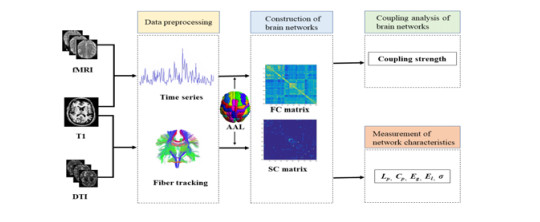
The coupling between functional and structural brain networks is difficult to clarify due to the complicated alterations in gray matter and white matter for the development of Alzheimer's disease (AD). A cohort of 112 participants [normal control group (NC, 62 cases), mild cognitive impairment group (MCI, 31 cases) and AD group (19 cases)], was recruited in our study. The brain networks of rsfMRI functional connectivity (rsfMRI-FC) and diffusion tensor imaging structural connectivity (DTI-SC) across the three groups were constructed, and their correlations were evaluated by Pearson's correlation analyses and multiple comparison with Bonferroni correction. Furthermore, the correlations between rsfMRI-SC/DTI-FC coupling and four neuropsychological scores of mini-mental state examination (MMSE), clinical dementia rating-sum of boxes (CDR-SB), functional activities questionnaire (FAQ) and montreal cognitive assessment (MoCA) were inferred by partial correlation analyses, respectively. The results demonstrated that there existed significant correlation between rsfMRI-FC and DTI-SC (p < 0.05), and the coupling of rsfMRI-FC/DTI-SC showed negative correlation with MMSE score (p < 0.05), positive correlations with CDR-SB and FAQ scores (p < 0.05), and no correlation with MoCA score (p > 0.05). It was concluded that there existed FC/SC coupling and varied network characteristics for rsfMRI and DTI, and this would provide the clues to understand the underlying mechanisms of cognitive deficits of AD.
Citation: Xia Xu, Song Xu, Liting Han, Xufeng Yao. Coupling analysis between functional and structural brain networks in Alzheimer's disease[J]. Mathematical Biosciences and Engineering, 2022, 19(9): 8963-8974. doi: 10.3934/mbe.2022416
[1] | Zhengtao Xi, Tongqiang Liu, Haifeng Shi, Zhuqing Jiao . Hypergraph representation of multimodal brain networks for patients with end-stage renal disease associated with mild cognitive impairment. Mathematical Biosciences and Engineering, 2023, 20(2): 1882-1902. doi: 10.3934/mbe.2023086 |
[2] | Wei Yin, Tao Yang, GuangYu Wan, Xiong Zhou . Identification of image genetic biomarkers of Alzheimer's disease by orthogonal structured sparse canonical correlation analysis based on a diagnostic information fusion. Mathematical Biosciences and Engineering, 2023, 20(9): 16648-16662. doi: 10.3934/mbe.2023741 |
[3] | Wei Kong, Feifan Xu, Shuaiqun Wang, Kai Wei, Gen Wen, Yaling Yu . Application of orthogonal sparse joint non-negative matrix factorization based on connectivity in Alzheimer's disease research. Mathematical Biosciences and Engineering, 2023, 20(6): 9923-9947. doi: 10.3934/mbe.2023435 |
[4] | Dominique Duncan, Thomas Strohmer . Classification of Alzheimer's disease using unsupervised diffusion component analysis. Mathematical Biosciences and Engineering, 2016, 13(6): 1119-1130. doi: 10.3934/mbe.2016033 |
[5] | Atsushi Kawaguchi . Network-based diagnostic probability estimation from resting-state functional magnetic resonance imaging. Mathematical Biosciences and Engineering, 2023, 20(10): 17702-17725. doi: 10.3934/mbe.2023787 |
[6] | Yujie Kang, Wenjie Li, Jidong Lv, Ling Zou, Haifeng Shi, Wenjia Liu . Exploring brain dysfunction in IBD: A study of EEG-fMRI source imaging based on empirical mode diagram decomposition. Mathematical Biosciences and Engineering, 2025, 22(4): 962-987. doi: 10.3934/mbe.2025035 |
[7] | Zhi Yang, Kang Li, Haitao Gan, Zhongwei Huang, Ming Shi, Ran Zhou . An Alzheimer's Disease classification network based on MRI utilizing diffusion maps for multi-scale feature fusion in graph convolution. Mathematical Biosciences and Engineering, 2024, 21(1): 1554-1572. doi: 10.3934/mbe.2024067 |
[8] | Xiang Li, Jinyu Cong, Kunmeng Liu, Pingping Wang, Min Sun, Benzheng Wei . Aberrant intrinsic functional brain topology in methamphetamine-dependent individuals after six-months of abstinence. Mathematical Biosciences and Engineering, 2023, 20(11): 19565-19583. doi: 10.3934/mbe.2023867 |
[9] | Zijian Wang, Yaqin Zhu, Haibo Shi, Yanting Zhang, Cairong Yan . A 3D multiscale view convolutional neural network with attention for mental disease diagnosis on MRI images. Mathematical Biosciences and Engineering, 2021, 18(5): 6978-6994. doi: 10.3934/mbe.2021347 |
[10] | Song Xu, Xufeng Yao, Liting Han, Yuting Lv, Xixi Bu, Gan Huang, Yifeng Fan, Tonggang Yu, Gang Huang . Brain network analyses of diffusion tensor imaging for brain aging. Mathematical Biosciences and Engineering, 2021, 18(5): 6066-6078. doi: 10.3934/mbe.2021303 |
The coupling between functional and structural brain networks is difficult to clarify due to the complicated alterations in gray matter and white matter for the development of Alzheimer's disease (AD). A cohort of 112 participants [normal control group (NC, 62 cases), mild cognitive impairment group (MCI, 31 cases) and AD group (19 cases)], was recruited in our study. The brain networks of rsfMRI functional connectivity (rsfMRI-FC) and diffusion tensor imaging structural connectivity (DTI-SC) across the three groups were constructed, and their correlations were evaluated by Pearson's correlation analyses and multiple comparison with Bonferroni correction. Furthermore, the correlations between rsfMRI-SC/DTI-FC coupling and four neuropsychological scores of mini-mental state examination (MMSE), clinical dementia rating-sum of boxes (CDR-SB), functional activities questionnaire (FAQ) and montreal cognitive assessment (MoCA) were inferred by partial correlation analyses, respectively. The results demonstrated that there existed significant correlation between rsfMRI-FC and DTI-SC (p < 0.05), and the coupling of rsfMRI-FC/DTI-SC showed negative correlation with MMSE score (p < 0.05), positive correlations with CDR-SB and FAQ scores (p < 0.05), and no correlation with MoCA score (p > 0.05). It was concluded that there existed FC/SC coupling and varied network characteristics for rsfMRI and DTI, and this would provide the clues to understand the underlying mechanisms of cognitive deficits of AD.
Alzheimer's disease (AD) is one kind of neurodegenerative disease, which is clinically accompanied by typical progressive cognitive decline and behavioral impairments. It not only affects the daily life of patients but also brings a heavy burden on families, public healthcare systems and society. The AD affected more than 15% of people aged 65 years and older and its annual healthcare cost had reached more than $ 300 billion worldwide [1].
Alzheimer's changes typically begin in the human brain with the slow accumulation of abnormal structures, namely plaques and tangles, which was suspected to be the prime contributor to the damage and death of nerve cells in the human brain. The plaques in extracellular β-amyloid protein and tangles in intracellular twisted fibers of tau protein somehow played an important role in blocking the communication among nerve cells and disrupting processes needed for cell survival [2]. Previous studies confirmed that these pathological changes started decades ago before the first signs of memory loss [3].
Besides the structural MRI, the diffusion tensor imaging (DTI) and resting-state functional MRI (rsfMRI) had been proposed to evaluate the brain morphological and functional changes. Recently, the DTI and rsfMRI brain networks based on graph theory had been applied in the AD studies. According to the structural connectivity (SC) and functional connectivity (FC), the human brain was assumed as one assembly of highly interconnected networks or connectomes. The brain networks of SC were reconstructed by abundant cortical-cortical and subcortical WM axonal pathways, while the brain FC networks in vivo mapped the statistical connections between the cortical nodes in the complex neural systems, i.e., functional connectome [4,5]. The graph-based network analyses promised to reliably quantify brain SC and FC with a cluster of neurobiologically meaningful and easily computable brain network measures. Therefore, the relevant studies revealed that the capability of information transmission across brain networks could be assessed by common network characteristics of path length (Lp), clustering coefficient (Cp), global efficiency (Eg), local efficiency (El) and small-worldness (σ) [6,7,8].
DTI-SC networks demonstrated unique values in the evaluation of AD [9]. The global DTI-SC demonstrated that there were increased Lp and reduced Eg for AD [10]. Moreover, the DTI-SC within the intra-occipital lobe gradually became weakened during the progression of AD [11]. Another study found that the hemispheric asymmetry of brain networks could be taken as a potential biomarker for AD [12]. Again, WM seeded networks had approximately more than 400 connections and presented stronger connections than GM seeded networks for both AD and control groups [13]. Accordingly, the network of rsfMRI-FC showed specific characteristics of random network topology with powerless connections at anterior, posterior, and prefrontal brain regions. Nevertheless, the FC at the occipital and frontal lobes did not change with significant large-scale SC alterations during the transition from mild cognitive impairment (MCI) to AD [14,15,16,17,18]. Related studies verified that the cognitive and memory impairments were strongly correlated with the decreased σ, increased Lp and Cp for AD [19].
Till now, the analysis of FC-SC coupling based on the graph theory has become a hot spot of brain studies. Since the default mode network (DMN) had been highlighted as a large-scale network of brain regions that were highly correlated with each other in terms of neural activity and the changes within this network had been described in healthy aging as well as in AD [20]. It was proved that the core regions of the DMN were associated with brain regions having memory function [21]. At the mode of DMN, the coupling strength between DTI-SC and rsfMRI-FC was significantly correlated, and the comparison of brain FC and SC networks for healthy participants indicated that pairs of nodes had stronger SCs and FCs [22]. The decreased coupling of FC-SC might indicate more stringent and less dynamic brain function for AD [23]. The coupling between rsfMRI-FC and DTI-SC was obviously increased for the connections of DMN in AD [24]. It was evident that the correlations between FC and SC could provide more comprehensive and sensitive biomarkers, and helped to reveal the variations of network connectivity and functional interactivity of AD [25,26].
In our study, an optimized scheme for brain network coupling was proposed with the aim to investigate the coupling correlation between DTI-SC and rsfMRI-FC. Via the measured brain network characteristics, the coupling of rsfMRI-FC/DTI-SC was evaluated by Pearson's correlation analyses and multiple comparison with Bonferroni correction. Furthermore, the correlations between the rsfMRI-FC/DTI-SC coupling and four neuropsychological scores, including mini-mental state examination (MMSE), clinical dementia rating-sum of boxes (CDR-SB), functional activities questionnaire (FAQ) and montreal cognitive assessment (MoCA), were inferred by partial correlation analyses, respectively. This study would provide new insights to explore the changes in FC-SC coupling during AD progression.
A cohort of 112 participants enrolled from the the Alzheimer's disease neuroimaging initiative (ADNI) database was divided into three groups: NC (62 cases), MCI (31 cases) and AD (19 cases). The demographic and neuropsychological information of the participants is shown in Table 1. The inclusion criteria were listed as follows: (1) age between 55 and 90 years, (2) visual and auditory acuity sufficient for neuropsychological testing, (3) good general health and no diseases that could interfere with testing, (4) at least sixth-grade education or good work history (excluding mental retardation), (5) no contraindications to MRI, and (6) all participants who were willing and able to take part in relevant follow-up studies, including blood collection for genomic analysis and biomarker testing, cerebrospinal fluid collection and neuropsychological testing.
Three MR protocols of rsfMRI, DTI and T1-weighted imaging (T1WI) were conducted by Siemens 3.0T MR scanners. The rsfMRI scans were acquired using echo planar imaging sequence with the following parameters: flip angle (FA) = 90°; time of repetition (TR) = 3000 ms; time of echo (TE) = 30 ms; slice thickness = 3.4 mm; pixel spacing = 3.4 × 3.4 mm2; matrix size = 448 × 448; and time points = 197. The imaging parameters for DTI were listed as follows: gradient directions = 54 (94 cases) and 30 (18 cases); FA = 90°; TR = 7200/12, 400 ms; TE = 56/95 ms; slice thickness = 2.0 mm; pixel size = 2.0 × 2.0 mm2; and matrix size = 1044 × 1044. The main imaging parameters for T1WI were: FA = 90°; TR = 2300 ms; TE = 3 ms; slice thickness = 1.0 mm; voxel size = 1.0 × 1.0 mm2; and matrix size = 240 × 256.
The pipeline of brain network coupling of rsfMRI-FC/DTI-SC mainly involved four steps: data preprocessing, construction of brain networks, coupling analysis of brain networks and measurement of network characteristics, as shown in Figure 1.
For the construction of rsfMRI and DTI-SC networks, the data preprocessing of rsfMRI and T1WI was performed by the package of data processing assistant for resting-state fMRI (DPARSF) [27]; and the preprocessing of DTI and T1WI was conducted via the software of pipeline for analyzing brain diffusion images (PANDA) [28], respectively.
The preprocessing of rsfMRI data included: removal of the first 10 time points, time correction, correction of head movement, regression of interference signal, normalization of voxel size to 3 × 3 × 3 mm3, spatial smoothing with a kernel of 4 × 4 × 4 mm3 and high-pass filtering (0.01-0.1 Hz). Participants were excluded in cases of head translation greater than 3 mm and head rotation greater than 3°. At the preprocessing of DTI data, it consisted of format conversion [(digital imaging and communications in medicine) DICOM to (neuroimaging informatics technology initiative) NIFTI], resampling, removal of brain tissues, segmentation, local diffusion homogeneity, eddy current calibration, standardization, smoothing and deterministic fiber tracking (DFT). During the DFT, the tracking termination criteria were set as: FA < 0.2 or tracking angle > 45° [29].
The construction of brain networks of rsfMRI-FC and DTI-SC mainly composed of three steps: brain parcellation, definition of brain nodes and edges, and connection of brain nodes via edges. Firstly, the brain MR images were parcellated into 90 cerebral regions by the atlas of anatomical automatic labeling (AAL) from montreal neurological institute (MNI) [30]. Secondly, the nodes and edges of the rsfMRI-FC network were defined by extracting the time series of the 90 cerebral regions and the normalized correlation matrix with Fisher Z-transformation was constructed by Pearson's correlation coefficients [31]. Similarly, the nodes and edges of the DTI-SC network were defined as mentioned above. The T1WI images registered to the original B0 image were mapped to the MNI space by nonlinear transformation. Then, the AAL template was inverted from the MNI space to the original DTI space with the implementation of nearest neighbor interpolation. Here, the product of fractional anisotropy (FA) matrix and fiber number (FN) matrix was used to define the nodes and edges. To reduce the effect of pseudo-junctions during whole-brain fiber tracking, only the nodes connected with fiber bundles (edges) having more than three fibers were regarded as effective connections. Thirdly, the non-directional and weighted brain networks were delineated by defined nodes and edges.
At the DMN, 32 common brain regions of rsfMRI-FC and DTI-SC were selected as interested nodes to reconstruct the functional and structural connection matrices. The two FC and SC matrices were quantitatively evaluated by the metric of coupling strength.
Five brain network characteristics, including path length (Lp), clustering coefficient (Cp), global efficiency (Eg), local efficiency (El) and small-worldness (σ), were measured for evaluating the two brain networks across there groups. The definition of network characteristics was summarized as follows: (1) σ reflected the transmission efficiency of human brain network, (2) Eg quantified the efficiency of parallel information transfer for global brain network, (3) El denoted the mean value of the sums of neighboring sub-networks from Eg, (4) Lp represented the shortest path length between brain neighboring nodes, and (5) Cp indicated the degree of node clustering. The network characteristics of rsfMRI-FC and DTI-SC were measured by GRETNA (https://www.nitrc.org/projec-ts/gretna/) [32] at the sparsity threshold of 0.05-0.4 with stable step size of 0.01.
The statistical analyses were performed in four parts: demographic evaluation, brain network characteristic analyses, coupling analysis of brain networks and evaluation of network coupling with neuropsychological scores. The statistical analyses were performed using the software of SPSS [33]. The independent-sample t tests were performed to assess the statistical difference for any two groups among NC, MCI and AD. The p value < 0.05 indicated statistically significant difference for all tests.
Firstly, the differences of demographic and clinical variables were evaluated. The chi-square tests were used for the gender variable, and the variance analyses were used for other variables. Secondly, the brain network characteristics of rsfMRI-FC and DTI-SC were also compared. The network characteristics of rsfMRI-FC and DTI-SC were evaluated by variance analyses. If the results were significantly different between two groups, the Bonferroni tests would be further used to evaluate the differences. Thirdly, the coupling strength between rsfMRI-FC and DTI-SC were evaluated by Pearson's correlation analyses and multiple comparison with Bonferroni correction. Especially, the area under the curves (AUC) derived from receiving operating characteristic curves were used for the intergroup comparison of rsfMRI-FCs because of the sensitivity for detecting topological differences in brain FC networks [34]. Finally, the correlations between the coupling of rsfMRI-FC/DTI-SC and neuropsychological scores (MMSE, CDR-SB, FAQ and MoCA) were assessed by the partial correlation analyses, respectively.
It was clear that there were no significant differences for age and gender ratio, and significant differences for the education level among the three groups. Furthermore, the scores of MMSE, CDR-SB, FAQ and MoCA presented significant differences across the three groups (Table 1).
Variables | NC (n = 62) | MCI (n = 31) | AD (n = 19) | p value |
Age (year) | 71.75 ± 6.47 | 70.71 ± 7.77 | 74.8 ± 8.49 | 0.154 |
Gender (M/F) | 24/38 | 16/15 | 10/9 | 0.371 |
Education (year) | 16.77 ± 2.29 | 15.65 ± 2.81 | 15.21 ± 2.50 | 0.024 |
MMSE | 28.74 ± 1.23 | 28.04 ± 1.43 | 22.04 ± 2.46 | < 0.050 |
CDR-SB | 0.11 ± 0.23 | 1.29 ± 0.85 | 5.01 ± 1.58 | < 0.050 |
FAQ | 0.15 ± 0.35 | 4.13 ± 3.97 | 15.28 ± 7.04 | < 0.050 |
MoCA | 25.78 ± 2.61 | 22.82 ± 2.87 | 15.96 ± 4.45 | < 0.050 |
Abbreviations: MMSE: mini-mental state exam; CDR-SB: clinical dementia rating-sum of boxes; FAQ: functional activities questionnaire; MoCA: montreal cognitive assessment. |
In Table 2, the brain network characteristics of rsfMRI-FC demonstrated that the σ and Eg of NC group were greater than those of MCI and AD groups, but the Lp of NC group was significantly lower than those of MCI and AD groups. Moreover, there existed significant differences for Lp and Eg between NC and AD groups and between NC and MCI groups, and no statistically significant differences were observed for all network characteristics between MCI and AD groups.
Network characteristics | NC | MCI | AD | p value | ||
NC:MCI | MCI:AD | NC:AD | ||||
Lp | 0.761 ± 0.026 | 0.775 ± 0.030 | 0.791 ± 0.037 | 0.021* | 0.103 | 0.003* |
Cp | 0.198 ± 0.009 | 0.199 ± 0.009 | 0.201 ± 0.009 | 0.886 | 0.310 | 0.230 |
Eg | 0.180 ± 0.004 | 0.178 ± 0.004 | 0.178 ± 0.006 | 0.006* | 1.000 | 0.035* |
El | 0.256 ± 0.007 | 0.254 ± 0.007 | 0.256 ± 0.007 | 0.189 | 0.178 | 0.681 |
σ | 0.699 ± 0.085 | 0.648 ± 0.099 | 0.689 ± 0.101 | 0.012* | 0.173 | 0.664 |
*Note: *p < 0.05. |
Accordingly, the brain network characteristics of DTI-SC were shown in Table 3. There were no significant differences for σ, El and Cp; the Lp of AD group was higher than those of MCI and NC groups, and the Eg of AD group was lower than those of other two groups. No significant differences were found for El between any two of the three groups. However, there were significant differences for Lp and Eg between MCI and AD groups, Cp and σ between MCI and NC groups, and Lp and Eg between MCI and AD groups.
Network characteristics | NC | MCI | AD | p value | ||
NC:MCI | MCI:AD | NC:AD | ||||
Lp | 0.270 ± 0.060 | 0.275 ± 0.062 | 0.331 ± 0.066 | 0.862 | 0.003* | 0.000* |
Cp | 0.020 ± 0.900 | 0.024 ± 0.009 | 0.020 ± 0.006 | 0.042* | 0.126 | 0.660 |
Eg | 3.895 ± 0.900 | 3.818 ± 0.809 | 3.152 ± 0.660 | 0.811 | 0.003* | 0.001* |
El | 5.718 ± 1.324 | 5.661 ± 1.175 | 5.055 ± 1.025 | 0.992 | 0.054 | 0.051 |
σ | 3.471 ± 0.388 | 3.709 ± 0.454 | 3.632 ± 0.628 | 0.020* | 0.722 | 0.187 |
*Note: *p < 0.05. |
It was demonstrated that the rsfMRI-FC was positively correlated with DTI-SC and there were significant differences for FC-SC coupling across the three groups and the coupling strength in AD group was greater than those of NC and MCI. With the coupling analysis, the coupling strengths (mean ± variance) were obtained with the NC of 0.272 ± 0.062, MCI of 0.297 ± 0.050 and AD of 0.328 ± 0.075, respectively. There were significant differences among the three groups for FC-SC coupling at the p-value of 0.003.
The correlations between FC-SC coupling and MMSE, CDR-SB, FAQ and MoCA scores were shown in Figure 2. The coupling of rsfMRI-FC/DTI-SC was negatively correlated with MMSE score, positively correlated with CDR-SB and FAQ scores, and no correlation with MoCA score, respectively.
In this study, the network coupling of FC-SC based on graph theory was evaluated via the three aspects of network characteristics, coupling analysis and correlations with the neuropsychological scores. The results showed that the coupling strength varied across three groups and this network coupling was proved to be correlated with neuropsychological scores in different trends.
According to the graph theory of regular and random networks, any small-world networks could facilitate the efficiency of information transmission and processing at lower processing cost and capacity consumption [35]. The inconsistency change of Cp among groups may be related to the differences in sample size, network node and threshold selection. During the AD progression, increased Lp and decreased Eg suggested that the information transmission rate and integration capacity were obviously reduced, and this would in turn lead to the observed impairments in cognitive and executive functions [36]. Indeed, the structural core of the brain played a great role in integrating information across functionally segregated brain regions [37,38]. The changes of the damaged FC and SC networks provided not only the critical imaging markers but also the potential clues to understand the underlying mechanism of cognitive deficits of AD [39].
For the rsfMRI-FC, the NC had larger σ and smaller Lp, while the AD had larger Lp. It was consistent with the previous neuroscientific findings [19]. In addition, the efficiency of the rsfMRI-FC decreased along with the smaller Eg in AD. It was indicated that the topology of damaged brain FC would certainly lead to the decrease of global network processing efficiency in AD [10]. Similarly for the DTI-SC network, there were no significant difference for the σ among the three groups. It was hinted that the human brain might maintain the optimal network topology to achieve the most efficient information transmission for the most efficient information transmission during progressive neurodegeneration. While the increased Lp and decreased Eg reflected the impairment of global connectivity of DTI-SC in AD [36].
With the coupling analysis, there were significant correlations for rsfMRI-FC/DTI-SC. The correlations between FC-SC coupling and neuropsychological scores (MMSE, CDR-SB, FAQ and MoCA) provided further support for the assumption that these changes in network coupling contributed to the cognitive decline in AD. It was reported that the coupling strength of rsfMRI-FC/DTI-SC was increased with the known greater severity of cognitive deficits in AD [40,41]. It might be explained by the reasons that the loss of plasticity within pathways connecting different brain regions (nodes) for constrained brain network dynamics [42]. The integrity of SC might reflect the ability of the cerebral cortex to maintain functional diversity and neural activity interactions [43]. Therefore, the increased coupling indicated that AD would produce more direct functional interactions related to anatomical connections, which implied that brain function became more rigid, less dynamic and adaptable. Moreover, it reduced the restructuring capacity of functional network and mitigated the effects of brain regional degeneration [44,45].
This study had several limitations. First, the gender effect of participants was not considered due to the limited sample size. Second, DTI-SC was certainly underestimated for the missed information of crossover fibers during DFT. More accurate SC would be achieved by the betterment of fiber tracking algorithms on higher resolution data sets. Finally, multiple modalities of brain networks should be involved for convincing results, such as the metabolism connectivity of positron emission tomography (PET) and FC of electroencephalography. It would be of further interest to investigate disease-related changes in these dynamic properties and their relationship to cognitive decline on more larger data samples.
It was concluded that there existed FC/SC coupling and varied network characteristics for rsfMRI and DTI, and this would provide the clues to understand the underlying mechanisms of cognitive deficits of AD.
This study was funded by the grants of the National Key Research and Development Program of China (2020YFC2008700) and the National Natural Science Foundation of China (Nos 61971275, 81830052, and 82072228).
The authors declare no conflict of interest.
[1] |
N. Hao, Z. Wang, P. Liu, R. Becker, S. Yang, K. Yang, et al., Acoustofluidic multimodal diagnostic system for Alzheimer's disease, Biosens. Bioelectron., 196 (2022), 113730. https://doi.org/10.1016/j.bios.2021.113730 doi: 10.1016/j.bios.2021.113730
![]() |
[2] |
B. T. Hyman, C. H. Phelps, T. G. Beach, E. H. Bigio, N. J. Cairns, M. C. Carrillo, et al., National institute on aging-Alzheimer's association guidelines for the neuropathologic assessment of Alzheimer's disease, Alzheimer's Dementia, 8 (2012), 1-13. https://doi.org/10.1016/j.jalz.2011.10.007 doi: 10.1016/j.jalz.2011.10.007
![]() |
[3] |
V. L. Villemagne, S. Burnham, P. Bourgeat, B. Brown, K. A. Ellis, O. Salvado, et al., Amyloid β deposition, neurodegeneration, and cognitive decline in sporadic Alzheimer's disease: a prospective cohort study, Lancet Neurol., 12 (2013), 357-367. https://doi.org/10.1016/s1474- 4422(13)70044-9 doi: 10.1016/s1474-4422(13)70044-9
![]() |
[4] |
O. Sporns, G. Tononi, R. Kötter, The human connectome: A structural description of the human brain, PLoS Comput. Biol., 1 (2005), e42. https://doi.org/10.1371/journal.pcbi.0010042 doi: 10.1371/journal.pcbi.0010042
![]() |
[5] |
B. B. Biswal, M. Mennes, X. N. Zuo, S. Gohel, C. Kelly, S. M. Smith, et al., Toward discovery science of human brain function, PNAS, 107 (2010), 4734-4739. https://doi.org/10.1073/pnas.0911855107 doi: 10.1073/pnas.0911855107
![]() |
[6] |
F. Agosta, S. Galantucci, M. Filippi, Advanced magnetic resonance imaging of neurodegenerative diseases, Neurol. Sci., 38 (2017), 41-51. https://doi.org/10.1007/s10072-016-2764-x doi: 10.1007/s10072-016-2764-x
![]() |
[7] |
M. W. Cho, M. Y. Choi, Brain networks: Graph theoretical analysis and development models, Int. J. Imaging Syst. Technol., 20 (2010), 108-116. https://doi.org/https://doi.org/10.1002/ima.20229 doi: 10.1002/ima.20229
![]() |
[8] |
J. delEtoile, H. Adeli, Graph ttheory and brain connectivity in Alzheimer's disease, Neuroscientist, 23 (2017), 616-626. https://doi.org/10.1177/1073858417702621 doi: 10.1177/1073858417702621
![]() |
[9] |
A. M. Tuladhar, I. W. M. Van Uden, L. C. A. Rutten-Jacobs, A. Lawrence, H. Van Der Holst, A. Van Norden, et al., Structural network efficiency predicts conversion to dementia, Neurology, 86 (2016), 1112-1119. https://doi.org/10.1212/wnl.0000000000002502 doi: 10.1212/wnl.0000000000002502
![]() |
[10] |
F. U. Fischer, D. Wolf, A. Scheurich, A. Fellgiebel, Altered whole-brain white matter networks in preclinical Alzheimer's disease, NeuroImage: Clin., 8 (2015), 660-666. https://doi.org/10.1016/j.nicl.2015.06.007 doi: 10.1016/j.nicl.2015.06.007
![]() |
[11] |
A. Wada, O. Abe, Graph theoretic analysis of structural connectivity of Alzheimer's disease by using diffusion MR imaging, Med. Imaging Technol., 34 (2016), 18-21. https://doi.org/10.11409/mit.34.18 doi: 10.11409/mit.34.18
![]() |
[12] |
C. Yang, S. Zhong, X. Zhou, L. Wei, L. Wang, S. Nie, The abnormality of topological asymmetry between hemispheric brain white matter networks in Alzheimer's disease and mild cognitive impairment, Front. Aging Neurosci., 9 (2017), 261. https://doi.org/10.3389/fnagi.2017.00261 doi: 10.3389/fnagi.2017.00261
![]() |
[13] |
L. Zajac, B. B. Koo, C. Bauer, R. Killiany, Seed location impacts whole-brain structural network comparisons between healthy elderly and individuals with Alzheimer's disease, Brain Sci., 7 (2017), 37. https://doi.org/10.3390/brainsci7040037 doi: 10.3390/brainsci7040037
![]() |
[14] |
E. S. Lee, K. Yoo, Y. B. Lee, J. Chung, J. E. Lim, B. Yoon, et al., Default mode network functional connectivity in early and late mild cognitive impairment: Results from the Alzheimer's disease neuroimaging initiative, Alzheimer Dis. Assoc. Disord., 30 (2016), 289-296. https://doi.org/10.1097/wad.0000000000000143 doi: 10.1097/wad.0000000000000143
![]() |
[15] |
L. Chuanming, Z. Jian, W. Jian, G. Li, L. Chuan, An fMRI stroop task study of prefrontal cortical function in normal aging, mild cognitive impairment, and Alzheimer's disease, Curr. Alzheimer Res., 6 (2009), 525-530. https://doi.org/10.2174/156720509790147142 doi: 10.2174/156720509790147142
![]() |
[16] | K. Çiftçi, Graph theoretical analysis of functional brain networks during Alzheimer's disease, in 2010 IEEE 18th Signal Processing and Communications Applications Conference, (2010), 925-928. https://doi.org/10.1109/SIU.2010.5651274. |
[17] |
K. Hahn, N. Myers, S. Prigarin, K. Rodenacker, A. Kurz, H. Förstl, et al., Selectively and progressively disrupted structural connectivity of functional brain networks in Alzheimer's disease-Revealed by a novel framework to analyze edge distributions of networks detecting disruptions with strong statistical evidence, NeuroImage, 81 (2013), 96-109. https://doi.org/10.1016/j.neuroimage.2013.05.011 doi: 10.1016/j.neuroimage.2013.05.011
![]() |
[18] |
R. Balachandar, J. P. John, J. Saini, K. J. Kumar, H. Joshi, S. Sadanand, et al., A study of structural and functional connectivity in early Alzheimer's disease using rest fMRI and diffusion tensor imaging, Int. J. Geriatr. Psychiatry, 30 (2015), 497-504. https://doi.org/10.1002/gps.4168 doi: 10.1002/gps.4168
![]() |
[19] |
Z. Liu, Y. Zhang, H. Yan, L. Bai, R. Dai, W. Wei, et al., Altered topological patterns of brain networks in mild cognitive impairment and Alzheimer's disease: A resting-state fMRI study, Psychiatry Res.: Neuroimaging, 202 (2012), 118-125. https://doi.org/10.1016/j.pscychresns.2012.03.002 doi: 10.1016/j.pscychresns.2012.03.002
![]() |
[20] |
K. Mevel, G. Chételat, F. Eustache, B. Desgranges, The default mode network in healthy aging and Alzheimer's disease, Int. J. Alzheimers Dis., 2011 (2011), 535816. https://doi.org/10.4061/2011/535816 doi: 10.4061/2011/535816
![]() |
[21] |
J. S. Lim, D. W. Kang, Stroke connectome and its implications for cognitive and behavioral sequela of stroke, J. Stroke, 17 (2015), 256-267. https://doi.org/10.5853/jos.2015.17.3.256 doi: 10.5853/jos.2015.17.3.256
![]() |
[22] |
S. Khalsa, S. D. Mayhew, M. Chechlacz, M. Bagary, A. P. Bagshaw, The structural and functional connectivity of the posterior cingulate cortex: Comparison between deterministic and probabilistic tractography for the investigation of structure-function relationships, NeuroImage, 102 (2014), 118-127. https://doi.org/10.1016/j.neuroimage.2013.12.022 doi: 10.1016/j.neuroimage.2013.12.022
![]() |
[23] |
Y. Sun, Q. Yin, R. Fang, X. Yan, Y. Wang, A. Bezerianos, et al., Disrupted functional brain connectivity and its association to structural connectivity in amnestic mild cognitive impairment and Alzheimer's disease, PloS one, 9 (2014), e96505. https://doi.org/10.1371/journal.pone.0096505 doi: 10.1371/journal.pone.0096505
![]() |
[24] |
Z. Dai, Q. Lin, T. Li, X. Wang, H. Yuan, X. Yu, et al., Disrupted structural and functional brain networks in Alzheimer's disease, Neurobiol. Aging, 75 (2019), 71-82. https://doi.org/10.1016/j.neurobiolaging.2018.11.005 doi: 10.1016/j.neurobiolaging.2018.11.005
![]() |
[25] |
J. J. Crofts, M. Forrester, R. D. O'Dea, Structure-function clustering in multiplex brain networks, Europhys. Lett., 116 (2016), 18003. https://doi.org/10.1209/0295-5075/116/18003 doi: 10.1209/0295-5075/116/18003
![]() |
[26] |
J. Zhao, X. Ding, Y. Du, X. Wang, G. Men, Functional connectivity between white matter and gray matter based on fMRI for Alzheimer's disease classification, Brain Behav., 9 (2019), https://doi.org/10.1002/brb3.1407 doi: 10.1002/brb3.1407
![]() |
[27] |
C. Yan, Y. Zang, DPARSF: a MATLAB toolbox for "pipeline" data analysis of resting-state fMRI, Front. Syst. Neurosci., 4 (2010), 13. https://doi.org/10.3389/fnsys.2010.00013 doi: 10.3389/fnsys.2010.00013
![]() |
[28] |
Z. Cui, S. Zhong, P. Xu, G. Gong, Y. He, PANDA: a pipeline toolbox for analyzing brain diffusion images, Front. Hum. Neurosci., 7 (2013), 42. https://doi.org/10.3389/fnhum.2013.00042 doi: 10.3389/fnhum.2013.00042
![]() |
[29] |
S. Mori, W. E. Kaufmann, C. Davatzikos, B. Stieltjes, L. Amodei, K. Fredericksen, et al., Imaging cortical association tracts in the human brain using diffusion-tensor-based axonal tracking, Magn. Reson. Med., 47 (2002), 215-223. https://doi.org/10.1002/mrm.10074 doi: 10.1002/mrm.10074
![]() |
[30] |
N. Tzourio-Mazoyer, B. Landeau, D. Papathanassiou, F. Crivello, O. Etard, N. Delcroix, et al., Automated anatomical labeling of activations in SPM using a macroscopic anatomical parcellation of the MNI MRI single-subject brain, NeuroImage., 15 (2002), 273-289. https://doi.org/https://doi.org/10.1006/nimg.2001.0978 doi: 10.1006/nimg.2001.0978
![]() |
[31] |
C. F. Bond, K. Richardson, Seeing the FisherZ-transformation, Psychometrika., 69 (2004), 291-303. https://doi.org/10.1007/bf02295945 doi: 10.1007/bf02295945
![]() |
[32] |
J. Wang, L. Wang, Y. Zang, H. Yang, H. Tang, Q. Gong, et al., Parcellation-dependent small-world brain functional networks: A resting-state fMRI study, Hum. Brain Mapp., 30 (2009), 1511-1523. https://doi.org/10.1002/hbm.20623 doi: 10.1002/hbm.20623
![]() |
[33] | P. Hinton, C. Brownlow, I. McMurray, B. Charlotte, SPSS Explained, 1st Edition, 2004. https://doi.org/10.4324/9780203642597 |
[34] |
J. Wang, X. Wang, M. Xia, X. Liao, A. Evans, Y. He, GRETNA: a graph theoretical network analysis toolbox for imaging connectomics, Front. Hum. Neurosci., 9 (2015), 386. https://doi.org/10.3389/fnhum.2015.00386 doi: 10.3389/fnhum.2015.00386
![]() |
[35] |
X. Liao, A. V. Vasilakos, Y. He, Small-world human brain networks: Perspectives and challenges, Neurosci. Biobehav. Rev., 77 (2017), 286-300. https://doi.org/10.1016/j.neubiorev.2017.03.018 doi: 10.1016/j.neubiorev.2017.03.018
![]() |
[36] |
L. J. Zhang, G. Zheng, L. Zhang, J. Zhong, Q. Li, T. Z. Zhao, et al., Disrupted small world networks in patients without overt hepatic encephalopathy: A resting state fMRI study, Eur. J. Radiol., 83 (2014), 1890-1899. https://doi.org/10.1016/j.ejrad.2014.06.019 doi: 10.1016/j.ejrad.2014.06.019
![]() |
[37] |
Y. Li, Y. Liu, J. Li, W. Qin, K. Li, C. Yu, et al., Brain anatomical network and intelligence, PLoS Comput. Biol., 5 (2009), e1000395. https://doi.org/10.1371/journal.pcbi.1000395 doi: 10.1371/journal.pcbi.1000395
![]() |
[38] |
P. Hagmann, L. Cammoun, X. Gigandet, R. Meuli, C. J. Honey, V. J. Wedeen, et al., Mapping the structural core of human cerebral cortex, PLoS Biol., 6 (2008), e159. https://doi.org/10.1371/journal.pbio.0060159 doi: 10.1371/journal.pbio.0060159
![]() |
[39] |
Z. Wang, Z. Dai, G. Gong, C. Zhou, Y. He, Understanding structural-functional relationships in the human brain: A large-scale network perspective, Neuroscientist, 21 (2014), 290-305. https://doi.org/10.1177/1073858414537560 doi: 10.1177/1073858414537560
![]() |
[40] |
J. Wang, R. Khosrowabadi, K. K. Ng, Z. Hong, J. S. X. Chong, Y. Wang, et al., Alterations in brain network topology and structural-functional connectome coupling relate to cognitive impairment, Front. Aging Neurosci., 10 (2018), 404. https://doi.org/10.3389/fnagi.2018.00404 doi: 10.3389/fnagi.2018.00404
![]() |
[41] |
Z. Dai, Y. He, Disrupted structural and functional brain connectomes in mild cognitive impairment and Alzheimer's disease, Neurosci. Bull., 30 (2014), 217-232. https://doi.org/10.1007/s12264-013-1421-0 doi: 10.1007/s12264-013-1421-0
![]() |
[42] |
S. Gardini, A. Venneri, F. Sambataro, F. Cuetos, F. Fasano, M. Marchi, et al., Increased functional connectivity in the default mode network in mild cognitive impairment: A maladaptive compensatory mechanism associated with poor semantic memory performance, J. Alzheimers Dis., 45 (2015), 457-470. https://doi.org/10.3233/JAD-142547 doi: 10.3233/JAD-142547
![]() |
[43] |
J. Zimmermann, P. Ritter, K. Shen, S. Rothmeier, M. Schirner, A. R. McIntosh, Structural architecture supports functional organization in the human aging brain at a regionwise and network level, Hum. Brain Mapp., 37 (2016), 2645-2661. https://doi.org/10.1002/hbm.23200 doi: 10.1002/hbm.23200
![]() |
[44] |
R. Cao, X. Wang, Y. Gao, T. Li, H. Zhang, W. Hussain, et al., Abnormal anatomical rich-club organization and structural-functional coupling in mild cognitive impairment and Alzheimer's disease, Front. Neurol., 11 (2020), 53. https://doi.org/10.3389/fneur.2020.00053 doi: 10.3389/fneur.2020.00053
![]() |
[45] |
M. P. Van Den Heuvel, O. Sporns, G. Collin, T. Scheewe, R. C. W. Mandl, W. Cahn, et al., Abnormal rich club organization and functional brain dynamics in schizophrenia, JAMA Psychiatry, 70 (2013), 783. https://doi.org/10.1001/jamapsychiatry.2013.1328 doi: 10.1001/jamapsychiatry.2013.1328
![]() |
1. | Xinyue Teng, Chaoyue Guo, Xiaoxia Lei, Fuyin Yang, Zenan Wu, Lingfang Yu, Juanjuan Ren, Chen Zhang, Comparison of brain network between schizophrenia and bipolar disorder: A multimodal MRI analysis of comparative studies, 2023, 327, 01650327, 197, 10.1016/j.jad.2023.01.116 | |
2. | Shuheng Zhang, Yan Zhang, Jie Pan, Xintian Lin, Xuemei Ding, Hongqin Yang, Ming Li, Hossein Asghari, Kebin Shi, Nuannuan Shi, 2023, Novelty detection technology for Alzheimer's disease based on autoencoder feature extraction and MCD classification, 9781510667938, 16, 10.1117/12.2687619 | |
3. | Xiaoli Yang, Yuxin Xia, Zhenwei Li, Lipei Liu, Zhipeng Fan, Jiayi Zhou, Classification of Alzheimer’s Disease Based on White Matter Connectivity Network, 2023, 13, 2076-3417, 12030, 10.3390/app132112030 | |
4. | Yan Zhang, Xin Liu, Zuping Zhang, 2024, DDN-Net: Deep Residual Shrinkage Denoising Networks with Channel-Wise Adaptively Soft Thresholds for Automated Major Depressive Disorder Identification, 979-8-3503-4485-1, 1626, 10.1109/ICASSP48485.2024.10447988 | |
5. | Daisuke Yoshimaru, Tomokazu Tsurugizawa, Junichi Hata, Kanako Muta, Takuto Marusaki, Naoya Hayashi, Shuhei Shibukawa, Kei Hagiya, Hideyuki Okano, Hirotaka James Okano, Similarity and characterization of structural and functional neural connections within species under isoflurane anesthesia in the common marmoset, 2024, 300, 10538119, 120854, 10.1016/j.neuroimage.2024.120854 | |
6. | Hossein Mohammadi, Armin Ariaei, Zahra Ghobadi, Enam Alhagh Charkhat Gorgich, Auob Rustamzadeh, Which neuroimaging and fluid biomarkers method is better in theranostic of Alzheimer’s disease? An umbrella review, 2024, 16, 26672421, 403, 10.1016/j.ibneur.2024.02.007 | |
7. | Yong-Wen Sun, Xin-Yue Lyu, Xiao-Yang Lei, Ming-Ming Huang, Zhen-Min Wang, Bo Gao, Association study of brain structure–function coupling and glymphatic system function in patients with mild cognitive impairment due to Alzheimer’s disease, 2024, 18, 1662-453X, 10.3389/fnins.2024.1417986 | |
8. | Xiaojie Yu, Jingyuan Liu, Yinping Lu, Shintaro Funahashi, Toshiya Murai, Jinglong Wu, Qi Li, Zhilin Zhang, Early diagnosis of Alzheimer’s disease using a group self-calibrated coordinate attention network based on multimodal MRI, 2024, 14, 2045-2322, 10.1038/s41598-024-74508-z | |
9. | Yinping Lu, Luyao Wang, Toshiya Murai, Jinglong Wu, Zhilin Zhang, Detection of structural-functional coupling abnormalities using multimodal brain networks in Alzheimer’s disease: A comparison of three computational models, 2025, 22131582, 103764, 10.1016/j.nicl.2025.103764 |
Variables | NC (n = 62) | MCI (n = 31) | AD (n = 19) | p value |
Age (year) | 71.75 ± 6.47 | 70.71 ± 7.77 | 74.8 ± 8.49 | 0.154 |
Gender (M/F) | 24/38 | 16/15 | 10/9 | 0.371 |
Education (year) | 16.77 ± 2.29 | 15.65 ± 2.81 | 15.21 ± 2.50 | 0.024 |
MMSE | 28.74 ± 1.23 | 28.04 ± 1.43 | 22.04 ± 2.46 | < 0.050 |
CDR-SB | 0.11 ± 0.23 | 1.29 ± 0.85 | 5.01 ± 1.58 | < 0.050 |
FAQ | 0.15 ± 0.35 | 4.13 ± 3.97 | 15.28 ± 7.04 | < 0.050 |
MoCA | 25.78 ± 2.61 | 22.82 ± 2.87 | 15.96 ± 4.45 | < 0.050 |
Abbreviations: MMSE: mini-mental state exam; CDR-SB: clinical dementia rating-sum of boxes; FAQ: functional activities questionnaire; MoCA: montreal cognitive assessment. |
Network characteristics | NC | MCI | AD | p value | ||
NC:MCI | MCI:AD | NC:AD | ||||
Lp | 0.761 ± 0.026 | 0.775 ± 0.030 | 0.791 ± 0.037 | 0.021* | 0.103 | 0.003* |
Cp | 0.198 ± 0.009 | 0.199 ± 0.009 | 0.201 ± 0.009 | 0.886 | 0.310 | 0.230 |
Eg | 0.180 ± 0.004 | 0.178 ± 0.004 | 0.178 ± 0.006 | 0.006* | 1.000 | 0.035* |
El | 0.256 ± 0.007 | 0.254 ± 0.007 | 0.256 ± 0.007 | 0.189 | 0.178 | 0.681 |
σ | 0.699 ± 0.085 | 0.648 ± 0.099 | 0.689 ± 0.101 | 0.012* | 0.173 | 0.664 |
*Note: *p < 0.05. |
Network characteristics | NC | MCI | AD | p value | ||
NC:MCI | MCI:AD | NC:AD | ||||
Lp | 0.270 ± 0.060 | 0.275 ± 0.062 | 0.331 ± 0.066 | 0.862 | 0.003* | 0.000* |
Cp | 0.020 ± 0.900 | 0.024 ± 0.009 | 0.020 ± 0.006 | 0.042* | 0.126 | 0.660 |
Eg | 3.895 ± 0.900 | 3.818 ± 0.809 | 3.152 ± 0.660 | 0.811 | 0.003* | 0.001* |
El | 5.718 ± 1.324 | 5.661 ± 1.175 | 5.055 ± 1.025 | 0.992 | 0.054 | 0.051 |
σ | 3.471 ± 0.388 | 3.709 ± 0.454 | 3.632 ± 0.628 | 0.020* | 0.722 | 0.187 |
*Note: *p < 0.05. |
Variables | NC (n = 62) | MCI (n = 31) | AD (n = 19) | p value |
Age (year) | 71.75 ± 6.47 | 70.71 ± 7.77 | 74.8 ± 8.49 | 0.154 |
Gender (M/F) | 24/38 | 16/15 | 10/9 | 0.371 |
Education (year) | 16.77 ± 2.29 | 15.65 ± 2.81 | 15.21 ± 2.50 | 0.024 |
MMSE | 28.74 ± 1.23 | 28.04 ± 1.43 | 22.04 ± 2.46 | < 0.050 |
CDR-SB | 0.11 ± 0.23 | 1.29 ± 0.85 | 5.01 ± 1.58 | < 0.050 |
FAQ | 0.15 ± 0.35 | 4.13 ± 3.97 | 15.28 ± 7.04 | < 0.050 |
MoCA | 25.78 ± 2.61 | 22.82 ± 2.87 | 15.96 ± 4.45 | < 0.050 |
Abbreviations: MMSE: mini-mental state exam; CDR-SB: clinical dementia rating-sum of boxes; FAQ: functional activities questionnaire; MoCA: montreal cognitive assessment. |
Network characteristics | NC | MCI | AD | p value | ||
NC:MCI | MCI:AD | NC:AD | ||||
Lp | 0.761 ± 0.026 | 0.775 ± 0.030 | 0.791 ± 0.037 | 0.021* | 0.103 | 0.003* |
Cp | 0.198 ± 0.009 | 0.199 ± 0.009 | 0.201 ± 0.009 | 0.886 | 0.310 | 0.230 |
Eg | 0.180 ± 0.004 | 0.178 ± 0.004 | 0.178 ± 0.006 | 0.006* | 1.000 | 0.035* |
El | 0.256 ± 0.007 | 0.254 ± 0.007 | 0.256 ± 0.007 | 0.189 | 0.178 | 0.681 |
σ | 0.699 ± 0.085 | 0.648 ± 0.099 | 0.689 ± 0.101 | 0.012* | 0.173 | 0.664 |
*Note: *p < 0.05. |
Network characteristics | NC | MCI | AD | p value | ||
NC:MCI | MCI:AD | NC:AD | ||||
Lp | 0.270 ± 0.060 | 0.275 ± 0.062 | 0.331 ± 0.066 | 0.862 | 0.003* | 0.000* |
Cp | 0.020 ± 0.900 | 0.024 ± 0.009 | 0.020 ± 0.006 | 0.042* | 0.126 | 0.660 |
Eg | 3.895 ± 0.900 | 3.818 ± 0.809 | 3.152 ± 0.660 | 0.811 | 0.003* | 0.001* |
El | 5.718 ± 1.324 | 5.661 ± 1.175 | 5.055 ± 1.025 | 0.992 | 0.054 | 0.051 |
σ | 3.471 ± 0.388 | 3.709 ± 0.454 | 3.632 ± 0.628 | 0.020* | 0.722 | 0.187 |
*Note: *p < 0.05. |