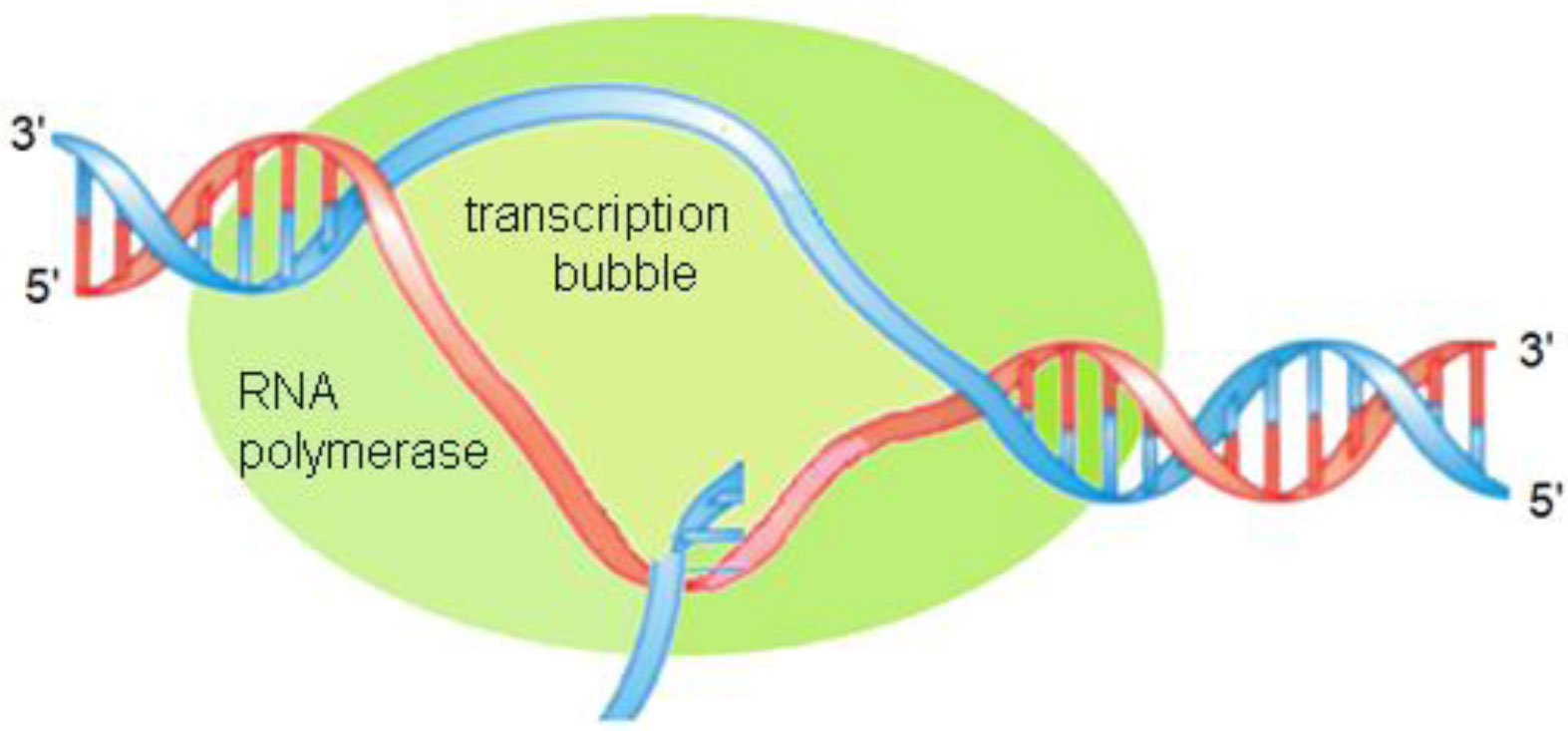
Getting to Zero (GTZ) initiatives focus on expanding use of antiretroviral treatment (ART) and pre-exposure prophylaxis (PrEP) to eliminate new HIV infections. Computational models help inform policies for implementation of ART and PrEP continuums. Such models, however, vary in their design, and may yield inconsistent predictions. Using multiple approaches can help assess the consistency in results obtained from varied modeling frameworks, and can inform optimal implementation strategies.
A study using three different modeling approaches is conducted. Two approaches use statistical time series analysis techniques that incorporate temporal HIV incidence data. A third approach uses stochastic stimulation, conducted using an agent-based network model (ABNM). All three approaches are used to project HIV incidence among a key population, young Black MSM (YBMSM), over the course of the GTZ implementation period (2016–2030).
All three approaches suggest that simultaneously increasing PrEP and ART uptake is likely to be more effective than increasing only one, but increasing ART and PrEP by 20% points may not eliminate new HIV infections among YBMSM. The results further suggest that a 20% increase in ART is likely to be more effective than a 20% increase in PrEP. All three methods consistently project that increasing ART and PrEP by 30% simultaneously can help reach GTZ goals.
Increasing PrEP and ART uptake by about 30% might be necessary to accomplish GTZ goals. Such scale-up may require addressing psychosocial and structural barriers to engagement in HIV and PrEP care continuums. ABNMs and other flexible modeling approaches can be extended to examine specific interventions that address these barriers and may provide important data to guide the successful intervention implementation.
Citation: Aditya Subhash Khanna, Mert Edali, Jonathan Ozik, Nicholson Collier, Anna Hotton, Abigail Skwara, Babak Mahdavi Ardestani, Russell Brewer, Kayo Fujimoto, Nina Harawa, John A. Schneider. Projecting the number of new HIV infections to formulate the 'Getting to Zero' strategy in Illinois, USA[J]. Mathematical Biosciences and Engineering, 2021, 18(4): 3922-3938. doi: 10.3934/mbe.2021196
[1] | Larisa A. Krasnobaeva, Ludmila V. Yakushevich . DNA kinks behavior in the potential pit-trap. AIMS Biophysics, 2022, 9(2): 130-146. doi: 10.3934/biophy.2022012 |
[2] | Larisa A. Krasnobaeva, Ludmila V. Yakushevich . On the dimensionless model of the transcription bubble dynamics. AIMS Biophysics, 2023, 10(2): 205-219. doi: 10.3934/biophy.2023013 |
[3] | Audrey Lejart, Gilles Salbert, Sébastien Huet . Cytosine hydroxymethylation by TET enzymes: From the control of gene expression to the regulation of DNA repair mechanisms, and back. AIMS Biophysics, 2018, 5(3): 182-193. doi: 10.3934/biophy.2018.3.182 |
[4] | Roman Marks . Bubble mediated polymerization of RNA and DNA. AIMS Biophysics, 2022, 9(2): 96-107. doi: 10.3934/biophy.2022009 |
[5] | Michael J. Hamilton, Matthew D. Young, Silvia Sauer, Ernest Martinez . The interplay of long non-coding RNAs and MYC in cancer. AIMS Biophysics, 2015, 2(4): 794-809. doi: 10.3934/biophy.2015.4.794 |
[6] | Yuri M. Moshkin . Chromatin—a global buffer for eukaryotic gene control. AIMS Biophysics, 2015, 2(4): 531-554. doi: 10.3934/biophy.2015.4.531 |
[7] | Arsha Moorthy, Alireza Sarvestani, Whitney Massock, Chamaree de Silva . Twisting and extension: Application of magnetic tweezers to DNA studies. AIMS Biophysics, 2023, 10(3): 317-346. doi: 10.3934/biophy.2023020 |
[8] | Davood Norouzi, Ataur Katebi, Feng Cui, Victor B. Zhurkin . Topological diversity of chromatin fibers: Interplay between nucleosome repeat length, DNA linking number and the level of transcription. AIMS Biophysics, 2015, 2(4): 613-629. doi: 10.3934/biophy.2015.4.613 |
[9] | Théo Lebeaupin, Hafida Sellou, Gyula Timinszky, Sébastien Huet . Chromatin dynamics at DNA breaks: what, how and why?. AIMS Biophysics, 2015, 2(4): 458-475. doi: 10.3934/biophy.2015.4.458 |
[10] | Sarada Ghosh, Guruprasad Samanta, Manuel De la Sen . Feature selection and classification approaches in gene expression of breast cancer. AIMS Biophysics, 2021, 8(4): 372-384. doi: 10.3934/biophy.2021029 |
Getting to Zero (GTZ) initiatives focus on expanding use of antiretroviral treatment (ART) and pre-exposure prophylaxis (PrEP) to eliminate new HIV infections. Computational models help inform policies for implementation of ART and PrEP continuums. Such models, however, vary in their design, and may yield inconsistent predictions. Using multiple approaches can help assess the consistency in results obtained from varied modeling frameworks, and can inform optimal implementation strategies.
A study using three different modeling approaches is conducted. Two approaches use statistical time series analysis techniques that incorporate temporal HIV incidence data. A third approach uses stochastic stimulation, conducted using an agent-based network model (ABNM). All three approaches are used to project HIV incidence among a key population, young Black MSM (YBMSM), over the course of the GTZ implementation period (2016–2030).
All three approaches suggest that simultaneously increasing PrEP and ART uptake is likely to be more effective than increasing only one, but increasing ART and PrEP by 20% points may not eliminate new HIV infections among YBMSM. The results further suggest that a 20% increase in ART is likely to be more effective than a 20% increase in PrEP. All three methods consistently project that increasing ART and PrEP by 30% simultaneously can help reach GTZ goals.
Increasing PrEP and ART uptake by about 30% might be necessary to accomplish GTZ goals. Such scale-up may require addressing psychosocial and structural barriers to engagement in HIV and PrEP care continuums. ABNMs and other flexible modeling approaches can be extended to examine specific interventions that address these barriers and may provide important data to guide the successful intervention implementation.
The construction of energy profiles and their analysis is a new method for studying the physical and functional properties of plasmids. This method came to DNA science from theoretical physics and nonlinear mathematics. In the frameworks of the method, the DNA molecule is considered as a complex dynamic system in which nonlinear conformational perturbations-kinks, can arise and propagate [1]–[4].
Since the work [5], kinks have been actively used to simulate the DNA open states which are small (only a few base pairs long) unwound DNA regions inside which hydrogen bonds between complementary bases are broken. The behavior of the kinks is in many ways similar to that of ordinary particles moving in the DNA potential field. Having calculated the energy profile of the potential field, one can completely solve the problem of the kink dynamics including calculation of time dependence of the kink velocity and construction of the kink trajectory.
A particular example of the open state is the transcription bubble (Figure 1) which is formed at the initial stage of the transcription process as a result of the interaction of RNA polymerase with DNA promoter region [6],[7]. One of the first attempts to calculate the DNA potential field in which the transcriptional bubble moves was made by Salerno [8]. However, the absence at that time of a complete set of dynamic parameters of DNA, as well as the computer power required for such calculations, forced the author to confine himself to only a small fragment of a DNA molecule, which did not allow to obtain reliable results on the kink dynamics and to relate them with functional properties of the molecule.
In this work, we solve this problem on the basis of new data and new approaches to the study of the dynamics of open states in inhomogeneous DNA. In the calculations we use the full set of dynamic parameters of DNA, which was first obtained in [9] and later refined in [10]. The block method and the method of constructing the kinks trajectories are also applied [11],[12]. In contrast to work [12], where only one plasmid profile was calculated, here we use the method of the double profile, which makes it possible to compare the dynamic characteristics of kinks activated in the main and complementary DNA strands. Such a comparison makes it possible to explain in which region of the plasmid the formation of a transcription bubble is most likely, as well to understand in which direction the bubble will move and the transcription process will go.
Plasmid pBR322 [13] is a small circular DNA the sequence of which consists of 4361 bases [14] (Appendix). This sequence contains three coding regions:
CDS-1, encoding tetracycline resistance protein,
CDS-2, encoding ROP protein,
CDS-3, encoding beta-lactamase,
and three promoters: P1, P2 and P3 marked in Appendix by grey. Coordinates of the CDS regions are (86..1276), (1915..2106) and (3293..4153). Coordinates of promoters are (27-33), (43-49) and (4188-4194). The double-stranded scheme of the plasmid is shown in Figure 2a. A linear version of the scheme obtained by cutting at the point Q, is presented in Figure 2b.
Region number | Region coordinates | NA | NT | NG | NC | N |
1 | 1..85 | 22/29 | 29/22 | 17/17 | 17/17 | 85 |
2 (CDS-1) | 86..1276 | 190/268 | 268/190 | 353/381 | 381/353 | 1192 |
3 | 1277..1914 | 142/145 | 145/142 | 160/191 | 191/160 | 638 |
4 (CDS-2) | 1915..2106 | 54/37 | 37/54 | 48/53 | 53/48 | 192 |
5 | 2107..3292 | 294/268 | 268/294 | 319/305 | 305/319 | 1186 |
6 (CDS-3) | 3293..4153 | 213/223 | 223/213 | 203/222 | 222/203 | 861 |
7 | 4154-4361 | 67/64 | 64/67 | 34/42 | 42/34 | 207 |
It is convenient to renumber all regions including CDS regions and the regions between them, starting from the point S. A total of 7 regions turned out. Data on their composition are presented in Table 1.
However, the splitting of the sequence into 7 regions does not reflect the circular nature of the plasmid. To take this into account, we combined the 1st and 7th regions into a single region with number (7 + 1). As a result, the total number of regions decreased by one and became equal to six. In what follows, these six regions will be referred to as blocks.
To describe angular oscillations of nitrous bases in a separate block of the DNA molecule consisting of N bp, let us use the improved Englander model [5], which is a system of 2N coupled nonlinear differential equations [15]:
Here
where
After the averaging procedure (3), equations (1)–(2) are transformed to the form:
In the continuum approximation, these equations take the following form:
In the approximation of average field [17], two coupled equations (6) and (7) are transformed into two independent equations:
where a is the distance between the nearest base pairs. In this approximation, the movement of kinks in the main and complementary chains can be considered separately. The validity of this approximation for DNA was shown in [18], where it was demonstrated numerically that taking into account the interaction between kinks activated in the main and complementary chains leads only to a slight perturbation of the kink shape.
One-soliton solutions of equations (8)–(9), kinks, have then the form :
Here
Equation for the kink velocity was obtained by McLaughlin and Scott [19]. In the case of plasmid pBR322, this equation has the form:
The analytical solution of equation (1) found in [20], has the form :
where
The solution for the kink coordinate
where z0 is the coordinate of the kink at the initial moment of time t0.
The total kink energy is determined by the following formula [21]:
where
In the case of small velocities (
where (
To construct the energy profile, we first calculated the averaged values of the main dynamic parameters of the plasmid:
and also calculated the values of the rest energies of the kinks activated in each of these regions:
Here
Number of the region | Coordinate of the region | ||||
1+7 | (1..85)+(4154..4361) | 6.13/6.28 | 1.94/1.97 | 2.06/2.10 | 1.60/1.63 |
2 (CDS-1) | 86..1276 | 6.05/6.33 | 1.90/1.97 | 2.26/2.32 | 1.66/1.71 |
3 | 1277..1914 | 6.09/6.30 | 1.92/1.96 | 2.21/2.26 | 1.65/1.68 |
4 (CDS-2) | 1915..2106 | 6.26/6.13 | 1.97/1.92 | 2.23/2.19 | 1.67/1.64 |
5 | 2107..3292 | 6.25/6.14 | 1.95/1.93 | 2.22/2.20 | 1.67/1.65 |
6 (CDS-3) | 3293..4153 | 6.14/6.25 | 1.93/1.95 | 2.17/2.20 | 1.64/1.66 |
With the help of the data from the last column of Table 2 we constructed the double energy profile of the potential field of the plasmid (Figure 3). The energy was calculated not at every point, but for each range into which we divided the plasmid. The coordinates of the bases are plotted in Figure 3 along the horizontal in units of base pairs (bp), 1 bp = 3.4×10–10 m. The values of the rest energy of kinks are plotted along the vertical.
Figure 3 shows that the deepest well in the energy profile of both the main (solid line) and complementary (dashed line) sequences corresponds to the (7 + 1)-th region. This means that the probability of kink activation in this region is the highest. This result is in good agreement with the experimental data summarized in [14]. Indeed. the transcription process usually begins with the binding of RNA polymerase to the promoter region of the DNA sequence, and this event is accompanied by local unwinding double-stranded DNA. According to [14] there are three promoters in the (7 + 1)-th region: the P2 promoter located in the main chain, and the P1 and P3 promoters located in the complementary chain. That saturation of the (7 + 1)-th region with promoters indicates that just in this region with high probability the formation of local unwound regions - kinks, takes place.
It can be seen also that in the (7 + 1)-th region, the rest energy of the kinks activated in the main strand is less than the rest energy of the kinks activated in the complementary strand. This means that the probability of the kink activation in the main strand containing P2 promoter is greater than in the complementary strand containing P1 and P3 promoters. This allows us to suggest that the P1 and P3 promoters are much weaker than the P2 promoter.
Using formulas (2)-(4) and the data of Table 2, we plotted the time dependence of the velocity, coordinates and total energy of the plasmid pBR322 kinks (Figures 4–6).
Figure 4 shows that the velocity of the kinks activated in both strands decreases with time, which is explained by the effects of dissipation. The velocity of the kink activated in the complementary strand, decreases faster. The zigzags observed on both curves are explained by abrupt changes in the velocity of the kinks when passing the boundaries between the regions.
Figure 5 shows that the coordinate of the kinks grows smoothly and reaches a constant value, which means that the kink movement stops. Kink activated in the main strand stops at the point 2332 bp, and kink activated in the complementary strand stops at 2558 bp.
From Figure 6 it can be seen that at the same value of the initial kink velocity (1881 m/s), the values of the initial total energy of the kinks activated in different strands differ. For kinks activated in the main strand, the initial total energy is 10.42×10−18 (J), and for kinks activated in the complementary strand, the initial total energy is 12.25×10−18 (J). Later both energy curves decreases and reaches the values of the rest energies.
To construct trajectories of kinks in the 3D space, we solved numerically a system of coupled differential equations for the velocity and coordinates of the kinks:
The results are presented in the form of trajectories of the kink motion in the space {v, z, t} (Figure 7).
In the present work, we investigated the dynamics of the nonlinear conformation distortions – kinks, activated in the plasmid pBR322. The kinks were considered as quasi particles moving in the potential field of the plasmid. The key moment of the method used is the construction of the double energetic profile the analysis of which gives valuable information about the kink behavior.
To imitate the internal dynamics of the plasmid we used mathematical model consisting of two coupled sine-Gordon equations that in the average field approximation were transformed to two sine-Gordon independent equations with renormalized coefficients. The first equation described angular oscillations of nitrous bases of the main chain in the complementary chain. The second equation described angular oscillations of nitrous bases in the complementary chain. As a result, we obtained two types of kink-like solutions: one type are kinks which were the solutions of the first equation, and the other are kinks which were the solutions of the second equation.
To take into account the circular nature of DNA, we combined the end regions into a single block. Using calculated values of the rest energies of the kinks we constructed two energy profiles: the first for the main sequence, the second for the complementary one.
Analysis of these profiles showed that the deepest well in the energy profiles corresponds to the (7 + 1)-th region. This means that the probability of kink activation in this region is the highest.
Because the kink rest energy in the (7 + 1)-th region of the main sequence is less than in that of the complementary sequence we concluded that the P2 promoter located in the (7 + 1)-th region of the main sequence is stronger than the P1 and P3 promoters located in the (7 + 1)-th region of the complementary sequence. Therefore, it can be assumed that the transcription bubble formed in the (7 + 1)-th region is most likely to start moving in the direction of the CDS-1 region.
We calculated the main characteristics of the kink motion, including the time dependences of the kink velocity, coordinates, and total energy. These calculations were performed at the initial velocity equal to 1881 m/s which was chosen to avoid reflections from energy barriers corresponding to CDS-1 and CDS-2. Despite the fact that here we have considered only one value of the initial kink velocity the proposed approach is applicable to any values of the initial kink velocity. It is necessary only to keep in mind one important condition: the initial values should be less than the sound velocity. This condition arises from the mathematical requirements for the existence and stability of the solution in the form of kink.
It should be noted, also, that these results were obtained under three additional constraints. First, we used a simplified model that took into account angular oscillations of the nitrous bases in only one of the two DNA strands. Second, only one type of internal DNA motions-angular oscillations of the nitrous bases, was taken into accountю. Third, the model used did not take into account the DNA torque. In spite of all these limitations, we can expect that the results of the investigations obtained by simple and convenient method correctly convey the basic laws of the kink dynamic behavior in plasmid pBR322 and can be applied to another DNA sequences.
[1] | Joint United Nations Programme on HIV/AIDS (UNAIDS), Getting to Zero: 2011-2015 Strategy. 2010. Available from: http://files.unaids.org/en/media/unaids/contentassets/documents/unaidspublication/2010/20101221_JC2034E_UNAIDS-Strategy_en.pdf. |
[2] | The White House, National HIV/AIDS strategy for the United States, Washington, DC, 2010. |
[3] | National Alliance of State and Territorial AIDS Directors (NASTAD), Ending the HIV epidemic: jusrisdictional plans, Washington, D.C., 2018. Available from: https://www.nastad.org/maps/ending-hiv-epidemic-jurisdictional-plans. |
[4] |
A. S. Fauci, H. D. Marston, Focusing to Achieve a World Without AIDS, JAMA, 313 (2015), 357-358. doi: 10.1001/jama.2014.17454
![]() |
[5] | J. R. Hargreaves, S. Delany-Moretlwe, T. B. Hallett, S. Johnson, S. Kapiga, P. Bhattacharjee, et al., The HIV prevention cascade: integrating theories of epidemiological, behavioural, and social science into programme design and monitoring, Lancet HIV, 7 (2016), e318-e322. |
[6] |
A. Jones, I. Cremin, F. Abdullah, J. Idoko, P. Cherutich, N. Kilonzo, et al., Transformation of HIV from pandemic to low-endemic levels: a public health approach to combination prevention, Lancet, 384 (2014), 272-279. doi: 10.1016/S0140-6736(13)62230-8
![]() |
[7] |
B. C. Zanoni, K. H. Mayer, The adolescent and young adult HIV cascade of care in the United States: exaggerated health disparities, AIDS Patient Care STDS, 28 (2014), 128-135. doi: 10.1089/apc.2013.0345
![]() |
[8] | K. Risher, K. H. Mayer, C. Beyrer, HIV treatment cascade in MSM, people who inject drugs, and sex workers, Curr. Opin. HIV AIDS, 10 (2015), 420-429. |
[9] | Centers for Disease Control and Prevention, HIV and Gay and Bisexual Men. Available from: https://www.cdc.gov/hiv/group/msm/index.html. |
[10] | S. Cassels, S. J. Clark, M. Morris, Mathematical models for HIV transmission dynamics: tools for social and behavioral science research, J. Acquired Immune Defic. Syndr., 47 (2008), S34. |
[11] |
E. T. Lofgren, M. E. Halloran, C. M. Rivers, J. M. Drake, T. C. Porco, B. Lewis, et al., Opinion: Mathematical models: A key tool for outbreak response, Proc. Natl. Acad. Sci., 111 (2014), 18095-18096. doi: 10.1073/pnas.1421551111
![]() |
[12] | S. M. Jenness, S. M. Goodreau, E. Rosenberg, E. N. Beylerian, K. W. Hoover, D. K. Smith, et al., Impact of the Centers for Disease Control's HIV Preexposure Prophylaxis Guidelines for Men Who Have Sex With Men in the United States, J. Infect. Dis., 214 (2016), 1800-1807. |
[13] | S. M. Jenness, A. Sharma, S. M. Goodreau, E. S. Rosenberg, K. M. Weiss, K. W. Hoover, et al., Individual HIV risk versus population impact of risk compensation after HIV preexposure prophylaxis initiation among men who have sex with men, Plos One, 12 (2017), e0169484. |
[14] |
W. C. Goedel, M. R. F. King, M. N. Lurie, A. S. Nunn, P. A. Chan, B. D. L. Marshall, Effect of Racial Inequities in Pre-Exposure Prophylaxis Use on Racial Disparities in HIV Incidence Among Men Who Have Sex with Men, J. Acquired Immune Defic. Syndr., 79 (2018), 323-329. doi: 10.1097/QAI.0000000000001817
![]() |
[15] | R. Brookmeyer, D. Boren, S. D. Baral, L. G. Bekker, N. Phaswana-Mafuya, C. Beyrer, et al., Combination HIV prevention among MSM in South Africa: results from agent-based modeling, Plos One, 9 (2014), e112668. |
[16] | C. Celum, T. B. Hallett, J. M. Baeten, HIV-1 prevention with ART and PrEP: mathematical modeling insights into resistance, effectiveness, and public health impact, J. Infect. Dis., 208 (2013), 189-191. |
[17] | A. A. King, M. Domenech de Cellès, F. M. G. Magpantay, P. Rohani, Avoidable errors in the modelling of outbreaks of emerging pathogens, with special reference to Ebola, Proc. R. Soc. B, 282 (2015), 20150347. |
[18] |
A. S. Khanna, D. T. Dimitrov, S. M. Goodreau, What can mathematical models tell us about the relationship between circular migrations and HIV transmission dynamics?, Math. Biosci. Eng., 11 (2014), 1065-1090. doi: 10.3934/mbe.2014.11.1065
![]() |
[19] | V. A. Thurmond, The point of triangulation, J. Nurs. Scholarsh., 33 (2001), 253-258. |
[20] | M. Leach, I. Scoones, The social and political lives of zoonotic disease models: Narratives, science and policy, Soc. Sci. Med., 88 (2013), 10-17. |
[21] |
D. T. Campbell, D. W. Fiske, Convergent and discriminant validation by the multitrait-multimethod matrix, Psychol. Bull., 56 (1959), 81-105. doi: 10.1037/h0046016
![]() |
[22] |
J. C. Greene, V. J. Caracelli, W. F. Graham, Toward a conceptual framework for mixed-method evaluation designs, Educ. Eval. Policy Anal., 11 (1989), 255-274. doi: 10.3102/01623737011003255
![]() |
[23] | D. T. Halperin, O. Mugurungi, T. B. Hallett, B. Muchini, B. Campbell, T. Magure, et al., A Surprising Prevention Success: Why Did the HIV Epidemic Decline in Zimbabwe?, PLoS Med., 8 (2011), e1000414. |
[24] |
G. W. Rutherford, W. McFarland, H. Spindler, K. White, S. V. Patel, J. Aberle-Grasse, et al., Public health triangulation: approach and application to synthesizing data to understand national and local HIV epidemics, BMC Public Health, 10 (2010), 447. doi: 10.1186/1471-2458-10-447
![]() |
[25] | J. W. Eaton, L. F. Johnson, J. A. Salomon, T. Barnighausen, E. Bendavid, A. Bershteyn, et al., HIV treatment as prevention: systematic comparison of mathematical models of the potential impact of antiretroviral therapy on HIV incidence in South Africa, Plos Med., 9 (2012), e1001245. |
[26] |
D. A. M. C. van de Vijver, B. E. Nichols, U. L. Abbas, C. A. B. Boucher, V. Cambiano, J. W. Eaton, et al., Preexposure prophylaxis will have a limited impact on HIV-1 drug resistance in sub-Saharan Africa, AIDS, 27 (2013), 2943-2951. doi: 10.1097/01.aids.0000433237.63560.20
![]() |
[27] |
A. S. Khanna, J. A. Schneider, N. Collier, J. Ozik, R. Issema, A. di Paola, et al., A modeling framework to inform preexposure prophylaxis initiation and retention scale-up in the context of "Getting to Zero" initiatives, AIDS, 33 (2019), 1911-1922. doi: 10.1097/QAD.0000000000002290
![]() |
[28] | Getting to Zero Exploratory Workgroup, Getting To Zero: A Framework to Eliminate HIV in Illinois, 2017. Available from: https://www.aidschicago.org/resources/content/1/1/1/3/documents/GTZ_framework_August_draft.pdf. |
[29] | Getting to Zero Exploratory Working Group. Getting to Zero: A Framework to Eliminate HIV in Illinois, Chicago, 2017. Available from: http://www.dph.illinois.gov/topics-services/diseases-and-conditions/hiv-aids/getting-zero. |
[30] | Illinois Department of Public Health, Illinois Electronic HIV/ AIDS Reporting System. Available from: http://www.idph.state.il.us/ehrtf/ehrtf_home.htm. |
[31] |
A. S. Khanna, S. Michaels, B. Skaathun, E. Morgan, K. Green, L. Young, et al., Preexposure prophylaxis awareness and use in a population-based sample of young black men who have sex with men, JAMA Intern. Med., 176 (2016), 136-138. doi: 10.1001/jamainternmed.2015.6536
![]() |
[32] | J. Schneider, B. Cornwell, A. Jonas, N. Lancki, R. Behler, B. Skaathun, et al., Network dynamics of HIV risk and prevention in a population-based cohort of young Black men who have sex with men, Network Sci., 2 (2017), 247. |
[33] |
W. S. Cleveland, Robust locally weighted regression and smoothing scatterplots, J. Am. Stat. Assoc., 74 (1979), 829-836. doi: 10.1080/01621459.1979.10481038
![]() |
[34] | W. S. Cleveland, LOWESS: a program for smoothing scatterplots by robust locally weighted regression, Am. Stat., 35 (1981), 54. |
[35] |
W. S. Cleveland, S. J. Devlin, Locally weighted regression: an approach to regression analysis by local fitting, J. Am. Stat. Assoc., 83 (1988), 596-610. doi: 10.1080/01621459.1988.10478639
![]() |
[36] |
J. R. Wong, J. K. Harris, C. Rodriguez-Galindo, K. J. Johnson, Incidence of childhood and adolescent melanoma in the United States: 1973-2009, Pediatrics, 131 (2013), 846-854. doi: 10.1542/peds.2012-2520
![]() |
[37] |
J. F. Ludvigsson, A. Rubio-Tapia, C. T. van Dyke, L. J. Melton, A. R. Zinsmeister, B. D. Lahr, et al., Increasing incidence of celiac disease in a north American population, Am. J. Gastroenterol., 108 (2013), 818-824. doi: 10.1038/ajg.2013.60
![]() |
[38] | J. M. Brotherton, M. Fridman, C. L. May, G. Chappell, A. M. Saville, D. M. Gertig, Early effect of the HPV vaccination programme on cervical abnormalities in Victoria, Australia: an ecological study, Lancet, 377 (2011), 2085-2092. |
[39] | R. A. Bonacci, D. R. Holtgrave, Evaluating the impact of the US national HIV/AIDS strategy, 2010-2015, AIDS Behav., 20 (2016), 1383-1389. |
[40] | S. C. Kalichman, Pence, Putin, Mbeki and their HIV/AIDS-related crimes against humanity: call for social justice and behavioral science advocacy, AIDS Behav., 27 (2017), 963-967. |
[41] |
D. R. Holtgrave, R. A. Bonacci, R. O. Valdiserri, Presidential elections and HIV-related national policies and programs, AIDS Behav., 21 (2017), 611-614. doi: 10.1007/s10461-017-1703-z
![]() |
[42] | H. I. Hall, R. Song, T. Tang, Q. An, J. Prejean, P. Dietz, et al., HIV trends in the United States: Diagnoses and Estimated Incidence, JMIR Public Heal Surveill., 3 (2017), e8. |
[43] | R. O. Valdiserri, C. H. Maulsby, D. R. Holtgrave, Structural factors and the national HIV/AIDS strategy of the USA, Struct. Dyn. HIV, (2018), 173-194. |
[44] |
G. Robins, T. Snijders, P. Wang, M. Handcock, P. Pattison, Recent developments in exponential random graph (p*) models for social networks, Soc. Networks., 29 (2007), 192-215. doi: 10.1016/j.socnet.2006.08.003
![]() |
[45] | Statnet Development Team (Pavel N. Krivitsky, Mark S. Handcock, David R. Hunter, Carter T. Butts, Chad Klumb, Steven M. Goodreau, and Martina Morris) (2003-2020). statnet: Software tools for the Statistical Modeling of Network Data. URL http://statnet.org |
[46] |
N. Collier, M. North, Parallel agent-based simulation with repast for High Performance Computing, Simulation, 89 (2013), 1215-1235. doi: 10.1177/0037549712462620
![]() |
[47] | N. Collier, J. T. Murphy, J. Ozik, E. Tatara, Repast for High Performance Computing, 2018. Available from: https://repast.github.io/repast_hpc.html. |
[48] | A. S. Khanna, N. Collier, J. Ozik, BARS: Building Agent-based Models for Racialized Justice Systems, 2017. Available from: https://github.com/khanna7/BARS. |
[49] | D. R. Holtgrave, H. I. Hall, L. Wehrmeyer, C. Maulsby, Costs, Consequences and Feasibility of Strategies for Achieving the Goals of the National HIV/AIDS Strategy in the United States: A Closing Window for Success?, AIDS Behav., 16 (2012), 1365-1372. |
[50] |
J. Koopman, Modeling infection transmission, Annu. Rev. Public Health, 25 (2004), 303-326. doi: 10.1146/annurev.publhealth.25.102802.124353
![]() |
[51] |
J. S. Koopman, Modeling infection transmission-the pursuit of complexities that matter, Epidemiology, 13 (2002), 622-624. doi: 10.1097/00001648-200211000-00004
![]() |
[52] | R. A. Bonacci, D. R. Holtgrave, U.S. HIV incidence and transmission goals, 2020 and 2025, Am. J. Prev. Med., 53 (2017), 275-281. |
[53] |
T. M. Hammett, J. L. Gaiter, C. Crawford, Reaching seriously at-risk populations: health interventions in criminal justice settings, Health Educ. Behav., 25 (1998), 99-120. doi: 10.1177/109019819802500108
![]() |
[54] | L. Bowleg, M. Teti, D. J. Malebranche, J. M. Tschann, "It's an Uphill Battle Everyday": intersectionality, low-income black heterosexual men, and implications for HIV prevention research and interventions, Psychol. Men Masculinity, 14 (2013), 25-34. |
[55] |
R. A. Jenkins, Getting to zero: we can't do it without addressing substance use, AIDS Educ. Prev., 30 (2018), 225-231. doi: 10.1521/aeap.2018.30.3.225
![]() |
[56] | J. Seeley, C. H. Watts, S. Kippax, S. Russell, L. Heise, A. Whiteside, Addressing the structural drivers of HIV: a luxury or necessity for programmes?, J. Int. AIDS Soc., 15 (2012). |
[57] | D. V. Havlir, S. P. Buchbinder, Ending AIDS in the United States-if not now, when?, JAMA Intern. Med., 179 (2019), 1165-1166. |
[58] | E. H. Layer, C. E. Kennedy, S. W. Beckham, J. K. Mbwambo, S. Likindikoki, W. W. Davis, et al., Multi-level factors affecting entry into and engagement in the HIV continuum of care in Iringa, Tanzania, Plos One, 9 (2014), e104961. |
[59] | C. H. Logie, V. L. Kennedy, W. Tharao, U. Ahmed, M. R. Loutfy, Engagement in and continuity of HIV care among African and Caribbean black women living with HIV in Ontario, Canada, Int. J. STD AIDS, 28 (2017), 969-974. |
[60] | O. Bonnington, J. Wamoyi, W. Ddaaki, D. Bukenya, K. Ondenge, M. Skovdal, et al., Changing forms of HIV-related stigma along the HIV care and treatment continuum in sub-Saharan Africa: a temporal analysis, Sex. Trans. Infect., 93 (2017), e052975. |
[61] | R. Sutton, M. Lahuerta, F. Abacassamo, L. Ahoua, M. Tomo, M. R. Lamb, et al., Feasibility and acceptability of health communication interventions within a combination intervention strategy for improving linkage and retention in HIV care in Mozambique, J. Acquired Immune Defic. Syndr., 74 (2017), S29-S36. |
[62] | C. H. Brown, D. C. Mohr, C. G. Gallo, C. Mader, L. Palinkas, G. Wingood, et al., A computational future for preventing HIV in minority communities: how advanced technology can improve implementation of effective programs, J. Acquired Immune Defic. Syndr., 63 (2013), S72-S84. |
[63] | R Core Team, The R project for statistical computing, 2019. Available from: https://www.r-project.org/. |
[64] |
A. Y. Liu, S. E. Cohen, E. Vittinghoff, P. L. Anderson, S. Doblecki-Lewis, O. Bacon, et al., Preexposure prophylaxis for HIV infection integrated with municipal- and community-based sexual health services, JAMA Intern. Med., 176 (2016), 75-84. doi: 10.1001/jamainternmed.2015.4683
![]() |
[65] |
L. K. Rusie, C. Orengo, D. Burrell, A. Ramachandran, M. Houlberg, K. Keglovitz, et al., Preexposure prophylaxis initiation and retention in care over 5 years, 2012-2017: are quarterly visits too much?, Clin. Infect. Dis., 67 (2018), 283-287. doi: 10.1093/cid/ciy160
![]() |
1. | L. Krasnobaeva, L. Yakushevich, FEATURES OF THE POTENTIAL FIELD STRUCTURE OF THE PPF1 PLASMID AND THEIR INFLUENCE ON THE CHARACTER OF MOTION OF NONLINEAR CONFORMATIONAL PERTURBATIONS – KINKS, 2022, 7, 2499-9962, 99, 10.29039/rusjbpc.2022.0490 |
Region number | Region coordinates | NA | NT | NG | NC | N |
1 | 1..85 | 22/29 | 29/22 | 17/17 | 17/17 | 85 |
2 (CDS-1) | 86..1276 | 190/268 | 268/190 | 353/381 | 381/353 | 1192 |
3 | 1277..1914 | 142/145 | 145/142 | 160/191 | 191/160 | 638 |
4 (CDS-2) | 1915..2106 | 54/37 | 37/54 | 48/53 | 53/48 | 192 |
5 | 2107..3292 | 294/268 | 268/294 | 319/305 | 305/319 | 1186 |
6 (CDS-3) | 3293..4153 | 213/223 | 223/213 | 203/222 | 222/203 | 861 |
7 | 4154-4361 | 67/64 | 64/67 | 34/42 | 42/34 | 207 |
Number of the region | Coordinate of the region | ||||
1+7 | (1..85)+(4154..4361) | 6.13/6.28 | 1.94/1.97 | 2.06/2.10 | 1.60/1.63 |
2 (CDS-1) | 86..1276 | 6.05/6.33 | 1.90/1.97 | 2.26/2.32 | 1.66/1.71 |
3 | 1277..1914 | 6.09/6.30 | 1.92/1.96 | 2.21/2.26 | 1.65/1.68 |
4 (CDS-2) | 1915..2106 | 6.26/6.13 | 1.97/1.92 | 2.23/2.19 | 1.67/1.64 |
5 | 2107..3292 | 6.25/6.14 | 1.95/1.93 | 2.22/2.20 | 1.67/1.65 |
6 (CDS-3) | 3293..4153 | 6.14/6.25 | 1.93/1.95 | 2.17/2.20 | 1.64/1.66 |
Region number | Region coordinates | NA | NT | NG | NC | N |
1 | 1..85 | 22/29 | 29/22 | 17/17 | 17/17 | 85 |
2 (CDS-1) | 86..1276 | 190/268 | 268/190 | 353/381 | 381/353 | 1192 |
3 | 1277..1914 | 142/145 | 145/142 | 160/191 | 191/160 | 638 |
4 (CDS-2) | 1915..2106 | 54/37 | 37/54 | 48/53 | 53/48 | 192 |
5 | 2107..3292 | 294/268 | 268/294 | 319/305 | 305/319 | 1186 |
6 (CDS-3) | 3293..4153 | 213/223 | 223/213 | 203/222 | 222/203 | 861 |
7 | 4154-4361 | 67/64 | 64/67 | 34/42 | 42/34 | 207 |
Number of the region | Coordinate of the region | ||||
1+7 | (1..85)+(4154..4361) | 6.13/6.28 | 1.94/1.97 | 2.06/2.10 | 1.60/1.63 |
2 (CDS-1) | 86..1276 | 6.05/6.33 | 1.90/1.97 | 2.26/2.32 | 1.66/1.71 |
3 | 1277..1914 | 6.09/6.30 | 1.92/1.96 | 2.21/2.26 | 1.65/1.68 |
4 (CDS-2) | 1915..2106 | 6.26/6.13 | 1.97/1.92 | 2.23/2.19 | 1.67/1.64 |
5 | 2107..3292 | 6.25/6.14 | 1.95/1.93 | 2.22/2.20 | 1.67/1.65 |
6 (CDS-3) | 3293..4153 | 6.14/6.25 | 1.93/1.95 | 2.17/2.20 | 1.64/1.66 |