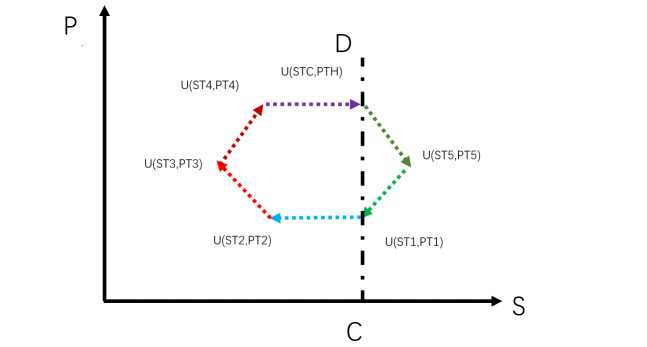
Pork makes up the highest proportion of household expenditure on meat in China and supply and demand have been basically stable in the past decade. However, the catastrophic outbreak of African swine fever (ASF) in August 2018 disrupted the balance and reduced the national herd by half within six months. The consequence was a gross lack of supply to the market and consumer demand was unable to be met. Accordingly, live pig prices rose sharply from 2019. In order to assess the influence of ASF on the price of the live pigs, we use a price function to characterize the relationship between price of the live pigs and the nation's pig stock, and then establish a time delay ASF epidemic dynamical model with the price function. By analyzing the dynamical behaviors of the model, we calculate the basic reproductive number, discuss the stability of equilibrium, and obtain the critical conditions for Hopf bifurcation. The model reasonableness is confirmed by carrying out data fitting and parameter estimation based on price data of the live pigs, the pig stock data and the outbreak data of ASF. By performing sensitivity analysis, we intuitively show the impact of ASF on the price of live pigs and the pig stocks, and assess the key factors affecting the outbreak of ASF. The conclusion is drawn that, with the control measures adopted by related government department in China, the basic reproductive number (R0=0.6005) means that the ASF epidemic has been controlled. Moreover, the price of the live pig increases linearly with R0, while the effect of the number of infected pigs on the subsequent price is non-linear related. Our findings suggest that society and the government should pay more attention to the prevention of animal disease epidemics.
Citation: Yihao Huang, Jing Li, Juan Zhang, Zhen Jin. Dynamical analysis of the spread of African swine fever with the live pig price in China[J]. Mathematical Biosciences and Engineering, 2021, 18(6): 8123-8148. doi: 10.3934/mbe.2021403
[1] | Haitao Song, Lirong Guo, Zhen Jin, Shengqiang Liu . Modelling and stability analysis of ASFV with swill and the virus in the environment. Mathematical Biosciences and Engineering, 2022, 19(12): 13028-13049. doi: 10.3934/mbe.2022608 |
[2] | Fangyuan Chen, Rong Yuan . Dynamic behavior of swine influenza transmission during the breed-slaughter process. Mathematical Biosciences and Engineering, 2020, 17(5): 5849-5863. doi: 10.3934/mbe.2020312 |
[3] | Vanessa Steindorf, Sergio Oliva, Jianhong Wu . Cross immunity protection and antibody-dependent enhancement in a distributed delay dynamic model. Mathematical Biosciences and Engineering, 2022, 19(3): 2950-2984. doi: 10.3934/mbe.2022136 |
[4] | Najat Ziyadi . A male-female mathematical model of human papillomavirus (HPV) in African American population. Mathematical Biosciences and Engineering, 2017, 14(1): 339-358. doi: 10.3934/mbe.2017022 |
[5] | Bevina D. Handari, Dipo Aldila, Bunga O. Dewi, Hanna Rosuliyana, Sarbaz H. A. Khosnaw . Analysis of yellow fever prevention strategy from the perspective of mathematical model and cost-effectiveness analysis. Mathematical Biosciences and Engineering, 2022, 19(2): 1786-1824. doi: 10.3934/mbe.2022084 |
[6] | Haitao Song, Dan Tian, Chunhua Shan . Modeling the effect of temperature on dengue virus transmission with periodic delay differential equations. Mathematical Biosciences and Engineering, 2020, 17(4): 4147-4164. doi: 10.3934/mbe.2020230 |
[7] | Zuohuan Zheng, Kaihua Wang, Daiyu Yang, Feifei Yin, Dingwei Sun, Weiyan Yu, Jialun Lin, Ying Liu, Changkuan Chen, Zehui Yang, Biao Wu . Factors affecting the transmission of dengue fever in Haikou city in 2019. Mathematical Biosciences and Engineering, 2023, 20(9): 16045-16059. doi: 10.3934/mbe.2023716 |
[8] | Zhenzhen Liao, Shujing Gao, Shuixian Yan, Genjiao Zhou . Transmission dynamics and optimal control of a Huanglongbing model with time delay. Mathematical Biosciences and Engineering, 2021, 18(4): 4162-4192. doi: 10.3934/mbe.2021209 |
[9] | Rajanish Kumar Rai, Arvind Kumar Misra, Yasuhiro Takeuchi . Modeling the impact of sanitation and awareness on the spread of infectious diseases. Mathematical Biosciences and Engineering, 2019, 16(2): 667-700. doi: 10.3934/mbe.2019032 |
[10] | Toshikazu Kuniya, Hisashi Inaba . Hopf bifurcation in a chronological age-structured SIR epidemic model with age-dependent infectivity. Mathematical Biosciences and Engineering, 2023, 20(7): 13036-13060. doi: 10.3934/mbe.2023581 |
Pork makes up the highest proportion of household expenditure on meat in China and supply and demand have been basically stable in the past decade. However, the catastrophic outbreak of African swine fever (ASF) in August 2018 disrupted the balance and reduced the national herd by half within six months. The consequence was a gross lack of supply to the market and consumer demand was unable to be met. Accordingly, live pig prices rose sharply from 2019. In order to assess the influence of ASF on the price of the live pigs, we use a price function to characterize the relationship between price of the live pigs and the nation's pig stock, and then establish a time delay ASF epidemic dynamical model with the price function. By analyzing the dynamical behaviors of the model, we calculate the basic reproductive number, discuss the stability of equilibrium, and obtain the critical conditions for Hopf bifurcation. The model reasonableness is confirmed by carrying out data fitting and parameter estimation based on price data of the live pigs, the pig stock data and the outbreak data of ASF. By performing sensitivity analysis, we intuitively show the impact of ASF on the price of live pigs and the pig stocks, and assess the key factors affecting the outbreak of ASF. The conclusion is drawn that, with the control measures adopted by related government department in China, the basic reproductive number (R0=0.6005) means that the ASF epidemic has been controlled. Moreover, the price of the live pig increases linearly with R0, while the effect of the number of infected pigs on the subsequent price is non-linear related. Our findings suggest that society and the government should pay more attention to the prevention of animal disease epidemics.
In the food culture of the Chinese, pork is the favorite meat food, and the amount of pork on the dining table in the reception of guests determines the level of the guest's status and also reflects the economic strength of the host's family. Therefore, the amount of pig breeding, pork production, pork prices are directly related to people's livelihood and social stability. From a demand perspective, the number of pigs that the Chinese consumed every year had increased from 400 million in 1995 to 700 million in 2014, and then was stable at more than 600 million. All in all, from 2014 to 2018, China's annual pork consumption has been basically stable and it can be assumed that the demand for pork has not changed from an economic point of view. In 2018, the world produced a total of 113 million tons of pork, of which 55.95 million tons were consumed by the Chinese. In other words, China consumes nearly half of the world's pork despite having less than one-fifth of the population. In terms of pork supply, the farms' ability to supply pork in China is second to none. Of the 113 million tons of pork in the world in 2018, the Chinese produced 54.04 million tons, twice that of the entire European Union and four times that of the United States. In the past few decades, three major pig-raising provinces in the country (Sichuan, Henan and Guangdong) have developed large-scale pig breeding companies such as New Hope, Muyuan, and Wen's[1,2]. In general, from 2014 to 2018, China's pork supply and demand regionally balanced. Along with the relationship between supply and demand, the price of live pigs and pork from 2014 to 2018 remained stable, but it was broke by African swine fever (ASF) in August 2018.
African swine fever virus (ASFV) was first discovered in Kenya, Africa in 1921 and has a history of about 100 years. In August 2018, the African swine fever epidemic began to outbreak in China. The virulent strain of African swine fever virus can cause high fever, loss of appetite, bleeding of internal organs and skin, and death within 2–10 days, with a mortality rate as high as 100%[4,5,6]. Due to the complication of the disease on the animal, all infected pigs should be required to culled. Under normal circumstances, if the biological control associated with African swine fever is not carried out in time, African swine fever continues to prevail and can cause a long-term impact on the development of China's breading industry. In addition, the way of transmission of African swine fever virus is mainly through contact with the infected pig, or ingestion of items contaminated with African swine fever virus which is another reason for harmless disposal of the infected pig[7,8,9].
According to the epidemic situation and corresponding control measures in China, with the spread of the African swine fever epidemic, a large number of the infected live pigs and healthy pigs in the same group were culled, and small farms that did not meet the government standard were forced to close down, which caused a large reduction in pig stock. Since November 2018, the domestic pork stock reduced by almost half within six months, and the pork stock that can be supplied to the market is far from being insufficient. Thus, all these lead to the decimation of the pork market commodity supply chain. After March 2019, pork price and price of the live pig began to rise, almost rose every day, and price of piglet soared rises from about 13 yuan/kg to 38 yuan/kg. Although there has occasionally appeared a downward trend since then, it hovered at 35 yuan/kg. As of January 2021, price of the live pig still maintained at 34.8 yuan/kg[3]. It is obvious that African swine fever plays an important role in price rise of the live pig and the pork, which is directly related to safe and stable operation of the national economy. However, the internal interaction mechanism between African swine fever and price needs to be further studied quantitatively by applying mathematical methods.
Mathematical methods to investigate the spread of the epidemic can be divided into dynamics and statistics. The dynamical method is based on inner transmission mechanism of the epidemic to establish equations to understand its laws and characteristics. Among them, Nielsen et al. proposed a SEIR model to study the prevalence of African swine fever in farms[10]. Barongo et al. established a differential equation model to predict and evaluate the effect of bio-security measures and vaccination intervention[11,12]. Lee et al. established a stochastic network model with various farms in Vietnam and drew a conclusion that besides improving bio-security, culling pigs in infected farms as early as possible is critical to minimize the spread of ASF epidemic[13]. These works investigated the effectiveness of various prevention and control measures, but did not consider the price factor. Zhan et al. applied PVAR model and obtained that African swine fever had most important impact on pork prices, such influence relationship was lagging[14]. Statistics are based on data to establish models without considering mechanism. In the study of price of the live pig, Tan et al. used existing live pig price data to establish statistical models and found that the price and demand of the live pig were directly affected by African swine fever, while the supply of pigs was indirectly affected from related data[15,16]. So, in this paper, based on the data about the pig stock number, the live pig price and ASF pig cases, we combine the transmission dynamics of ASF and the price factor to establish a new dynamical model.
Pork commodity is a product with a long production cycle. The farm will determine the output of the next period according to the current price, and the current pig breeding quantity determines the next period of pork price conversely. Before establishing dynamical model, we should understand relationship of pork price and breeding quantity of pigs. Based on the hexagonal spider web model and combined with the actual situation, we interpreter the relationship as follows (see Figure 1).
(1) Before the outbreak of African swine fever, the total number of live pigs in the country and its market demand were in a state of balance. The status of the stock of live pigs and prices of pig is at point U(ST1, PT1).
(2) With spread of the epidemic, a large number of live pigs were killed, and many small and medium-sized private pig farms were closed, so the stock of live pigs began to decrease. However, due to the time period that live pigs are transformed to pork products that is delivered to the market for sale, the price change lags behind the change in the stock volume. In this way, the status of the stock of live pigs and the price of live pigs moves from point U(ST1, PT1) to point U(ST2, PT2).
(3) Since the reduced amount of pig herds led to the decline of the volume of pork market supply in the next period, the market demand for pork products cannot be met and the price of pigs will increase. At this time, the status of pig stock and pig price moves from point U(ST2, PT2) to point U(ST3, PT3).
(4) The increase of the pork price will drive farms to increase breeding quantity of pigs. However, most of them are piglets, which need go through the process of growth and cannot be put on the market directly, so the price of pigs is still rising. So, point U(ST3, PT3) moves to point U(ST4, PT4).
(5) When it reaches point U(ST4, PT4), the piglets from the previous period have grown into fattening pigs and can be sold for processing. At this time, the supply of live pigs can meet the market demand, so the price of pigs will not change. So, the status of the stock of live pigs and the price of live pigs moves from point U(ST4, PT4) to U(STC, PTH).
(6) When the supply of live pigs reaches market demand, because the price is still at a relatively high position, farms still increase sows farrowing for profit. However, superabundant stocks will cause oversupply. In order to sell pork products faster, the price will start to fall. At this time, the status of the stock of pigs and the price of pig will move from point U(STC, PTH) to point U(ST5, PT5).
(7) When the price of live pigs begins to fall, the farm will correspondingly reduce the stock of live pigs to reduce the cost of breeding. At this time, the status of the stock of live pigs and the price of live pigs moves from point U(ST5, PT5) to point U(ST1, PT1), and supply and demand of pigs finally reach the balance to make the price be stable.
From Figure 1, it can be seen that the reason to cause this cycle are the lag from sows' pregnancy to piglets and lag from piglets to pork products for sale[17,18,19,20,21]. So, in this paper, considering the two time delays, we introduce a logistic growth function and a price function to establish a novel time delay dynamical model, and investigate the influence of ASF on the pork price. First, we analyse the dynamical behavior of the model without delay and with delay. Secondly, the actual data of pigs stock, pigs price and reported cases during the onset period of ASF are used to verify the rationality of model and estimate parameter values. Finally, we carry out the sensitivity analysis of the parameters to assess impact of ASF on the stock and the price of live pigs.
In this paper we take all the live pigs in China as research objects. The stock quantity of live pigs, pig price and the cumulative number of reported infected pigs with ASF are adopted (see Table 1). The data in Table 1 are interpreted as follows.
(1) The stock of live pigs is the existing number of all pigs in the farm, including sows, piglets, and pigs that can be put on the market, which can be obtained from the web of the Iimedia[22] (data.iimedia.cn) and the Ministry of Rural Agriculture of China (www.moa.gov.cn). The data in the Iimedia is on the monthly bases, but the data in the Ministry of Rural Agriculture of China is on a quarterly basis, so we adopt the former to fit model.
Time | The stock of live pigs (Unit: million) | The price of live pigs (Unit: China Yuan/kg) | Cumulative number of reported infected pigs by ASF (Unit: Individual) |
2017.03 | 41305.41 | 16.71 | |
2017.04 | 41466.79 | 16 | |
2017.05 | 41010.73 | 14.63 | |
2017.06 | 40845.35 | 13.78 | |
2017.07 | 40596.74 | 13.96 | |
2017.08 | 40407.91 | 14.42 | |
2017.09 | 40357.91 | 14.75 | |
2017.10 | 40322.69 | 14.52 | |
2017.11 | 40356.89 | 14.47 | |
2017.12 | 39900.84 | 15.07 | |
2018.01 | 41529.58 | 15.25 | |
2018.02 | 40668.07 | 14.06 | |
2018.03 | 41334.64 | 11.91 | |
2018.04 | 41196.72 | 10.93 | |
2018.05 | 40452.93 | 10.57 | |
2018.06 | 39811.29 | 11.32 | |
2018.07 | 39464.27 | 12.02 | |
2018.08 | 39340.35 | 13.6 | 1277 |
2018.09 | 39558.9 | 13.95 | 2246 |
2018.10 | 39581.39 | 14.03 | 5991 |
2018.11 | 39353.89 | 13.99 | 7143 |
2018.12 | 38299.13 | 13.98 | 8097 |
2019.01 | 36459.77 | 13.87 | 15448 |
2019.02 | 33493.43 | 12.68 | 17849 |
2019.03 | 33638.04 | 14.35 | 17982 |
2019.04 | 32712.57 | 15 | 18878 |
2019.05 | 31493.42 | 15 | 19087 |
2019.06 | 29830.47 | 16 | 19292 |
2019.07 | 27176.34 | 17.6 | 19391 |
2019.08 | 24431.67 | 21.85 | 19506 |
2019.09 | 23517.14 | 26.8 | 19521 |
2019.10 | 23227.07 | 33.38 | 19546 |
2019.11 | 23743.76 | 34.89 | 19704 |
2019.12 | 24095.79 | 33.3 | 19793 |
2020.01 | 19580.44 | 34.2 | |
2020.02 | 20128.69 | 36.6 | |
2020.03 | 20873.45 | 35.4 | |
2020.04 | 21812.76 | 33.6 | |
2020.05 | 22663.46 | 30.2 | |
2020.06 | 23660.65 | 32.3 | |
2020.07 | 24796.36 | 35.8 | |
2020.08 | 25961.79 | 37.1 | |
2020.09 | 27156.03 | 36.5 | |
2020.10 | 28296.59 | 32.7 | |
2020.11 | 29513.34 | 29.7 | |
2020.12 | 30280.69 | 33.1 | |
2021.01 | 29188.69 | 35.5 | |
2021.02 | 29101.13 | 31.8 | |
2021.03 | 29974.16 | 28.2 | |
2021.04 | 23.8 | ||
2021.05 | 19.7 | ||
2021.06 | 15.6 | ||
2021.07 | 15.9 |
(2) The price of live pigs is the price at which the farm sells live pigs to the slaughterhouse. The data on the price of live pigs in the Iimedia is consistent with monthly data in the Ministry of Agriculture of China.
(3) Cumulative number of reported infected pigs by ASF is the cumulative number of African swine fever cases that comes from the Official Veterinary Bulletin (www.moa.gov.cn/gk/sygb/), which is the official website that belongs to China's Ministry of Rural Agriculture.
Denote N(t) to be the total number of live pigs at time t. With the short disease duration of African swine fever virus, once pigs show related clinical symptoms, they will be culled immediately, which can be described by classical SI epidemic model. According to the infection status, all pigs at time t are divided into two categories: S(t) that represents the number of susceptible pigs at time t that are healthy and may be infected in future, and I(t) that represents the number of infected pigs by ASF at time t, but have not been found and confirmed. Since the amount of pig breeding is limited by resources such as China's grain, breeding area and breeding level, the import term in SI model should adopt a more reasonable logistic rate, which is given in the model (3.1).
{dSdt=rS(1−SK)−βSI−θS,dIdt=βSI−αI, | (3.1) |
where, K is the maximum breeding amount of pigs that is affordable in China and it is positive, r is the intrinsic growth rate, β is the infection rate coefficient of the epidemic, α is the clinical outbreak rate or the confirmed rate of infected pigs, and θ is the conversion rate of live pigs in the breeding farm into pork products in market and it is also called as sale rate or marketing rate, then θS is the amount of live pigs that are transformed to pork product and put into the market at time t. All parameters except K are non-negative constants.
Assume τ1 is the pregnant time of sow from insemination to birthing. The price of pig at time t−τ1, denoted by P(t−τ1), will affect the growth rate at time t, r(t), whose relationship can be described as follows.
r(t)=bP(t−τ1)−a0, | (3.2) |
where, b is the scaling factor and a0/b is the price floor. Only the pig price is above a certain price, farms can continue to breed pigs and r(t) is greater than 0.
Since there is a production process of pigs from breeding to fattening and to final slaughter, and it leads to the lagging in price changes, that is, P(t+τ2) the price of pigs at time t+τ2, will be determined by S(t) breeding stock at time t, whose relationship is established as follows.
P(t+τ2)=eCS(t)−a1. | (3.3) |
Substituting Eq (3.3) into the model (3.2), we have
r(t)=b[eCS(t−τ1−τ2)−a1]−a0. |
Among them, e is the conversion coefficient of the price. C is the average monthly demand amount in the pig market. a1 is a adjustment parameter. Substituting Eqs (3.2) and (3.3) into the model (3.1), we obtain the following model.
{dSdt=b[hS(t−τ)−a]S(t)(1−S(t)K)−βS(t)I(t)−θS(t),dIdt=βS(t)I(t)−αI(t), | (3.4) |
where, a=a0/b+a1, h=eC and τ=τ1+τ2.
In this section, dynamical behaviors of system (3.4) with delay and without delay are analyzed. System (3.4) without delay corresponds to the following ordinary differential equations.
{dSdt=b(hS(t)−a)S(t)(1−S(t)K)−βS(t)I(t)−θS(t)≜f1(S,I),dIdt=βS(t)I(t)−αI(t)≜f2(S,I). | (4.1) |
By solving the equilibria of system (4.1), one can obtain that system (4.1) has two disease-free equilibria E01=(S01,0) and E02=(S02,0) (see Figure 2(a)), where
S01=12ab[(bh+abK+Kθ)−√(bh+abK+Kθ)2−4ab2hK] |
and
S02=12ab[(bh+abK+Kθ)+√(bh+abK+Kθ)2−4ab2hK]. |
Obviously, S02>S01>0. According to the equation dIdt=βS(t)I(t)−αI(t) of system (3.4), let dIdt|S=S01=0 and eliminate I, one can also obtain the expression of the basic reproductive number
R0=βS01α. |
R0>1 shows that the number of I(t) will increases with time at the initial time, which implies the infectious disease will outbreak, while R0<1 indicates the opposite case[23,24].
Besides, if the condition b(hKβ2+aα2)−αβ(abK+Kθ+bh)>0, that is ˆR0=b(hKβ2+aα2)αβ(abK+Kθ+bh)>1 holds, there exists an endemic equilibrium E∗=(S∗,I∗) (see Figure 2(a)), where
S∗=αβ, I∗=1Kαβ2(b(hKβ2+aα2)−αβ(abK+Kθ+bh))=(abK+Kθ+bh)Kβ(ˆR0−1). |
The following Lemma will give the relationship between R0 and ˆR0.
Lemma 4.1. R0>1⇒ˆR0>1.
Remark 4.1. When K is sufficiently large in model (4.1), namely (1−S(t)K)→1, then the original two disease-free equilibria became one equilibrium E0=(S0,0), where S0=bhab+θ, and endemic equilibrium is transformed as E∗=(S∗,I∗) (see Figure 2(b)), where
S∗=αβ, I∗=bhβ−α(ab+θ)αβ=ab+θβ(ˆR0−1). |
Concurrently, the corresponding the basic reproductive number becomes
ˆR0=bhβα(ab+θ), for sufficiently large K. |
Theorem 4.1. When R0<1, the disease-free equilibrium E01 is locally asymptotically stable. When R0>1, E01 is unstable. In addition, E02 is always unstable.
Proof: The Jacobian matrix of system (4.1) at E01 is
J|E01=(−bKS01(hK−aS201)−βS010β(S01−αβ)), | (4.2) |
and it has two eigenvalues:
λ1=−bKS01(hK−aS201), λ2=β(S01−αβ). |
We can obtain hK−aS201>0, then λ1<0 holds. When R0<1, λ2<0, which indicates that the disease-free equilibrium E01 is locally asymptotically stable. Otherwise, E01 becomes unstable.
Similarly, the Jacobian matrix of system (4.1) at E02 is
J|E02=(−bKS02(hK−aS202)−βS020β(S02−αβ)), | (4.3) |
and it has two eigenvalues:
λ1=−bKS02(hK−aS202), λ2=β(S02−αβ). |
Since hK−aS202<0, it is obvious that λ1<0. Thus E02 is always unstable.
Theorem 4.2. When R0>1, the endemic equilibrium E∗ is locally asymptotically stable.
Proof: The Jacobian matrix of system (4.1) at the endemic equilibrium E∗ is
J|E∗=(b11b12b21b22), | (4.4) |
where
b11=−b(hKβ2−aα2)αβK, b12=−α<0, |
b21=(abK+Kθ+bh)K(ˆR0−1)>0, b22=0. |
Then the characteristic equation is given by
λ2+b1λ+b0=0, | (4.5) |
here, b1=b(hKβ2−aα2)αβK=bβ(hK−a(S∗)2)αK>b(hK−aS201)αK>0, b0=α(abK+Kθ+bh)K(ˆR0−1). When R0>1, ˆR0>1 holds, then one can obtain that b0>0. Based on Routh-Hurwitz criterion, it is obtained that the endemic equilibrium E∗ is always locally asymptotically stable.
Through the similar theoretical analysis process, one can give dynamical behavior for sufficiently large K.
Remark 4.2. For sufficiently large K, the disease-free equilibrium E0 is locally asymptotically stable when ˆR0<1, but becomes unstable when ˆR0>1. The endemic equilibrium E∗ is locally asymptotically stable when ˆR0>1.
For the convenience of the subsequent theoretical analysis, the disease-free equilibrium E01 and endemic equilibrium E∗ are unified to mark as E1=(S1,I1). By inserting ˉS=S−S1 and ˉI=I−I1 into system (3.4) and linearizing this system, then one can give
{dˉSdt=c11ˉS(t)+c12ˉS(t−2τ)+c13ˉI(t),dˉIdt=c21ˉS(t)+c22ˉI(t), | (4.6) |
where c11=b(hS1−a)(1−2S1K)−βI1−θ, c12=−bh(K−S1)KS1, c13=−βS1, c21=βI1, c22=β(S1−αβ).
Let ˉS=S1eλt,ˉI=I1eλt, and insert ˉS and ˉI into system (4.4). One can further derive the following characteristic equation:
λ2−(c11+c22)λ−c12λe−2λτ+c11c22−c13c21+c12c22e−2λτ=0. | (4.7) |
Assume that λ=iω(ω>0) is a root of characteristic equation (4.7), one can get
−ω2−(c11+c22)iω−c12iωe−iωτ+c11c22−c13c21+c12c22e−iωτ=0. | (4.8) |
The real part and the imaginary part of Eq (4.8) are separated as
{−(c11+c22)ω=c12ωcosωτ+c12c22sinωτ,−ω2+c11c22−c13c21=c12ωsinωτ−c12c22cosωτ, | (4.9) |
from Eq (4.9), we can compute
cos(ωτ)=−(c11c222+c11ω2−c13c21c22)c12(c222+ω2),sin(ωτ)=−ω(c13c21+c222+ω2)c12(c222+ω2). |
By adding the squares of both sides of the above two Eq (4.9), we can get
ω4+C1ω2+C0=0, | (4.10) |
where C1=c211−c212+c222+2c13c21, C01=(c11c22−c12c22−c13c21), C02=(c11c22+c12c22−c13c21), C0=C01C02.
Set Z=ω2, Eq (4.10) can transformed as
Z2+C1Z+C0=0. | (4.11) |
Note that E02 is always unstable, then we discuss the local stabilities of E01 and E∗ in the following part.
Case I: When we focus on E01, it is easy to obtain
c11=−b(h−aS01)K, c12=−bh(k−S01)KS01, c13=−βS01, c21=0, c22=β(S01−αβ), |
and c22<0 because of R0<1. Then the coefficients of Eq (4.11) are C1=c211−c212+c222, C01=(c11−c12)c22 and C02=(c11+c12)c22. Obviously, C02=(c11+c12)c22>0 because of the stability of E01 in system (4.1). The following conclusion is given:
Lemma 4.2. If condition (H2) K(ab+θ)bh>1 holds, then characteristic equation (4.7) at E01 has a pair of pure imaginary roots ±iω0, where ω0=√−C1+√C21−4C02.
Proof: By inserting S01 into the expression c11−c12, one can get c11−c12=(ab+θ−bhK). Since condition (H2) holds, one can obtain c11−c12>0, then one can further derive C01<0. Thus the expression C0<0 is given. According to Weida's theorem, a quadratic equation (4.11) with one variable has two real roots:
Z01=−C1+√C21−4C02, Z02=−C1−√C21−4C02, |
and Z01>0>Z02. We thus obtain that characteristic equation (4.7) has a pair imaginary roots ±iω0, where ω0=√Z01, namely, ω0=√−C1+√C21−4C02.
On the basis of Lemma 4.2, the values of cos and sin functions at ω0 are
cos(ω0τ)=−c11c12, sin(ω0τ)=−ω0c12. |
Then we can derive
τj0={1ω0(arccos(−c11c12)+2jπ), sin(ω0)≥0,1ω0(2π−arccos(−c11c12)+2jπ), sin(ω0)<0, j=0,1,2,⋯. | (4.12) |
Lemma 4.3. Define λ(τ)=α(τ)+iω(τ) as a root of characteristic equation (4.7) at τj0, and α(τj0)=0, ω(τj0)=ω0 for j=0,1,2,⋯. Assume that condition (H2) K(ab+θ)bh>1 holds, then the transversality condition
Re(dλ(τ)dτ)|τ=τj0>0. |
is obtained.
Proof: By deriving the two sides of Eq (4.7) with respect to τ, we can get
Re(dλdτ|τ=τj0)−1=Re{2λ−(c11+c22)−c12e−λτ+c12τ(λ−c22)e−λτ−c12λ(λ−c12)e−λτ|τ=τj0}=Re{(2λ−(c11+c22))eλτ−c12)−c12λ(λ−c12)|τ=τj0−τλ|τ=τj0}=Re{2iω(cos(ωτ)+isin(ωτ))−(c11+c22)(cos(ωτ)+isin(ωτ))−c12)−c12iω(iω−c12)|τ=τj0}=2ω20+C1ω20+c222=√C21−4C0ω20+c222, |
obviously, one can get Re(dλdτ|τ=τj0)−1>0, then the transversality condition Re(dλdτ|τ=τj0)>0 holds.
Through further collation, the expression C21−4C0=(c211−c212−c222)2>0. One can get the following result.
Lemma 4.4. If condition (H3) K(ab+θ)bh<1 holds, then Real parts of all roots of characteristic equation (4.7) at E01 are negative.
The proof process is similar to Lemma 4.2, We don't elaborate here. Note that Lemma 4.4 show that E01 is always locally asymptotically stable for τ≥0.
Theorem 4.3. Suppose that R0<1 and (H2) K(ab+θ)bh>1 hold, then one can get the following results:
(i) The disease-free equilibrium E01 of system (3.4) is locally asymptotically stable for τ∈[0,τ00), and is unstable when τ∈(τ00,+∞).
(ii) System (3.4) undergoes a Hopf bifurcation at the E01 when τ=τj0 (j=0,1,2,⋯).
Remark 4.3. On the basis of similar theoretical analysis, system (3.4) at E0 undergoes same dynamic behavior when K is sufficiently large.
Case II: When we consider E∗, it is easy to calculate
c11=b(aα−βh)Kβ, c12=−bh(Kβ−α)Kα, c13=−α, c21=(abK+Kθ+bh)K(ˆR0−1), c22=0. |
Then C1=c211−c212+2c13c21, C0=c213c221>0.
Lemma 4.5. If condition (H4) C1>0 and C21−4C0>0 holds, then all roots of characteristic equation (4.7) at E∗ have negative real parts for τ≥0.
Proof: If condition (H4) holds, then Eq (4.11) has two roots:
Z∗1=−C1+√C21−4C02, Z∗2=−C1−√C21−4C02, |
and Z∗2<Z∗1<0 based on Weida's theorem. By combining the stability of E∗ with respect to τ=0, then the real parts of all roots of characteristic equation (4.7) at E∗ are negative for τ≥0.
Lemma 4.5. indicates that E∗ is always locally asymptotically stable for τ≥0.
Lemma 4.6. If condition (H5) C1<0 and C21−4C0>0 holds, then characteristic equation (4.7) at E∗ has two pairs of pure imaginary roots, defined by
ω∗1=√22√−C1+√C21−4C0, ω∗2=√22√−C1−√C21−4C0. |
Proof: When condition (H5) holds, the two roots of Eq (4.11) are
Z∗1=−C1+√C21−4C02, Z∗2=−C1−√C21−4C02, |
it is easy to obtain that Z∗1>Z∗2>0. We thus get that ω∗i=√Z∗i>0(i=1,2), which indicates that Eq (4.7) at E∗ has two pairs of pure imaginary roots.
According to Eq (4.9) and Lemma 4.6, we give two critical bifurcation values corresponding to ω∗1 and ω∗2:
τm∗1=1ω∗1(arccos(−c11c12)+2mπ), m=0,1,2,⋯, |
τn∗2=1ω∗2(arccos(−c11c12)+2nπ), n=0,1,2,⋯. |
Lemma 4.7. Assume that the conditions of Lemma 4.6 are satisfied, then τ0∗1<τ0∗2.
Proof: Denote
F(ω)=1ω(arccos(−c11c12)), |
it is obvious that F(ω) is a monotonically decreasing function with respect to ω. Thus one can obtain τ0∗1<τ0∗2 when ω0∗1>ω0∗2.
Theorem 4.4. On the basis of Lemma 4.6 and Lemma 4.7, then there exist a integer r≥0 such that
(i) when τ∈[0,τ0∗1)⋃(τ0∗2,τ1∗1)⋃(τ1∗2,τ2∗1)⋃⋯⋃(τr−1∗2,τr∗1), the endemic equilibrium E∗ is locally asymptotically stable;
(ii) when τ∈(τ0∗1,τ0∗2)⋃(τ1∗1,τ1∗2)⋃(τ2∗1,τ2∗2)⋃⋯⋃(τr−1∗1,τr−1∗2) and τ>τr∗1, the endemic equilibrium E∗ becomes unstable.
Proof: The transversality conditions
Re(dλdτ)|τ=τm∗1,λ=iω∗1>0, Re(dλdτ)|τ=τn∗2,λ=iω∗2<0 |
are easy to prove, which indicates that a pair of roots of Eq (4.7) cross the imaginary axis at iω∗1 into the right-plane for each τ=τm∗1, and a pair of roots cross the imaginary axis at iω∗2 and then return to left-half plane for each τ=τn∗2, then system (3.4) experiences stability switches with the increase of τ. Moreover, we further derive
τm+1∗1−τm∗1=2πω∗1<τn+1∗2−τn∗2=2πω∗2, |
due to ω∗1>ω∗2, which clearly shows that such alternation can't continue for entire sequences. So there exists a finite integer r such that
τr−1∗1<τr−1∗2<τr∗1<τr+1∗1<τr∗2, |
and the multiplicity of roots in right-half plane is at least two when τ>τr∗1, then E∗ of system (3.4) is unstable.
Remark 4.4. According to the above dynamic analysis, we can deduce that stability switches occurs at E∗ for system (3.4) for sufficiently large K.
For the parameter values in the system (3.4) shown in Table 2, we give the following explanation.
Parameters | Unit | value |
b | month | 0.0027 95%CI: [0.002,0.0034] |
e | month | 201.8030 95%CI: [190.8515,213.025] |
C | month | 5×107 |
τ1 | month | 6 |
τ2 | month | 6 |
K | month | 5.4372×108 |
β | month | 5.1928×10−9 95%CI:[4.9923×10−9,5.2409×10−9] |
θ | month | 0.052 |
α | month | 2 |
a1 | / | 10.1148 95%CI: [8.4408,11.8373] |
a0 | / | 0 |
(1) According to the price function P(t+τ2)=eCS(t)−a1 and τ2=6, we construct the objective function
min∑t[P(t+τ2)−ˆP(t+τ2)]2, |
where ˆP(t+τ2) is the actual price of live pigs, P(t+τ2) is the theoretical price. Based on live pig price from September 2017 to July 2021 and inventory data from March 2017 to January 2021 in Table 1, we apply the least square estimation method to estimate the parameters e and a1. Suppose that the actual pig price ˆP(t+τ2)×10 has an error that obeys to the Poisson distribution. we carry out random simulation for 1000 times, and obtain the data fitting result in Figure 3 and the values of e and a1 in Figure 4.
(2) Since the number of culled infected pigs due to ASF is very small compared to the total number of breeding, we ignore the number of culled infected pigs, then the total breeding volume satisfies the Eq (5.1). The number of culled susceptible pigs due to the infected pigs in the same group is implied in parameters b or θ.
dSdt=b[eCS(t−τ)−a]S(t)(1−S(t)K)−θS(t). | (5.1) |
We build the objective function
min∑t[S(t)−ˆS(t)]2, |
where, ˆS(t) is the actual inventory of pigs in China, and S(t) is the theoretical inventory. Similarly, according to the inventory data from August 2018 to December 2019 in Table 1, we apply the least squares estimation method to estimate the parameter b. Assume that the actual stock data ˆS(t)/106 has an error with a Poisson distribution. Random simulation is performed 1000 times, and the data fitting result is shown in Figure 5. The estimated parameter value is shown in Table 2 and its histogram is given in Figure 6.
(3) C is the market demand for pork products in the current. Since 2014, the pork market in China has been very stable. According to statistics, the Chinese consume more than 600 million pigs per year. Therefore, the monthly consumption of pigs can be taken as 5×107.
(4) K is the environmental capacity. Here we refer to the highest peak value of domestic pigs raised in a single month, 5.4372×108.
(5) There are two litters a year for one sow, and each pregnancy period is more than 5 months, so the value of τ1 is 6 months.
(6) θ can be derived from the ratio of monthly sold pigs to whole stocks. By comparing with the data, it is found that the ratio of monthly sold pigs to stocks is basically stable, except for months that Chinese Spring Festival and Mid-Autumn Festival come in. The fluctuation of the ratio is very small, so here the average value is adopted.
(7) African swine fever causes high fever, loss of appetite, internal organs and skin bleeding. The mortality rate is as high as 100% and infected pigs will die within half a month. So, α=1/2.
(8) β is the infection rate coefficient between susceptible pigs and infected pigs. According to the model (3.4),
W(t)≜∑tαI(t) |
is the theoretical cumulative number of infected and culled pigs, and the actual data is denoted by ˆW(t). By establishing an objective function
min∑t[W(t)−ˆW(t)]2, |
we estimate the parameter β by applying the least square estimation. It is assumed that the actual infection data ˆW(t)/100 has an error with a Poisson distribution. The parameter estimation result is shown in Table 2 and its histogram is given in Figure 8. The data fitting results are shown in Figure 7.
Since protective immunity is not considered in this paper, all existing pigs can be divided into two categories: susceptible pigs (S) healthy pigs but may be infected with African swine fever in the future; the infected (I) are already infected with African swine fever but have not been reported and confirmed. In the initial stage of the disease, the number of infected pigs is small, so it is assumed that all pigs are susceptible. In this case, a index, the basic reproduction number, can be applied to judge whether the epidemic can persist with time. According to [15,16,17], the expression of R0 is given as
R0=βαS01. |
During the pandemic phase, the effective reproduction number R0(t)=βαS(t) can be used to judge the real-time development trend of the epidemic. The graph of R0 in term of the pig stock S is given in Figure 9. As can be seen from Figure 8, in the presence of diseases, the amount of breeding is positively related to the basic reproductive number. The greater the amount of breeding is, the less conducive to disease control. When the stock of live pigs is 385 million, the basic reproductive number R0=1. That is to say, with the raising quantity less than 385 million, the disease can be controlled with time. From August 2018 to the end of 2019, due to the outbreak of African swine fever, a large number of live pigs were culled, and many small-scale pig farms with smaller scale were forced to shut down, resulting in a reduction in the number of live pigs. The stock of live pigs at the end of 2019 was only over 220 million, and the corresponding the effective reproductive number R0=0.6005. The currently available data show that by the end of 2020, the number of live pigs will be 300 million, and the corresponding the basic reproductive number R0<1, which means that the epidemic cannot re-outbreak temporarily. But, it is important to notice that when raising quantity is more than 385 million as the livestock breeding industry recovers, if the virus is not completely eradicated, the disease may rebound.
Now, when African swine fever persists with time, we can apply the basic reproduction number R0 to assess the effect of the epidemic on the price of the live pigs. The stock of live pigs and the price of live pigs interact each other, and in the steady state they satisfy
P∗=eCS∗−a1. |
Then, we have
S∗=αβ=S01R0. |
Substituting the above formula into the expression of P∗, we can get
P∗=eCR0S01−a1, |
and the function graph of P∗, the pig price in the steady state, in term of R0 can be given in Figure 10. It can be seen that the price P∗ will increases linearly with the basic reproductive number. When the basic reproductive number R0=1, the corresponding live pig price is 33.5 yuan/kg. When R0=1.5, the corresponding live pig price can arrive more than 55 yuan/kg.
In this subsection, we show the influence of critical parameters on the R0 in Figure 11(a)–(c).
(1) The increasing of b can lead to an increase in the stock of live pigs, which leads to an increase in R0. When b=0.0111, R0 = 1. When b is smaller, variation amplitude of R0 is larger. When b is larger, with the increase of b, the variation amplitude of R0 becomes smaller.
(2) The infection rate coefficient has a linear relationship with R0. As β increases, R0 also increases. When β=8.65×10−9, R0=1.
(3) The disease elimination rate α and R0 show an inverse relationship. When α=1.2, R0 = 1. When α is small, as α increases, R0 decreases fast. When α is larger, as α increases, the reduction amplitude of R0 becomes smaller.
Combining with Figure 9 and Figure 10, it is concluded that in order to ensure that the disease can be effectively controlled when it occurs, it need to manage the stock of live pigs and eliminate the infected pigs timely.
The values of parameters in system (3.4) are given in Table 2, then we calculate that system (4.1) without delay only has two disease-free equilibria E01=(2.3335×108,0), E02=(2.3814×109,0) and the basic reproductive number R0=0.6934 based on section 4. We further find that E02 is unstable, while E01 is locally asymptotically stable, which is independent of the initial value from Figure 12(a), (b). According to the expression of the critical value τ in (4.12), we obtain τ00=39.8972. Figure 12 shows that E01 of system (3.4) shall become unstable, and a family of asymptotically stable periodic solutions occurs, namely, the number of S(t) oscillates periodically with time. In view of the magnitude of the oscillation amplitude of I(t) is about 10−7, so we don't show the variation of I(t) here.
In order to observe the influence of the change of transmission rate β on the dynamic behavior of system (3.4). Figure 13(a) shows that the relation τj∗1<τj∗2 always holds with increase of β for j=0,1,2, while the sequence τ0∗1<τ0∗2<τ1∗1<τ1∗2<τ2∗1<τ2∗2 is not always satisfied, which can be observed that the red solid line (τ1∗1) is lower than the dark green dashed line (τ0∗2) when β>β=4×10−8, and the blue solid line (τ2∗1) gradually approaches to less than the magenta dashed line (τ1∗2) with the increase of β, such phenomenon verifies the content of Theorem 4.4. In addition, the basic reproductive number R0 increases linearly with increase of transmission rate β from Figure 13 (b).
The relationship between supply and demand of live pigs directly affects its price, further affects people's daily life. From 2014 to 2018, the amount of the pigs stock always maintained at more than 4×108 and the price of live pigs in China was relatively stable. The outbreak of African swine fever in 2018 caused a huge decrease in the breeding volume and the shortage of pork, which broke the balance of supply and demand for pork products, and ultimately caused pork prices to rise in 2019. Based on the traditional SI epidemic model to describe the spread of the African swine fever, we add a price function to construct a new time delay dynamical model to explore the relationship between African swine fever, the number of pigs in stock, and the price of live pigs. It is showed that from November 2018, the number of live pigs was falling for more than one year. From January 2020, the number of live pigs begin to increase. In Figure 14, we predict that between September to December 2021, the stock of live pigs will arrive the peak value about 365 million. In this case, combined with Figure 9, when the stock of live pigs is 365 million, the value of R0 is less than 1, but nears 1. So it is obtained that there is also the possibility of an outbreak of an African swine fever with the recovery of pig feeding number. Since the model in this paper does not consider the seasonal demand of live pigs in the Spring Festival, so the fitting result of model in January 2020 is higher than the actual data. From Figure 15, it shows that due to a period of time lag τ2, the price of live pigs (red dot) can reach the minimum value in March to May 2022.
R0 is the index to judge whether the disease will eventually become epidemic. In Figure 10, we give the effect of R0 on the price of the live pigs in the steady state under the case that African swine fever becomes endemic disease. In fact, what we want to know is the real-time effect of the epidemic on the price of live pigs, which can be assessed by I(t). According to the African Swine Fever Prevention and Control Law, if there appear one confirmed case of African Swine Fever in a farm, all pigs in the entire farm must be culled and subjected to biological isolation. Since most of live pig farms in China in recent years are medium and large farms, the number of raised live pigs generally exceeds 10, 000. It can be obtained that one African swine fever case will lead to reduce the number of susceptible pigs by 10, 000. According to the Ministry of Agriculture of China, 19, 793 cumulative cases of African swine fever disease has been reported, which will lead to about 2×108 susceptible pigs to be culled. From Table 1, the number of live pigs before the occurrence of the African swine fever in August 2018 was 393 million, and the number of live pigs in January 2020 was only 196 million, which is also in line with above assumptions.
Then, we have
P(t+τ2)=eCS(t)−a1=eCS(0)−10000×I(t)−a1. | (6.1) |
The effect of I(t) on the price at time P(t+τ2) can be see Figure 16, and they are non-linear related. It shows that when there is 2, 000 pigs to be infected, the subsequent price will be about 16.5 yuan/kg. If the number of the infected arrives 10000, the price will be 23.5 yuan/kg. If the number of the infected arrives 20, 000, the price will be more than 40 yuan/kg.
From above analysis, it can be seen that the model we establish not only can be used to excavate inner relationship between the epidemic and the price of live pigs, but also can predict their development trend. By fitting history data to confirm the reasonability of model, we can predict the trend of pig stock and price in the future, and propose macroscopic suggestions on the forecast of pig market. In addition, the model we establish can be used to simulate similar situations of other animal infectious disease: After the outbreak of an animal disease, the model can predict the price trend of the animal product based on the number of animal stocks, the price of the animal product, and the scale of the disease outbreak (the number of the infected or the positive rate). In this way, the impact of the epidemic on the economy can be assessed.
This article studies the impact of African swine fever on the price of live pigs and the stock of live pigs. Regarding the prevention of African swine fever, Benoit et al. studied the trade of live pigs on the network, and believed that they were more likely to be infected with African swine fever during the transportation of live pigs[25]. Lisa and Tomasz et al. concluded that wild boar activities have a great influence on the spread of African swine fever virus[26,27]. From the above assumptions, is the African swine fever disease in China caused by the African swine fever virus carried by wild boar? Breeding farms are mostly built in remote suburbs away from the city center. Can reducing the number of wild boars around the farms reduce the infection rate of African swine fever? What kind of bio-epidemic prevention and road supervision should be implemented during the transportation of live pigs to reduce the exposure rate of live pigs to the African swine fever virus. These are all valuable tasks that can be investigated in future.
This work was supported by the National Natural Science Foundation of China (Grant Nos. 61873154, and 12001340), the Natural Science Foundation of Shanxi Province (Grant No. 201901D211411), the Universities' Science and Technology Innovation Item of Shanxi Province (2019L0472), the Shanxi Scholarship Council of China (HGKY2019004), the Scientific and Technological Innovation Programs (STIP) of Higher Education Institutions in Shanxi (2019L0082, 2019L0114), General Youth Fund project in Shanxi Province(201901D211158). We would like to thank the anonymous referee for the careful reading and helpful suggestions which lead to a significant improvement of our original manuscript.
The authors have declared that no competing interests exist.
[1] | P. Guo, Chinese people eat 600 million pigs per year, which is 52% of the world's pigs, Available from: https://www.pig66.com/2018/145_1018/17343759.html. |
[2] | J. B. Zhang, China is short of pork, and the whole world can't help, Available from: https://baijiahao.baidu.com/s?id = 1643664282919321235&wfr = spider&for = pc. |
[3] | Sina Finance, China's pork consumption hit a 15-year low in 2019, Available from: http://finance.sina.com.cn/roll/2020-05-10/doc-iirczymk0905792.shtml. |
[4] | Baidu, Shenyang occur one cases of African swine fever epidemic 913 pigs have been culled, Available from: https://baijiahao.baidu.com/s?id = 1607763410109998748&wfr = spider&for = pc. |
[5] | Information Office of the Ministry of Agriculture and Rural Affairs, Live pigs transferred from Heilongjiang to Zhengzhou, Henan were diagnosed with African swine fever, Available from: http://www.moa.gov.cn/gk/yjgl_1/yqfb/201808/t20180816_6155815.htm. |
[6] | X. T. Zhou, N. Li, Y. Z. Luo, R. L. Hu, Emergence of African Swine Fever in China, Transboundary Emerging Dis., 2018 (2018). |
[7] | S. N. Xiao, J. Wang, S. Y. Wang, L. F. Yang, B. Geng, M. X. Li, et al., Analysis of the existing problems and countermeasures in the prevention and control of African swine fever at the grassroots level, Chi. Veter. J., 54 (2018), 112–114. |
[8] | China Business Network, African swine fever triggers major changes in the Chinese pig industry chain, Jiangxi Feed, 06 (2018), 50–52. |
[9] | J. F. Ding, Stopping the leak from the source, cutting off the feeding chain of "swill pigs", China Business Daily, 31 October 2018, P02. |
[10] |
J. Nielsen, T. Larsen, T. Halasa, L. Christianse, Estimation of the transmission dynamics of African swine fever virus within a swine house, Epidemiol. Infect., 145 (2017), 2787–2796. doi: 10.1017/S0950268817001613
![]() |
[11] |
M. B. Barongo, R. P. Bishop, E. M. Fevre, D. L. Knobel, A. Ssematimba, A mathematical model that simulates control options for African swine fever virus (ASFV), PLoS One, 11 (2016), e0158658. doi: 10.1371/journal.pone.0167791
![]() |
[12] |
A. Kouidere, O. Balatif, M. Rachik, Analysis and optimal control of a mathematical modeling of the spread of African swine fever virus with a case study of South Korea and cost-effectiveness, Chaos Solitons Fractals, 146 (2021), 110867. doi: 10.1016/j.chaos.2021.110867
![]() |
[13] |
H. S. Lee, K. K. Thakur, L. Pham-Thanh, T. D. Dao, A. N. Bui, V. N. Bui, et al., A stochastic network-based model to simulate farm-level transmission of African swine fever virus in Vietnam, Plos one, 16 (2021), e0247770. doi: 10.1371/journal.pone.0247770
![]() |
[14] | Z. Zhan, M. Q. Li, Y. Ji, H. S. Li, Study on the Influence of African Swine Fever on the Fluctuation of Meat Prices in China: Eemprical Analysis Based on PVAR Model, Chi. J. Agric. Mach. Chem., 42 (2021), 173–178. |
[15] | Y. Tan, X. N. Wang, Research on the time-varying impact of African swine fever public opinion on pork price fluctuations in my country, South. Rural Areas, 37 (2021), 22–36. |
[16] | Q. Xiao, Y. Zhou, The impact of disease on pork price fluctuations——based on the perspective of supply and demand, Heilongjiang Anim. Husb. Vet. Med., 02 (2019), 12–16. |
[17] | D. B. Gao, Research on cobweb economic model with type Ⅱ functional response, Math. Pract. Knowl., 50 (2020), 133–142. |
[18] | B. D. Li, Cobweb model and its mathematical mechanism analysis, J. Lanzhou Commer. Coll., 5 (2001), 75–77. |
[19] | L. Lin, Several differential equation models in economic systems, J. Grad. Sch. Chin. Acad. Sci., 3 (2003), 273–278. |
[20] | M. H. Su, C. C. Hua, Cobweb model in the form of differential equations, J. Yunnan Univ., 2007 (2007), 20–23. |
[21] | L. Ming, Z. J. Yang, Qualitative analysis of continuous cobweb model, Stat. Decis., 2 (2013), 14–17. |
[22] | Iime data center, Available from: https://data.iimedia.cn/. |
[23] |
P. Van den Driessche, J. Watmough, Reproduction numbers and sub-threshold endemic equilibria for compartmental models of disease transmission, Math. Biosci., 180 (2002), 29–48. doi: 10.1016/S0025-5564(02)00108-6
![]() |
[24] |
O. Diekmann, J. A. P. Heesterbeek, M. G. Roberts, The construction of nextgeneration matrices for compartmental epidemic models, J. R. Soc. Interface, 7 (2010), 873–885. doi: 10.1098/rsif.2009.0386
![]() |
[25] |
B. Govoeyi, A. M. Agbokounou, Y. Camara, S. G. Ahounou, I. O. Dotche, P. S. Kiki, et al., Social network analysis of practice adoption facing outbreaks of African Swine Fever, Prev. Vet. Med., 179 (2020), 105008. doi: 10.1016/j.prevetmed.2020.105008
![]() |
[26] |
L. A. Pollock, E. J. Newton, E. L. Koen, Predicting high-risk areas for African swine fever spread at the wild-domestic pig interface in Ontario, Prev. Vet. Med., 191 (2021), 105341. doi: 10.1016/j.prevetmed.2021.105341
![]() |
[27] |
T. Podgorski, T. Borowik, M. Lyjak, G. Wozniakowski, Spatial epidemiology of African swine fever: Host, landscape and anthropogenic drivers of disease occurrence in wild boar, Prev. Vet. Med., 177 (2020), 104691. doi: 10.1016/j.prevetmed.2019.104691
![]() |
1. | Asier Minondo, Exporters' reaction to positive foreign demand shocks, 2023, 0965-7576, 10.1111/roie.12663 | |
2. | Jordi Casal, Damián Tago, Pilar Pineda, Blagojcho Tabakovski, Imelda Santos, Carolyn Benigno, Tran Huynh, Giovanna Ciaravino, Daniel Beltran‐Alcrudo, Evaluation of the economic impact of classical and African swine fever epidemics using OutCosT, a new spreadsheet‐based tool, 2022, 69, 1865-1674, 10.1111/tbed.14590 | |
3. | Din Prathumwan, Inthira Chaiya, Kamonchat Trachoo, Study of Transmission Dynamics of Streptococcus suis Infection Mathematical Model between Pig and Human under ABC Fractional Order Derivative, 2022, 14, 2073-8994, 2112, 10.3390/sym14102112 | |
4. | Huaicheng Liu, Jianwen Zou, Rongchao Liu, Jing Chen, Xiaohan Li, Haixue Zheng, Long Li, Bin Zhou, Development of a TaqMan-Probe-Based Multiplex Real-Time PCR for the Simultaneous Detection of African Swine Fever Virus, Porcine Circovirus 2, and Pseudorabies Virus in East China from 2020 to 2022, 2023, 10, 2306-7381, 106, 10.3390/vetsci10020106 | |
5. | Penglu Zhang, Tong Nie, Jun Ma, Hao Chen, Identification of suitable areas for African swine fever occurrence in china using geographic information system-based multi-criteria analysis, 2022, 209, 01675877, 105794, 10.1016/j.prevetmed.2022.105794 | |
6. | Senada Kalabušić, Esmir Pilav, Global behaviour of a class of discrete epidemiological SI models with constant recruitment of susceptibles, 2022, 28, 1023-6198, 259, 10.1080/10236198.2022.2042277 | |
7. | Huan Wang, Meijun Chen, Ziyao Guo, Yangyang Shen, Yufan Chen, Ting Luo, Yuying Liu, Jianqiang Li, Fang Wang, Jiangjun Wan, The Influencing Factors of “Post-African Swine Fever” Pig Farm Biosecurity: Evidence from Sichuan Province, China, 2023, 13, 2076-2615, 3053, 10.3390/ani13193053 | |
8. | Shraddha Tiwari, Thakur Dhakal, Tae-Su Kim, Seong-Hyeon Kim, Sang-Joon Lee, Dae-Sung Yoo, Ho-Seong Cho, Gab-Sue Jang, Yeonsu Oh, Jordi Casal, Global Basic Reproduction Number of African Swine Fever in Wild Boar and a Mental Model to Explore the Disease Dynamics, 2024, 2024, 1865-1682, 1, 10.1155/2024/1046866 | |
9. | Ing-Marie Gren, Hans Andersson, Lars Jonasson, Benefits and costs of measures to tackle the outbreak of African swine fever in Sweden, 2024, 233, 01675877, 106353, 10.1016/j.prevetmed.2024.106353 | |
10. | Mengying Dou, Md. Abul Kalam Azad, Yating Cheng, Sujuan Ding, Yang Liu, Bo Song, Xiangfeng Kong, Expressions of Insulin-like Growth Factor System among Different Breeds Impact Piglets’ Growth during Weaning, 2023, 13, 2076-2615, 3011, 10.3390/ani13193011 | |
11. | Dana C. Pittman Ratterree, Sapna Chitlapilly Dass, Martial L. Ndeffo-Mbah, The Reproduction Number of Swine Viral Respiratory Diseases: A Systematic Review, 2024, 11, 2306-7381, 300, 10.3390/vetsci11070300 | |
12. | Juan Li, Junhui Zhang, Lu Gao, Bingxin Nie, Huaiping Zhu, Evaluating the impact of swill-feeding on the control of African swine fever in China with a dynamic model, 2024, 186, 09600779, 115262, 10.1016/j.chaos.2024.115262 | |
13. | Melissza Salling, Assessing the price effects of African swine fever in the China market, 2025, 3, 10.37349/eff.2025.101064 | |
14. | Dapeng Zhou, Jing Zhang, Honghua Huan, Nanyan Hu, Yinqiu Li, Jinhua Cheng, Assessing the Impact of External Shocks on Prices in the Live Pig Industry Chain: Evidence from China, 2025, 17, 2071-1050, 1934, 10.3390/su17051934 | |
15. | Arianna Ceruti, Rea Maja Kobialka, Ahmed Abd El Wahed, Uwe Truyen, African Swine Fever: A One Health Perspective and Global Challenges, 2025, 15, 2076-2615, 928, 10.3390/ani15070928 |
Time | The stock of live pigs (Unit: million) | The price of live pigs (Unit: China Yuan/kg) | Cumulative number of reported infected pigs by ASF (Unit: Individual) |
2017.03 | 41305.41 | 16.71 | |
2017.04 | 41466.79 | 16 | |
2017.05 | 41010.73 | 14.63 | |
2017.06 | 40845.35 | 13.78 | |
2017.07 | 40596.74 | 13.96 | |
2017.08 | 40407.91 | 14.42 | |
2017.09 | 40357.91 | 14.75 | |
2017.10 | 40322.69 | 14.52 | |
2017.11 | 40356.89 | 14.47 | |
2017.12 | 39900.84 | 15.07 | |
2018.01 | 41529.58 | 15.25 | |
2018.02 | 40668.07 | 14.06 | |
2018.03 | 41334.64 | 11.91 | |
2018.04 | 41196.72 | 10.93 | |
2018.05 | 40452.93 | 10.57 | |
2018.06 | 39811.29 | 11.32 | |
2018.07 | 39464.27 | 12.02 | |
2018.08 | 39340.35 | 13.6 | 1277 |
2018.09 | 39558.9 | 13.95 | 2246 |
2018.10 | 39581.39 | 14.03 | 5991 |
2018.11 | 39353.89 | 13.99 | 7143 |
2018.12 | 38299.13 | 13.98 | 8097 |
2019.01 | 36459.77 | 13.87 | 15448 |
2019.02 | 33493.43 | 12.68 | 17849 |
2019.03 | 33638.04 | 14.35 | 17982 |
2019.04 | 32712.57 | 15 | 18878 |
2019.05 | 31493.42 | 15 | 19087 |
2019.06 | 29830.47 | 16 | 19292 |
2019.07 | 27176.34 | 17.6 | 19391 |
2019.08 | 24431.67 | 21.85 | 19506 |
2019.09 | 23517.14 | 26.8 | 19521 |
2019.10 | 23227.07 | 33.38 | 19546 |
2019.11 | 23743.76 | 34.89 | 19704 |
2019.12 | 24095.79 | 33.3 | 19793 |
2020.01 | 19580.44 | 34.2 | |
2020.02 | 20128.69 | 36.6 | |
2020.03 | 20873.45 | 35.4 | |
2020.04 | 21812.76 | 33.6 | |
2020.05 | 22663.46 | 30.2 | |
2020.06 | 23660.65 | 32.3 | |
2020.07 | 24796.36 | 35.8 | |
2020.08 | 25961.79 | 37.1 | |
2020.09 | 27156.03 | 36.5 | |
2020.10 | 28296.59 | 32.7 | |
2020.11 | 29513.34 | 29.7 | |
2020.12 | 30280.69 | 33.1 | |
2021.01 | 29188.69 | 35.5 | |
2021.02 | 29101.13 | 31.8 | |
2021.03 | 29974.16 | 28.2 | |
2021.04 | 23.8 | ||
2021.05 | 19.7 | ||
2021.06 | 15.6 | ||
2021.07 | 15.9 |
Parameters | Unit | value |
b | month | 0.0027 95%CI: [0.002,0.0034] |
e | month | 201.8030 95%CI: [190.8515,213.025] |
C | month | 5×107 |
τ1 | month | 6 |
τ2 | month | 6 |
K | month | 5.4372×108 |
β | month | 5.1928×10−9 95%CI:[4.9923×10−9,5.2409×10−9] |
θ | month | 0.052 |
α | month | 2 |
a1 | / | 10.1148 95%CI: [8.4408,11.8373] |
a0 | / | 0 |
Time | The stock of live pigs (Unit: million) | The price of live pigs (Unit: China Yuan/kg) | Cumulative number of reported infected pigs by ASF (Unit: Individual) |
2017.03 | 41305.41 | 16.71 | |
2017.04 | 41466.79 | 16 | |
2017.05 | 41010.73 | 14.63 | |
2017.06 | 40845.35 | 13.78 | |
2017.07 | 40596.74 | 13.96 | |
2017.08 | 40407.91 | 14.42 | |
2017.09 | 40357.91 | 14.75 | |
2017.10 | 40322.69 | 14.52 | |
2017.11 | 40356.89 | 14.47 | |
2017.12 | 39900.84 | 15.07 | |
2018.01 | 41529.58 | 15.25 | |
2018.02 | 40668.07 | 14.06 | |
2018.03 | 41334.64 | 11.91 | |
2018.04 | 41196.72 | 10.93 | |
2018.05 | 40452.93 | 10.57 | |
2018.06 | 39811.29 | 11.32 | |
2018.07 | 39464.27 | 12.02 | |
2018.08 | 39340.35 | 13.6 | 1277 |
2018.09 | 39558.9 | 13.95 | 2246 |
2018.10 | 39581.39 | 14.03 | 5991 |
2018.11 | 39353.89 | 13.99 | 7143 |
2018.12 | 38299.13 | 13.98 | 8097 |
2019.01 | 36459.77 | 13.87 | 15448 |
2019.02 | 33493.43 | 12.68 | 17849 |
2019.03 | 33638.04 | 14.35 | 17982 |
2019.04 | 32712.57 | 15 | 18878 |
2019.05 | 31493.42 | 15 | 19087 |
2019.06 | 29830.47 | 16 | 19292 |
2019.07 | 27176.34 | 17.6 | 19391 |
2019.08 | 24431.67 | 21.85 | 19506 |
2019.09 | 23517.14 | 26.8 | 19521 |
2019.10 | 23227.07 | 33.38 | 19546 |
2019.11 | 23743.76 | 34.89 | 19704 |
2019.12 | 24095.79 | 33.3 | 19793 |
2020.01 | 19580.44 | 34.2 | |
2020.02 | 20128.69 | 36.6 | |
2020.03 | 20873.45 | 35.4 | |
2020.04 | 21812.76 | 33.6 | |
2020.05 | 22663.46 | 30.2 | |
2020.06 | 23660.65 | 32.3 | |
2020.07 | 24796.36 | 35.8 | |
2020.08 | 25961.79 | 37.1 | |
2020.09 | 27156.03 | 36.5 | |
2020.10 | 28296.59 | 32.7 | |
2020.11 | 29513.34 | 29.7 | |
2020.12 | 30280.69 | 33.1 | |
2021.01 | 29188.69 | 35.5 | |
2021.02 | 29101.13 | 31.8 | |
2021.03 | 29974.16 | 28.2 | |
2021.04 | 23.8 | ||
2021.05 | 19.7 | ||
2021.06 | 15.6 | ||
2021.07 | 15.9 |
Parameters | Unit | value |
b | month | 0.0027 95%CI: [0.002,0.0034] |
e | month | 201.8030 95%CI: [190.8515,213.025] |
C | month | 5×107 |
τ1 | month | 6 |
τ2 | month | 6 |
K | month | 5.4372×108 |
β | month | 5.1928×10−9 95%CI:[4.9923×10−9,5.2409×10−9] |
θ | month | 0.052 |
α | month | 2 |
a1 | / | 10.1148 95%CI: [8.4408,11.8373] |
a0 | / | 0 |