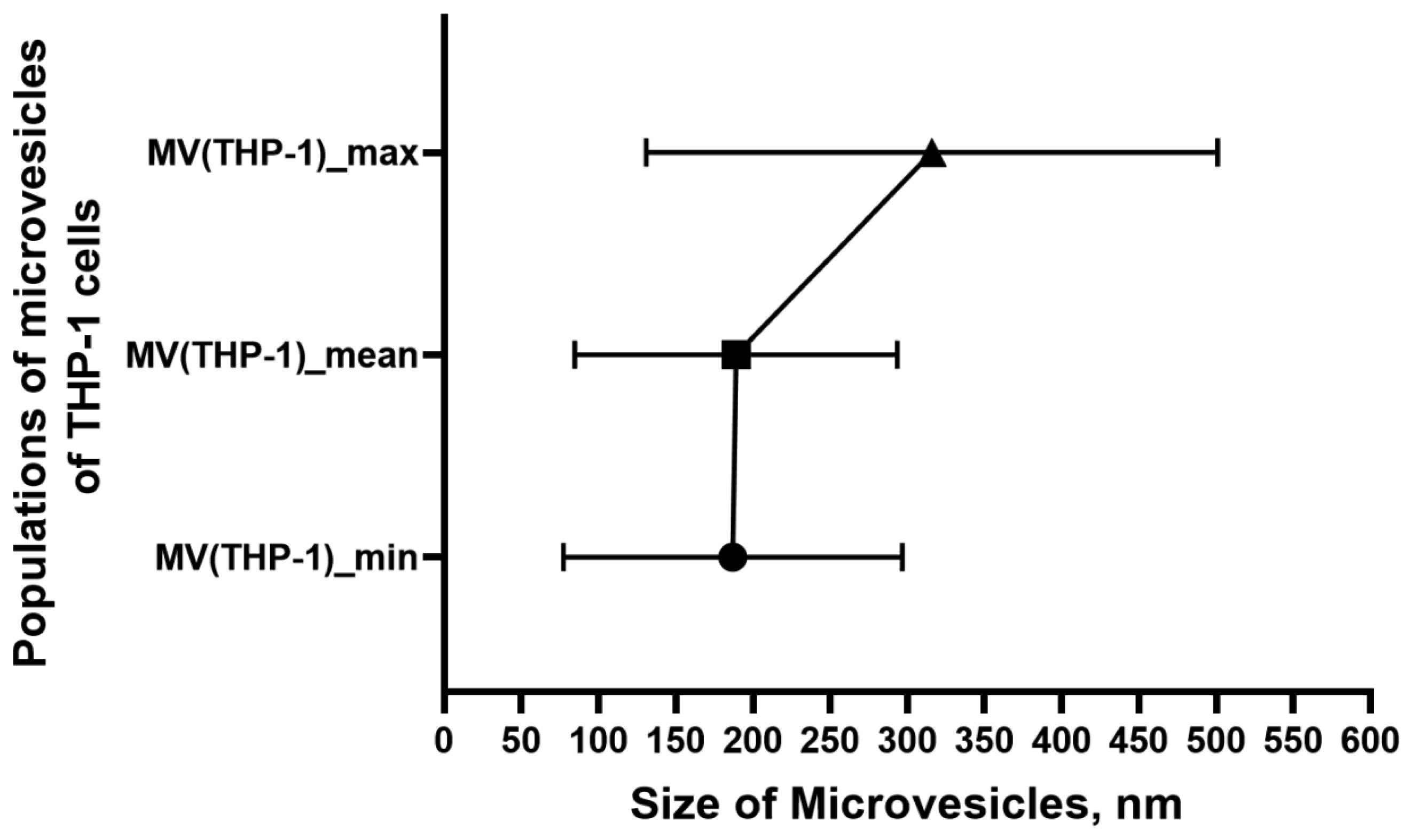
Citation: Muhammad Sadeqi Nezhad, Farhad Seif, Ilad Alavi Darazam, Azam Samei, Monireh Kamali, Hossein Aazami, Monireh Mohsenzadegan, Yaghoub Mollaei-Kandelousi, Pegah Babaheidarian, Majid Khoshmirsafa, Mohsen Fateh. An overview of the prominence of current diagnostic methods for diagnosis of COVID-19[J]. AIMS Allergy and Immunology, 2020, 4(3): 60-74. doi: 10.3934/Allergy.2020006
[1] | Cameron Browne . Immune response in virus model structured by cell infection-age. Mathematical Biosciences and Engineering, 2016, 13(5): 887-909. doi: 10.3934/mbe.2016022 |
[2] | Kaushik Dehingia, Anusmita Das, Evren Hincal, Kamyar Hosseini, Sayed M. El Din . Within-host delay differential model for SARS-CoV-2 kinetics with saturated antiviral responses. Mathematical Biosciences and Engineering, 2023, 20(11): 20025-20049. doi: 10.3934/mbe.2023887 |
[3] | Yan Li, Kuan Wang, Lejun Wang, Tongbo Chang, Shengnian Zhang, Wenxin Niu . Biomechanical analysis of the meniscus and cartilage of the knee during a typical Tai Chi movement—brush-knee and twist-step. Mathematical Biosciences and Engineering, 2019, 16(2): 898-908. doi: 10.3934/mbe.2019042 |
[4] | Jun Wang, Mingzhi Gong, Zhenggang Xiong, Yangyang Zhao, Deguo Xing . Immune-related prognostic genes signatures in the tumor microenvironment of sarcoma. Mathematical Biosciences and Engineering, 2021, 18(3): 2243-2257. doi: 10.3934/mbe.2021113 |
[5] | Bindu Kumari, Chandrashekhar Sakode, Raghavendran Lakshminarayanan, Prasun K. Roy . Computational systems biology approach for permanent tumor elimination and normal tissue protection using negative biasing: Experimental validation in malignant melanoma as case study. Mathematical Biosciences and Engineering, 2023, 20(5): 9572-9606. doi: 10.3934/mbe.2023420 |
[6] | Cameron J. Browne, Chang-Yuan Cheng . Age-structured viral dynamics in a host with multiple compartments. Mathematical Biosciences and Engineering, 2020, 17(1): 538-574. doi: 10.3934/mbe.2020029 |
[7] | A. M. Elaiw, N. H. AlShamrani, A. D. Hobiny . Stability of an adaptive immunity delayed HIV infection model with active and silent cell-to-cell spread. Mathematical Biosciences and Engineering, 2020, 17(6): 6401-6458. doi: 10.3934/mbe.2020337 |
[8] | Andrea Bondesan, Antonio Piralla, Elena Ballante, Antonino Maria Guglielmo Pitrolo, Silvia Figini, Fausto Baldanti, Mattia Zanella . Predictability of viral load dynamics in the early phases of SARS-CoV-2 through a model-based approach. Mathematical Biosciences and Engineering, 2025, 22(4): 725-743. doi: 10.3934/mbe.2025027 |
[9] | Huan Kong, Guohong Zhang, Kaifa Wang . Stability and Hopf bifurcation in a virus model with self-proliferation and delayed activation of immune cells. Mathematical Biosciences and Engineering, 2020, 17(5): 4384-4405. doi: 10.3934/mbe.2020242 |
[10] | Peter S. Kim, Joseph J. Crivelli, Il-Kyu Choi, Chae-Ok Yun, Joanna R. Wares . Quantitative impact of immunomodulation versus oncolysis with cytokine-expressing virus therapeutics. Mathematical Biosciences and Engineering, 2015, 12(4): 841-858. doi: 10.3934/mbe.2015.12.841 |
Monocytes\macrophages are actively involved in the angiogenesis regulation [1]–[3]. They regulate the growth and remodeling of blood and lymphatic vessels in different ways. Macrophages can inhibit angiogenesis by triggering apoptosis in ECs and phagocytizing the resulting cellular detritus. This is characteristic of classically activated macrophages (M1). Alternatively, activated macrophages stimulate angiogenesis by producing angiogenic factors such as VEGF-A, VEGF-C, as well as participating in the selection of the leading cell of the growing vessel [4]. Macrophages are involved in the control of angiogenesis at all stages via the production of cytokines and other substances. They secrete angiogenin and human angiogenic factor (h-AF), which stimulate EC migration [5]–[7]. Macrophages secrete vasoactive substances such as VEGF and prostaglandins that increase vascular permeability. Macrophages produce VEGF, bFGF, PDGF, IGF, IL-8, IL-6, and FGF10 cytokines that control EC proliferation and migration [6],[8]. IFNγ, TNFα, and TGFβ have the opposite effect on the proliferation and migration of ECs [6]. Macrophages are actively involved in both degradation and synthesis of extracellular matrix (ECM) components, which are the most important participant in angiogenesis. Macrophages are the sources for two types of proteases—metalloproteinases (MMPs): MMP2, MMP9 [9], and serine proteases: urokinase-type plasminogen activator (uPA) and its inhibitor (PAI). These enzymes control ECM degradation and release of matrix-related growth factors [10].
Monocytes and macrophages [11], along with many other cells, such as ECs, epithelial cells, nerve cells, tumor cells of various origins, stem cells, cells of the immune system, are producers of MVs. MVs are relatively new objects of biological research, and therefore in the literature, this group of extracellular vesicles is denoted by various terms: shedding vesicles, shedding bodies, ectosomes, MVs [12], and exovesicles [13]. The diameter of MVs varies between studies in the range of 100–1000 nm [14]. They form everywhere on the plasma membrane, budding out into the extracellular space. Proteins and nucleic acids are believed to enter MVs by targeted transport [12],[14],[15]. Unlike exosomes, the molecular composition of MVs is less well understood, but it is known that depending on the type of producing cells, MVs can be enriched with matrix metalloproteinases [16]–[18], glycoproteins such as GPIb, GPIIb-IIIa, P-selectin [19]–[21], and integrins such as Mac-1 [19],[22]. The protein profile of MVs is believed to be highly dependent on the type of source cells. It was found that depending on the type of macrophage activation, the characteristics of the produced MVs can vary [23].
The biological effects of macrophage-derived MVs are studied using cell lines, such as THP-1 cell line. The use of cell lines is justified by the fact that it is extremely difficult to isolate MVs derived from a certain cell population from biological fluids. One of the areas of research on MVs composition is the identification of microRNAs in MV fractions obtained from both cell cultures and peripheral blood. For example, it was shown that MVs derived from monocytic leukemia THP-1 cells contain miRNA-150, and thereby contribute to an increase in the EC transmigration activity. In addition to miRNA-150, the following microRNAs were found in MVs produced by THP-1 cells: miRNA-20a, miRNA-21, miRNA-23a, miRNA-26b, miRNA-27b, miRNA-30d, miRNA-25, miRNA-29d, miRNA-146a, miRNA-181b, miRNA-221, miRNA-320 [24]. The content of some microRNAs in MVs increased, when cells were exposed to LPS, hydrogen peroxide, and oleic/palmitic acids [24],[25]. It is believed that microRNAs are selectively packed into MVs, and the vesicular microRNA profile may differ from cellular microRNAs [26]. It was found that MVs derived from THP-1 cells contain Argonaute 2 protein, which is required for microRNA activity [24]. Activation of THP-1 cells with LPS resulted in MV production, which induced expression of adhesion receptors by ECs [27]. Yet, possible influence of MVs derived from unstimulated THP-1 cells on cell phenotype and functions needs to be defined more precisely.
Thus, macrophages are widely represented in the human body and serve as a source of a wide range of biologically active molecules, in particular, cytokines and growth factors, microRNAs. Macrophages are involved in a number of physiological processes, including the regulation of angiogenesis. Monocytes\macrophages produce MVs containing a variety of substances. Currently, the protein component and functions of MVs produced by monocytes\macrophages are poorly understood. In this research, we studied the effect of MVs produced by monocytic leukemia THP-1 cells on the phenotype, proliferation, migration, and vascular formation of EA.Hy926 ECs.
ТНР-1 cell line was obtained from the ATCC (USA). The cell culture was kept in the suspension form at a concentration of 0.7–1 × 106 cells/mL in a complete RPMI 1640 medium with 10% fetal calf serum (FCS) (Sigma, USA).
EA.Hy926 ECs were provided by Dr. Edgel (University of North Carolina, NC, USA). The main characteristics of these cells are typical for ECs [28]–[30]. The cells were cultured as previously reported [31]. Cell viability was checked using Trypan blue solution and comprised an average 96%. All experiments involving cell culturing were performed under the same incubation conditions (humid environment, 37 °C, 5% CO2).
Before the experiments, FCS was frozen while stored and then thawed and inactivated by the standard protocol (heating to 56 °C). The heating of FCS might lead to the destruction of MVs, which are possibly present in the media. All solutions (including culture media and FCS) for isolating MVs and experimenting on them were filtered through a 0.2 µm pore size filter (Corning, Germany) before the experiments [32]. ТНР-1 cells were cultured in flasks in DMEM/F-12 supplemented with 10% FCS, 2 mM L-glutamine, 100 U/mL penicillin, and 100 µg/mL streptomycin (Sigma, USA) if else not specified. Further in the article, cells cultured under these conditions are defined as unstimulated. The day before MV separation, the medium was completely replaced with fresh culture medium filtered through a 0.2 µm pore size filter. THP-1 cells were diluted to a concentration of 1 × 106/mL. Cell viability was assessed the day after culture initiation. We centrifuged the culture media obtained from flasks at 200 g (22 °C) for 10 min allowing cell removal. Currently, due to the absence of a single standard method for the separation of MVs, various approaches to obtain MV fractions with different purity and enrichment levels are used [33],[34]. To separate MVs, we used previously reported differential centrifugation method [35],[36]. Briefly, supernatants containing MVs were centrifuged at 500, 9900 and 19800 g. The supernatant was then discarded, and the pellet was resuspended in Hanks' solution without Ca2+ and Mg2+.
As we previously reported, to control the size of the isolated MVs we used the laser correlation spectrometer (Zetаsizer NanoZS, Malvern Instruments, UK) [37]. The experiments were repeated five times. The size of the MVs produced by THP-1 cells was in the range of 169–400 nm (Figure 1).
The peak of the MV quantity distribution was 200 nm. These data complied with previous works [32],[36],[38],[39], which ascertained the size of MVs.
The total protein concentration in the cell and MV lysates was determined by the Bradford method [40] using a NanoDrop One spectrophotometer (Thermo Scientific, MA, USA). After culturing for 24 h (as described above), the total protein amount in MVs produced by source THP-1 cells was 0.15 ± 0.036 µg/106. The obtained data allowed us to calculate the protein load of microchips for gel electrophoresis. Based on the total protein amount in MV probes of unstimulated THP-1 cells, we prepared the dilutions of MVs in culture media for further experiments: 3.276 µg/100 µL, 1.638 µg/100 µL, 0.819 µg/100 µL and 0.410 µg/100 µL.
For phenotype evaluation, THP-1 cells were centrifuged for 10 min at 200 g, then cells were diluted to a concentration of 1 × 106 cells per 300 µL of Hanks' solution. MVs isolated from 30 mL of THP-1 cell culture medium were diluted in 1 mL of Hanks' solution containing 0.35% BSA (Sigma, USA). The 100 µL of MV suspension was used for phenotype analysis. THP-1 cells and their MVs were labeled with monoclonal antibodies against CD11a PE-Cy7 clone HI111 (BD, USA), CD11b PerCP clone 238446 (RD, USA), CD11с Alexa Fluor 700 clone ICRF 3.9 (RD, USA), CD18 PE clone 212701 (RD, USA), CD29 PE clone P5D2 (RD, USA), CD54 adhesion molecules FITC clone BBIG-I1 (11C81) (RD, USA); CD119 (IFNγR) FITC clone 92101 (RD, USA), CD120a (TNFαR1) Alexa Fluor 488 clone 16803 (RD, USA), CD120b (TNFαR2) Alexa Fluor 700 clone 22235 (RD, USA), VEGF-R1 PE clone 49560 (RD, USA), VEGF-R2 PerCP clone 89106 (RD, USA), VEGF-R3 APC clone 54733 (RD, USA), TGFβR2 cytokine receptors (RD, USA) Alexa Fluor 700, clone 25508 (RD, USA); HLA-DR phenotypic marker APC-H7 clone L243 (BD, USA) and CD105 APC clone 166707 (RD, USA) according to the manufacturer's instructions. The antibodies were combined into tree panels that we used from MV treatment. Each panel was used to treat 100 µL of MV suspension or 1 × 106 cells in 300 µL of Hanks' solution. The gating strategy used can be found in Figures S1 and S2. The experiments were repeated four times. The receptor expression was assessed using unstimulated THP-1 cells and MVs. Isotype controls (BD and RD, USA) and additionally cells and MVs not treated with antibodies were used. Flow cytometry was conducted using BD FACS Canto II flow cytometer as shown previously [41], which allows detecting particles greater than 0.2 µm in size. To adjust the device, we used additional protocols for cleaning the flowing liquid and sample washing buffers as well as calibration particles of 0.1, 0.2, 0.5 and 1.0 µm (Invitrogen, USA) in size according to the device manufacturer's instructions.
The method was reported previously by us [42]. Briefly, ECs were seeded into 96-well flat-bottom plate. After 24 hours, the medium was replaced with dilutions of MVs derived from unstimulated THP-1 cells prepared using the ECs medium containing 2.5% FCS (3.276 µg/100 µL, 1.638 µg/100 µL, 0.819 µg/100 µL and 0.410 µg/100 µL). After 24 hours, ECs were next stained with 0.2% crystal violet solution as reported previously [43]. Optical density was measured using Microplate Reader (Thermo Labsystems), and converted to the cell number. Changes in cell proliferation were assessed by comparing changes in sample optical density and the cell number with that of the ECs incubated in MV-free culture medium containing 2.5% FCS. When culturing ECs with 10% FCS (positive control), stimulation of ECs proliferative activity (17671 (13637, 34043) cells/well) was observed, compared with ECs culturing in medium with 2.5% FCS (10022 (9990, 10513) cells/well, p < 0.001). Experiments were carried out three times with each MV concentration analyzed in four replicates.
The method was reported previously by us [42]. Briefly, ECs were cultured for 24 hours in 96-well flat-bottom plate. The monolayer was then disrupted by partial cell scraping, the width of the obtained line was photographed (Figure 2).
Then, the medium was replaced with dilutions of MVs derived from THP-1 cells prepared using the EC medium containing 2.5% FCS (3.276 µg/100 µL, 1.638 µg/100 µL, 0.819 µg/100 µL and 0.410 µg/100 µL). After 24 hours, ECs were incubated with 100 µL of crystal violet solution (0.2%, Sigma-Aldrich Chem. Co., USA), washed and dried. Three fields of view were photographed in each well. Data analysis was performed using MarkMigration (Russia) software [44], which considers the residual area of the disrupted monolayer line after migration. Experiments determining EC migratory activity in the presence of MVs were performed three times. Each MV concentration was analyzed four times. Median area of the initial line after monolayer scraping was 0.88 mm2 (interquartile range (IQR), 0.88–0.89). No cells in the disrupted monolayer zone were detected. We noted an increase in the number (579 (504, 635), p < 0.001) of migrated ECs and a decrease in the area (0.2 (0.14, 0.25), p < 0.01) mm2 of the disrupted monolayer line after cell migration in the presence of 2.5% FCS. An increase in the FCS concentration in cell culture medium to 10% (positive control) caused an increase in the number of migrated ECs (657 (588, 762), p < 0.01) and a decrease in the residual area of the disrupted monolayer line after cell migration (0.12 (0.83, 0.18), p < 0.001) mm2. Thus, in the experimental model, EA.Hy926 cells responded to a higher FCS concentration with increased migratory activity, which is consistent with the results described previously [45],[46]. This allows the evaluation of changes in cell migratory activity in the presence of MVs derived from THP-1 cells.
Wells of a 96-well plate were pretreated with the Matrigel Growth Factors Reduced matrix (BD, USA) as we reported earlier [47]. Matrigel is a mixture of extracellular matrix proteins, and also contains minor levels of cytokines (TGFβ, EGF, IGF, bFGF, and PA) [48]. Then, EA.Hy926 ECs were added to the wells (150000 cells/mL in 200 µL of culture medium). MVs derived from THP-1 cells at different concentrations in 100 µL of culture medium were added to some of the wells (3.276 µg/100 µL, 1.638 µg/100 µL, 0.819 µg/100 µL and 0.410 µg/100 µL). The ECs culturing with 2.5% FCS determined baseline parameters. For use as positive controls, ECs were cultured with 20 ng/mL bFGF (RD, USA) or with 10% FCS that was free of MVs. The cells were then incubated for 24 hours. The experiments were performed four times with three replicates for each MV concentration. Data were recorded in all experiments using the AxioObserver.Z1 microscope (Zeiss, Germany) and the Axiovision image analysis software (Zeiss, Germany). In each well, one field of view was taken into consideration, in which length (in micrometers (µm)) and number of vessels were estimated using the ImagePro software.
We used the method described earlier [42]. Briefly, ECs were seeded into a 96-well plate (3.5 × 104 cells/well in 100 µL of medium) and cultured for 24 hours. To stain intracellular protein, THP-1 cells were treated with a carboxyfluorescein diacetate succinimidyl ester (CFSE, Sigma, USA) solution at concentration of 5 µM (n = 5) for use as positive controls. Some THP-1 cells were left unstimulated. Unstimulated and stained THP-1 cells were then cultured in 75 cm2 flasks (BD, USA) in 40 mL of complete RPMI1640 for 24 hours. The cell concentration was 1 × 106/mL. MVs were then isolated (as described above), added to ECs (3.276 µg of total protein in 100 µL of medium), and incubated for 24 hours. ECs were washed three times with Versene solution and removed from the plate surface. Then, ECs were resuspended twice in Hanks' solution without Ca2+ and Mg2+, and centrifuged at 200 g for 10 min. Fluorescent CFSE inclusions in ECs were evaluated using the FACS Canto II flow cytometer (Becton Dickinson, USA). The experiments were repeated five times.
The detailed method was described earlier [42]. Briefly, MVs derived from THP-1 cells were added to ECs at a total protein concentration of 3.276 µg in 100 µL of medium (in three repetitions). Unstimulated ECs were used as controls. ECs incubated with phorbol 12-myristate 13-acetate (10 ng/mL, Sigma, USA) were used as positive controls. The next day, ECs were washed, removed from the plate with Versene solution and stained with 7-AAD dye (Biolegend, USA). Cell death was assessed using the BD FACS Canto II flow cytometer by 7-AAD inclusion, as described [49],[50]. The median pool of nonviable ECs after culturing with MVs derived from THP-1 cells was 8.9% (IQR 4.5–9.5). Viability experiments were repeated four times. After incubation with MVs, ECs were treated with monoclonal antibodies against CD45 PerCP clone 2D1, CD54 APC clone HA58, CD29 PE clone MAR4, CD11a FITC clone HI111, CD11b PE clone ICRF44, and CD18 APC clone 6.7 (Becton Dickinson, USA), as well as with isotypic antibodies. Antibody selection was based on phenotyping results of ТНР-1 cells and their MVs. The fluorescence was analysed using the BD FACS Canto II cytometer. Analysis of the receptor expression in ECs was repeated four times.
Unstimulated THP-1 cells and their MVs were washed with cooled phosphate buffer (0.01 M PBS, pH 7.4) and then lysed in RIPA buffer 50 mM Tris-HCl, pH 8.1, Triton X-100 (1%), sodium deoxycholate (0.5%), sodium dodecyl sulfate (0.1%), sodium chloride (150 mM), and ethylenediaminetetraacetic acid (EDTA) (1 mM) containing a protease and phosphatase inhibitor mixture (Sigma, USA) with intermittent shaking for 30 min. Cell debris was removed by centrifugation (16000 g, 4 °C, 10 min). Proteins were normalized for cells and their MVs by 20 µg total protein per assay. Then they were separated by their molecular weight as we previously reported [42]. The proteins were then incubated with primary monoclonal antibodies to Erk1/2 (p44/42 MAPK (Erk1/2), rabbit Ab, 1:1000, Cell Signaling, MA, USA), Phospho-Erk1/2 (phospho-p44/42 MAPK) ((Thr202/Tyr204) (20G11), rabbit Ab, 1:1000, Cell Signaling, USA), Akt (Akt (pan)), (C67E7), rabbit Ab, 1:1000, Cell Signaling, MA, USA), Phospho-Akt ((Ser473) (193H12), rabbit Ab, 1:1000, Cell Signaling, MA, USA) or TGF-β (TGF-beta rabbit Ab 1:1000, Cell Signaling, MA, USA) at 4 °C for one night on MR-12 Rocker-Shaker (BioSan, Latvia). After reaction with an appropriate secondary antibody (1:1000; Cell Signaling Technology, MA, USA), signals were visualised on ChemiDoc™ Touch Gel Imaging System (Bio-Rad Laboratories, USA) using enhanced chemiluminescence (ECL) with ECL reagents (Bio-Rad Laboratories, USA). The results were evaluated using ImageLab software (Bio-Rad Laboratories, USA). Based on existing recommendations [51], the intensity of the bands of investigated proteins detected by the Western blotting was normalized by the total protein content in the sample, which was recorded with a gel using stain-free technology (Bio-Rad Laboratories, USA). Samples of three independent experiments (n = 3) were analysed to determine each protein.
The procedure was conducted as previously reported [42]. Briefly, the sediments containing cells and MVs were stored at –80 °C until assay. The cell membranes were disrupted with five freeze-thaw cycles and mechanical disruption, and the obtained lysates were then centrifuged (16000 g, 4 °C, 10 min). The obtained supernatants were discarded, and the sediment was dried at room temperature. Next, the dry residue was dissolved in a minimal amount of 0.1 M sodium bicarbonate (Sigma). After determining the total protein amount (as described in Section 2.4), the concentrations of obtained protein solutions were aligned, focusing on the lowest value of the measured protein amount. Purified proteins in obtained solutions were then separated in Agilent 2100 bioanalyzer (Agilent Technologies, USA). All experiments were repeated six times independently.
MV proteins were fractionated in a 10% polyacrylamide gel under Laemmli denaturing conditions at a load of 42 µg total protein. After electrophoretic separation of proteins, they were visualized by Coomassie G250 staining, then the bands on a gel corresponding to the protein bands were cut out in a number of 35 pieces. Next, the strips were crushed and washed three times to remove the dye and sodium dodecyl sulfate with a solution of 50% acetonitrile in 30 mM Tris (pH 8.2) for 15 min at room temperature. After removing the solution, the gel pieces washed from the dye were dehydrated in 100% acetonitrile. Then, after removing acetonitrile, the samples were dried for 40 min at 4 °C. Bovine trypsin solution (20 ng/mL; Promega, United States) was added to the dried samples and incubated for 1 hour on ice until the gel was completely rehydrated. After that, the excess trypsin was removed. 50 µL of 30 mM Tris (pH 8.2) were added to the samples and incubated for 16–18 hours at 37 °C. Tryptic peptide mixture was extracted three times from the gel with a 50% aqueous acetonitrile solution containing 0.1% formic acid (Sigma-Aldrich Chem. Co., USA) in an ultrasonic bath for 20 min. The peptides in the resulting solution were dried at 4 °C and frozen at −80 °C until further analysis.
After one-dimensional gel electrophoresis and subsequent sample preparation, the dried tryptic peptides were dissolved in 50 µL of a 50% aqueous solution of acetonitrile containing 0.1% trifluoroacetic acid (Sigma-Aldrich Chem. Co., USA), and stirred until complete dissolution. The solutions were applied to metal plates for MALDI-TOF mass spectrometry analysis as we previously reported [42]. Mass spectra of the tryptic peptides were obtained using the Axima Resonance MALDI-TOF mass spectrometer (Shimadzu/Kratos Analytical Ltd., UK). Protein identification was undertaken relative to the SwissProt and NCBI databases using taxonomic constraints for the Homo sapiens species, the Mascot software (www.matrixscience.com) and the peptide fingerprinting method. A parallel search was performed using a database of inverted and random (decoy) amino acid sequences. After peptide identification, the correspondence between an identified protein and its actual position on a gel was checked.
Statistical analysis was performed in Statistica 10 software. We used the nonparametric Mann–Whitney U test. Data were presented as a median (upper quartile, lower quartile). Results of western blot analysis and enzyme activity assessment were presented as a mean ± standard error of the mean (SEM) within at least three independent experiments. T-test for independent samples was used to analyze the experimental results. The value p < 0.05 was considered statistically significant.
We found that THP-1 cells carry on their surface CD54 [52],[53], CD18 [53], CD11b [52], CD11a [53],[54], CD29 adhesion molecules, CD120a (TNFαR1) [55], CD120b (TNFαR2) [56], VEGFR1 [57], CD105 [58], CD119 (IFNγR1) [59], TGFR2 [60] cytokine receptors, HLA-DRA [61] major histocompatibility complex (Figure 3). Expression of VEGF-R2 [57], CD11c [62], and VEGF-R3 [63] by THP-1 cells was very low. Our findings are consistent with published data (references are indicated above next to each receptor). Analysis of the expression of cell surface molecules on MVs produced by THP-1 cells showed that they expressed CD54, CD18, CD11a (Figure 3), CD11b, CD29 adhesion molecules, CD120a, VEGFR1, VEGFR2, CD105, CD119, TGFR2 cytokine receptors, as well as HLA-DR molecule.
We established that EA.Hy926 ECs that were incubated with MVs derived from THP-1 cells and pretreated with CFSE solution, included fluorescent CFSE (Figure 4).
Culturing of ECs with MVs derived from THP-1 cells did not affect the constitutive expression of CD29 and CD54 (Figure 5). After ECs culturing with MVs derived from THP-1 cells, CD45, CD11a, CD11b, and CD18 receptors were not transferred to ECs.
Culturing of ECs with MVs with a total protein content of 3.276 µg/100 µL, 1.368 µg/100 µL, and 0.819 µg/100 µL showed a decrease in EC proliferation compared with EC culturing in a medium without MVs (Figure 6).
ECs cultured with MVs derived from THP-1 cells (total protein content 3.276 µg/100 µL) decreased the migratory activity due to a lower number of migrated ECs compared with ECs cultured without MVs (Figure 7). ECs cultured with MVs derived from THP-1 cells (total protein content 3.276 µg/100 µL and 0.410 µg/100 µL) increased the migratory activity due to an increased number of migrated ECs and in parallel due to a decreased residual area after cell migration to the disrupted monolayer zone (Figure 7).
In the presence of bFGF, as well as in the presence of 10% FCS, the length of vessels was greater compared to ECs culturing with 2.5% FCS. Culturing of ECs with bFGF lowered the number of vessels, compared to ECs culturing with 2.5% FCS, while culturing in a medium supplemented with 10% FCS did not change their number (Figures 8 and 9). MVs derived from THP-1 cells, at a concentration of 3.276 µg per 100 µL increased the length, and at a concentration of 1.638 µg per 100 µL, on the contrary, decreased the length of vessels, as compared with ECs culturing with 2.5% FCS. The length of vessels formed by ECs in the presence of MVs at a concentration of 1.638 µg per 100 µL was also shorter compared to the length in the presence of MVs at a concentration of 3.276 µg per 100 µL. The number of vessels in the presence of MVs at a concentration of 3.276 µg per 100 µL was lower, and in the presence of MVs at a concentration of 1.638 µg per 100 µL was higher compared to ECs culturing with 2.5% FCS.
Protein extraction from lysates with microelectrophoresis revealed that 17 and 5 major (>3% of the total intensity) protein groups were released in THP-1 cells and in their MVs, respectively (Figure 10, Table 1). The group with a mean molecular weight of 60.5 ± 0.27 kDa is common for cells (4.4 ± 0.46%) and their MVs (62.0 ± 2.85%). The groups with the following masses also predominated in cells: 42.7 ± 0.28 kDa (22.6 ± 2.25%); 12.8 ± 0.09 kDa (11.3 ± 1.41%); 11.9 ± 0.04 kDa (7.2 ± 0.85%); 28.4 ± 0.19 kDa (6.6 ± 0.27%) and 66.6 ± 0.45 kDa (6.8 ± 0.62%). Protein groups with masses of 98.0±1.03 kDa (12.1 ± 0.87%) and 84.7 ± 0.17 kDa (6.9 ± 0.44%) predominated in MVs. Thus, protein profiles of THP-1 cells and their MVs differed: cells contained a greater variety of proteins than MVs did, while proteins with a higher molecular weight predominated in MVs compared to that in THP-1 cells.
No. | Molecular weight, kDa | Pool, % | |
Cells, n = 6 | 1 | 11.2 ± 0.05 | 4.1 ± 0.31 |
2 | 11.9 ± 0.04 | 7.2 ± 0.85 | |
3 | 12.8 ± 0.09 | 11.3 ± 1.41 | |
4 | 14.5 ± 0.06 | 5.6 ± 0.36 | |
5 | 18.1 ± 0.01 | 5.0 ± 0.46 | |
6 | 20.1 ± 0.07 | 3.9 ± 0.18 | |
7 | 23.0 ± 0.18 | 3.5 ± 0.23 | |
8 | 24.9 ± 0.14 | 4.2 ± 0.53 | |
9 | 28.4 ± 0.19 | 6.6 ± 0.27 | |
10 | 34.4 ± 0.20 | 5.6 ± 0.67 | |
11 | 42.7 ± 0.28 | 22.6 ± 2.25 | |
12 | 50.7 ± 0.27 | 3.6 ± 0.18 | |
13 | 54.7 ± 0.07 | 5.1 ± 0.77 | |
14 | 57.0 ± 0.35 | 4.5 ± 1.74 | |
15 | 59.9 ± 0.33 | 4.4 ± 0.46 | |
16 | 66.6 ± 0.45 | 6.8 ± 0.62 | |
17 | 83.2 ± 0.31 | 3.6 ± 0.46 | |
MVs, n = 5 | 1 | 61.1 ± 0.45 | 62.0 ± 2.85 |
2 | 84.7 ± 0.17 | 6.9 ± 0.44 | |
3 | 98.0 ± 1.03 | 12.1 ± 0.87 | |
4 | 120.7 ± 0.67 | 5.8 ± 2.02 | |
5 | 138.2 ± 0.68 | 6.6 ± 1.83 |
It was shown that apart from other proteins identified, MVs derived from THP-1 cells presumably contained fibroblast growth factor (fibroblast growth factor 10, encoded by the FGF10 gene; SwissProt entry O15520, MW 23.4 kDa, pI 9.61, 7 tryptic peptides, overlapping 9% of the amino acid sequence of the protein) and endothelin precursor (endothelin-2 isoform 2 preproprotein, encoded by the EDN2 gene; NCBI entry NP_001289198, MW 16.5 kDa, pI 10.19, 7 tryptic peptides, overlapping 11% of the amino acid sequence of the protein). These two proteins were recognized as associated with angiogenesis. The other proteins identified included cytoskeleton proteins, protein biosynthesis enzymes, energy metabolism enzymes, etc.
THP-1 cells and their MVs contained ERK1/2, Akt, and TGFβ. Regarding the total protein in MVs and THP-1 cells, respectively, the total ERK1/2 kinase content in MB was 6.4 times less than its content in THP-1 cells. However, it was shown that a significant amount of phosphorylated form of ERK1/2 (pERK1/2) was present in MVs produced by THP-1 cells, compared to THP-1 cells. It was shown that MVs contained protein kinase Akt in the amount comparable to that in THP-1 cells, while there were no endogenous levels of Akt phosphorylated by Ser473 revealed (Figure 11).
The interaction of monocytes\macrophages with ECs determines the course of many biological processes, such as inflammation, angiogenesis, carcinogenesis, atherogenesis [64]–[67]. The possibility of interaction of these cells using MVs is of particular interest. Therefore, we attempted to describe this interaction. Monocytic leukemia THP-1 cells were selected as source cells of MVs, and EA.Hy926 ECs were selected as target cells.
Comparison of the phenotype of monocyte leukemia THP-1 cells and their MVs indicated a coincidence of profiles of the molecules they express (Figure 3). The presence of CD54, CD18, CD11a, CD11b, and CD29 adhesion receptors on the MVs surface may determine their further interaction with target cells [68]–[70].
Due to the expression of ligands for these molecules [71], ECs can be targets for MVs derived from monocytes/macrophages. The presence of CD120a (TNFαR1), CD120b (TNFαR2), VEGFR1, VEGFR2, CD105, CD119 (IFNγR1), TGFR2 receptors on MVs derived from THP-1 cells can be a trap for the corresponding cytokines similar to soluble receptors capable of binding cytokines and canceling their effect on target cells [72]. On the other hand, being part of MV membrane, these receptors may be shed from monocytes/macrophages, thus decreasing the sensitivity of cells to the corresponding cytokines.
Currently, there are literature data describing several potential ways for MVs to interact with target cells: simple membrane fusion, clathrin- and caveolae-mediated endocytosis, lipid raft-dependent endocytosis, phagocytosis, micropinocytosis, destruction of MVs near target cells followed by the effect of the contents on target cells via surface receptors [12],[73]–[75]. The pathway of MVs internalization in target cells depends largely on which proteins and glycoproteins are expressed both on MVs themselves and on recipient cells [76]. We established the transfer of an intracellular protein labeled with a fluorescent dye from MVs produced by THP-1 cells to EA.Hy926 ECs (Figure 4). This coincides with previously obtained data on the protein transfer by MVs derived from natural killer cells to ECs [42]. Despite this, we were not able to detect the transfer of CD45, CD11a, CD11b, and CD18 receptors to the endothelium by MVs derived from THP-1 cells. In a similar model, the transfer of CD45 receptors by MVs derived from natural killer cells to ECs was previously established [42]. In the case of MVs produced by THP-1 cells, the absence of transfer of surface receptors to the endothelium can be explained by their low concentration on the MVs membrane or by differences in the uptake mechanisms of MVs derived from monocytes\macrophages and natural killer cells by ECs, which requires additional study. Surface receptor transfer from MVs towards target cells can also be influenced by presence or absence of activation triggers for MV source cells. For example, THP-1 cells cultured in the presence of LPS produced MVs, which influenced adhesion receptor expression by ECs [27]. The amount of CD54+ MVs obtained from LPS-stimulated monocytes differed from MVs of unstimulated cells [77]. Further experiments are needed to explore the impact of inducers on MV receptor transfer to recipient cells.
We established the fundamental possibility of the influence of MVs produced by THP-1 cells on the processes of angiogenesis. Thus, MV derived from THP-1 cells inhibited ECs proliferation. In high concentrations, MVs reduced ECs migration, increased the length but decreased the number of vessels, promoting the development of non-branching angiogenesis. On the contrary, in low concentrations, MVs increased ECs migration, reduced the length, and increased the number of vessels, promoting the development of branching angiogenesis. This opposite effect of different MV concentrations on the type of angiogenesis may be associated with several factors. On the one hand, an increased number of MVs with VEGFR1 and VEGFR2 receptors may promote binding of VEGF [78] from the medium, which is produced by ECs themselves [79]. A decreased concentration of free VEGF can help reduce the vascular branching [80],[81]. An increased content of MVs carrying CD105 on their surface can affect ECs in the same way as the secretory form of this receptor, sCD105 (Endoglin), does. In the literature, we found no data on the difference in effects of these forms of CD105 on ECs.
However, Endoglin was found to dose-dependently inhibit EC migration and the branching pathway of angiogenesis [82]. Using Western Blot Analysis, we established the presence of TGFβ in MVs produced by THP-1 cells. With an increased MV content, the probability of TGFβ destruction near target cells also increased. In our model, this may contribute to a local increase in the TGFβ content near ECs. Previously, TGFβ was found to inhibit EC proliferation and migration [83], promote vascular stabilization, inhibit vascular branching in the absence of VEGF [84], and suppress FGF signaling in ECs. On the other hand, in our model, a decreased number of MVs derived from THP-1 cells may reduce VEGF binding, reduce the effect of Endoglin and change the effect of TGFβ on ECs, and bring to the fore the effect of substances contained in MVs.
Comparison of proteomic profiles of THP-1 cells and their MVs showed a significant difference in their proteomic profiles. Using Western Blot Analysis, we established the presence of ERK1/2 and its phosphorylated form (pERK1/2), unphosphorylated Akt, in MVs produced by THP-1 cells. Using MALDI-TOF mass spectrometry, we found that MVs derived from THP-1 cells contained fibroblast growth factor 10 (FGF10) and endothelin precursor (ET)-2 (endothelin-2 isoform 2 preprotein).
Endothelin-2 found in MVs produced by THP-1 cells, as well as ET-1, acted on ECs via ETA and ETB receptors [85]. Activation of these receptors stimulated endothelium NO synthase-mediated NO production by ECs as well as prostacyclin synthesis [86]. ET-1 has been shown to directly promote tumor angiogenesis by inducing EC survival, proliferation, and invasion in an ETBR-dependent manner [87]. In this context, monocytes\macrophages, due to the production of MVs with ET, can act as regulators of physiological and pathological angiogenesis [87].
Previously, FGF10 was found to enhance the stimulating effect of VEGF on EC proliferation [88]. In lung development, FGF10 enhances airway branching via ERK1/2 and mTORC1 [89]. The receptors for FGF10 are FGFR1b and FGFR2b [90], which are expressed on ECs [91],[92]. It is possible that FGF10 is able [93] to stimulate branching angiogenesis by acting on ECs via these receptors in the same way as bFGF [94]. Despite the fact that FGF10 and bFGF can affect ECs via the same receptors, no direct evidence has been found in the literature in favor of the effect of FGF10 on branching angiogenesis. FGF signals through the RAS-MAPK and PI3K-AKT-GSK3β signaling pathways. FGF10 signaling through FGFR2b to SRC induce F-actin binding protein phosphorylation as well as cortactin phosphorylation, which participates in endocytosis and clathrin-dependent internalization [95]. FGF10 signaling stimulates the polarization of FGFR2b to the leading edge for the regulation of cellular migration [95]. A triple membrane signaling pathway has been described, in which stimulation of FGFR2b or VEGFR2 receptor tyrosine kinases activates ADAM17 followed by HB-EGF release, EGFR activation, and ERK1/2 activation. The result of this signal is stimulation of EC migration [91]. Thus, the observed effects of migration, stimulation, and activation of branching angiogenesis in the presence of small numbers of MVs may be the result of a predominant effect of FGF10 and pERK1/2 localized in MVs on ECs. Despite the presence of TGFβ in MVs and its ability to inhibit branching angiogenesis in the absence of VEGF [84], its ability to stimulate this type of angiogenesis has been established in certain cases. It was found that TGFβ stimulated vascular branching in the conditioned medium of glial cells [96]. Low extracellular TGFβ levels also promote EC proliferation and migration and new blood vessel formation [84]. High TGFβ concentrations induce EC differentiation, tube formation reduction, and impaired invasion in artificial matrix. Previously it was shown that TGFβ similarly to VEGF is able to induce angiogenesis and that VEGF can be a target for TGFβ [84]. TGFβ is known to crosstalk between different signaling pathways and to promote expression of other factors that affect angiogenesis, e.g., VEGF [97], FGF [98]. In this context, a decrease in the concentration of MVs in the EC microenvironment may lead to a decrease in the release of TGFβ, which, under conditions of a decreased VEGF deprivation by VEGFR receptors on MVs and in the presence of FGF10, may stimulate branching angiogenesis.
Thus, MVs derived from monocytes\macrophages have the ability to regulate angiogenesis, i.e., affect proliferation, migration, determine the method of vascular formation, and stimulate branching or non-branching angiogenesis. MVs produced by THP-1 cells are able to interact with ECs and transfer protein into them due to the receptor expression on the membrane. CD105, TGFβ, pERK1/2, FGF10, and preproprotein endothelin-2, which are potentially capable of changing the behavior of ECs in the manner we discovered, and correcting angiogenesis, were found in the composition of MVs derived from THP-1 cells.
[1] |
Surveillances V (2020) The epidemiological characteristics of an outbreak of 2019 novel coronavirus diseases (COVID-19)—China, 2020. CCDC Weekly 2: 113-122. doi: 10.46234/ccdcw2020.032
![]() |
[2] |
Bai Y, Yao L, Wei T, et al. (2020) Presumed asymptomatic carrier transmission of COVID-19. Jama 323: 1406-1407. doi: 10.1001/jama.2020.2565
![]() |
[3] | Dehghanbanadaki H, Seif F, Vahidi Y, et al. (2020) Bibliometric analysis of global scientific research on coronavirus (COVID-19). MJIRI 34: 354-362. |
[4] |
Wang S, Zhou X, Zhang T, et al. (2020) The need for urogenital tract monitoring in COVID-19. Nat Rev Urol 17: 314-315. doi: 10.1038/s41585-020-0319-7
![]() |
[5] |
Rothan HA, Byrareddy SN (2020) The epidemiology and pathogenesis of coronavirus disease (COVID-19) outbreak. J Autoimmun 109: 102433. doi: 10.1016/j.jaut.2020.102433
![]() |
[6] |
Seif F, Aazami H, Khoshmirsafa M, et al. (2020) JAK inhibition as a new treatment strategy for patients with COVID-19. Int Arch Allergy Imm 181: 467-475. doi: 10.1159/000508247
![]() |
[7] | Zhang H, Kang Z, Gong H, et al. (2020) The digestive system is a potential route of 2019-nCov infection: a bioinformatics analysis based on single-cell transcriptomes. BioRxiv In press. |
[8] |
Chen Y, Chen L, Deng Q, et al. (2020) The presence of SARS-CoV-2 RNA in the feces of COVID-19 patients. J Med Virol 92: 833-840. doi: 10.1002/jmv.25825
![]() |
[9] | Backer JA, Klinkenberg D, Wallinga J (2020) The incubation period of 2019-nCoV infections among travellers from Wuhan, China. MedRxiv In press. |
[10] |
Lei J, Li J, Li X, et al. (2020) CT imaging of the 2019 novel coronavirus (2019-nCoV) pneumonia. Radiology 295: 18. doi: 10.1148/radiol.2020200236
![]() |
[11] |
Li Q, Guan X, Wu P, et al. (2020) Early transmission dynamics in Wuhan, China, of novel coronavirus—infected pneumonia. N Engl J Med 382: 1199-1207. doi: 10.1056/NEJMoa2001316
![]() |
[12] |
Adhikari SP, Meng S, Wu YJ, et al. (2020) Epidemiology, causes, clinical manifestation and diagnosis, prevention and control of coronavirus disease (COVID-19) during the early outbreak period: a scoping review. Infect Dis Poverty 9: 1-12. doi: 10.1186/s40249-020-00646-x
![]() |
[13] |
Guan CS, Lv ZB, Yan S, et al. (2020) Imaging features of coronavirus disease 2019 (COVID-19): evaluation on thin-section CT. Acad Radiol 27: 609-613. doi: 10.1016/j.acra.2020.03.002
![]() |
[14] | Centers for Disease Control and Prevention, Management of Patients with Confirmed 2019-nCoV, 2020. National Center for Immunization and Respiratory Diseases (NCIRD), Division of Viral Diseases, 2020 Available from: https://www.cdc.gov/coronavirus/2019-ncov/hcp/clinical-guidance-management-patients.html. |
[15] |
Ai T, Yang Z, Hou H, et al. (2020) Correlation of chest CT and RT-PCR testing in coronavirus disease 2019 (COVID-19) in China: a report of 1014 cases. Radiology 296: E32-E40. doi: 10.1148/radiol.2020200642
![]() |
[16] |
Denison MR, Graham RL, Donaldson EF, et al. (2011) Coronaviruses: an RNA proofreading machine regulates replication fidelity and diversity. RNA Biol 8: 270-279. doi: 10.4161/rna.8.2.15013
![]() |
[17] |
Zhu N, Zhang D, Wang W, et al. (2020) A novel coronavirus from patients with pneumonia in China, 2019. N Engl J Med 382: 727-733. doi: 10.1056/NEJMoa2001017
![]() |
[18] | Zhang Y (2020) Novel 2019 coronavirus genome Shanghai Public Health Clinical Center & School of Public Health, Fudan University, Available from: https://virological.org/t/novel-2019-coronavirus-genome/319. |
[19] | Corman VM, Landt O, Kaiser M, et al. (2020) Detection of 2019 novel coronavirus (2019-nCoV) by real-time RT-PCR. Eurosurveillance 25: 2000045. |
[20] |
Chan JFW, Kok KH, Zhu Z, et al. (2020) Genomic characterization of the 2019 novel human-pathogenic coronavirus isolated from a patient with atypical pneumonia after visiting Wuhan. Emerg Microbes Infect 9: 221-236. doi: 10.1080/22221751.2020.1719902
![]() |
[21] |
Kim H (2020) Outbreak of novel coronavirus (COVID-19): What is the role of radiologists? Eur Radiol 30: 3266-3267. doi: 10.1007/s00330-020-06748-2
![]() |
[22] | Ora J, Puxeddu E, Cavalli F, et al. (2020) Does bronchoscopy help the diagnosis in Covid-19 infection? Eur Respir J In press. |
[23] | Tan FR, Qiu YL, Xu Z (2020) Bronchoalveolar lavage fluid was used to diagnose two cases of 2019-nCoV infection. Chin J Tuberc Respir Dis 43: 337-339. |
[24] |
Winichakoon P, Chaiwarith R, Liwsrisakun C, et al. (2020) Negative nasopharyngeal and oropharyngeal swabs do not rule out COVID-19. J Clin Microbiol 58: e00297-20. doi: 10.1128/JCM.00297-20
![]() |
[25] |
He JL, Luo L, Luo ZD, et al. (2020) Diagnostic performance between CT and initial real-time RT-PCR for clinically suspected 2019 coronavirus disease (COVID-19) patients outside Wuhan, China. Respir Med 168: 105980. doi: 10.1016/j.rmed.2020.105980
![]() |
[26] |
Sethuraman N, Jeremiah SS, Ryo A (2020) Interpreting diagnostic tests for SARS-CoV-2. Jama 323: 2249-2251. doi: 10.1001/jama.2020.8259
![]() |
[27] |
Dai W, Zhang H, Yu J, et al. (2020) CT imaging and differential diagnosis of COVID-19. Can Assoc Radiol J 71: 195-200. doi: 10.1177/0846537120913033
![]() |
[28] |
Fang Y, Zhang H, Xie J, et al. (2020) Sensitivity of chest CT for COVID-19: comparison to RT-PCR. Radiology 296: E115-E117. doi: 10.1148/radiol.2020200432
![]() |
[29] |
Chung M, Bernheim A, Mei X, et al. (2020) CT imaging features of 2019 novel coronavirus (2019-nCoV). Radiology 295: 202-207. doi: 10.1148/radiol.2020200230
![]() |
[30] |
Bernheim A, Mei X, Huang M, et al. (2020) Chest CT findings in coronavirus disease-19 (COVID-19): relationship to duration of infection. Radiology 295: 685-691. doi: 10.1148/radiol.2020200463
![]() |
[31] |
Xie X, Zhong Z, Zhao W, et al. (2020) Chest CT for typical 2019-nCoV pneumonia: relationship to negative RT-PCR testing. Radiology 296: E41-E45. doi: 10.1148/radiol.2020200343
![]() |
[32] | Chana JFW, Yip CCY, To KKW, et al. (2020) Improved molecular diagnosis of COVID-19 by the novel, highly sensitive and specific COVID-19-RdRp/Hel realtime reverse transcription-polymerase chain reaction assay validated in vitro and with clinical specimens. J Clin Microbiol 10: 00310-20. |
[33] |
Tavare AN, Braddy A, Brill S, et al. (2020) Managing high clinical suspicion COVID-19 inpatients with negative RT-PCR: a pragmatic and limited role for thoracic CT. Thorax 75: 531. doi: 10.1136/thoraxjnl-2020-214916
![]() |
[34] |
Houben PHH, Winkens RAG, Van der Weijden T, et al. (2010) Reasons for ordering laboratory tests and relationship with frequency of abnormal results. Scand J Prim Health Care 28: 18-23. doi: 10.3109/02813430903281758
![]() |
[35] | Sikaris KA (2017) Enhancing the clinical value of medical laboratory testing. Clin Biochem Rev 38: 107. |
[36] |
Singhal T (2020) A review of coronavirus disease-2019 (COVID-19). Indian J Pediatr 87: 281-286. doi: 10.1007/s12098-020-03263-6
![]() |
[37] |
Chen N, Zhou M, Dong X, et al. (2020) Epidemiological and clinical characteristics of 99 cases of 2019 novel coronavirus pneumonia in Wuhan, China: a descriptive study. Lancet 395: 507-513. doi: 10.1016/S0140-6736(20)30211-7
![]() |
[38] |
Wu J, Liu J, Zhao X, et al. (2020) Clinical characteristics of imported cases of COVID-19 in Jiangsu province: a multicenter descriptive study. Clin Infect Dis 71: 706-712. doi: 10.1093/cid/ciaa199
![]() |
[39] |
Yang S, Shi Y, Lu H, et al. (2020) Clinical and CT features of early stage patients with COVID-19: a retrospective analysis of imported cases in Shanghai, China. Eur Respir J 55: 2000407. doi: 10.1183/13993003.00407-2020
![]() |
[40] |
Zhang J, Dong X, Cao Y, et al. (2020) Clinical characteristics of 140 patients infected with SARS-CoV-2 in Wuhan, China. Allergy 75: 1730-1741. doi: 10.1111/all.14238
![]() |
[41] |
Shi H, Han X, Jiang N, et al. (2020) Radiological findings from 81 patients with COVID-19 pneumonia in Wuhan, China: a descriptive study. Lancet Infect Dis 20: 425-434. doi: 10.1016/S1473-3099(20)30086-4
![]() |
[42] |
Song F, Shi N, Shan F, et al. (2020) Emerging 2019 novel coronavirus (2019-nCoV) pneumonia. Radiology 295: 210-217. doi: 10.1148/radiol.2020200274
![]() |
[43] |
Zhang R, Wang X, Ni L, et al. (2020) COVID-19: Melatonin as a potential adjuvant treatment. Life Sci 250: 117583. doi: 10.1016/j.lfs.2020.117583
![]() |
[44] | Bahrami M, Kamalinejad M, Latifi SA, et al. (2020) Cytokine storm in COVID-19 and parthenolide: preclinical evidence. Phytother Res In press. |
[45] |
Emameh RZ, Nosrati H, Eftekhari M, et al. (2020) Expansion of Single Cell Transcriptomics Data of SARS-CoV Infection in Human Bronchial Epithelial Cells to COVID-19. Biol Proced Online 22: 16. doi: 10.1186/s12575-020-00127-3
![]() |
[46] | Roshanravan N, Seif F, Ostadrahimi A, et al. (2020) Targeting Cytokine Storm to Manage Patients with COVID-19: A Mini-Review. Arch Med Res In press. |
[47] |
Vashist SK (2020) In vitro diagnostic assays for COVID-19: recent advances and emerging trends. Diagnostics 10: 202. doi: 10.3390/diagnostics10040202
![]() |
[48] |
Xiao AT, Gao C, Zhang S (2020) Profile of specific antibodies to SARS-CoV-2: the first report. J Infect 81: 147-178. doi: 10.1016/j.jinf.2020.03.012
![]() |
[49] | Lou B, Li T, Zheng S, et al. (2020) Serology characteristics of SARS-CoV-2 infection since the exposure and post symptoms onset. Eur Respir J In press. |
[50] | Xiang F, Wang X, He X, et al. (2020) Antibody detection and dynamic characteristics in patients with COVID-19. Clin Infect Dis In press. |
[51] |
Zhang W, Du R H, Li B, et al. (2020) Molecular and serological investigation of 2019-nCoV infected patients: implication of multiple shedding routes. Emerg Microbes Infect 9: 386-389. doi: 10.1080/22221751.2020.1729071
![]() |
[52] |
Patel R, Babady E, Theel ES, et al. (2020) Report from the American Society for Microbiology COVID-19 International Summit, 23 March 2020: Value of diagnostic testing for SARS–CoV-2/COVID-19. mBio 11: e00722-20. doi: 10.1128/mBio.00722-20
![]() |
[53] | Rajendran K, Narayanasamy K, Rangarajan J, et al. (2020) Convalescent plasma transfusion for the treatment of COVID-19: Systematic review. J Med Virol 2020: 25961. |
[54] |
Azkur AK, Akdis M, Azkur D, et al. (2020) Immune response to SARS-CoV-2 and mechanisms of immunopathological changes in COVID-19. Allergy 75: 1564-1581. doi: 10.1111/all.14364
![]() |
[55] |
Mo H, Zeng G, Ren X, et al. (2006) Longitudinal profile of antibodies against SARS-coronavirus in SARS patients and their clinical significance. Respirology 11: 49-53. doi: 10.1111/j.1440-1843.2006.00783.x
![]() |
[56] | Shi YL, Li LH, Sun ZH, et al. (2010) Study on the changing regularity of special antibody and expression of stomach and enteric involvement on SARS-coronavirus infection in the recovery period of severe acute respiratory syndrome. Chin J Epidemiol 31: 795-799. |
[57] |
Chen H, Guo J, Wang C, et al. (2020) Clinical characteristics and intrauterine vertical transmission potential of COVID-19 infection in nine pregnant women: a retrospective review of medical records. Lancet 395: 809-815. doi: 10.1016/S0140-6736(20)30360-3
![]() |
[58] | Simpson S, Kay FU, Abbara S, et al. (2020) Radiological Society of North America Expert Consensus Statement on reporting chest CT findings related to COVID-19. Endorsed by the Society of Thoracic Radiology, the American College of Radiology, and RSNA. J Thorac Imaging 2: e200152. |
[59] |
Zhou S, Wang Y, Zhu T, et al. (2020) CT features of coronavirus disease 2019 (COVID-19) pneumonia in 62 patients in Wuhan, China. Am J Roentgenol 214: 1287-1294. doi: 10.2214/AJR.20.22975
![]() |
[60] |
Yang W, Cao Q, Qin L, et al. (2020) Clinical characteristics and imaging manifestations of the 2019 novel coronavirus disease (COVID-19): A multi-center study in Wenzhou city, Zhejiang, China. J Infect 80: 388-393. doi: 10.1016/j.jinf.2020.02.016
![]() |
[61] |
Pan F, Ye T, Sun P, et al. (2020) Time course of lung changes on chest CT during recovery from 2019 novel coronavirus (COVID-19) pneumonia. Radiology 295: 715-721. doi: 10.1148/radiol.2020200370
![]() |
[62] | Jin YH, Cai L, Cheng ZS, et al. (2020) A rapid advice guideline for the diagnosis and treatment of 2019 novel coronavirus (2019-nCoV) infected pneumonia (standard version). Mil Med Res 7: 4. |
[63] | Kalra MK, Homayounieh F, Arru C, et al. (2020) Chest CT practice and protocols for COVID-19 from radiation dose management perspective. Eur Radiol In press. |
[64] | Hoffman T, Nissen K, Krambrich J, et al. (2020) Evaluation of a COVID-19 IgM and IgG rapid test; an efficient tool for assessment of past exposure to SARS-CoV-2. Infect Ecol Epidemiol 10: 1754538. |
[65] | To KKW, Tsang OTY, Leung WS, et al. (2020) Temporal profiles of viral load in posterior oropharyngeal saliva samples and serum antibody responses during infection by SARS-CoV-2: an observational cohort study. Lancet Infect Dis 20: 567-574. |
[66] | Zhao R, Li M, Song H, et al. (2020) Serological diagnostic kit of SARS-CoV-2 antibodies using CHO-expressed full-length SARS-CoV-2 S1 proteins. MedRxiv In press. |
[67] |
Kim H, Hong H, Yoon SH (2020) Diagnostic performance of CT and reverse transcriptase-polymerase chain reaction for coronavirus disease 2019: a meta-analysis. Radiology 296: E145-E144. doi: 10.1148/radiol.2020201343
![]() |
[68] |
Kasteren PBV, Veer BVD, Brink SVD, et al. (2020) Comparison of seven commercial RT-PCR diagnostic kits for COVID-19. J Clin Virol 128: 104412. doi: 10.1016/j.jcv.2020.104412
![]() |
1. | Benjamin Wacker, Revisiting the classical target cell limited dynamical within-host HIV model - Basic mathematical properties and stability analysis, 2024, 21, 1551-0018, 7805, 10.3934/mbe.2024343 |
No. | Molecular weight, kDa | Pool, % | |
Cells, n = 6 | 1 | 11.2 ± 0.05 | 4.1 ± 0.31 |
2 | 11.9 ± 0.04 | 7.2 ± 0.85 | |
3 | 12.8 ± 0.09 | 11.3 ± 1.41 | |
4 | 14.5 ± 0.06 | 5.6 ± 0.36 | |
5 | 18.1 ± 0.01 | 5.0 ± 0.46 | |
6 | 20.1 ± 0.07 | 3.9 ± 0.18 | |
7 | 23.0 ± 0.18 | 3.5 ± 0.23 | |
8 | 24.9 ± 0.14 | 4.2 ± 0.53 | |
9 | 28.4 ± 0.19 | 6.6 ± 0.27 | |
10 | 34.4 ± 0.20 | 5.6 ± 0.67 | |
11 | 42.7 ± 0.28 | 22.6 ± 2.25 | |
12 | 50.7 ± 0.27 | 3.6 ± 0.18 | |
13 | 54.7 ± 0.07 | 5.1 ± 0.77 | |
14 | 57.0 ± 0.35 | 4.5 ± 1.74 | |
15 | 59.9 ± 0.33 | 4.4 ± 0.46 | |
16 | 66.6 ± 0.45 | 6.8 ± 0.62 | |
17 | 83.2 ± 0.31 | 3.6 ± 0.46 | |
MVs, n = 5 | 1 | 61.1 ± 0.45 | 62.0 ± 2.85 |
2 | 84.7 ± 0.17 | 6.9 ± 0.44 | |
3 | 98.0 ± 1.03 | 12.1 ± 0.87 | |
4 | 120.7 ± 0.67 | 5.8 ± 2.02 | |
5 | 138.2 ± 0.68 | 6.6 ± 1.83 |
No. | Molecular weight, kDa | Pool, % | |
Cells, n = 6 | 1 | 11.2 ± 0.05 | 4.1 ± 0.31 |
2 | 11.9 ± 0.04 | 7.2 ± 0.85 | |
3 | 12.8 ± 0.09 | 11.3 ± 1.41 | |
4 | 14.5 ± 0.06 | 5.6 ± 0.36 | |
5 | 18.1 ± 0.01 | 5.0 ± 0.46 | |
6 | 20.1 ± 0.07 | 3.9 ± 0.18 | |
7 | 23.0 ± 0.18 | 3.5 ± 0.23 | |
8 | 24.9 ± 0.14 | 4.2 ± 0.53 | |
9 | 28.4 ± 0.19 | 6.6 ± 0.27 | |
10 | 34.4 ± 0.20 | 5.6 ± 0.67 | |
11 | 42.7 ± 0.28 | 22.6 ± 2.25 | |
12 | 50.7 ± 0.27 | 3.6 ± 0.18 | |
13 | 54.7 ± 0.07 | 5.1 ± 0.77 | |
14 | 57.0 ± 0.35 | 4.5 ± 1.74 | |
15 | 59.9 ± 0.33 | 4.4 ± 0.46 | |
16 | 66.6 ± 0.45 | 6.8 ± 0.62 | |
17 | 83.2 ± 0.31 | 3.6 ± 0.46 | |
MVs, n = 5 | 1 | 61.1 ± 0.45 | 62.0 ± 2.85 |
2 | 84.7 ± 0.17 | 6.9 ± 0.44 | |
3 | 98.0 ± 1.03 | 12.1 ± 0.87 | |
4 | 120.7 ± 0.67 | 5.8 ± 2.02 | |
5 | 138.2 ± 0.68 | 6.6 ± 1.83 |