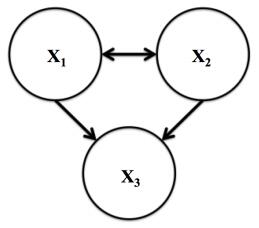
Citation: Tin Phan, Changhan He, Alejandro Martinez, Yang Kuang. Dynamics and implications of models for intermittent androgen suppression therapy[J]. Mathematical Biosciences and Engineering, 2019, 16(1): 187-204. doi: 10.3934/mbe.2019010
[1] | Alacia M. Voth, John G. Alford, Edward W. Swim . Mathematical modeling of continuous and intermittent androgen suppression for the treatment of advanced prostate cancer. Mathematical Biosciences and Engineering, 2017, 14(3): 777-804. doi: 10.3934/mbe.2017043 |
[2] | Cassidy K. Buhler, Rebecca S. Terry, Kathryn G. Link, Frederick R. Adler . Do mechanisms matter? Comparing cancer treatment strategies across mathematical models and outcome objectives. Mathematical Biosciences and Engineering, 2021, 18(5): 6305-6327. doi: 10.3934/mbe.2021315 |
[3] | Zhimin Wu, Tin Phan, Javier Baez, Yang Kuang, Eric J. Kostelich . Predictability and identifiability assessment of models for prostate cancer under androgen suppression therapy. Mathematical Biosciences and Engineering, 2019, 16(5): 3512-3536. doi: 10.3934/mbe.2019176 |
[4] | Avner Friedman, Harsh Vardhan Jain . A partial differential equation model of metastasized prostatic cancer. Mathematical Biosciences and Engineering, 2013, 10(3): 591-608. doi: 10.3934/mbe.2013.10.591 |
[5] | Rujing Zhao, Xiulan Lai . Evolutionary analysis of replicator dynamics about anti-cancer combination therapy. Mathematical Biosciences and Engineering, 2023, 20(1): 656-682. doi: 10.3934/mbe.2023030 |
[6] | Abeer S. Alnahdi, Muhammad Idrees . Nonlinear dynamics of estrogen receptor-positive breast cancer integrating experimental data: A novel spatial modeling approach. Mathematical Biosciences and Engineering, 2023, 20(12): 21163-21185. doi: 10.3934/mbe.2023936 |
[7] | Heyrim Cho, Allison L. Lewis, Kathleen M. Storey, Anna C. Zittle . An adaptive information-theoretic experimental design procedure for high-to-low fidelity calibration of prostate cancer models. Mathematical Biosciences and Engineering, 2023, 20(10): 17986-18017. doi: 10.3934/mbe.2023799 |
[8] | Leonardo Schultz, Antonio Gondim, Shigui Ruan . Gompertz models with periodical treatment and applications to prostate cancer. Mathematical Biosciences and Engineering, 2024, 21(3): 4104-4116. doi: 10.3934/mbe.2024181 |
[9] | Erin N. Bodine, K. Lars Monia . A proton therapy model using discrete difference equations with an example of treating hepatocellular carcinoma. Mathematical Biosciences and Engineering, 2017, 14(4): 881-899. doi: 10.3934/mbe.2017047 |
[10] | Lu Gao, Yuanshun Tan, Jin Yang, Changcheng Xiang . Dynamic analysis of an age structure model for oncolytic virus therapy. Mathematical Biosciences and Engineering, 2023, 20(2): 3301-3323. doi: 10.3934/mbe.2023155 |
Prostate cancer (PCa) is a major health concern in the US. It is the second most common cancer in men with the chance of life-time diagnosis being 1 in 7. PCa is more common in men over 50, and especially over 65. Recent developments in prostate cancer treatments have increased the rate of treatment and survival rate greatly, evidenced by a 5/10/15-year survival rate of 99%/98%/95%. However, for metastatic prostate tumors, the 5-year survival rate is 28%, which is among the lowest of all cancers [1,25]. The only known risk factors are age and heredity, while race and living situation are possible risk factors [1,27].
Most prostate cancers are found during screening with a prostate-specific antigen (PSA) blood test or a digital rectal exam (DRE). The fact that PSA level can reflect the size of the tumor and act as a predictor of relapse makes PSA the standard biomarker for PCa. Furthermore, PSA is frequently used as a proxy for the progression of a tumor, especially in mathematical modeling [14,20]. Androgens like testosterones and 5
For ADT, the reduction in androgen during treatment can cause undesirable side-effects such as impotence, depression, bone demineralization, and an increased risk of dementia. As a counter measure, intermittent androgen suppression (IAS) was introduced in the mid-1980s. Under IAS, the patient is put on ADT until his PSA level decreases to a predetermined lower threshold, which often corresponds to tumor regression and the PSA thresholds can be varied by the clinicians. Then, ADT is temporarily stopped until the PSA level rises to another predetermined upper threshold [1]. IAS has been shown to improve quality of life for patients; however, it remains controversial whether IAS is superior to continuous androgen suppression (CAS) in prolonging patient survival [4].
The lack of a solid understanding and a gold standard in treatment of prostate cancer drives the need for mathematical models. Especially in the last 15 years, many mathematical models have emerged to help explain the progression dynamics of prostate cancer in hope of answering some of the aforementioned questions [20].
Jackson [18] developed the first experimentally driven PCa model under CAS in 2004, which inspired later modeling efforts. In 2008, Ideta et al. [17] introduced the first mathematical model for PCa under IAS treatment with androgen dependent (AD) cell to androgen independent (AI) cell mutation. Guo et al. [13] formulated a partial differential equation version of the Ideta's model. Shimada and Aihara [24] studied the competition between different populations of PCa cells. A different approach to the formulation of a competition model based on Ideta's work was proposed by Yang et al. [28]. Eikenberry et al. [7] extended Ideta's model with intracellular signal of androgens to study its role in PCa. This was extended by Portz et al. [22] to a model that can fit clinical PSA data. And Portz's model was later simplified by Baez and Kuang [2] to also fit androgen data. Another extension of Ideta's model and the ideas of Eikenberry et al. [7] was presented by Jain et al. in [26], whose model captures the detailed biochemical dynamics of PCa. On the other hand, Hirata et al. [15] constructed a piecewise linear model based on the phenomenological behaviors of PSA to fit and describe the dynamics of PCa.
Additionally, numerous attempts have been done to study various clinical aspects such as an optimal schedule using an adaptive threshold and a stochastic hybrid automaton model [12], or a discrete hybrid cellular automaton model for a similar purpose for bone metastasized PCa [5]. Elishmereni et al. developed an algorithm that takes a mechanistic model in combination with the tumor Gleason score, a classification of the severity of prostate cancer, to predict when castration resistance will occur [9].
In this work, we rigorously exam and extend two previous approaches, which were developed by Baez and Kuang [2], Hirata and his colleagues [15], to further our understanding of the dynamics of prostate cancer and provide better tools for clinical applications.
The data we use to study the models comes from Vancouver Prostate Center [3], which contains the information of 109 patients. Similar to [2], we require the patient data to have at least 20 data points for both androgen and PSA in the first 1.5 cycles, the number of eligible patient data for comparison purpose is reduced to 62. Additionally, we need sufficient data for an additional treatment cycle for the forecasting comparison, so we select 26 of these 62 patient data.
Our model is built upon previous models which were based on a more complex but mechanistic framework. This is necessary because unlike phenomenological and statistical models, mechanistic models are created with sound hypotheses or well-known laws observed in nature [19].
Baez and Kuang [2] recently introduced an improved version of the model in [22] with the main emphasis of developing a simple model that can be readily used by physicians. This model is the first one to successfully take into account of clinical androgen data via fitting and forecasting. This is an important feature since the incorporation of androgen data fitting allows for better fitting and forecasting. In this model,
dx1dt=μm(1−q1Q)x1⏟growth−(D1(Q)+δ1x1)x1⏟death−λ(Q)x1⏟AD to AI | (2.1) |
dx2dt=μm(1−q2Q)x2⏟growth−(D2(Q)+δ2x2)x2⏟death+λ(Q)x1⏟AD to AI | (2.2) |
dQdt=γ(Qm−Q)⏟diffusion−μ(Q−q1)x1+μ(Q−q2)x2x1+x2⏟Uptake | (2.3) |
dPdt=bQ⏟baseline production+σ(Qx1+Qx2)⏟tumor production−ϵP⏟clearance | (2.4) |
Di(Q)=diRiQ+Ri, λ(Q)=cKQ+K | (2.5) |
γ=γ1u(t)+γ2, u(t)={0,on treatment1,off treatment. | (2.6) |
One important parameter constraint in the BK model is the inequality involving the cell quotas of AI and AD cells,
The validation of a model goes beyond how well it fits the observed data. This is especially true for mechanistic model, which can be used as direct test of well-founded scientific theories. Although the BK model has an impressive fitting and forecasting power [2], it may not have the appropriate underlying population assumption. In other word, the population structure in the BK model may not be flexible enough to allow for different cancer dynamics. This is especially crucial when the correct prostate cancer cell population structure and interaction are not yet fully known. Thus, this population structure simplicity may compromise the BK model's ability to appropriately represent the dynamics of the cancer growth and its forecasting potential.
As noted by Hirata et al. [15], a system with three distinguished populations is perhaps optimal in the case of metastasized prostate cancer because such a system --- under linear rates of change --- can reproduce the bi-phasic decline that is observed in the PSA data when the treatment starts.
The difference between the two population model in [2] and the three population model in [15] is an intermediate population between an androgen-dependent (AD) cell population and a strongly androgen-independent (strong AI) cell population. We call this new cell population the weakly androgen-independent (weak AI) cell population. Unlike the strong AI cell population, this weak AI population has the ability to revert back to the AD cell population. Due to permanent nature of the transformation, we consider the transition from
Param | Description | Range | Unit |
| max proliferation rate | 0.001 - 0.09 | day |
| min AD cell quota | 0.1-0.7 | nM |
| min w.AI cell quota | 0.1-0.5 | nM |
| min s.AI cell quota | 0.1-0.5 | nM |
| prostate baseline PSA | 0.1-2.5 | |
| tumor PSA production rate | 0.001-0.9 | |
| PSA clearance rate | 0.001-0.01 | day |
| max AD cell death rate | 0.001-0.09 | day |
| max w.AI cell death rate | 0.0001-0.001 | day |
| max s.AI cell death rate | 0.0001-0.001 | day |
| density death rate | 0.1-9 | 1/day/mm |
| density death rate | 0.01-4.5 | 1/day/mm |
| density death rate | 0.01-4.5 | 1/day/mm |
| AD death rate half-saturation | 0-3 | nM |
| w.AI death rate half-saturation | 0-3 | nM |
| s.AI death rate half-saturation | 0-3 | nM |
| maximum mutation rate | | day |
| mutation rate half-saturation | 0-1 | nM |
| testes androgen production | 20 | day |
| secondary androgen production | 0.001-0.01 | day |
| maximum androgen | 15-30 | nM |
x′1=μ1(Q)x1⏟growth−(D1(Q)+δ11x1)x1⏟death−(λ12(Q)+λ13(Q))x1⏟AD to AI+λ21(Q)x2⏟w.AI to AD | (2.7) |
x′2=μ2(Q)x2⏟growth−(D2(Q)+δ22x2)x2⏟death−(λ21(Q)+λ23(Q))x2⏟w.AI to AD and s.AI+λ12(Q)x1⏟AD to w.AI | (2.8) |
x′3=μ3(Q)x3⏟growth−(D3(Q)+δ33x3)x3⏟death+λ13(Q)x1+λ23(Q)x2⏟AD and w.AI to s.AI | (2.9) |
Q′=γ(Qm−Q)⏟diffusion−μ(Q−q1)x1+μ(Q−q2)x2+μ(Q−q3)x3x1+x2+x3⏟uptake | (2.10) |
P′=bQ⏟baseline production+σQ(x1+x2+x3)⏟tumor production−ϵP⏟clearance | (2.11) |
Di(Q)=diRiQ+Ri , λij(Q)=cijKQ+K, μi=μ(1−qiQ) | (2.12) |
γ=γ1u(t)+γ2 , u(t)={0 , on treatment1 , off treatment. | (2.13) |
where "w.AI" and "s.AI" stands for weak AI and strong AI cells. Due to insufficient data to support differentiating "mutation/adaption" rates in prostate cancer cell, we take the "mutation rates" to be the same, e.g.
We adapt the approach of the BK model formulation to derive our three population model. Recall that the three-population-model contains three different types of prostate cancer cells: the androgen dependent type (
μi(Q)=μ(1−qiQ), i∈{1,2,3}, |
where
Di(Q)=diRiQ+Ri, i∈{1,2,3}, |
where
λ(Q)=cKQ+K, |
where
We first derive the rate of change for the androgen cell quota
Qx=Q(t)x(t) nmol |
where
x=x1+x2+x3. |
Let
(Q(t)x(t))′=γ(Qm−Q(t))x(t)−(νRQ(t)+R+δx(t))Q(t)x(t). |
Differentiate and solve for
∑i∈{1,2,3}(ciRiQ(t)+Ri+δiixi(t))Q(t)xi(t). |
Finally, we assume the PSA level is produced at a baseline rate of
For parameter fitting, we use MATLAB (version 2017a) function fmincon, which utilizes interior point algorithm to minimize an objective error function within the range provided in Table 1. The objective error function is calculated as the sum of the mean squared error (MSE) fitting error for PSA and androgen since particularly large error is undesirable in clinical application.
ErrorMSE=1N∑Ni=1(yi−ˆyi)2, |
where N is the total number of data points,
ObjectiveMSE=ErrorMSE,PSA+ErrorMSE,androgen |
The range for the parameter is adapted from [2] under the assumption that the parameter constraints for the weak and strong AI cells are the same. The initial guesses of the parameters are chosen by hand within the established range to obtain the best fit.
In this section, we provide some analytical insights into the three population model. Additionally, we explore a condition on the population structure that ensures the consistency with what is observed in clinical studies.
Hatano et al. [14] points out one important flaw with the PKN model (the predecessor of the BK model). Under CAS treatment, PKN model fails to reproduce relapse both analytically and numerically within the given parameter constraints. Note that this does not mean conclusively that the model fails intrinsically. In fact, BK model allows for possible relapse under a similar analysis. If the choice of treatment is CAS, then the constant suppression of androgen production will result in a near minimum level of androgen in the body. Thus we can approximate
ddt(x1(t)x2(t))=(−D1(Q)−δ1x1−λ(Q)0λ(Q)T−D2(Q)−δ2x2)(x1(t)x2(t)), | (3.1) |
where
J(0,0)=(−D1(Q)−λ(Q)0λ(Q)T−D2(Q)). | (3.2) |
There are many ways to force instability at extinction. One such condition arises from having the determinant to be negative, e.g.
Hatano et al. [14] pointed out that the reasons behind PKN model not being able to reproduce relapse under CAS is either because it does not have an absorbing state (with respect to the direction of mutation, see Figure 1 for an example of absorbing state) or the parameter constraints are not optimal. However, the BK model is capable of reproducing the relapse within the provided parameter constraints, this suggests that the appropriate structure of the cell dynamics in the case of prostate tumor should have at least one absorbing state.
In this section, we provide some standard analysis for our model to justify its biological validity. First, we establish an invariant positive region under biologically reasonable assumptions. Additionally, we show that the population of a cell is also bounded above, which is coherent with reality. While the PSA level is an indicator of the growth of the tumor, it has negligible effect on the growth of the tumor cells and the production of androgen. This is reflected in the mathematical form, where the dynamics of
Proposition 1. Assume
Proof. If
dQdt=γ(Qm−Q)−1x3∑i=1μ(Q−qi)xi, |
it is clear that
dQdt=γ(Qm−q3)−1xμ[(q3−q1)x1+(q3−q2)x2]>0, |
when
Since
x′=3∑i=1μi(Q)xi−3∑i=1(Di(Q)+δiixi)xi≤(μ3(Q)−Dm(q3))x−δ33(x21+x22+x23)≤(μ3(Qm)−Dm(q3))x−δ33x2. |
This implies the limit supremum of
The number and locations of nonnegative steady states are natural but challenging mathematical questions that maybe of interest to readers. Here our focus is to exam the existence and local stability of an absorbing steady state where both the AD and reversible AI cells are extinct.
Proposition 2. Assume
Proof. Let
Thus
The next proposition presents a set of intuitive but unrealistic sufficient conditions for the extinction of some or all cancer populations.
Proposition 3. Define
Proof. Define
{S1(Q)=μ1(Q)−D1(Q)−λ12(Q)−λ13(Q)S2(Q)=μ2(Q)−D2(Q)−λ21(Q)−λ23(Q)S3(Q)=μ3(Q)−D3(Q). |
Then the rate of change of each population can be expressed as
{x′1(t)=(S1(Q)−δ11(Q)x1(t))x1(t)+λ21(Q)x2(t)x′2(t)=(S2(Q)−δ22(Q)x2(t))x2(t)+λ12(Q)x1(t)x′3(t)=(S3(Q)−δ33(Q)x3(t))x3(t)+λ13(Q)x1(t)+λ23(Q)x2(t). |
By our definition of
x′1(t)+x′2(t)=(T1(Q)−δ11x1(t))x1(t)+(T2(Q)−δ22x2(t))x2(t). |
Since
x′1(t)+x′2(t)≤min{T1(Qm),T2(Qm)}(x1(t)+x2(t)). |
This leads to the estimate
x1(t)+x2(t)≤(x1(0)+x2(0))e−|min{T1(Qm),T2(Qm)}|t. |
This implies the extinction of the AD and weak-AI populations.
Additionally if
For stability analysis, the following proposition extends Proposition 2 by establishing sufficient conditions of asymptotic stability of the equilibrium state with only the strong AI population.
Proposition 4. The steady state
(i)S1(Q∗)+S2(Q∗)<0(ii)S1(Q∗)S2(Q∗)>λ21(Q∗)λ12(Q∗). |
Furthermore, a set of sufficient conditions for the locally asymptotically stability of
S3(Q∗)>0,T1(Q∗)<0,T2(Q∗)<0. |
Proof. The Jacobian matrix evaluated at
J(E1)=(M11M12M21M22) |
where
M11=(S1(Q∗)λ21(Q∗)λ12(Q∗)S2(Q∗)) |
M21=(λ13(Q∗)λ23(Q∗)μδ33(q1−Q∗)μ3(Q∗)−D3(Q∗)μδ33(q2−Q∗)μ3(Q∗)−D3(Q∗)) |
M22=(−S3(Q∗)(μq3Q2∗+d3R3(Q∗+R3)2)μ3(Q∗)−D3(Q∗)δ330−(γ+μ)) |
and
In this section, we present key numerical results comparing the two and three population models against clinical data. Additionally, we provide a sensitivity analysis to further enlighten the important parameters of the three population model. The sensitivity analysis for the two population model can be found in [2].
We calculate the error of the fitting and forecasting parts for a quantitative comparison, where we used the predetermined on-treatment time given in the data to make the forecast. Table 2 shows comparable errors in the fitting of the first 1.5 cycles of both models. Additional criterion for comparison, such as the Akaike Information Criterion (AIC) that was used in similar contexts [14], could be used to strengthen the comparison. It is worth pointing out that information theoretic comparison like the AIC does not directly take into account of how well a model describes the dynamics of the natural phenomenon, for instance how well a model can follow the trend of the data. On the other hand, Table 3 shows the forecasting ability of both models are in comparable range. Instances where the models give large forecasts errors are likely due to the uncertainty associated with the number of parameters. Examples of the fitting and forecasting are presented in Figure 2. In addition, we also studied the case where
Model | PSA | Androgen | |||||
Median | | | Median | | | ||
3 Populations | 1.18 | 0.69 | 2.11 | 2.68 | 1.88 | 4.50 | |
2 Populations | 1.14 | 0.78 | 2.04 | 2.68 | 1.88 | 4.50 |
Model | PSA | Androgen | |||||
Median | | | Median | | | ||
3 Populations | 5.32 | 3.76 | 15.61 | 4.26 | 2.30 | 6.45 | |
2 Populations | 4.52 | 2.94 | 8.10 | 4.19 | 2.31 | 6.62 |
We present selected figures of androgen levels and cell populations corresponding to the forecasting in Figure 2.
Sensitivity serves an important part in the analysis of any mechanistic model. Sometimes, by performing sensitivity analysis, we can pinpoint parameters that do not serve important parts in the dynamics of the models. While for nonlinear models, global sensitivity analysis techniques are often preferred, local sensitivity analysis serves as a good first step to disclose the relationship of small variation in parameter values and the variable of interest. Since sensitivity analysis is often affected by the magnitude of the parameter or the variable, we will use the normalized sensitivity as presented in section 5.5 in [23], which takes the form:
Sp=∂x∂ppx. |
Here,
Figure 5 shows a dominating influence of the maximum androgen
There is a need for mathematical modeling effort for understanding and treating (metastasized) prostate cancer. The aim of this work is to compare two different cell population structures and explore key aspects that could help future prostate cancer modeling effort. We summarize key results and discuss our findings here:
(A1) The data fitting part for both models are similarly accurate, especially when there is sufficient and clear regularity in the data as can be seen in the fitting and forecasting of patient 28 data in Figure 2. On the other hand, the two population presents better forecasting ability, perhaps due to having a smaller number of parameters. Since the incorporation of an additional cell population does not significantly improve fitting and worsen forecasting, it suggests that to model clinical PSA dynamics in the absence of extensive data, the cancer cells can be assumed to fall into two types, androgen dependent and androgen independent.
(A2) A delay of PSA relapse from the onset of the off-treatment period can be seen for many patients. The sudden switch in the androgen available between the off and on treatment periods does not account for this, leading to situations where the forecast could have been stronger had such measures have been taken, for instance patients 77 and 100 in Figure 2. The delay, which can be up to several months if present, can be seen as a sign of drug residual in the patient's body interfering with the production of androgen, or perhaps a more complex mechanism that delays the availability of androgen in the tumor. While incorporating a delay term to account for the observed delay is straightforward, a more biologically motivated alternative would be to use the interpolation of androgen introduced in ref. [22]; however, the latter approach may require additional assumption on the shape of androgen data for forecasting.
(A3) It is possible to obtain highly accurate fitting and forecasting with a small number of PSA data points (around 10), example can be found in simulation of patient 100 in Figure 2. Aside from the obvious assistance of concurrent fitting of androgen data, having a balanced ratio of high and low data points and regularity in patient data may play the key role. Although using a small number of data points may lead to over fitting, most parameters are relatively not sensitive. Thus a thorough sensitivity analysis follow by fixing the non-sensitive parameters is a possible method to deal with the problem of problem of over fitting. On the other hand, the disproportional number of data points will possibly effect the fitting and forecasting (see the three population model fitting and forecasting for patient 29). This suggests that a resampling technique should be applied and recent approaches in this direction can be found in [16].
Preliminary dynamical and sensitivity analysis of the model are also provided, which shows that the model structure is biologically sound. While some conditions for local stability and existence of a coexistence of all cancer cells are established, a global study is still needed to explore this further. The simulations in Figure 3 and Figure 4 suggest the global stability for the on and off-treatment connected by a switch. According to the simulation results, periodic switching of treatment may allow the model to generate a stable limit cycle solution. Furthermore, although a local technique has been used to explore the relationship between variables and parameters, global techniques is highly desirable for the sensitivity analysis due to the nonlinearity in the model [23]. In addition to data, it will be helpful to perform parameter identification analysis to exam the credibility of the parameter values identified via model data fitting prcess [8].
From the perspective of physicians and patients, the following questions could be useful for planning the course of treatment to optimize the effect. What are the ideal thresholds for PSA level in IAS? Under optimal treatment schedule and dosage, can IAS prolong a patient's life in comparison to CAS? What is a good metric to compare different treatments? When will the tumor become resistant to hormonal therapy? What triggers the development of hormonal refractory and how to prevent it? Each question by itself poses difficult practical and theoretical problems, for example the information on the types of cancer cells and how they interact is difficult to obtain. But perhaps, the most serious problem is the lack of a good practical method to track the development of a metastasized prostate tumor. In practice, PSA is primarily used as a direct correspondence to the total tumor population. This has led to the almost exclusive use of PSA-data-fitting as the only justification for mathematical models [20].
This work is partially supported by NSF grants DMS-1148771, 1518529 and 1615879. We are profoundly grateful to Dr. Nicholas Bruchovsky for the clinical data set and his encouragement. In addition, the authors would like to thank the reviewers for many helpful comments that improved the presentation of this manuscript.
All authors declare no conflicts of interest in this paper.
[1] | Prostate cancer, Am. Cancer Soc., 4–18, 28–29, 56–57.0 |
[2] | J. Baez and Y. Kuang, Mathematical models of androgen resistance in prostate cancer patients under intermittent androgen suppression therapy, Appl. Sci., 6 (2016), 352. |
[3] | N. Bruchovsky, L. Klotz, J. Crook, S. Malone, C. Ludgate, W. J. Morris, M. E. Gleave and S. L. Goldenberg, Final results of the canadian prospective phase ii trial of intermittent androgen suppression for men in biochemical recurrence after radiotherapy for locally advanced prostate cancer, Cancer, 107 (2006), 389–395. |
[4] | N. Buchan and S. Goldenberg, Intermittent versus continuous androgen suppression therapy: do we have consensus yet?, Cur. Oncol., 17 (2010), S45. |
[5] | L. M. Cook, A. Araujo, J. M. Pow-Sang, M. M. Budzevich, D. Basanta and C. C. Lynch, Predictive computational modeling to define effective treatment strategies for bone metastatic prostate cancer, Sci. Rep., 6 (2016), 29384. |
[6] | M. Droop, Some thoughts on nutrient limitation in algae, J. Phycol., 9 (1973), 264–272. |
[7] | S. E. Eikenberry, J. D. Nagy and Y. Kuang, The evolutionary impact of androgen levels on prostate cancer in a multi-scale mathematical model, Biol. Direct, 5 (2010), 24. |
[8] | M. C. Eisenberg and H. V. Jain, A confidence building exercise in data and identifiability: Modeling cancer chemotherapy as a case study, J. Theor. Biol., 431 (2017), 63–78. |
[9] | M. Elishmereni, Y. Kheifetz, I. Shukrun, G. H. Bevan, D. Nandy, K. M. McKenzie, M. Kohli and Z. Agur, Predicting time to castration resistance in hormone sensitive prostate cancer by a personalization algorithm based on a mechanistic model integrating patient data, The Prostate, 76 (2016), 48–57. |
[10] | R. Everett, A. Packer and Y. Kuang, Can mathematical models predict the outcomes of prostate cancer patients undergoing intermittent androgen deprivation therapy?, Biophys. Rev. Letters, 9 (2014), 173–191. |
[11] | B. J. Feldman and D. Feldman, The development of androgen-independent prostate cancer, Nat. Rev. Cancer, 1 (2001), 34–45. |
[12] | J. L. Fleck and C. G. Cassandras, Optimal design of personalized prostate cancer therapy using infinitesimal perturbation analysis, Nonlin. Analysis Hybrid System., 25 (2017), 246–262. |
[13] | Q. Guo, Y. Tao and K. Aihara, Mathematical modeling of prostate tumor growth under intermittent androgen suppression with partial differential equations, lnt. J. Bifurcat. Chaos, 18 (2008), 3789– 3797. |
[14] | T. Hatano, Y. Hirata, H. Suzuki and K. Aihara, Comparison between mathematical models of intermittent androgen suppression for prostate cancer, J. Theor. Biol., 366 (2015), 33–45. |
[15] | Y. Hirata, N. Bruchovsky and K. Aihara, Development of a mathematical model that predicts the outcome of hormone therapy for prostate cancer, J. Theor. Biol., 264 (2010), 517–527. |
[16] | Y. Hirata, K. Morino, K. Akakura, C. S. Higano and K. Aihara, Personalizing androgen suppression for prostate cancer using mathematical modeling, Sci. Rep., 8 (2018), 2673. |
[17] | A. M. Ideta, G. Tanaka, T. Takeuchi and K. Aihara, A mathematical model of intermittent androgen suppression for prostate cancer, J. Nonlin. Sci., 18 (2008), 593–614. |
[18] | T. L. Jackson, A mathematical model of prostate tumor growth and androgen-independent relapse, Discrete Cont. Dyn. B, 4 (2004), 187–202. |
[19] | Y. Kuang, Basic properties of mathematical population models, J. Biomath., 17 (2002), 129–142. |
[20] | Y. Kuang, J. D. Nagy and S. E. Eikenberry, Introduction to mathematical oncology, vol. 59, CRC Press, 2016. |
[21] | J. D. Morken, A. Packer, R. A. Everett, J. D. Nagy and Y. Kuang, Mechanisms of resistance to intermittent androgen deprivation in patients with prostate cancer identified by a novel computational method, Cancer Res., 74 (2014), 3673–3683. |
[22] | T. Portz, Y. Kuang and J. D. Nagy, A clinical data validated mathematical model of prostate cancer growth under intermittent androgen suppression therapy, Aip. Advances, 2 (2012), 011002. |
[23] | A. Saltelli, K. Chan and E. M. Scott, Sensitivity analysis, Wiley New York, 2000. |
[24] | T. Shimada and K. Aihara, A nonlinear model with competition between prostate tumor cells and its application to intermittent androgen suppression therapy of prostate cancer, Math. Biosci., 214 (2008), 134–139. |
[25] | R. L. Siegel, K. D. Miller and A. Jemal, Cancer statistics, 2016, CA Cancer J. Clinic., 66 (2016), 7–30. |
[26] | H. Vardhan Jain and A. Friedman, Modeling prostate cancer response to continuous versus intermittent androgen ablation therapy, Discrete Cont. Dyn. B, 18. |
[27] | A. Wolf, R. C. Wender, R. B. Etzioni, I. M. Thompson, A. V. D'Amico, R. J. Volk, D. D. Brooks, C. Dash, I. Guessous, K. Andrews et al., American cancer society guideline for the early detection of prostate cancer: update 2010, CA Cancer J. Clinic., 60 (2010), 70–98. |
[28] | J. Yang, T.J. Zhao, C.Q. Yuan, J.H. Xie and F.F. Hao, A nonlinear competitive model of the prostate tumor growth under intermittent androgen suppression, J. Theor. Biol., 404 (2016), 66– 72. |
1. | Tin Phan, Sharon M. Crook, Alan H. Bryce, Carlo C. Maley, Eric J. Kostelich, Yang Kuang, Review: Mathematical Modeling of Prostate Cancer and Clinical Application, 2020, 10, 2076-3417, 2721, 10.3390/app10082721 | |
2. | Tin Phan, Kyle Nguyen, Preeti Sharma, Yang Kuang, The Impact of Intermittent Androgen Suppression Therapy in Prostate Cancer Modeling, 2018, 9, 2076-3417, 36, 10.3390/app9010036 | |
3. | Paul A. Valle, Luis N. Coria, Karla D. Carballo, Chemoimmunotherapy for the treatment of prostate cancer: Insights from mathematical modelling, 2021, 90, 0307904X, 682, 10.1016/j.apm.2020.09.021 | |
4. | William Meade, Allison Weber, Tin Phan, Emily Hampston, Laura Figueroa Resa, John Nagy, Yang Kuang, High Accuracy Indicators of Androgen Suppression Therapy Failure for Prostate Cancer—A Modeling Study, 2022, 14, 2072-6694, 4033, 10.3390/cancers14164033 | |
5. | Guillermo Lorenzo, Nadia di Muzio, Chiara Lucrezia Deantoni, Cesare Cozzarini, Andrei Fodor, Alberto Briganti, Francesco Montorsi, Víctor M. Pérez-García, Hector Gomez, Alessandro Reali, Patient-specific forecasting of postradiotherapy prostate-specific antigen kinetics enables early prediction of biochemical relapse, 2022, 25, 25890042, 105430, 10.1016/j.isci.2022.105430 | |
6. | S. Pasetto, H. Enderling, R. A. Gatenby, R. Brady-Nicholls, Intermittent Hormone Therapy Models Analysis and Bayesian Model Comparison for Prostate Cancer, 2022, 84, 0092-8240, 10.1007/s11538-021-00953-w | |
7. | Tin Phan, Justin Bennett, Taylor Patten, Practical Understanding of Cancer Model Identifiability in Clinical Applications, 2023, 13, 2075-1729, 410, 10.3390/life13020410 | |
8. | Miaoran Yao, Yongxin Zhang, Wendi Wang, Selection of prostate cancer therapy strategy under early androgen suppression treatment, 2024, 132, 10075704, 107914, 10.1016/j.cnsns.2024.107914 |
Param | Description | Range | Unit |
| max proliferation rate | 0.001 - 0.09 | day |
| min AD cell quota | 0.1-0.7 | nM |
| min w.AI cell quota | 0.1-0.5 | nM |
| min s.AI cell quota | 0.1-0.5 | nM |
| prostate baseline PSA | 0.1-2.5 | |
| tumor PSA production rate | 0.001-0.9 | |
| PSA clearance rate | 0.001-0.01 | day |
| max AD cell death rate | 0.001-0.09 | day |
| max w.AI cell death rate | 0.0001-0.001 | day |
| max s.AI cell death rate | 0.0001-0.001 | day |
| density death rate | 0.1-9 | 1/day/mm |
| density death rate | 0.01-4.5 | 1/day/mm |
| density death rate | 0.01-4.5 | 1/day/mm |
| AD death rate half-saturation | 0-3 | nM |
| w.AI death rate half-saturation | 0-3 | nM |
| s.AI death rate half-saturation | 0-3 | nM |
| maximum mutation rate | | day |
| mutation rate half-saturation | 0-1 | nM |
| testes androgen production | 20 | day |
| secondary androgen production | 0.001-0.01 | day |
| maximum androgen | 15-30 | nM |
Model | PSA | Androgen | |||||
Median | | | Median | | | ||
3 Populations | 1.18 | 0.69 | 2.11 | 2.68 | 1.88 | 4.50 | |
2 Populations | 1.14 | 0.78 | 2.04 | 2.68 | 1.88 | 4.50 |
Model | PSA | Androgen | |||||
Median | | | Median | | | ||
3 Populations | 5.32 | 3.76 | 15.61 | 4.26 | 2.30 | 6.45 | |
2 Populations | 4.52 | 2.94 | 8.10 | 4.19 | 2.31 | 6.62 |
Param | Description | Range | Unit |
| max proliferation rate | 0.001 - 0.09 | day |
| min AD cell quota | 0.1-0.7 | nM |
| min w.AI cell quota | 0.1-0.5 | nM |
| min s.AI cell quota | 0.1-0.5 | nM |
| prostate baseline PSA | 0.1-2.5 | |
| tumor PSA production rate | 0.001-0.9 | |
| PSA clearance rate | 0.001-0.01 | day |
| max AD cell death rate | 0.001-0.09 | day |
| max w.AI cell death rate | 0.0001-0.001 | day |
| max s.AI cell death rate | 0.0001-0.001 | day |
| density death rate | 0.1-9 | 1/day/mm |
| density death rate | 0.01-4.5 | 1/day/mm |
| density death rate | 0.01-4.5 | 1/day/mm |
| AD death rate half-saturation | 0-3 | nM |
| w.AI death rate half-saturation | 0-3 | nM |
| s.AI death rate half-saturation | 0-3 | nM |
| maximum mutation rate | | day |
| mutation rate half-saturation | 0-1 | nM |
| testes androgen production | 20 | day |
| secondary androgen production | 0.001-0.01 | day |
| maximum androgen | 15-30 | nM |
Model | PSA | Androgen | |||||
Median | | | Median | | | ||
3 Populations | 1.18 | 0.69 | 2.11 | 2.68 | 1.88 | 4.50 | |
2 Populations | 1.14 | 0.78 | 2.04 | 2.68 | 1.88 | 4.50 |
Model | PSA | Androgen | |||||
Median | | | Median | | | ||
3 Populations | 5.32 | 3.76 | 15.61 | 4.26 | 2.30 | 6.45 | |
2 Populations | 4.52 | 2.94 | 8.10 | 4.19 | 2.31 | 6.62 |