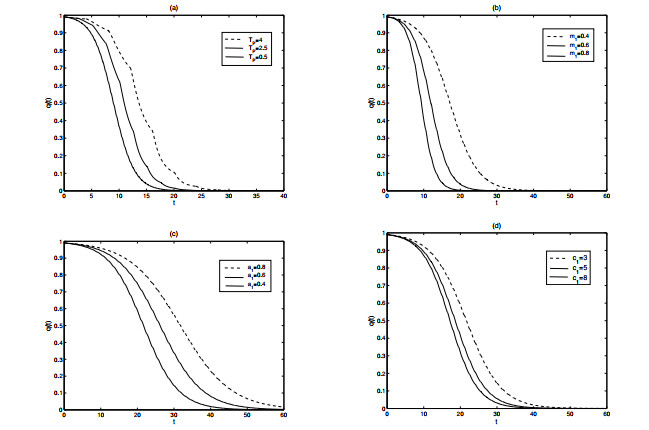
The complexity of biological systems suggests that current definitions of molecular dysfunctions are essential distinctions of a complex phenotype. This is well seen in neurodegenerative diseases (ND), such as Alzheimer's disease (AD) and Parkinson's disease (PD), multi-factorial pathologies characterized by high heterogeneity. These challenges make it necessary to understand the effectiveness of candidate biomarkers for early diagnosis, as well as to obtain a comprehensive mapping of how selective treatment alters the progression of the disorder. A large number of computational methods have been developed to explain network-based approaches by integrating individual components for modeling a complex system. In this review, high-throughput omics methodologies are presented for the identification of potent biomarkers associated with AD and PD pathogenesis as well as for monitoring the response of dysfunctional molecular pathways incorporating multilevel clinical information. In addition, principles for efficient data analysis pipelines are being discussed that can help address current limitations during the experimental process by increasing the reproducibility of benchmarking studies.
Citation: Marios G. Krokidis, Themis P. Exarchos, Panagiotis Vlamos. Data-driven biomarker analysis using computational omics approaches to assess neurodegenerative disease progression[J]. Mathematical Biosciences and Engineering, 2021, 18(2): 1813-1832. doi: 10.3934/mbe.2021094
[1] | Wenjie Qin, Yue Xia, Yi Yang . An eco-epidemic model for assessing the application of integrated pest management strategies. Mathematical Biosciences and Engineering, 2023, 20(9): 16506-16527. doi: 10.3934/mbe.2023736 |
[2] | Yuan Tian, Sanyi Tang . Dynamics of a density-dependent predator-prey biological system with nonlinear impulsive control. Mathematical Biosciences and Engineering, 2021, 18(6): 7318-7343. doi: 10.3934/mbe.2021362 |
[3] | Liping Wu, Zhongyi Xiang . A study of integrated pest management models with instantaneous and non-instantaneous impulse effects. Mathematical Biosciences and Engineering, 2024, 21(2): 3063-3094. doi: 10.3934/mbe.2024136 |
[4] | Yanyun Li, Juhua Liang . Models for determining the optimal switching time in chemical control of pest with pesticide resistance. Mathematical Biosciences and Engineering, 2021, 18(1): 471-494. doi: 10.3934/mbe.2021026 |
[5] | Yi Yang, Lirong Liu, Changcheng Xiang, Wenjie Qin . Switching dynamics analysis of forest-pest model describing effects of external periodic disturbance. Mathematical Biosciences and Engineering, 2020, 17(4): 4328-4347. doi: 10.3934/mbe.2020239 |
[6] | Xinli Hu, Wenjie Qin, Marco Tosato . Complexity dynamics and simulations in a discrete switching ecosystem induced by an intermittent threshold control strategy. Mathematical Biosciences and Engineering, 2020, 17(3): 2164-2178. doi: 10.3934/mbe.2020115 |
[7] | Bruno Buonomo , Francesco Giannino , Stéphanie Saussure , Ezio Venturino . Effects of limited volatiles release by plants in tritrophic interactions. Mathematical Biosciences and Engineering, 2019, 16(5): 3331-3344. doi: 10.3934/mbe.2019166 |
[8] | Baolin Kang, Xiang Hou, Bing Liu . Threshold control strategy for a Filippov model with group defense of pests and a constant-rate release of natural enemies. Mathematical Biosciences and Engineering, 2023, 20(7): 12076-12092. doi: 10.3934/mbe.2023537 |
[9] | Hao Zhou, Xia Wang, Sanyi Tang . Global dynamics of non-smooth Filippov pest-natural enemy system with constant releasing rate. Mathematical Biosciences and Engineering, 2019, 16(6): 7327-7361. doi: 10.3934/mbe.2019366 |
[10] | Luis F. Gordillo . Optimal sterile insect release for area-wide integrated pest management in a density regulated pest population. Mathematical Biosciences and Engineering, 2014, 11(3): 511-521. doi: 10.3934/mbe.2014.11.511 |
The complexity of biological systems suggests that current definitions of molecular dysfunctions are essential distinctions of a complex phenotype. This is well seen in neurodegenerative diseases (ND), such as Alzheimer's disease (AD) and Parkinson's disease (PD), multi-factorial pathologies characterized by high heterogeneity. These challenges make it necessary to understand the effectiveness of candidate biomarkers for early diagnosis, as well as to obtain a comprehensive mapping of how selective treatment alters the progression of the disorder. A large number of computational methods have been developed to explain network-based approaches by integrating individual components for modeling a complex system. In this review, high-throughput omics methodologies are presented for the identification of potent biomarkers associated with AD and PD pathogenesis as well as for monitoring the response of dysfunctional molecular pathways incorporating multilevel clinical information. In addition, principles for efficient data analysis pipelines are being discussed that can help address current limitations during the experimental process by increasing the reproducibility of benchmarking studies.
As we all know, crop pests have been the biggest obstacle to agricultural development. The existence of pests will not only reduce the yield of crops but also affect their quality, resulting in economic losses. Therefore, the control of pests is an important research subject in agriculture all over the world.
The most common method of pest control is spraying pesticide. Its advantages are convenient and efficient, and the effects on pests can be seen in a short time. However, with a large amount of pesticide use, people have to face many serious problems, such as the development of pest resistance, reduction of beneficial organisms, the resurgence of major pests, the outbreak of secondary pests, residues in food, environmental pollution and so on. Faced with these serious consequences, people have begun to explore new pest control methods. Biological control is also an important method to control pests [1,2,3]. In augmentative biological control, natural enemies are mass-reared in biofactories for release in certain numbers to obtain immediate control of pests, which can not only control pests but also reduce environmental pollution [4,5]. According to statistics, there are about 500 kinds of pests in the world controlled by imported natural enemies, and at least 300 kinds of pests have been effectively controlled. However, natural enemies often need to breed in captivity, and it is costly. Therefore, people often combine these two methods, that is, adopt integrated pest management (IPM) [3,6]. In the process of IPM, economic threshold (ET) and economic injury level (EIL) are two important concepts. EIL is defined as the lowest pest density which will cause economic damage, and ET is defined as the pest density at which control action should be taken to prevent pests from reaching the EIL [6,7]. More realistically, we need to control the density of the pest population below EIL rather than to make them extinct.
Based on the above biological background, many scholars used mathematical models to simulate the process of spraying pesticide and releasing natural enemies, including impulsive differential equations at the fixed time [8,9,10,11,12,13,14] or state-dependent [6,15,16,17,18] and Filippov switching systems [7,19,20,21,22,23], etc. These studies assumed that the pesticides acted in a way that killed pests instantaneously and proportionately. However, studies have shown that the effects of pesticides on pests are not always instantaneous, but have certain delayed responses. In many cases, the pesticides may remain on or in a crop or pest after they have been treated [25,26], which indicates that the pesticides have residual and delayed effects on the process of pest control. So the density of pest population could increase for a time even after the pesticide application has been made before it decreases. A more realistic method of modelling pesticide action is to assume that the effects of pesticide are simulated by continuous or piecewise-continuous periodic functions which affect the growth rate in the Logistic growth model [27,28]. For example, Liang et al. [27] developed mathematical models to evaluate the residual and delayed effects on an integrated pest management strategy which combined piecewise-continuous periodic functions for chemical control with pulse actions for releasing natural enemies.
In previous studies, most researchers also ignored the development of pest resistance. Pesticide control in the initial phase has very good effects. However, in agricultural production, due to the improper applications of pesticides and the long-term use of the single pesticide in the same area, pests are prone to developing resistance to pesticide [29,30,31,32], which eventually loses its effects. Therefore, to reduce or delay the development of pest resistance and make the best use of pesticides, we should avoid unnecessary pesticide applications or switch to apply different types of pesticides [29]. Once pest resistance has developed, we could also use natural enemies to suppress the outbreaks of pests. The release amount of natural enemies needed to be adjusted according to the evolution of pesticide resistance. If the released amount was too large, then it was not cost-effective and may cause secondary outbreaks or pest resurgence [30].
Considering the residual and delayed effects of pesticide and pest resistance to pesticide, one purpose of this paper is to use a mathematical model to help determine how the spraying frequency of pesticides and the residual and delayed effects of pesticide affect the results of pest control in the process of integrated pest management. Another purpose of this paper is how we can take effective strategies to reduce or delay the development of the pest resistance or adjust the release amount of natural enemies to the extent that the pests become extinct or do not exceed the EIL when pests develop resistance.
The organization of this paper is as follows. A hybrid dynamical model is established to simulate the process of integrated pest management in section 2. It assumes that spraying pesticide is more frequently used than releasing natural enemies. A piecewise-continuous periodic function to model the residual and delayed effects of pesticides on pests and natural enemies and the development of pest resistance to pesticides are introduced into the pest control model. In section 3, the threshold condition for pest-eradication is obtained, and we compare the integrated pest management strategy with any single control strategy. In section 4, the effects of chemical control factors on the threshold are analysed, including the frequency of spraying pesticide, the killing efficiency rate of pesticide to pests, the decay rate of pesticide to pests, and the delayed response rate on pests. In section 5, we give three different control strategies to reduce or delay the development of pest resistance and control the outbreaks of pest, including switching pesticides strategy and strategy for releasing natural enemies elastically for the pest-eradication, and the state feedback strategy for controlling pests not exceeding the EIL. Finally, a brief discussion is given in the last section.
To address the effects of integrated control tactics on the pest-natural enemy dynamic model and consider the effects of residual pesticide effects and delayed responses to the pesticide on successful pest control, Liang et al. [27] proposed the following pest-natural enemy model with residual pesticidal actions and delayed responses to the pesticide applications.
{dx(t)dt=rx(t)(1−x(t)K)−b1(t)x(t)−αx(t)y(t),dy(t)dt=λαx(t)y(t)−dy(t)−b2(t)y(t)),}t≠nT,x(t+)=x(t),y(t+)=y(t)+μ,}t=nT, | (2.1) |
where x(t) and y(t) represent the densities of the pests and natural enemies at time t, respectively; r>0 is the intrinsic growth rate of the pest population; K>0 is the carrying capacity of the pest population; α>0 is the predation rate per natural enemy on pests; λ>0 denotes the effective conversion rate of natural enemies; d>0 denotes the death rate of the natural enemies; μ>0 is the release amount of natural enemies at time t=nT; bi(t)(i=1,2) refers to the effects of pesticide on pests and natural enemies, respectively.
In this paper, we suppose that pesticides are applied more frequently than natural enemies release. In any interval [nT,(n+1)T), we spray pesticides p times periodically at t=nT+kTP(k=0,1,2,⋯p−1), and take on the following exponentially decaying piecewise periodic function:
bi(t)=mi(e−ai(t−(nT+kTP))−e−ci(t−(nT+kTp)),nT+kTP≤t<nT+(k+1)TP, | (2.2) |
where mi≥0(i=1,2) represents the the killing efficiency rate of pesticide to pests and natural enemies, respectively; ai(i=1,2) represents the positive decay rate of pesticide to pests and natural enemies, respectively; ci(i=1,2) is the delayed response rate with ci>ai>0(i=1,2).
The model established by Liang et al. [27], as quoted above, did not consider pest resistance to pesticides. In this paper, based on the above model, we assume that pests population x is made up of two parts: sensitive to pesticides (denoted by xs) and resistant to pesticides, that is, pesticides have no effects on them (denoted by xr), and q(t) refers to the proportion of sensitive pests at time t (i.e. q(t)=xs/x), which changes over time, then 1−q(t) is the proportion of resistant pests. Let q(0)=q0 denotes the proportion of sensitive pests at the initial time. Then xs(t) and xr(t) meet the following equations:
{dxs(t)dt=rxs(t)(1−x(t)K)−b1(t)xs(t)−αxs(t)y(t),dxr(t)dt=rxr(t)(1−x(t)K)−αxr(t)y(t), | (2.3) |
and we have
dx(t)dt=rx(t)(1−x(t)K)−b1(t)q(t)x(t)−αx(t)y(t). |
Since q(t)=xs/x, by simple calculations, we obtain
dq(t)dt=b1(t)q(t)(q(t)−1). | (2.4) |
Then we set up the following hybrid pest control model with resistance evolution:
{dx(t)dt=rx(t)(1−x(t)K)−b1(t)q(t)x(t)−αx(t)y(t),dy(t)dt=λαx(t)y(t)−dy(t)−b2(t)y(t)),}t≠nT,x(t+)=x(t),y(t+)=y(t)+μ,}t=nT,dq(t)dt=b1(t)q(t)(q(t)−1), | (2.5) |
where n∈N={0,1,2,...}, and the implications of all other parameters are the same with those in model (2.1), and q(t) satisfies equation (2.4).
By calculations, the analytic solution of q(t) at interval [nT,(n+1)T)(n∈N) is
q(t)=q(nT+kTP)q(nT+kTP)+(1−q(nT+kTP))exp(m1a1(1−e−a1(t−(nT+kTP)))−m1c1(1−e−c1(t−(nT+kTP)))), |
where
q(nT+kTP)=q0q0+(1−q0)exp((np+k)(m1a1(1−e−a1TP)−m1c1(1−e−c1TP))). | (2.6) |
To illustrate the effects of spraying period TP, the killing efficiency rate m1, the decay rate a1 and the delayed response rate c1 on the development of pest resistance, we simulate the changing graph q(t) with time t, respectively. The results indicate that shortening the period of spraying pesticide, increasing the killing efficiency rate, small decay rate and large delayed response rate could result in the development of pest resistance (see Figure 1).
Firstly, we consider the following subsystems of system (2.5):
{dy(t)dt=−(d+b2(t)y(t),t≠nT,y(nT+)=y(nT)+μ,t=nT, | (3.1) |
Similar to the proof of Appendix B in [27], the following theorem can be obtained:
Theorem 3.1 System (3.1) has a unique globally asymptotically stable positive T-periodic solution ˜y(t), and for any solution y(t) of system (3.1), we have y(t)→˜y(t) as t→∞.
where,
˜y(t)=y∗exp(∫tnT−(d+b2(s))ds),t∈[nT,(n+1)T), | (3.2) |
y∗=μ1−exp(−dT−m2p(1a2(1−e−a2Tp)−1c2(1−e−c2Tp)). | (3.3) |
Therefore, in any given time interval [nT,(n+1)T), there exists a pest-eradication periodic solution (0,˜y(t)) of system (2.5).
Denote
R0(n,T)=exp(∫(n+1)TnT(r−b1(t)q(t))dt), |
M(T)=exp(∫T0(−α˜y(t))dt), |
R1(n,T)=R0(n,T)M(T), |
we have,
Theorem 3.2 If R1(n,T)<1 holds, then the pest-eradication periodic solution (0,˜y(t)) of system (2.5) is globally attractive.
The proof of Theorem 3.2 is given in Appendix A.
By direct calculation, we obtain
R0(n,T)=erTexp∫(n+1)TnT(−b1(t)q(t))dt=erTp−1∏k=0[(q(nT+kTp)e(m1a1e−a1Tp−m1c1e−c1Tp)+(1−q(nT+kTp)e(m1a1−m1c1)]e−(m1a1−m1c1), |
where q(nT+kTp) is given in (2.6).
M(T)=exp(∫T0(−α˜y(t))dt)=exp(−αy∗(p−1∑k=0∫(k+1)⋅Tpk⋅Tpexp(−dt−(km2a2(1−e−a2Tp)−km2c2(1−e−c2Tp))−(m2a2(1−e−a2(t−kTp))−m2c2(1−e−c2(t−kTp))))dt)), |
where y∗ is given in (3.3). From above we can get the exact expression of R1(n,T).
Remark 3.1 The first part R0(n,T) of R1(n,T) shows the effects of the development of pest resistance on the threshold; the second part M(TN) reflects the effects of natural enemies on pests control, including the predation rate α, the impulsive release period T, the release amount μ. Without biological control, the threshold condition of pest eradication becomes R0(n,T), and it is clear that R1(n,T)<R0(n,T). Since q(t) is decreasing with time t, R0(n,T) and R1(n,T) are increasing functions with respect to n, and R1(n,T) tends to erTM(T) (see Figure 2(a)), which means that at last chemical control does not work and natural enemies are killed. So in the short term, the integrated pest control strategy works better than chemical and biological control. Still, pests eventually break out because of pest resistance to pesticides (see Figure 2(b)). Therefore, we need to take more effective measures to control pests.
What is interesting here is how the control strategy affects the threshold R1(h,TN), and consequently on the success of pest control.
From the expression of R1(n,T), we know ∂R1(n,T)∂μ<0, which implies that the larger the release amount μ of natural enemies, the smaller the threshold R1(n,T), and then the pest population can be more easily controlled by biological control applications. In the following, we focus on the effects of chemical control factors on the R1(n,T), including the frequency p of spraying pesticide, the killing efficiency rate m1 of pesticide to pests, the decay rate a1 of pesticide to pests, and the delayed response rate c1 on pests.
First of all, we want to know if it's better to spray pesticide as often as possible during the period of releasing natural enemies. In Figure 3 (a)–(d), we fix all parameters except for the period T, parameters which describe the residual and delayed effects of pesticides on pest (m1,a1 and c1), and choose different spraying pesticide frequencies, respectively. It shows that the minimum value of the threshold R1(n,T) depends on the above key parameters. When the pesticide has a weak effect on the pests, the pest resistance develops slowly and the threshold R1(n,T) decreases with the increase of frequency p. The more frequently the pesticide is sprayed, the better the pest control will be (see Figure 3(a) T=1.7, Figure 3(b) m1=0.4, Figure 3(c) a1=1.2, Figure 3(d) c1=0.3); when the pesticide effect increases to a certain extent, the resistance of pests gradually develops. The threshold R1(n,T) is not a monotonous function, and in this case there is an optimal spraying frequency to control pests (see Figure 3(a) T=1, Figure 3(b) m1=0.8, Figure 3(c) a1=0.8, Figure 3(d) c1=0.7). When the pesticide has a strong effect on the pests, the pest resistance develops rapidly. With the increase of p, R1(n,T) increases, which indicates spraying pesticides frequently is not conducive to pest control, and repeated use of the same pesticide can cause revival of the pests (see Figure 3(a) T=0.6, Figure 3(b) m1=1.1, Figure 3(c) a1=0.4, Figure 3(d) c1=1.2). Therefore, in the process of pest control, due to the development of pest resistance, we should carefully choose the frequency of spraying pesticide, not that the more frequently pesticides are sprayed, the better the pest control is.
Next, the effects of other chemical control factors to pests on the threshold R1(n,T) are considered. In Figures 4 and 5, we simulate the contours of R1(n,T) with respect to m1, a1 and c1, respectively. It can be seen that in the early stages of pest control (h=4, see Figure 4(a) and Figure 5(a)), pest resistance has not yet developed, so R1(n,T) decreases with the increase of m1 or c1 and increases with the increase of a1, which implies that strong effects of pesticides are helpful to control pests at the beginning of pest control. However, with time passing (see Figure 5(b) and Figure 6(b)), R1(n,T) is no longer a monotone function with respect to m1, a1 and c1. For higher killing efficiency rate m1, lower decay rate a1 and larger delayed response rate c1, repeated use of the same insecticide causes the pest to develop resistance rapidly. The strong effects of pesticides lead to the increase of R1(n,T), which are not better for pest control. Therefore, for the long term of pest control, we should be careful in choosing pesticides, not that the stronger effect of pesticide, the better the pest control.
From the analysis in section 3, we know that R1(n,T) is a monotonic increasing function concerning n. At first, R1(n,T)<1, under the effects of biological and chemical control, the density of pest populations decrease rapidly. However, with time passing, pests gradually develop resistance to pesticide, R1(n,T) will be greater than 1, and the density of pests population will increase gradually after decreasing to a certain level. Pests will eventually break out (see Figure 6(b), dashed line). Therefore, to reduce or delay the development of pest resistance and control the outbreaks of pest, effective measures must be taken.
To avoid the development of the pest resistance, the most common method used by farmers is to switch to another pesticide with different action mechanisms to achieve the purpose of pest control. Then how to choose the switching time is an important question. To answer this question, based on model (2.5) we set up the following pest control model with resistance development and switching pesticides. Here, for the sake of convenience, it assumes that each pesticide has the same strength of action and the proportion q0 of sensitive pests at the initial time is also the same.
{dx(t)dt=rx(t)(1−x(t)K)−b1(t)q(t)x(t)−αx(t)y(t),dy(t)dt=λαx(t)y(t)−dy(t)−b2(t)y(t)),}t≠nT,x(t+)=x(t),y(t+)=y(t)+μ,}t=nT,dq(t)dt=b1(t)q(t)(q(t)−1),t≠hTN,q(t+)=q0,t=hTN, | (5.1) |
where, n∈N,h∈N, and we assume that each pesticide is applied for ω periods of releasing natural enemies, that is, TN=ωT,ω∈N+={1,2,⋯,}.
Denote
R2(ω,T)=R1(1,T)R1(2,T)…R1(ω,T) |
we have the following threshold for eradication of pests,
Theorem 5.1 If R2(ω,T)<1 holds, then the pest-eradication periodic solution (0,˜y(t)) of System (5.1) is globally attractive.
The proof of Theorem 5.1 is similar to the proof of Theorem 3.2, here we omit it.
Now we determine the switching time for each pesticide. First, take the threshold R2(ω,T) as a guide. Once the threshold R2(ω,T) reaches one for the first time, we switch to another new type of pesticide. Select the parameters in Figure 6(a), we find that R1(ω,T) reaches one for the first time after 15 periods of releasing natural enemies. So we can switch to another kind of pesticide after 45 pesticide applications for each pesticide. By switching pesticides, the pests are finally controlled (see Figure 6(b), thin line). We can also take the threshold R1(n,T) as a guide for switching pesticides. Once the threshold R1(n,T) reaches one for the first time, we switch to another pesticide, then from Figure 6(a) we can see that after 9 periods R1(n,T) reaches one for the first time. So by switching to another pesticide after 27 applications for each pesticide, the pests tend to be extinct (see Figure 6(b), bold line). Therefore, taking threshold R2(ω,T) as a guide for switching pesticides, each pesticide can be used more often, and we can also achieve the purpose of pest control.
As previous analysis indicates, if we take chemical control only or integrated pest control but release a constant amount of natural enemies periodically described by the model (2.5), then pests eventually break out because of pest resistance to pesticides (see Figure 7(b) dashed line and thin line, respectively). To reduce or delay the increase of pest resistance, the frequency and dosage of pesticide should be reduced as much as possible, and more biological control should be taken. So if the pesticide spraying pattern is not changed, the release amount μ of natural enemies is no longer constant, but elastic with the increase of n to adapt to the development of the pest resistance and ensure that the threshold R1(n,T) is always less than 1, and the pests will be eradicated. Then the question is how to determine the release amount of natural enemies?
To achieve this goal, we choose a constant RC<1 and assume that the release amount of natural enemies is μn at time t=nT. Let
R1(n,T)=R0(n,T)M(T)=R0(n,T)exp(∫T0(−αy∗B(t))dt)≐RC |
and
B(t)=exp∫t0(−d+b2(s))ds), |
then we have,
μn=−1−exp(−dT−m2p(1a2(1−e−a2Tp)−1c2(1−e−c2Tp))α∫T0B(t)dtlnRCR0(n,T). | (5.2) |
Our ultimate goal is to control outbreaks of pests. If R1(n,T)≤RC, we choose μn=0. Otherwise, if R1(n,T)>RC, chemical control can no longer control pests, and natural enemies should be released. At t=nT, the release amount μn of natural enemies is determined by equation (13) which guarantees that R1(n,T)=RC<1, and the pests will eventually become extinct. Taking the parameters in Figure 7 as an example, set RC=0.96. If we choose μn as Table 1, then we can maintain R1(n,T) to RC (see Figure 7(a)), accordingly pests tend to be eradicated (see Figure 7(b), bold line).
Time nT of the releasing natural enemies | The releasing amount un of natural enemies |
T | 0.038 |
2T | 0.0385 |
3T | 0.039 |
4T | 0.0396 |
5T | 0.04 |
6T | 0.0405 |
7T | 0.041 |
8T | 0.049 |
9T | 0.0429 |
10T | 0.044 |
11T | 0.0454 |
12T | 0.047 |
13T | 0.049 |
14T | 0.0513 |
15T | 0.054 |
16T | 0.0567 |
17T | 0.06 |
18T | 0.0635 |
19T | 0.0675 |
20T | 0.0716 |
21T | 0.076 |
22T | 0.08 |
23T | 0.0846 |
24T | 0.089 |
In the first two strategies, we are more concerned about how to take effective measures to eradicate pests. However, ecologically and economically, the strategy of eradicating pests is not desirable because the right amount of pests is beneficial for the protection of natural enemies and the maintenance of economic compensation for crops. Therefore, a more reasonable pest control strategy is to take control measures when the density of pests reaches ET to prevent the density of pests from increasing to the EIL. This strategy maintains the sensitivity of pests to pesticides. It avoids the development of pest resistance caused by excessive pesticide use and the adverse effects of pesticides on the environment and natural enemies, here we call it state feedback control.
In this subsection, it is assumed that the integrated pest control strategy is applied when the pest density increases and reaches ET, and corresponding time series are τ1,τ2,…, τ1<τ2<…<τj<τj+1<…, then x(τj)=ET for j=1,2,…. By these assumptions, the following integrated pest management model with ET and resistance development is established.
{dx(t)dt=rx(t)(1−x(t)K)−b1(t)q(t)x(t)−αx(t)y(t),dy(t)dt=λαx(t)y(t)−dy(t)−b2(t)y(t)),}t≠τj,x(t+)=x(t),y(t+)=y(t)+μ,}t=τj,dq(t)dt=b1(t)q(t)(q(t)−1),bi(t)=mi(e−ai(t−τj)−e−ci(t−τj)),i=1,2,τj≤t<τj+1,j∈N+, | (5.3) |
where the initial values are x(0)=x0<ET,y(0)=y0,q(0)=q0, the biological meanings of other parameters are identical to those in model (2.5).
It is very difficult to investigate model (5.3) theoretically, as we can not determine the exact time of τj,j=1,2,…, and the times of pesticide applications for any solution with initial value (x0,y0). For simplification of analysis, we assume that the initial value x0 of the density of pests is ET. In the following, we turn to numerical simulations to investigate how this control strategy affects the success of the pest control within a given time.
From Figure 8(a), for a given time t∈[0,200], we can see that if we don't take any pest control strategy, pest populations will eventually break out and exceed the EIL (see Figure 8(a), thin blue line). So the state feedback strategy based on model (5.3) is applied once the pest population increases and reaches ET=0.6. The density of the pest population can be eventually maintained below the EIL after the integrated pest control strategy is applied for 13 times, but exceeds the ET and oscillates periodically (see Figure 8, bold black line). If we take periodically pest control strategy based on model (2.5), although we can control the pests below EIL, we apply the integrated control strategy for 50 times (see Figure 8(b), thin red line). Therefore, from the point of reality, the state feedback control strategy is more desirable, saves natural enemies and causes less harm to the environment.
Next, we investigate the effects of the release amount of natural enemies on this feedback control strategy. We denote Δτj=τj−τj−1,j=1,2,…,n with τ0=0, where n denotes the maximum number at which the pest population x(t) increases and reaches ET within the given time, which we called the outbreak period. We take the same parameters as those in Figure 8 except for the release amount μ of natural enemies. In Figure 9(a) and Figure 9(b), we plot the time series of pest population and the outbreak period of pests for μ=0.1,μ=0.3,μ=0.6, respectively. The simulation results indicate that the pest population eventually oscillate periodically and can be maintained below EIL. When the release amount μ=0.1, although the amplitude of density the pest is the largest, the period of pest outbreak Δτi is the longest, and the pest control strategies are only used 13 times. When the release amount μ=0.3, the pest outbreak period is the shortest, and the pest control strategy is the most frequently used. When the release amount μ=0.6, compared with μ=0.3, the pest outbreak period is longer, and the pest control strategy is less used, but we need to release more natural enemies. Therefore, if we want to prevent the density of pests from increasing to the level of EIL, from an ecological and economic perspective, it is not that the more natural enemies are released, the better the results are.
Many scholars used impulsive differential equations at the fixed time or with state feedback to describe biological phenomena that are subject to instantaneous disturbances, such as vaccination strategy [33,34], pollutants discharge [35,36], harvesting [37,38] and the culture of microorganisms in chemostats[39,40]. In the pest control, the two most common methods are spraying insecticides and releasing natural enemies, which were also simulated by many mathematical models with impulsive differential equation [6,8,9,10,11,12,13,14,15,16,17,18,29,30,31]. Those studies mainly focused on the chemical and biological control with instantaneous disturbances, or failed to take into account pesticide resistance. However, as the introduction pointed out that the pesticides had residual and delayed effects, and the pests were apt to develop resistance to pesticides, while the release of natural enemies can be instantaneous. So it is more reasonable to use a hybrid dynamic system to describe this process. In some cases, pesticides can be successfully integrated into the pest management program without harming natural enemies or having less effects on natural enemies. This can be done by using selective pesticides such as Bacillus thuringiensis (B.t.), choosing the time of pesticides applications to avoid the presence of important natural enemies or keeping pesticides out of the reach of natural enemies. In this case, the effects of pesticides on natural enemies are greatly reduced and the development of resistance is negligible. Therefore, in this paper, we developed a hybrid pest management model incorporating pest resistance to investigate how the frequency of the pesticide applications and the effects of pesticides affected the success or failure of pest control. Our results indicated that the development of pest resistance was related to the frequency of pesticide applications, the killing efficiency rate m1, the decay rate a1 and the delayed response rate c1. It was not that the more frequently the pesticides were sprayed and the stronger effects the pesticides had on pests, the more successful pest control became.
In particular, to control the outbreaks of pest, we provided three possible pest control strategies. If we want to completely eradicate the pest population, the simple and direct method for successful pest control is switching to another kind of pesticide. Our results indicated that taking threshold R2(ω,T) as a guide for switching pesticides, each pesticide could be used more often than taking the threshold R1(n,T) as a guide. However, the pesticide switching method will still result in the pest resistance to pesticide and even damage to natural enemies. Therefore, to reduce or delay the emergence of pest resistance and avoid harm to natural enemies, we should use less pesticides and adopt biological control whenever possible. We have shown that the constant release of natural enemies was insufficient to suppress the outbreaks of pest population once pest resistance developed, so we could take the releasing-natural-enemies-elastically strategy, that is, the amount of natural enemies to be released was determined according to the evolution of pest resistance, which was dynamic and varied with the development of resistance.
If we want to control the density of pests population below the EIL rather than eradicating them, the state feedback control strategy could be taken. From an ecological and economic perspective, the state feedback control strategy is more desirable. It avoids the development of pest resistance caused by excessive pesticide applications and the negative effects of pesticides on the environment and natural enemies. Further, our results obtained in the present work showed that it was not that the more natural enemies were released, the better results were obtained.
The results obtained here can provide theoretical guidance for relevant decision-making departments in the face of pesticide resistance. Note that only the simplest pest-natural enemy system has been employed to discuss the key issues related to pest resistance and pest management, and we only considered the pest resistance to pesticides. However, in some cases, natural enemies can develop resistance to pesticides. Some effects of environmental variations including climate, food availability and so on should be taken into account in the established model, which could significantly affect the outcome of pest control. We will leave these in the future work.
This work is supported by National Natural Science Foundation of China(11371030), Natural Science Foundation of Liaoning Province (20170540001) and Liaoning Bai Qian Wan Talents Program.
No potential conflict of interest was reported by the authors.
Since R(n,T)<1, we can choose a ϵ>0 small enough such that
χ=R1(n,T)exp(αεT)<1 | ( |
holds.
From the second equation of system (2.5), we know
dy(t)dt≥−(d+b2(t))y(t). |
Consider the following nonautonomous impulsive system:
{dN1(t)dt=−(d+b2(t))N1(t),t≠nT,N1(nT+)=N1(nT)+μ,t=nT, | ( |
By comparison theorem of impulsive differential equations and Theorem 3.1, we get y(t)≥N(t)>˜y(t)−ϵ for t large enough. For simplification, we assume y(t)>˜y(t)−ϵ for all t>0. Then from the first equation of system (2.5), we have
dx(t)dt≤rx(t)(1−x(t)K)−b1(t)q(t)x(t)−αx(t)(˜y(t)−ε). |
For t∈[nT,(n+1)T), since q(t) decreases with time t, we obtain
x((n+1)T))≤x(nT)(exp∫(n+1T)nT(r(1−x(t)K)−b1(t)q(t)−α(˜y(t)−ε))≤x(nT)exp∫(n+1T)nT(r−b1(t)q(t))dtexp∫(n+1T)nT(−α˜y(t))dtexp(αεT)=x(nT)R1(n,T)exp(αεT)≤x(nT)χ≤x((n−1)T)exp∫(nT)(n−1)T(r−b1(t)q(t))dtexp∫nT(n−1)T(−α˜y(t))dtexp(αεT)χ≤x((n−1)T)exp∫(n+1T)nT(r−b1(t)q(t))dtexp(∫(n+1T)nT(−α˜y(t))dtexp(αεT)χ=x((n−1)T)χ2≤⋯≤x(0)χn+1, |
so we have x(nT)→0 as n→∞. For t∈[nT,(n+1)T), 0<x(t)<x(nT)exp(rT), thus x(t)→0 as t→∞.
Next, we will prove y(t)→˜y(t) as t→∞. From the above conclusion, we know for any 0<ε1<dλα, there exists a t1>0 such that 0<x(t)<ε1 for t>t1. Without loss of generality, we assume 0<x(t)<ε1 for all t≥0. By System (2.5), we obtain
−(d+b2(t))y(t)≤dy(t)dt≤−((d−λαε1)+b2(t))y(t). | ( |
From the left-hand side of the above inequality and system (3.1), we have y(t)≥˜y(t)−ε2 for any ε2>0 and t large enough. For the right-hand side of System (A.3), consider the following impulsive system
{dN2(t)dt=−((d−λαε1)+b2(t))N2(t),t≠nT,N2(t+)=N2(t)+μ,t=nT, | ( |
Similar to system (3.1), system (A.4) has a unique globally asymptotically stable positive T-periodic solution ~N2(t), where
~N2(t)=N∗2exp(∫tnT(−((d−λαε1)+b2(s)))ds),t∈[nT,(n+1)T), |
N∗2=μ1−exp(−(d−λαε1)T−m2a2(1−e−a2T)+m2c2(1−e−c2T)). |
Thus, for ε2>0, there exists a t2>0 such that
y(t)≤~N2(t)+ε2. |
Let ε1→0, we have
˜y(t)−ε2≤y(t)≤˜y(t)+ε2 |
for t large enough, which implies y(t)→˜y(t) as t→∞.
Therefore, if R1(n,T)<1 holds, the pest-eradication periodic solution (0,˜y(t) of system (2.5) is globally attractive. This completes the proof.
[1] | C. A. Lane, J. Hardy, J. M Schott, Alzheimer's disease, Eur. J. Neurol., 25 (2017), 59-70. |
[2] | H. S. Kwon, S. H. Koh, Neuroinflammation in neurodegenerative disorders: the roles of microglia and astrocytes, Transl. Neurodegener., 9 (2020), 42. |
[3] | C. Marogianni, M. Sokratous, E. Dardiotis, G. M. Hadjigeorgiou, D. Bogdanos, G. Xiromerisiou, Neurodegeneration and inflammation—an interesting interplay in Parkinson's disease, Int. J. Mol. Sci., 21 (2020), 8421. |
[4] |
S. H. Kim, M. Y. Noh, H. J. Kim, K. W. Oh, J. Park, S. Lee, et al., A therapeutic strategy for Alzheimer's disease focused on immune-inflammatory modulation, Dement Neurocogn. Disord., 18 (2019), 33-46. doi: 10.12779/dnd.2019.18.2.33
![]() |
[5] | Z. Han, R. Tian, P. Ren, W. Zhou, P. Wang, M. Luo, et al., Parkinson's disease and Alzheimer's disease: a Mendelian randomization study, BMC Med. Genet., 19 (2018), 215. |
[6] | A. Xie, J. Gao, L. Xu, D. Meng, Shared mechanisms of neurodegeneration in Alzheimer's disease and Parkinson's disease, Biomed. Res. Int., 2014 (2014), 648740. |
[7] | J. Montaner, L. Ramiro, A. Simats, S. Tiedt, K. Makris, G. C. Jickling, et al., Multilevel omics for the discovery of biomarkers and therapeutic targets for stroke, Nat. Rev. Neurol., 16 (2020), 247-264. |
[8] | W. J. Lukiw, A. Vergallo, S. Lista, H. Hampel, Y. Zhao, Biomarkers for Alzheimer's disease (AD) and the application of precision medicine, J. Pers. Med., 10 (2020), 138. |
[9] |
E. M. Cilento, L. Jin, T. Stewart, M. Shi, L. Sheng, J. Zhang, Mass spectrometry: A platform for biomarker discovery and validation for Alzheimer's and Parkinson's diseases, J. Neurochem., 151 (2019), 397-416. doi: 10.1111/jnc.14635
![]() |
[10] |
J. Verheijen, K. Sleegers, Understanding Alzheimer disease at the interface between genetics and transcriptomics, Trends Genet., 34 (2018), 434-447. doi: 10.1016/j.tig.2018.02.007
![]() |
[11] |
E. Cuyvers, K. Sleegers, Genetic variations underlying Alzheimer's disease: evidence from genome-wide association studies and beyond, Lancet Neurol., 15 (2016), 857-868. doi: 10.1016/S1474-4422(16)00127-7
![]() |
[12] | F. L. Heppner, R. M. Ransohoff, B. Becher, Immune attack: The role of inflammation in Alzheimer disease, Nature, 16 (2015), 358-372. |
[13] | Y. Yan, A. Zhao, Y. Qui, Y. Li, R. Yan, Y. Wang, et al., Genetic association of FERMT2, HLA-DRB1, CD2AP, and PTK2B polymorphisms with Alzheimer's disease risk in the southern Chinese population, Front. Aging Neurosci., 12 (2020), 16. |
[14] | V. Escott-Price, C. Bellenguez, L. S. Wang, S. H. Choi, D. Harold, L. Jones, et al., Gene-wide analysis detects two new susceptibility genes for Alzheimer's disease. PLoS One, 9 (2014), e94661. |
[15] | I. López González, P. Garcia-Esparcia, F. Llorens, I. Ferrer, Genetic and transcriptomic profiles of inflammation in neurodegenerative diseases: Alzheimer, Parkinson, Creutzfeldt-Jakob and Tauopathies, Int. J. Mol. Sci., 17 (2016), 206. |
[16] | Z. P. Van Acker, M. Bretou, Wim Annaert, Endo-lysosomal dysregulations and late-onset Alzheimer's disease: impact of genetic risk factors, Mol. Neurodegener., 14 (2019), 20. |
[17] | A. De Roeck, C. Van Broeckhoven, K. Sleegers, The role of ABCA7 in Alzheimer's disease: evidence from genomics, transcriptomics and methylomics, Acta Neuropathol., 138 (2019), 201-220. |
[18] |
C. Reitz, Novel susceptibility loci for Alzheimer's disease, Future Neurol., 10 (2015), 547-558. doi: 10.2217/fnl.15.42
![]() |
[19] | C. Bellenguez, C. Charbonnier, B. Grenier-Boley, O. Quenez, K. Le Guennec, G. Nicolas, et al., Contribution to Alzheimer's disease risk of rare variants in TREM2, SORL1, and ABCA7 in 1779 cases and 1273 controls, Neurobiol. Aging, 59 (2017), 220.e1-220.e9. |
[20] |
P. Ciryam, R. Kundra, R. Freer, R. I. Morimoto, C. M. Dobson, M. Vendruscolo, A transcriptional signature of Alzheimer's disease is associated with a metastable subproteome at risk for aggregation, Proc. Natl. Acad. Sci. USA, 113 (2016), 4753-4758. doi: 10.1073/pnas.1516604113
![]() |
[21] | L. V. Kalia, A. E. Lang, Parkinson's disease, Lancet, 386 (2015), 896-912. |
[22] | A. Masato, N. Plotegher, D. Boassa, L. Bubacco, Impaired dopamine metabolism in Parkinson's disease pathogenesis, Mol. Neurodegener., 14 (2019), 35. |
[23] |
M. G. Krokidis, Identification of biomarkers associated with Parkinson's disease by gene expression profiling studies and bioinformatics analysis, AIMS Neurosci., 6 (2019), 333-345. doi: 10.3934/Neuroscience.2019.4.333
![]() |
[24] | A. Grünewald, K. R. Kumar, C. M. Sue, New insights into the complex role of mitochondria in Parkinson's disease, Prog. Neurobiol., 177 (2019), 79-93. |
[25] | C. A. D. Costa, W. E. Manaa, E. Duplan, F. Checler, The endoplasmic reticulum stress/unfolded protein response and their contributions to Parkinson's disease physiopathology, Cells, 9 (2020), 2495. |
[26] | M. Deffains, M. H. Canron, M. Teil, Q. Li, B. Dehay, E. Bezard, et al., L-DOPA regulates α-synuclein accumulation in experimental parkinsonism, Neuropathol. Appl. Neurobiol., 2020. |
[27] |
L. P. Dolgacheva, A. V. Berezhnov, E. I. Fedotova, V. P. Zinchenko, A. Y. Abramov, Role of DJ-1 in the mechanism of pathogenesis of Parkinson's disease, J. Bioenerg. Biomembr., 51 (2019), 175-188. doi: 10.1007/s10863-019-09798-4
![]() |
[28] | A. Urbizu, K. Beyer, Epigenetics in Lewy body diseases: Impact on gene expression, utility as a biomarker, and possibilities for therapy, Int. J. Mol. Sci., 21 (2020), 4718. |
[29] | D. M. O'Hara, G. Pawar, S. K. Kalia, L. V. Kalia, LRRK2 and α-Synuclein: Distinct or synergistic players in Parkinson's disease?, Front. Neurosci., 14 (2020), 577. |
[30] | T. K. Lin, K. J. Lin, K. L. Lin, C. W. Liou, S. D. Chen, Y. C. Chuang, et al., When friendship turns sour: effective communication between mitochondria and intracellular organelles in Parkinson's disease, Front. Cell. Dev. Biol., 8 (2020), 607392. |
[31] | B. Wang, K. R. Stanford, M. Kundu, ER-to-Golgi trafficking and its implication in neurological diseases, Cells, 9 (2020), 408. |
[32] | T. M. Axelsen, D. P. D. Woldbye, Gene therapy for Parkinson's disease, an update, J. Parkinsons Dis., 8 (2018), 195-215. |
[33] | T. J. Collier, D. E. Jr. Redmond, K. Steece-Collier, J. W. Lipton, F. P. Manfredsson, Is a-synuclein loss-of-function a contributor to parkinsonian pathology? Evidence from non-human primates, Front. Neurosci., 10 (2016), 12. |
[34] | C. Klein, N. Hattori, C. Marras, MDSGene: Closing data gaps in genotype-phenotype correlations of monogenic Parkinson's disease, J. Parkinsons Dis., 8 (2018), S25-S30. |
[35] | J. Chi, Q. Xie, J. Jia, X. Liu, J. Sun, Y. Deng, L. Yi, Integrated analysis and identification of novel biomarkers in Parkinson's disease, Front. Aging Neurosci., 10 (2018), 178. |
[36] | Y. I. Li, G. Wong, J. Humphrey, T. Raj, Prioritizing Parkinson's disease genes using population-scale transcriptomic data, Nat. Commun., 10 (2019), 994. |
[37] |
K. J. Karczewski, M. P. Snyder, Integrative omics for health and disease, Nat. Rev. Genet., 19 (2018), 299-310. doi: 10.1038/nrg.2018.4
![]() |
[38] | B. Wang, V. Kumar, A. Olson, D. Ware, Reviving the transcriptome studies: an insight into the emergence of single-molecule transcriptome sequencing, Front. Genet., 10 (2019), 384. |
[39] | F. R. Pinu, S. A. Goldansaz, J. Jaine, Translational metabolomics: current challenges and future opportunities, Metabolites, 9 (2019), 108. |
[40] | J. L. Ren, A. H. Zhang, L. Kong, X. J. Wang, Advances in mass spectrometry-based metabolomics for investigation of metabolites, RSC Adv., 8 (2018), 22335. |
[41] | J. M. Wilkins, E. Trushina, Application of metabolomics in Alzheimer's disease, Front. Neurol., 8 (2018), 719. |
[42] | E. Trushina, T. Dutta, X. M. Persson, M. M. Mielke, R. C. Petersen, Identification of altered metabolic pathways in plasma and CSF in mild cognitive impairment and Alzheimer's disease using metabolomics, PLoS One, 8 (2013), e63644. |
[43] |
G. Paglia, M. Stocchero, S. Cacciatore, S. Lai, P. Angel, M. T. Alam, et al., Unbiased metabolomic investigation of Alzheimer's disease brain points to dysregulation of mitochondrial aspartate metabolism, J. Proteome Res., 15 (2016), 608-618. doi: 10.1021/acs.jproteome.5b01020
![]() |
[44] | S. G. Snowden, A. A. Ebshiana, A. Hye, Y. An, O. Pletnikova, R. O'Brien, et al., Association between fatty acid metabolism in the brain and Alzheimer disease neuropathology and cognitive performance: a nontargeted metabolomic study, PLoS Med., 14 (2017), e1002266. |
[45] |
S. P. Guiraud, I. Montoliu, L. Da Silva, L. Dayon, A. N. Galindo, J. Corthesy, et al., High-throughput and simultaneous quantitative analysis of homo-cysteine-methionine cycle metabolites and co-factors in blood plasma and cerebrospinal fluid by isotope dilution LC-MS/MS, Anal. Bioanal. Chem., 409 (2017), 295-305. doi: 10.1007/s00216-016-0003-1
![]() |
[46] |
J. B. Toledo, M. Arnold, G. Kastenmuller, R. Chang, R. A. Baillie, X. Han, et al., Metabolic network failures in Alzheimer's disease-a biochemical road map, Alzheimers Dement., 13 (2017), 965-84. doi: 10.1016/j.jalz.2017.01.020
![]() |
[47] | S. F. Graham, O. P. Chevallier, C. T. Elliott, C. Holscher, J. Johnston, B. McGuinness, et al., Untargeted metabolomic analysis of human plasma indicates differentially affected polyamine and l-arginine metabolism in mild cognitive impairment subjects converting to Alzheimer's disease, PLoS One, 10 (2015), e0119452. |
[48] |
M. Mapstone, F. Lin, M. A. Nalls, A. K. Cheema, A. B. Singleton, M. S. Fiandaca, et al., What success can teach us about failure: the plasma metabolome of older adults with superior memory and lessons for Alzheimer's disease, Neurobiol. Aging, 51 (2017), 148-55. doi: 10.1016/j.neurobiolaging.2016.11.007
![]() |
[49] |
M. Benson, Clinical implications of omics and systems medicine: focus on predictive and individualized treatment, J. Intern. Med., 279 (2016), 229-240. doi: 10.1111/joim.12412
![]() |
[50] |
C. Evans, J. Hardin, D. M. Stoebel, Selecting between-sample RNA-Seq normalization methods from the perspective of their assumptions, Brief Bioinform., 19 (2018), 776-792. doi: 10.1093/bib/bbx008
![]() |
[51] | F. Maleki, K. Ovens, D. J. Hogan, A. J. Kusalik, Gene set analysis: challenges, opportunities, and future research, Front. Genet., 11 (2020), 654. |
[52] | I. Ihnatova, V. Popovici, E. Budinska, A critical comparison of topology-based pathway analysis methods, PLoS One, 13 (2018), e0191154. |
[53] | N. Xie, L. Zhang, W. Gao, C. Huang, P. E. Huber, X. Zhou, et al., Subpathway-GM: identification of metabolic subpathways via joint power of interesting genes and metabolites and their topologies within pathways, Signal. Transduct. Target. Ther., 5 (2020), 227. |
[54] |
Q. Yang, S. Wang, E. Dai, S. Zhou, D. Liu, H. Liu, et al., Pathway enrichment analysis approach based on topological structure and updated annotation of pathway, Brief Bioinform., 20 (2019), 168-177. doi: 10.1093/bib/bbx091
![]() |
[55] |
P. Kong, P. Lei, S. Zhang, D. Li, J. Zhao, B. Zhang, Integrated microarray analysis provided a new insight of the pathogenesis of Parkinson's disease, Neurosci. Lett., 662 (2018), 51-58. doi: 10.1016/j.neulet.2017.09.051
![]() |
[56] | M. G. Krokidis, P. Vlamos, Transcriptomics in amyotrophic lateral sclerosis, Front. Biosci. (Elite Ed), 10 (2018), 103-121. |
[57] | L. Bravo-Merodio, L. A. Williams, G. V. Gkoutos, A. Acharjee, Omics biomarker identification pipeline for translational medicine. J. Transl. Med., 17 (2019), 155. |
[58] |
M. A. Myszczynska, P. N. Ojamies, A. M. B. Lacoste, D. Neil, A. Saffari, R. Mead, et al., Applications of machine learning to diagnosis and treatment of neurodegenerative diseases, Nat. Rev. Neurol., 16 (2020), 440-456. doi: 10.1038/s41582-020-0377-8
![]() |
[59] | J. Xin, X. Ren, L. Chen, Y. Wang, Identifying network biomarkers based on protein-protein interactions and expression data, BMC Med. Genomics, 8 (2015), S11. |
[60] |
L. Fu, K. Fu, Analysis of Parkinson's disease pathophysiology using an integrated genomics-bioinformatics approach, Pathophysiology, 22 (2015), 15-29. doi: 10.1016/j.pathophys.2014.10.002
![]() |
[61] | R. Al-Ouran, Y. W. Wan, C. G. Mangleburg, T. V. Lee, K. Allison, J. M. Shulman, et al., A portal to visualize transcriptome profiles in mouse models of neurological disorders, Genes (Basel), 10 (2019), E759. |
[62] | J. Kelly, R. Moyeed, C. Carroll, D. Albani, X. Li, Gene expression meta-analysis of Parkinson's disease and its relationship with Alzheimer's disease, Mol. Brain., 12 (2019), 16. |
[63] | E. Mariani, F. Frabetti, A. Tarozzi, M. C. Pelleri, F. Pizzetti, R. Casadei, Meta-analysis of Parkinson's disease transcriptome data using TRAM software: whole substantia nigra tissue and single dopamine neuron differential gene expression, PLoS One, 11 (2016), e0161567. |
[64] |
P. Kong, P. Lei, S. Zhang, D. Li, J. Zhao, B. Zhang, Integrated microarray analysis provided a new insight of the pathogenesis of Parkinson's disease, Neurosci. Lett., 662 (2018), 51-58. doi: 10.1016/j.neulet.2017.09.051
![]() |
[65] |
E. Glaab, Computational systems biology approaches for Parkinson's disease, Cell Tissue Res., 373 (2018), 91-109. doi: 10.1007/s00441-017-2734-5
![]() |
[66] | T. M. Nguyen, A. Shafi, T. Nguyen, S. Draghici, Identifying significantly impacted pathways: a comprehensive review and assessment, Genome Biol., 20 (2019), 203. |
[67] | J. Ma, A. Shojaie, G. Michailidis, A comparative study of topology-based pathway enrichment analysis methods, BMC Bioinform., 20 (2019), 546. |
[68] | C. Gao, H. Sun, T. Wang, M. Tang, N. I. Bohnen, M. L. T. M. Müller, et al., Model-based and model-free machine learning techniques for diagnostic prediction and classification of clinical outcomes in Parkinson's disease, Sci. Rep., 8 (2018), 7129. |
[69] |
Y. Hu, Z. Pan, Y. Hu, L. Zhang, J. Wang, Network and pathway-based analyses of genes associated with Parkinson's disease, Mol. Neurobiol., 54 (2017), 4452-4465. doi: 10.1007/s12035-016-9998-8
![]() |
1. | Jingna Liu, Qi Qi, Bing Liu, Shujing Gao, Pest control switching models with instantaneous and non-instantaneous impulsive effects, 2023, 205, 03784754, 926, 10.1016/j.matcom.2022.10.027 | |
2. | Airen Zhou, Analysis of an Integrated Pest Management Model with Impulsive Diffusion between Two Regions, 2023, 11, 2227-7390, 2970, 10.3390/math11132970 | |
3. | Liping Wu, Zhongyi Xiang, A study of integrated pest management models with instantaneous and non-instantaneous impulse effects, 2024, 21, 1551-0018, 3063, 10.3934/mbe.2024136 | |
4. | Zeli Zhou, Jianjun Jiao, Xiangjun Dai, Lin Wu, Dynamics of a Predator–Prey System with Impulsive Stocking Prey and Nonlinear Harvesting Predator at Different Moments, 2024, 12, 2227-7390, 2369, 10.3390/math12152369 | |
5. | Xiangjun Dai, Jianjun Jiao, Qi Quan, Airen Zhou, Dynamics of a predator–prey system with sublethal effects of pesticides on pests and natural enemies, 2024, 17, 1793-5245, 10.1142/S1793524523500079 | |
6. | Bing Liu, Jiani Jin, Haokun Qi, Study on an integrated pest management switching model with pesticide resistance development and limited resources of natural enemies, 2025, 1598-5865, 10.1007/s12190-025-02540-x |
Time nT of the releasing natural enemies | The releasing amount un of natural enemies |
T | 0.038 |
2T | 0.0385 |
3T | 0.039 |
4T | 0.0396 |
5T | 0.04 |
6T | 0.0405 |
7T | 0.041 |
8T | 0.049 |
9T | 0.0429 |
10T | 0.044 |
11T | 0.0454 |
12T | 0.047 |
13T | 0.049 |
14T | 0.0513 |
15T | 0.054 |
16T | 0.0567 |
17T | 0.06 |
18T | 0.0635 |
19T | 0.0675 |
20T | 0.0716 |
21T | 0.076 |
22T | 0.08 |
23T | 0.0846 |
24T | 0.089 |
Time nT of the releasing natural enemies | The releasing amount un of natural enemies |
T | 0.038 |
2T | 0.0385 |
3T | 0.039 |
4T | 0.0396 |
5T | 0.04 |
6T | 0.0405 |
7T | 0.041 |
8T | 0.049 |
9T | 0.0429 |
10T | 0.044 |
11T | 0.0454 |
12T | 0.047 |
13T | 0.049 |
14T | 0.0513 |
15T | 0.054 |
16T | 0.0567 |
17T | 0.06 |
18T | 0.0635 |
19T | 0.0675 |
20T | 0.0716 |
21T | 0.076 |
22T | 0.08 |
23T | 0.0846 |
24T | 0.089 |