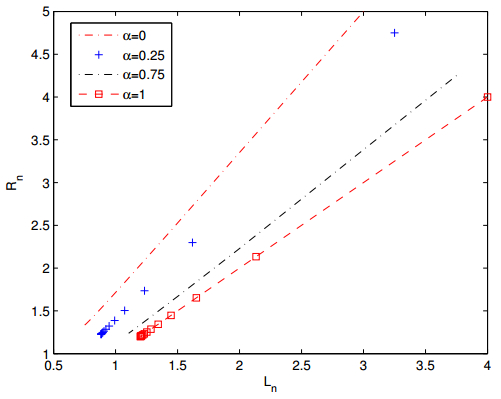
Citation: Qianhong Zhang, Fubiao Lin, Xiaoying Zhong. On discrete time Beverton-Holt population model with fuzzy environment[J]. Mathematical Biosciences and Engineering, 2019, 16(3): 1471-1488. doi: 10.3934/mbe.2019071
[1] | Martin Bohner, Sabrina Streipert . Optimal harvesting policy for the Beverton--Holt model. Mathematical Biosciences and Engineering, 2016, 13(4): 673-695. doi: 10.3934/mbe.2016014 |
[2] | Martin Bohner, Jaqueline Mesquita, Sabrina Streipert . The Beverton–Hold model on isolated time scales. Mathematical Biosciences and Engineering, 2022, 19(11): 11693-11716. doi: 10.3934/mbe.2022544 |
[3] | John E. Franke, Abdul-Aziz Yakubu . Periodically forced discrete-time SIS epidemic model with disease induced mortality. Mathematical Biosciences and Engineering, 2011, 8(2): 385-408. doi: 10.3934/mbe.2011.8.385 |
[4] | Yang Li, Jia Li . Stage-structured discrete-time models for interacting wild and sterile mosquitoes with beverton-holt survivability. Mathematical Biosciences and Engineering, 2019, 16(2): 572-602. doi: 10.3934/mbe.2019028 |
[5] | Guilherme M Lopes, José F Fontanari . Influence of technological progress and renewability on the sustainability of ecosystem engineers populations. Mathematical Biosciences and Engineering, 2019, 16(5): 3450-3464. doi: 10.3934/mbe.2019173 |
[6] | Marcin Choiński, Mariusz Bodzioch, Urszula Foryś . A non-standard discretized SIS model of epidemics. Mathematical Biosciences and Engineering, 2022, 19(1): 115-133. doi: 10.3934/mbe.2022006 |
[7] | Ming Chen, Meng Fan, Congbo Xie, Angela Peace, Hao Wang . Stoichiometric food chain model on discrete time scale. Mathematical Biosciences and Engineering, 2019, 16(1): 101-118. doi: 10.3934/mbe.2019005 |
[8] | Benjamin Wacker, Jan Schlüter . An age- and sex-structured SIR model: Theory and an explicit-implicit numerical solution algorithm. Mathematical Biosciences and Engineering, 2020, 17(5): 5752-5801. doi: 10.3934/mbe.2020309 |
[9] | Ming Chen, Menglin Gong, Jimin Zhang, Lale Asik . Comparison of dynamic behavior between continuous- and discrete-time models of intraguild predation. Mathematical Biosciences and Engineering, 2023, 20(7): 12750-12771. doi: 10.3934/mbe.2023569 |
[10] | Ning Yu, Xue Zhang . Discrete stage-structured tick population dynamical system with diapause and control. Mathematical Biosciences and Engineering, 2022, 19(12): 12981-13006. doi: 10.3934/mbe.2022606 |
Discrete time single-species model is the most appropriate mathematical description of life histories of organism whose reproduction occurs only once a year during a very short season. It is assumed that, in the rest of the year, the population is only subjected to mortality, but not to births. Therefore the between year dynamics is characterized by a first order difference equation xn+1=f(xn), where xn denotes population at the nth generation. The production function f is usually density-dependent, and the strength of density dependence is determined by several parameters including growth rate, the probability of surviving the reproductive season, the carrying capacity of surrounding environment, and intraspecific cooperation or competition factors. These models are widely used in fisheries and many organisms [1]. Beverton-Holt model [2] is one of classic population model which has been studied
xn+1=βxn1+δxn,n=0,1,⋯, |
where xn is population at the nth generation, β represents a productivity parameter, and δ controls the level of density dependence. Since then, many results on the model and the generation of the model have been widely obtained by some researchers [3,4,5].
In fact, the identification of the parameters of the model is usually based on statistical method, starting from data experimentally obtained and on the choice of some method adapted to the identification. These models, even the classic deterministic approach, are subjected to inaccuracies (fuzzy uncertainty) that can be caused by the nature of the state variables, by parameters as coefficients of the model and by initial conditions.
In our real life, we have learned to deal with uncertainty. Scientists also accept the fact that uncertainty is very important study in most applications. Modeling the real life problems in such cases, usually involves vagueness or uncertainty in some of the parameters. The concept of fuzzy set and system was introduced by Zadeh [6] and its development has been growing rapidly to various situation of theory and application including fuzzy differential and fuzzy difference equations. It is well known that fuzzy difference equation is a difference equation whose parameters or the initial values are fuzzy numbers, and its' solutions are sequences of fuzzy numbers. It has been used to model a dynamical systems under possibility uncertainty [7]. Due to the applicability of fuzzy difference equation for the analysis of phenomena where imprecision is inherent, this class of difference equation is a very important topic from theoretical point of view and also its applications. Recently there has been an increasing interest in the study of fuzzy difference equations (see [8,9,10,11,12,13,14,15,16,17,18,19,20,21,22,23]).
Inspired with the previous, by virtue of the theory of fuzzy difference equation, in this work, we consider the following discrete time Beverton-Holt model with fuzzy uncertainty parameters and initial conditions.
xn+1=Axn˜1+Bxn,n=0,1,⋯, | (1.1) |
where xn is population at the nth generation, A denotes a productivity parameter, B controls the level of density dependence. Furthermore A,˜1,B and the initial value x0 are positive fuzzy numbers.
The main aim of this work is to study the existence of positive solutions of Beverton-Holt population model (1.1). Furthermore, according to a generation of division (g-division) of fuzzy numbers, we derive some conditions so that every positive solution of Beverton-Holt population model (1.1) is bounded and persistent. Finally, under some conditions we prove that Beverton-Holt population model (1.1) has a unique positive equilibrium x and every positive solution tends to x as n→∞.
Firstly, we give the following definitions.
Definition 2.1. [24] u:R→[0,1] is said to be a fuzzy number if it satisfies conditions (i)-(iv) written below:
(i) u is normal, i. e., there exists an x∈R such that u(x)=1;
(ii) u is fuzzy convex, i. e., for all t∈[0,1] and x1,x2∈R such that
u(tx1+(1−t)x2)≥min{u(x1),u(x2)}; |
(iii) u is upper semicontinuous;
(iv) The support of u, suppu=¯⋃α∈(0,1][u]α=¯{x:u(x)>0} is compact.
For α∈(0,1], the α−cuts of fuzzy number u is [u]α={x∈R:u(x)≥α} and for α=0, the support of u is defined as suppu=[u]0=¯{x∈R|u(x)>0}.
Definition 2.2. Fuzzy Number (Parametric form): [24] A fuzzy number u in a parametric form is a pair (u_,¯u) of functions u_(r),¯u(r),0≤r≤1, which satisfies the following requirements:
(1) u_(r) is a bounded monotonic increasing left continuous function,
(2)¯u(r) is a bounded monotonic decreasing left continuous function,
(3) u_(r)≤¯u(r),0≤r≤1.
A crisp (real) number x is simply represented by (u_(r),¯u(r))=(x,x),0≤r≤1. The fuzzy number space {(u_(r),¯u(r))} becomes a convex cone E1 which could be embedded isomorphically and isometrically into a Banach space [24].
Definition 2.3. [24] The distance between two arbitrary fuzzy numbers u and v is defined as follows:
D(u,v)=supα∈[0,1]max{|ul,α−vl,α|,|ur,α−vr,α|}. | (2.1) |
It is clear that (E1,D) is a complete metric space.
Definition 2.4. [24] Let u=(u_(r),¯u(r)),v=(v_(r),¯v(r))∈E1,0≤r≤1, and arbitrary k∈R. Then
(i) u=v iff u_(r)=v_(r),¯u(r)=¯v(r),
(ii) u+v=(u_(r)+v_(r),¯u(r)+¯v(r)),
(iii) u−v=(u_(r)−¯v(r),¯u(r)−v_(r)),
(iv) ku={(ku_(r),k¯u(r)),k≥0;(k¯u(r),ku_(r)),k<0,
(v) uv=(min{u_(r)v_(r),u_(r)¯v(r),¯u(r)v_(r),¯u(r)¯v(r)},max{u_(r)v_(r),u_(r)¯v(r),¯u(r)v_(r),¯u(r)¯v(r)}).
Definition 2.5. Triangular Fuzzy Number. [24] A triangular fuzzy number (TFN) denoted by A is defined as (a,b,c) where the membership function
A(x)={0,x≤a;x−ab−a,a≤x≤b;1,x=b;c−xc−b,b≤x≤c;0,x≥c.
The α−cuts of A=(a,b,c) are described by [A]α={x∈R:A(x)≥α}=[a+α(b−a),c−α(c−b)]=[Al,α,Ar,α], α∈[0,1], it is clear that the [A]α are closed interval. A fuzzy number is positive if suppA⊂(0,∞).
The following proposition is fundamental since it characterizes a fuzzy set through the α-levels.
Proposition 2.1 [24] If {Aα:α∈[0,1]} is a compact, convex and not empty subset family of Rn such that
(i) ¯⋃Aα⊂A0.
(ii) Aα2⊂Aα1 if α1≤α2.
(iii) Aα=⋂k≥1Aαk if αk↑α>0.
Then there is u∈En(En denotes n dimensional fuzzy number space) such that [u]α=Aα for all α∈(0,1] and [u]0=¯⋃0<α≤1Aα⊂A0.
Definition 2.6. [25] Suppose that A,B∈E1 have α-cuts [A]α=[Al,α,Ar,α],[B]α=[Bl,α,Br,α], with 0∉[B]α,∀α∈[0,1]. The g-division ÷g is the operation that calculates the fuzzy number C=A÷gB having level cuts [C]α=[Cl,α,Cr,α](here [A]α−1=[1/Ar,α,1/Al,α]) defined by
[C]α=[A]α÷g[B]α⟺{(i)[A]α=[B]α[C]α,or(ii)[B]α=[A]α[C]α−1 | (2.2) |
provided that C is a proper fuzzy number (Cl,α is nondecreasing, Cr,α is nondecreasing, Cl,1≤Cr,1).
Remark 2.1. According to [25], in this paper the fuzzy number is positive, if A÷gB=C∈E1 exists, then the following two cases are possible
Case Ⅰ. if Al,αBr,α≤Ar,αBl,α,∀α∈[0,1], then Cl,α=Al,αBl,α,Cr,α=Ar,αBr,α,
Case Ⅱ. if Al,αBr,α≥Ar,αBl,α,∀α∈[0,1], then Cl,α=Ar,αBr,α,Cr,α=Al,αBl,α.
The fuzzy analog of the boundedness and persistence (see [9,10]) is as follows:
Definition 2.7. A sequence of positive fuzzy numbers (xn) is persistence (resp. bounded) if there exists a positive real number M (resp. N) such that
supp xn⊂[M,∞)(resp. supp xn⊂(0,N]),n=1,2,⋯, |
A sequence of positive fuzzy numbers (xn) is bounded and persistence if there exist positive real numbers M,N>0 such that
supp xn⊂[M,N],n=1,2,⋯. |
A sequence of positive fuzzy numbers (xn),n=1,2,⋯, is an unbounded if the norm ‖xn‖,n=1,2,⋯, is an unbounded sequence.
Definition 2.8. xn is a positive solution of (1.1) if (xn) is a sequence of positive fuzzy numbers which satisfies (1.1). A positive fuzzy number x is called a positive equilibrium of (1.1) if
x=Ax˜1+Bx. |
Let (xn) be a sequence of positive fuzzy numbers and x is a positive fuzzy number, xn→x as n→∞ if limn→∞D(xn,x)=0.
Firstly we study the existence of positive solutions of Beverton-Holt population model (1.1). We need the following lemma.
Lemma 3.1. [24] Let f:R+×R+×R+→R+ be continuous, A,B,C are fuzzy numbers. Then
[f(A,B,C)]α=f([A]α,[B]α,[C]α), α∈(0,1] | (3.1) |
Theorem 3.1. Consider Beverton-Holt population model (1.1) with fuzzy uncertainty parameters and initial condition. Then, for any positive fuzzy number x0, there exists a unique positive solution xn of Beverton-Holt population model (1.1) with initial conditions x0.
Proof. The proof is similar to those of Proposition 2.1 [9]. Suppose that there exists a sequence of fuzzy numbers (xn) satisfying (1.1) with initial condition x0. Consider the α−cuts, α∈(0,1],
[xn]α=[Ln,α,Rn,α],n=0,1,2,⋯, [A]α=[Al,α,Ar,α], [B]α=[Bl,α,Br,α], [˜1]α=[˜1l,α,˜1r,α], | (3.2) |
It follows from (1.1), (3.2) and Lemma 3.1 that
[xn+1]α=[Ln+1,α,Rn+1,α]=[Axn˜1+Bxn]α=[A]α×[xn]α[˜1]α+[B]α×[xn]α=[Al,αLn,α,Ar,αRn,α][˜1l,α+Bl,αLn,α,˜1r,α+Br,αRn,α] |
Noting Remark 2.1, one of the following two cases holds
Case Ⅰ
[xn+1]α=[Ln+1,α,Rn+1,α]=[Al,αLn,α˜1l,α+Bl,αLn,α,Ar,αRn,α˜1r,α+Br,αRn,α] | (3.3) |
Case Ⅱ
[xn+1]α=[Ln+1,α,Rn+1,α]=[Ar,αRn,α˜1r,α+Br,αRn,α,Al,αLn,α˜1l,α+Bl,αLn,α] | (3.4) |
If Case Ⅰ holds true, it follows that for n∈{0,1,2,⋯},α∈(0,1]
Ln+1,α=Al,αLn,α˜1l,α+Bl,αLn,α, Rn+1,α=Ar,αRn,α˜1r,α+Br,αRn,α | (3.5) |
Then it is obvious that, for any initial condition (L0,α,R0,α),α∈(0,1], there is a unique solution (Ln,α,Rn,α). Now we prove that [Ln,α,Rn,α],α∈(0,1], where (Ln,α,Rn,α) is the solution of system (3.5) with initial conditions (L0,α,R0,α), determines the solution xn of (1.1) with initial conditions x0 such that
[xn]α=[Ln,α,Rn,α], α∈(0,1], n=0,1,2,⋯. | (3.6) |
For n=1, since A,B,˜1 and x0 are positive fuzzy numbers, it is easy to see that [L1,α,R1,α] is the α-cuts of x1=Ax0˜1+Bx0, for any α∈(0,1], we have
[L1,α,R1,α]=[Al,αL0,α˜1l,α+Bl,αL0,α,Ar,αR0,α˜1r,α+Br,αR0,α]=[A]α[x0]α[˜1]α+[B]α[x0]α |
Working inductively, let [Lk,α,Rk,α],k≥1, be the α-cuts of fuzzy number xk as [xk]α=[Lk,α,Rk,α], we show that [Lk+1,α,Rk+1,α] determines the α-cuts of fuzzy number xk+1=Axk˜1+Bxk.
According to (3.5), for α∈(0,1], we have
[Lk+1,α,Rk+1,α]=[Al,αLk,α˜1l,α+Bl,αLk,α,Ar,αRk,α˜1r,α+Br,αRk,α]=[Al,αLk,α,Ar,αRk,α][˜1l,α+Bl,αLk,α,˜1r,α+Br,αRk,α]=[A]α[xk]α[˜1]α+[B]α[xk]α. | (3.7) |
Then [Lk+1,α,Rk+1,α] determines the α-cuts of fuzzy number xk+1=Axk˜1+Bxk. Then for each n, [Ln,α,Rn,α],α∈(0,1] determines the α-cuts of fuzzy number xn satisfying with (3.6).
Next we prove the uniqueness of the solution. Suppose that there exists another solution ¯xn of (1.1) with the initial condition x0. Then arguing as above, it is easy to get
[¯xn]α=[Ln,α,Rn,α], α∈(0,1], n=0,1,⋯. | (3.8) |
Then from (3.6) and (3.8) we have [xn]α=[¯xn]α,α∈(0,1],n=0,1,2,⋯, from which it follows that xn=¯xn,n=0,1,⋯. Thus the proof is completed.
If Case Ⅱ holds true, the proof is similar to those of Case Ⅰ. Thus the proof of Theorem 3.1 is completed.
Remark 3.1. From a theoretical point of view, the existence of solution for fuzzy difference equation is very important and meaningful with initial condition. Therefore, in that sense, the existence of positive fuzzy solution for Beverton-Holt population model is of vital importance and practical significance. In fact, it can see that the positive solution of Beverton-Holt population model with fuzzy environment is a sequence of positive fuzzy numbers describing the fuzzy uncertainty.
To study the dynamical behavior of the positive solutions of Beverton-Holt population model (1.1), according to Definition 2.3, we consider two cases.
Firstly, if Case Ⅰ holds true, we need the following lemma.
Lemma 3.2 Consider the system of difference equations
yn+1=aynp+cyn, zn+1=bznq+dzn, n=0,1,⋯, | (3.9) |
where p∈(0,1),q∈(1,+∞),a,b,c,d∈(0,+∞),y0,z0∈(0,+∞), if a>p,b>q, then the following statements are true:
(i) The system exists unique positive equilibrium (a−pc,b−qd) which is globally asymptotically stable.
(ii) yn and zn are bounded and persistent.
Proof. (ⅰ) Let (¯y,¯z) be equilibrium point of (3.9). It is easy to get positive equilibrium (¯y,¯z)=(a−pc,b−qd). The linearized equation associated with (3.9) about equilibrium (¯y,¯z) is
yn+1=payn, zn+1=qbzn. |
Since a>p,b>q, it follows that the system is locally asymptotically stable.
On the other hand, set f(y)=ayp+cy,g(z)=bzq+dz, then
f′(y)=ap(p+cy)2>0, g′(z)=bq(q+dz)2>0. | (3.10) |
Namely, the sequences (yn) and (zn) are increasing and
yn=ayn−1p+cyn−1<ac, zn=bzn−1q+dzn−1<bd. | (3.11) |
Therefore, from (3.10) and (3.11), it follows that the limitation of (yn),(zn) exist. Set limn→∞yn=y,limn→∞zn=z, integrating with (3.9), it can follows that
limn→∞yn=a−pc, limn→∞zn=b−qd. | (3.12) |
Therefore, it follows that the positive equilibrium (a−pc,b−qd) is globally asymptotically stable.
(ⅱ) Set Yn=1yn, Zn=1zn, then (3.9) can be transformed into
Yn+1=ca+paYn, Zn+1=db+qbZn, n=0,1,⋯, | (3.13) |
It follows from (3.13) that
Yn=ca+pca2+p2a2Yn−2=ca+pca2+⋯+pn−1can+pnanY0=ca[1−(pa)n−1]1−pa+pnanY0≤ca−p+Y0:=1δ | (3.14) |
Zn=db+qdb2+q2b2Zn−2=db+qdb2+⋯+qn−1dbn+qnbnZ0=db[1−(qb)n−1]1−qb+qnbnZ0≤db−q+Z0:=1γ | (3.15) |
From (3.11), (3.14) and (3.15), This implies
δ≤yn≤ac, γ≤zn≤bd. |
This completes the proof of Lemma 3.2.
Theorem 3.2 Consider Beverton-Holt population model (1.1) with fuzzy uncertainty parameters and initial condition. If
0<˜1l,α≤Al,α, ˜1r,α≤Ar,α, α∈(0,1], | (3.16) |
then the following statements are true
(i) Every positive solution xn of Beverton-Holt population model (1.1) is bounded and persistent.
(ii) Every positive solution xn of Beverton-Holt population model (1.1) tends to the positive equilibrium point x as n→∞.
Proof. (ⅰ) Since A,˜1,B and the initial value x0 are positive fuzzy numbers, there exist positive real numbers MA,NA,MB,NB,M0,N0,P,Q, such that, for all α∈(0,1],
[Al,α,Ar,α]⊂[MA,NA], [Bl,α,Br,α]⊂[MB,NB], [L0,α,R0,α]⊂[M0,N0],[˜1l,α,˜1r,α]⊂[P,Q], | (3.17) |
Let xn be a positive solution of Beverton-Holt population model (1.1), from (3.16), (3.17) and Lemma 3.2, we get
Ln,α>(Al,α−˜1l,α)L0,αBl,αL0,α+Al,α−˜1l,α>(MA−Q)M0NBN0+NA−Q:=M, Rn,α<Ar,αBr,α<NAMB:=N | (3.18) |
From which, we get for n≥1,⋃α∈(0,1][Ln,α,Rn,α]⊂[M,N], and so ¯⋃α∈(0,1][Ln,α,Rn,α]⊆[M,N]. Thus the positive solution is bounded and persistent.
(ⅱ) Suppose that there exists a fuzzy number x such that
x=Ax˜1+Bx, [x]α=[Lα,Rα], α∈(0,1]. | (3.19) |
where Lα,Rα≥0. Then from (3.19) we can prove that
Lα=Al,αLα˜1l,α+Bl,αLα, Rα=Ar,αRα˜1r,α+Br,αRα. | (3.20) |
Hence from (3.20), we have
Lα=Al,α−˜1l,αBl,α, Rα=Ar,α−˜1r,αBr,α. | (3.21) |
Let xn be a positive solution of Beverton-Holt population model (1.1). Since (3.16) holds true, we can apply Lemma 3.2 to system (3.5), and so we have
limn→∞Ln,α=Lα, limn→∞Rn,α=Rα, | (3.22) |
Therefore from (3.22) we have
limn→∞D(xn,x)=limn→∞supα∈(0,1]{max{|Ln,α−Lα|,|Rn,α−Rα|}}=0. |
This completes the proof of the Theorem.
Secondly, if Case Ⅱ holds true, it follows that for n∈{0,1,2,⋯},α∈(0,1]
Ln+1,α=Ar,αRn,α˜1r,α+Br,αRn,α, Rn+1,α=Al,αLn,α˜1l,α+Bl,αLn,α | (3.23) |
We need the following lemmas.
Lemma 3.3. Consider the system of difference equations
yn+1=bznq+dzn, zn+1=aynp+cyn, n=0,1,⋯, | (3.24) |
where p∈(0,1),q∈(1,+∞),a,b,p,q,c,d,y0,z0∈(0,+∞). If
a>p, b>q. | (3.25) |
Then the following statements are true
(i) The solution of (3.24) is bounded and persistent.
(ii) Furthermore suppose that
a−pc<b−qd. | (3.26) |
Then system exists unique positive equilibrium point (ab−pqqc+ad,ab−pqpd+bc) which is globally asymptotically stable.
Proof. (ⅰ) It is clear from (3.24) that
yn≤bd, zn≤ac. | (3.27) |
Setting Yn=1yn, Zn=1zn, then (3.24) can be transformed to
Yn=db+qbZn−1, Zn=ca+paYn−1. | (3.28) |
Working inductively, for n−2k≥0, it can conclude that
Yn=db+qb(ca+paYn−2)=db+qcab+pqabYn−2=db+qcab+pqdab2+pq2ab2Zn−3=db+qcab+pqdab2+pq2ca2b2+p2q2a2b2Yn−4=⋯=db[1−(pqab)k]1−pqab+qcab[1−(pqab)k]1−pqab+(pqab)kYn−2k≤db+qcab1−pqab+Y0=bc+pdab−pq+Y0:=1ξ | (3.29) |
Zn=ca+pa(db+qbZn−2)=αa+pdab+pqabZn−2=ca+pdab+pqca2b+p2qa2bZn−3=ca+pdab+pqca2b+p2qda2b2+p2q2a2b2Zn−4=⋯=ca[1−(pqab)k]1−pqab+pdab[1−(pqab)k]1−pqab+(pqab)kZn−2k≤ca+pdab1−pqab+Z0=bc+pdab−pq+Z0:=1ρ | (3.30) |
It follows from (3.27), (3.29) and (3.30) that
ξ≤yn≤bd, ρ≤zn≤ac, for n>0. | (3.31) |
This completes the proof of (ⅰ).
(ⅱ) Let (¯y,¯z) be an equilibrium point of (3.24). It is clear to obtain that (¯y,¯z)=(ab−pqqc+ad,ab−pqpd+bc). The linearized equation of system (3.24) about the equilibrium point (¯y,¯z) is
Ψn+1=GΨn. | (3.32) |
where Ψn=(yn,zn)T and
G=(0qb(q+d¯z)2pa(p+c¯y)20) |
Thus the characteristic equation of (3.32) is
λ2−pqab(p+c¯y)2(q+d¯z)2=0. |
Since (3.25) and (3.26) hold true, it is easy to obtain that the root of characteristic equation |λ|<1. From [26,27], thus the unique positive equilibrium point (¯y,¯z) is locally asymptotically stable.
Next, let (yn,zn) be an arbitrary positive solution of (3.24). From (3.29) and (3.30), we have {Yn}, {Zn} are monotone increasing and have a upper bound. Namely, {yn}, {zn} are monotone decreasing and have lower bound. So, set limn→∞yn=y,limn→∞zn=z., From (3.24), it can follow that
limn→∞yn=y=¯y=ab−pqqc+ad, limn→∞zn=z=¯z=ab−pqpd+bc. |
Therefore it implies that the unique positive equilibrium point (¯y,¯z) is globally asymptotically stable.
Theorem 3.3. Consider Beverton-Holt population model (1.1) with fuzzy uncertainty parameters and initial condition. If (3.16) and the following condition are satisfied
Bl,αBr,α≤Al,α−˜1l,αAr,α−˜1r,α, ∀α∈(0,1]. | (3.33) |
Then the following statements are true
(i) Every positive solution xn of Beverton-Holt population model (1.1) is bounded and persistence.
(ii) Every positive solution xn of Beverton-Holt population model (1.1) tends to the positive equilibrium point x as n→+∞.
Proof. (ⅰ) The proof is similar to those of Theorem 3.2. Let xn be a positive solution of (1.1), from (3.16), (3.17) and Lemma 3.3, we get
Ln,α≥L0,α(Al,αAr,α−˜1l,α˜1r,α)L0,α(Ar,αBl,α+˜1l,αBr,α)+(Al,αAr,α−˜1l,α˜1r,α)≥M0(M2A−Q2)N0(NANB+QNB)+(N2A−P2)=:K | (3.34) |
Rn,α≤Al,αBl,α≤NAMB=:L | (3.35) |
From (3.34) and (3.35), we get, for n≥1,⋃α∈(0,1][Ln,α,Rn,α]⊂[K,L]. And so
¯⋃α∈(0,1][Ln,α,Rn,α]⊆[K,L]. |
Thus the positive solution xn of (1.1) is bounded and persistent.
(ⅱ) Suppose that there exists a fuzzy number x such that (3.19) is satisfied. Then from (3.19) and Case Ⅱ, we have
Lα=Ar,αRα˜1r,α+Br,αRα, Rα=Al,αLα˜1l,α+Bl,αLα. | (3.36) |
It follows from (3.36) that
Lα=Al,αAr,α−˜1l,α˜1r,α˜1r,αBl,α+Al,αBr,α, Rα=Al,αAr,α−˜1l,α˜1r,α˜1l,αBr,α+Ar,αBl,α. | (3.37) |
Let xn be a positive solution of (1.1) such that Case Ⅱ holds. Namely.
Ln+1,α=Ar,αRn,α˜1r,α+Br,αRn,α, Rn+1,α=Al,αLn,α˜1l,α+Bl,αLn,α. | (3.38) |
Since (3.38) is satisfied, we can apply Lemma 3.3 to system (3.38) and so we have
limn→∞Ln,α=Lα, limn→∞Rn,α=Rα, | (3.39) |
Therefore from (3.39) we have
limn→∞D(xn,x)=limn→∞supα∈(0,1]{max{|Ln,α−Lα|,|Rn,α−Rα|}}=0. |
This completes the proof of Theorem 3.3.
Remark 3.2. In population dynamical model, the parameters of model derived from statistic data with vagueness or uncertainty. It corresponds to reality to use fuzzy parameters in population dynamical model. In contrast with classic population model, the solution of fuzzy population model is within a range of value (approximate value), which are taken into account fuzzy uncertainties. Furthermore the global asymptotic behaviour of discrete Beverton-Holt population model are obtained in fuzzy context.
Example 4.1 Consider the following fuzzy discrete time Beverton-Holt population model
xn+1=Axn˜1+Bxn, n=0,1,⋯, | (4.1) |
we take A,B,˜1 and the initial values x0 such that
A(x)={54x−1,0.8≤x≤1.6−52x+5,1.6≤x≤2,˜1(x)={2x−1,0.5≤x≤1−5x+6,1≤x≤1.2 | (4.2) |
B(x)={10x−4,0.4≤x≤0.5−10x+6,0.5≤x≤0.6,x0(x)={x−3,3≤x≤4−x+5,4≤x≤5 | (4.3) |
From (4.2), we get
[A]α=[0.8+45α,2−25α], [˜1]α=[0.5+12α,1.2−15α], α∈(0,1]. | (4.4) |
From (4.3) we get
[B]α=[0.4+110α,0.6−110α], [x0]α=[3+α,5−α], α∈(0,1]. | (4.5) |
Therefore, it follows that
¯⋃α∈(0,1][A]α=[0.8,2], ¯⋃α∈(0,1][˜1]α=[0.5,1.2], ¯⋃α∈(0,1][B]α=[0.4,0.6],¯⋃α∈(0,1][x0]α=[3,5]. | (4.6) |
From (4.1), it results in a coupled system of difference equations with parameter α,
Ln+1,α=Al,αLn,α˜1l,α+Bl,αLn,α, Rn+1,α=Ar,αRn,α˜1r,α+Br,αRn,α, α∈(0,1]. | (4.7) |
Therefore, Al,α≥˜1l,α>0,Ar,α≥˜1r,α,∀α∈(0,1], and initial values x0 are positive fuzzy numbers, so from Theorem 3.2, we have that every positive solution xn of Eq.(4.1) is bounded and persistence.
In addition, from Theorem 3.2, Eq.(4.1) has a unique positive equilibrium ¯x=(0.75,1.2,1.333). Moreover every positive solution xn of Eq.(4.1) converges the unique equilibrium ¯x with respect to D as n→∞. (see Figure 1–Figure 3)
Example 4.2 Consider the following fuzzy discrete time Beverton-Holt population model (4.1).
where A,˜1,B and the initial values x0 are satisfied
A(x)={103x−3,0.9≤x≤1.2−103x+5,1.2≤x≤1.5,˜1(x)={103x−73,0.7≤x≤1−103x+133,1≤x≤1.3 | (4.8) |
B(x)={5x−2,0.4≤x≤0.6−5x+4,0.6≤x≤0.8,x0(x)={52x−1.5,0.6≤x≤1−52x+3.5,1≤x≤1.4 | (4.9) |
From (4.8), we get
[A]α=[0.9+310α,1.5−310α], [˜1]α=[0.7+310α,1.3−310α], α∈(0,1]. | (4.10) |
From (4.9), we get
[B]α=[0.4+15α,0.8−15α], [x0]α=[0.6+25α,1.4−25α], α∈(0,1]. | (4.11) |
Therefore, it follows that
¯⋃α∈(0,1][A]α=[0.9,1.5], ¯⋃α∈(0,1][˜1]α=[0.7,1.3], ¯⋃α∈(0,1][B]α=[0.4,0.8],¯⋃α∈(0,1][x0]α=[0.6,1.4]. | (4.12) |
From (4.1), it results in a coupled system of difference equation with parameter α,
Ln+1,α=Ar,αRn,α˜1r,α+Br,αRn,α, Rn+1,α=Al,αLn,α˜1l,α+Bl,αLn,α, α∈(0,1]. | (4.13) |
It is clear that (3.33) is satisfied and initial values x0 are positive fuzzy numbers, so from Theorem 3.3, Eq.(4.1) has a unique positive equilibrium ¯x=(0.333,0.3548,0.3793). Moreover every positive solution xn of Eq.(4.1) converges the unique equilibrium ¯x with respect to D as n→∞. (see Figure 4–Figure 6)
In this work, according to a generalization of division (g-division) of fuzzy number, we study the fuzzy discrete time Beverton-Holt population model xn+1=Axn˜1+Bxn. The existence of positive solution and qualitative behavior to (1.1) are investigated. The main results are as follows
(1) Under Case Ⅰ, the positive solution is bounded and persists if Al,α>˜1l,α,Ar,α>˜1r,α,α∈(0,1]. Every positive solution xn tends to the unique equilibrium x as n→∞.
(2) Under Case Ⅱ, the positive solution is bounded and persists if Al,α>˜1l,α,Ar,α>˜1r,α, and Bl,αBr,α≤Al,α−˜1l,αAr,α−˜1r,α,α∈(0,1]. Every positive solution xn tends to the unique equilibrium x as n→∞.
The authors would like to thank the editor and anonymous reviewers for their helpful comments and valuable suggestions, which have greatly improved the quality of this paper. This work was financially supported by the National Natural Science Foundation of China (Grant No.11761018)), by the Priority Projects of Science Foundation at Guizhou University of Finance and Economics (No. 2018XZD02) and by the Innovative Exploration and Academic Planted Talent Project of the Guizhou University of Finance and Economics.
The authors declare that they have no competing interests in this paper.
[1] | M. Kot, Elements of Mathematical Ecology, Cambridge University Press, New York, 2001. |
[2] | R. Beverton and S. Holt, On the dynamics of exploited fish populations, Fish. Invest. Ser 2, 19 (1957), 1–533. |
[3] | M. D. L. Sen, The generalized Beverton-Holt equation and the control of populations, Appl. Math. Model., 32 (2008), 2312–2328. |
[4] | M. D. L. Sen and S. Alonso-Quesada, Control issues for the Beverton-Holt equation in ecology by locally monitoring the environment carrying capacity: Nonadaptive and adaptive cases, Appl. Math. Comput., 215 (2009), 2616–2633. |
[5] | M. Bohner and S. Streipert, Optimal harvesting policy for the Beverton-Holt model, Math. Biosci. Eng., 13 (2016), 673–695. |
[6] | L. A. Zadeh, Fuzzy set, Inf. Control, 8 (1965), 338–353. |
[7] | E. Y. Deeba, A. De Korvin and E. L. Koh, A fuzzy difference equation with an application, J. Differ. Equ. Appl., 2 (1996), 365–374. |
[8] | E. Y. Deeba and A. De Korvin, Analysis by fuzzy difference equations of a model of CO2 level in the blood, Appl. Math. Lett., 12 (1999), 33–40. |
[9] | G. Papaschinopoulos and B. K. Papadopoulos, On the fuzzy difference equation xn+1=A+B/xn, Soft Comput., 6 (2002), 456--461. |
[10] | G. Papaschinopoulos and B. K. Papadopoulos, On the fuzzy difference equation xn+1=A+xn/xn−m, Fuzzy Set. Syst., 129 (2002), 73--81. |
[11] | G. Stefanidou, G. Papaschinopoulos and C. J. Schinas, On an exponential-type fuzzy difference equation, Adv. Differ. Equ., 2010 (2010), 1–19. |
[12] | Q. Din, Asymptotic behavior of a second order fuzzy rational difference equations, J. Discrete Math., 2015 (2015), 1–7. |
[13] | R. Memarbashi and A. Ghasemabadi, Fuzzy difference equations of volterra type, Int. J. Nonlinear Anal. Appl., 4 (2013), 74–78. |
[14] | K. A. Chrysafis, B. K. Papadopoulos and G. papaschinopoulos, On the fuzzy difference equations of finance, Fuzzy Set. Syst., 159 (2008), 3259–3270. |
[15] | Q. Zhang, L. Yang and D. Liao, Behaviour of solutions of to a fuzzy nonlinear difference equation, Iranian J. Fuzzy Syst., 9 (2012), 1–12. |
[16] | Q. Zhang, L. Yang and D. Liao, On first order fuzzy riccati difference equation, Inform. Sciences, 270 (2014), 226–236. |
[17] | Q. Zhang, J. Liu and Z. Luo, Dynamical behaviour of a third-order rational fuzzy difference equation, Adv. Differ. Equ., 2015 (2015). |
[18] | S. P. Mondal, D. K. Vishwakarma and A. K. Saha, Solution of second order linear fuzzy difference equation by Lagranges multiplier method, J. Soft Comput. Appl., 1 (2016), 11–27. |
[19] | Z. Alijani and F. Tchier, On the fuzzy difference equation of higher order, J. Comput. Complex. Appl., 3 (2017), 44–49. |
[20] | A. Khastan, Fuzzy Logistic difference equation, Iranian J. Fuzzy Syst., (2017), In Press. |
[21] | C. Wang, X. Su, P. Liu, et al., On the dynamics of a five-order fuzzy difference equation, J. Nonlinear Sci. Appl., 10 (2017), 3303–3319. |
[22] | A. Khastan and Z. Alijani, On the new solutions to the fuzzy difference equation xn+1=A+B/xn, Fuzzy Set. Syst., (2018), In Press. |
[23] | A. Khastan, New solutions for first order linear fuzzy difference equations, J. Comput. Appl. Math., 312 (2017), 156–166. |
[24] | D. Dubois and H. Prade, Possibility theory: an approach to computerized processing of uncertainty, Plenum Publishing Corporation, New York, 1998. |
[25] | L. Stefanini, A generalization of Hukuhara difference and division for interval and fuzzy arithmetic, Fuzzy Set. Sys., 161 (2010), 1564–1584. |
[26] | V. L. Kocic and G. Ladas, Global behavior of nonlinear difference equations of higher order with application, Kluwer Academic Publishers, Dordrecht, 1993. |
[27] | M. R. S. Kulenonvic and G. Ladas, Dynamics of second order rational difference equations with open problems and conjectures, Chapaman & Hall/CRC, Boca Raton, 2002. |
1. | Lili Jia, Dynamic Behaviors of a Class of High-Order Fuzzy Difference Equations, 2020, 2020, 2314-4629, 1, 10.1155/2020/1737983 | |
2. | Lili Jia, Changyou Wang, Qiang Yang, Existence and uniqueness of positive fuzzy solution for a three-order fuzzy difference equation, 2020, 1592, 1742-6588, 012050, 10.1088/1742-6596/1592/1/012050 | |
3. | Changyou Wang, Jiahui Li, Lili Jia, DYNAMICS OF A HIGH-ORDER NONLINEAR FUZZY DIFFERENCE EQUATION, 2021, 11, 2156-907X, 404, 10.11948/20200050 | |
4. | Abdul Alamin, Sankar Prasad Mondal, Shariful Alam, Ali Ahmadian, Soheil Salahshour, Mehdi Salimi, Solution and Interpretation of Neutrosophic Homogeneous Difference Equation, 2020, 12, 2073-8994, 1091, 10.3390/sym12071091 | |
5. | Qianhong Zhang, Ouyang Miao, Fubiao Lin, Zhongni Zhang, On discrete-time laser model with fuzzy environment, 2021, 6, 2473-6988, 3105, 10.3934/math.2021188 | |
6. | Qianhong Zhang, Wenzhuan Zhang, Fubiao Lin, Dongyang Li, On dynamic behavior of second-order exponential-type fuzzy difference equation, 2020, 01650114, 10.1016/j.fss.2020.07.021 | |
7. | Lili Jia, Xiaojuan Zhao, Changyou Wang, Qiyu Wang, DYNAMIC BEHAVIOR OF A SEVEN-ORDER FUZZY DIFFERENCE EQUATION, 2023, 13, 2156-907X, 486, 10.11948/20220340 | |
8. | Lili Jia, Changyou Wang, Xiaojuan Zhao, Wei Wei, Dynamic Behavior of a Fractional-Type Fuzzy Difference System, 2022, 14, 2073-8994, 1337, 10.3390/sym14071337 | |
9. | Qianhong Zhang, Miao Ouyang, Bairong Pan, Fubiao Lin, Qualitative analysis of second-order fuzzy difference equation with quadratic term, 2022, 1598-5865, 10.1007/s12190-022-01793-0 | |
10. | Muhammad Usman, Abdul Khaliq, Muhammad Azeem, Senesie Swaray, Mohamed Kallel, Kavikumar Jacob, The dynamics and behavior of logarithmic type fuzzy difference equation of order two, 2024, 19, 1932-6203, e0309198, 10.1371/journal.pone.0309198 | |
11. | Miao Ouyang, Qianhong Zhang, Mingji Cai, Zihao Zeng, Dynamic analysis of a fuzzy Bobwhite quail population model under g-division law, 2024, 14, 2045-2322, 10.1038/s41598-024-60178-4 | |
12. | Qianhong Zhang, Bairong Pan, Miao Ouyang, Fubiao Lin, Large time behavior of solution to second-order fractal difference equation with positive fuzzy parameters, 2023, 45, 10641246, 5709, 10.3233/JIFS-224099 | |
13. | Ibtissem Redjam, Yacine Halim, Michal Fečkan, On a higher order fuzzy difference equation with a quadratic term, 2024, 1598-5865, 10.1007/s12190-024-02243-9 | |
14. | Xu Teng, Changyou Wang, Lian Li, Unique Existence of Solution for A Fifth-order Fractional-type Fuzzy Difference Equation, 2024, 2890, 1742-6588, 012007, 10.1088/1742-6596/2890/1/012007 | |
15. | Asifa Tassaddiq, Muhammad Tanveer, Muhammad Usman, Dalal Khalid Almutairi, Rabab Alharabi, On the Global Dynamics of a Fourth-Order Riccati-Type Exponential Fuzzy Difference Equation, 2025, 14, 2075-1680, 118, 10.3390/axioms14020118 | |
16. | Osman Topan, Yasin Yazlik, Sevda Atpinar, Dynamics of fuzzy difference equations system with higher-order, 2025, 1598-5865, 10.1007/s12190-025-02440-0 |