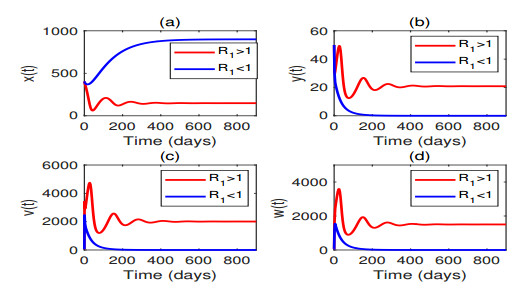
In this article, we investigate the dynamics of a stochastic HIV model with a Hill-type infection rate and distributed delay, which are better choices for mass action laws. First, we transform a stochastic system with weak kernels into a degenerate high-dimensional system. Then the existence of a stationary distribution is obtained by constructing a suitable Lyapunov function, which determines a sharp critical value Rs0 corresponding to the basic reproduction number for the determined system. Moreover, the sufficient condition for the extinction of diseases is derived. More importantly, the exact expression of the probability density function near the quasi-equilibrium is obtained by solving the Fokker-Planck equation. Finally, numerical simulations are illustrated to verify the theoretical results.
Citation: Wenjie Zuo, Mingguang Shao. Stationary distribution, extinction and density function for a stochastic HIV model with a Hill-type infection rate and distributed delay[J]. Electronic Research Archive, 2022, 30(11): 4066-4085. doi: 10.3934/era.2022206
[1] | Yifan Wu, Xiaohui Ai . Analysis of a stochastic Leslie-Gower predator-prey system with Beddington-DeAngelis and Ornstein–Uhlenbeck process. Electronic Research Archive, 2024, 32(1): 370-385. doi: 10.3934/era.2024018 |
[2] | Jia Chen, Yuming Chen, Qimin Zhang . Ergodic stationary distribution and extinction of stochastic pertussis model with immune and Markov switching. Electronic Research Archive, 2025, 33(5): 2736-2761. doi: 10.3934/era.2025121 |
[3] | Meng Gao, Xiaohui Ai . A stochastic Gilpin-Ayala nonautonomous competition model driven by mean-reverting OU process with finite Markov chain and Lévy jumps. Electronic Research Archive, 2024, 32(3): 1873-1900. doi: 10.3934/era.2024086 |
[4] | Wenhui Niu, Xinhong Zhang, Daqing Jiang . Dynamics and numerical simulations of a generalized mosquito-borne epidemic model using the Ornstein-Uhlenbeck process: Stability, stationary distribution, and probability density function. Electronic Research Archive, 2024, 32(6): 3777-3818. doi: 10.3934/era.2024172 |
[5] | Zhimin Li, Tailei Zhang, Xiuqing Li . Threshold dynamics of stochastic models with time delays: A case study for Yunnan, China. Electronic Research Archive, 2021, 29(1): 1661-1679. doi: 10.3934/era.2020085 |
[6] | Yuan Tian, Jing Zhu, Jie Zheng, Kaibiao Sun . Modeling and analysis of a prey-predator system with prey habitat selection in an environment subject to stochastic disturbances. Electronic Research Archive, 2025, 33(2): 744-767. doi: 10.3934/era.2025034 |
[7] | Bing Zhao, Shuting Lyu, Qimin Zhang . Dynamics and density function for a stochastic anthrax epidemic model. Electronic Research Archive, 2024, 32(3): 1574-1617. doi: 10.3934/era.2024072 |
[8] | Wenxuan Li, Suli Liu . Dynamic analysis of a stochastic epidemic model incorporating the double epidemic hypothesis and Crowley-Martin incidence term. Electronic Research Archive, 2023, 31(10): 6134-6159. doi: 10.3934/era.2023312 |
[9] | Tao Zhang, Mengjuan Wu, Chunjie Gao, Yingdan Wang, Lei Wang . Probability of disease extinction and outbreak in a stochastic tuberculosis model with fast-slow progression and relapse. Electronic Research Archive, 2023, 31(11): 7104-7124. doi: 10.3934/era.2023360 |
[10] | Ziang Chen, Chunguang Dai, Lei Shi, Gaofang Chen, Peng Wu, Liping Wang . Reaction-diffusion model of HIV infection of two target cells under optimal control strategy. Electronic Research Archive, 2024, 32(6): 4129-4163. doi: 10.3934/era.2024186 |
In this article, we investigate the dynamics of a stochastic HIV model with a Hill-type infection rate and distributed delay, which are better choices for mass action laws. First, we transform a stochastic system with weak kernels into a degenerate high-dimensional system. Then the existence of a stationary distribution is obtained by constructing a suitable Lyapunov function, which determines a sharp critical value Rs0 corresponding to the basic reproduction number for the determined system. Moreover, the sufficient condition for the extinction of diseases is derived. More importantly, the exact expression of the probability density function near the quasi-equilibrium is obtained by solving the Fokker-Planck equation. Finally, numerical simulations are illustrated to verify the theoretical results.
Human immunodeficiency virus (HIV) is the one of the most harmful diseases, and thus has always been a hot topic worthy of attention. Considering the crowding effect of the CD4+T cells (prey of virus), Bairagi and Adak[1] proposed the HIV-1 system with a Hill type function based on the following mass action principle:
dxdt=s−μx−βxnvan+xn,dydt=βxnvan+xn−(α+μ)y,dvdt=cαy−γv. | (1.1) |
Here x(t),y(t) and v(t) denote the concentrations of activated CD4+T cells, infected CD4+T cells and virus particles, respectively. n≥1 is a Hill constant. Other parameters can be referred to in [1]. Besides, from [1], the basic reproductive number for System (1.1) is as follows:
R1=βcαsnγ(α+μ)(anμn+sn). | (1.2) |
And when R1<1, the disease-free equilibrium E0(sμ,0,0) is globally asymptotically stable, and when R1>1, E0 is unstable and there exists a unique endemic equilibrium, which is globally asymptotically stable. Considering that the growth of infected CD4+T cells is affected not only by the number of normal cells, but also by the number of previous cells, we investigate the HIV system with distributed delay:
dxdt=s−μx−βxnvan+xn,dydt=β∫t−∞f(t−s)xn(s)v(s)an+xn(s)ds−(α+μ)y,dvdt=cαy−γv, | (1.3) |
where the kernel f(t)=tkσk+1e−σtk! is a gamma distribution initially proposed by Macdonald[2] and σ>0 is a mean delay. For convenience, we choose the weak kernel case (k=0), that is, f(t)=σe−σt. Let
w(t)=∫t−∞f(t−s)xn(s)v(s)an+xn(s)ds. |
Then System (1.3) is transformed into the equivalent form:
dxdt=s−μx−βxnvan+xn,dydt=βw−(α+μ)y,dvdt=cαy−γv,dwdt=−σw+σxnvan+xn. | (1.4) |
Xu [3] investigated the global asymptotic stability of an HIV-1 infection model with distributed intracellular delays. Using methods similar to those in Theorems 2.3 and 2.4 of [1] and Theorems 3.1 and 3.2 of [3], we obtain the following results.
Theorem 1.1. (i) The disease-free equilibrium point E0(sμ,0,0,0) of System (1.4) is globally asymptotically stable when R1<1 and unstable when R1>1, where R1 is defined in Eq.(1.2);
(ii) The endemic equilibrium point E∗(x∗,y∗,v∗,w∗) of System (1.4) is globally asymptotically stable when R1>1, where
x∗=a(Aβ−A)1n,y∗=γAcα(s−μa(Aβ−A)1n),v∗=cαγy∗,w∗=α+μβy∗, |
with A=(μ+α)γcα.
In addition, the infectious diseases are inevitably affected by environmental white noises[4,5,6]. In view of the above, we consider the following stochastic HIV-1 model with distributed delay:
dx=(s−μx−βxnvan+xn)dt+σ1xdB1(t),dy=(β∫t−∞σe−σ(t−s)xn(s)v(s)an+xn(s)ds−(α+μ)y)dt+σ2ydB2(t),dv=(cαy−γv)dt+σ3vdB3(t), | (1.5) |
where Bj(t),j=1,2,3 represent independent Brownion motions whose noise intensities are expressed as σ2j,j=1,2,3. In [7,8,9], the existence of a stationary distribution and the extinction of stochastic systems are studied based on the theory of Khasminskii [10] by constructing the suitable Lyapunov function, which implies that the diseases will be prevalent or tend to extinction. In [11], Guo and Zhang gave the numerical approximation for an HIV infection model incorporating the mean-reverting Ornstein-Uhlenbeck process. In [12], the extinction and the existence of a unique invariant probability measure for a stochastic HIV-1 infection model with degenerate diffusion were obtained. In [13], a group of stochastic dynamic models of the HIV/AIDS infection in a host population are presented, and global asymptotic and p−exponential stability of the disease-free equilibrium in probability was investigated. The most difficulty with our work is determining how to deal with the Hill-type infection rate and distributed delay when constructing the Lyapunov function and proving the positive definiteness.
Similarly, let
w(t)=∫t−∞σe−σ(t−s)xn(s)v(s)an+xn(s)ds. |
Then
dwdt=−σ2e−σt∫t−∞eσsxn(s)v(s)an+xn(s)ds+σxn(t)v(t)an+xn(t)=−σw(t)+σxn(t)v(t)an+xn(t). |
Thus, System (1.5) is transformed into the following equivalent form:
dx=(s−μx−βxnvan+xn)dt+σ1xdB1(t),dy=(βw−(α+μ)y)dt+σ2ydB2(t),dv=(cαy−γv)dt+σ3vdB3(t),dw=(−σw+σxnvan+xn)dt. | (1.6) |
In comparison with other existing results, the achieved contributions and innovations can be summarized as follows:
● A stochastic HIV model with a Hill-type infection rate and distributed delay is proposed, which may reflect more reality than the existing ones.
● The existence of a stationary distribution for System (1.6) is obtained by constructing a suitable Lyapunov function, which determines a critical value R∗0 corresponding to the basic reproduction number.
● The exact density function near the endemic quasi-equilibrium is given by solving the Fokker-Planck equation.
● Our main innovation is the development of a technique to deal with the Hill-type infection rate, which is different with the existing ones.
The rest of the article is organized as follows. In Section 2, the sufficient condition of the existence of a stationary distribution for the stochastic system given by Eq.(1.5) is derived; it determines a sharp critical value Rs0. In Section 3, the extinction of the diseases is investigated. In Section 4, the exact probability density function at the quasi-endemic equilibrium is derived. In Section 5, the numerical results are illustrated. Finally, the conclusion is given briefly.
The existence and uniqueness of the global positive solution of System (1.6) will be given. Since this is standard, we omit it.
Theorem 2. For any initial value (x(0),y(0),v(0),w(0))∈R4+, there exists a unique positive solution (x(t),y(t),v(t),w(t)) for System (1.6) on t≥0 and the solution will remain in R4+ with a probability of one.
Consider the following auxilary Logistic equation:
dXdt=s−μX+σ1X(t)dB1(t). | (2.1) |
Similar to Lemma 4.1 in [14] and [15], we have the following lemma:
Lemma 1. [14,15] Eq (2.1) has a unique stationary distribution with the density function f∗(⋅) defined by
f∗(z)=ba11Γ(a1)z−(a1+1)e−b1z,z>0, | (2.2) |
where a1=2μ+σ21σ21,b1=2sσ21 and Γ(⋅) is a Gamma function and the following equalities hold:
∫∞0zf∗(z)dz=limt→+∞1t∫t0X(t)dt=EX(t)=sμ,a.s. | (2.3) |
∫∞0znf∗(z)dz=bn1Γ(a1−n)Γ(a1),a.s.ifμ>(n−1)σ212. |
Proof. Eq.(2.1) has a unique stationary distribution with the density function f∗(z) on (0,∞), which is defined by Eq.(2.2) and Eq.(2.3) holds. In addition,
∫∞0znf∗(z)dz=ba11Γ(a1)∫∞0zn−a1−1e−b1zdz=bn1Γ(a1)∫∞0˜za1−n−1e−˜zd˜z=bn1Γ(a1−n)Γ(a1). |
Next, we consider the following integral equation:
X(t)=X(t0)+∫tt0b(s,X(s))ds+k∑r=1∫tt0σr(s,X(s))dBr(s),t≥t0≥0. | (2.4) |
Lemma 2. [10,16] Suppose that System (2.4) has a global positive solution and there exists a non-negative function V∈C2(R4+,R+) and a bounded closed set D such that LV≤−1 for R4+∖D. Then System (2.4) has a stationary distribution.
Next by applying a method similar to those in [7,8,9], which are based on the theory of Khasminskii [10] and combining Theorem 2.1 and Lemmas 2.1 and 2.2, we obtain the following main result.
Theorem 3. Assuming that Rs0>1 and μ>(n−1)σ212, System (1.6) has a unique stationary distribution π(⋅), where
Rs0=sμ+σ212(βcα(α+μ+σ222)(γ+σ232)(an+bn1Γ(a1−n)Γ(a1)))1n, | (2.5) |
where a1=2μ+σ21σ21 and b1=2sσ21.
Proof. By Theorem 2 and Lemma 2, we only need to construct a Lyapunov function. For convenience, denote F(x)=xnan+xn. Noting that ddx(F(x)x)=xn−2((n−1)an−xn)(an+xn)2, we have that F(x)x≤{1/a,n=1(F((n−1)1na))/((n−1)1na),n>1≜C1forx∈(0,+∞). By the first equation of Eq.(1.6), we have dx≤(s−μx)dt+σ1xdB1(t). By the comparison principle, we have that x(t)≤X(t),a.s. Define
¯V1=−lnx−c1lny−c2lnv−c3lnw+βC1γv, |
where
c1=sn(α+μ+σ222)n√βcα(α+μ+σ222)(γ+σ232)(an+∫+∞0xnπ(x)dx),c2=sn(γ+σ232)n√βcα(α+μ+σ222)(γ+σ232)(an+∫+∞0xnπ(x)dx),c3=sσnn√βcα(α+μ+σ222)(γ+σ232)(an+∫+∞0xnπ(x)dx). |
By Ito's formula, Lemma 1 and F(x)x≤C1, we have that,
L¯V1=−sx−c1βwy−c2cαyv−c3σF(x)vw+βF(x)vx+βC1cαyγ−βC1v+c1(α+μ+σ222)+c2(γ+σ232)+c3σ+(μ+σ212)≤−n∑k=1snx−c1βwy−c2cαyv−c3σF(x)vw+βF(x)vx+βC1cαyγ−βF(x)vx+(μ+σ212)+c1(α+μ+σ222)+c2(γ+σ232)+c3σ−c4(an+xn)+c4(an+xn) |
≤−n∑k=1snx−c1βwy−c2cαyv−c3σF(x)vw−c4(an+xn)+βC1cαyγ+(μ+σ212)+c1(α+μ+σ222)+c2(γ+σ232)+c3σ+c4(an+∫∞0znf∗(z)dz)+c4(Xn−∫∞0znf∗(z)dz)≤−(n+4)(c1c2c3c4βcασsnnn)1n+4+c1(α+μ+σ222)+c2(γ+σ232)+c3σ+c4(an+∫∞0znf∗(z)dz)+βC1cαyγ+(μ+σ212)+c4(Xn−∫∞0znf∗(z)dz)≤−s(βcα(α+μ+σ222)(γ+σ232)(an+∫∞0znf∗(z)dz))1n+(μ+σ212)+βC1cαyγ+c4(Xn−∫∞0znf∗(z)dz)=(1−Rs0)(μ+σ212)+βC1cαyγ+c4(Xn−∫∞0znf∗(z)dz), | (2.6) |
where Rs0 is defined in Eq.(2.5), and
c4=sn(an+∫∞0znf∗(z)dz)n√βcα(α+μ+σ222)(γ+σ232)(an+∫∞0znf∗(z)dz). |
The Itˆo formula is applied to
¯V2=−lnx+βC1γv−lnv−lnw,¯V3=1θ+1(x+y2+(α+μ)v4cα+βwσ)θ+1, |
where
0<θ<2kp,withp=max{σ21,σ22,σ23},k=min{μ,α+μ2,γ,σ2}. |
Then
L¯V2=−sx+βv(F(x)x−C1)+βC1cαyγ−cαyv−σF(x)vw+μ+γ+σ+12(σ21+σ23)≤−sx+βC1cαyγ−cαyv−σF(x)vw+μ+γ+σ+12(σ21+σ23), | (2.7) |
and
L¯V3≤(s−μx−βw2−(α+μ)y4−(α+μ)γv4cα)(x+y2+(α+μ)v4cα+βwσ)θ+θ2(x+y2+(α+μ)v4cα+βwσ)θ−1(σ21x2+σ224y2+σ23(α+μ)2(4cα)2v2)≤(s−μx−βw2−(α+μ)y4−(α+μ)γv4cα)(x+y2+(α+μ)v4cα+βwσ)θ+θ2p(x+y2+(α+μ)v4cα+βwσ)θ−1(x2+(y2)2+((α+μ)v4cα)2+(βwσ)2)≤s(x+y2+(α+μ)v4cα+βwσ)θ−k(x+y2+(α+μ)v4cα+βwσ)θ+1+θp2(x+y2+(α+μ)v4cα+βwσ)θ+1≤A−ρ2(x+y2+(α+μ)v4cα+βwσ)θ+1≤A−ρ2(xθ+1+(y2)θ+1+((α+μ)v4cα)θ+1+(βwσ)θ+1), | (2.8) |
where ρ=k−θp2>0, and
A:=max(x,y,v,w)∈R4+{s(x+y2+(α+μ)v4cα+βwσ)θ−ρ2(x+y2+(α+μ)v4cα+βwσ)θ+1}.
Define
¯V(x,y,v,w)=M¯V1+¯V2+¯V3, | (2.9) |
where M is a positive constant sufficiently enough satisfying
−M(Rs0−1)(μ+σ212)+μ+σ212+σ232+γ+σ+A≤−2. | (2.10) |
By Eqs.(2.6)–(2.10), we have that
L¯V(x,y,v,w)≤G(x,y,v,w)+Mc4(Xn−∫∞0znf∗(z)dz), | (2.11) |
where
G(x,y,v,w)=−2+(M+1)βC1cαyγ−sx−cαyv−σF(x)vw−ρ2(xθ+1+(y2)θ+1+((α+μ)v4cα)θ+1+(βwσ)θ+1). |
First, we consider the expression G(x,y,v,w) in two cases:
Case 1. If y→0+, then G(x,y,v,w)≤−2+(M+1)βC1cαyγ→−2.
Case 2. If x→∞ or x→0+ or y→∞ or v→∞ or w→∞ or y↛0+,v→0+ or x↛0+,v↛0+,w→0+, then
G(x,y,v,w)≤−2+H−sx−cαyv−σF(x)vw−ρ4(xθ+1+(y2)θ+1+((α+μ)v4cα)θ+1+(βwσ)θ+1)→−∞, |
where H=supy∈(0,+∞){(M+1)βC1cαyγ−ρ4(y2)θ+1}.
In light of the above, there exists a sufficiently small constant ε>0 such that
G(x,y,v,w)<−1,for(x,y,v,w)∈R4+∖Dε, |
where Dε={(x,y,v,w)∈R4+|ε≤x≤1ε,ε≤y≤1ε,ε2≤v≤1ε2,ε3≤w≤1ε3}.
By the continuity of G(x,y,v,w), there exists a positive constant K such that
G(x,y,v,w)≤K, for(x,y,v,w)∈R4+. |
Hence, integrating from 0 to t and taking the expectation for both sides of Eq.(2.11) give that
−E(¯V(x0,,y0,v0,w0))≤∫t0E(L(¯V(x(τ),y(τ),v(τ),w(τ))))dτ+Mc4E(∫t0Xn(τ)dτ−∫t0∫∞0znf∗(z)dzdτ). |
Let t→∞, then,
0≤lim inft→∞1t∫t0E(L(¯V(x(τ),y(τ),v(τ),w(τ))))dτ=lim inft→∞1t∫t0E(L(¯V(x(τ),y(τ),v(τ),w(τ)))I(x,y,v,w)∈R4+∖Dε)dτ+lim inft→∞1t∫t0E(L(¯V(x(τ),y(τ),v(τ),w(τ)))I(x,y,v,w)∈Dε)dτ≤lim inft→∞1t∫t0(−P((x(τ),y(τ),v(τ),w(τ))∈R4+∖Dε)+KP((x(τ),y(τ),v(τ),w(τ))∈Dε))dτ=lim inft→∞1t∫t0(−1+P((x(τ),y(τ),v(τ),w(τ))∈Dε)+KP((x(τ),y(τ),v(τ),w(τ))∈Dε))dτ≤−1+(1+K)lim inft→∞1t∫t0P((x(τ),y(τ),v(τ),w(τ))∈Dε)dτ, |
which implies that,
lim inft→∞1t∫t0P((x,y,v,w)∈Dε)dτ≥11+K, | (2.12) |
where P(t,(x,y,v,w),⋅) is the transition probability of (x(t),y(t),v(t),w(t)). By the invariance of R4+ and the inequality given by Eq.(2.12), there exists an invariant probability measure π(⋅) on R4+, (see [17,18]). The result is confirmed.
Theorem 4. Let (x(t),y(t),v(t),w(t)) be a solution of System (1.6) with any initial value (x(0),y(0),v(0),w(0))∈R4+. Then the following results will hold:
limt→+∞supln(δ1y(t)+δ2v(t)+δ3w(t))t≤ˉμ, |
where
δ1=1α+μ,δ2=λcα,δ3=β(α+μ)λσ,λ=3√R1,κ=max{α+μ,γ,σ)}(λ−1)I{λ≥1}+min{α+μ,γ,σ}(λ−1)I{λ≤1},ˉμ=κ+σδ3δ2∫∞0|F(X)−F(sμ)|f∗(X)dX, | (3.1) |
where R1 is defined by Eq.(1.2).
Especially, if ˉμ<0, then the infected CD4+T cells population y(t) and virus particle v(t) will die out exponentially with a probability of one.
Proof. Define
z(t)=δ1y(t)+δ2v(t)+δ3w(t). |
Applying the Itˆo formula,
d(lnz)=L(lnz)dt+δ1σ2yzdB2(t)+δ2σ3vzdB3(t), | (3.2) |
where
L(lnz)=1z(δ1βw+δ2cαy+δ3σF(x)v−δ1(α+μ)y−δ2γv−δ3σw)−12z2(δ21σ22y2+δ22σ23v2)≤1z(δ1βw+δ2cαy+δ3σF(sμ)v−δ1(α+μ)y−δ2γv−δ3σw)+vzσδ3(F(x)−F(sμ))=1z(δ1(α+μ),δ2γ,δ3σ)(Ms(y,v,w)T−(y,v,w)T)+vzσδ3(F(x)−F(sμ))≤1z(λ−1)(δ1(α+μ)y+δ2γv+δ3σw)+vzσδ3(F(X)−F(sμ))≤max{α+μ,γ,σ)}(λ−1)I{λ≥1}+min{α+μ,γ,σ}(λ−1)I{λ≤1}+σδ3δ2|F(X)−F(sμ)|, |
and the monotone increasing property of F(X) with Ms=(00βα+μcαγ000F(sμ)0), and λ=3√R1=3√βcαF(sμ)(α+μ)γ satisfies (δ1(α+μ),δ2γ,δ3σ)Ms=λ(δ1(α+μ),δ2γ,δ3σ). Integrating both sides of Eq.(3.2) yields that,
lnz(t)t≤lnz(0)t+max{α+μ,γ,σ)}(λ−1)I{λ≥1}+min{α+μ,γ,σ}(λ−1)I{λ≤1}+σδ3δ21t∫t0|F(X(τ))−F(sμ)|dτ+M1(t)t+M2(t)t, | (3.3) |
where M1(t)=∫t0δ1σ2y(τ)z(τ)dB2(τ)andM2(t)=∫t0δ2σ3v(τ)z(τ)dB3(τ). Noting that the quadratic variation ⟨M1,M1⟩tt=σ22t∫t0δ21y2(τ)z2(τ)dτ≤σ22<∞,⟨M2,M2⟩tt≤σ23<∞,
limt→∞Mi(t)t=0,a.s.,i=1,2. | (3.4) |
From the ergodic theorem and Eq.(3.3) and Eq.(3.4), it follows that,
limt→+∞suplnz(t)t≤max{α+μ,γ,σ)}(λ−1)I{λ≥1}+min{α+μ,γ,σ}(λ−1)I{λ≤1}+σδ3δ2∫∞0|F(x)−F(sμ)|f∗(x)dx:=ˉμ,a.s.. |
If ˉμ<0, it is obvious to get limt→+∞z(t)=0, a.s., which implies that limt→+∞y(t)=limt→+∞v(t)=limt→+∞w(t)=0, a.s. In the other words, infected cells will exponentially decrease to zero with a probability of one.
We have obtained the existence of the stationary distribution of System (1.6). Next we give the details about the local probability density function of System (1.6).
First, let ˉx=lnx, ˉy=lny, ˉv=lnv, ¯w=lnw, then by Itˆo's formula, System (1.6) is transformed into the following form:
dˉx=(se−ˉx−βe(n−1)ˉx+ˉvan+enˉx−μ1)dt+σ1dB1(t),dˉy=(βeˉw−ˉy−μ2−α)dt+σ2dB2(t),dˉv=(cαeˉy−ˉv−μ3)dt+σ3dB3(t),dˉw=(σenˉx+ˉv−ˉwan+enˉx−σ)dt, | (4.1) |
where μi=μ+σ2i2,i=1,2andμ3=γ+σ232.
Define
Rs1=βcαsn(anμn1+sn)(μ2+α)μ3, | (4.2) |
which is the same as Eq.(1.2) when σi=0(i=1,2,3). Define a quasi-endemic equilibrium ~E∗=(~x∗,~y∗,~v∗,~w∗)=(e¯x∗,e¯y∗,e¯v∗,e¯w∗), where
~x∗=a(˜Aβ−˜A)1n,~y∗=μ3˜Acα(s−μ1a(˜Aβ−˜A)1n),~v∗=cαμ3~y∗,~w∗=α+μ2β~y∗, | (4.3) |
where ˜A = (μ2+α)μ3cα. And ~E∗ exists if and only if Rs1>1, and ~E∗ is the same with the endemic equilibrium E∗ of the determined system given by Eq.(1.4) when there is no white noises.
Let (ˆx,ˆy,ˆv,ˆw) = (ˉx−¯x∗,ˉy−¯y∗,ˉv−¯v∗,ˉw−¯w∗), then the linearized system of Eq.(4.1) at (¯x∗,¯y∗,¯v∗,¯w∗) is as follows:
dˆx=(−a11ˆx−a13ˆv)dt+σ1dB1(t),dˆy=(−a22ˆy+a22ˆw)dt+σ2dB2(t),dˆv=(a32ˆy−a32ˆv)dt+σ3dB3(t),dˆw=(a41ˆx+a43ˆv−a43ˆw)dt, | (4.4) |
where, combining (4.3),
a11=μ1+nβane(n−1)¯x∗+¯v∗(an+en¯x∗)2>0,a13=βe¯w∗−¯x∗>0,a22=μ2+α>0,a32=cαe¯y∗−¯v∗=μ3>0,a41=nanσen¯x∗+¯v∗−¯w∗(an+en¯x∗)2>0,a43=σen¯x∗+¯v∗−¯w∗an+en¯x∗=σ>0. |
It is obvious that
a11a43=μ1σ+a41a13>a41a13. | (4.5) |
Theorem 5. Let Y=(ˆx,ˆy,ˆv,ˆw) be a solution to Eq.(4.4) with the initial value (ˆx(0),ˆy(0),ˆv(0),ˆw(0))∈R4. If Rs1>1, then there exists a unique density function Φ(Y) around the quasi-equilibrium ~E∗, which can be approximately expressed in the following form
Φ(Y)=(2π)−2|Σ|−12e−12(ˆx,ˆy,ˆv,ˆw)Σ−1(ˆx,ˆy,ˆv,ˆw), |
in which Σ=Σ1+Σ2+Σ3 is positive definite, Rs1 is defined by Eq.(4.2), Σ1=ρ21M−11Θ1(M−11)T and Σ2,Σ3 will be described below for different cases.
Proof. System (4.4) can be rewritten into dY=AYdt+ΛdB(t), where Y=(ˆx,ˆy,ˆv,ˆw)T, Λ=diag(σ1,σ2,σ3,0), B(t)=(B1(t),B2(t),B3(t),0) and
A=(−a110−a1300−a220a220a32−a320a410a43−a43). |
According to [19], the four dimensional Fokker-Planck equation to describe a density function Φ(Y)=Φ(ˆx,ˆy,ˆv,ˆw) of the stationary distribution of Eq.(4.4) around the quasi-equilibrium ~E∗ is as follows:
−σ21∂2Φ2∂ˆx2−σ22∂2Φ2∂ˆy2−σ23∂2Φ2∂ˆv2+∂∂ˆx((−a11ˆx−a13ˆv)Φ)+∂∂ˆy((−a22ˆy+a22ˆw)Φ)+∂∂ˆv((a32ˆy−a32ˆv)Φ)+∂∂ˆw((a41ˆx+a43ˆv−a43ˆw)Φ)=0, |
which is an approximate representation of the Gaussian distribution Φ(Y)=ke−12(Y−Y∗)Q(Y−Y∗)T, with Y∗=(0,0,0,0); also, Q is a real symmetric matrix, which satisfies QΛ2Q+ATQ+QA=0. If Q is positive-definite, let Q−1=Σ, then Λ2+AΣ+ΣAT=0. By the finite independent superposition principle,
Λ2i+AΣi+ΣiAT=0,i=1,2,3, | (4.6) |
where Σ=Σ1+Σ2+Σ3,Λ2=Λ21+Λ22+Λ23,Λ1=diag(σ1,0,0,0),Λ2=diag(0,σ2,0,0), Λ3=diag(0,0,σ3,0).
Step 1. For System (4.4), we consider
Λ21+AΣ1+Σ1AT=0. | (4.7) |
Let
M1=(a22a32a41a222a32+a22a232+a332−a332+a22a43a32−a222a32−a22a232−a43a22a320−a232−a22a32a232a22a320a32−a3200010), |
then
B1=M1AM−11=(−N1−N2−N3−N4100001000010), | (4.8) |
where
N1=a11+a22+a32+a43>0,N2=a11a22+a11a32+a11a43+a22a32+a22a43+a32a43>0,N3=a11(a43a22+a43a32+a22a32)>0,N4=a13a22a32a41>0. | (4.9) |
Then, by incorporating Eq.(4.8), Eq.(4.7) is transformed into the following form:
M1Λ21MT1+B1(M1Σ1MT1)+(M1Σ1MT1)BT1=0, |
which implies that
1σ21Λ21+B1Θ1+Θ1BT1=0, | (4.10) |
where Θ1=1ρ21M1Σ1MT1 with ρ1=a22a32a41σ1.
By tedious and complex computation and the incorporation of (4.5), we get that
N1(N2N3−N1N4)−N23>a311(a32+a43)(a22(a22+a32+a43)+a32a43)+a211(a22a43((a22+a43)2+3a32(a22+a32+a43))+a22a32(a22+a32)2+a32a43(a32+a43)2)+a11(a243(a222(a22+a43)+a232(a32+a43))+a222a232(a22+a32+3a43)+a43a22a32(a43(3a32+a22)+(a43+a22)2))>0. |
By Lemma 3.1 of [20], Θ1 is positive definite. Then Σ1=ρ21M−11Θ1(M−11)T is also a positive definite matrix.
Step 2. For System (4.4), we consider
Λ22+AΣ2+Σ2AT=0. | (4.11) |
Let
T1=M2AM−12=(−a220−a22a43a13a22a32−a32000−a13−a11000ω1a13−a43)withM2=(010000101000a43a13001), |
where ω1=a243−a11a43+a13a41.
Case 2.1. If ω1≠0, we find the standard matrix M21 such that B1=M21T1M−121, where
M21=(−a32ω1(a11+a32+a43)ω1(a211+a11a43+a243)ω1a13−a3430−ω1−(a11+a43)ω1a13a24300ω1a13−a430001), |
and B1 is defined in Eq.(4.8). Similar to Step 1, Eq.(4.11) is transformed into the following equation:
1σ22Λ22+B1Θ1+Θ1BT1=0, |
which is the same with Eq.(4.10), and Θ1 is positive definite which implies that Σ2=ρ221(M21M2)−1Θ1(M−12M−121)T with ρ21=a32|ω1|σ2 is also positive definite.
Case 2.2. If ω1=0, there exists a new standard matrix M22 such that B2=M22T1M−122, where
M22=(−a13a32a11a13+a13a32a21100−a13−a11000100001),B2=(−H11−H12−H13−H1410000100000−a43). |
Similarly, Eq (4.11) is transformed into the following form
(M22M2)Λ22(M22M2)T+B2(M22M2)Σ2(M22M2)T+(M22M2)Σ2(M22M2)TBT2=0, |
which implies that
1σ22Λ22+B2Θ2+Θ2BT2=0, | (4.12) |
where Θ2=1ρ222(M22M2)Σ2(M22M2)T with ρ22=a13a32σ2. Direct computation induces that
H11=a11+a22+a32>0,H13=a22a32(a11−a43)=a22a32a13a41a43>0,and byω1=0,H11H12−H13=a211(a22+a32)+a222(a11+a32)+a22a32(2a11+a43+a32)+a11a232>0. |
By Lemma 3.2 of [20], Θ2 is semi-positive definite. Then Σ2=ρ222(M22M2)−1Θ2((M22M2)−1)T is also semi-positive definite.
Hence, for System (4.4), Σ2 is positive definite for ω1≠0 or semi-positive definite for ω1=0.
Step 3. For System (4.4), we consider the following algebraic equation:
Λ23+AΣ3+Σ3AT=0. | (4.13) |
Likewise, let
T2=M3AM−13=(−a3200a32a43−a43−a13a41a43a4100−a13ω1a243a13a41a43−a1100a220−a22)withM3=(00100001100a13a430100). |
Next, we discuss them in three cases.
Case 3.1. If ω1≠0, let C3=P3T2P3−1, where
P3=(10000100001000a22a243a13ω11),C3=(−a320−a22a32a243a13ω1a32a43−a43−a13a41a43a4100−a13ω1a243a13a41a43−a11000a22a43ω2a13ω1−a22), |
with ω2=a13a41−a11a43+a22a43.
Case 3.1.1. If ω2≠0, there exists a standard matrix M31 such that B1=M31C3(M−131), where
M31=(−a22ω2a22(a11+a22+a43)ω2a43ω31−a3220−a22ω2a43−a22ω2(a11a43−a13a41+a22a43)a13ω1a22200a22a43ω2a13ω1−a220001) |
with
ω31=−a22a13ω1(a11(a11a43−a13a41)2−a13a41(−a22ω1+a11a243−a13a41a43)−a322a243), |
and B1 is defined in Eq.(4.8). Similar to Step 1, Eq.(4.13) is transformed into the following equation:
1σ23Λ23+B1Θ1+Θ1BT1=0, |
which is the same with Eq.(4.10), and Θ1 is positive definite which implies that Σ3=ρ231(M31P3M3)−1Θ1((M31P3M3)−1)T with ρ31=a22|ω2|σ3>0 is also positive definite.
Case 3.1.2. If ω2=0, then
B3≜M32C3M−132=(−H21−H22−H23−H2410000100000−a22), |
where
M32=(−a13ω1a43a13(a11+a43)ω1a243a43a211−a13a41a11−a13a41a43a4300−a13ω1a243a13a41a43−a11000100001). |
Direct computation yields that
H21=a11+a32+a43>0,H23=a32a43(a11−a22)=a32a13a41>0,and byω2=0,H21H22−H23=(a11+a32)(a11a32+a11a43+a243)+a22a32a43>0. |
Similarly, Eq.(4.13) is transformed into the following equation:
1σ23Λ23+B3Θ3+Θ3BT3=0. |
By Lemma 3.2 of [20], Θ3 is semi-positive definite, which implies that Σ3=ρ232(M32P3M3)−1Θ2((M32P3M3)−1)T with ρ32=a13|ω1|a43σ3>0 is also semi-positive definite.
Case 3.2. If ω1=0, then
B4≜M33T2M−133=(−H31−H32−H33−H3410000100000a13a41a43−a11), |
where
M33=(a22a43−a222−a22(a43+a13a41a43)a22a41a2220a220−a2200010010), |
and
H31=1a43(a13a41+a22a43+a32a43+a243)>0,H33=a13a22a32a41a43>0,H31H32−H33=1a243(a22a43+a32a43+a13a41+a243)(a22a243+a13a41(a22+a32))+a22a32a43(a22+a32+a43)>0. |
Similarly, Eq.(4.13) is transformed into the following form:
1σ23Λ23+B4Θ4+Θ4BT4=0. |
By Lemma 3.2 of [20], Θ4 is semi-positive definite, which implies that Σ3=ρ233(M33M3)−1Θ4((M33M3)−1)T with ρ33=a22a43σ3>0 is also semi-positive definite.
Hence, for System (4.4), Σ3 is positive definite for ω1≠0,ω2≠0 and semi-positive definite for ω1≠0 and ω2=0 or ω1=0.
With all the things above, Σ=Σ1+Σ2+Σ3 in Eq.(4.6) is positive definite. Thus, there is a local asymptotic density function Φ(ˆx,ˆy,ˆv,ˆw) near the quasi-endemic equilibrium ˜E∗.
In this section, we give some numerical simulations to illustrate our theoretical results.
For the ODE system given by Eq.(1.4), the parameters refer to the data in [1] as follows
s=9,μ=0.01,β=0.005,a=50,c=550,σ=0.5,α=0.35,γ=2,n=1. | (5.1) |
By Eq.(1.2), we obtain R1=βcαsnγ(α+μ)(anμn+sn)≈1.2664>1; then, there exists an endemic equilibrium point E∗(x∗,y∗,v∗,w∗), where x∗=a(Aβ−A)1n≈148.45,y∗=γAcα(s−μx∗)≈20.8763, v∗=cαγy∗≈2009.3andw∗=α+μβy∗≈1503.1, with A=(μ+α)γcα≐0.0037. By Theorem 1.1(ii), the endemic equilibrium point E∗ is globally asymptotically stable, as illustrated in Figure 1 (red lines). By decreasing the value c by c=400, we obtain R1=0.9211<1. By Theorem 1.1(i), the disease-free equilibrium point E0(900,0,0,0) is globally asymptotically stable, as illustrated in Figure 1 (blue lines).
In the subsection, we consider the effect of white noises on the HIV system and establish the discretized system by Milstein method[21]:
xk+1=xk+(s−μxk−βxnkvkan+xnk)Δt+σ1xk√Δtξk+12σ21xk(ξ2k−1)Δt,yk+1=yk+(βwk−(α+μ)yk)Δt+σ2yk√Δtζk+12σ22yk(ζ2k−1)Δt,vk+1=vk+(cαyk−γvk)Δt+σ3vk√Δtςk+12σ23vk(ς2k−1)Δt,wk+1=wk+(−σwk+σxnkvkan+xnk)Δt, |
where ξk,ζk,ςk(k=1,2,⋯) are independent Gaussian random variables, which satisfy the standard normal distribution N(0,1).
Fixing the same parameters as Eq.(5.1), we choose the noise intensities σ1=0.05,σ2=0.08,σ3=0.08. By Eq.(2.5), we get that
Rs0=sμ+σ212(βcα(α+μ+σ222)(γ+σ232)(an+bn1Γ(a1−n)Γ(a1)))1n=1.2451>1. |
Then by Theorem 3, there exists a stationary distribution for the degenerate system given by Eq.(1.6), which implies the persistence of the disease, as illustrated in the left graph of Figure 2. The right graph of Figure 2 demonstrates the distribution of a density function near the deterministic steady state.
Further increasing the noise intensities σ1=σ2=σ3=0.3 and choosing μ=0.07,β=0.005,c=50,α=0.5,a=100andσ=0.9, we obtain from Eq.(3.1) that ˉμ=−0.1715<0. From Theorem 4, we know that the infected CD4+T cell population y(t) and virus particle v(t) tend to extinction (see Figure 3).
Some authors give the numerical simulations and theoretical analysis for an HIV infection model with CD4+ T-cells. For example, Evirgen et al.[22] gave the existence and uniqueness of the solutions for a fractionalized HIV infection model with the Atangana-Baleanu fractional derivative by using the Arzela-Ascoli theorem. Umar et al. [23] provided the numerical outcomes of a nonlinear HIV infection system, which is different from the Runge-Kutta method. Dewasurendra et al.[24] applied the MDDiM method to an HIV infection model of CD4+ T-cells, which shows the advantages over HAM.
Our main difference is the proposal of an HIV model with a Hill-type infection rate xnan+xn(n≥1) and distributed delay under the disturbance of white noise and proof of the existence of a stationary distribution by constructing a suitable Lyapunov function, which is a vast challenge. More importantly, we have given the exact local probability density function near the quasi-equilibrium by solving the corresponding Fokker-Planck equation. We have given the rigorous mathematical proof by describing the dynamics of the system, not only the numerical simulations.
In this paper, we first demonstrated the global asymptotical stability of the disease-free equilibrium and endemic equilibrium for the deterministic system. Second, the existence of a stationary distribution for an equivalent degenerate stochastic system was derived to obtain the sharp critical value Rs0 by using the theory of Khasminskii. Rs0 is consistent with the basic reproduction number without the white noises. Under a certain condition, the sufficient conditions for the extinction of the diseases have been given. In the part of numerical simulation, by incorporating the experimental data [25], we have applied the default parameter values given in Table 1 of [1] to verify the effectiveness of a stochastic system with degenerate diffusion.
There are still many interesting and instructive issues worthy of further study. For example, we consider the existence and uniqueness of the positive periodic solutions for the complex periodic system.
The work was supported by the Fundamental Research Funds for the Central Universities (No. 22CX03013A) and Shandong Provincial Natural Science Foundation (Nos. ZR2020MA039, ZR202102250288).
The authors declare that there is no conflicts of interest.
[1] |
N. Bairagi, D. Adak, Global analysis of HIV-1 dynamics with Hill type infection rate and intracellular delay, Appl. Math. Model., 38 (2014), 5047–5066. https://doi.org/10.1016/j.apm.2014.03.010 doi: 10.1016/j.apm.2014.03.010
![]() |
[2] | N. Macdonald, Time Lags in Biological Models, in: Lecture Notes in Biomathematics, Springer-Verlag, Heidelberg, 27 (1978). |
[3] |
R. Xu, Global dynamics of an HIV-1 infection model with distributed intracellular delays, Comput. Math. Appl., 61 (2011), 2799–2805. https://doi.org/10.1016/j.camwa.2011.03.050 doi: 10.1016/j.camwa.2011.03.050
![]() |
[4] |
X. Zhang, Q. Yang, Threshold behavior in a stochastic SVIR model with general incidence rates, Appl. Math. Lett., 121 (2021), 107403. https://doi.org/10.1016/j.aml.2021.107403 doi: 10.1016/j.aml.2021.107403
![]() |
[5] |
W. Zuo, Y. Zhou, Density function and stationary distribution of a stochastic SIR model with distributed delay, Appl. Math. Lett., 129 (2022), 107931. https://doi.org/10.1016/j.aml.2022.107931 doi: 10.1016/j.aml.2022.107931
![]() |
[6] | X. Mu, D. Jiang, A. Alsaedi, Analysis of a Stochastic Phytoplankton-Zooplankton Model under Non-degenerate and Degenerate Diffusions, J. Nonlinear Sci., 32 (2022). https://doi.org/10.1007/s00332-022-09787-9 |
[7] |
Y. Wang, D. Jiang, H. Tasawar, A. Alsaedi, Stationary distribution of an HIV model with general nonlinear incidence rate and stochastic perturbations, J. Franklin Inst., 356 (2019), 6610–6637. https://doi.org/10.1016/j.jfranklin.2019.06.035 doi: 10.1016/j.jfranklin.2019.06.035
![]() |
[8] |
Q. Liu, D. Jiang, Stationary distribution and extinction of a stochastic SIR model with nonlinear perturbation, Appl. Math. Lett., 73 (2017), 8–15. https://doi.org/10.1016/j.aml.2017.04.021 doi: 10.1016/j.aml.2017.04.021
![]() |
[9] |
M. Song, W. Zuo, D. Jiang, H. Tasawar, Stationary distribution and ergodicity of a stochastic cholera model with multiple pathways of transmission, J. Franklin Inst., 357 (2020), 10773–10798. https://doi.org/10.1016/j.jfranklin.2020.04.061 doi: 10.1016/j.jfranklin.2020.04.061
![]() |
[10] | R. Khasminskii, Stochastic Stability of Differential Equations, Springer, Heidelberg, 1980. https://doi.org/10.1007/978-3-642-23280-0 |
[11] |
W. Guo, Q. Zhang, Explicit numerical approximation for an impulsive stochastic age-structured HIV infection model with Markovian switching, Math. Comput. Simulat., 182 (2021), 86–115. https://doi.org/10.1016/j.matcom.2020.10.015 doi: 10.1016/j.matcom.2020.10.015
![]() |
[12] |
T. Feng, Z. Qiu, X. Meng, L. Rong, Analysis of a stochastic HIV-1 infection model with degenerate diffusion, Appl. Math. Comput., 348 (2019), 437–455. https://doi.org/10.1016/j.amc.2018.12.007 doi: 10.1016/j.amc.2018.12.007
![]() |
[13] |
Y. Emvudu, D. Bongor, R. Koïna, Mathematical analysis of HIV/AIDS stochastic dynamic models, Appl. Math. Model., 40 (2016), 9131–9151. https://doi.org/10.1016/j.apm.2016.05.007 doi: 10.1016/j.apm.2016.05.007
![]() |
[14] |
D. Nguyen, G. Yin, C. Zhu, Long-term analysis of a stochastic SIRS model with general incidence rates, SIAM J. Appl. Math., 80 (2020), 814–838. https://doi.org/10.1137/19M1246973 doi: 10.1137/19M1246973
![]() |
[15] |
Y. Lin, D. Jiang, P. Xia, Long-time behavior of a stochastic SIR model, Appl. Math. Comput., 236 (2014), 1–9. https://doi.org/10.1016/j.amc.2014.03.035 doi: 10.1016/j.amc.2014.03.035
![]() |
[16] |
D. Xu, Y. Huang, Z. Yang, Existence theorems for periodic Markov process and stochastic functional differential equations, Discrete Contin. Dyn. Syst., 24 (2009), 1005–1023. https://doi.org/10.3934/dcds.2009.24.1005 doi: 10.3934/dcds.2009.24.1005
![]() |
[17] |
N. Du, N. Dang, G. Yin, Conditions for permanence and ergodicity of certain stochastic predator-prey models, J. Appl. Probab., 53 (2016), 187–202. https://doi.org/10.1017/jpr.2015.18 doi: 10.1017/jpr.2015.18
![]() |
[18] |
S. P. Meyn, R. L. Tweedie, Stability of Markovian processes III: Foster-Lyapunov criteria for continuous-time processes, Adv. Appl. Probab., 25 (1993), 518–548. https://doi.org/10.2307/1427522 doi: 10.2307/1427522
![]() |
[19] | H. Roozen, An asymptotic solution to a two-dimensional exit problem arising in population dynamics, SIAM J. Appl. Math., 49 (1989). https://doi.org/10.1137/0149110 |
[20] |
J. Ge, W. Zuo, D. Jiang, Stationary distribution and density function analysis of a stochastic epidemic HBV model, Math. Comput. Simulat., 191 (2022), 232–255. https://doi.org/10.1016/j.matcom.2021.08.003 doi: 10.1016/j.matcom.2021.08.003
![]() |
[21] |
D. Higham, An algorithmic introduction to numerical simulation of stochastic differential equations, SIAM Rev., 43 (2001), 525–546. https://doi.org/10.1137/S0036144500378302 doi: 10.1137/S0036144500378302
![]() |
[22] |
F. Evirgen, S. Ucar, N. ¨Ozdemir, System analysis of HIV infection model with CD4+ T under Non-Singular kernel derivative, Appl. Math. Nonlinear Sci., 5 (2020), 139–146. https://doi.org/10.2478/amns.2020.1.00013 doi: 10.2478/amns.2020.1.00013
![]() |
[23] |
M. Umar, Z. Sabir, M. A. Z. Raja, H. M. Baskonus, S. W. Yao, E. Ilhan, A novel study of Morlet neural networks to solve the nonlinear HIV infection system of latently infected cells, Results Phys., 25 (2021), 104235. https://doi.org/10.1016/j.rinp.2021.104235 doi: 10.1016/j.rinp.2021.104235
![]() |
[24] |
M. Dewasurendra, Y. Zhang, N. Boyette, I. Islam, K. Vajravelu, A method of directly defining the inverse mapping for a HIV infection of CD4+ T-cells model, Appl. Math. Nonlinear Sci., 6 (2021), 469–482. https://doi.org/10.2478/amns.2020.2.00035 doi: 10.2478/amns.2020.2.00035
![]() |
[25] |
D. Ho, A. Neumann, A. Perelson, W. Chen, J. Leonard, M. Markowitz, Rapid turnover of plasma virons and CD4 lymphocytes in HIV-1 infection, Nature, 373 (1995), 123–126. https://doi.org/10.1038/373123a0 doi: 10.1038/373123a0
![]() |
1. | Baoquan Zhou, Hao Wang, Tianxu Wang, Daqing Jiang, Stochastic generalized Kolmogorov systems with small diffusion: I. Explicit approximations for invariant probability density function, 2024, 382, 00220396, 141, 10.1016/j.jde.2023.10.057 | |
2. | Ziang Chen, Chunguang Dai, Lei Shi, Gaofang Chen, Peng Wu, Liping Wang, Reaction-diffusion model of HIV infection of two target cells under optimal control strategy, 2024, 32, 2688-1594, 4129, 10.3934/era.2024186 |