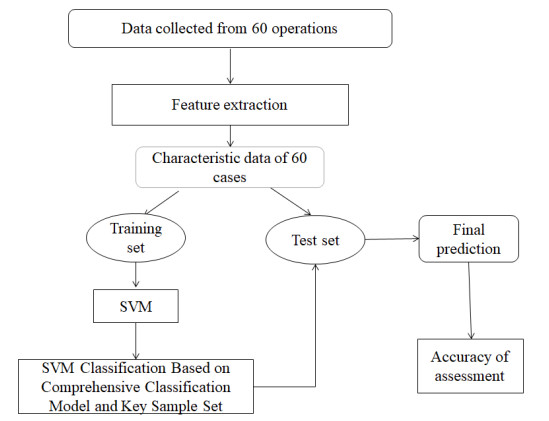
Citation: Jin Hwan Do. Apomorphine-induced pathway perturbation in MPP+-treated SH-SY5Y cells[J]. AIMS Molecular Science, 2017, 4(3): 271-287. doi: 10.3934/molsci.2017.3.271
[1] | Hasanen A. Hammad, Doha A. Kattan . Strong tripled fixed points under a new class of F-contractive mappings with supportive applications. AIMS Mathematics, 2025, 10(3): 5785-5805. doi: 10.3934/math.2025266 |
[2] | Monairah Alansari, Mohammed Shehu Shagari, Akbar Azam, Nawab Hussain . Admissible multivalued hybrid Z-contractions with applications. AIMS Mathematics, 2021, 6(1): 420-441. doi: 10.3934/math.2021026 |
[3] | Dong Ji, Yao Yu, Chaobo Li . Fixed point and endpoint theorems of multivalued mappings in convex b-metric spaces with an application. AIMS Mathematics, 2024, 9(3): 7589-7609. doi: 10.3934/math.2024368 |
[4] | Tahair Rasham, Muhammad Nazam, Hassen Aydi, Abdullah Shoaib, Choonkil Park, Jung Rye Lee . Hybrid pair of multivalued mappings in modular-like metric spaces and applications. AIMS Mathematics, 2022, 7(6): 10582-10595. doi: 10.3934/math.2022590 |
[5] | Tahair Rasham, Abdullah Shoaib, Shaif Alshoraify, Choonkil Park, Jung Rye Lee . Study of multivalued fixed point problems for generalized contractions in double controlled dislocated quasi metric type spaces. AIMS Mathematics, 2022, 7(1): 1058-1073. doi: 10.3934/math.2022063 |
[6] | Nehad Abduallah Alhajaji, Afrah Ahmad Noman Abdou, Jamshaid Ahmad . Application of fixed point theory to synaptic delay differential equations in neural networks. AIMS Mathematics, 2024, 9(11): 30989-31009. doi: 10.3934/math.20241495 |
[7] | Muhammad Tariq, Eskandar Ameer, Amjad Ali, Hasanen A. Hammad, Fahd Jarad . Applying fixed point techniques for obtaining a positive definite solution to nonlinear matrix equations. AIMS Mathematics, 2023, 8(2): 3842-3859. doi: 10.3934/math.2023191 |
[8] | Mustafa Mudhesh, Hasanen A. Hammad, Eskandar Ameer, Muhammad Arshad, Fahd Jarad . Novel results on fixed-point methodologies for hybrid contraction mappings in Mb-metric spaces with an application. AIMS Mathematics, 2023, 8(1): 1530-1549. doi: 10.3934/math.2023077 |
[9] | Rashid Ali, Faisar Mehmood, Aqib Saghir, Hassen Aydi, Saber Mansour, Wajdi Kallel . Solution of integral equations for multivalued maps in fuzzy b-metric spaces using Geraghty type contractions. AIMS Mathematics, 2023, 8(7): 16633-16654. doi: 10.3934/math.2023851 |
[10] | Azhar Hussain, Saman Yaqoob, Thabet Abdeljawad, Habib Ur Rehman . Multivalued weakly Picard operators via simulation functions with application to functional equations. AIMS Mathematics, 2021, 6(3): 2078-2093. doi: 10.3934/math.2021127 |
As a result of failure of the umbilical ring to close completely after birth, the umbilical hernia is one common abdominal hernia in adults, and occur in approximately one of six patients around the world [1,2]. So far, the true prevalence of umbilical hernias is still unknown, because it appears to be age and gender specific in international populations. As we all know, by the first month of infants, the umbilical hernias reach a maximal size, but most umbilical hernias can close spontaneously before one year old [3,4]. Therefore, despite their relatively high prevalence, the umbilical hernias in adults are poorly studied, especially among patients.
With respect to umbilical hernia repair, the majority of umbilical hernias can be corrected by elective surgical repair in day surgery center, for instance, the customary open surgery or laparoscopic operation which represents a minimally invasive treatment option [5]. It should be noted that the excellent visual exposure, minor incisions, fewer complications, comparable recurrence rates, quicker recovery, less pain, and fewer wound complications are the advantages of laparoscopic hernia repair compared with the traditional open approach [6,7]. On the basis of authoritative data, the total abdominal wall herniorrhaphies are nearly 1 million per year, and the umbilical hernias attribute approximately 175,000 [8]. Although umbilical herniorrhaphy is one common outpatient surgical procedure, it may be associated with significant postoperative complications [9,10]. During umbilical herniorrhaphy, the supraglottic airway devices (SADs) have been described as a missing link between facemask and endotracheal intubation [11,12,13]. And, the ProSeal Laryngeal Mask Airway (PLMA) is the modification of LMA classic and has been extensively studied [14]. Thanks to the presence of the posterior cuff and other potential advantages, PLMA has gained widespread popularity among anaesthetists [15,16].
However, to the best of our knowledge, the effectiveness of using a PLMA has not been fully evaluated in patients undergoing day surgery for herniorrhapy [17,18]. Therefore, this study aimed to examine the effectiveness of using PLMA in a day surgery center, and the authors designed this prospective randomized trial to collect all kinds of data during operation and classify them with SVM and compare the performance of PLMA and endotracheal tube (ETT) for patients who underwent herniorrhaphy [19,20,21]. The basic model of Support Vector Machine (SVM) is to find the best separating hyperplane in the feature space to maximize the interval between positive and negative samples in the training set. SVM is a supervised learning algorithm to solve binary classification problems. After introducing the kernel method, SVM can also be used to solve non-linear problems.
Generally, there are two kinds of SVM, Hard-spaced support vector machine (LSSVM) is a kind of support vector machine, which can obtain LSSVM by maximizing the hard-spaced when the training data are linearly separable. Soft-spaced support vector machine is a classification method of linear support vector machine by maximizing the soft-spaced when the training data are approximately linear separable. Nonlinear Support Vector Machine (NLSVM) is a kind of Support Vector Machine (SVM), which can obtain NLSVM by kernel method and maximizing the soft interval when the training data is linear and inseparable.
The main idea of SVM can be summarized as two points (1) it is aimed at the linear separable case. For the linear separable case, the linear non-separable sample in the low-dimensional input space is transformed into the high-dimensional feature space by using the non-linear mapping algorithm, so that the high-dimensional feature space can be linearly separable by using the linear algorithm to analyze the non-linear feature of the sample (2) SVM plays an important role in data processing of medical imaging and clinical medicine.
Data mining includes many methods such as machine learning, expert systems, and pattern recognition. Support Vector Machine (SVM) is a new machine learning method based on the theory of Vapnik-Chervonenkis dimension and the principle of structural risk minimization. It has applications in imaging medicine and laboratory medicine. Developments in recent years include: research on improved methods for SVM, such as least squares SVM [34], related SVM [34], mainly used to improve computing speed; select appropriate input features, or SVM and other The method is combined, such as combined with evidence theory [34], to improve the classification accuracy; SVM for different parameter training is integrated to reduce the number of missing judgments.
This paper proposes a new idea to improve the classification performance of SVM: Based on the key sample set, several SVM classification models with different feature quantities are constructed, and the classification results are synthesized. And the paper will first introduce the basic principles of SVM, then describe the construction method of SVM comprehensive classification model and the generation method of key sample sets. Then introduce the SVM classification step based on comprehensive classification model and key sample set and the index classification weight based on information entropy.
The support vector machine was first proposed by Cortes and Vapnik in 1995. It is a new machine learning method based on statistical learning theory, which follows the principle of structural risk minimization. It exhibits many unique advantages in solving small sample, nonlinear and high dimensional pattern recognition. The main idea of the support vector classification method is to find a hyperplane as the two types of segmentation planes in the high-dimensional space for the two-class problem, and to maximize the classification interval.
The support vector machine classification problem can be described as: given training set S = {(xi, yi)| xi∈Rn, yi∈ R}, solve Optimal classification hyperplane. Where xi is the i-th input feature The support vector machine classification problem can be described as: given training set S = {(xi, yi)| xi∈Rn, yi∈ R}, solve Optimal classification hyperplane. Where xi is the i-th input feature
(x)=sgn[(w∙x)]+b | (1) |
min||w||22+Cl∑i=1εis.t. yi[(w∙xi)+b]1−εi,i=1,2,…,lεi⪖0,1,2…,l |
Where h and b are the coefficient vector and the deviation term respectively; C is the penalty factor; εi is the relaxation variable.
Construct the Lagrangian function as follows:
L(w,b,ε,α,γ)=(w∙w)2+C∑li=1εi−∑li=1αi{yi[(w∙xi)+b]−l+εi}−∑li=1γiεi | (2) |
In the equation, αi and γi are Lagrangian multipliers. Can be transformed into a solution to its dual problem, ie
max∑li=1αi−∑li=1,j=1yiyjαiαj2(xi∙xj) | (3) |
s.t.0<=xαi<=xC,i=1,2…,l∑li=1αiyi=0 | (4) |
This is a problem of quadratic function optimization under inequality constraints, only a part of αi in the solution is not 0, and the corresponding sample is the support vector.
After obtaining a, b can
−maxi−yi=−l(w∙xi) | (5) |
+mini−yi=l(w∙xi)2 | (6) |
get. Obtaining the optimal classification function gives
f(x)=sgn[(w∙x)]+b | (7) |
sgn[∑li=1αiyi(x∙xi)+b | (8) |
When the data in the training set is linearly inseparable, it can be mapped to a high-dimensional space by the nonlinear mapping Φ: Rn→H, making it linearly separable in the high-dimensional space. Since the training algorithm in high-dimensional space only needs to perform inner product operation Φ(xi) •Φ(xi), when there is a function that satisfies
K(xi,xi)=Φ(xi)∙Φ(xi) | (9) |
The inner product of high dimensional space can be calculated by the function of the original space. Such a function is called a kernel function. The basic function of the kernel function is to accept the vector in the two low-dimensional spaces as input, and to calculate the inner product. Value of the vector in the high-dimensional space after a certain transformation.
Commonly used kernel functions are mainly
(1) Linear kernel function
K(x,y)=(x∙y) | (10) |
(2) Polynomial kernel function
K(x,y)=[b(x∙y)+c]d | (11) |
In the equation, b, c, and d are all constants.
(3) Radial basis kernel function
K(x,y)=exp−||x−y|22σ | (12) |
Where σ is the product of the number of sample input features and the width of the radial basis kernel function, which is a constant.
(4) Sigmoid kernel function
K(x,y)=tanh[b(x∙y)+c] | (13) |
Where b, c are constants.
After introducing the kernel function, equations (3) and (4) are respectively
max∑li=1αi−(x+a)n∑li=1yiαjαiαjK(xi,x) | (14) |
s.t.0<=xαi<=xC,i=1,2,…l∑li=1αiyi=0 | (15) |
f(x)=sgn[∑li=1αiyiK(xi,x)+b] | (16) |
The specific choice on which type of kernel function and how to choose the parameters in the kernel function are currently lacking specific operational principles. The test method can only be used in the application process. That is to say, different kernel functions and different parameter combinations are tested, and one of the better effects is selected according to the test results. In general, the radial basis kernel function is a kernel function that does not deviate too much.
The selection of the feature quantity is crucial for the SVM classification effect. When the feature quantity is large, the main features are sometimes masked, and the classification effect is not ideal. When the feature quantity is small, the system state may not be fully characterized, and the classification effect is not satisfactory. To this end, some scholars have studied genetic algorithm, simulated annealing, principal component analysis and other methods for feature quantity selection and feature extraction. The use of genetic algorithm and other intelligent optimization algorithms to select feature quantities has the disadvantages of slow calculation speed. Principal component analysis can replace the original with a small number of representative features without losing the main information of the original data and affecting the evaluation effect. Some input features significantly reduce the input space dimension, but lose the original physical meaning of the feature quantity.
This paper is inspired by the comprehensive idea of multiple different parameter classifiers in literature. Several SVM classification models with different feature quantities are constructed. According to the principle of conservation, the classification results of multiple classification models are integrated and avoided. The so-called conservative principle is to minimize the number of samples of the leak classification (determining unstable samples as stable). To this end, the classification results of multiple SVM classification models are summarized as follows: for a test sample, if one classification model is determined to be unstable, it is attributed to the unstable classification; only when all classification models are judged as when it is stable, it is attributed to the stable classification. This reduces the number of leak classifications at the expense of increased misclassification (determining stable samples as unstable). The reason for this is that the tolerance for leak classification is lower when performing a stable assessment, and the impact of miss classification on the superiority of surgical methods is much greater.
For each patient, the following demographic data was collected, including gender, age, and weight. In addition, monitoring of surgery time, heart rate, systolic blood pressure, diastolic blood pressure, mean arterial pressure, respiratory parameters (peak airway pressure, altitude airway pressure, mean airway pressure, end-tidal co2 and respiratory rate), respectively. In addition, secondary outcomes were postoperative complication rates associated with PLMA and tracheal catheters on day 7 postoperatively. The incidence of complications was recorded: nausea and vomiting, cough, sore throat, hoarseness and leakage.
Generally, the weight of the index that people understand refers to the "importance weight" of the indicator, which is the proportional distribution of the "importance degree" of the indicator, and the indicator "classification weight" refers to the "measurement" of the indicator value of the sample. The degree is that the weight information is uncertain, but in the case of the decision maker's bounded rationality, the use of the information output by the decision data itself to extract weights is a scientific and effective objective weighting method.
Let γ(ij) denote the index Ij (j = 1, 2, ..., m) for the classification of the hernia surgery Xi by different methods (referred to as the degree of discrimination), w (j i) for the indicator Ij classification weight for xenon surgery Xi performed by different methods. Consider the jth row vector of matrix (4) borrowing the concept and properties of information entropy, (μij1, μ (The degree of dispersion and concentration of each component in TF185) j2...μijp) is described as follows:
γ(i)j=1+1logp∑pk=1μijk∙log∑pk=1μijki=1,2,…,n;j=1,2,…m | (17) |
Known by the entropy:
If and only if μijk=1pμijk(p=1,2,…,p), γ(ij)takes a minimum value of 0;
γ(ij) takes a maximum value of 1 if and only if μijk = 1 remaining p - 1 μijk = 0 (l ≠k).
Moreover, when the value of μijk is more dispersed, γ(ij) is smaller; when the value of μijk is more concentrated, γ(ij) is larger, so γ (The value of TF210) reflects the degree of concentration and dispersion of μijk, which reflects the classification weight of index Ij.
w(i)j=γ(i)j∑mj=1γ(i)j(i=1,2,…,m) | (18) |
Since 0 ≤ w(ji) ≤ 1, w(ji) is the index weight of the indicator ij with respect to the sample.
Obviously, the indicator "classification weight" and the indicator "importance weight" are two different concepts and have essential differences. The indicator classification weight is the concept that is generated after the indicator measurement value is provided according to the sample measurement value of the sample. The classification information is calculated and calculated. This indicates that the index weight of the index is hidden in the actual observation data of the sample. It cannot be subjectively equation ted by experts, and the importance weight of the indicator has nothing to do with the measured value of the indicator.
After obtaining the patient's written informed consent in advance, The Second Affiliated Hospital of Fujian Medical University performed the study. According to the established selection and exclusion criteria, all possible eligible patients were strictly screened. A total of 60 patients met the following criteria: (1) 18 years of age or older; (2) diagnosed as umbilical hernia. The exclusion criteria are as follows: (1) Patients with coexisting respiratory diseases, congenital diseases or chronic drugs; (2) patients with upper respiratory tract infection on the day of surgery; (3) patients with expected airway difficulties; (4) patients with increased risk of aspiration Such as non-fasting state, hiatal hernia, lung disease and so on.
The 60 patients underwent hernia repair, including routine laparotomy or laparoscopic surgery. According to the random number table, eligible patients were randomly divided into two groups: group A received PLMA, group B received ETT as airway device. Researchers and statisticians turned a blind eye to randomized grouping when pediatric patients were included in the study. Specific selection criteria are as follows: (1) over 18 years old; (2) diagnosis of umbilical hernia. The exclusion criteria are as follows. (1) patients with respiratory diseases, congenital diseases or chronic drugs; (2) patients with upper respiratory tract infection on the day of operation; (3) patients with expected airway difficulties; (4) patients with increased inhalation risk per patient, such as non-fasting state, hiatal hernia, pulmonary disease, etc. The following demographic data were collected, including gender, age and weight. In addition, the operation time, heart rate, systolic blood pressure, diastolic blood pressure, mean arterial pressure, respiratory parameters (peak airway pressure, high altitude airway pressure, mean airway pressure, end tidal carbon dioxide and respiratory rate) were monitored. In addition, the second outcome was the incidence of complications associated with PLMA and tracheal intubation on the seventh day after surgery.
Before herniorrhaphy, each patient underwent comprehensive physical examinations and preanesthetic evaluation by the surgeons and anesthetists in The Second Affiliated Hospital of Fujian Medical University. All the patients were given tablet alprazolam (0.2 mg, orally) at bedtime on the previous night of surgery. On the day of surgery, the patients were fasted 6 h for solid food, 4 h for milk and 2 h for clear water. During the operation, the patient was placed in supine position, and 100% oxygen and sevoflurane (6%-8%) were utilized to induce general an aesthesia. And then, the standard monitoring instruments including three-lead electrocardiogram, pulse oximeter, and noninvasive blood pressure were connected. The monitoring indicators during operations were as follows. (1) non-invasive arterial blood pressure; (2) heart rate; (3) respiratory parameters, including peak airway pressure, plateau airway pressure, mean airway pressure, end-tidal CO2, and respiratory rate. And when the patients were under anesthesia, the medical engineering device PLMA and ETT were inserted by trained anesthesia providers with significant experience and expertise in insertion. Less than two insertion attempts were allowed before considering it as failure to insert the device. Afterwards, whether the PLMA and ETT devices were placed correctly was confirmed by manual ventilation, auscultation, and square wave capnography. And, the adequacy of ventilation was assessed based on two criteria: (1) No leakage with an airway pressure of 15 cm H2O, and (2) Bilateral chest excursion with a PIP of 20 cm H2O. After the surgery finished, the patients were followed up for 7 days to watch for postoperative complications, including nausea and vomiting, bucking, pharyngulas, hoarse, and leakage.
SPSS software version 16.0 (IBM Corp., Armonk, NY, USA) was utilized to carry out statistical analysis. Continuous variables are expressed as mean ± standard deviation, and categorical and ordinal variables are expressed as numbers and percentages. The Student's t-test was adopted to compare data between PLMA and endotracheal tube groups, and the Pearson's Chi-square test was used to compare postoperative complications between the two groups. P < 0.05 was considered statistically significant.
Sample set 1 of patients in ETT group and PLMA group was used as training sample set. Sample set 2 of patients was used as testing sample set. Sample set 1 was divided into five subsets according to the severity of complications after operation. For each sub-sample set, 10 sets of features are selected, and the radial basis function is used as the kernel function to construct the SVM classification model with penalty coefficient C = 100. In this way, a total of 5 × 10 × 10 classification models are obtained. Ten sets of feature quantities were included in 10 information collected in clinical trials. During the test, the classification results of 10 SVM classification models with different features should be synthesized according to the key sample sets and the corresponding effects of the test samples. Figure 2, 3 and 4 show the comparison of the classification results of the three folds, five folds and ten folds cross validation. From Table 3, 4 and 5, it can be seen that the proposed method based on SVM comprehensive classification model and key sample set has better classification effect. For 60 test samples, there are 17 misclassifications in Tri-Fold cross-validation, 12 misclassifications in five-fold cross-validation and 6 misclassification in ten-fold cross-validation. Generally speaking, the accuracy rate is higher.
Parameter | Baseline | Maximum |
Peak airway pressure (mmHg) | 12.48 ± 1.84 | 26.31 ± 1.39 |
Plateau airway pressure (mmHg) | 13.89 ± 1.20 | 19.88 ± 2.69 |
Mean airway pressure (mmHg) | 6.21 ± 1.19 | 8.99 ± 0.67 |
Group | Complications | Incidence | |||||
PONV* | Bucking | Pharyngalgia | Hoarseness | Leakage | Total | ||
ProSeal LMA | 2 | 0 | 2 | 1 | 1 | 6 | 20.00 % |
Endotracheal tube airway | 6 | 2 | 4 | 4 | 3 | 19 | 63.33 % |
Predicted | ||||
Cross validation | Good | Secondary | Wrong | |
Triple fold | Good | 20 | 6 | 4 |
Secondary | 1 | 10 | 2 | |
Wrong | 1 | 3 | 13 | |
Five folds | Good | 25 | 3 | 2 |
Secondary | 1 | 11 | 1 | |
Wrong | 2 | 3 | 12 | |
Ten folds | Good | 27 | 2 | 1 |
Secondary | 1 | 12 | 0 | |
Wrong | 1 | 1 | 15 |
The above results verify the validity of the method in data classification.A total of 60 patients undergoing hernia repair under general anesthesia were randomly divided into two groups, PLMA (N = 30) and ETT (N = 30), for airway management. Heart rate, systolic pressure, diastolic pressure, mean arterial pressure, respiratory parameters (peak airway pressure, high altitude airway pressure, mean airway pressure, end-expiratory CO2 and respiratory frequency), and postoperative complications associated with PLMA and PLMA were monitored for ETT (nausea and vomiting, vomiting, pharyngalgia, hoarseness and leakage), respectively.
There was no significant difference in systolic blood pressure trend between the two groups. Compared with PLMA group, the heart rate of ETT group was higher in the middle period (P < 0.05). In addition, the diastolic blood pressure in ETT group increased by 30% from baseline (P < 0.05), and the mean arterial pressure in ETT group increased by 24.3% from baseline (P < 0.05). In addition, compared with PLMA group, the maximum respiratory parameters such as peak airway pressure, platform airway pressure, mean airway pressure, end-expiratory carbon dioxide and respiratory frequency in ETT group increased (P < 0.05). Postoperative complications related to ProSeal LMA and ETT, such as nausea and vomiting, spasm, pharyngalgia, hoarseness and leakage, were 20.00% in PLMA group and 63.33% in ETT group (P < 0.05). For airway management, compared with traditional ETT, PLMA is a safe supraglottic airway device, which is suitable for patients undergoing hernia surgery. It has the advantages of stable heart rate, respiratory parameters and blood pressure, and fewer complications.
Thirty patients were included in each group. Demographic data of age, sex, weight was comparable between the two groups, as shown in Table 1. The majority of patients who required herniorrhaphy were males (N = 55), and no significant differences were found between the groups with regard to their demographic data. In terms of duration of operation, the time in PLMA group was much shorter than it in ETT group (39.69 ± 6.21 versus 98.52 ± 6.53, P < 0.05), as shown in Table 1.
ProSeal-LMA | Endotracheal tube | |
Age (year) | 55.97 ± 3.21 | 55.92 ± 3.89 |
Sex (male/female) | 28/2 | 27/3 |
Weight (kg) | 68.92 ± 4.59 | 67.84 ± 5.61 |
Parameter | Baseline | Maximum |
Peak airway pressure (mmHg) | 13.22 ± 1.99 | 19.21 ± 2.31 |
Plateau airway pressure (mmHg) | 14.23 ± 1.39 | 17.52 ± 2.68 |
Mean airway pressure (mmHg) | 6.32 ± 1.20 | 7.86 ± 0.78 |
As shown in Figure 1, the heart rate trend during operation was illustrated, PLMA group versus ETT group. It could be deduced that the heart rate was comparable in both the groups at the beginning and end period, but a considerable fluctuation of heart rate appeared at the intermediate stage in both groups, on account of the specific operative procedures.
It can be put forward that the systolic blood pressure increased by 5% from baseline in ETT group. And, on the other hand, the fluctuation range in PLMA group was lower than it in the ETT group, which indicated the systolic blood pressure in PLMA group was more stable during the whole operation. In addition, according to the data recorded during the operation, the diastolic blood pressure trend during operation was illustrated, PLMA group versus ETT group. It could be put forward that the diastolic blood pressure increased by 30% from baseline in ETT group. And, on the other hand, the fluctuation range in PLMA group was much lower than it in the ETT group, which indicated the diastolic blood pressure in PLMA group was more stable during the whole operation. In terms of mean arterial pressure trend, according to the data recorded during the operation, the mean arterial pressure trend during operation was illustrated, PLMA group versus ETT group. It could be put forward that the mean arterial pressure increased by 24.3% from baseline in ETT group. And, on the other hand, the fluctuation range in PLMA group was much lower than it in the ETT group, which indicated the mean arterial pressure in PLMA group was more stable during the whole operation. In a word, the PLMA helped to maintain the stability of blood pressure during the whole operation, when compared with ETT device.
The increase rates of peak airway pressure, plateau airway pressure, mean airway pressure, end-tidal CO2, and respiratory rate in PLMA group were 19.54 %, 20.31 %, 16.23 %, 19.21 %, and 42.89 %, respectively (P < 0.001). And the increase rates of peak airway pressure, plateau airway pressure, mean airway pressure, end-tidal CO2, and respiratory rate in ETT group were 38.41 %, 29.87 %, 18.52 %, 29.80 %, and 60.33 %, respectively (P < 0.001). From the data described above, it can be deduced that the PLMA device contributed to maintain steady breathing during the whole operation.
The total number of postoperative complications in PLMA group was 6, and the total number of postoperative complications in ETT group was 19. And, the incidence rates of complications in PLMA group and ETT group were 20.00 % and 63.33 %, respectively (P < 0.05).
Generally speaking, umbilical hernia refers to the exit of abdominal wall viscera through umbilical cord ring. From an anatomical point of view, the umbilical ring consists of umbilical scar, circular ligament and umbilical fascia [22,23]. Normally, the circular ligament passes through the upper edge of the umbilical cord ring and attaches to the lower edge [24,25]. However, if it only attaches to the upper edge of the ring, then the bottom of the umbilical ring is formed only by the umbilical fascia and peritoneum, so it will cause weakness, thus vulnerable to umbilical hernia [26]. As mentioned above, umbilical hernia is a common pathology and its incidence is estimated to be about 15% of that of abdominal wall hernia repair. In addition, they may become more pronounced during crying, tension and defecation, and may lead to prominent cysts and their contents [24]. Umbilical hernia is common, but many spontaneously subside within the first five years of life. Therefore, some surgeons recommend conservative treatment rather than traditional laparotomy and laparoscopic surgery. However, it is also pointed out that umbilical hernia may lead to serious complications, such as incarcerated and incarcerated hernia. Therefore, in clinical practice, for most symptomatic and asymptomatic umbilical hernia, timely surgical repair is recommended.
It is well known that the most basic aspect of hernioplasty is general sensation. Keeping the upper respiratory tract unobstructed is essential for the entire surgical operation [27,28]. Currently, PLMA and ETT are considered acceptable alternatives to airway management in patients undergoing hernioplasty [29,30]. Therefore, it is imperative to choose a more effective treatment method. Because of the wide application of support vector machines in clinical medicine, we try to verify the superiority of support vector machines in clinical practice and improve the performance of support vector machines through experimental methods.
Moreover, patients' airway management has evolved from traditional ETT to minimally invasive LMA [31]. As far as we know, the best ventilation parameters include proper chest movement, stable oxygenation and square wave coronary angiography [14,32,33]. Therefore, in order to obtain better data and train support vector machine for its future clinical application, we conducted these prospective randomized trials in The Second Affiliated Hospital of Fujian Medical University.
A total of 60 patients were enrolled in this study. The demographic data of age, sex and weight of the two groups were comparable. Firstly, data were collected by operation. In terms of operation time, PLMA group was significantly shorter than ETT group. In addition, it can be inferred that the heart rates of the two groups are comparable at the beginning and the end, but due to the specific surgical procedures, the heart rates of the two groups fluctuate considerably in the middle stage. According to the data, systolic blood pressure in PLMA group was more stable during the whole operation and diastolic blood pressure was more stable during the whole operation. From the trend of mean arterial pressure, the mean arterial pressure in PLMA group was more stable during the whole operation. In conclusion, compared with ETT devices, PLMA helps maintain blood pressure stability throughout the operation.
After collecting reliable data, according to the method of index classification weight, the data are classified and feature extraction is carried out. The unascertained measure evaluation model satisfies the "normality" and "additivity", so the evaluation result is credible. In addition, the unascertained measure model pays attention to the "orderliness" of the evaluation space, gives a reasonable confidence, and trains and tests the subsequent SVM. Trial and evaluation provide reliable data. Compared with the traditional SVM method, the classification accuracy is increased and the number of missing classes is greatly reduced, which improves the practicability of the SVM method in stability assessment. As shown in Table 5, 6, 7 and Figure 4.
We conclude that the advantages and disadvantages of SVM in airway management and other clinical applications of hernioplasty under general anesthesia with PLMA and ETT are acceptable. The results of this study prove the superiority and practicability of SVM in data classification. In this experiment, PLMA is superior to ETT in terms of more stable heart rate, respiratory parameters, blood pressure and fewer complications.
This study was approved by the Research Ethics Committee in The Second Affiliated Hospital of Fujian Medical University. All ethical procedures conformed with the principles of 1964 Declaration of Helsinki and its latest 2008 amendments. Written informed consent was obtained from all participants beforehand.
ZSD and QMW contributed to the study design and overall coordination of the project. QWY, MXQ, HF. He was involved in the project implementation and data collection. ZSD, ZYC, QFH, QMW, ZPZ, QHL, LYH, YJH contributed to the data analysis, article writing and modification. All the authors checked and approved the final manuscript.
The authors acknowledge the cooperation and support of the patients, their parents and medical staffs in the Second Affiliated Hospital of Fujian Medical University.
The datasets used or analysed during the current study are available from the corresponding author upon reasonable request.
The study was done after agreement from thepatients' informed consent.
The authors declared that there was no conflict of interest in this study.
[1] |
Ribarič S (2012) The pharmacological properties and therapeutic use of apomorphine. Molecules 17: 5289-5309. |
[2] |
Deleu D, Hanssens Y, Northway MG (2004) Subcutaneous apomorphine: an evidence-based review of its use in Parkinson's disease. Drugs Aging 21: 687-709. doi: 10.2165/00002512-200421110-00001
![]() |
[3] |
Sydow O (2008) Parkinson's disease: recent development in therapies for advanced disease with a focus on deep brain stimulation (DBS) and duodenal levodopa infusion. FEBS J 275: 1370-1376. doi: 10.1111/j.1742-4658.2008.06295.x
![]() |
[4] |
Haeri M, Sarbaz Y, Gharibzadeh S (2005) Modeling the Parkinson's tremor and its treatments. J Theor Biol 236: 311-322. doi: 10.1016/j.jtbi.2005.03.014
![]() |
[5] |
Antonini A, Isaias IU, Rodolfi G, et al. (2011) A 5-year prospective assessment of advanced Parkinson disease patients treated with subcutaneous apomorphine infusion or deep brain stimulation. J Neurol 258: 579-585. doi: 10.1007/s00415-010-5793-z
![]() |
[6] |
Carron R, Fraix V, Maineri C, et al. (2011) High frequency deep brain stimulation of the subthalamic nucleus versus continuous subcutaneous apomorphine infusion therapy: A review. J Neural Transm 118: 915-924. doi: 10.1007/s00702-010-0556-7
![]() |
[7] |
De Gaspari D, Siri C, Landi A, et al. (2006) Clinical and neuropsychological follow up at 12 months in patients with complicated Parkinson's disease treated with subcutaneous apomorphine infusion or deep brain stimulation of the subthalamic nucleus. J Neurol Neurosurg Psychiatry 77: 450-453. doi: 10.1136/jnnp.2005.078659
![]() |
[8] |
Merello M, Lees AJ, Balej J, et al. (1999) GPi firing rate modification during beginning-of-dose motor deterioration following acute administration of apomorphine. Mov Disord 14: 481-483. doi: 10.1002/1531-8257(199905)14:3<481::AID-MDS1015>3.0.CO;2-H
![]() |
[9] |
Gassen M, Glinka Y, Pinchasi B, et al. (1996) Apomorphine is a highly potent free radical scavenger in rat brain mitochondrial fraction. Eur J Pharmacol 308: 219-225. doi: 10.1016/0014-2999(96)00291-9
![]() |
[10] | Grunblatt E, Mandel S, Gassen M, et al. (1999) Potent neuroprotective and antioxidant activity of apomorphine in MPTP and 6-hydroxydopamine induced neurotoxicity. J Neural Transm Suppl 55: 57-70. |
[11] |
Do JH (2014) Neurotoxin-induced pathway perturbation in human neuroblastoma SH-EP cells. Mol Cells 37: 672-684. doi: 10.14348/molcells.2014.0173
![]() |
[12] |
Kim IS, Choi DK, Do JH (2013) Genome-wide temporal responses of human neuroblastoma SH-SY5Y cells to MPP+ neurotoxity. BioChip J 7: 247-257. doi: 10.1007/s13206-013-7308-3
![]() |
[13] | Nakamura K, Bindokas VP, Marks JD, et al. (2000) The selective toxicity of 1-methyl-4-phenylpyridinium to dopaminergic neurons: The role of mitochondria complex I and reactive oxygen species revisited. Mol Pharmacol 58: 271-278. |
[14] | Lotharius J, O'Malley KL (2000) The parkinsonisminducing drug 1-methyl-4-phenylpyridinium triggers intracellular dopamine oxidation. A novel mechanism of toxicity. J Biol Chem 275: 38581-38588. |
[15] |
Moon HE, Paek SH (2015) Mitochondrial dysfunction in Parkinson's disease. Exp Neurobiol 24: 103-116. doi: 10.5607/en.2015.24.2.103
![]() |
[16] |
Choi DK, Kim IS, Do JH (2014) Signaling pathway analysis of MPP+-treated human neuroblastoma SH-SY5Y cells. Biotechnol Bioprocess Eng 19: 332-340. doi: 10.1007/s12257-013-0754-x
![]() |
[17] |
Du P, Kibbe WA, Lin SM (2008) Lumi: a pipeline for processing Illumina microarray. Bioinformatics 24: 1547-1548. doi: 10.1093/bioinformatics/btn224
![]() |
[18] |
Conesa A, Nueda MN, Ferrer A, et al. (2006) maSigPro: a method to identify significantly differential expression profiles in time-course microarray experiments. Bioinformatics 22: 1096-1102. doi: 10.1093/bioinformatics/btl056
![]() |
[19] |
Yu G, Wang LG, Han Y, et al. (2012) clusterProfiler: an R package for comparing biological themes among clusters. Omics 16: 284-287. doi: 10.1089/omi.2011.0118
![]() |
[20] |
Tarca AL, Draghici S, Khatri P, et al. (2009) A novel signaling pathway impact analysis. Bioinformatics 25: 75-82. doi: 10.1093/bioinformatics/btn577
![]() |
[21] | Csárdi G, Nepusz T (2006) The igraph software package for complex network research. Inter J Complex Syst 1695. |
[22] |
Pepe D, Do JH (2015) Estimation of dysregulated pathway regions in MPP+ treated human neuroblastoma SH-EP cells with structural equation model. BioChip J 9: 131-138. doi: 10.1007/s13206-015-9206-3
![]() |
[23] | Pepe D, Do JH (2016) Comparison of perturbed pathways in two different cell models for Parkinson's disease with structural equation model. J Comput Biol 23: 90-101. |
[24] |
Newman ME, Girvan M (2004) Finding and evaluating community structure in networks. Phys Rev E Stat Nonlin Soft Matter Phys 69: 026113. doi: 10.1103/PhysRevE.69.026113
![]() |
[25] |
Benjamini Y, Yekutieli D (2001) The control of the false discovery rate in multiple testing under dependency. Ann Stat 29: 1165-1188. doi: 10.1214/aos/1013699998
![]() |
[26] |
Yu G, Wang KG, Yan GR, et al. (2015) DOSE: an R/Bioconductor package for disease ontology semantic and enrichment analysis. Bioinformatics 31: 608-609. doi: 10.1093/bioinformatics/btu684
![]() |
[27] | Okun MS, Foote KD (2010) Parkinson's disease DBS: what, when, who and why? The time has come to tailor DBS targets. Expert Rev Neurother 10: 1847-1857. |
1. | Mohammed Shehu Shagari, Trad Alotaibi, OM Kalthum S. K. Mohamed, Arafa O. Mustafa, Awad A. Bakery, On existence results of Volterra-type integral equations via C∗-algebra-valued F-contractions, 2023, 8, 2473-6988, 1154, 10.3934/math.2023058 |
Parameter | Baseline | Maximum |
Peak airway pressure (mmHg) | 12.48 ± 1.84 | 26.31 ± 1.39 |
Plateau airway pressure (mmHg) | 13.89 ± 1.20 | 19.88 ± 2.69 |
Mean airway pressure (mmHg) | 6.21 ± 1.19 | 8.99 ± 0.67 |
Group | Complications | Incidence | |||||
PONV* | Bucking | Pharyngalgia | Hoarseness | Leakage | Total | ||
ProSeal LMA | 2 | 0 | 2 | 1 | 1 | 6 | 20.00 % |
Endotracheal tube airway | 6 | 2 | 4 | 4 | 3 | 19 | 63.33 % |
Predicted | ||||
Cross validation | Good | Secondary | Wrong | |
Triple fold | Good | 20 | 6 | 4 |
Secondary | 1 | 10 | 2 | |
Wrong | 1 | 3 | 13 | |
Five folds | Good | 25 | 3 | 2 |
Secondary | 1 | 11 | 1 | |
Wrong | 2 | 3 | 12 | |
Ten folds | Good | 27 | 2 | 1 |
Secondary | 1 | 12 | 0 | |
Wrong | 1 | 1 | 15 |
ProSeal-LMA | Endotracheal tube | |
Age (year) | 55.97 ± 3.21 | 55.92 ± 3.89 |
Sex (male/female) | 28/2 | 27/3 |
Weight (kg) | 68.92 ± 4.59 | 67.84 ± 5.61 |
Parameter | Baseline | Maximum |
Peak airway pressure (mmHg) | 13.22 ± 1.99 | 19.21 ± 2.31 |
Plateau airway pressure (mmHg) | 14.23 ± 1.39 | 17.52 ± 2.68 |
Mean airway pressure (mmHg) | 6.32 ± 1.20 | 7.86 ± 0.78 |
Parameter | Baseline | Maximum |
Peak airway pressure (mmHg) | 12.48 ± 1.84 | 26.31 ± 1.39 |
Plateau airway pressure (mmHg) | 13.89 ± 1.20 | 19.88 ± 2.69 |
Mean airway pressure (mmHg) | 6.21 ± 1.19 | 8.99 ± 0.67 |
Group | Complications | Incidence | |||||
PONV* | Bucking | Pharyngalgia | Hoarseness | Leakage | Total | ||
ProSeal LMA | 2 | 0 | 2 | 1 | 1 | 6 | 20.00 % |
Endotracheal tube airway | 6 | 2 | 4 | 4 | 3 | 19 | 63.33 % |
Predicted | ||||
Cross validation | Good | Secondary | Wrong | |
Triple fold | Good | 20 | 6 | 4 |
Secondary | 1 | 10 | 2 | |
Wrong | 1 | 3 | 13 | |
Five folds | Good | 25 | 3 | 2 |
Secondary | 1 | 11 | 1 | |
Wrong | 2 | 3 | 12 | |
Ten folds | Good | 27 | 2 | 1 |
Secondary | 1 | 12 | 0 | |
Wrong | 1 | 1 | 15 |
ProSeal-LMA | Endotracheal tube | |
Age (year) | 55.97 ± 3.21 | 55.92 ± 3.89 |
Sex (male/female) | 28/2 | 27/3 |
Weight (kg) | 68.92 ± 4.59 | 67.84 ± 5.61 |
Parameter | Baseline | Maximum |
Peak airway pressure (mmHg) | 13.22 ± 1.99 | 19.21 ± 2.31 |
Plateau airway pressure (mmHg) | 14.23 ± 1.39 | 17.52 ± 2.68 |
Mean airway pressure (mmHg) | 6.32 ± 1.20 | 7.86 ± 0.78 |