we briefly review the related work of crack detection; In the third section, we provide the details of the proposed model; In the fourth section, we present and discuss the experimental result on three public datasets; In the last section, we conclude this paper.
The crack segmentation methods can be categorized as the traditional method and the deep-learning-based method. In recent years, deep learning technology has been applied in image segmentation. Since it can automatically extract useful features at multiple scales and significantly improve performance, deep learning-based methods have become the mainstream for crack segmentation.
The early crack method mainly relied on thresholding [1,2] that has low robustness. To overcome this problem, some research combined gray values [3], the standard deviation of neighboring pixels [4] to avoid the influence of noise. Besides, some researchers proposed Minimal Path Selection (MPS) [5,6], Minimum Spanning Tree (MST) [7,8], Crack Fundamental Element (CFE) [9,10] to enhance the continuity of crack. The minimum path-based method is to find the shortest path length between two specific nodes and to extract the structure similar to the curve in the image. Chen et al. [11] used the shortest path for crack detection but a high error detection rate. Although considerable efforts had been made, the pixel threshold-based methods are still difficult to get satisfied segmentation results of complex cracks with bad road conditions. The texture analysis-based method [12,13] firstly captures the gray-scale spatial distributions to characterize the texture pattern in the image and then uses texture patterns to predict if the pixel belongs to a crack or normal road surface. However, this method cannot capture local information and cannot well segment the irregular cracks. The wavelet transform method [14,15] assumed that a crack in structure would change the structure's natural frequencies and vibration, so it can be used for detecting the crack location and depth. Although the wavelet-based method can avoid the influence of noise in the image, it cannot work well for discontinuous cracks. Another traditional method is saliency detection [16], which aims to identify image salient areas by fusing multi-scale image features. Wei et al. [17] used saliency detection to detect road cracks, but it was difficult to obtain a complete and continuous crack.
In recent years, the deep learning method has been applied in road image segmentation and has become mainstream image processing. The deep learning method can automatically extract target features at multiple scales and significantly improve performance compared to the traditional image process methods. Dan et al. [18] firstly proposed a Convolutional Neural Network (CNN)-based method for semantic segmentation, which uses the sliding window to identify each crack pixel concerning its neighboring pixels around them. However, if there are errors in initial labels, it may have poor predictions and high computation costs. Cha et al. [19] proposed a crack segmentation method that used a deep CNN combined with sliding windows for the cracks with different scales. The method is robust to noise and can work well for complex road conditions. Then Cha et al. [20] proposed a crack segmentation method by combining Regional Proposal Network (RPN) and Faster Regional CNN (R-CNN), in which the RPN network is used for target extraction, and the Faster R-CNN is used to locate the extracted target. Liu et al. [21] proposed an end-to-end deep hierarchical CNN to segment the road crack, consisting of a fully connected neural network and a deep supervision network. Long et al. [22] proposed the Fully Connected Network (FCN) by replacing the full connection layer in CNN with a convolutional layer. As a result, both efficiency and accuracy of pixel-level segmentation are simultaneously improved a lot. Islam et al. [23] proposed an FCN-based crack detection method that used an encoder for feature extraction and a decoder for pixel-level classification. FCN showed that different stages of convolutional layers, but the coarse feature maps of the top layer are not enough to obtain the refined segmentation result. Based on the FCN model, many types of segmentation networks were proposed for medical image segmentation. In recent years, the U-Net network has been widely used in the field of medical image segmentation. Ronneberger et al. [24] firstly proposed U-Net and applied it to medical image segmentation. With data augmentation and appropriate loss function, the U-Net can realize end-to-end training and get a good prediction with fewer train images. Oktay et al. [25] proposed a model for medical image segmentation based on U-Net by combining with an attention mechanism, significantly improving segmentation accuracy. Inspired by the successful application of U-Net in medical image segmentation, Liu et al. [26] firstly used the U-Net to detect concrete cracks. The trained model can accurately identify the cracks in images. Compared with FCNs, it can obtain better results but with fewer training sets. Badrinarayanan et al. [27] proposed SegNet consisted of an encoding network and a decoding network. The multi-scale deep architecture was developed by using pooling indices for up-sampling and finally realized pixel-level classification. Zou et al.[28] proposed DeepCrack model based on SegNet can capture the line structures through an end-to-end trainable deep convolutional neural network. With larger-scale feature maps and more holistic representations, the model can detect more detail of crack. Liu et al.[29] proposed DeepCrack based on FCN and used DSN to supervise features of each convolution layer. And it also refines the prediction results by using guided filtering and Conditional Random Fields(CRFs). The residual network [30,31] can help solve gradient disappearance and gradient explosion in deep neural networks. Huyan et al.[32] proposed CrackU-Net, which achieved pixel-level crack detection through convolution, pooling, transpose convolution, and concatenation operations. This model was based on U-Net and did not change the structure too much. What the difference is that a transposed convolution layer was introduced into CrackU-Net. Fan et al.[33] proposed an ensemble of convolutional neural networks based on probability fusion for automated pavement crack detection and measurement. The network can identify the structure of small cracks with raw images. Song et al.[34] established a multi-scale dilated convolution module and introduced an attention module to refine the features further. These researches demonstrate that the attention mechanism is useful for extracting image features. But there is still plenty of room for improvement of precision and F1-score. The Generative Adversarial Network (GAN) was first proposed by Goodfellow et al. [35], and it has been applied for medical image segmentation [36–39]. Gao et al.[40] proposed a GAN-based method for segmenting crack of concrete pavement, which combines segmentation network CU-Net and FU-Net with GAN. Many types of research of GAN indicate that combining the segmentation network with the GAN principle can improve the accuracy and robustness of the segmentation network.
The segmentation network structure is illustrated in Figure 3. The segmentation network is a fully convolutional encoder-decoder structure that uses 6-layer convolution to extract image features. A multi-scale skip-connection structure is used in up-sampling. The input image size is adjusted to 128×128×3, and the encoder uses convolutional layers with a convolution kernel size of 7, 5, 4, respectively, and stride 2 to perform down-sampling to extract image features. The decoder uses global convolution with a convolution kernel size of 3, 7, 9, 11, respectively, and stride 1. At the same time, a residual convolution module is added after each convolution layer with kernel sizes 1, 3, 1, respectively. The channels of each convolutional layer in the encoder are 64,128,256,512, 1024, and 2048, respectively. Based on FCN, a visual attention mechanism is added in the segmentation network's upsampling to preserve more image details, while the residual structure is added after each convolution layer to make the network deeper to get more features.
Attention mechanism was firstly proposed by Bahdanau et al. [41] for machine translation. In recent years, it has been applied in computer vision and Natural Language Processing (NLP), similar to the visual attention that humans only pay attention to the part they are interested in of the image. Adding the attention mechanism into the deep neural network can make the network pay more attention to the current target information, and the influence of irrelevant information appears insignificant.
The attention mechanism can be expressed in the following form:
A=N(X) | (1) |
FA=A⊗F | (2) |
where X refers to the input, N(X) refers to the output of attention network denoted as A; F refers to the feature matrix obtained by the input X through the convolutional neural network; ⊗ denotes matrix concatenation operation on A and F; FA is the feature matrix result from A⊗F. The diagram of the attention mechanism is illustrated in Figure 4.
The residual module can deepen the network to capture richer feature information and avoid the network's degradation problem as the layers increase. The residual structure is shown in Figure 5, which consists of three layers of convolution with convolution kernel sizes of 1, 3, and 1, respectively, using the Leaky Relu activation function after each layer of convolution.
Our proposed method can capture richer local features and more global semantic features by adding the above modules.
The Generative Adversarial Network (GAN) is composed of a generator and a discrimination network. The principle of GAN is that: the generator generates an image as close as possible to the real image, while the discrimination network discriminates whether the input is real or fake. The adversarial training between the generator and discrimination network can continuously enhance their abilities until Nash equilibrium. The GANs' objective loss function is defined as follows:
minθGmaxθDL(θG,θD)=Ex∼Pdata[logD(x)]+Ez∼Pz[log(1−D(G(z)))] | (3) |
where, θG and θD represent the parameters for the generator and discrimination network, respectively. x is a real image from an unknown distribution Pdata, and z is a random input for the generator G, drawn from a probability distribution Pz. The objective of GANs is to minimize the generator's loss function and maximize the discrimination network's loss function. The former makes the generator generate the predicted label as close as the groundtruth and later makes the discrimination network cannot accurately distinguish the input is predicted label or groundtruth.
Adversarial training is proposed by Goodfellow et al.[42]. By using adversarial training not only can it improve the robustness of the model but also can improve the ability of generalization capability. In a word, adversarial training is used adversarial samples, which is produced by adding a noise radv to the original input to the trained model compared with the original input. The model can be expressed as follows:
minθ−logP(y∣x+radv;θ) | (4) |
where, y is the label, θ is the model parameters. The theory of adversarial training is further elaborated by Madry et al. [43]. To optimize the adversarial training theory, Madry proposed a new formula which is called Min-Max. The Min-Max is defined as follows:
minθE(x,y)∼D[maxradv∈SL(θ,x+radv,y)] | (5) |
where L is the loss function, S is the range of values of radv.
As the formula shows, the Min-Max has two parts: the max is called 'attack', which is to find disturbance radv and maximize the loss, and the min is called 'defense' which minimizes the outer loss and gets model parameters with the highest robustness.
To guide the training of the segmentation network, we formulate an adversarial network Inspired by Min-Max. The network includes six convolutional layers with a kernel size of 3, 7, 9, 11, respectively. The inputs of the discrimination network are the label image and the predicted image segmented by the segmentation network. The discrimination network is trained to maximize the loss and pass gradients to the segmentation network when the segmentation network is trained to minimize the loss. The structure of the discrimination network is illustrated in Figure 6.
The discrimination network has two inputs: the original image masked by the predicted image generated from the segmentation network and the original image masked by the groundtruth. The loss function of the discrimination network is defined as follows:
minθSmaxθDL(θS,θD)=1NN∑n=1lmae(fD(xn∘S(xn)),fD(xn∘yn)) | (6) |
where, lmae refers to Mean Absolute Error (MAE), xn denotes the input image, yn denotes groundtruth, and S(xn) denotes the output prediction map of the input image from the segmentation network, xn∘S(xn)refers to pixel-level multiplication of origin image and predicted image, and xn∘yn refers to pixel-level multiplication of origin image and groundtruth. What's more, The lmae is formulated as:
lmae(fD(x),fD(x'))=1LL∑i=1|fiD(x)−fiD(x')| | (7) |
where, L denotes the number of discrimination network layers, and fiD(x) denotes the feature map of image x at layer i of the discrimination network. The pseudo algorithm of the proposed model for crack segmentation is provided as follows:
Algorithm: Road crack segmentation with generative adversarial learning. |
1: Input:Original image 2: Output: Predicted image 3: for the number of iterations do 4: for k steps do 5: Predicted image S(xn) from Segmentation network 6: Original image xn from training dataset 7: Label image yn from training dataset 8: Compute the Mean Absolute Error lmae 9: Update the discrimination network by ascending its stochastic gradient 10: end for 11: Update the Segmentation network by ascending its stochastic gradient 12: end forTraining of Segmentation network aim at getting the smallest value of the loss, training of discrimination network aim at getting the biggest value of the loss |
We evaluate the performance of our method on three public datasets: Crack Forest Dataset(CFD) [44], GAPs284, and CRACK500, respectively. CFD includes 118 road crack images with 480×320 resolution; The GAPs284 includes 509 different resolutions road crack images. The CRACK500 dataset includes 1896 road crack images with 648×484 resolution. All datasets provide the groundtruth for each image. Some examples of these three datasets are illustrated in Figure 7. All images for training, evaluation, and testing are uniformly resized to the size of 128 × 128. The proposed model is trained and evaluated on the above three separate datasets. All three data sets are divided into training and validation sets in a 7:3 ratio.
The experimental environment is Intel(R) Core(TM) i5-9400F CPU, 6GB memory, Geforce GTX1660S GPU, Windows 10 operating system, program based on Pytorch. During the experiment, the epoch is set to 300; batch size is set to 8; shuffle is set to True; the initial learning rate is set to 0.0002 reduced by the decay rate 0.5 after every 50 epochs until the learning rate is 0.00000001; Adam optimization algorithm betas is set as (0.5, 0.999).
The commonly used criteria, i.e., Precision, Recall, F1 Score, mIoU (Mean Intersection over Union), are used for evaluation and comparison. The Precision and Recall are computed as follows:
Precision=TPTP+FP | (8) |
Recall=TPTP+FN | (9) |
where TP, FN, and FP refer to True Positive, False Negative, and False Positive, respectively.
F1-Score is a criterion used in statistics to measure the accuracy of the binary classification model, which is calculated as a weighted average of precision and recall and is defined as:
F1=2×P×RP+R=2×TP2×TP+FN+FP | (10) |
where P and R refer to Precision and Recall, respectively.
mIoU is a common criterion for semantic segmentation evaluation, aiming to calculate the intersection ratio between true and predicted labels. mIoU is computed as follows:
mIoU=1k+1k∑i=0TPFN+FP+TP | (11) |
where k refers to the number of samples.
The experimental results of our proposed network on the CFD, GAPs284, and CRACK500 public datasets are shown in Figure 8.
As is shown in the above images, the predicted results on CFD have smooth and consistent cracks. But when the crack is too complex, like the last image that has too many horizontal and vertical interlaced shapes, the model can detect the main crack, but the prediction is not as detailed enough as the label image. When the model is tested on GAPs284, it can segment the insignificant crack which is not labeled in the groundtruth, as is shown in the first image. The predicted images on CRACK500 also show that the model can produce the segmentation results which look better than the groundtruth. The above experience results demonstrate that the model has a good ability to segment road crack images.
To demonstrate the effectiveness of our method for pixel-level crack segmentation, we compare the experimental results with other state-of-the-art methods under the criterion of Precision, Recall, and F1 Score, and the quantitative results are listed in Table 1.
Model | CFD | GAPs284 | CRACK500 | ||||||
Precision | Recall | F1-score | Precision | Recall | F1-score | Precision | Recall | F1-score | |
Nguyen et al. [45] | 0.8567 | 0.9132 | 0.8745 | - | - | - | 0.6954 | 0.6744 | 0.6895 |
David et al. [46] | 0.8517 | 0.9155 | 0.8727 | - | - | - | 0.6811 | 0.6629 | 0.6788 |
Weng et al. [47] | 0.8682 | 0.8873 | 0.8776 | 0.6980 | 0.7055 | 0.7022 | 0.7565 | 0.7871 | 0.7715 |
Proposed-method | 0.8746 | 0.8955 | 0.8849 | 0.7720 | 0.7542 | 0.7630 | 0.9653 | 0.8197 | 0.8865 |
The quantitative comparisons demonstrate that the accuracy of our method outperforms or is comparable to the state-of-the-art methods. For example, the performance of our method on the CRACK500 dataset got the best result than other methods.
To demonstrate the effect of the attention mechanism, we compare the performance of the proposed network with and without the attention module in Table 2.
Dataset | CFD | GAPs284 | CRACK500 | |||
Proposed method | With attention | Without attention | With attention | Without attention | With attention | Without attention |
Precision | 0.8746 | 0.6849 | 0.7720 | 0.7205 | 0.9653 | 0.9063 |
Recall | 0.8955 | 0.8427 | 0.7542 | 0.7399 | 0.8197 | 0.8104 |
F1-score | 0.8849 | 0.7557 | 0.7630 | 0.7300 | 0.8865 | 0.8557 |
mIoU | 0.6754 | 0.6073 | 0.6619 | 0.6395 | 0.8296 | 0.7478 |
The quantitative comparisons demonstrate that the accuracy of our method outperforms or is comparable to the state-of-the-art methods. Compared with the network without the attention module, the improvement is noticeable: the mIoU of three datasets is increased by about 7%, 3%, and 17%, respectively.
To demonstrate the effect of generative adversarial guided training in the proposed method, we use our proposed method, U-Net and Attention-based U-Net, to conduct comparative experiments on three public datasets CFD, GAPs284, and CRACK500, respectively, under the same experimental environment and settings. We use mIoU and F1 scores as experimental evaluation criteria. As is shown in Table 3, it is obvious that generative adversarial guided learning can improve the accuracy compared with a single segmentation network. The comparative experiments prove that generative adversarial learning plays a significant role in improving the accuracy of road crack segmentation.
Method | Evaluation Criteria | CFD | GAPs284 | CRACK500 |
Proposed method | mIoU | 0.6754 | 0.6619 | 0.8296 |
F1-scre | 0.8849 | 0.7630 | 0.8865 | |
U-Net | mIoU | 0.5253 | 0.1766 | 0.7543 |
F1-scre | 0.6698 | 0.6789 | 0.6058 | |
Attention U-Net | mIoU | 0.4549 | 0.2519 | 0.5244 |
F1-scre | 0.6094 | 0.3833 | 0.6527 |
Qualitative and quantitative comparisons of experimental results demonstrated that the proposed method achieves good performances on different datasets. The reasons are that: 1) we perform the crack segmentation under the guidance of generative adversarial learning framework, the adversarial mechanism makes us can obtain an optimal segmentation network even if the number of training samples is relatively small; 2) we combine the residual modular and attention mechanism in the segmentation network, which can capture richer information, preserve more detail of crack and obtain refined segmentation results. Although the groundtruth of the crack is discontinuous and rough, the segmentation results are still robust, continuous, and smooth, which is close to the crack in reality. Although the experimental results demonstrate that the generative adversarial learning framework and the attention mechanism positively affect crack segmentation, the segmentation result may miss some crack details if the road crack pattern is highly complicated.
Road crack detection plays a significant role in road maintenance and is a challenge in computer vision due to the complexity and diversity of crack and the condition of the road. This paper tackled the challenge problem of pixel-level road crack segmentation by proposing attention residual U-Net with generative adversarial guided learning. The segmentation network can capture richer and important information by adding the residual modular and attention mechanism. Under the generative adversarial learning framework, the optimal segmentation network can be obtained and can achieve high performance. We verified the performance of this model on three public road crack data sets, and our method outperforms or is comparable to the state-of-the-art methods. Experimental results show that the proposed model can effectively and accurately achieve high-quality crack segmentation by improving the segmentation network through adversarial training.
The network proposed in this paper has achieved idea results for crack detection, but further research work is needed in the following aspects: The crack width is not measured in this paper. Future research work will focus on measuring and evaluating road damage ratings. This paper only performs crack detection on static images, but future research will realize real-time video crack detection.
This work is supported by the national key research and development program (2019YFB1705702).
The authors declared that they have no conflicts of interest in this work.
[1] | Li. Q, Liu. X, Novel approach to pavement image segmentation based on neighboring difference histogram method, in 2008 Congress on Image and Signal Processing, IEEE, (2008), 792–796. |
[2] | M. S. Kaseko, S. G. Ritchie, A neural network-based methodology for pavement crack detection and classification, Transport. Res. C.-Emer., 1 (1993), 275–291. |
[3] | M. Gavilán, D. Balcones, O. Marcos, Adaptive road crack detection system by pavement classification, Sensors, 11 (2011), 9628–9657. |
[4] | T. S. Nguyen, S. Begot, F. Duculty, Free-form anisotropy: A new method for crack detection on pavement surface images, in 2011 18th IEEE International Conference on Image Processing, IEEE, (2011), 1069–1072. |
[5] | R. Amhaz, S. Chambon, J. Idier, Automatic crack detection on two-dimensional pavement images: An algorithm based on minimal path selection, IEEE. T. Intell. Transp., 17 (2016), 2718–2729. |
[6] | M. Avila, S. Begot, F. Duculty, 2D image based road pavement crack detection by calculating minimal paths and dynamic programming, in 2014 IEEE International Conference on Image Processing (ICIP), IEEE, (2014), 783–787. |
[7] | Q. Li., D. Zhang, Q. Zou, 3D laser imaging and sparse points grouping for pavement crack detection, In: A. Scarpas, N. Kringos, I. Al-Qadi, Loizos A, eds, in 2017 25th European Signal Processing Conference (EUSIPCO), (2017), 2036–2040. |
[8] | Q. Zou, Y. Cao, Q. Li, CrackTree: Automatic crack detection from pavement images, Pattern. Recogn. Lett., 33 (2012), 227–238. |
[9] | Y. Huang, Y. J. Tsai, Crack fundamental element (CFE) for multi-scale crack classification, in 7th RILEM International Conference on Cracking in Pavements, (2012), 419–428. |
[10] | Y. J. Tsai, C. Jiang, Z. Wang, Implementation of automatic crack evaluation using crack fundamental element, in 2014 IEEE International Conference on Image Processing (ICIP), IEEE, (2014), 773–777. |
[11] | Y. Chen, Y. Zhang, J. Yang, Curve-like structure extraction using minimal path propagation with backtracking, IEEE T. Image. Process, 25 (2015), 988–1003. |
[12] | K. Y. Song, M. Petrou, J. Kittler, Texture crack detection, Mach. Vision. Appl, 8 (1995): 63–75. |
[13] | M. Petrou, J. Kittler, K. Y. Song, Automatic surface crack detection on textured materials, J. Mater. Process. Tech., 56 (1996), 158–167. |
[14] | E. Douka, S. Loutridis, A. Trochidis, Crack identification in plates using wavelet analysis, J. Sound. Vib., 270 (2004), 279–295. |
[15] | P. Subirats, J. Dumoulin, V. Legeay, Automation of pavement surface crack detection using the continuous wavelet transform, in 2006 International Conference on Image Processing, IEEE, (2006), 3037–3040. |
[16] | L. Itti, C. Koch, E. Niebur, A model of saliency-based visual attention for rapid scene analysis, IEEE T. Pattern. Anal., 20 (1998), 1254–1259. |
[17] | W. Xu, Z. Tang, J. Zhou, Pavement crack detection based on saliency and statistical features, in 2013 IEEE International Conference on Image Processing, IEEE, (2013), 4093–4097. |
[18] | D. Ciresan, A. Giusti, L. Gambardella, Deep neural networks segment neuronal membranes in electron microscopy images, Adv. Neural Inform. Process Syst., 25 (2012), 2843–2851. |
[19] | Y. J. Cha, W. Choi, O. Büyüköztürk, Deep learning-based crack damage detection using convolutional neural networks, Computer‐Aided Civil Infrast. Eng., 32 (2017), 361–378. |
[20] | Y. J. Cha, W. Choi, G. Suh, Autonomous structural visual inspection using region-based deep learning for detecting multiple damage types, Computer‐Aided Civil Infrast. Eng., 33 (2018), 731–747. |
[21] | Y. Liu, J. Yao, X. Lu, DeepCrack: A deep hierarchical feature learning architecture for crack segmentation, Neurocomputing, 338 (2019), 139–153. |
[22] | J. Long, E. Shelhamer, T. Darrell, Fully convolutional networks for semantic segmentation, in Proceedings of the IEEE conference on computer vision and pattern recognition, IEEE, (2015), 3431–3440. |
[23] | M. M. Islam, J. M. Kim, Vision-based autonomous crack detection of concrete structures using a fully convolutional encoder–decoder network, Sensors, 19 (2019), 4251. |
[24] | O. Ronneberger, P. Fischer, T. Brox, U-net: Convolutional networks for biomedical image segmentation, Springer International Publishing, 2015. |
[25] | O. Oktay, J. Schlemper, L. L. Folgoc, Attention u-net: Learning where to look for the pancreas, 2018. |
[26] | Z. Liu, Y. Cao, Y. Wang, Computer vision-based concrete crack detection using U-net fully convolutional networks, Automat. Constr., 104 (2019), 129–139. |
[27] | V. Badrinarayanan, A. Kendall, R. Cipolla, Segnet: A deep convolutional encoder-decoder architecture for image segmentation, IEEE T. Pattern. Anal., 39 (2017), 2481–2495. |
[28] | Q. Zou, Z. Zhang, Q. Li, Deepcrack: Learning hierarchical convolutional features for crack detection, IEEE T. Image. Process, 28 (2018), 1498–1512. |
[29] | Y. Liu, J. Yao, X. Lu, DeepCrack: A deep hierarchical feature learning architecture for crack segmentation, Neurocomputing, 338 (2019), 139–153. |
[30] | F. Wang, M. Jiang, C. Qian, Residual attention network for image classification, in 2017 IEEE Conference on Computer Vision and Pattern Recognition (CVPR), IEEE, (2017), 3156–3164. |
[31] | D. Yang, H. R. Karimi, K. Sun, Residual wide-kernel deep convolutional auto-encoder for intelligent rotating machinery fault diagnosis with limited samples, Neural Networks, 141 (2021), 133–144. |
[32] | J. Huyan, W. Li, S. Tighe, CrackU‐net: A novel deep convolutional neural network for pixelwise pavement crack detection, Struct. Contro. Hlth., 27 (2020), e2551. |
[33] | Z. Fan, C. Li, Y. Chen, Ensemble of deep convolutional neural networks for automatic pavement crack detection and measurement, Coatings, 10 (2020), 152. |
[34] | W. Song, G. Jia, D. Jia, Automatic pavement crack detection and classification using multiscale feature attention network, IEEE Access, 7 (2019), 171001–171012. |
[35] | I. Goodfellow, J. Pouget-Abadie, M. Mirza, Generative adversarial networks, Commun. ACM, 63 (2020), 139–144. |
[36] | P. Luc, C. Couprie, S. Chintala, Semantic segmentation using adversarial networks, in NIPS Workshop on Adversarial Training, 2016. |
[37] | N. Souly, C. Spampinato, M. Shah, Semi supervised semantic segmentation using generative adversarial network, in Proceedings of the IEEE conference on computer vision and pattern recognition, IEEE, (2017), 5688–5696. |
[38] | G. Wu, Q. Wang, D. Zhang, A generative probability model of joint label fusion for multi-atlas based brain segmentation, Med. Image. Anal., 18 (2014), 881–890. |
[39] | V. Alex, M. S. KP, S. S. Chennamsetty, Generative adversarial networks for brain lesion detection, in Medical Imaging 2017: Image Processing-International Society for Optics and Photonics, (2017), 10133: 101330G. |
[40] | Z. Gao, B. Peng, T. Li, Generative adversarial networks for road crack image segmentation, in 2019 International Joint Conference on Neural Networks (IJCNN), IEEE, (2019), 1–8. |
[41] | D. Bahdanau, K. Cho, Y. Bengio, Neural machine translation by jointly learning to align and translate, in 3rd International Conference on Learning Representations(ICLR), 2015. |
[42] | I. J. Goodfellow, J. Shlens, C. Szegedy, Explaining and harnessing adversarial examples, 2014. |
[43] | A. Madry, A. Makelov, L. Schmidt, Towards deep learning models resistant to adversarial attacks, in International Conference on Learning Representations, 2018. |
[44] | Y. Shi, L. Cui, Z. Qi, Automatic road crack detection using random structured forests, IEEE T. Intell. Transp., 17 (2016), 3434–3445. |
[45] | N. T. H. Nguyen, T. H. Le, S. Perry, Pavement crack detection using convolutional neural network, in Proceedings of the Ninth International Symposium on Information and Communication Technology, (2018), 251–256. |
[46] | M. D. Jenkins, T. A. Carr, M. I. Iglesias, A deep convolutional neural network for semantic pixel-wise segmentation of road and pavement surface cracks, in 2018 26th European Signal Processing Conference (EUSIPCO), IEEE, (2018), 2120–2124. |
[47] | X. Weng, Y. Huang, W. Wang, Segment-based pavement crack quantification, Automat. Constr., 105 (2019), 102819. |
1. | Lei Yang, Hanyun Huang, Shuyi Kong, Yanhong Liu, Hongnian Yu, PAF-Net: A Progressive and Adaptive Fusion Network for Pavement Crack Segmentation, 2023, 24, 1524-9050, 12686, 10.1109/TITS.2023.3287533 | |
2. | Suli Bai, Mingyang Ma, Lei Yang, Yanhong Liu, Pixel-wise crack defect segmentation with dual-encoder fusion network, 2024, 426, 09500618, 136179, 10.1016/j.conbuildmat.2024.136179 |
Algorithm: Road crack segmentation with generative adversarial learning. |
1: Input:Original image 2: Output: Predicted image 3: for the number of iterations do 4: for k steps do 5: Predicted image S(xn) from Segmentation network 6: Original image xn from training dataset 7: Label image yn from training dataset 8: Compute the Mean Absolute Error lmae 9: Update the discrimination network by ascending its stochastic gradient 10: end for 11: Update the Segmentation network by ascending its stochastic gradient 12: end forTraining of Segmentation network aim at getting the smallest value of the loss, training of discrimination network aim at getting the biggest value of the loss |
Model | CFD | GAPs284 | CRACK500 | ||||||
Precision | Recall | F1-score | Precision | Recall | F1-score | Precision | Recall | F1-score | |
Nguyen et al. [45] | 0.8567 | 0.9132 | 0.8745 | - | - | - | 0.6954 | 0.6744 | 0.6895 |
David et al. [46] | 0.8517 | 0.9155 | 0.8727 | - | - | - | 0.6811 | 0.6629 | 0.6788 |
Weng et al. [47] | 0.8682 | 0.8873 | 0.8776 | 0.6980 | 0.7055 | 0.7022 | 0.7565 | 0.7871 | 0.7715 |
Proposed-method | 0.8746 | 0.8955 | 0.8849 | 0.7720 | 0.7542 | 0.7630 | 0.9653 | 0.8197 | 0.8865 |
Dataset | CFD | GAPs284 | CRACK500 | |||
Proposed method | With attention | Without attention | With attention | Without attention | With attention | Without attention |
Precision | 0.8746 | 0.6849 | 0.7720 | 0.7205 | 0.9653 | 0.9063 |
Recall | 0.8955 | 0.8427 | 0.7542 | 0.7399 | 0.8197 | 0.8104 |
F1-score | 0.8849 | 0.7557 | 0.7630 | 0.7300 | 0.8865 | 0.8557 |
mIoU | 0.6754 | 0.6073 | 0.6619 | 0.6395 | 0.8296 | 0.7478 |
Method | Evaluation Criteria | CFD | GAPs284 | CRACK500 |
Proposed method | mIoU | 0.6754 | 0.6619 | 0.8296 |
F1-scre | 0.8849 | 0.7630 | 0.8865 | |
U-Net | mIoU | 0.5253 | 0.1766 | 0.7543 |
F1-scre | 0.6698 | 0.6789 | 0.6058 | |
Attention U-Net | mIoU | 0.4549 | 0.2519 | 0.5244 |
F1-scre | 0.6094 | 0.3833 | 0.6527 |
Algorithm: Road crack segmentation with generative adversarial learning. |
1: Input:Original image 2: Output: Predicted image 3: for the number of iterations do 4: for k steps do 5: Predicted image S(xn) from Segmentation network 6: Original image xn from training dataset 7: Label image yn from training dataset 8: Compute the Mean Absolute Error lmae 9: Update the discrimination network by ascending its stochastic gradient 10: end for 11: Update the Segmentation network by ascending its stochastic gradient 12: end forTraining of Segmentation network aim at getting the smallest value of the loss, training of discrimination network aim at getting the biggest value of the loss |
Model | CFD | GAPs284 | CRACK500 | ||||||
Precision | Recall | F1-score | Precision | Recall | F1-score | Precision | Recall | F1-score | |
Nguyen et al. [45] | 0.8567 | 0.9132 | 0.8745 | - | - | - | 0.6954 | 0.6744 | 0.6895 |
David et al. [46] | 0.8517 | 0.9155 | 0.8727 | - | - | - | 0.6811 | 0.6629 | 0.6788 |
Weng et al. [47] | 0.8682 | 0.8873 | 0.8776 | 0.6980 | 0.7055 | 0.7022 | 0.7565 | 0.7871 | 0.7715 |
Proposed-method | 0.8746 | 0.8955 | 0.8849 | 0.7720 | 0.7542 | 0.7630 | 0.9653 | 0.8197 | 0.8865 |
Dataset | CFD | GAPs284 | CRACK500 | |||
Proposed method | With attention | Without attention | With attention | Without attention | With attention | Without attention |
Precision | 0.8746 | 0.6849 | 0.7720 | 0.7205 | 0.9653 | 0.9063 |
Recall | 0.8955 | 0.8427 | 0.7542 | 0.7399 | 0.8197 | 0.8104 |
F1-score | 0.8849 | 0.7557 | 0.7630 | 0.7300 | 0.8865 | 0.8557 |
mIoU | 0.6754 | 0.6073 | 0.6619 | 0.6395 | 0.8296 | 0.7478 |
Method | Evaluation Criteria | CFD | GAPs284 | CRACK500 |
Proposed method | mIoU | 0.6754 | 0.6619 | 0.8296 |
F1-scre | 0.8849 | 0.7630 | 0.8865 | |
U-Net | mIoU | 0.5253 | 0.1766 | 0.7543 |
F1-scre | 0.6698 | 0.6789 | 0.6058 | |
Attention U-Net | mIoU | 0.4549 | 0.2519 | 0.5244 |
F1-scre | 0.6094 | 0.3833 | 0.6527 |