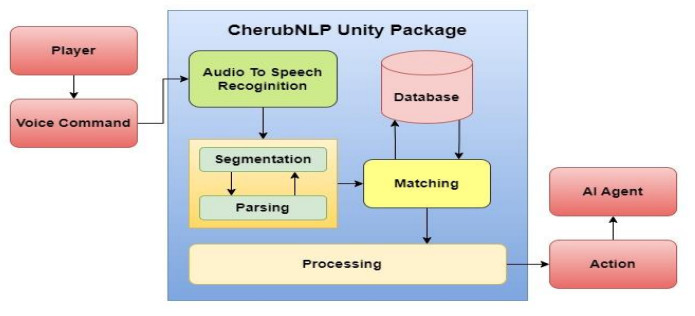
Some of the most significant computational ideas in neuroscience for learning behavior in response to reward and penalty are reinforcement learning algorithms. This technique can be used to train an artificial intelligent (AI) agent to serve as a virtual assistant and a helper. The goal of this study is to determine whether combining a reinforcement learning-based Virtual AI assistant with play therapy. It can benefit wheelchair-bound youngsters with Down syndrome. This study aims to employ play therapy methods and Reinforcement Learning (RL) agents to aid children with Down syndrome and help them enhance their abilities like physical and mental skills by playing games with them. This Agent is designed to be smart enough to analyze each patient's lack of ability and provide a specific set of challenges in the game to improve that ability. Increasing the game's difficulty can help players develop these skills. The agent should be able to assess each player's skill gap and tailor the game to them accordingly. The agent's job is not to make the patient victorious but to boost their morale and skill sets in areas like physical activities, intelligence, and social interaction. The primary objective is to improve the player's physical activities such as muscle reflexes, motor controls and hand-eye coordination. Here, the study concentrates on the employment of several distinct techniques for training various models. This research focuses on comparing the reinforcement learning algorithms like the Deep Q-Learning Network, QR-DQN, A3C and PPO-Actor Critic. This study demonstrates that when compared to other reinforcement algorithms, the performance of the AI helper agent is at its highest when it is trained with PPO-Actor Critic and A3C. The goal is to see if children with Down syndrome who are wheelchair-bound can benefit by combining reinforcement learning with play therapy to increase their mobility.
Citation: Joypriyanka Mariselvam, Surendran Rajendran, Youseef Alotaibi. Reinforcement learning-based AI assistant and VR play therapy game for children with Down syndrome bound to wheelchairs[J]. AIMS Mathematics, 2023, 8(7): 16989-17011. doi: 10.3934/math.2023867
[1] | Khaled Tarmissi, Hanan Abdullah Mengash, Noha Negm, Yahia Said, Ali M. Al-Sharafi . Explainable artificial intelligence with fusion-based transfer learning on adverse weather conditions detection using complex data for autonomous vehicles. AIMS Mathematics, 2024, 9(12): 35678-35701. doi: 10.3934/math.20241693 |
[2] | Ghazanfar Latif, Jaafar Alghazo, Majid Ali Khan, Ghassen Ben Brahim, Khaled Fawagreh, Nazeeruddin Mohammad . Deep convolutional neural network (CNN) model optimization techniques—Review for medical imaging. AIMS Mathematics, 2024, 9(8): 20539-20571. doi: 10.3934/math.2024998 |
[3] | Fahad F. Alruwaili . Ensuring data integrity in deep learning-assisted IoT-Cloud environments: Blockchain-assisted data edge verification with consensus algorithms. AIMS Mathematics, 2024, 9(4): 8868-8884. doi: 10.3934/math.2024432 |
[4] | Thavavel Vaiyapuri, Prasanalakshmi Balaji, S. Shridevi, Santhi Muttipoll Dharmarajlu, Nourah Ali AlAseem . An attention-based bidirectional long short-term memory based optimal deep learning technique for bone cancer detection and classifications. AIMS Mathematics, 2024, 9(6): 16704-16720. doi: 10.3934/math.2024810 |
[5] | Huihui Zhong, Weijian Wen, Jianjun Fan, Weijun Yang . Reinforcement learning-based adaptive tracking control for flexible-joint robotic manipulators. AIMS Mathematics, 2024, 9(10): 27330-27360. doi: 10.3934/math.20241328 |
[6] | Lingtao Wen, Zebo Qiao, Jun Mo . Modern technology, artificial intelligence, machine learning and internet of things based revolution in sports by employing graph theory matrix approach. AIMS Mathematics, 2024, 9(1): 1211-1226. doi: 10.3934/math.2024060 |
[7] | Alaa O. Khadidos . Advancements in remote sensing: Harnessing the power of artificial intelligence for scene image classification. AIMS Mathematics, 2024, 9(4): 10235-10254. doi: 10.3934/math.2024500 |
[8] | Efrén Pérez-Santín, Luis de-la-Fuente-Valentín, Mariano González García, Kharla Andreina Segovia Bravo, Fernando Carlos López Hernández, José Ignacio López Sánchez . Applicability domains of neural networks for toxicity prediction. AIMS Mathematics, 2023, 8(11): 27858-27900. doi: 10.3934/math.20231426 |
[9] | Xufeng Tan, Yuan Li, Yang Liu . Stochastic linear quadratic optimal tracking control for discrete-time systems with delays based on Q-learning algorithm. AIMS Mathematics, 2023, 8(5): 10249-10265. doi: 10.3934/math.2023519 |
[10] | Jian Qi . Artificial intelligence-based intelligent computing using circular q-rung orthopair fuzzy information aggregation. AIMS Mathematics, 2025, 10(2): 3062-3094. doi: 10.3934/math.2025143 |
Some of the most significant computational ideas in neuroscience for learning behavior in response to reward and penalty are reinforcement learning algorithms. This technique can be used to train an artificial intelligent (AI) agent to serve as a virtual assistant and a helper. The goal of this study is to determine whether combining a reinforcement learning-based Virtual AI assistant with play therapy. It can benefit wheelchair-bound youngsters with Down syndrome. This study aims to employ play therapy methods and Reinforcement Learning (RL) agents to aid children with Down syndrome and help them enhance their abilities like physical and mental skills by playing games with them. This Agent is designed to be smart enough to analyze each patient's lack of ability and provide a specific set of challenges in the game to improve that ability. Increasing the game's difficulty can help players develop these skills. The agent should be able to assess each player's skill gap and tailor the game to them accordingly. The agent's job is not to make the patient victorious but to boost their morale and skill sets in areas like physical activities, intelligence, and social interaction. The primary objective is to improve the player's physical activities such as muscle reflexes, motor controls and hand-eye coordination. Here, the study concentrates on the employment of several distinct techniques for training various models. This research focuses on comparing the reinforcement learning algorithms like the Deep Q-Learning Network, QR-DQN, A3C and PPO-Actor Critic. This study demonstrates that when compared to other reinforcement algorithms, the performance of the AI helper agent is at its highest when it is trained with PPO-Actor Critic and A3C. The goal is to see if children with Down syndrome who are wheelchair-bound can benefit by combining reinforcement learning with play therapy to increase their mobility.
Down syndrome is a genetic condition that has an impact on a person's development. It can lead to several health issues, delays in development, and physical impairments. It is possible to be born with it or to acquire it later in life. Some people will feel different effects, but some will always need care and support from others. Having an extra copy of chromosome 21 is a hallmark of Down syndrome. A child can be born with two copies of chromosome 21. Characteristic facial traits and growth abnormalities, as well as developmental delays, cardiac defects, and gastrointestinal issues, are the results of this excess material. The genetic condition known as Down syndrome affects people of every racial and ethnic background equally. India is home to the largest concentration of people with Down syndrome in the world. About 1 in every 166 births in India is affected by a Downs Syndrome disease, and 1 in every 830 births is a kid with Down syndrome. Those figures are scary enough, but the reality that this disease is so often fatal in India due to a lack of attention, education, and modern medical technology is beyond terrifying. Congenital heart abnormalities are more common in children with Down syndrome. Most babies born with Down syndrome are perfectly healthy upon arrival, but they face a higher risk of developing infections and several types of cancer in infancy. Just around half of the 23,000 to 29,000 children born with Down syndrome in India each year will live to adulthood. In countries with more sophisticated medical facilities and technology, such as the United States, patients have a better chance of survival. Prenatal screening using a blood test or ultrasound can identify babies at risk for Down syndrome. This genetic ailment has no known origin or treatment, but the older the mother, the greater the risk for her offspring to develop the disorder. Although many medical complications might arise from having a child with Down syndrome, the focus of this study is on the physical impairments these kids face [1].
Lower body weakness is the most frequent physical impairment in children with Down syndrome. Because of this, they may have trouble walking, running, and jumping. Likewise, their leg muscles could be weak. They will be confined to a wheelchair and miss out on all fun that other kids have playing games. Children with Down syndrome can have cognitive difficulties due to learning problems. Hearing loss or issues with their peripheral vision are also possibilities. Most kids will not know how to make the most of their arms or how to synchronize their hands and eyes. Because of this, they will always be dependent on other people for help with basic tasks and care [2,3]. Play therapy is a modality of care typically employed with kids. This is done so that they will not always feel comfortable talking to grown-ups about how they are feeling or the problems they are having. A play therapy session may look like any other time spent playing, but it serves a much greater purpose. The therapist's ability to observe the child at play allows for greater insight into the youngster's difficulties. If the child has experienced trauma, the therapist will be able to help them work through their emotions and heal. Through play, kids can figure out how to handle stressful situations and fix their bad habits. Several professionals in the field of mental health, such as psychologists and psychiatrists, employ play therapy. Professionals in fields such as physical therapy, social work, occupational therapy, and behavioural therapy are all engaged in this field [4].
Researchers and medical professionals have shown that games can be highly beneficial in assisting children with Down syndrome with physical and social tasks. As a bonus, some games can help kids develop better hand-eye coordination. One of gaming's best features is how many types of people can find enjoyment from it. You can find games that are appropriate for both sexes and playable by people of all ages, from infants to the elderly. The market, however, offers little choice for the disabled community. This is because the average person has no real-world experience with them and hence has no notion of what will or will not work. However, there is a dearth of options for disabled children with Down syndrome who are confined to wheelchairs. Some games on the market are tailored to the needs of children with disabilities. Games that involve physical movement or the use of physical objects (such as toys) are also included. They are geared towards enhancing the participant's ability to move and think quickly on their feet. Children with Down syndrome benefit from them because they boost their confidence and social abilities. These activities almost always necessitate the presence of an adult or other responsible guardian. Most parents of children with Down syndrome simply cannot afford to devote their lives to taking care of their kids, and they may not even be able to afford any paid help. The ideal method to address this issue is to develop a game that can be played independently under the guidance of an AI Helper. Children with Down syndrome can play this game unattended and it should help them develop their cognitive, physical, and social abilities [5,6].
The earlier research uses the Unity Game Engine, the Unity ML-Agents, and the HTC Vive Head-Mounted Display to power an AI-driven game feature that aids players' visual navigation. We discuss the use of deep reinforcement learning for completing physical activities in the same virtual reality game using Proximal Policy Optimisation and Generative Adversarial Imitation Learning. We put our mechanisms to the test by having four people work together to keep a digital butterfly safe, with the aid of an agent that may appear both as a friendly "ghost arm" and a fierce foe. Based on our findings, deep learning bots may be useful for learning game tasks and offering players fresh perspectives [7]. This study investigates the feasibility of developing a virtual reality (VR) game for children with Down syndrome who are confined to wheelchairs. The VR game would feature an AI-powered virtual assistant. The hope is that a person with Down syndrome can use this game to boost their cognitive, physical, and interpersonal skills. The player's task in this game is to keep the ball balanced on a board, but the game is cleverly programmed to use the player's virtual AI assistant to detect their areas of weakness and to continuously test them on those areas by increasing the game's difficulty and randomness. A player with Down syndrome may have a greater chance at skill development if the game is changed to increase its difficulty and challenge. In this study, numerous algorithms are used to teach the Virtual AI Assistant how to keep tabs on the player's performance and adjust the game's parameters accordingly. To determine the most effective approach to learning and developing a more capable AI assistant, this study examines the efficiency and speed with which various Reinforcement Learning algorithms such as Deep Q-Network (DQN), Asynchronous Advantage Actor Critic (A3C), Quantile Regression Deep Q-Network (QR-DQN), Proximal Policy Optimization with Actor-Critic (PPO-AC) acquire new knowledge to play in this environment efficiently.
The remaining portions of the essay are structured as follows: Section 2 analyses the related works and discuss the Down syndrome, VR technology and AI assistance. The comparison of the DQN, A3C, QR-DQN and PPO-AC models is described in Section 3. The Results and Discussion are then analyzed with the proposed VR game in Section 4, along with a performance comparison with alternative techniques. The essential findings of the suggested research are then summarized in Section 5.
Analyzed results of letting a kid pick his or her own Wii game and having family and friends join in to help. Self-efficacy, exercise adherence, and changes in physical activity and body composition and function were among the outcomes measured. Strength, speed, agility, coordination, balance, depth perception, and body composition were all evaluated. This work postulated that after the intervention period, these metrics would improve due to Wii use. Several researchers hypothesized that if kids played games that required them to use specific motor skills and talents, they would get better at using those skills and get better at those games. Keeping a child with Down syndrome interested and enthusiastic about an activity that can help with limitations in activity and impairments in body structure and function can be facilitated by letting the child make choices about which games to play and by incorporating multiplayer/family sessions. The Wii gaming system could be a great way for a child with DS to get some much-needed exercise at home, while also providing a pleasant and sociable activity the whole family can enjoy together [8,9]. The goal of this research was to see how playing Wii-Fit would affect children's balance who were diagnosed with DS. I hypothesized that providing Down syndrome children undergoing normal rehabilitation in Saudi Arabia with virtual reality (VR) using Wii game technology will improve their balance when compared to only providing them with traditional physical treatment. Thirty kids with Down syndrome had their equilibrium analyzed using the Bruininks-Oseretsky Test of Motor Proficiency [10,11], Game is designed to help people with Down syndrome learn and practice prosody-related speech skills, claims that children with Down syndrome can benefit from playing serious video games which have been proven to help keep players engaged and interested. Unfortunately, nothing has been done to aid people with Down syndrome in developing emotional awareness using interactive technology. In this study, the paper details the creation of Emotion Down, a serious video game meant to improve the emotional awareness of people with Down syndrome through testing and implementation [12].
The interface has been designed with Down syndrome users in mind, considering their limited cognitive abilities, learning capabilities, and attention span. Activities in the creation and perception of prosodic phenomena are used to convey the learning content and boost students' communicative abilities. The use of a video game to develop the oral and communication skills of people with Down syndrome is a promising area of research since the activities are introduced inside the narrative of the game, rather than as a basic succession of learning tasks [13], In a 2017 study how people with Down syndrome engage with video games, how the neuroplastic changes that occur during gaming may improve memory and learning, and how a person's affinity for a particular video game evolves. The work then goes into detail about the gaming interests of people with Down syndrome, as reported by their parents. The effects of video games on people are another area of study that sheds light on how people with Down syndrome engage with games. Physical functioning has been linked to video game play in studies assessing the effects of video games on people with Down syndrome. These investigations have focused on the potential of Wii games to enhance sensorimotor abilities, balance, and coordination. Look at the results of playing Wii-Fit, a game that uses the player's natural movements as a form of engagement. Those who were allocated to play the game showed significant gains in balance and agility, while individuals in the usual therapy group showed less significant changes. Berg, Becker and Martlan, where hopes to pinpoint the processes of video games that lead to alterations in brain plasticity in gamers, including those with Down syndrome [14].
The purpose of this research is to create a machine learning classifier that can predict the prosodic quality of utterances made by people with Down syndrome and to examine the impact of inter- individual variation on evaluation outcomes. A therapist and a prosody specialist evaluated a corpus of utterances made by people with Down syndrome while playing a video game to determine how prosodically suitable they were. An artificial classifier was educated on the expert's evaluations to make predictions about prosodic quality using basic frequency, duration, and intensity parameters. Looked at how different people with Down syndrome can be in terms of how their speech is evaluated for prosody. So, the study demonstrates some of the factors that account for the challenges of conducting an automatic evaluation of prosody in individuals with Down syndrome [15]. To compare the original developmental elements that drove the design of JECRIPE with the actual behaviour observed in a group of children playing the game, the suggested method adopts qualitative and quantitative criteria. The findings of this case study show that the evaluation method is credible and capable of identifying usability and fun issues that can be considered and fixed in subsequent game iterations [16]. There has been a lot of research done on the topic of using cutting-edge digital tools in pedagogy by the year 2023. While it is true that virtual reality (VR) can help students learn, and that some studies have shown that using VR to teach ophthalmology improves student performance, 15, it is also true that while digital methods may represent a paradigm shift in the way dental students are educated, the human tutor factor cannot be ignored, even when the results of classical and digital approaches are comparable. Therefore, the choice of when to implement digital technologies into dentistry education must be approached with extreme care.
The technology incorporates a virtual reality simulation of a ball-catching game to provide rehabilitative action practice. The patient's movements, facial expressions, and vocal commands are all picked up by the Kinect-sensing gadget. The interface is lively and visually appealing, transforming a mundane situation into a joyful one. The game has been shown to aid in the restoration of physical function, provide psychological reinforcement, boost feelings of community, and lower depressive, anxious, and tense states. In other words, the patient's upper-limb dexterity and equilibrium have much improved [17]. This research provides a reinforcement-learning-based solution to this problem by dynamically establishing a safety strategy that facilitates recovery from potentially harmful conditions. The result of our efforts will be the specification of a smart recovery procedure. The initial version of our solution is currently being put through its paces on a test bed. When a potentially harmful condition is identified, we are primarily concerned with assigning the automatic specification of a strategy to restore safety. Instead of making these decisions at runtime, the state-of-the-art relies on a predetermined strategy set during the design phase. An agent has been trained to learn how to solve a certain risky issue, and this training serves as the first validation of our work [18].
The purpose of the RL agent is to protect the patient from unfavorable and potentially harmful situations. Meanwhile, the time it takes the agent to get to a secure state or an exit must be kept to a minimum. If a person performing a nuclear examination makes a mistake in the proper sequence of actions, a software agent can help them get to a safe place. It needs to function in a challenging and uncertain setting [19]. This research analyses the effects of the Evenness VR Sensory Room based on the user's age, the type of impairment they have, how often they use the room, and their original requirements [20]. This discussion of advantages includes input from service recipients, carers, and employees. Further research is required, ideally with a bigger sample size, a type of control group, and an examination of longer-term results. As such, it is hoped that the current findings would prompt additional thought into the installation and evaluation of VR sensory rooms for adults with disabilities to determine the most effective means by which to improve their health and social inclusion [21]. This research combines motion trackers, an eye tracker, and a facial tracker to simulate the user's face in an immersive virtual reality setting, to examine the effects of this customization on the user's experience of presence and embodiment. Participants were split into two groups: one used an avatar based on their facial images, while the other used avatars based on the faces of strangers of the same gender.
Perceptions and opinions were collected using a mixed-methods strategy using a questionnaire and interview. This work adds the following to the existing literature on immersive VR, and examines the connection between embodiment and presence, as well as the consequences of an avatar's facial appearance on both concepts. The findings suggest that letting the user create an avatar based on their appearance improves embodiment. On the contrary, when it came to the second research question, from the result it is discovered that no statistically significant differences between the two categories [22].
Currently, studies show that digital games are especially suited to promote the growth of 21st- century competencies (such as communication, collaboration, systems thinking, and creativity) by encouraging the active construction of new knowledge and by providing the opportunity for players to experiment with new identities [23,24]. A gaming virtual assistant is an artificial intelligence (AI)-powered virtual assistant designed to aid players with in-game activities like delivering hints, recommendations, and strategies, answering questions, and performing tasks based on voice or text instructions. The term "presence" refers to the user's subjective experience of "being there" in the virtual world. The fundamental idea is that users will act similarly in a virtual environment as they would in a similar real-world situation if they feel as though they are truly present there. Using natural language processing and other auxiliary algorithms, conversational AI can comprehend the context of a conversation. Its core features enable it to digest information, gain insight, and respond in a completely organic manner. Automated Speech Recognition (ASR), Natural Language Understanding (NLU), Advanced Dialog Management (ADM), and Machine Learning (ML) form the foundation of the technology, together with NLP and other, more fundamental technologies. To ensure that AI algorithms are always at their most effective, natural language processing (NLP) processes are always communicating with machine learning (ML) procedures. Every interaction should be understood, interpreted, and returned to a suitable response [25,26].
To implement the Virtual assistant that understands and communicates with players is shown in Figure 1. This research will be using the CherubNLP package from SciSharp. SciSharp is a Net package mainly used for Data Science and Machine Learning. This package is used for processing the player's voice command. The package simply takes the player's voice command and converts it into an action that can have some meaning in the game. To analyse language with the use of machine learning, conversational AI currently employs a technique called natural language processing. Language processing approaches progressed from linguistics to computational linguistics to statistical natural language processing before machine learning was introduced. Eventually, deep learning will significantly improve conversational AI's natural language processing skills. A more in-depth breakdown of these four NLP stages is as follows:
1. Input Generation is the method through which the user delivers their message, be it verbally or in a written form.
2. Input Analysis, which interacts (if textual) through NLU, is a subset of NLP (NLP). This way, the original meaning and intent of the words are preserved. Automatic speech recognition (ASR) is used to interpret spoken information and transform it into language tokens for further processing when the input is verbal.
3. Dialogue Management permits a machine to respond to a user's questions in natural language by using a computer's processing power.
4. Reinforcement Learning oversees figuring out how to get better over time and enhancing the program. This feature examines input from the user to fine-tune the interaction and strengthen the appropriate response.
The complexity level reflected in conversational AI applications is entirely up to the developer. That opens the door for a wide range of products, such as virtual assistants, to carry out customer-business interactions and automate internal processes. A virtual chatbot with awareness of its surroundings is called a conversational virtual assistant. A combination of natural language understanding, natural language processing, and machine learning allows this chatbot to learn as it converses. They can tailor interactions based on a user's profile or other data provided, and they use predictive intelligence and analytics to do so. They may be able to anticipate a user's requirements and even start a dialogue with them based on their past preferences and actions [27].
Asynchronous implementations of four widely used reinforcement learning algorithms demonstrate the training-stabilizing effects of parallel actor learners, allowing for the successful training of neural network controllers using all four approaches. The top performing technique, an asynchronous variant of actor-critic, outperforms the present state-of-the-art on the Atari domain while training in half time on a single multicore CPU rather than a GPU. Furthermore, this demonstrates the success of an asynchronous actor-critic on a novel challenge involving the visual navigation of random 3D mazes [28,29]. As a result, each agent can update the gradient of its policy more frequently, which may lead to a more rapid convergence to optimal policy settings. Furthermore, using such agent's increases exploration, as each agent will be making discoveries about its environment and establishing connections between previously unrelated data. [30,31]. Information is passed from one group of interconnected neurons in an A3C to the next as it travels through the network's three layers: the input layer, the hidden layer, and the output layer. The input layer is the point of contact between the A3C algorithm and the outside world, collecting data about external events and feeding it to the hidden layer. This data is then processed by the hidden layer, which then passes along a representation of it to the output layer. This representation is then mapped to the output layer of the meaning or action that agents can take to accomplish their objectives [32].
The Q-Learning reinforcement learning technique updates its Q-values using Bellman's equation. The Q-value [Q(s, a)] represents the value of the agent being in a particular state and performing a particular action to enter that state. The Q-values for each action at each state are determined by this algorithm, which helps the agent decide what to do next. If an agent is required to carry out activities and solve complex problems like a person and perhaps even better than a human, this model must more closely match human behaviour.
NewQ(s,a)=Q(s,a)+α[Reward(s,a)+γmaxQ′(s′,a′)−Q(s,a)] | (1) |
Q target, which is the highest value that can be acquired from the subsequent state, is subtracted from Q to produce the error (or TD error), which is then calculated (current prediction of the Q-value) Our neural network can then forecast which behaviour will lead to rewards by updating the weights using the error or loss function.
Δω=α[(R+γmaxaˆQ(s′,a,ω))−ˆQ(s′,a,ω)]∇ωˆQ(s,a,ω) | (2) |
ˆAt=−V(st)+rt+γrt+1+…+γT−t+1rT−1+γT−tV(sT) | (3) |
The empirical return, indicated as ^At for a specific time step t in a finite-horizon Markov decision process, is expressed in equation (3). (MDP). The A3C algorithm and other reinforcement learning methods frequently use it.
Algorithm: Asynchronous Advantage Actor Critic (A3C)
1. Initialize global shared parameters theta and empty shared memory M
2. For each training agent i:
a. Initialize the local copy of network parameters θi=θ
b. Initialize the empty list of episode rollouts R
c. Set initial observation s=env.reset()
d. For each training step:
i. Perform timesteps in the environment using the current policy πθi
ii. Collect the experience tuple (s, a, r, s', done)
iii. Add a tuple to local rollout R
iv. If done or the end of the episode, calculate the discounted rewards and add R to M
v. If enough experience has been collected, update the global policy π_θ using the data in M.
vi. Synchronize the local copy of network parameters θi with the global copy theta
3. Repeat from step 2 until convergence or a desired number of iterations is reached.
Q Learning is a value-based technique for RL, and its deep variant, Deep Q Learning, is an extension of that technique. The goal of reinforcement learning is to train a model to predict, given a state and a set of actions and rewards, the set of actions that would yield the highest expected reward over time. Q Learning is an iterative process where the agent takes an action and then evaluates the results. An agent's actions are rewarded by the environment, which then transitions to a new state. All while the environment is being "solved, " this process is ongoing. Learning the optimal order of actions is the goal of reinforcement learning. This is accomplished by having the model try out many permutations of actions, first at random and then with a policy based on what it has learnt through rewards thus far. This process continues until the final stage of the ecosystem is reached. In Deep Q Networks, a Neural Network is utilized to train the Q-value function in place of a mapping table that would otherwise be used to keep track of the returns. Networks like these outperform traditional on/off policy-based approaches in high-dimensional state spaces. The Q-value function calculates the estimated cumulative benefit of an action and in-state s and subsequent adherence to a particular policy.
Q(s,a)=V(s)+(A(s,a)−1|A|∑aA(s,a)) | (4) |
where V(s) is the state value function, which calculates the anticipated cumulative benefit of a state 's' adhering to a particular policy. The advantage function, A(s, a), calculates the benefit of action "a" in the state "s" in comparison to the typical action value in that state. The average advantage value overall actions in state "s" is represented by the expression 1|A|∑aA(s,a)).
Loss=(r+γ∗maxa′Q(s′,a′)−Q(s,a))2 | (5) |
Where maxa′Q(s′,a′) is the maximum reward that may be gained after acting (a) in state (s), is the discount factor, and s' is the following state. The greatest Q-value for all feasible actions in the following state s' is Q(s′, a′). The estimated Q-value for the current state-action pair is represented by Q(s′, a′).
Q(s,a)=Q(s,a)+α(r+γmaxQ(s′a′)−Q(s,a) | (6) |
The update rule for the Q-value function in the Q-learning algorithm is expressed in equation (6). The Q-learning algorithm is a model-free reinforcement learning method that iteratively updates the Q-values in response to experience to determine the best Q-value function
Algorithm: Deep Q-Learning (DQN)
1. Initialize replay memory D with capacity N
2. Initialize action value function Q with random weight theta
3. Initialize the target action value function Q' with weights θ^' = θ
4. For each episode:
a. Set initial state s
b. For each timestep:
i. With probability epsilon, select a random action 'a'
ii. Otherwise select action a=argmaxaQ(s,a,θ)
iii. Execute the action in the environment and observe the reward r and next state 's''
iv. Store the transition (s, a, r, s', done) in replay memory 'D'
v. Sample random minibatch of transitions (sj,aj,rj,s′j,donej) from 'D'
vi. Set target yj=rj+γ∗maxa′Q(s′j,a,θ)
vii. Compute loss L=(yj−Q(sj,aj,θ))2
viii. Update Q function by minimizing the loss: θ←θ−(α×∂L∂θ)
ix. Every C step, set θ′=θ
x. If done, exit loop
5. Repeat from step 4 until convergence or a desired number of episodes is reached.
The method involves gathering a sample of data from its interactions with the environment and then using that data to modify its decision-making strategy. After the policy has been modified with this set of experiences, they are discarded and a fresh set of experiences is gathered according to the updated policy. This is because it is an "on-policy learning" approach, in which the collected experience samples are only good for one round of policy revision. PPO's main contribution is making sure that updates to a policy do not drastically alter its original intent. In exchange for introducing some bias, this results in more consistent training and prevents the agent from wandering down an irreversible road of stupid behaviour. Further moving down into AI agent and examine how it sets and revises its policy [33]. The value network estimates the state value function while the policy network generates a probability distribution over the possible courses of action. The following objective functions are used by the PPO-AC algorithm to update both networks:
Policy objective function:
Lclip=min(rt,clip(rt,1−ε,1+ε)×A(s,a)) | (7) |
(s, a) is the advantage function, and the clip is a clipping function that limits the update to a small range. Where are the policy network parameters, rt is the ratio of the probability of choosing an action under the new policy and the old policy, (s, a) is the advantage function, and clip is the clipping function. Value function objective function:
ˆL(V)(θV)=E[(V(s)−V∧∧(s))2] | (8) |
where V(s) is the estimated state value function, V^̂(s) is the target value calculated as the discounted sum of rewards plus the estimated value of the next state, and V(s) is the network parameters for the value.
Entropy bonus:
E(π)(θ)=E[−π(a∣s)×logπ(a∣s)] | (9) |
where π(a|s) is the probability of selecting an action 'a' in state 's'.
The total loss function for the PPO-AC algorithm is defined as:
L(θ,θV)=L(π)(θ)−c1×LV(θV)+c2×E(π)(θ) | (10) |
where the loss function's entropy, bonus term and the value function's relative weight are controlled by the hyperparameters c1 and c2.
The PPO agent will adopt an Actor-Critic methodology. The Actor and the Critic models, both Deep Neural Networks, are used. Learning what to do in response to a specific observable condition of the environment is the job of the actor model. Actors can learn from their mistakes by sending their predicted actions to the environment and watching what happens in-game. When the actions produce desirable results, such as a successful aim, the environment provides a reward. There is a negative payoff if the activity results in an own goal. The Critic paradigm consumes this incentive [34,35]. During a period, PPO remember details about the interactions with the environment, such as the observed states, actions, rewards, etc., This provides us with 128 training examples for the actor and the critic neural networks [36,37].
Algorithm: Proximal Policy Optimization with Actor-Critic (PPO-AC)
1. Initialize actor network πθ(a,s) and critic network Vπ(s)
2. Initialize the old actor network πθold to be identical to πθ
3. Initialize an empty buffer of experience 'B'
4. For each episode:
a. Set initial state 's'
b. For each timestep:
i. Sample an action a∼πθ(s,a)
ii. Execute action 'a' in the environment and observe the reward 'r' and next state 's''
iii. Store transition (s, a, r, s', done) in buffer B
iv. Set s ← s′
v. If done, exit loop
c. Compute advantages At=Rt−Vπ(st)+γ×Vπ(st+1)
d. Compute target values Yt=At+Vπ(st)
e. Update critic network by minimizing the mean squared error between
Vπ(st) and Yt:π←argminπ1/N×Σ(Yt−Vπ(st))2
f. Update the actor network using the clipped surrogate objective:
i. Compute old probabilities πθold(st,at)
ii. Compute new probabilities πθ(st,at)
iii. Compute advantage-weighted probability ratio
rt=πθ(st,at)/πθold(st,at)×At
iv. Compute clipped surrogate Lclip=min(rt,clip(rt,1−ε,1+ε))
v. Compute entropy bonus H=−β×sum(πθ(st)×log(πθ(st))
vi. Compute total objective L=Lclip−(c1×Vπ(st))+(c2×H)
vii. Update actor network by maximizing L with respect θ:θ←argmaxθL
g. Every K episode, set πθold=πθ
5. Repeat from step 4 until convergence or a desired number of episodes is reached.
The reinforcement learning technique known as QR-DQN expands upon the original DQN by employing quantile regression to estimate the distribution of the expected return rather than just a single value. QR-central DQN's tenet can use a set of quantiles to estimate the distribution of expected returns. This allows the algorithm to consider the degree of uncertainty surrounding the predicted return, allowing it to make decisions that are more robust in the face of this uncertainty. When compared with the mean squared error loss utilized by the traditional DQN method, the Huber loss function adopted by QR-DQN is more resistant to extreme data points [38]. The QR-DQN technique is effective because it learns a collection of quantiles that characterize the distribution of the expected return. To do this, the algorithm minimizes the Huber loss function during training and uses the resulting values to update the quantiles. Following this, the expected return for each action is estimated using the quantile values, and the action with the highest expected return is chosen. When compared to other reinforcement learning algorithms, QR-DQN excels in its ability to deal with non-stationary settings and distributional shifts [39]. In conclusion, QR-DQN is an efficient and adaptable reinforcement learning method that works well in highly unpredictable and non-stationary environments. Its usefulness has been shown in a wide variety of applications, and its stability and resilience have been improved using quantile regression and the Huber loss function.
L(θ)=Ez((z−Qθ(s,a))×D(z−Qθ(s′,argmax′aQθ(s′,a′))<0)) | (11) |
where
● θ is a representation of the neural network's parameters used to estimate the action value function.
● Action a s' is the next state, and Qθ(s,a) is the projected action-value function for state s.
● The move that maximises the action value function in the following state is called a'.
● z is a random sample from the quantile distribution, and D(condition) is the indicator function that equals 1 when the condition is true and 0 otherwise.
● The goal of the loss function L(θ) is to reduce the error between the target quantiles z−Qθ(s′,argmax′aQθ(s′,a′)) a and the anticipated quantiles Qθ(s,a).
● To make better decisions in the long run, the network learns to estimate the distribution of the expected returns for each action and state by minimising this error.
Algorithm: Quantile Regression Deep Q-Network (QR-DQN)
1. Initialize Q-network Q (s, a, τ) with randomly initialized parameters.
2. Initialize target Q-network QTarget((s, a, τ) with the same parameters as Q.
3. Initialize replay buffer D.
4. For each episode:
a. Initialize state 's'.
b. For each time step t:
i. With probability epsilon, select a random action a, otherwise select
a=argmaxaQ(s,a,τ)
ii. Execute the action in the environment and observe the reward r and next state s'.
iii. Store transition (s, a, r, s', done) in replay buffer D.
iv. Sample batch of transitions from D.
v. Compute the target values for each transition:
Yi=ri+γ×QTarget(s′i,argmaxaQ(s′i,a,τ′), τ')
vi. Compute the quantile regression target values for each transition:
Zi,k=ri+γ×QTarget(s′a,argmaxaQ(s′a,a,τ′),τ′a)
vii. Compute the quantile regression loss for each transition and quantile:
Li,k=∑(ρij×(Zij−Yi)), where ρij is the Huber loss function applied to the difference between the jth quantile of Q (Si,ai,τi) and Zi, k.
viii. Compute the mean loss over all quantiles: Li=(1/K)×sum(Li,k)
ix. Update Q-network by minimizing tLih respect to Q-network parameters.
x. Update target Q-network parameters QTarget←τ×QTarget+ (1 - τ) × Q
xi. If done, exit loop
5. Repeat from step 4 until convergence or a desired number of episodes is reached.
This research presents a virtual reality (VR) game that employs reinforcement learning algorithms to teach the AI companion how to interact with disabled children (such as those with Down syndrome and who use wheelchairs). This VR game is developed by using Unity3D application, a virtual reality headset and two hand controllers are required for the player (a youngster with Down syndrome) in this game as shown in Figure 2. This will facilitate their mental imaging of the VR setting and interaction with the AI helper. This game has a major advantage over the other games as this can be played without any adult supervision and can also be played at home.
This game is a conventional ball game. The ball is hurled downward from a higher location and lands on a board. To maintain the ball's equilibrium and keep it on the table, this board can be adjusted in a diagonal direction up or down. The basic components of the game are two baskets. The colours are red and green, in that order. The objective of the game is for the player to keep the ball on the board while balancing it and moving it to the green basket in some way. Which will result in a score of ten points being given to the player. If the ball goes into the red basket, there will be a negative score of ten points for the player. If the ball lands in any other location, then the player receives a deducted score of one point. The player can have a better sense of their surroundings thanks to the VR headgear. Using the functions of the hand controller, the player can engage and move the board as required. The player will be able to balance the ball on the board by moving the hand controllers in whatever way they see fit throughout this game. The artificial intelligence that serves as the player's virtual helper will initially help in reaching the objective. This is done to accumulate additional score points. Nevertheless, after a few rounds of play, the AI assistance will begin to present increasingly difficult tasks, such as not coordinating with the player's movements or moving the ball in a random pattern to hinder the player's ability to win the game.
Inside this virtual reality setting, as shown in Figure 3 the player can manipulate the hand controls to move their hand within the surroundings. It is the player's job to keep the game under control, but the ball is constantly produced one after the other to make it impossible for them to do so. Both the players' hand-eye coordination and their confidence will improve as a direct result of this. At the beginning of the game, the level of difficulty will be very low. As more of the game is played. The AI assistance will take on the player's flaws and will begin to present challenges based on the player's previous games and the weaknesses they revealed in those game.
In Figure 4, shows the objectives of the game where it specifies the game's scoring place and losing place. The AI will initially assist the player to move the ball towards the goal. That is to the green bucket. By doing this the AI will also understand the players play strategy and player's weakness.
From the Figure 5 footage, the player and the AI assistant are being competent and making a team effort to score in the game by moving the ball towards the scoring area. The AI helper will automatically make the game easier if the player is unable to come out on top after a predetermined number of attempts at playing the game. The game's difficulty is increased when the player performs well. The difficulty is gradually increased by the AI either by not cooperating with the player or by playing randomly which makes the player lose more points. This will engage the player to put more effort and thinking ability to score in the game. The Figure 6 Shows the highest level of difficulty in the game, where the board is like a maze and the player has to move the ball in the maze to the scoring bucket. The player will also be given a time lime where the player has to score within the time limit otherwise the score will be reduced by ten points.
In Figure 7, the game was completely developed using Unity Game Engine (Unity 3D) Software. The Unity software has the option to create games in 2D, 3D, 3D - Virtual Reality and so on. In this research, the game was created using the 3D - Virtual Reality option. Unity software automatically initialises all the required settings for a 3D VR environment.
Unity software also provides an option to download and integrate with third-party software packages. Here, this game makes use of 2 such packages, Unity Virtual Reality Tool Kit is a package provides the necessary interface to connect with Virtual Reality devices such as Oculus Headset, PlayStation VR Headset, VR Hand Controllers or any other VR devices. The package simply captures all the inputs or actions from VR devices and translates them into Unity game inputs. This will allow the players to interact with the VR Game Environment created by Unity using VR devices. Unity Machine Learning (ML) agents is a Package provides all the tools required to create Artificial Intelligent Agents in Unity Game. This package also the agent to train and interact with the game environment in Unity. The package comes with a predefined set of frequently used algorithms like A3C and DQN. It also provides the necessary setting to adjust and modify the hyperparameters. With the help of Python and the "ml-agents-trainer-plugin" package custom algorithm can be implemented and can also be integrated with the Unity Machine Learning Agents package. This will allow the Unity engine to train the agents in the game environment using the custom algorithm.
The agent's primary goals and work objectives are set in a separate training environment. Training the agent and defining reward/penalty functions consider the following. The agent's goal is to score. The agent gets a reward if it scores. Otherwise, the agent will get a penalty. Ball spawns randomly in the table. At Initial state, the agent can tilt the table to move the ball. The agent must always balance the ball in the table. The ball should move every second. Agents should not idle about the table. These task-specific limitations considerably affect reward/penalty functions. The agent receives a reward or a penalty if it meets the limitations. The reward function rewards actions that move the ball closer to the primary task objective. The reward function is highly tailored to reward limitations like saving the ball from going off the table and regaining control. Penalty Function penalises activities that take the ball away from the primary task aim, have a bad outcome, or violate limitations. To optimise learning and efficiency, reward and penalty levels are carefully modified from trials and errors. A virtual reality game with a trained AI aid bot can compete against a Down syndrome player. This section compares many algorithms' problem-solving abilities. First, find the best learning rate. As illustrated in Figure 8, this study compares SGD, Adagrad, vSGD, and Adam optimizers with their learning rates to find the best optimizer for all training processes. The ideal learning rate for this issue is 5 × 10−3, which minimises loss.
Five times the normal learning rate results in a 4% loss. Adam's optimiser will be used to train all algorithms for this problem with a learning rate of 5 × 10−3. When the virtual AI helper agent is trained with PPO and Actor-Critic algorithms, the loss is decreased at a rate that is consistent with respect to the conclusion of each episode. After 100 episodes, the accuracy achieved a maximum of 87 %. At the beginning of the process, the accuracy increased to between 70 and 80 % after only 25 to 30 episodes. After going through training for a total of one hundred different scenarios, it improved its accuracy to 87 %. Further training consisting of more episodes did not result in any improvement in accuracy. This demonstrates that the agent, when trained with the PPO algorithm and Actor-Critic algorithm together, can generate a powerful AI virtual assistant with an accuracy of 87% that will compete against a player with Down syndrome and help the player improve their weaknesses and skills. In Figure 9 further demonstrates that the PPO method, when paired with the Actor-Critic algorithm, has a higher performance than any other algorithm and outperforms the accuracy of the A3C algorithm, the QR-DQN algorithm, and the DQN algorithm. In complex, continuous action spaces, PPO with Actor-Critic architecture outperforms A3C, DQN, and QR-DQN. This game has continuous action space. PPO directly optimises the policy without approximating the Q-function, which is difficult for continuous action spaces. PPO also learns the best policy faster with less training samples than other algorithms in this research. Thus, the PPO Actor-Critic method outperforms all other algorithms in this study.
After that, machine learning algorithms train a second agent using the most common reinforcement strategy. "A3C" stands for asynchronous advantage actor-critic. The loss dropped below 30% in less than 30 sessions. In Figure 10, reward loss did not decrease by more than 20% after 100 episodes. The 45th episode had a 60% accuracy improvement. 74% accuracy after 100 episodes. Training on a few hundred episodes didn't improve the system's accuracy. The A3C outperforms the DQN and QR-DQN in this task. The A3C Algorithm is a parallelized version of the Actor-Critic Algorithm. It is well-suited for discrete and continuous action spaces. The current game has a complex environment. Therefore, A3C is not suited. However, the A3C algorithm performance came close to that of the PPO Actor critic.
Agent training uses Quantile Regression - Deep Q-Network. For at least 80 episodes, this strategy did not reduce loss. After a few episodes, starting with the 80th, the loss dropped to 22%. In Figure 10, the graph shows a steep loss drop. However, accuracy plateaued at 63%. This technique outperforms the DQN algorithm, however its 63% accuracy is insufficient for the VR game where the AI must play against a person with Down syndrome. Aim for 80% accuracy for a smooth experience. Distributional reinforcement learning method QR-DQN learns return distribution instead of expected value. QR-DQN works in sparse reward situations with stochastic optimum policies. In discrete action spaces with numerous optimal policies, it helps agents balance exploration and exploitation. In Figure 11, there are many rewards for an action and the action space is continuous thus the QR-DQN is not optimal for the game.
The DQN Algorithm is a value-based algorithm that uses deep neural networks to approximate the optimal Q-function. It has been shown to achieve human-level performance on Atari games and other tasks. However, in this game, the action space is continuous and the optimal policy is complex and nonlinear. DQN algorithm is well suited for discrete action space. Hence this algorithm is not optimal for this game in Figure 12.
In Figure 13, the comparison graphs visualize how the PPO algorithm when combined with Actor-Critic was able to outperform other algorithms like A3C, QR-DQN, and DQN. The highest accuracy reached was around 87%. This is considered fit for the Virtual AI Assistant and this can be integrated with the Virtual reality game to compete with Down syndrome children, thereby helping them to improve their physical and mental skills.
Reinforcement learning, play therapy, and VR games are used to create a smart AI helper in this study. Single-agent training data suggests the agent can support the Down syndrome child. It even learns gaming strategy. This study shows that the agent can increase the player's score and act as a crutch, but it needs further training to replace a human player. Designers can experiment with level layouts and player difficulties thanks to easily added support agents that can help players complete difficult stages. Reinforcement learning to generate self-learning agents instead of hard-coded bots helps shorten the development time, as shown by this work. Deep Q-learning performs best in simple circumstances and struggles when the game is slightly complicated. With future development of environment-oriented algorithms like the A3C algorithms, it could repeat earlier results. In this game, A3C and PPO with Actor-critic are more efficient than DQN and QR-DQN. The proposed approach is under development. Gameplay mechanics and AI agents need more research to accurately promote Down syndrome therapeutic advantages. One such mechanic is to progressively increase the difficulty by putting barriers in the game's easy portions. The AI Agent will analyse the player's weaknesses and challenge them accordingly. The difficult atmosphere can boost self-confidence and self-reliance. The programme can also target specific or several disabled down syndromes. This game can be modified to an online multiplayer game where several Down syndrome youngsters can play and communicate with their online friends and AI companions.
The authors extend their appreciation to the Deanship for Research & Innovation, Ministry of Education in Saudi Arabia for funding this research work through project number: IFP22UQU4281768DSR159.
Conceptualization, Y.A., and J.M.; methodology, S.R.; software, J.M.; validation, Y A., S.R. and J.M.; formal analysis, Y.A.; investigation, S.R.; resources, Y.A.; data curation, J.M.; writing—original draft preparation, S.R.; writing—review and editing, Y.A.; visualization, Y.A.; supervision, J.M.; project administration, S.R.; funding acquisition, Y.A. All authors have read and agreed to the published version of the manuscript.
The authors declare no conflict of interest.
[1] | V. Oriol, I. Babuschkin, W. M. Czarnecki, M. Mathieu, A. Dudzik, J. Chung, et al., Grandmaster level in starcraft ii using multi-agent reinforcement learning, Nature, 575 (2019), 350–54, https://doi.org/10.1038/s41586-019-1724-z |
[2] |
Q. Shunian, Z. Li, Z. Pang, Z. Li, Y. Tao, Multi-Agent optimal control for central chiller plants using reinforcement learning and game theory, Systems, 11 (2023), 136. https://doi.org/10.3390/systems11030136 doi: 10.3390/systems11030136
![]() |
[3] | S. Konstantinos, G. K. Sidiropoulos, G. A. Papakostas, Reinforcement learning in game industry-review, prospects and challenges, Appl. Sci., 13 (2023), 2443. https://doi.org/10.3390/app13042443 |
[4] | L. Hyun-Kyo, J. Kim, J. Heo, Y. H. Han, Federated reinforcement learning for training control policies on multiple IoT devices, Sensors, 20 (2020). https://doi.org/10.3390/s20051359 |
[5] |
G. Yang, Y. Cheng, C. L. P. Chen, X. Wang, Proximal policy optimization with policy feedback, IEEE T. Syst. Man Cy-S., 52 (2022), 4600–4610. https://doi.org/10.1109/TSMC.2021.3098451 doi: 10.1109/TSMC.2021.3098451
![]() |
[6] |
D. A. Elena, F. Vallone, M. C. Zurlo, D. Marocco, SG-ACCORD: Designing virtual agents for soft skills training in the school context, Educ. Sci., 12 (2022), 174. https://doi.org/10.3390/educsci12030174 doi: 10.3390/educsci12030174
![]() |
[7] | A. Elor, S. Kurniawan, Deep reinforcement learning in immersive virtual reality exergame for agent movement guidance, IEEE 8th International Conference on Serious Games and Applications for Health (SeGAH), (2020), 1–7. https://doi.org/10.1109/SeGAH49190.2020.9201901 |
[8] |
K. A. Ogudo, R. Surendran, O. I. Khalaf, Optimal artificial intelligence based automated skin lesion detection and classification model, Comput. Syst. Sci. Eng., 44 (2023), 693–707, https://doi.org/10.32604/csse.2023.024154 doi: 10.32604/csse.2023.024154
![]() |
[9] |
B. Patti, T. Becker, A. Martian, K. D. Primrose, J. Wingen, Motor control outcomes following nintendo wii use by a child with down syndrome, Pediatric Physical Therapy: The Official Publication of the Section on Pediatrics of the American Physical Therapy Association, 24 (2012), 78–84. https://doi.org/10.1097/PEP.0b013e31823e05e6 doi: 10.1097/PEP.0b013e31823e05e6
![]() |
[10] |
C. Mario, P. M. Castilla, D. E. Mancebo, L. Aguilar, C. G. Ferreras, V. C. Payo, Automatic assessment of prosodic quality in Down syndrome: Analysis of the impact of speaker heterogeneity, NATO Adv. Sci. Instit. Series E: Appl. Sci., 9 (2019), 1440. https://doi.org/10.3390/app9071440 doi: 10.3390/app9071440
![]() |
[11] | G. F. César, D. E. Mancebo, M. C. Astorgano, L. A. Cuevas, V. F. Lucas, Engaging adolescents with down syndrome in an educational video game, Int. J. Human-Comput. Int., 33 (2017), 693–712. |
[12] | M. H. Lara, A. I. Martinez-García, K. Caro, Emotion4Down: A serious video game for supporting emotional awareness of people with down syndrome. In Proceedings of the 8th Mexican Conference on Human-Computer Interaction, MexIHC '21 5. New York, NY, USA: Association for Computing Machinery, 2 (2022), 1–5. https://doi.org/10.1145/3492724.3492729 |
[13] | M. Isys, D. G. Trevisan, C. N. Vasconcelos, Esteban, Observed interaction in games for down syndrome children, In 2015 48th Hawaii International Conference on System Sciences, (2015) 662–671. https://doi.org/10.1109/HICSS.2015.86 |
[14] |
C. J. Mills, D. Tracey, R. Kiddle, R. Gorkin, Evaluating a virtual reality sensory room for adults with disabilities, Sci. Rep-UK., 13 (2023), 495. https://doi.org/10.1038/s41598-022-26100-6 doi: 10.1038/s41598-022-26100-6
![]() |
[15] |
P. Kelsey, J. L. Sherry, Parental perspectives on video game genre preferences and motivations of children with down syndrome, J. Enabling Technol., 12 (2023), 1–9. https://doi.org/10.1108/JET-08-2017-0034 doi: 10.1108/JET-08-2017-0034
![]() |
[16] | R. Pedro, F. Nicolau, M. Norte, E. Zorzal, J. Botelho, V. Machado, et al., Preclinical dental students self-assessment of an improved operative dentistry virtual reality simulator with haptic feedback, Sci. Rep-UK, 13 (2023), 2823. https://doi.org/10.1038/s41598-023-29537-5 |
[17] | Y. Shih-Ching, W. Hwang, T. Huang, W. Liu, Y. Chen, Y. Hung, A study for the application of body sensing in assisted rehabilitation training, International Symposium on Computer, Consumer and Control, (2012), 922–925. https://doi.org/10.1109/IS3C.2012.240 |
[18] | P. Giovanni, A. Coronato. A Reinforcement-Learning-Based Approach for the Planning of Safety Strategies in AAL Applications, Intelligent Environments Amsterdam, (2018), 498–505. https://doi.org/10.3233/978-1-61499-874-7-498 |
[19] | P. Giovanni, A. Coronato, M. Naeem, G. D. Pietro, A reinforcement learning-based approach for the risk management of e-health environments: A case study. 14th International Conference on Signal-Image Technology & Internet-Based Systems (SITIS). (2018), 711–716. https://doi.org/10.1109/SITIS.2018.00114 |
[20] |
T. Thanarajan, Y. Alotaibi, S. Rajendran, K. Nagappan, Improved wolf swarm optimization with deep-learning-based movement analysis and self-regulated human activity recognition, AIMS Math., 8 (2023), 12520–12539. https://doi.org/10.3934/math.2023629 doi: 10.3934/math.2023629
![]() |
[21] | S. Haejung, T. H. Laine, Influence of avatar facial appearance on users' perceived embodiment and presence in immersive virtual reality, Electronics, (2023). https://doi.org/10.3390/electronics12030583 |
[22] |
P. Adéla, T. Morton, F. J. A. Perez-Cueto, G. Makransky, A randomized trial testing the effectiveness of virtual reality as a tool for pro environmental dietary change, Sci. Rep-UK., 12 (2022), 14315. https://doi.org/10.1038/s41598-022-18241-5 doi: 10.1038/s41598-022-18241-5
![]() |
[23] | N. Takashi, H. Sato, Y. Asa, T. Koike, K. Miyata, E. Nakagawa, et al., Achieving affective human–virtual agent communication by enabling virtual agents to imitate positive expressions, Sci. Rep-UK., 10 (2020), 5977. https://doi.org/10.1038/s41598-020-62870-7 |
[24] | O. J. van, F. Dignum, Agent communication for believable human-like interactions between virtual characters, Cognitive Agents for Virtual Environments, (2013). 37–54. https://doi.org/10.1007/978-3-642-36444-0_3 |
[25] | C. Karina, I. A. Encinas-Monroy, V. L. Amado-Sanchez, O. I. Islas-Cruz, E. A. Ahumada-Solorza, L. A. Castro, Using a gesture-based videogame to support eye-hand coordination and pre-literacy skills of children with down, 79 (2020), 1–28. https://doi.org/10.1007/s11042-020-09452-x |
[26] |
T. Tamilvizhi, R. Surendran, K. Anbazhagan, K. Rajkumar, Quantum behaved particle swarm Optimization-Based deep transfer learning model for sugarcane leaf disease detection and classification, Math. Probl. Eng., 2022 (2022), 3452413. https://doi.org/10.1155/2022/3452413 doi: 10.1155/2022/3452413
![]() |
[27] |
W. Linwan, N. A. Dodoo, T. J. Wen, L. Ke, Understanding twitter conversations about artificial intelligence in advertising based on natural language processing, Int. J. Adver., 41 (2022), 685–702. https://doi.org/10.1080/02650487.2021.1920218 doi: 10.1080/02650487.2021.1920218
![]() |
[28] | M. Corrales-Astorgano, Prosody training of people with down syndrome using an educational video game, In Iber SPEECH ISCA: ISCA. 2021-37, Syndrome, Multimed. Tools Appl., 79 (2021), 34101–34128. https://doi.org/10.21437/iberspeech |
[29] | E. David, M. Corrales-Astorgano, V. Cardeñoso-Payo, L. Aguilar, C. González-Ferreras, P. Martínez-Castilla, et al., PRAUTOCAL Corpus: A corpus for the study of down syndrome prosodic aspects, Lang. Resour. Eval., 56 (2022), 191–224. https://doi.org/10.1007/s10579-021-09542-8 |
[30] |
S. S. Rawat, S. Singh, Y. Alotaibi, S. Alghamdi, G. Kumar, Infrared target-background separation based on weighted nuclear norm minimization and robust principal component analysis, Mathematics, 10 (2022), 2829. https://doi.org/10.3390/math10162829 doi: 10.3390/math10162829
![]() |
[31] |
R. Meenakshi, R. Ponnusamy, S. Alghamdi, O. Ibrahim Khalaf, Y. Alotaibi, Development of mobile app to support the mobility of visually impaired people, Comput. Mater. Con., 73 (2022), 3473–3495. https://doi.org/10.32604/cmc.2022.028540 doi: 10.32604/cmc.2022.028540
![]() |
[32] | Y. A. Alotaibi, New Meta-Heuristics Data Clustering Algorithm Based on Tabu Search and Adaptive Search Memory. Symmetry. 14 (2022), 623. https://doi.org/10.3390/sym14030623 |
[33] | C. Hyunji, S. Lee. Intelligent Virtual Assistant Knows Your Life (2018). http://arXiv.org/abs/1803.00466 |
[34] |
N. Krishnaraj, S. Rajendran, Y. Alotaibi. Trust aware multi-objective metaheuristic optimization based secure route planning technique for cluster based iiot environment, IEEE Access., 10 (2022), 112686–112694. https://doi.org/10.1109/ACCESS.2022.3211971 doi: 10.1109/ACCESS.2022.3211971
![]() |
[35] |
S. Rajagopal, T. Thanarajan, Y. Alotaibi, S. Alghamdi, Brain tumor: Hybrid feature extraction based on UNET and 3DCNN, Comput. Syst. Sci. Eng., 45 (2023), 2093–2109. https://doi.org/10.32604/csse.2023.032488 doi: 10.32604/csse.2023.032488
![]() |
[36] | R. T. Radha, R. Surendran, A. Meshal, Penguin Search Optimization Algorithm with Multi-agent Reinforcement Learning for Disease Prediction and Recommendation Model, J. Intell. Fuzzy Sys., (2023), 1–13. https://doi.org/10.3233/JIFS-223933 |
[37] |
S. S. Rawat, S. Alghamdi, G. Kumar, Y. Alotaibi, O. I. Khalaf, L. P. Verma, Infrared small target detection based on partial sum minimization and total variation, Mathematics, 10 (2022), 671. https://doi.org/10.3390/math10040671 doi: 10.3390/math10040671
![]() |
[38] |
C. Taehyeok, K. Cho, Y. Sung, Approaches that use domain-specific expertise: behavioral-cloning-based advantage actor-critic in basketball games, Sci. China, Ser. A, 11 (2023), 1110. https://doi.org/10.3390/math11051110 doi: 10.3390/math11051110
![]() |
[39] |
F. Jie, Y. Rao, Q. Luo, J. Xu, Solving one-dimensional cutting stock problems with the deep reinforcement learning, Sci. China, Ser. A, 11 (2023), 1028. https://doi.org/10.3390/math11041028 doi: 10.3390/math11041028
![]() |
1. | Joypriyanka M, Surendran R, 2023, Reinforcement Learning in Supermarket Tycoon to Enhance Multitasking and Problem-Solving in Autistic Children, 979-8-3503-0777-1, 234, 10.1109/3ICT60104.2023.10391564 | |
2. | Pradeep Surya Dadi, Geetha Rani K, Sathish Kumar P. J, Rajasekar B, Surendran R, 2024, Exoskeleton Pysiotherapy and Assistive Robotic Arm, 979-8-3503-7999-0, 138, 10.1109/ICSCSS60660.2024.10625414 | |
3. | Youseef Alotaibi, Arun Mozhi Selvi Sundarapandi, Subhashini P, Surendran Rajendran, Computational linguistics based text emotion analysis using enhanced beetle antenna search with deep learning during COVID-19 pandemic, 2023, 9, 2376-5992, e1714, 10.7717/peerj-cs.1714 | |
4. | Joypriyanka M, Surendran R, 2023, CheckersMind: Enhancing Cognitive Ability in Dimentia Patients Through Checkers Game Therapy with Chatbot, 979-8-3503-0088-8, 924, 10.1109/ICOSEC58147.2023.10275905 | |
5. | Logapriya E, Surendran R, 2023, Hybrid Recommendations System for Women Health Nutrition at Menstruation Cycle, 979-8-3503-0777-1, 357, 10.1109/3ICT60104.2023.10391518 | |
6. | Joypriyanka M, Surendran R, 2023, Checkers Game Therapy to Improve the Mental Ability Of Alzheimer’s Patient using AI Virtual Assistant, 979-8-3503-2579-9, 96, 10.1109/ICAISS58487.2023.10250519 | |
7. | Umamah Khalid, Muddasar Naeem, Fabrizio Stasolla, Madiha Syed, Musarat Abbas, Antonio Coronato, Impact of AI-Powered Solutions in Rehabilitation Process: Recent Improvements and Future Trends, 2024, Volume 17, 1178-7074, 943, 10.2147/IJGM.S453903 | |
8. | Logapriya E, Surendran R, 2024, Combination of Food Nutrition Recommend for Women Healthcare at Menstrual Bleeding, 979-8-3503-7999-0, 1438, 10.1109/ICSCSS60660.2024.10624744 | |
9. | Youseef Alotaibi, R Deepa, K Shankar, Surendran Rajendran, Inverse chi-square-based flamingo search optimization with machine learning-based security solution for Internet of Things edge devices, 2024, 9, 2473-6988, 22, 10.3934/math.2024002 | |
10. | Rishi Saravanan, Nidhi Saravanan, 2024, Extended Reality for Neuro-Diverse Children: A Personalized Approach Using XR Data Collection and Reinforcement Learning, 979-8-3315-0691-9, 411, 10.1109/ISMAR-Adjunct64951.2024.00116 | |
11. | Mirko Casu, Salvatore Bellissima, Giorgia Farruggio, Chiara Farrauto, Pasquale Caponnetto, 2024, A Case Study on the Use of Hypnosis Combined with Virtual Reality in Psychological Partner Violence, 979-8-3503-7800-9, 793, 10.1109/MetroXRAINE62247.2024.10797144 | |
12. | Joypriyanka M, Surendran R, Sathish Kumar P. J, Sivasangari A, 2024, Game Therapy for Specially-Abled Individuals with PPO Reinforcement Learning in VR-based Educational Games, 979-8-3503-8960-9, 1126, 10.1109/ICDICI62993.2024.10810847 | |
13. | Suresh Maruthai, Tamilvizhi Thanarajan, T Ramesh, Surendran Rajendran, Multi-axis transformer based U-Net with class balanced ensemble model for lung disease classification using X-ray images, 2025, 0895-3996, 10.1177/08953996251317416 | |
14. | Raveena Selvanarayanan, Surendran Rajendran, Youseef Alotaibi, Early Detection of Colletotrichum Kahawae Disease in Coffee Cherry Based on Computer Vision Techniques, 2024, 139, 1526-1506, 759, 10.32604/cmes.2023.044084 | |
15. | Naveen Kumar R, Surendran R, Sumathy K, 2024, Improved AI-Generated Multitrack Music with Variational Autoencoders (VAE) for Harmonious Balance Compared to Recurrent Neural Network for Coherence, 979-8-3503-6472-9, 1281, 10.1109/AECE62803.2024.10911702 | |
16. | H. Christopher, Surendran R, Madhusundar. N, 2024, Improving memory and problem-solving skills of socially anxious people using game therapy, 979-8-3503-6472-9, 1350, 10.1109/AECE62803.2024.10911097 | |
17. | Abinaya M, Suneel Kumar Duvvuri, Sathish Kumar P. J, Surendran R, K S. Balamurugan, 2025, Improving Early Cardiomyopathy Diagnosis with CNNs: A Deep Learning-Based Approach, 979-8-3315-2076-2, 1, 10.1109/IEMENTech65115.2025.10959655 |