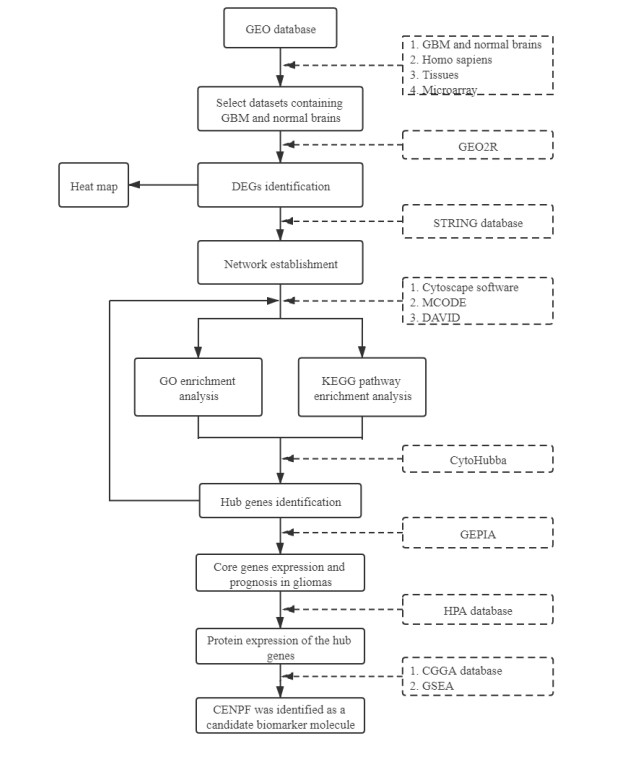
Gliomas are common malignant tumors of the central nervous system. Despite the surgical resection and postoperative radiotherapy and chemotherapy, the prognosis of glioma remains poor. Therefore, it is important to reveal the molecular mechanisms that promotes glioma progression. Microarray datasets were obtained from the Gene Expression Omnibus (GEO) database. The GEO2R tool was used to identify 428 differentially expressed genes (DEGs) and a core module from three microarray datasets. Heat maps were drawn based on DEGs. Gene Ontology (GO) and Kyoto Encyclopedia of Genes and Genomes (KEGG) pathway enrichment analysis were performed using the DAVID database. The core module was significantly involved in several KEGG pathways, such as "cell cycle", "viral carcinogenesis", "progesterone-mediated oocyte maturation", "p53 signaling pathway". The protein-protein interaction (PPI) networks and modules were built using the STRING database and the MCODE plugin, respectively, which were visualized using Cytoscape software. Identification of hub genes in the core module using the CytoHubba plugin. The top modular genes AURKA, CDC20, CDK1, CENPF, and TOP2A were associated with glioma development and prognosis. In the Human Protein Atlas (HPA) database, CDC20, CENPF and TOP2A have significant protein expression. Univariate and multivariate cox regression analysis showed that only CENPF had independent influencing factors in the CGGA database. GSEA analysis found that CENPF was significantly enriched in the cell cycle, P53 signaling pathway, MAPK signaling pathway, DNA replication, spliceosome, ubiquitin-mediated proteolysis, focal adhesion, pathway in cancer, glioma, which was highly consistent with previous studies. Our study revealed a core module that was highly correlated with glioma development. The key gene CENPF and signaling pathways were identified through a series of bioinformatics analysis. CENPF was identified as a candidate biomarker molecule.
Citation: Moxuan Zhang, Quan Zhang, Jilin Bai, Zhiming Zhao, Jian Zhang. Transcriptome analysis revealed CENPF associated with glioma prognosis[J]. Mathematical Biosciences and Engineering, 2021, 18(3): 2077-2096. doi: 10.3934/mbe.2021107
[1] | Xiaowei Zhang, Jiayu Tan, Xinyu Zhang, Kritika Pandey, Yuqing Zhong, Guitao Wu, Kejun He . Aggrephagy-related gene signature correlates with survival and tumor-associated macrophages in glioma: Insights from single-cell and bulk RNA sequencing. Mathematical Biosciences and Engineering, 2024, 21(2): 2407-2431. doi: 10.3934/mbe.2024106 |
[2] | Chen Ma, Zhihao Yao, Qinran Zhang, Xiufen Zou . Quantitative integration of radiomic and genomic data improves survival prediction of low-grade glioma patients. Mathematical Biosciences and Engineering, 2021, 18(1): 727-744. doi: 10.3934/mbe.2021039 |
[3] | Leibo Wang, Xuebin Zhang, Jun Liu, Qingjun Liu . RETRACTED ARTICLE: Kinesin family member 15 can promote the proliferation of glioblastoma. Mathematical Biosciences and Engineering, 2022, 19(8): 8259-8272. doi: 10.3934/mbe.2022384 |
[4] | Yuexian Li, Zhou Su, Biwei Wei, Mengbin Qin, Zhihai Liang . Bioinformatics analysis identified MMP14 and COL12A1 as immune-related biomarkers associated with pancreatic adenocarcinoma prognosis. Mathematical Biosciences and Engineering, 2021, 18(5): 5921-5942. doi: 10.3934/mbe.2021296 |
[5] | Lifeng Han, Steffen Eikenberry, Changhan He, Lauren Johnson, Mark C. Preul, Eric J. Kostelich, Yang Kuang . Patient-specific parameter estimates of glioblastoma multiforme growth dynamics from a model with explicit birth and death rates. Mathematical Biosciences and Engineering, 2019, 16(5): 5307-5323. doi: 10.3934/mbe.2019265 |
[6] | Jingren Niu, Qing Tan, Xiufen Zou, Suoqin Jin . Accurate prediction of glioma grades from radiomics using a multi-filter and multi-objective-based method. Mathematical Biosciences and Engineering, 2023, 20(2): 2890-2907. doi: 10.3934/mbe.2023136 |
[7] | Ahmed Hammad, Mohamed Elshaer, Xiuwen Tang . Identification of potential biomarkers with colorectal cancer based on bioinformatics analysis and machine learning. Mathematical Biosciences and Engineering, 2021, 18(6): 8997-9015. doi: 10.3934/mbe.2021443 |
[8] | Jie Wang, Md. Nazim Uddin, Rehana Akter, Yun Wu . Contribution of endothelial cell-derived transcriptomes to the colon cancer based on bioinformatics analysis. Mathematical Biosciences and Engineering, 2021, 18(6): 7280-7300. doi: 10.3934/mbe.2021360 |
[9] | Tongmeng Jiang, Pan Jin, Guoxiu Huang, Shi-Cheng Li . The function of guanylate binding protein 3 (GBP3) in human cancers by pan-cancer bioinformatics. Mathematical Biosciences and Engineering, 2023, 20(5): 9511-9529. doi: 10.3934/mbe.2023418 |
[10] | Qinyan shen, Jiang wang, Liangying zhao . To investigate the internal association between SARS-CoV-2 infections and cancer through bioinformatics. Mathematical Biosciences and Engineering, 2022, 19(11): 11172-11194. doi: 10.3934/mbe.2022521 |
Gliomas are common malignant tumors of the central nervous system. Despite the surgical resection and postoperative radiotherapy and chemotherapy, the prognosis of glioma remains poor. Therefore, it is important to reveal the molecular mechanisms that promotes glioma progression. Microarray datasets were obtained from the Gene Expression Omnibus (GEO) database. The GEO2R tool was used to identify 428 differentially expressed genes (DEGs) and a core module from three microarray datasets. Heat maps were drawn based on DEGs. Gene Ontology (GO) and Kyoto Encyclopedia of Genes and Genomes (KEGG) pathway enrichment analysis were performed using the DAVID database. The core module was significantly involved in several KEGG pathways, such as "cell cycle", "viral carcinogenesis", "progesterone-mediated oocyte maturation", "p53 signaling pathway". The protein-protein interaction (PPI) networks and modules were built using the STRING database and the MCODE plugin, respectively, which were visualized using Cytoscape software. Identification of hub genes in the core module using the CytoHubba plugin. The top modular genes AURKA, CDC20, CDK1, CENPF, and TOP2A were associated with glioma development and prognosis. In the Human Protein Atlas (HPA) database, CDC20, CENPF and TOP2A have significant protein expression. Univariate and multivariate cox regression analysis showed that only CENPF had independent influencing factors in the CGGA database. GSEA analysis found that CENPF was significantly enriched in the cell cycle, P53 signaling pathway, MAPK signaling pathway, DNA replication, spliceosome, ubiquitin-mediated proteolysis, focal adhesion, pathway in cancer, glioma, which was highly consistent with previous studies. Our study revealed a core module that was highly correlated with glioma development. The key gene CENPF and signaling pathways were identified through a series of bioinformatics analysis. CENPF was identified as a candidate biomarker molecule.
Glioma is the most common and fatal primary tumor of the central nervous system, accounting for more than 80% of malignant brain tumors [1]. Among these, glioblastoma multiforme (GBM, World Health Organization grade IV glioma) is the most agammaessive and most common primary tumor in adults, with an average incidence rate of 4 to 5 per 100, 000 people per year [2]. Although we have made great progress in the past few decades and have made great progress in understanding the basic mechanisms of glioma development, the median survival of glioblastoma is only 12 to 15 months [3,4,5]. The 3-year survival rate of patients with glioblastoma is only 4 to 15% [6]. Therefore, there is an urgent need for developing new therapeutics for glioma patients.
The current care for glioma consists of maximal resection combined with radiotherapy and chemotherapy [7]. Surgery can delay clinical symptoms, prolong survival, and obtain enough tumor specimens to identify tissue and molecular pathology diagnoses. At present, molecular pathology of nervous system malignant tumors have made great progress and found a series of molecular markers that are helpful for clinical diagnosis and predicting formation, invasion, progression and prognosis of glioma. The main molecular markers, such as IDH mutation, Codeletion of chromosomal arms 1p and 19q, MGMT promoter methylation, EGFRvIII, ATRX mutations, TERT promoter mutation and Ki-67 are being used in molecular pathological diagnosis, treatment options, and prognostic evaluation with glioma patients [8]. These molecular markers play a central role in regulating tumor cell proliferation and apoptosis. In recent years, the cancer genome atlas (TCGA) has identified many core signaling pathways for the pathogenesis of malignant glioma: RTK/RAS/PI3K signal pathway, P53 signal pathway, retinoblastoma protein (RB) signal pathway [9]. These molecular markers and signal pathways are important for individualized treatment and clinical prognosis of glioma. Although the classification of tumors in the central nervous system is still based on morphological criteria, molecular phenotypic changes have provided some important supporting basis for the differential diagnosis of tumors. For example, integrated mutations in IDH, TP53 and ATRX suggest astrogliomas. IDH mutation, 1p/19q co-deletion, and TERT promoter mutation suggest oligoglioma. Mutations in the IDH wild-type and TERT promoter suggest adult glioblastoma. IDH wild-type and BRAF mutations are indicative of low-grade gliomas in children or adolescents. Integrating molecular subtypes into the classification of gliomas helps to deepen the understanding of the nature of gliomas. And this huge progress is inseparable from the development of bioinformatics. Many therapies targeting these molecular markers have been used in clinical trials, but few have ultimately succeeded. Therefore, it is urgent to identify new therapeutic targets for glioma.
In recent years, the development of high-throughput sequencing technology and gene chip technology has promoted the molecular revolution to provide an unprecedented opportunity for the study of molecular mechanisms in cancer biology [10]. Through these new technologies, more and more candidate genes for diseases have been discovered [11,12]. Gene chips, also known as DNA microarrays, make it possible to obtain a large number of effective cancer-related gene expression profiles [13]. Thus, DNA microarrays play an important role in the discovery of molecular markers for predicting cancer prognosis [14,15]. The Gene Expression Omnibus (GEO) database is a major repository that stores high-throughput functional genomic data sets generated using microarray-based and sequence-based techniques for the identification of key genes involved in tumorigenesis, progression, prognosis, and resistance [16]. Identification of glioma-related potential molecular biomarkers is essential for improving the clinical efficacy of glioma [17].
Bioinformatics analysis of multiple datasets may help us identify molecular biomarkers associated with glioma development. In this study, a comprehensive bioinformatics analysis of GBM gene expression profiles was performed to identify potential key biomarkers of GBM. Microarray datasets obtained from the GEO database were used to identify differentially expressed genes (DEGs) between GBM and normal brain tissues. Through enrichment analysis, the biological functions of the resulting DEGs were clarified and a protein-protein interaction (PPI) network was established. Key genes were screened through a series of integrated bioinformatics analyses.
We selected microarray data from the Gene Expression Omnibus (GEO) database (http://www.ncbi.nlm.nih.gov/geo), which is a visual, friendly and free public database. The keyword we searched for is "glioma". If the following conditions are met, it is considered that the next step can be analyzed: 1) Studies included both glioblastoma and normal brain tissue; 2) Species are limited to Homo sapiens; 3) Attribute name was limited tissue; 4) Platform was microarray. Then, three gene expression profiles (GSE108476, GSE50161 and GSE116520) were obtained from the GEO database. The GSE108476 dataset submitted by Gusev Y based on the GPL570 platform (Affymetrix Human Genome U133 Plus 2.0 Array) was selected 221 glioblastomas and 28 normal brains. The GSE50161 dataset submitted by Griesinger AM based on the GPL570 platform (Affymetrix Human Genome U133 Plus 2.0 Array) was selected 34 glioblastomas and 13 normal brains. The GSE116520 dataset submitted by Kruthika BS based on the GPL10558 platform (Illumina HumanHT-12 V4.0 expression beadchip) was selected 17 glioblastomas and 8 normal brains. The flow chart for bioinformatics analysis of public datasets is shown in Figure 1.
The Chinese Glioma Genome Atlas (CGGA) database (http://www.cgga.org.cn) is a user-friendly web application for data storage and analysis to explore glioma datasets from Chinese samples [18]. This study retrospectively collected RNA sequencing data from 693 glioma patients from the CGGA database, ranging from WHO grade II-IV, as a validation cohort. Information on these patients is available from the online database website.
GEO2R (http://www.ncbi.nlm.nih.gov/geo/geo2r) is an interactive web tool that allows users to compare two or more sets of samples in the GEO series to identify DEGs under different experimental conditions. Adjusted P value was used to reduce the false discovery rate (FDR). The cut-off values for DEGs screening were based on FDR < 0.05 and |logFC| ≧ 1.0. The overlapping DEGs in the three datasets were retained for further analysis.
Gene ontology (GO) is a database established by the Gene Ontology Consortium to describe the function of gene products, covering biological processes, molecular functions and cellular components [19]. Kyoto Encyclopedia of Genes and Genomes (KEGG) is a database resource that integrates genomic, chemical, and system function information to explain biological genome sequences and other high-throughput sequencing data [20]. GO and KEGG enrichment analysis was performed using the DAVID (https://david.ncifcrf.gov) online tool, which is a bioinformatics database that integrates biological data and analysis tools to provide biofunctional annotations for a large number of genes or proteins, helping users extract biological information from them [21]. P-value < 0.05 was set as the cut-off criterion.
The STRING website (http://string-db.org/cgi/) is an online tool that evaluates the protein-protein interaction information. To analyze the relationship between DEGs, the PPI network was built using the STRING online tool and the interaction score > 0.4 was chosen as the cutoff criterion. Subsequently, the PPI network was analyzed using the Molecular Complex Detection (MCODE) tool in Cytoscape [22]. Finally, the cytohubba tool was used to select the hub genes in Cytoscape [23].
Gene difference analysis and Overall survival curve were obtained from database using Gene Expression Profiling Interactive Analysis (GEPIA) online tools. GEPIA is a web server for cancer and normal gene expression profiling and interaction analysis. GEPIA provides key interactive and customizable functions, including differential expression analysis, correlation analysis, patient survival analysis, and similar gene detection. GEPIA, based on Cancer Genome Atlas database (TCGA) and Genotype-Tissue Expression (GTEx) database, was used to analyze differential expression levels and survival of the hub genes [24]. P < 0.05 was considered statistically significant.
The Human Protein Atlas (HPA) database is a friendly database dedicated to providing tissue and cell distribution information for all human proteins and providing public access for free [25]. The HPA database was used to examine the expression of hub genes in gliomas. Antibodies used in the HPA database were: AURKA (CAB001454), CDC20 (HPA055288), CDK1 (HPA003387), CENPF (HPA064308), TOP2A (HPA006458).
GSEA was used for KEGG enrichment analysis of target genes based on TCGA database. The screening criteria were based on 25%, 75% cut-off values. GSEA was used to gene sets from the Molecular Signatures Database. P-value < 0.05 and FDR < 0.25 was considered statistically significant.
The chi-square analysis was used to analyze the correlation between gene expression and clinicopathological features. The Cox proportional hazards model was used in the multivariate prognostic analysis. P-value < 0.05 was considered to be statistically significant in all of the statistical analyses. For data analysis, we were all performed using GraphPad Prism 6 and SPSS 20 software.
According to the search criteria, 3 microarray datasets were retrieved from the GEO database, including 272 glioblastomas and 49 normal brain tissues (Table 1). GSE108476 dataset was produced by Affymetrix Human Genome U133 Plus 2.0 Array (GPL570); GSE50161 dataset by Affymetrix Human Genome U133 Plus 2.0 Array (GPL570); and GSE116520 dataset by Illumina HumanHT-12 V4.0 expression beadchip (GPL10558).
ID | Platform | Sample | References |
GSE108476 | GPL570 | GBM = 221, NB = 28 | [26] |
GSE50161 | GPL570 | GBM = 34, NB = 13 | [27] |
GSE116520 | GPL10558 | GBM = 17, NB = 8 | [28] |
GBM, glioblastoma multiforme; NB, normal brain. |
428 differentially expressed genes (DEGs) were found using the GEO2R online tool analysis (adjusted P < 0.05 and |FC| > 2). These DEGs were shared by GSE108476, GSE50161 and GSE116520 datasets (Figure 2). Subsequently, to test DEGs, we mapped the heat map of the differential genes through these 3 datasets (Figure 3).
To further predict the biological mechanism of DEGs, we performed functional and pathway enrichment analysis, including GO and KEGG. The GO term enrichment analysis showed that in the biological processes-associated category, the DEGs were enriched in the interferon-γ-mediated signaling pathway, positive regulation of GTPase activity, signal transduction, microtubule cytoskeleton organization (Figure 4A and Table S1). Also, cell component analysis showed that the DEGs were enriched in the cytoplasm, the perinuclear region of cytoplasm, cell junction, plasma membrane and cytosol (Figure 4A and Table S1). Besides, in terms of molecular function, the DEGs were enriched in protein binding, protein kinase activity, receptor binding, ATP binding, GTPase activator activity and drug binding (Figure 4A and Table S1). Finally, as shown in Figure 4B, KEGG pathway analysis showed that the DEGs were enriched in the ErbB signaling pathway, GABAergic synapse, progesterone-mediated oocyte maturation, cAMP signaling pathway, microRNAs in cancer. Detailed information of these pathways was listed in Table S2.
Catelogy | The rank method in cytoHubba | |||||
MCC | MNC | Degree | EPC | Closeness | Radiality | |
Gene symbol top 10 | CDCA5 | MYC | MYC | CCNA2 | MYC | MYC |
PBK | SNAP25 | SNAP25 | CDK1 | SNAP25 | SNAP25 | |
MELK | CDK1 | CDK1 | TOP2A | CDK1 | CENPF | |
CENPF | CCNA2 | CCNA2 | AURKA | AURKA | CDK1 | |
TOP2A | AURKA | AURKA | CDC20 | CENPF | AURKA | |
CDC20 | TOP2A | CDC20 | PBK | CDC20 | NES | |
CDK1 | CDC20 | TOP2A | CENPF | CCNA2 | CD44 | |
CCNA2 | CDKN3 | CDKN3 | CDKN3 | CD44 | CDC20 | |
AURKA | CENPF | CENPF | MYC | TOP2A | CDK4 | |
HJURP | PBK | CD44 | HJURP | CDKN3 | TOP2A | |
Selected genes were the overlap hub genes in the top 10 by six ranked methods respectively in cytoHubba. MCC: Maximal clique centrality; MNC: Maximum neighborhood component; Degree: Node connect degree; EPC: Edge percolated component. |
The PPI network was analyzed by STRING which contains 421 nodes and 1083 edges. The k-means clustering of DEGs by STRING was divided into 2 categories (Figure 5A). We found a cluster of genes in the glioma-related network, all of which were up-regulated and play a central role in the entire network. Then, we used the MCODE in Cytoscape software to mine highly clustered modules from this network. As expected, a module with higher connectivity (cluster score = 20.3) was identified, the module has 23 nodes and 223 edges (Figure 5B). To further predict the biological mechanism of the core molecule, we performed pathway enrichment analysis. The module molecules were involved in several KEGG pathways including "cell cycle", "viral carcinogenesis", "progesterone-mediated oocyte maturation", "oocyte meiosis", "p53 signaling pathway" and "cellular senescence" (Figure 5C and Table S3). These results indicated that the highly clustered modules may play an important role in the development of glioma.
Clinicopathological Parameter, value | CENPF expression | ||||
N | Low expression (%) | High expression (%) | χ2 | P value | |
Gender | |||||
Male | 398 (57.4) | 206 (51.8) | 192 (48.2) | ||
Female | 295 (42.6) | 140 (47.5) | 155 (52.5) | 1.254 | 0.263 |
Age | |||||
≥ 43 | 349 (50.4) | 167 (47.9) | 182 (52.1) | ||
< 43 | 343 (49.5) | 179 (52.2) | 164 (47.8) | 1.301 | 0.254 |
Missing | 1 (0.1) | 0 (0.0) | 1 (100.0) | ||
WHO grade | |||||
II | 188 (27.1) | 137 (72.9) | 51 (27.1) | ||
III | 255 (36.8) | 124 (48.6) | 131 (51.4) | ||
IV | 249 (36.0) | 85 (34.1) | 164 (65.9) | 64.597 | < 0.001* |
Missing | 1 (0.1) | 0 (0.0) | 1 (100.0) | ||
IDH mutation | |||||
Wildtype | 286 (41.3) | 110 (38.5) | 176 (61.5) | ||
Mutant | 356 (51.4) | 188 (52.8) | 168 (47.2) | 13.126 | < 0.001* |
Missing | 51 (7.3) | 48 (94.1) | 3 (5.9) | ||
1p, 19q codeletion status | |||||
Non-codel | 478 (69.0) | 198 (41.4) | 280 (58.6) | ||
Codel | 145 (20.9) | 85 (58.6) | 60 (41.4) | 13.273 | < 0.001* |
Missing | 70 (10.1) | 63 (90.0) | 7 (10.0) | ||
Recurrent | |||||
Yes | 271 (39.1) | 98 (36.2) | 173 (63.8) | ||
No | 422 (60.9) | 248 (58.8) | 174 (41.2) | 33.731 | < 0.001* |
Radiotherapy status | |||||
Yes | 509 (73.5) | 246 (48.3) | 263 (51.7) | ||
No | 113 (16.3) | 72 (63.7) | 41 (36.3) | 8.762 | 0.003* |
Missing | 71 (10.2) | 28 (39.4) | 43 (60.6) | ||
Chemotherapy status | |||||
Yes | 457 (65.9) | 209 (45.7) | 248 (54.3) | ||
No | 151 (21.8) | 100 (66.2) | 51 (33.8) | 19.07 | < 0.001* |
Missing | 85 (12.2) | 37 (43.5) | 48 (56.5) |
We next focused on the top module genes, as their expression in glioma tissues was highly upregulated in all datasets considered in this study. We used cytoHubba from Cytoscape software to evaluate the role of molecules in PPI networks by different scoring methods. As shown in Table 2, five hub genes were selected by cytoHubba using six methods, including AURKA, CDC20, CDK1, CENPF and TOP2A.
GEPIA, an online database of data from the TCGA and GTEx databases, included 207 normal subjects and 681 glioma patients. As shown in Figure 6A, all the hub genes were significantly upregulated in glioma, compared with the normal brain (P < 0.05). By overall survival (OS) analysis, we found that high expression of hub genes was associated with poor survival (Figure 6B). These results suggested that the five hub genes were closely related to the occurrence and development of glioma.
In the HPA database, we were able to find pathological sections of normal and glioma patients for Hub gene protein staining. Immunohistochemical results of five hub genes in the HPA database showed that CENPF and CDC20 were highly expressed in the nucleus and cytoplasm of glioma cells, but were almost undetectable in normal brain tissues (Figure 7). TOP2A is highly expressed in the nucleus of glioma cells and is not detected in normal brain tissues. Although AURKA and CDK1 are expressed in the nucleus of glioma cells, the positive rate is lower (Figure 7). This result shows that CDC20, CENPF and TOP2A may have more research significance in glioma.
693 cases of glioma patients with high-throughput sequencing were selected from the CGGA database. Divided into high expression group and low expression group by the median of expression level. Chi-square test results showed that CDC20, TOP2A and CENPF were associated with glioma WHO grade, IDH mutation, 1p, 19q co-deletion, recurrence and chemoradiotherapy (Tables 3, S4 and S5). P < 0.05 was considered statistically significant. Univariate and multivariate cox regression analysis showed that only CENPF was the same with IDH status and 1p19q co-deletion status has the independent influencing factors (P < 0.01) (Table 4). CDC20 and TOP2A have no independent influencing factors (P > 0.05) (Table 4). Hence, CENPF has the potential to be a marker for predicting the prognosis of glioma.
Parameter | Univariate analysis | Multivariate analysis | ||||
P-value | HR | 95% CI | P-value | HR | 95% CI | |
Gender(Female vs. male) | 0.907 | 1.012 | 0.826-1.241 | 0.100 | 1.226 | 0.962-1.562 |
Age (years)(≧ 43 vs. < 43) | < 0.001* | 1.732 | 1.411-2.125 | 0.001* | 1.474 | 1.161-1.873 |
WHO grade(II vs. III vs. IV) | < 0.001* | 2.740 | 2.359-3.182 | < 0.001* | 2.128 | 1.719-2.636 |
IDH mutation(Wildtype vs. Mutant) | < 0.001* | 3.237 | 2.615-4.008 | < 0.001* | 1.749 | 1.318-2.319 |
1p, 19q codeletion status(Non-codel vs. Codel) | < 0.001* | 0.263 | 0.187-0.369 | < 0.001* | 0.439 | 0.292-0.659 |
Recurrent(Primary vs. Recurrent) | < 0.001* | 2.089 | 1.702-2.564 | < 0.001* | 2.444 | 1.924-3.103 |
Radiotherapy status(Yes vs. No) | 0.020 | 1.430 | 1.057-1.935 | 0.333 | 0.829 | 0.568-1.212 |
Chemotherapy status(Yes vs. No) | 0.003 | 1.506 | 1.154-1.966 | 0.010 | 0.635 | 0.449-0.899 |
CENPF expression(high expression vs. low expression) | < 0.001* | 2.942 | 2.374-3.645 | < 0.001* | 2.403 | 1.736-3.325 |
CDC20 expression(high expression vs. low expression) | < 0.001* | 2.536 | 2.054-3.131 | 0.920 | 0.982 | 0.688-1.401 |
TOP2A expression(high expression vs. low expression) | < 0.001* | 2.142 | 1.739-2.639 | 0.617 | 1.097 | 0.763-1.576 |
To further understand CENPF, we analyzed its role in glioma by GSEA based on the TCGA database. P-value < 0.05 and FDR < 0.25 was considered statistically significant. As shown in Figure 8, the results indicated that the high expression of CENPF exhibited appreciable relevance to cell cycle, P53 signaling pathway, MAPK signaling pathway, DNA replication, spliceosome, ubiquitin-mediated proteolysis, focal adhesion, pathway in cancer, glioma, which were highly consistent with previous results.
With the progress of a series of large-scale scientific research projects such as the Human Genome Project and the Brain Project, glioma treatment will face new challenges and opportunities. At present, the research hotspots of scientists all over the world mainly focus on molecular targeted therapy, immunotherapy, understanding of tumor microenvironment, glioma stem cells and exosomes [1,29]. Although glioma research has achieved unprecedented development and progress in many disciplines, glioma transcriptome analysis remains the focus of current research.
To explore key genes that significantly affect the prognosis of gliomas, our current study systematically integrates three independent microarray datasets containing GBM and normal brain tissue from the GEO database. Through a series of bioinformatics analyses, we found a high-linking module containing 23 glioma risk genes that better distinguish between glioma and normal brain tissue. The top genes seem to be related to the development, progression and prognosis of glioma.
The modules identified in this study were highly connected. Through functional enrichment analysis, we found that the module participates in a variety of KEGG pathways. The occurrence and development of gliomas are inseparable from the regulation of the cell cycle. The proteins involved in the cell cycle are called cyclin proteins and are classified into cyclins A, B, D, E, G, and H [30]. They bind to key protein kinases (cyclin-dependent kinases, CDKs) and regulate their enzymatic activity, helping to advance and coordinate the cell cycle [31]. Many studies now have found that cycle arrest in the G2/M phase inhibits the progression of glioma through targeting molecules [32,33,34]. Other studies have found that targeted inhibition of certain proteins can arrest the cycle of GO/G1 phase and lead to inhibition of glioma progression [35,36,37]. Besides, maintenance and repair of DNA telomeres is an important process to prevent genomic instability and cancer [38]. P53 signaling pathway inhibition has been widely reported as necessary for glioma development [39,40]. Cellular senescence refers to the process in which cell proliferation and differentiation abilities and physiological functions gradually decline overtime during the execution of life activities. Recent research shows that induced cell senescence has anti-tumor effects [41]. There is increasing evidence that human cytomegalovirus (HCMV) infection is associated with GBM and leads to the growth and metastasis of GBM cells [42]. Stoichioproteomics reveal that oocyte meiosis and progesterone-mediated oocyte maturation pathways are also associated with gliomas. However, the relationship with glioma needs further study.
The top module genes of AURKA, CDC20, CDK1, TOP2A and CENPF were highly correlated with the development and progression of glioma. In the glioma TCGA database, high expression of AURKA, CDC20, CDK1, CENPF and TOP2A is associated with poor prognosis, which means they may become new prognostic markers. Recent studies have found that ARUKA promotes glioma cell proliferation and resistance to PI3K inhibitors through the PLK1/CDK1 signaling pathway [43,44]. Yiming Ding et al. found that high expression of CDC20 in glioma patients is associated with poor prognosis [45]. Yunqiu Zhang et al. investigated that application of the CDC20 gene module co-expressed signature may provide more selective adjuvant therapy for glioma patients [46]. Zhenhua Song et al. showed that activation of survivin signaling by overexpression CDK1, contributed to the suppression of the senescence process in senescence-escaping cells [47]. S. Deguchi et al. found that down-regulation of TOP2A expression in glioma cell lines resulted in reduced proliferation of glioma cells [48].
In subsequent experiments, TOP2A, CENPF and CDC20 were screened by TCGA and HPA databases. RNA sequencing data of 693 glioma patients were obtained from the CGGA database for data analysis. Chi-square test found that CENPF, CDC20 and TOP2A were all statistically significant. Univariate and multivariate cox regression analysis found that only CENPF was the same with IDH status and 1p19q co-deletion status has the independent influencing factors. Centromere protein F (CENPF), a protein involved in centromere-centromere complexation and chromosome segregation during mitosis, is involved in tumor progression [49]. The latest studies found that CENPF may become an important regulator of prostate cancer metabolism through its role in mitochondria [49,50]. CENPF is highly expressed in hepatocellular carcinoma and promotes growth and invasion [51]. However, it has not been reported that CENPF is associated with glioma. The role of CENPF in gliomas was further analyzed by GSEA. The results showed that the high expression of CENPF exhibited appreciable relevance to cell cycle, P53 signaling pathway, MAPK signaling pathway, DNA replication, spliceosome, ubiquitin-mediated proteolysis, focal adhesion, pathway in cancer, glioma, which were overlap with the enrichment pathways in the DEGs and hub genes. In the next step, we will conduct experiments to further verify the role of CENPF in gliomas.
Our current research systematically integrates multiple microarray gene expression profiles and discovers a module related to the development of gliomas. The expression of the top module gene is closely related to the occurrence, development and prognosis of glioma. Our work may provide a deeper understanding of the molecular mechanism of glioma. Finally, through bioinformatics analysis, we found that CENPF is the core molecule in the top module molecule. For the next step, experiments will be carried out to verify the specific mechanism of CENPF in glioma.
All authors declare no conflicts of interest in this paper.
[1] |
M. Qian, S. Wang, X. Guo, J. Wang, Z. Zhang, W. Qiu, et al., Hypoxic glioma-derived exosomes deliver microRNA-1246 to induce M2 macrophage polarization by targeting TERF2IP via the STAT3 and NF-κB pathways, Oncogene, 39 (2020), 428-442. doi: 10.1038/s41388-019-0996-y
![]() |
[2] |
Z. P. Wen, W. J. Zeng, Y. H. Chen, H. Li, J. Y. Wang, Q. Cheng, et al., Knockdown ATG4C inhibits gliomas progression and promotes temozolomide chemosensitivity by suppressing autophagic flux, J. Exp. Clin. Cancer Res., 38 (2019), 1-15. doi: 10.1186/s13046-018-1018-6
![]() |
[3] |
X. Chen, M. Zhang, H. Gan, H. Wang, J. H. Lee, D. Fang, et al., A novel enhancer regulates MGMT expression and promotes temozolomide resistance in glioblastoma, Nat. Commun., 9 (2018), 1-14. doi: 10.1038/s41467-017-02088-w
![]() |
[4] |
Y. Chen, P. Liu, P. Sun, J. Jiang, Y. Zhu, T. Dong, et al., Oncogenic MSH6-CXCR4-TGFB1 feedback loop: a novel therapeutic target of photothermal therapy in glioblastoma multiforme, Theranostics, 9 (2019), 1453-1473. doi: 10.7150/thno.29987
![]() |
[5] | T. Liu, A. Li, Y. Xu, Y. Xin, Momelotinib sensitizes glioblastoma cells to temozolomide by enhancement of autophagy via JAK2/STAT3 inhibition, Oncol. Rep., 41 (2019), 1883-1892. |
[6] |
J. Ruiz, D. Case, G. Enevold, R. Rosdhal, S. B. Tatter, T.L. Ellis, et al., A phase Ⅱ trial of thalidomide and procarbazine in adult patients with recurrent or progressive malignant gliomas, J. Neurooncol, 106 (2012), 611-617. doi: 10.1007/s11060-011-0698-y
![]() |
[7] |
M. Nagane, R. Nishikawa, Y. Narita, H. Kobayashi, S. Takano, N. Shinoura, et al., Phase Ⅱ study of single-agent bevacizumab in Japanese patients with recurrent malignant glioma, Jpn. J. Clin. Oncol., 42 (2012) 887-895. doi: 10.1093/jjco/hys121
![]() |
[8] |
T. Jiang, Y. Mao, W. Ma, Q. Mao, Y. You, X. Yang, et al., CGCG clinical practice guidelines for the management of adult diffuse gliomas, Cancer Lett, 375 (2016) 263-273. doi: 10.1016/j.canlet.2016.01.024
![]() |
[9] | Cancer Genome Atlas Research Network, Comprehensive genomic characterization defines human glioblastoma genes and core pathways, Nature, 455 (2008), 1061. |
[10] |
H. Wang, A. K. Diaz, T. I. Shaw, Y. Li, M. Niu, J. H. Cho, et al., Deep multiomics profiling of brain tumors identifies signaling networks downstream of cancer driver genes, Nat. Commun., 10 (2019) 1-15. doi: 10.1038/s41467-018-07882-8
![]() |
[11] |
B. Zhang, Q. Wu, R. Xu, X. Hu, Y. Sun, Q. Wang, et al., The promising novel biomarkers and candidate small molecule drugs in lower-grade glioma: Evidence from bioinformatics analysis of high-throughput data, J. Cell. Biochem., 120 (2019), 15106-15118. doi: 10.1002/jcb.28773
![]() |
[12] |
B. Kamaraj, R. Purohit, Mutational analysis on membrane associated transporter protein (MATP) and their structural consequences in oculocutaeous albinism type 4 (OCA4)-a molecular dynamics approach, J. Cell. Biochem., 117 (2016), 2608-2619. doi: 10.1002/jcb.25555
![]() |
[13] |
X. Fan, L. Shi, H. Fang, Y. Cheng, R. Perkins, W. Tong, DNA microarrays are predictive of cancer prognosis: a re-evaluation, Clin. Cancer Res., 16 (2010), 629-636. doi: 10.1158/1078-0432.CCR-09-1815
![]() |
[14] |
L. Ein-Dor, O. Zuk, E. Domany, Thousands of samples are needed to generate a robust gene list for predicting outcome in cancer, Proc. Natl. Acad. Sci., 103 (2006), 5923-5928. doi: 10.1073/pnas.0601231103
![]() |
[15] |
S. Michiels, S. Koscielny, C. Hill, Prediction of cancer outcome with microarrays: a multiple random validation strategy, Lancet, 365 (2005), 488-492. doi: 10.1016/S0140-6736(05)17866-0
![]() |
[16] |
S. E. Wilhite, T. Barrett, Strategies to explore functional genomics data sets in NCBI's GEO database, Methods Mol. Biol., 802 (2012), 41-53. doi: 10.1007/978-1-61779-400-1_3
![]() |
[17] |
S. Venneti, J. T. Huse, The evolving molecular genetics of low-grade glioma, Adv. Anat. Pathol., 22 (2015), 94-101. doi: 10.1097/PAP.0000000000000049
![]() |
[18] |
F. Wu, R. C. Chai, Z. Wang, Y. Q. Liu, Z. Zhao, G. Z. Li, et al., Molecular classification of IDH-mutant glioblastomas based on gene expression profiles, Carcinogenesis, 40 (2019), 853-860. doi: 10.1093/carcin/bgz032
![]() |
[19] |
J. Lomax, Get ready to GO! A biologist's guide to the Gene Ontology, Briefings Bioinf., 6 (2005), 298-304. doi: 10.1093/bib/6.3.298
![]() |
[20] |
M. Kanehisa, S. Goto, KEGG: kyoto encyclopedia of genes and genomes, Nucleic Acids Res., 28 (2000), 27-30. doi: 10.1093/nar/28.1.27
![]() |
[21] |
L. Slemc, T. Kunej, Transcription factor HIF1A: downstream targets, associated pathways, polymorphic hypoxia response element (HRE) sites, and initiative for standardization of reporting in scientific literature, Tumour Biol., 37 (2016), 14851-14861. doi: 10.1007/s13277-016-5331-4
![]() |
[22] |
M. Kohl, S. Wiese, B. Warscheid, Cytoscape: software for visualization and analysis of biological networks, Methods Mol. Biol., 696 (2011), 291-303. doi: 10.1007/978-1-60761-987-1_18
![]() |
[23] |
C. H. Chin, S. H. Chen, H. H. Wu, C. W. Ho, M. T. Ko, C.Y. Lin, CytoHubba: identifying hub objects and sub-networks from complex interactome, BMC Syst. Biol., 8 (2014), 1-7. doi: 10.1186/1752-0509-8-1
![]() |
[24] |
Z. Tang, C. Li, B. Kang, G. Gao, C. Li, Z. Zhang, GEPIA: a web server for cancer and normal gene expression profiling and interactive analyses, Nucleic Acids Res., 45 (2017), W98-W102. doi: 10.1093/nar/gkx247
![]() |
[25] | M. Uhlen, L. Fagerberg, B. M. Hallstrom, C. Lindskog, P. Oksvold, A. Mardinoglu, Tissue-based map of the human proteome, Science, 347 (2015). |
[26] |
Y. Gusev, K. Bhuvaneshwar, L. Song, J. C. Zenklusen, H. Fine, S. Madhavan, The REMBRANDT study, a large collection of genomic data from brain cancer patients, Sci. Data, 5 (2018), 180158. doi: 10.1038/sdata.2018.158
![]() |
[27] |
A. M. Griesinger, D. K. Birks, A. M. Donson, V. Amani, L. M. Hoffman, A. Waziri, et al., Characterization of distinct immunophenotypes across pediatric brain tumor types, J. Immunol., 191 (2013), 4880–4888. doi: 10.4049/jimmunol.1301966
![]() |
[28] |
B. S. Kruthika, R. Jain, A. Arivazhagan, R. D. Bharath, T. C. Yasha, P. Kondaiah, et al., Transcriptome profiling reveals PDZ binding kinase as a novel biomarker in peritumoral brain zone of glioblastoma, J. Neurooncol., 141 (2019), 315–325. doi: 10.1007/s11060-018-03051-5
![]() |
[29] |
Q. Ma, W. Long, C. Xing, J. Chu, M. Luo, H. Y. Wang, et al., Cancer stem cells and immunosuppressive microenvironment in glioma, Front. Immunol., 9 (2018), 2924. doi: 10.3389/fimmu.2018.02924
![]() |
[30] |
M. K. Kalita, U. K. Nandal, A. Pattnaik, A. Sivalingam, G. Ramasamy, M. Kumar, et al., CyclinPred: a SVM-based method for predicting cyclin protein sequences, PLoS One, 3 (2008), e2605. doi: 10.1371/journal.pone.0002605
![]() |
[31] |
Y. Liao, Y. Feng, J. Shen, F. J. Hornicek, Z. Duan, The roles and therapeutic potential of cyclin-dependent kinases (CDKs) in sarcoma, Cancer Metastasis Rev., 35 (2016), 151-163. doi: 10.1007/s10555-015-9601-1
![]() |
[32] |
F. Brand, A. Forster, A. Christians, M. Bucher, C. M. Thome, M. S. Raab, et al., FOCAD loss impacts microtubule assembly, G2/M progression and patient survival in astrocytic gliomas, Acta Neuropathol., 139 (2020), 175-192. doi: 10.1007/s00401-019-02067-z
![]() |
[33] |
A. C. Cheng, Y. C. Hsu, C. C. Tsai, The effects of cucurbitacin E on GADD45beta-trigger G2/M arrest and JNK-independent pathway in brain cancer cells, J. Cell. Mol. Med., 23 (2019), 3512-3519. doi: 10.1111/jcmm.14250
![]() |
[34] |
N. Liu, G. Hu, H. Wang, Z. Li, Z. Guo, PLK1 inhibitor facilitates the suppressing effect of
temozolomide on human brain glioma stem cells, J. Cell. Mol. Med., 22 (2018), 5300–5310. doi: 10.1111/jcmm.13793
![]() |
[35] | Q. K. Ji, J. W. Ma, R. H. Liu, X. S. Li, F. Z. Shen, L. Y. Huang, et al., CDCA7L promotes glioma proliferation by targeting CCND1 and predicts an unfavorable prognosis, Mol. Med. Rep., 20 (2019), 1149-1156. |
[36] |
T. Yawata, Y. Higashi, Y. Kawanishi, T. Nakajo, N. Fukui, H. Fukuda, et al., CD146 is highly expressed in glioma stem cells and acts as a cell cycle regulator, J. Neurooncol., 144 (2019), 21-32. doi: 10.1007/s11060-019-03200-4
![]() |
[37] |
H. Fan, L. Geng, F. Yang, X. Dong, D. He, Y. Zhang, Ursolic acid derivative induces apoptosis in glioma cells through down-regulation of cAMP, Eur. J. Med. Chem., 176 (2019), 61-67. doi: 10.1016/j.ejmech.2019.04.059
![]() |
[38] |
J. B. Vannier, G. Sarek, S. J. Boulton, RTEL1: functions of a disease-associated helicase, Trends Cell Biol., 24 (2014), 416-425. doi: 10.1016/j.tcb.2014.01.004
![]() |
[39] |
T. Wang, X. Li, S. L. Sun, EX527, a Sirt-1 inhibitor, induces apoptosis in glioma via activating the p53 signaling pathway, Anti-cancer Drugs, 31 (2020), 19-26. doi: 10.1097/CAD.0000000000000824
![]() |
[40] |
L. X. Xu, Z. H. Li, Y. F. Tao, R. H. Li, F. Fang, H. Zhao, et al., Histone acetyltransferase inhibitor Ⅱ induces apoptosis in glioma cell lines via the p53 signaling pathway, J. Exp. Clin. Cancer Res., 33 (2014), 1-15. doi: 10.1186/1756-9966-33-1
![]() |
[41] |
X. L. Zhang, X. T. Ji, B. Sun, L. L. Qian, X. L. Hu, H. X. Lou, et al., Anti-cancer effect of marchantin C via inducing lung cancer cellular senescence associated with less secretory phenotype, Biochim. Biophys. Acta Gen. Subj., 1863 (2019), 1443-1457. doi: 10.1016/j.bbagen.2019.05.006
![]() |
[42] |
J. Song, Q. Ma, M. Hu, D. Qian, B. Wang, N. He, The inhibition of miR-144-3p on cell proliferation and metastasis by targeting TOP2A in HCMV-positive glioblastoma cells, Molecules, 23 (2018), 3259. doi: 10.3390/molecules23123259
![]() |
[43] |
X. Li, E. Martinez-Ledesma, C. Zhang, F. Gao, S. Zheng, J. Ding, et al., TIE2-FGFR1 interaction induces adaptive PI3K inhibitor resistance by upregulating Aurora A/PlK1/CDK1 signaling in glioblastoma, Cancer Res., 79 (2019), 5088-5101. doi: 10.1158/0008-5472.CAN-19-0325
![]() |
[44] |
L. Xu, H. Liu, Z. Yan, Z. Sun, S. Luo, Q. Lu, Inhibition of the Hedgehog signaling pathway suppresses cell proliferation by regulating the Gli2/miR-124/AURKA axis in human glioma cells, Int. J. Oncol., 50 (2017), 1868-1878. doi: 10.3892/ijo.2017.3946
![]() |
[45] |
Y. Ding, S. Yu, Z. Bao, Y. Liu, T. Liang, CDC20 with malignant progression and poor prognosis of astrocytoma revealed by analysis on gene expression, J. Neurooncol., 133 (2017), 87-95. doi: 10.1007/s11060-017-2434-8
![]() |
[46] |
Y. Zhang, J. Li, K. Yi, J. Feng, Z. Cong, Z. Wang, et al., Elevated signature of a gene module coexpressed with CDC20 marks genomic instability in glioma, Proc. Natl. Acad. Sci., 116 (2019), 6975-6984. doi: 10.1073/pnas.1814060116
![]() |
[47] | Z. Song, Y. Pan, G. Ling, S. Wang, M. Huang, X. Jiang, et al., Escape of U251 glioma cells from temozolomide-induced senescence was modulated by CDK1/survivin signaling, Am. J. Transl. Res., 9 (2017), 2163-2180. |
[48] |
S. Deguchi, K. Katsushima, A. Hatanaka, K. Shinjo, F. Ohka, T. Wakabayashi, et al., Oncogenic effects of evolutionarily conserved noncoding RNA ECONEXIN on gliomagenesis, Oncogene, 36 (2017), 4629-4640. doi: 10.1038/onc.2017.88
![]() |
[49] |
M. Shahid, M. Y. Lee, H. Piplani, A. M. Andres, B. Zhou, A. Yeon, et al., Centromere protein F (CENPF), a microtubule binding protein, modulates cancer metabolism by regulating pyruvate kinase M2 phosphorylation signaling, Cell Cycle, 17 (2018), 2802-2818. doi: 10.1080/15384101.2018.1557496
![]() |
[50] |
M. Shahid, M. Kim, M. Y. Lee, A. Yeon, S. You, H. L. Kim, et al., Downregulation of CENPF remodels prostate cancer cells and alters cellular metabolism, Proteomics, 19 (2019), 1900038. doi: 10.1002/pmic.201900038
![]() |
[51] |
X. Yang, B. S. Miao, C. Y. Wei, R. Z. Dong, P. T. Gao, X. Y. Zhang, et al., Lymphoid-specific helicase promotes the growth and invasion of hepatocellular carcinoma by transcriptional regulation of centromere protein F expression, Cancer Sci., 110 (2019), 2133-2144. doi: 10.1111/cas.14037
![]() |
![]() |
![]() |
1. | Haibin Wu, Chengcheng Guo, Chaoye Wang, Jiang Xu, Suyue Zheng, Jian Duan, Yiyun Li, Hongming Bai, Qiuyan Xu, Fangling Ning, Feng Wang, Qunying Yang, Single‐cell RNA sequencing reveals tumor heterogeneity, microenvironment, and drug‐resistance mechanisms of recurrent glioblastoma , 2023, 1347-9032, 10.1111/cas.15773 | |
2. | Dan Yang, Jia Wang, Mingqiu Hu, Feng Li, Feifei Yang, Youcai Zhao, Yanli Xu, Xuezhong Zhang, Lijun Tang, Xiuqun Zhang, Combined multiomics analysis reveals the mechanism of CENPF overexpression-mediated immune dysfunction in diffuse large B-cell lymphoma in vitro, 2022, 13, 1664-8021, 10.3389/fgene.2022.1072689 | |
3. | Lei Cao, Xu Lu, Xia Wang, Hao Wu, Xiaye Miao, From single-cell to spatial transcriptomics: decoding the glioma stem cell niche and its clinical implications, 2024, 15, 1664-3224, 10.3389/fimmu.2024.1475235 | |
4. | Jianming Peng, Qing Zhang, Xiaofeng Zhu, Zhu Yan, Meng Zhu, Single-cell profiling uncovers proliferative cells as key determinants of survival outcomes in lower-grade glioma patients, 2024, 15, 2730-6011, 10.1007/s12672-024-01302-8 | |
5. | Sofia Martins, Roberta Coletti, Marta B. Lopes, Disclosing transcriptomics network-based signatures of glioma heterogeneity using sparse methods, 2023, 16, 1756-0381, 10.1186/s13040-023-00341-1 | |
6. | Jianfang Wang, Juntao Guo, Shengchen Yu, Hengwei Yu, Abebe Belete Kuraz, Diba Dedacha Jilo, Gong Cheng, Anning Li, Cunling Jia, Linsen Zan, Knockdown of NFIC Promotes Bovine Myoblast Proliferation through the CENPF/CDK1 Axis, 2024, 72, 0021-8561, 12641, 10.1021/acs.jafc.4c01811 | |
7. | Tathiane M. Malta, Thais S. Sabedot, Natalia S. Morosini, Indrani Datta, Luciano Garofano, Wies Vallentgoed, Frederick S. Varn, Kenneth Aldape, Fulvio D'Angelo, Spyridon Bakas, Jill S. Barnholtz-Sloan, Hui K. Gan, Mohammad Hasanain, Ann-Christin Hau, Kevin C. Johnson, Simona Cazacu, Ana C. deCarvalho, Mustafa Khasraw, Emre Kocakavuk, Mathilde C.M. Kouwenhoven, Simona Migliozzi, Simone P. Niclou, Johanna M. Niers, D. Ryan Ormond, Sun Ha Paek, Guido Reifenberger, Peter A. Sillevis Smitt, Marion Smits, Lucy F. Stead, Martin J. van den Bent, Erwin G. Van Meir, Annemiek Walenkamp, Tobias Weiss, Michael Weller, Bart A. Westerman, Bauke Ylstra, Pieter Wesseling, Anna Lasorella, Pim J. French, Laila M. Poisson, Adelheid Woehrer, Allison K Lowman, Ana C deCarvalho, Ana Valeria Castro, Andrea Transou, Andrew R Brodbelt, Ann-Christin Hau, Anna Lasorella, Anna Golebiewska, Annemiek Walenkamp, Annette M Molinaro, Antonio Iavarone, Azzam Ismail, Bart A Westerman, Bauke Ylstra, Christoph Bock, D. Ryan Ormond, Daniel J Brat, Emre Kocakavuk, Erwin G Van Meir, Floris P Barthel, Frederick S Varn, Fulvio D'Angelo, Gaetano Finocchiaro, Ganesh Rao, Gelareh Zadeh, Guido Reifenberger, Ho Keu ngNg, Hoon Kim, Houtan Noushmehr, Hrvoje Miletic, Hui K Gan, Indrani Datta, Jack Rock, James M Snyder, Jason T Huse, Jennifer M Connelly, Jill S Barnholtz-Sloan, Johanna M Niers, John F deGroot, Kadir C Akdemir, Kasthuri S Kannan, Keith L Ligon, Kenneth Aldape, Ketan R Bulsara, Kevin C Johnson, Kristin D Alfaro, Laila M Poisson, Luciano Garofano, Lucy F Stead, MacLean P Nasrallah, Marion Smits, Martin J van den Bent, Mathilde CM Kouwenhoven, Michael Weller, Mohammad Hasanain, Mustafa Khasraw, Peter V Gould, Peter A Sillevis Smitt, Peter S LaViolette, Philip D Tatman, Pieter Wesseling, Pim J French, Rameen Beroukhim, Roel G.W. Verhaak, Simona Migliozzi, Simone P Niclou, Spyridon Bakas, Steven Kalkanis, Sun Ha Paek, Susan C Short, Tabatabai Ghazaleh, Tathiane M Malta, Thais S Sabedot, Tobias Weiss, Tobias Walbert, Ujjwal Baid, Wies Vallentgoed, W. K. Alfred Yung, Roel G.W. Verhaak, Antonio Iavarone, Houtan Noushmehr, The Epigenetic Evolution of Glioma Is Determined by the IDH1 Mutation Status and Treatment Regimen, 2024, 84, 0008-5472, 741, 10.1158/0008-5472.CAN-23-2093 | |
8. | Hong-jie Wen, Shou-yan Zhu, Hua-gang Yang, Feng-yong Guo, Investigation on the molecular mechanism of SPA interference with osteogenic differentiation of bone marrow mesenchymal stem cells, 2024, 14, 2045-2322, 10.1038/s41598-024-66502-2 | |
9. | Zhiqiang Yi, Yanfei Jia, Runchun Lu, Chunwei Li, Long Wen, Xiangdong Yin, Junfei Yi, Liang Li, E2F1-driven CENPM expression promotes glycolytic reprogramming and tumorigenicity in glioblastoma, 2024, 41, 1573-6822, 10.1007/s10565-024-09945-7 | |
10. | Junhong Li, Moxuan Zhang, Qiang Sun, Xinglan Li, Fei Du, Yanhao Cheng, Shuzhi Li, Jian Zhang, CENPF interaction with PLA2G4A promotes glioma growth by modulating mTORC1 and NF-κB pathways, 2025, 25, 1475-2867, 10.1186/s12935-025-03700-6 | |
11. | Edgar G. Ordóñez-Rubiano, Nicolás Rincón-Arias, William J. Shelton, Andres F. Salazar, María Alejandra Sierra, Raphael Bertani, Diego F. Gómez-Amarillo, Fernando Hakim, Matías Baldoncini, César Payán-Gómez, Alba Lucia Cómbita, Sandra C. Ordonez-Rubiano, Rafael Parra-Medina, Current Applications of Single-Cell RNA Sequencing in Glioblastoma: A Scoping Review, 2025, 15, 2076-3425, 309, 10.3390/brainsci15030309 |
Catelogy | The rank method in cytoHubba | |||||
MCC | MNC | Degree | EPC | Closeness | Radiality | |
Gene symbol top 10 | CDCA5 | MYC | MYC | CCNA2 | MYC | MYC |
PBK | SNAP25 | SNAP25 | CDK1 | SNAP25 | SNAP25 | |
MELK | CDK1 | CDK1 | TOP2A | CDK1 | CENPF | |
CENPF | CCNA2 | CCNA2 | AURKA | AURKA | CDK1 | |
TOP2A | AURKA | AURKA | CDC20 | CENPF | AURKA | |
CDC20 | TOP2A | CDC20 | PBK | CDC20 | NES | |
CDK1 | CDC20 | TOP2A | CENPF | CCNA2 | CD44 | |
CCNA2 | CDKN3 | CDKN3 | CDKN3 | CD44 | CDC20 | |
AURKA | CENPF | CENPF | MYC | TOP2A | CDK4 | |
HJURP | PBK | CD44 | HJURP | CDKN3 | TOP2A | |
Selected genes were the overlap hub genes in the top 10 by six ranked methods respectively in cytoHubba. MCC: Maximal clique centrality; MNC: Maximum neighborhood component; Degree: Node connect degree; EPC: Edge percolated component. |
Clinicopathological Parameter, value | CENPF expression | ||||
N | Low expression (%) | High expression (%) | χ2 | P value | |
Gender | |||||
Male | 398 (57.4) | 206 (51.8) | 192 (48.2) | ||
Female | 295 (42.6) | 140 (47.5) | 155 (52.5) | 1.254 | 0.263 |
Age | |||||
≥ 43 | 349 (50.4) | 167 (47.9) | 182 (52.1) | ||
< 43 | 343 (49.5) | 179 (52.2) | 164 (47.8) | 1.301 | 0.254 |
Missing | 1 (0.1) | 0 (0.0) | 1 (100.0) | ||
WHO grade | |||||
II | 188 (27.1) | 137 (72.9) | 51 (27.1) | ||
III | 255 (36.8) | 124 (48.6) | 131 (51.4) | ||
IV | 249 (36.0) | 85 (34.1) | 164 (65.9) | 64.597 | < 0.001* |
Missing | 1 (0.1) | 0 (0.0) | 1 (100.0) | ||
IDH mutation | |||||
Wildtype | 286 (41.3) | 110 (38.5) | 176 (61.5) | ||
Mutant | 356 (51.4) | 188 (52.8) | 168 (47.2) | 13.126 | < 0.001* |
Missing | 51 (7.3) | 48 (94.1) | 3 (5.9) | ||
1p, 19q codeletion status | |||||
Non-codel | 478 (69.0) | 198 (41.4) | 280 (58.6) | ||
Codel | 145 (20.9) | 85 (58.6) | 60 (41.4) | 13.273 | < 0.001* |
Missing | 70 (10.1) | 63 (90.0) | 7 (10.0) | ||
Recurrent | |||||
Yes | 271 (39.1) | 98 (36.2) | 173 (63.8) | ||
No | 422 (60.9) | 248 (58.8) | 174 (41.2) | 33.731 | < 0.001* |
Radiotherapy status | |||||
Yes | 509 (73.5) | 246 (48.3) | 263 (51.7) | ||
No | 113 (16.3) | 72 (63.7) | 41 (36.3) | 8.762 | 0.003* |
Missing | 71 (10.2) | 28 (39.4) | 43 (60.6) | ||
Chemotherapy status | |||||
Yes | 457 (65.9) | 209 (45.7) | 248 (54.3) | ||
No | 151 (21.8) | 100 (66.2) | 51 (33.8) | 19.07 | < 0.001* |
Missing | 85 (12.2) | 37 (43.5) | 48 (56.5) |
Parameter | Univariate analysis | Multivariate analysis | ||||
P-value | HR | 95% CI | P-value | HR | 95% CI | |
Gender(Female vs. male) | 0.907 | 1.012 | 0.826-1.241 | 0.100 | 1.226 | 0.962-1.562 |
Age (years)(≧ 43 vs. < 43) | < 0.001* | 1.732 | 1.411-2.125 | 0.001* | 1.474 | 1.161-1.873 |
WHO grade(II vs. III vs. IV) | < 0.001* | 2.740 | 2.359-3.182 | < 0.001* | 2.128 | 1.719-2.636 |
IDH mutation(Wildtype vs. Mutant) | < 0.001* | 3.237 | 2.615-4.008 | < 0.001* | 1.749 | 1.318-2.319 |
1p, 19q codeletion status(Non-codel vs. Codel) | < 0.001* | 0.263 | 0.187-0.369 | < 0.001* | 0.439 | 0.292-0.659 |
Recurrent(Primary vs. Recurrent) | < 0.001* | 2.089 | 1.702-2.564 | < 0.001* | 2.444 | 1.924-3.103 |
Radiotherapy status(Yes vs. No) | 0.020 | 1.430 | 1.057-1.935 | 0.333 | 0.829 | 0.568-1.212 |
Chemotherapy status(Yes vs. No) | 0.003 | 1.506 | 1.154-1.966 | 0.010 | 0.635 | 0.449-0.899 |
CENPF expression(high expression vs. low expression) | < 0.001* | 2.942 | 2.374-3.645 | < 0.001* | 2.403 | 1.736-3.325 |
CDC20 expression(high expression vs. low expression) | < 0.001* | 2.536 | 2.054-3.131 | 0.920 | 0.982 | 0.688-1.401 |
TOP2A expression(high expression vs. low expression) | < 0.001* | 2.142 | 1.739-2.639 | 0.617 | 1.097 | 0.763-1.576 |
ID | Platform | Sample | References |
GSE108476 | GPL570 | GBM = 221, NB = 28 | [26] |
GSE50161 | GPL570 | GBM = 34, NB = 13 | [27] |
GSE116520 | GPL10558 | GBM = 17, NB = 8 | [28] |
GBM, glioblastoma multiforme; NB, normal brain. |
Catelogy | The rank method in cytoHubba | |||||
MCC | MNC | Degree | EPC | Closeness | Radiality | |
Gene symbol top 10 | CDCA5 | MYC | MYC | CCNA2 | MYC | MYC |
PBK | SNAP25 | SNAP25 | CDK1 | SNAP25 | SNAP25 | |
MELK | CDK1 | CDK1 | TOP2A | CDK1 | CENPF | |
CENPF | CCNA2 | CCNA2 | AURKA | AURKA | CDK1 | |
TOP2A | AURKA | AURKA | CDC20 | CENPF | AURKA | |
CDC20 | TOP2A | CDC20 | PBK | CDC20 | NES | |
CDK1 | CDC20 | TOP2A | CENPF | CCNA2 | CD44 | |
CCNA2 | CDKN3 | CDKN3 | CDKN3 | CD44 | CDC20 | |
AURKA | CENPF | CENPF | MYC | TOP2A | CDK4 | |
HJURP | PBK | CD44 | HJURP | CDKN3 | TOP2A | |
Selected genes were the overlap hub genes in the top 10 by six ranked methods respectively in cytoHubba. MCC: Maximal clique centrality; MNC: Maximum neighborhood component; Degree: Node connect degree; EPC: Edge percolated component. |
Clinicopathological Parameter, value | CENPF expression | ||||
N | Low expression (%) | High expression (%) | χ2 | P value | |
Gender | |||||
Male | 398 (57.4) | 206 (51.8) | 192 (48.2) | ||
Female | 295 (42.6) | 140 (47.5) | 155 (52.5) | 1.254 | 0.263 |
Age | |||||
≥ 43 | 349 (50.4) | 167 (47.9) | 182 (52.1) | ||
< 43 | 343 (49.5) | 179 (52.2) | 164 (47.8) | 1.301 | 0.254 |
Missing | 1 (0.1) | 0 (0.0) | 1 (100.0) | ||
WHO grade | |||||
II | 188 (27.1) | 137 (72.9) | 51 (27.1) | ||
III | 255 (36.8) | 124 (48.6) | 131 (51.4) | ||
IV | 249 (36.0) | 85 (34.1) | 164 (65.9) | 64.597 | < 0.001* |
Missing | 1 (0.1) | 0 (0.0) | 1 (100.0) | ||
IDH mutation | |||||
Wildtype | 286 (41.3) | 110 (38.5) | 176 (61.5) | ||
Mutant | 356 (51.4) | 188 (52.8) | 168 (47.2) | 13.126 | < 0.001* |
Missing | 51 (7.3) | 48 (94.1) | 3 (5.9) | ||
1p, 19q codeletion status | |||||
Non-codel | 478 (69.0) | 198 (41.4) | 280 (58.6) | ||
Codel | 145 (20.9) | 85 (58.6) | 60 (41.4) | 13.273 | < 0.001* |
Missing | 70 (10.1) | 63 (90.0) | 7 (10.0) | ||
Recurrent | |||||
Yes | 271 (39.1) | 98 (36.2) | 173 (63.8) | ||
No | 422 (60.9) | 248 (58.8) | 174 (41.2) | 33.731 | < 0.001* |
Radiotherapy status | |||||
Yes | 509 (73.5) | 246 (48.3) | 263 (51.7) | ||
No | 113 (16.3) | 72 (63.7) | 41 (36.3) | 8.762 | 0.003* |
Missing | 71 (10.2) | 28 (39.4) | 43 (60.6) | ||
Chemotherapy status | |||||
Yes | 457 (65.9) | 209 (45.7) | 248 (54.3) | ||
No | 151 (21.8) | 100 (66.2) | 51 (33.8) | 19.07 | < 0.001* |
Missing | 85 (12.2) | 37 (43.5) | 48 (56.5) |
Parameter | Univariate analysis | Multivariate analysis | ||||
P-value | HR | 95% CI | P-value | HR | 95% CI | |
Gender(Female vs. male) | 0.907 | 1.012 | 0.826-1.241 | 0.100 | 1.226 | 0.962-1.562 |
Age (years)(≧ 43 vs. < 43) | < 0.001* | 1.732 | 1.411-2.125 | 0.001* | 1.474 | 1.161-1.873 |
WHO grade(II vs. III vs. IV) | < 0.001* | 2.740 | 2.359-3.182 | < 0.001* | 2.128 | 1.719-2.636 |
IDH mutation(Wildtype vs. Mutant) | < 0.001* | 3.237 | 2.615-4.008 | < 0.001* | 1.749 | 1.318-2.319 |
1p, 19q codeletion status(Non-codel vs. Codel) | < 0.001* | 0.263 | 0.187-0.369 | < 0.001* | 0.439 | 0.292-0.659 |
Recurrent(Primary vs. Recurrent) | < 0.001* | 2.089 | 1.702-2.564 | < 0.001* | 2.444 | 1.924-3.103 |
Radiotherapy status(Yes vs. No) | 0.020 | 1.430 | 1.057-1.935 | 0.333 | 0.829 | 0.568-1.212 |
Chemotherapy status(Yes vs. No) | 0.003 | 1.506 | 1.154-1.966 | 0.010 | 0.635 | 0.449-0.899 |
CENPF expression(high expression vs. low expression) | < 0.001* | 2.942 | 2.374-3.645 | < 0.001* | 2.403 | 1.736-3.325 |
CDC20 expression(high expression vs. low expression) | < 0.001* | 2.536 | 2.054-3.131 | 0.920 | 0.982 | 0.688-1.401 |
TOP2A expression(high expression vs. low expression) | < 0.001* | 2.142 | 1.739-2.639 | 0.617 | 1.097 | 0.763-1.576 |