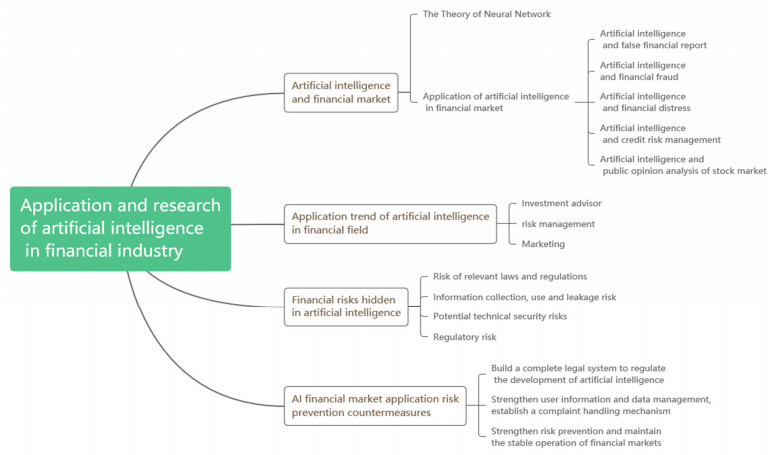
Artificial Intelligence (AI) is deemed to be the commanding point of science and technology in the next era. In recent years, with the enhancement of computer computing power, the improvement of the quantity and quality of big data, and the important breakthroughs in many research fields such as machine learning and speech recognition, AI technology has developed rapidly and has been widely used in all walks of life. In the financial industry, the application of AI technology in risk control, marketing, customer service, transaction, operation, and product optimization of financial institutions is becoming increasingly mature, and some new business models have been created. Starting from the application status and significance of AI in the international financial field, this paper expounds on the application, status quo, and development trend of AI in the financial industry. Then, in view of the risks and practical challenges existing in the development process of AI, based on the reality of international financial development, this paper summarizes the measures to promote the in-depth, healthy, and sustainable development of AI in the financial market. This paper aims to let readers understand the development status of AI in the financial field, and also provide theoretical reference for scholars in this field.
Citation: Yuxin Li, Jizheng Yi, Huanyu Chen, Duanxiang Peng. Theory and application of artificial intelligence in financial industry[J]. Data Science in Finance and Economics, 2021, 1(2): 96-116. doi: 10.3934/DSFE.2021006
[1] | Yue Yuin Lim, Sie Long Kek, Kok Lay Teo . Efficient state estimation strategies for stochastic optimal control of financial risk problems. Data Science in Finance and Economics, 2022, 2(4): 356-370. doi: 10.3934/DSFE.2022018 |
[2] | Katleho Makatjane, Ntebogang Moroke . Examining stylized facts and trends of FTSE/JSE TOP40: a parametric and Non-Parametric approach. Data Science in Finance and Economics, 2022, 2(3): 294-320. doi: 10.3934/DSFE.2022015 |
[3] | Dominic Joseph . Estimating credit default probabilities using stochastic optimisation. Data Science in Finance and Economics, 2021, 1(3): 253-271. doi: 10.3934/DSFE.2021014 |
[4] | Haihua Liu, Shan Huang, Peng Wang, Zejun Li . A review of data mining methods in financial markets. Data Science in Finance and Economics, 2021, 1(4): 362-392. doi: 10.3934/DSFE.2021020 |
[5] | Eid Elghaly Hassan, Diping Zhang . The usage of logistic regression and artificial neural networks for evaluation and predicting property-liability insurers' solvency in Egypt. Data Science in Finance and Economics, 2021, 1(3): 215-234. doi: 10.3934/DSFE.2021012 |
[6] | Kuo-Shing Chen . Interlinkages between Bitcoin, green financial assets, oil, and emerging stock markets. Data Science in Finance and Economics, 2024, 4(1): 160-187. doi: 10.3934/DSFE.2024006 |
[7] | Nitesha Dwarika . The risk-return relationship in South Africa: tail optimization of the GARCH-M approach. Data Science in Finance and Economics, 2022, 2(4): 391-415. doi: 10.3934/DSFE.2022020 |
[8] | Moses Khumalo, Hopolang Mashele, Modisane Seitshiro . Quantification of the stock market value at risk by using FIAPARCH, HYGARCH and FIGARCH models. Data Science in Finance and Economics, 2023, 3(4): 380-400. doi: 10.3934/DSFE.2023022 |
[9] | Michael Jacobs Jr. . Validation of corporate probability of default models considering alternative use cases and the quantification of model risk. Data Science in Finance and Economics, 2022, 2(1): 17-53. doi: 10.3934/DSFE.2022002 |
[10] | Man-Fai Leung, Abdullah Jawaid, Sai-Wang Ip, Chun-Hei Kwok, Shing Yan . A portfolio recommendation system based on machine learning and big data analytics. Data Science in Finance and Economics, 2023, 3(2): 152-165. doi: 10.3934/DSFE.2023009 |
Artificial Intelligence (AI) is deemed to be the commanding point of science and technology in the next era. In recent years, with the enhancement of computer computing power, the improvement of the quantity and quality of big data, and the important breakthroughs in many research fields such as machine learning and speech recognition, AI technology has developed rapidly and has been widely used in all walks of life. In the financial industry, the application of AI technology in risk control, marketing, customer service, transaction, operation, and product optimization of financial institutions is becoming increasingly mature, and some new business models have been created. Starting from the application status and significance of AI in the international financial field, this paper expounds on the application, status quo, and development trend of AI in the financial industry. Then, in view of the risks and practical challenges existing in the development process of AI, based on the reality of international financial development, this paper summarizes the measures to promote the in-depth, healthy, and sustainable development of AI in the financial market. This paper aims to let readers understand the development status of AI in the financial field, and also provide theoretical reference for scholars in this field.
Artificial Intelligence (AI) is a new technology science that integrates theory, method, technology, and application system by making computer simulation, extend and expand human intelligence, to make it similar to human thinking mode. Its essence wants to be a perfect and mature intelligent machine by continuously simulating and exploring human behavior. After years of development, the theory and technology of AI are becoming ever more mature, and the application field is also expanding. Now it has broken through the simple simulation of human behavior and developed into an integrated multi-functional application-oriented composite discipline. Together with virtual reality technology and 3D printing technology, it is known as "the hottest technology in the early 21st century".
With the development of digital technology and the increasing maturity of AI theory and technology, the roles of enterprises, the attributes of value creation, and even economic structures are changing rapidly (Schwab, 2017). In January 2016, in the Davos Forum, AI, as well as big data, were regarded as the core of the Fourth Industrial Revolution (Science and technology policy institute, 2016). Historically, the introduction of AI is an automated process that has gone on for a long time. Mechanization at the end of the 19th century and the end of the 20th century made part of the manual labor automation, and the progress of information technology in the middle and late 20th centuries made the standardized data processing automation (Korinek and Stiglitz, 2017). Based on Mustafa's research, expanding the application of modern information technology and automation will bring additional advantages (Mustafa and Rahman, 2015). Big Data, AI, blockchain, the Internet of Things (IoT), and other new technologies emerging in the Fourth Industrial Revolution are changing our economy and society. International Data Corporation (IDC), an American market research company specialized in IT, announced in October 2016 that cognitive systems and AI for integrating, learning, and analyzing data from a large number of vast and various information sources will be distributed across wide-ranging industries, and the global sales will increase from $8 billion in 2016 to $47 billion in 2020. This is equivalent to an annual growth rate of 55.1% (IDC, 2016). Companies from a wide range of industries, together with the government, get involved in research on AI and its applications, especially in the financial, stocks, and manufacturing industries (Kim, 2018). Following the report from the financial magazine Euromoney (McCauley, 2016), it is expected that the biggest changes will be achieved in the fields of credit evaluation, asset management, stock exchanges, and hedge fund management. They expect financial companies to conduct more effective portfolio and risk management through more precise and insightful data analysis. Tractica predicts that the enterprise AI system market will grow at an average annual rate of 56.1%, from $200 million in 2015 to $11.1 billion in 2024 (Tractica, 2015).
In the past 40 years, AI has made remarkable progress in imitating human reasoning and thought processes. Historically, the tools of AI were mainly limited to sequential processing and the very basic representation of knowledge and logic. A more modern method of AI is tantamount to constructing a computer with the structure and processing ability to imitate the human brain and thought process. These results provide the ability of knowledge representations based on a large amount of processing power, fast retrieval of large amounts of data, and pattern recognition based on experience. This AI technology is called neural computing or Artificial Neural Network (ANN). This paper summarizes the artificial neural network system and its wide application in the financial market, and briefly introduces the various uses of artificial neural networks in financial market modeling, and the successful application of artificial neural networks in various financial institutions.
Of course, AI has unprecedented advantages in the financial field but also has its own risks like all double-edged swords of science and technology. We must not ignore the risks inherent in the technology itself when we promote the development of AI technology in the financial industry.
The main content of this paper is illustrated in Figure 1. Firstly, starting from the international financial market, this paper summarizes the AI neural network theory and illustrates the wide application of AI in the financial market at five aspects: false financial report, financial fraud, financial distress, credit risk management, and stock market public opinion analysis. Secondly, this paper introduces the future application trend of AI in the financial field of three aspects of investment advisory, risk control, and marketing. Finally, it introduces four potential financial risks that AI may contain and puts forward policy suggestions. The structure of this paper is as follows: the second section mainly reviews the related concepts of AI and the application of AI in the financial field. The third section shows the future application trend of AI in the financial field from three aspects. The fourth section summarizes the hidden financial risks contained in AI. The fifth section puts forward the Countermeasures for the application risk prevention of AI in the financial market. The last section is the summary summarized the entire paper.
In recent years, artificial neural networks have been applied to develop various forms of research models. Neural network refers to AI technology. When there are multiple variables and complex interactions or multiple solutions, it can produce positive results. Therefore, artificial neural network technology is considered to be one of the methods used in the financial field (Cerullo and Cerullo, 1999; Chen and Du, 2009; Koskivaara, 2004; Tsai and Wu, 2008; Wong et al., 2000).
The basic idea of neural networks is to imitate biological neural networks. The neural network model is built on nodes (or processing units) to simulate the actions of neurons. The input and output links to these nodes simulate synapses. Assign weight to the input link to simulate neurotransmitter activity. Then use an algorithm to adjust the weight of the input link to make the neuron produce the required output, thereby simulating the learning process (Ibrahim, 2004). In general, a neural network is a kind of artificial system which can simulate the function of the human brain nervous system by modeling and connecting the basic unit of human brain neurons, and develop a kind of intelligent information processing function such as learning, association, memory and pattern recognition. An important characteristic of a neural network is that it can learn from the environment and store the learning results in the synaptic connections of the network. The neural network is a learning process. Under the stimulation of its environment, some sample patterns are successively inputted into the network, and the weight matrix of each layer of the network is adjusted according to certain rules (learning algorithm). When the weight of each layer of the network converges to a certain value, the learning process ends. Then we can use the generated neural network to classify the actual data. The basic flow chart of the neural network is presented in Figure 2.
The artificial neural network also has the capability of self-adaptation and self-organization. In the process of learning or training, change the synaptic weight value to be adapted to the requirements of the surrounding environment. The same network has different functions because of different learning methods and contents. The artificial neural network is a system with learning ability, which develops knowledge beyond the original knowledge level of the designer. Usually, its learning and training methods are divided into two kinds: one is supervised learning, in which case the given sample standard is used for classification or imitation. The other is unsupervised learning, in which case only learning methods or certain rules are specified, and the specific learning content varies with the environment of the system can automatically discover the characteristics and regularity of the environment. We add each input of the learning set for the neural network and tell the neural network what classification the output should be. After that, we use a neural network to test the examples. If the test passes (such as 80% or 90% accuracy), then the neural network is successfully constructed. For example, in financial applications, such as predicting the future price of stocks on stock exchanges, we may need a neural network to model the relationship between the pattern and the real value quantity (Anthony and Bartlett, 1999).
If the neural network is represented by a graph, Figure 3 shows the structure of the artificial neural network. It includes three main layers: the input layer, hidden layer, and output layer. The input layer allows the introduction of external data into the neural network, and the input external data is an independent variable. The function of the output layer is to transmit data, and the output data are the dependent variable. At the same time, the hidden layer has no strings attached to the external environment, and this function is only used to receive signals from the input layer and transmit them to the output layer (Küükkocaolu et al., 1997).
The data set is randomly divided into three parts: a training set, validity set, and test set. The training set data is used to train the network. The effective set is used together with the weight of the classifier, and the number of hidden units in the neural network is determined. The test set is used to evaluate the performance of training. In general, 80% of the data will be assigned to the training set, 10% to the effective set, and 10% to the test set (Küükkocaolu et al., 1997).
Compared with logistic regression, the advantage of ANN is that it needs less formal statistical training. Artificial neural networks can indirectly detect the complex nonlinear relationship between dependent variables and independent variables. In addition, artificial neural networks can detect all possible interactions between predictive variables and the availability of various training algorithms (TU, 1996). It is easier and faster to develop and implement artificial neural networks than to develop accounting expert system applications (Cerullo and Cerullo, 1999). The next section discusses some Ann applications from previous studies.
There are numerous fake listed companies in the history of Wall Street. Generations of investors should not only have a good eye on the Pearl, tap into potential listed companies, but also be clear about it, and avoid "mines" in the deep market. The fraud of Chinese listed companies is no less than Wall Street. At present, there are greater than 4000 listed companies with shares. They have read the thick financial reports and study the authenticity of the data, which is not covered by human resources. However, experts are indicating that the use of AI (particularly algorithms) can help to fight some of the risks (Chu, 2018; Killeen and Chan, 2018). Financial data fiction is the "mine" on the way of investors' investment. The ITL anti-financial fraud Intelligent Technology Committee was officially established, among which, the member units such as connected data will promote the use of new technologies such as big data and AI to discover and evaluate false financial reports.
Shizhong Huang, dean of Xiamen national accounting college, said big data and AI are powerful tools for anti-financial fraud. Although the anti-financial fraud data and algorithms are still in the initial stage, they have shown strong power. In the field of internet financial anti-fraud, researchers are trying to use Convolutional Neural Networks (Fu et al., 2016), Feature Engineering Strategies (Bahnsen et al., 2016), Cost-sensitive Decision Tree Approach (Sahin et al., 2013), Bayes Minimum Risk (Bahnsen et al., 2013) with Cost-sensitive and Hidden Markov Model (Bhingarde et al., 2015) to detect fraud transactions. In the future, anti-financial fraud will continue to exert its strength in the data warehouse, data processing, advanced algorithms, and other aspects. Li Feng, vice president of Shanghai Institute of finance, pointed out that the innovation of business models makes financial information more complex, and different enterprises have a different financial understanding of emerging business, which makes it more difficult to distinguish between financial fraud and normal financial business. In the face of such a complex environment, the traditional anti-fraud means have the characteristics of incomplete, less intuitive, low efficiency, and hard to see through. It is necessary to design and develop an anti-fraud system systematically, dynamically and in real-time by using the technical means of cloud computing, big data, and natural language processing, to bring about the business and management level within the regulatory departments, investors, investment institutions and enterprises promote.
Küükkocaolu et al. (1997) made an important step forward in determining the manipulated financial statements using an artificial neural network. They sampled 126 non-financial listed companies from the Istanbul stock exchange and used Beneish Model to provide input to ANN. Their results show that the probability of correct prediction is 86.17%, and the probability of false prediction is 13.82%. This shows that ANN forecasts the financial statements manipulated (Küükkocaolu et al., 1997).
Cerullo and Cerullo used artificial neural networks to predict false financial reports in their studies. They used seven variables as inputs to an: recording revenue when there is significant uncertainty, recording supplier refunds as revenue, failing to separate unusual and non-recurring income, the improper capitalization of assets, failure to accrue expected contingent liabilities, creating reserves, transferring sales revenue to later periods, and slow depreciation or amortization of assets. The results demonstrate that the artificial neural network predicts financial reporting fraud in management. In this case, compared with other methods, an artificial neural network provides prediction and accurate results in a short time (Cerullo and Cerullo, 1999).
Koskivaara used artificial neural networks in small samples from audit firms. They believe that using ANN for auditing could better serve the analysis review (AR) process, but only the financial ratio is used as the input of the artificial neural network. These financial ratios are based on the standards in the statement of audit standards: Analytical procedures. The results show that artificial neural network has the potential to improve the AR process. Although Kaminski et al. reported that financial ratios have limited ability to detect false financial reports, they are still widely used (Koskivaara, 2004).
Kotsiantis et al. (2006) used six financial ratios: profitability, leverage, liquidity, efficiency, cash flow, and financial distress. The sample included 41 fraud companies and 123 non-fraud companies. The paper compares the technology of Decision Tree, Artificial Neural Network, Bayesian Network, Logistic Regression Analysis, K-nearest Neighbor and Support Vector Machine and fraud detection technology, and establishes a hybrid decision system (Kotsiantis et al., 2006). The results of the experiment are consistent with the previous research. That is, published financial statements contain false financial indicators. However, due to the combination of technologies using financial data, the results of this study are restricted to financial data.
Companies such as Enron, Tyco, and WorldCom Inc. are estimated to have cost about $460 billion in market value due to financial fraud. In 2014, the Certified Fraud Association Examiner (ACFE) reported that U.S. organizations lost almost 5% of their revenue from fraud and that the gross domestic product is only in the U.S. The annual fraud based on the product is estimated to be approximately $3.7 trillion (Lin et al., 2015). Financial fraud is becoming an increasingly serious problem.
According to the "2018 intelligent anti-fraud insight report" released in Beijing on May 9, 2019, in the face of diversified financial and Telecommunications frauds, emerging technologies such as AI are being introduced by Chinese Internet enterprises and financial institutions for anti-fraud. The report believes that through the latest Internet technologies such as AI and blockchain, information collection sources will be more precise, risk pricing models will be personalized and targeted, investment decision-making processes will be more scientific and rigorous, and the role of credit intermediary will be more transparent and fairer.
Taking AI as an example, Zhang Feng, an expert of 360 financial anti-fraud technology, said that with the powerful data processing capacity of the machine, various information nodes of the applicant were constructed into a huge network diagram, on which an anti-fraud model based on machine learning was established, and repeated training and real-time identification were carried out. It is disclosed that at present, some domestic platforms have accumulated millions of blacklists and hundreds of millions of white list databases.
The nervous network system studied by Chen and Du (2009) was applied to predict fraud litigation to assist accountants in formulating audit strategies. The data used in the research are based on 74 prosecution cases and 148 non-prosecution cases, with SAS No.82 as the research tool, using indicators such as management characteristics and capabilities, operating characteristics and financial stability, and assets easy to be misappropriated. The empirical results show that, compared with the logistic model and auditor's judgment, the artificial neural network has not only higher prediction accuracy, but also better detection ability and lower misjudgment cost. This shows that AI technology is quite reliable in identifying fraud litigation and therefore becomes a supporting tool for practitioners (Chen and Du, 2009).
Lin et al. (2015) used expert questionnaires and data mining techniques to sort out different fraud factors and then ranked their importance. Among them, the data mining methods used include logistic regression, decision trees, and artificial neural networks (Lin et al., 2015).
Albashrawi studied 65 articles as far as possible to reveal the use of data mining in the field of financial fraud, showing the frequency, percentage of use of data mining technology and other general business applications. These data show the importance of AI technology in the field of financial fraud. It is worth noting that logistic regression, decision trees, SVM, NN, and Bayesian networks have been widely used to detect financial fraud, although they are not always associated with the best classification results (Albashrawi, 2021).
After the banking crisis in the 1980s and the early 1990s, the model composed of the nervous network system has played a new role. The prediction model can effectively prevent bank failure and promote economic stability. The latest prediction model technology was actively explored by Swicegood and compared with the existing methods. Their research has been used to predict the adverse performance of commercial banks in the banking industry through the use of discriminatory analysis, neural networks, and professional judgment methods (Swicegood et al., 2001).
Bankruptcy prediction and credit scoring have long been regarded as important topics and widely studied in accounting and financial literature (Du Jardin, 2010; Tsai and Wu, 2008). Initial financial ratios are utilized to represent the company's liquidity, solvency, financial structure, profitability, efficiency, and turnover by Du Jardin. The purpose of this study is to test the accuracy of artificial neural networks in bankruptcy prediction. The test sample included 250 bankrupt and 250 non-bankrupt retail companies with assets less than $750000. The validation consists of companies belonging to the same department and the same asset size category. The results show that the accuracy of the artificial neural network is 94.03%, while the accuracy of logistic regression is 90%.
At the same time, artificial neural network and data mining techniques are used by Chen and Du to build a financial distress prediction model by taking 34 companies in financial distress as samples and matching them with 34 non-bankrupt companies. The inputs of the neural network are profitability, financial structure, management ability, management performance, solvency, and the financial ratio of non-financial factors. These variables are selected based on previous studies. The results show that the ANN method has better prediction accuracy than the data mining clustering method, but its model cannot be directly applied to fraud detection (Chen and Du, 2009).
Lending is one of the important business of banks and financial institutions. The credit risk assessment and management risks of customers are faced by these institutions. Chinese bankers survey report (2018) was jointly issued by China Banking Association and PWC. The results show that more than 60% of the bankers believe that the concentrated outbreak of non-performing loans is the main risk faced by the world banking industry nowadays, and more than 30% of the bankers regard it as the biggest source of pressure faced by the bank operation. In the actual process of loan granting and management, people with insufficient records are often assessed by various credit risk assessment models, which makes the assessment process relatively loose. Therefore, since there are massive data of various types of users, AI takes advantage of its advantages in big data analysis, and various external unstructured data related to credit risk are analyzed. For example, judicial litigation data (court judicial website), industrial and commercial data, public opinion data of authorized media, etc. are used to carry out unified risk modeling, so as to achieve the effect of prejudgment and prevention of non-performing loans.
Pacelli established a credit risk model and applied artificial neural network methods to credit risk management investigations. Using the financial data of 24 companies as the input of ANN, the credit risk of the Italian manufacturing industry was predicted. The results show that artificial neural networks predict the credit risk of these companies (Pacelli, 2011). The credit risk model is a powerful tool for modeling and predicting the default of a company. This AI-based model is usually used for financial analysis and decision-making tasks (Khandani et al., 2010; Yu et al., 2010; Khashman, 2011; Lessmann et al., 2015; Abellán and Castellano, 2017).
Merchants and individuals are granted credit in advance by Alibaba and Jingdong using AI. Borrowers do not need a collateral guarantee, and the whole operation cycle can be monitored by the background. Before merchants apply for loans, customers' qualifications, assets, income level, behavior, and other characteristics are fully and effectively analyzed by the platform, and a customer access white list is formulated. At the same time, it designs a pre-credit model based on its risk preference, and actively determines a certain amount of pre-credit line for customers, which can be dynamically adjusted at different stages according to different scenarios and changes in demand. Its supply chain financial business has realized closed-loop management of full link, unified credit extension, and real-time conversion of the credit limit. Based on fragmented, scenario-based, and personalized financial services, it has formed an overall service plan of the full scenario and full link. Through mastering the customer data entry, the closed-loop management of the whole customer process within its system is realized, and the credit risk is effectively controlled.
With the development of the stock market becoming larger and larger, the number of participants is increasing, and the proportion of natural investors is relatively high. In response to the market demand, more and more AI-based models have come to the attention of the public, such as MACD (moving average index of smooth similarities and differences), KDJ (random index), RSI (relative strength index), etc. These parameters are often too old to adapt to the current market changes. Nowadays, many models based on AI have been applied to the analysis of public opinion in the stock market: the BP network prediction model was used by Wu Tao in 2014, and a stock simulation trading system was designed based on the prediction model of BP network. In 2015, the stock analysis system was designed by Hao Yuanyuan through the investment concept of various stock reviewers, providing reasonable investment suggestions for the system users. The prediction model of stock buy point was proposed by Zhang Yu in 2016, and more than 2000 stocks in Shanghai and Shenzhen stock markets were trained and studied by the BP network to predict the buy point and provide decision-making assistance for investors.
Patel et al. (2020) discussed the application of a machine learning framework to predict stock and stock price index changes. They used four prediction models to analyze data: Ann, SVM, Random Forest, and Naive Bayes. Reviews of two organization's stocks, namely Reliance Industries, and Infosys Ltd., and two stock price indices CNX Nifty and S & P Bombay Stock Exchange (BSE) are taken from 2003 to 2012 (Patel et al., 2015; Patel et al., 2020). Khedr et al. (2017) proposed an analysis and optimization framework to focus on the minimum error rate and improve the prediction accuracy when predicting the performance mode of stock price. The financial news method and historical value method are used to establish the prediction model. The above research effectively uses AI and various market and company data to establish a stock forecasting model with high accuracy.
Due to the uncertainty and volatility of stock market prices, stock market forecasting is a challenging task for global financial investors. ANN is adopted by Sureshkumar and Elango to forecast the future stock price more quickly and accurately. The daily stock data of Tata Consultancy Services Ltd (TCS) from 1 November 2009 to 12 December 2011 were used by them to forecast the future stock price of the company based on the previous closing price, opening price, high price, low price, and closing price. The analysis results show that compared with the existing tools and technologies, the accuracy of the prediction of the stock price by the artificial neural network is more than 20% (Sureshkumar and Elango, 2012).
AI investment advisor mainly refers to the use of intelligent algorithm technology and portfolio optimization theoretical model to provide users with investment decision-making information reference according to the risk preference, investment income requirements and investment style and other information provided by individual investors, and to provide suggestions for the improvement of the asset portfolio and allocation with the dynamic changes of the financial market (Jiang and Wu, 2016). Intelligent investment advisers can not only surpass human beings in investment allocation and transaction execution ability but also help investors overcome their emotional weaknesses (Yu and Peng, 2017). It is the field in which financial institutions apply AI technology more widely at present.AI investment banking emerged in the United States in 2008. Wall Street's enthusiasm for AI and big data and the growing market demand for wealth management have promoted the application of AI in the asset management industry. Currently, there are numerous mature smart investment platforms in the US market, such as Vanguard Fund, Charles Schwab, Betterment, Wealthfront, and so on. A.T.Kearney, a consulting firm, predicts that the industry's assets under management in the US will grow from $300bn in 2016 to $2.2tn in 2020, at a compound annual growth rate of 68%, or about 5.6% of all assets under management in the US.
Intelligent investment banking entered the Chinese market in 2014, initially dominated by Internet companies, and then major commercial banks and financial institutions in China gradually adopted the technology, which has grown rapidly in recent years. According to data from iResearch, the asset management scale of China's smart wealth management service market (based on the balance of wealth management products) has risen from 30.1 billion yuan in 2016 to 254.7 billion yuan in 2018 and is expected to reach 737.1 billion yuan in 2022. At present, the main participants of China's intelligent investment market are traditional financial institutions (securities companies, fund management companies, etc.), Internet companies, and financial IT companies. Compared with interconnected special planning networks and financial IT companies, the advantage of traditional financial institutions lies in their accumulated customer network, product resources, and business experience. In 2016, China Merchants Bank launched the first intelligent domestic investment platform "Capricorn Investment". Shanghai Pudong Development Bank followed suit with its "financial intelligence robot". In 2017, Industrial Bank's Industrial Intelligence Investment, Ping An Bank's Intelligent Investment, Everbright Bank's Guangyun Intelligent Investment, and Industrial and Commercial Bank of China's AI Investment were launched successively (Wang, 2017).
With the rise of Internet finance, the banking industry's demand for user credit qualification assessment increases, which promotes the development of intelligent risk control. Financial risk management can avoid losses and maximize profits for most enterprises. Since tasks rely heavily on information-driven decision-making, machine learning is an important task (Mashrur et al., 2020). In recent years, we have seen more and more people adopt Machine learning methods for various risk management tasks. Intelligent risk control by neural network, knowledge map, machine learning, data analysis and so on many technical structures, an into a system of the risk control system, the background for customer survey (Know-your-customer, KYC), credit evaluation, credit approval, after the credit collection, greatly improve the efficiency of each process, to improve the customer experience, In the process of use, the model is continuously iterated according to data feedback to increase the accuracy. AI systems also improve the efficiency of anti-money laundering and anti-fraud by learning from past cases, processing multi-dimensional structured and unstructured data, and monitoring and screening suspicious transactions. The intelligent risk control process is illustrated in Figure 4.
AI is already being used by regulators and financial institutions around the world to combat money laundering and fraud. HSBC partnered with Silicon Valley AI startup Ayasdi to automate the anti-money laundering investigation process. The Financial Crimes Enforcement Network (FinCEN) uses its AI system, FAIS, to identify potential money laundering activities (Gutierrez et al., 2015). The UK Financial Regulatory Authority (FCA) is considering the use of AI/machine learning in regulatory compliance enforcement processes, including KYC and AML. The Monetary Authority of Singapore and the Securities and Exchange Commission of the United States (SEC) are also using AI to identify suspicious transactions to improve their anti-money laundering efforts. In addition, AI is also being used to supervise the operations and financial performance of financial institutions and listed companies. The SEC uses AI to process and analyze unstructured data filed by the registration applicants, to analyze and predict the behaviors of the applicants from multiple dimensions, and to reflect the information into the risk level.
The marketing combination of AI and big data technology analyzes and classifies the data generated by users. Combining relevant data such as customers' consumption preferences, financial status, and behavior patterns, the platform realizes the precise positioning of customers' needs, build customer portraits, predict customer needs, match and push personalized products, and marketing copywriting. Smart marketing is gradually recognized by advertisers and marketers and has been invested in multiple fields, forming a mature system. The application architecture of the intelligent marketing push system is shown in Figure 5.
Compared with the traditional marketing form, AI marketing can analyze the existing data, and analyze the customer's price tolerance, brand preference elements, quality care degree from the micro-level. Such accurate positioning can better play the advantages of AI and improve the effectiveness of marketing, which is the effect that traditional marketing can not achieve (Yu, 2019).
Using a deep neural network to automatically extract features with depth and information from the original user data to construct a user feature representation helps make fuller use of user data and effectively improve the accuracy of user portraits. Using a user representation model based on deep neural networks can effectively overcome the shortcomings of the existing user portrait methods based on feature engineering and linear models. In 1996, PointCast Network proposed the information push technology for the first time, which sends the specific content to the client through the network software. Later, several tech companies, including Microsoft and Amazon, developed personalized push software built on a user's profile and interests. HURA model proposed by Wu, C. et al. based on the multi-layer attention mechanism and neural network structure, the user's personal attributes, and preferences are effectively predicted through search logs (Wu et al., 2019).
With the further application of AI technology, Kumar et al. (2019) proposed a comprehensive framework to understand the application of AI in personalized marketing: enterprises can use this technology to analyze consumer information and provide personalized products and services. In this process, AI can continue to learn and help managers improve the value proposition for customers. By designing and planning products in this way, they could increase their value to consumers and thus form a sustainable competitive advantage.
Marketers use AI to segment the market, predict consumers' personalized preferences, deploy targeted digital ads in real-time, or provide suppliers, with optimal promotion and pricing strategies. Timoshenko and Hauser (2019) use a convolutional neural network to filter out non-informative contents of UGC and cluster sentences to avoid repeating content sampling. It is proved that the machine learning method successfully eliminates the ineffective and repetitive information of UGC, and improve the efficiency of identifying customer requirements from UGC.
The global regulatory environment has grown in complexity and scope since the financial crisis in 2008. This is causing significant problems for organizations in the financial industry, as the complexity of hard and soft regulations little understood or appreciated (Brummer, 2010). The deep application of AI in the financial field needs the basis of legislation, However, whether in the standardization of regulatory laws or in the detailed regulatory policies and rules, it still stays at the level of Internet finance, and there are no systematic and standardized laws and regulations. The lack of laws and regulations makes the regulatory boundary vague. Many business forms related to AI can only be managed by referring to the laws and regulations in the field of traditional finance and internet finance. Once the disputes happened, the determination of legal liability is often lacking legal basis. For example, Cheng et al. pointed out that the decentralized nature of applicable laws and regulations and departmental rules for smart investment means that it is difficult for the supervisory department to grasp the boundaries of supervision in the actual operation process. This makes it easy for the AI investment platform to go beyond the scope of its main business and engage in illegal activities such as false propaganda, disguised fund absorption, illegal fund-raising, securities marketing, and illegal operations (Cheng et al., 2017). Secondly, the innovative application and development of AI pose new challenges to the financial regulatory system. Although a series of relevant guidelines have been issued in China, it is still necessary to further refine the relevant supporting policies of different industries, and improve the "legal gap" and "regulatory vacuum" of the industry. In addition, the deepening application of AI in the financial market, leading to changes in financial operations models and regulatory models, how to keep pace with the times, and constantly improve the legal system is also an important topic that needs attention to relevant agencies.
First of all, from the perspective of information collection, as an information-intensive industry, in order to ensure the smooth progress of financial transactions, it often needs to analyze, collect and handle a large number of customer behavior data in financial business, and the expansion of data collection range. Under the existing legal and regulatory system, it will be difficult to define the social problems caused by failure or behavior, such as responsibility and behavior supervision. For example, the behavior generated by the learning and decision-making mechanism of AI can not be traced back, the responsibility subject of malicious behavior caused by developers and designers is difficult to define, and the processing cost of its chain consequences will increase greatly. Due to the lack of relevant collection criteria and regulations, there is a legitimacy problem with data acquisition in specific practice. Secondly, from the perspective of data usage, once artificially intelligent communication and financial infrastructure are attacked, personal information is easily attacked by hackers or controlled by criminals, which will cause property losses to customers and even personal safety. In the 2015 data leakage cost survey: global analysis released by IBM and Ponemon Institute, it is pointed out that "the average total cost of data leakage of 350 companies participating in the study increased from 3.52 to 3.79 million US dollars, and the average cost of each lost or stolen record (including sensitive and confidential information) increased from 145 US dollars in 2014 to 154 US dollars in 2015 survey" (Ponemon et al., 2016). Moreover, since AI has not yet developed mature, the safety vulnerability has a slower repair rate, and the criminals may use related technologies to accurately identify potential goals, real time attack on the system, endangering the whole social credit system. The main objectives of the attackers are the theft, modification, and elimination of information, as well as the impersonation of individuals, which leads to fraud, economic losses, personal identification for a specific purpose, theft of devices to obtain control and information from those that are connected to the network (Alvarez et al., 2020). Under the existing legal and regulatory system, it will be difficult to define the social problems caused by failure or behavior, such as responsibility and behavior supervision (Cheng, 2016). Third, from the perspective of data storage. In the era of Internet, a large amount of data is usually stored in hard disk or floppy disk. If people intentionally or unintentionally lose data, it will cause the risk of data leakage. In addition, from the perspective of data transmission. At present, the standards of algorithm application, information control and disclosure adopted in China's financial business are inconsistent. On the one hand, it makes it difficult to guarantee the information confidentiality of the AI system and the right to know about the user. On the other hand, it also limits the ability of data integration and transmission, resulting in the uneven quality of historical data, which is prone to some data loss and errors.
First of all, the current AI is still in the exploration period, which inevitably meets many technical problems and brings some risks. For example, AI technology has been extensively used in the banking industry and many banks use face recognition to withdraw money from ATMs. So far, the maturity of face recognition technology is not high enough. If only face recognition is the only level, there are some risks in large-scale online face brushing transaction services. In addition, relying on AI technology, intelligent investment advisory also faces a series of technical challenges (Ma and Wei, 2018). Secondly, the technical depth is not enough. In recent years, the phenomenon of black swan often exists in the financial market, but the speed of machine deep learning is not enough to cope with the rhythm of the financial market, especially the rhythm of financial risks. At the same time, there are some defects and loopholes in the algorithm, which lead to the serious disconnection between the scheme and investment advice and the market reality, thus bringing huge investment losses to investors. Especially financial risks, and there are also certain defects and vulnerabilities in algorithms, leading to programs and investment recommendations and markets. Reality is seriously detached, which brings huge investment losses to investors. Third, due to the influence of technology reserve, capital scale, and talents, many small and medium-sized financial enterprises or institutions in the world have relatively low willingness or ability to apply AI, and the application process is slow and the effect is not obvious. For example, many cities commercial banks have not incorporated financial technology into their strategic planning. They are still passive in their subjective will and lack of supporting hardware or software architecture. Fourth, AI has a set pre-input algorithm program. If a large number of investors in the financial market use the same algorithm and operation mode, it will pose a challenge to financial stability. Moreover, if the AI transaction procedure fails or errors in algorithm transaction, it will not only lead to incorrect data analysis results and decision-making but also affect related business activities, which will not be conducive to the smooth completion of financial transactions, bring huge losses to financial consumers. And if it is serious, it will affect the stability of the whole financial market. Due to basic research and data limitations, AI will find it difficult to cope with the entire complex financial market alone. When encountering some emergencies, such as geological disasters, regulatory changes, and short-selling bans, the AI system may not be able to respond correctly (Wu et al., 2016).
At present, for the application of AI technology in the financial field, there is still a lack of relatively perfect regulatory rules. Once there is a business or service dispute, it will face a series of regulatory problems (Ma and Wei, 2018). On the one hand, financial risks become more concealed and complex. Although the application of AI in the financial market has spawned many new finance technologies, it has not changed the original risk attributes and types of the financial industry. On the contrary, it makes the risk characteristics more complex and difficult to identify. Specifically: First, modern technology such as AI promotes rapid changes in the financial industry, new products, new business, and new models, and integrates business in different fields, and increases the complexity of financial risks. Second, it reduces the risk controllability. Under the influence of AI and other financial technologies, financial transactions will be cleared in real-time. This will lead to a faster spread of risks and a wider scope, and it will be more difficult for financial regulators to rescue the market and isolate risks, and aggravate panic among market participants. Third, financial risks are more hidden. The application of AI in the financial field makes the participants of financial activities have multiple identities at the same time. The lack of supervision makes it difficult to identify the financial risks, and the financial risks are more hidden. In addition, the application of AI reduces the entry threshold of financial business, strengthens the motivation of financial institutions to engage in high-risk business activities, and highlights the risk preference of the whole financial system. On the other hand, with the continuous promotion of AI, financial regulators should not only deal with the old problems in the traditional financial business behavior and mode but also face new problems in the financial field with the development of technology, which puts forward new challenges to financial regulation. However, under the current financial regulatory system, it is not only relatively difficult to identify the risk responsibility, but also increases the cost of follow-up treatment. At the same time, there is a lack of AI simulation of specific scenarios, which may easily lead to deviations in the judgment and execution of tasks and lead to abnormal fluctuations in the financial market, thus endangering the stability of the financial market order.
The emergence of the intelligent revolution brings an unprecedented crisis and challenge to the current ethical standards, legal rules, social order, and public management system. Wu (2017) proposed that in terms of the legal system to adjust the social relations related to AI, there are mainly the issues of personality rights of human privacy protection, tort law of human damage caused by an intelligent system, and copyright of works generated by AI.
Establish and improve laws and regulations related to AI, refine specific policies, determine the scope and direction of application of policies and regulations, standardize the specific application of AI, and provide support for the wider and rapid promotion of AI in the financial market. At the same time, we should keep pace with the time, combined with the actual development of financial business, and constantly improve the legal system of new technology and new business, so as to avoid the phenomenon of disconnection between technology and law.
On the one hand, we should distinguish the types of personal information, strengthen the protection of personal information, refine the rules and technical rules for personal information, and ensure that the internal procedures of AI can be divided and applied according to the corresponding rules. On the other hand, we should stress the urgency of legislation, and make key legislation on AI through the pilot, improve the AI Intelligent regulation and responsibility bearing method can promote economic development while realizing AI, and ensure the sound operation of international financial market economy and steady development of economic order.
First of all, we should strengthen the financial risk knowledge of financial consumers, improve their risk identification ability, and guide them to make reasonable financial consumption. Establish and improve the complaint handling mechanism of financial consumers, strengthen the defense line of financial security, and protect the legitimate rights and interests of financial consumers. Secondly, financial enterprises have sensitive information, which needs to be transmitted and accessed on their own devices, such as personal health information, credit card information in banks, and top secrets information in government agencies. Such information needs to be protected against unauthorized disclosure (Abbadi and Alawneh, 2008). we should establish and improve the financial privacy protection system, strengthen the relevant administrative supervision, and clarify the relevant disclosure obligations, information security obligations, and compensation liabilities of financial institutions, to effectively ensure the information security of the application of AI in the financial field. Thirdly, financial institutions should take the initiative to strengthen communication and cooperation with AI technology development enterprises, complement each other's advantages, use AI technology to improve R & D institutions' ability in developing their customer information encryption technology, strengthen the management of user information and data, prevent and resolve information security risks, and protect user privacy. In addition, financial supervision departments should further improve the complaint handling mechanism of financial consumers, build a strong financial security defense line, protect the legitimate rights and interests of financial consumers to the greatest extent, and gradually promote the improvement of supervision mechanism, supervision content and supervision mode in combination with the guidance of complaint subject (Li, 2012).
From the perspective of financial institutions. To start with, we should realize the importance of AI, conform to its development trend, actively arrange corresponding new intelligent business forms, strengthen the level of financial infrastructure construction, and strengthen the independent innovation of financial technology. Secondly, we should improve the vulnerability of AI systems, strengthen the defense technology of AI systems, establish a self-healing system and data-sharing platform, regularly detect network security performance, actively protect data information and prevent virus intrusion (Marcus and Huang, 2019). To judge and monitor abnormal payment behavior and improve the security of financial transactions, a combination of biological features is used to prevent biological fraud. Thirdly, ensure the independence and controllability of financial technology, increase the support for innovation and R & D of financial technology enterprises, and strictly review and real-time monitor the software and hardware environment of AI applications. We should prevent the risk of financial fraud, make a scientific and reasonable forecast of its future trading trend, establish a security system, and constantly update technology to prevent criminals from taking advantage of it.
The introduction of AI into financial services is one of the more inclined forward-looking explorations in today's international financial institutions. AI has been increasingly introduced into financial asset trading, wealth and asset management, insurance and banking, customer service, credit lending, and other fields.
Based on the background and significance of international finance, this paper reviews the application and current situation of AI in the financial industry. This paper introduces the wide application of AI in the financial market from five aspects: the discovery and prediction of the false financial report, the discovery, and prediction of financial distress, the discovery and prediction of fraud cases, the prediction of credit risk management, and the prediction of the stock market price. More and more countries are actively participating in the AI financial market. At present, some developed countries' AI financial markets are at the forefront, while most emerging developing countries are in the stage of exploration and learning.
In addition, this paper points out four potential financial risks that AI may contain in the world and puts forward policy suggestions. First, the widespread application of AI has brought a great test to the use and management of financial data information in various countries. From the practical experience of supervision in various countries, it is found that all countries have made the moral standards for the development and application of AI before they promote the development and application of AI technology. From the perspective of financial risk prevention, many financial market participants may flood the mechanization transaction by using AI technology at the same time, and the possibility of financial crisis and instability will increase a lot. Second, the widespread use of AI will lead to the chaos of ethics, morality, law, and responsible subjects. Therefore, the application of AI in the financial field needs a strict legal system and ethical norms to clarify the rights, obligations, and responsibilities of legal subjects. Third, we must attach great importance to the security of personal privacy. This is part of the yardsticks of national and social civilization, which cannot be surpassed by any kind of science and technology. AI could collect massive data and make diversified analyses. As more and more data are collected, the risk of personal privacy leakage will certainly increase. It is urgent to establish a set of mechanisms to protect consumer privacy and data security. The review of AI data privacy settings becomes crucial. Fourth, it is necessary to carry out international AI security cooperation. On the one hand, the international community should unite to resist the moral hazard of AI technology and "encircle" the illegal international financial trends, to maintain the safe and healthy development of AI. On the other hand, the international financial community actively cooperates to share new scientific and technological achievements, to establish and improve the financial market of AI, so that the welfare of the society brought by financial AI will ultimately shine on mankind.
All authors declare no conflicts of interest in this paper.
[1] | Abbadi IM, Alawneh M (2008) Preventing insider information leakage for enterprises. 2008 Second International Conference on Emerging Security Information, Systems and Technologies, 99–106. |
[2] |
Abellán J, Castellano JG (2017) A comparative study on base classifiers in ensemble methods for credit scoring. Expert Syst Appl 73: 1–10. doi: 10.1016/j.eswa.2016.12.020
![]() |
[3] |
Albashrawi M (2021) Detecting financial fraud using data mining techniques: A decade review from 2004 to 2015. J Data Sci 14: 553–570. doi: 10.6339/JDS.201607_14(3).0010
![]() |
[4] | Anthony M, Bartlett P (1999) Neural Network Learning-Theoretical Foundations. |
[5] | Khedr AE, Salama SE, Yaseen N (2017) Predicting stock market behavior using data mining technique and news sentiment analysis. Int J Intell Syst Appl 9: 22–30. |
[6] |
Bahnsen AC, Aouada D, Stojanovic A (2016) Feature engineering strategies for credit card fraud detection. Expert Syst Appl 51: 134–142. doi: 10.1016/j.eswa.2015.12.030
![]() |
[7] | Bahnsen AC, Stojanovic A, Aouada D, et al. (2013) Cost sensitive credit card fraud detection using bayes minimum risk. 2013 12th International Conference on Machine Learning and Applications. |
[8] | Bhingarde A, Bangar A, Gupta K, et al. (2015) Credit card fraud detection using hidden markov model. Int J Comput Sci Inform Technol 76: 169–170. |
[9] | Brummer C (2010) How International Financial Law Works (and How it Doesn't). |
[10] | Cerullo MJ, Cerullo V (1999) Using neural networks to predict financial reporting fraud: part 2. Comput Fraud Secur 1999: 14–17. |
[11] |
Chen WS, Du YK (2009) Using neural networks and data mining techniques for the financial distress prediction model. Expert Syst Appl 36: 4075–4086. doi: 10.1016/j.eswa.2008.03.020
![]() |
[12] | Cheng DL (2016) Application status and security risk analysis of artificial intelligence in financial field. Financ Technol Era 2016: 47–49. |
[13] | Cheng J, He J, Yuan H, et al. (2017) Financial technology risks and regulatory countermeasures. China Financ 2017. |
[14] | Chu AB (2018) Mobile Technology and financial inclusion. In: Handbook of Blockchain, Digital Finance, and Inclusion, 1 Eds., Cambridge: Academic Press, 131–44. |
[15] |
Du Jardin P (2010) Predicting bankruptcy using neural networks and other classification methods: The influence of variable selection techniques on model accuracy. Neurocomputing 73: 2047–2060. doi: 10.1016/j.neucom.2009.11.034
![]() |
[16] | Fu K, Cheng D, Tu Y, et al. (2016) Credit card fraud detection using convolutional neural networks. International Conference on Neural Information Processing, 483–490. |
[17] | Gutierrez FH, Levin SC, Chambers MA, et al. (2015) FinCEN proposes AML requirements for registered investment advisers. Bank Financ Serv Policy Rep 34:15–18. |
[18] | Ibrahim A (2004) Chapter 6-Neural networks. |
[19] | IDC (2016) Worldwide Semiannual Cognitive/Artificial Intelligence Systems Spending Guide. |
[20] | Jiang HY, Wu CF (2016) Development status and regulatory suggestions of intelligent investment consultant. Secur Mark Guide. |
[21] | Kaminski KA, Wetzel TS, Guan L (2004) Can financial ratios detect fraudulent financial reporting? Managerial Auditing J 19: 15–28. |
[22] |
Khandani AE, Kim AJ, Lo AW (2010) Consumer credit-risk models via machine-learning algorithms. J Bank Financ 34: 2767–2787. doi: 10.1016/j.jbankfin.2010.06.001
![]() |
[23] |
Khashman A (2011) Credit risk evaluation using neural networks: Emotional versus conventional models. Appl Soft Comput 11: 5477–5484. doi: 10.1016/j.asoc.2011.05.011
![]() |
[24] | Killeen A, Chan R (2018) Global financial institutions 2.0, In: Handbook of Blockchain, Digital Finance, and Inclusion, 2 Eds., Amsterdam: Elsevier Inc, 213–242. |
[25] | Kim D, Kwahk K (2013) Investigating the global financial markets from a social network analysis perspective. J Korean Oper Res Manage Sci Soc 38: 11–33. |
[26] | Kim J (2018) Tasks and current status of the uses of artificial intelligence (fintech ai) in the fintech industry in the USA. J Payment Settlement 10: 185–214. |
[27] | Korinek A, Stiglitz JE (2017) Artificial intelligence and its implications for income distribution and unemployment. Natl Bur Econ Res. |
[28] |
Koskivaara E (2004) Artificial neural networks in analytical review procedures. Manage Auditing J 19: 191–223. doi: 10.1108/02686900410517821
![]() |
[29] | Kotsiantis S, Koumanakos E, Tzelepis D, et al. (2006) Forecasting fraudulent financial statements using data mining. Int J Comput Int 3: 104–110. |
[30] |
Kumar V, Rajan B, Venkatesan R, et al. (2019) Understanding the role of artificial intelligence in personalized engagement marketing. Calif Manage Rev 61: 135–155. doi: 10.1177/0008125619859317
![]() |
[31] | Küükkocaolu G, Benli Y, Küçüksözen C (1997) Detecting the manipulation of financial information by using artificial neural network models. Istanbul Stock Exc Rev 9. |
[32] |
Lessmann S, Baesens B, Seow HV, et al. (2015) Benchmarking state-of-the-art classification algorithms for credit scoring: An update of research. Eur J Oper Res 247: 124–136. doi: 10.1016/j.ejor.2015.05.030
![]() |
[33] | Li JZ (2012) Improving the management structure of consumer protection in banking institutions-Also commenting on the notice of China Banking Regulatory Commission on improving the customer complaint handling mechanism of banking financial institutions and doing a good job in financial consumer protection. Banker, 116–119. |
[34] |
Lin C, Chiu A, Huang SY, et al. (2015) Detecting the financial statement fraud: The analysis of the differences between data mining techniques and experts' judgments. Knowl Based Syst 89: 459–470. doi: 10.1016/j.knosys.2015.08.011
![]() |
[35] | Ma SL, Wei FY (2018) Application of artificial intelligence technology in financial field: main difficulties and countermeasures. South Financ, 78–84. |
[36] | Marcus C, Huang Z (2019) Artificial intelligence attacks: Artificial intelligence security vulnerabilities and countermeasures. Inform Secur Commun Secrecy, 72–81. |
[37] |
Mashrur A, Luo W, Zaidi NA, et al. (2020) Machine learning for financial risk management: A survey. IEEE Access 8: 203203–203223. doi: 10.1109/ACCESS.2020.3036322
![]() |
[38] | McCauley D (2016) Ghosts in the Machine: Artificial Intelligence, Risks and Regulation in Financial Markets. London Thought Leadership Consulting. Available from: https://www.euromoneythoughtleadership.com/ghostsinthemachine/. |
[39] |
Mustafa M, Rahman M (2015) Financial inclusion and per capita real GSP growth across fifty US states and the district of Columbia: Evidence from panel cointegration and GMM estimates. Glob Bus Financ Rev 20: 87–94. doi: 10.17549/gbfr.2015.20.1.87
![]() |
[40] | Pacelli V (2011) An artificial neural network approach for credit risk management. J Intell Learn Syst Appl 3: 103–112. |
[41] | Patel CI, Labana D, Pandya S, et al. (2020) Histogram of oriented gradient-based fusion of features for human action recognition in action video sequences. Sensors 20: 7299. |
[42] |
Patel J, Shah S, Thakkar P, et al. (2015) Predecting stock and stock price index movement using trend deterministic data preparation and machine learning techniques. Expert Syst Appl 42: 2162–2172. doi: 10.1016/j.eswa.2014.10.031
![]() |
[43] | Ponemon L, Julian T, Lalan C (2016) IBM & Ponemon Institute Study: Data Breach Costs Rising, Now $4 million per Incident. |
[44] |
Sahin Y, Bulkan S, Duman E (2013) A cost-sensitive decision tree approach for fraud detection. Expert Syst Appl 40: 5916–5923. doi: 10.1016/j.eswa.2013.05.021
![]() |
[45] | Schwab K (2017) Science and technology policy institute forum, In: The Fourth Industrial Revolution, 26 Eds., Geneva: Crown Publishing Group, 12–15. |
[46] | Sureshkumar K, Elango N (2012) Performance analysis of stock price prediction using artificial neural network. Glob J Comput Sci Technol 12. |
[47] | Swicegood P, Clark JA (2001) Off-site monitoring systems for predicting bank underperformance: a comparison of neural networks, discriminant analysis, and professional human judgment, intelligent. Syst Account Financ Manage 10: 169–186. |
[48] | Timoshenko A, Hauser JR (2019) Identifying customer needs from user-generated content. Mark Sci 38. |
[49] | Tractica (2015) Artificial Intelligence for Enterprise Applications: Deep Learning, Predictive Computing, Image Recognition, Speech Recognition, and Other AI Technologies for Enterprise Markets-Global Market Analysis and Forecasts. Available from: https://tractica.omdia.com/wp-content/uploads/2015/04/AIE-15-Brochure.pdf. |
[50] |
Tsai CF, Wu JW (2008) Using neural network ensembles for bankruptcy prediction and credit scoring. Expert Syst Appl 34: 2639–2649. doi: 10.1016/j.eswa.2007.05.019
![]() |
[51] |
Tu JV (1996) Advantages and disadvantages of using artificial neural networks versus logistic regression for predicting medical outcomes. J Clin Epidemiol 49: 1225–1231. doi: 10.1016/S0895-4356(96)00002-9
![]() |
[52] | Wang D (2017) Traditional financial institutions are ready to move. Is it more advantageous to set foot in intelligent investment advisory. China's Strategic Emerg Ind, 70–72. |
[53] |
Wong BK, Lai VS, Lam J (2000) A bibliography of neural network business applications research: 1994–1998. Comput Oper Res 27: 1045–1076. doi: 10.1016/S0305-0548(99)00142-2
![]() |
[54] | Wu HD (2017) Institutional arrangement and legal regulation in the age of artificial intelligence. Legal Sci, 128–136. |
[55] | Wu J, Chen L, Gao Y (2016) The application of foreign artificial intelligence in the field of financial investment advisory and its enlightenment to my country. Financ Asp, 88–92. |
[56] | Wu C, Wu F, Liu J, et al. (2019) Neural demographic prediction using search query. Proceedings of the Twelfth ACM International Conference on Web Search and Data Mining. |
[57] | Alvarez Y, Leguizamón-Páez MA, Londoño TJ (2020) Risks and security solutions existing in the Internet of things (IoT) in relation to Big Data. Ing Compet 23: 9–10. |
[58] | Yu M (2019) Research on the current situation and development trend of marketing industry under the background of artificial intelligence. Wealth Life, 82–83. |
[59] | Yu XJ, Peng YY (2017) Application and challenge of artificial intelligence in financial risk management. South Financ. |
[60] |
Yu L, Yue W, Wang S, et al. (2010) Support vector machine based multiagent ensemble learning for credit risk evaluation. Expert Syst Appl 37: 1351–1360. doi: 10.1016/j.eswa.2009.06.083
![]() |
1. | Fatima Tfaily, Mohamad M. Fouad, Multi-level stacking of LSTM recurrent models for predicting stock-market indices, 2022, 2, 2769-2140, 147, 10.3934/DSFE.2022007 | |
2. | Hassan Ali Al-Ababneh, Victoria Borisova, Alina Zakharzhevska, Polina Tkachenko, Natalia Andrusiak, Performance of Artificial Intelligence Technologies in Banking Institutions, 2022, 20, 2224-2899, 307, 10.37394/23207.2023.20.29 | |
3. | Leila Allahqoli, Antonio Simone Laganà, Afrooz Mazidimoradi, Hamid Salehiniya, Veronika Günther, Vito Chiantera, Shirin Karimi Goghari, Mohammad Matin Ghiasvand, Azam Rahmani, Zohre Momenimovahed, Ibrahim Alkatout, Diagnosis of Cervical Cancer and Pre-Cancerous Lesions by Artificial Intelligence: A Systematic Review, 2022, 12, 2075-4418, 2771, 10.3390/diagnostics12112771 | |
4. | Heiko Thimm, Karsten Boye Rasmussen, ChatGPT discovery of green image damaging information for large production companies, 2024, 478, 09596526, 143978, 10.1016/j.jclepro.2024.143978 | |
5. | Nurhadhinah Nadiah Ridzuan, Masairol Masri, Muhammad Anshari, Norma Latif Fitriyani, Muhammad Syafrudin, AI in the Financial Sector: The Line between Innovation, Regulation and Ethical Responsibility, 2024, 15, 2078-2489, 432, 10.3390/info15080432 | |
6. | Muhammad Naeem, Muzzammil Siraj, Shoukat Ali, Abdul Rehman, Sumair Farooq, 2024, chapter 4, 9798369337035, 83, 10.4018/979-8-3693-3703-5.ch004 | |
7. | Dong Chen, Yang Wu, Research on the use of communication big data and AI artificial intelligence technology to construct telecom fraud prevention behavior portrait, 2024, 18, 18724981, 2589, 10.3233/IDT-240386 | |
8. | Mohamad Zreik, 2024, chapter 4, 9798369311981, 55, 10.4018/979-8-3693-1198-1.ch004 | |
9. | Rafid Ullah, Hishamuddin Bin Ismail, Mohammad Tariqul Islam Khan, Ali Zeb, Nexus between Chat GPT usage dimensions and investment decisions making in Pakistan: Moderating role of financial literacy, 2024, 76, 0160791X, 102454, 10.1016/j.techsoc.2024.102454 | |
10. | Hao Zhao, Yu Wang, A Big Data-Driven Financial Auditing Method Using Convolution Neural Network, 2023, 11, 2169-3536, 41492, 10.1109/ACCESS.2023.3269438 | |
11. | Bilal Hassan Ahmed Khattak, Imran Shafi, Abdul Saboor Khan, Emmanuel Soriano Flores, Roberto García Lara, Md. Abdus Samad, Imran Ashraf, A Systematic Survey of AI Models in Financial Market Forecasting for Profitability Analysis, 2023, 11, 2169-3536, 125359, 10.1109/ACCESS.2023.3330156 | |
12. | Preeti Sharma, A. Shanker Prakash, Anjul Malhotra, 2024, Application of Advanced AI Algorithms for Fintech Crime Detection, 979-8-3503-7024-9, 1, 10.1109/ICCCNT61001.2024.10725857 | |
13. | Arun Agrawal, Saivya Bhadhouriya, Anand Kumar Pandey, Smriti Bhadoriya, Deshdeepak Shrivastava, Gaurav Dubey, Madhukar Dubey, Honey Sengar, Khemchand Shakywar, Vineet Shrivastava, 2024, chapter 13, 9798369361702, 363, 10.4018/979-8-3693-6170-2.ch013 | |
14. | Kudakwashe Maguraushe, Joanna Miriro Matanda, 2024, Chapter 15, 978-3-031-74049-7, 295, 10.1007/978-3-031-74050-3_15 | |
15. | Rahul Thakur, Jasneet Kaur, Harmanjeet Singh, 2024, Comparative Analysis of Artificial Intelligence Models in Stock Security Index Forecasting with Sentiment Analysis of Economic News, 979-8-3503-8747-6, 197, 10.1109/ICTACS62700.2024.10840980 | |
16. | Alejandro Ferrero, Gabriel Vásquez, Óscar Iván Franco, 2024, Creating a calculator for engineers with a graphical interface using python, 979-8-3315-3172-0, 1, 10.1109/CONIITI64189.2024.10854820 | |
17. | Exploring Artificial Intelligence and Data Science-Based Security and its Scope in IoT Use Cases, 2025, 11, 2149-9144, 10.22399/ijcesen.869 | |
18. | Nermin Nour El-din Farghaly, 2025, 978-1-83549-609-1, 123, 10.1108/978-1-83549-608-420251005 | |
19. | Peng Yang, Shanyue Jin, Does AI Adoption in Financial Management Enhance Corporate Risk Resilience?, 2025, 13, 2169-3536, 66211, 10.1109/ACCESS.2025.3560397 |