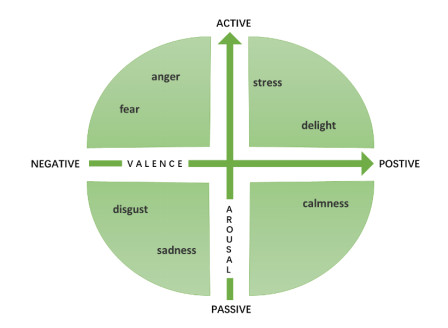
Brain network can be well used in emotion analysis to analyze the brain state of subjects. A novel dynamic brain network in arousal is proposed to analyze brain states and emotion with Electroencephalography (EEG) signals.
Time factors is integrated to construct a dynamic brain network under high and low arousal conditions. The transfer entropy is adopted in the dynamic brain network. In order to ensure the authenticity of dynamics and connections, surrogate data are used for testing and analysis. Channel norm information features are proposed to optimize the data and evaluate the level of activity of the brain.
The frontal lobe, temporal lobe, and parietal lobe provide the most information about emotion arousal. The corresponding stimulation state is not maintained at all times. The number of active brain networks under high arousal conditions is generally higher than those under low arousal conditions. More consecutive networks show high activity under high arousal conditions among these active brain networks. The results of the significance analysis of the features indicates that there is a significant difference between high and low arousal.
Compared with traditional methods, the method proposed in this paper can analyze the changes of subjects' brain state over time in more detail. The proposed features can be used to quantify the brain network for accurate analysis.
The proposed dynamic brain network bridges the research gaps in lacking time resolution and arousal conditions in emotion analysis. We can clearly get the dynamic changes of the overall and local details of the brain under high and low arousal conditions. Furthermore, the active segments and brain regions of the subjects were quantified and evaluated by channel norm information.This method can be used to realize the feature extraction and dynamic analysis of the arousal dimension of emotional EEG, further explore the emotional dimension model, and also play an auxiliary role in emotional analysis.
Citation: Yunyuan Gao, Zhen Cao, Jia Liu, Jianhai Zhang. A novel dynamic brain network in arousal for brain states and emotion analysis[J]. Mathematical Biosciences and Engineering, 2021, 18(6): 7440-7463. doi: 10.3934/mbe.2021368
[1] | Yanling An, Shaohai Hu, Shuaiqi Liu, Bing Li . BiTCAN: An emotion recognition network based on saliency in brain cognition. Mathematical Biosciences and Engineering, 2023, 20(12): 21537-21562. doi: 10.3934/mbe.2023953 |
[2] | Dingxin Xu, Xiwen Qin, Xiaogang Dong, Xueteng Cui . Emotion recognition of EEG signals based on variational mode decomposition and weighted cascade forest. Mathematical Biosciences and Engineering, 2023, 20(2): 2566-2587. doi: 10.3934/mbe.2023120 |
[3] | Tianhui Sha, Yikai Zhang, Yong Peng, Wanzeng Kong . Semi-supervised regression with adaptive graph learning for EEG-based emotion recognition. Mathematical Biosciences and Engineering, 2023, 20(6): 11379-11402. doi: 10.3934/mbe.2023505 |
[4] | Xiaowen Jia, Jingxia Chen, Kexin Liu, Qian Wang, Jialing He . Multimodal depression detection based on an attention graph convolution and transformer. Mathematical Biosciences and Engineering, 2025, 22(3): 652-676. doi: 10.3934/mbe.2025024 |
[5] | Zhongwen Jia, Lihan Tang, Jidong Lv, Linhong Deng, Ling Zou . Depression-induced changes in directed functional brain networks: A source-space resting-state EEG study. Mathematical Biosciences and Engineering, 2024, 21(9): 7124-7138. doi: 10.3934/mbe.2024315 |
[6] | Yujie Kang, Wenjie Li, Jidong Lv, Ling Zou, Haifeng Shi, Wenjia Liu . Exploring brain dysfunction in IBD: A study of EEG-fMRI source imaging based on empirical mode diagram decomposition. Mathematical Biosciences and Engineering, 2025, 22(4): 962-987. doi: 10.3934/mbe.2025035 |
[7] | Enas Abdulhay, Maha Alafeef, Hikmat Hadoush, V. Venkataraman, N. Arunkumar . EMD-based analysis of complexity with dissociated EEG amplitude and frequency information: a data-driven robust tool -for Autism diagnosis- compared to multi-scale entropy approach. Mathematical Biosciences and Engineering, 2022, 19(5): 5031-5054. doi: 10.3934/mbe.2022235 |
[8] | Gayathri Vivekanandhan, Mahtab Mehrabbeik, Karthikeyan Rajagopal, Sajad Jafari, Stephen G. Lomber, Yaser Merrikhi . Higuchi fractal dimension is a unique indicator of working memory content represented in spiking activity of visual neurons in extrastriate cortex. Mathematical Biosciences and Engineering, 2023, 20(2): 3749-3767. doi: 10.3934/mbe.2023176 |
[9] | Changwei Gong, Bing Xue, Changhong Jing, Chun-Hui He, Guo-Cheng Wu, Baiying Lei, Shuqiang Wang . Time-sequential graph adversarial learning for brain modularity community detection. Mathematical Biosciences and Engineering, 2022, 19(12): 13276-13293. doi: 10.3934/mbe.2022621 |
[10] | Song Xu, Xufeng Yao, Liting Han, Yuting Lv, Xixi Bu, Gan Huang, Yifeng Fan, Tonggang Yu, Gang Huang . Brain network analyses of diffusion tensor imaging for brain aging. Mathematical Biosciences and Engineering, 2021, 18(5): 6066-6078. doi: 10.3934/mbe.2021303 |
Brain network can be well used in emotion analysis to analyze the brain state of subjects. A novel dynamic brain network in arousal is proposed to analyze brain states and emotion with Electroencephalography (EEG) signals.
Time factors is integrated to construct a dynamic brain network under high and low arousal conditions. The transfer entropy is adopted in the dynamic brain network. In order to ensure the authenticity of dynamics and connections, surrogate data are used for testing and analysis. Channel norm information features are proposed to optimize the data and evaluate the level of activity of the brain.
The frontal lobe, temporal lobe, and parietal lobe provide the most information about emotion arousal. The corresponding stimulation state is not maintained at all times. The number of active brain networks under high arousal conditions is generally higher than those under low arousal conditions. More consecutive networks show high activity under high arousal conditions among these active brain networks. The results of the significance analysis of the features indicates that there is a significant difference between high and low arousal.
Compared with traditional methods, the method proposed in this paper can analyze the changes of subjects' brain state over time in more detail. The proposed features can be used to quantify the brain network for accurate analysis.
The proposed dynamic brain network bridges the research gaps in lacking time resolution and arousal conditions in emotion analysis. We can clearly get the dynamic changes of the overall and local details of the brain under high and low arousal conditions. Furthermore, the active segments and brain regions of the subjects were quantified and evaluated by channel norm information.This method can be used to realize the feature extraction and dynamic analysis of the arousal dimension of emotional EEG, further explore the emotional dimension model, and also play an auxiliary role in emotional analysis.
In recent years, emotion analysis has gained great traction in many interdisciplinary research areas. It can explore different changes in the brain when emotions alter, and help to understand the emotion mechanism. Emotion analysis based on EEG signals studies the brain function information processing mechanism of different human emotions, and the signal analysis results allow people to better understand the human brain information processing and perceptual feedback mechanisms. A well-structured emotion analysis can increase the accuracy of emotion recognition in research areas, like artificial intelligence, healthcare, therefore, greatly improving the efficiency of the applications. For instance, in the field of artificial intelligence, machines can better identify, explain and simulate human emotions with emotion analysis, to establish a better human-computer interaction [1]. Furthermore, emotion analysis can be used to diagnose and analyze the pathology of emotion-related diseases such as depression and autism [2], or assist in treating people's mental illness.
Electroencephalography (EEG) [3] is one of the widely used neuroimaging techniques in the research of brain information processing mechanisms [4]. It has the advantage of having no harm to humans and with a high temporal resolution. In emotion analysis, EEG can capture the brain signals that express and reflect human emotions.
In emotion analysis, emotion dimension theory is used in most research to describe the emotion space, consisting of valence, arousal, and other dimensions in modern emotion dimension models. A valence-arousal model [5]—the circumplex model, as shown in Figure 1. The circumplex model of emotion classification proposed by Russell has always been a research focus. The two-dimensional emotion model divides emotion into two dimensions, namely Valence and Arousal. These two dimensions are a unity of continuous numerical changes. Various emotional states can be characterized as a linear combination of these two dimensions. The valence spans from unpleasant to pleasant of emotions, and the arousal indicates emotions from passiveness to activeness. Although the model of emotion is not unique, in many models, valence and arousal are the most important components.
In current emotion analysis studies, researchers tend to focus on the valence of emotions but neglect the arousal of emotions, for the valence of emotions is easy to identify and quantify. However, emotion analysis is a series of extensive information processing, including analyses of emotion valence and arousal [6]. Deep processing of emotions arousal can improve the accuracy of emotion recognition. For instance, Andreas et al. [7] used experiments with the emotion arousal of subjects for emotion recognition with an accuracy of 89.7%. This showed that the role of emotion arousal is indispensable. Keil et al. [8] found that emotion arousing enhanced emotional processing in the higher gamma-band activity (46–65 Hz) on EEG at 500ms post-stimulus. In addition, the results of [9,10,11]verified the role of gamma band in emotional processing. And the results in [12,13] show that gamma has also achieved a better effect in the emotion classification task. Therefore, it is meaningful to study the arousal dimension of emotions in gamma band to enhance emotion recognition and emotional processing. This paper will mainly be carried out in the arousal dimension to explore the dynamic changes of brain states under different effects of arousal.
Furthermore, time is another influential factor for emotional analysis since the active areas of the brain responsible for emotional processing change over time. The brain constantly changes over time, and its connections will vary over time in different processing tasks. Human brain function networks on multiple time scales ranging from milliseconds to seconds have such dynamic changes. Research has also found that in the whole brain network, not only the nodes and the nodes themselves are dynamic on different time scales[14].
For arousal, the time component also has an indispensable relevance. Balsam et al. [15] examined the influence of emotional arousal and valence on estimating time intervals. The overestimation of the time interval of emotional arousal during encoding and reproduction indicates that emotional stimuli affect temporal information processing in qualitatively different ways at different stages of temporal information processing. Gil et al. [16] systematically examine the arousal effect on time perception when considering different discrete emotions and suggest that arousal is the basic mechanism that regulates the influence of emotion on time perception.To make a complete assessment of emotional processing, processes in different time periods should be considered.
In this work, the role of arousal in emotion analysis is investigated by incorporating time conditions. Specifically, we represent emotions with different brain states and use brain functional networks to express the state of the brain in different periods of time. The essence of constructing a brain functional network is to analyze the complex brain by defining nodes and edges based on graph theory. Channels in EEG signals from the brain are generally chosen as nodes, while edges refer to the connections between nodes. We refer to brain functional network as brain network for short.
Brain network are one of the major collaborative working models to describe the relationships between different brain areas (macroscopically) [17]. For instance, Gonuguntla et al. [18] used EEG phase synchronization (PLV) connectivity analysis on 60 seconds of the DEAP dataset to identify emotion-related functional connectivity patterns and their different associated regions. Bartolomei et al. [19] used a nonlinear measure of SEEG signal interdependencies at different time periods for different patients. So the interactions between regions were analyzed to form 'emotional networks'. Hou et al. [20] extracted a 2.5 seconds segment in the stimulus experiment. Then the phase lag index (PLI) between EEG channels was computed and an undirected network was constructed based on the PLI matrix.
However, as a method to analyze the brain states over a period of time, the traditional way of constructing a brain network is not enough to systematically study the effect of arousal during the whole stimulation process in detail [21]. Emotional processing is a series of broader information processing processes beyond emotional valence, including the deep processing of arousal [6,22]. Especially in terms of improving spatial and temporal memory [23], the increase in arousal can deepen memory more significantly, while the relative valence is not deeply affected. Although some studies have shown the importance of arousal, the effect of arousal under different conditions on the processing of emotional shadows is still unclear, especially for the dynamic changes of the brain state under arousal conditions over time. For example, in the high and low levels of arousal, the brain state changes over time, the activity of each brain area, the difference between subjects, and so on. In order to bridges the research gaps in lacking time resolution and arousal conditions in emotion analysis, a detailed display of the state of the brain over time under differrnt arousal conditon is necessary.
Compared with the traditional brain network, it is an idea to build a well-structured dynamic brain network under the condition of arousal and combining the time factor. A new evaluation method should be considered to quantify the results of the brain network state. Therefore, a novel dynamic brain network in arousal is proposed in this paper. The main technical challenges can be summarized. First, the time dynamics of the brain network need be expressed. Second, it is necessary to ensure the true effectiveness of the established brain state. Third, the complex dynamic brain network structure should be optimized for better evaluation. To address these challenges, constructing a dynamic brain network with a certain time resolution should be adopted to guarantee reliable emotion analysis. We have mainly done the following four tasks:
(1) Signal segmentation under different arousal levels with sliding time windows is applied to build dynamic brain network;
(2) Surrogate data was to generated to ensure the reliability of the connection;
(3) Features called channel norm information are proposed for better dynamic analysis and network structure optimization;
(4) Based on the above quantitative features, the experimental states of some subjects were compared. The differences in brain activity under different arousal conditions and the dynamic changes of the brain were obtained.
Through dynamic brain networks, dynamic changes and differences of the brain under different arousal conditions could be observed to analyze the role of arousal in emotional processing. According to the results of the analysis, we get the segments that we are interested in for more accurate emotional analysis.
The construction and analysis of a dynamic brain network in this paper are summarized in Figure 2. A dynamic brain network consists of several brain networks, each obtained from the gamma band of the EEG signal segment.
In this paper, each sample is a multi-channel EEG signal of an emotional stimulation experiment by a single subject. It is dynamically divided into several EEG signal segments. In previous studies, the activity in the gamma band is proved significant [24,25]. So the gamma band of each EEG signal segment is extracted and is used to construct the brain network.
To establish a brain network, the relationship between two channels is evaluated by transfer entropy, and a correlation matrix is formed and then abstracted as a brain network. Each brain network is connected in chronological order to form a dynamic brain network for dynamic verification and analysis.
Dynamic brain networks are obtained from different subjects during the experimental stimulation process under arousal conditions. Next, a number of surrogate data are generated for each connection and statistical tests are carried out to ensure the reliability of the connection to the maximum extent.
In addition, the features called channel norm information are proposed to reflect the amount of information transfer in the electrode. It could optimize the complex structure of the brain networks and be used as features to observe the dynamics of the network and the activity of the channels.
In summary, the construction and analysis of a dynamic brain network consist of four main steps:
(1) Dynamic segmentation: 3s sliding time windows are used to divide the EEG signals for modeling brain states at different periods;
(2) Transfer entropy was calculated and tested to form brain networks: Transfer entropy is used to calculate the connections between nodes. The corresponding surrogate data are generated to test transfer entropy;
(3) The dynamic analysis of brain network: The features of channel norm information are proposed to optimize the network structure by reducing the dimension.
The establishment of a dynamic brain network starts from dynamic signal segmentation. In dynamic brain networks, multi-channel EEG signals in each sample are segmented with a 3-second non-overlapping sliding time window as a segment to construct the brain network. The time window of 3-second is to ensure that the dynamic characteristics of the brain are captured under a certain amount of calculation and consumption, and this can be compared with the previous 3-second baseline state of the experiment. After segmentation, to better reflect the intensity of brain activity, the gamma band content of each segment is extracted. The resulted signals are used for subsequent transfer entropy calculation and network analysis. The brain network established in each window is a component of the dynamic brain network.
If a brain network changes dynamically during stimulation [21] (high arousal and low arousal stimulation), a network divided by non-overlapping sliding time windows can represent one of the dynamic processes. After establishing the dynamic brain network, we will use K-means clustering to verify the dynamics.
After dynamic signal segmentation, the brain network on each EEG signal segment is established. The EEG channel is selected as the node, and the transfer entropy is used to calculate the relationship between the nodes to get the edge.
Transfer entropy (TE) [26] is based on information theory. It measures how much information transfer between two processes by observing the transfer probability from one process to another [27]. Transfer entropy is a model-free realization of the Wiener principle, which is independent of the established models and therefore used for nonlinear quantitative analysis.
The estimation of transfer entropy is bi-directional and asymmetric. For instance, there are source process X and target process Y, and their corresponding time series are X={x1,x2,……xn} and Y={y1,y2,……,yn}, where n is the length of the time series. xi is the state variable of the i-th sampling point and yj is the same. The transfer entropy from the source process X to the target process Y can be described as:
TEX→Y=H(yt+u|yt)−H(yt+u|xt,yt) |
=∑xt+u,yt,ytp(yt+u,xt,yt)log2(p(yt+u|xt,yt)p(yt+u|yt)) | (1) |
where t is the discrete time index, u is the prediction time. p(·) is the joint probability and conditional probability between variables. H(yt+u|yt) is the conditional entropy of process Y, which depends on its past value. TEx→Y is the transfer entropy value from the source process X to the target process Y. Similarly, the estimated transfer entropy from Y to X can be obtained.
TEY→X=∑yt+u,xt,xtp(xt+u,xt,yt)log2(p(xt+u|yt,xt)p(xt+u|xt)) | (2) |
For specific calculations, we need to first calculate the joint probability and conditional probability of the internal state variables of the corresponding variables, and then calculate according to the formula to obtain the transfer entropy between the variables. If the number of channels is N, after calculating the transfer entropy per two channels, the transfer entropy matrix of N*N could be obtained. The values on the diagonal matrix of the transfer entropy matrix are 0.
In the transfer entropy matrix of each segment, each element TEij represents the information transfer value from i-th channel to j-th channel, and its corresponding row vector is the information transfer value from i-th channel to all channels. Similarly, the column vector represents the information transfer from all other channels to the i-th channel.
Surrogate data are generated for each estimate of TE to preserve a more efficient connection. They are time series, which are as close as possible to the original data but have no information transfer. They are used to detect and reject false connections between two completely unrelated time series of finite data in the estimation bias (i.e., a non-zero TE value occurs even with no information transfer). The expected TE under limited data should be estimated by surrogate data [28].
Corresponding surrogate data for each estimation are generated, as shown in Figure 3. The surrogate data generation method is to randomly assign a cut-in point in the source process X and exchange the two generated data segments. This method breaks the temporal precedence structure between the source and the target processes while having the same finite length and the same autocorrelation properties.
For each estimation of TE, 100 surrogate data are generated, and then the TE values of each surrogate data and the target process sequence are calculated. The original TE value is compared with the TE value distribution from the surrogate data by the Permutation test. The Permutation test is a nonparametric significance test [29] with the null hypothesis that two data samples come from the same population.
If the result from the Permutation test shows that the original TE value is quite different from the TE distribution generated by the surrogate data, the original TE value is retained and considered as an edge of the brain network nodes. Otherwise, the original TE value is set to 0, indicating no edges between nodes.
It can be expected that the structure of dynamic brain network is very complex, so effective and targeted analysis methods should be adopted. The information features of each channel are defined by using the TE matrix to observe the dynamic change process of the brain networks under the condition of different arousal degree.
The information transfer values in the TE matrix are mapped to scalars by calculating the Ln norm represented by row vectors and column vectors corresponding to each channel. In this paper, this feature is referred to as channel norm information. This feature not only realizes the quantification of information transfer but also reduces data dimension.
A norm is a function that assigns length or size to each vector in a vector space (or matrix). To some extent, this method also quantifies the sparsity of information transfer. The more connections and the higher the density, the greater its value. L2 norm is commonly used, which measures the length of the vector and can optimize the numerical calculation. It is expressed as
F(k)input/output=2√∑nm=1(TE(k)m)² | (7) |
Here these F(k)input/output are channel norm information. It is divided into input and output. It represents the information features of the k-th channel. TE(k)m represented the transfer entropy of the m-th channel and the k-th channel. The value of channel norm information is used to evaluate the activity of brain networks and channels.
For this study, the proposed dynamic brain network construction is shown in Figure 4. A sliding time window is used to obtain each segment of the stimulation process, and the gamma band information is mainly extracted. The directed brain functional connections of each segment are established through transfer entropy (TE) for the brain network, and '0' TE is excluded through corresponding surrogate data. Consequently, the brain networks are connected according to their time order to form a dynamic brain network.
To verify and evaluate the validity of the brain network in the sliding time windows, The L2 norm method is proposed to define the input and output information features of channels for each established transfer entropy information matrix. It could help to reduce the dimension and show a positive change process dynamically.
In this paper, the public dataset in the Database for Emotion Analysis using Physiological Signals (DEAP) [30] is utilized. The DEAP dataset is a multimodal affective EEG dataset for emotion analysis. This DEAP dataset contains experimental data on physiological signals of 15 women (average 25.4 years old) and 17 men (average 28.8 years old) during watching 40 music videos with different emotional markers. EEG signals are recorded at a sampling rate of 512 Hz from a 32-channel electrode cap according to the international 10–20 system, as shown in Figure 5. The electrode name represents the area and position where the electrode is located, FP stands for frontal pole, F for frontal, T for temporal, C for central, O for occipita, and P for parietal. The data is first downsampled to 128Hz. A bandpass frequency filter from 4–45Hz is applied. Experiments mainly include the following steps:
(1) The serial number of the experiment progression. In the screen, it will last for 2 seconds to inform the participant's current video order;
(2) Baseline recording. A cross prompt symbol appears on the screen for 5 seconds. At this time, the participants should keep calm, record the mark started;
(3) Music video playback. This process lasts for 63 seconds, the first 3 seconds is the time for each video conversion, and the last 60 seconds is the time of music video truly play. Participants need to keep their body balance and reduce movement;
(4) Self-assessment points. After watching a video, participants fill in the Self-assesSmentmanikins(SAM). This process will last for nearly 15 seconds. Participants need to evaluate the real emotional experience after reading music videos;
(5) Repeat steps (3) ~ (4) until 40 music video materials are all played.
Each participant needs 40 experiments and to perform a timely self-assessment t on the SAM questionnaire.
To construct a dynamic brain network, the TE between two channels is calculated in each 3s gamma band of EEG signals. After these values are tested with surrogate data, the TE matrix of this segment is obtained.
According to the TE matrix of each segment, the corresponding brain network topology could be constructed. In the time length of 0–60s, a total of 20 brain networks are obtained in each experiment. This means that the dynamic brain network of a sample contains 20 brain networks. For these 20 brain networks, use the numbers from 1 to 20 as their label in chronological order. An experimental result under high arousal condition is shown in Figure 7.
Compared with the method of threshold selection (i.e., to select a threshold to keep the edges with strong connections) based on sparsity, more effective connections are obtained by using surrogate data to test in the high arousal dynamic brain network. Besides, the activities of some channels and brain regions could be simply observed from the distribution of color blocks. For instance, segments 4, 8, 16 with many red nodes, marked by red boxes, have more connections among channels. Some consecutive segments (like 12, 13, 14, 15) with most blue and green nodes have fewer connections among channels.
It can be seen that even under the condition of high arousal, the brain would not be in an active state of information transfer all the time during the whole experiment.
By comparing with the brain topology of the experimental stimulation, the obvious changes are observed in the subjects' brain network in the resting state. Figure 8 shows the brain network before starting the first 3s stimulation (resting state). Significant activation occurred in the central area and temporal lobe under high arousal stimulation.
In these brain network topology under low arousal in Figure 9, more blue nodes and fewer red nodes than the phenomenon under high arousal conditions are shown in Figure 7. This indicates the degree of information transfer is not as high as that under high arousal conditions. In this way, compared with low arousal, high arousal is more likely to produce brain networks with more connections and more efficient information transfer. But the central part is still a relatively active area under low arousal in Figure 9.
From the results of Figure 7 and Figure 9, the nodes with the largest number of connections are observed near the center under both conditions (high and low arousal). The darker node indicates that it has more connections, indicating that it has more information exchange. The prefrontal lobe function related to cognition does not show strong function at the beginning of the experiment. And at the two moments at the beginning and end of the experiment under the two conditions, more light-colored nodes are presented, indicating the potential state changes of the subject during the experiment and the different activation of the brain.
Although the brain network topology gives an intuitive observation, the quantization effect is not enough. Therefore, active brain networks and active channels are analyzed in Sections 3.3 and 3.5.
To further explore the changes of each brain network in a dynamic brain network, the input and output channel norm information on 32 channels of each brain network is obtained after the TE matrix is calculated. The mean of channel norm information in 32 channels is regarded as the information feature of the network to indicate the degree of information transfer of the brain network. Different from the intuitive display of the brain network topology, the features are applied to quantify numerically to reflect the differences.
As shown in Figure 10, the degree of information processing in the brain varies over time in the stimulation experiment. It can be seen from the figure that the brain states at different moments have different special values. And similar to the results observed by the topological map, the brain state of the subjects showed a lower active state at the beginning and end of the experiment. For both high arousal and low arousal, the changes in Figure10 show a smaller value of information feature at the beginning of the experiment, which rises and fluctuates as the stimulation continues. In the latter part of the stimulation, the information feature value decreased. Even with the same stimulation, there are a variety of changes. Our information feature greatly reduces the data dimension from the original 32×32 dimensions to 32×1 dimensions compared with the brain network topology. It makes the results easier to analyze and observe the following active brain networks and channels.
To make a complete assessment of emotional processing in the brain, the time process of activation should be considered. Through this information feature, the active brain networks could be analyzed. Thus, each of the 20 networks is processed separately, and its TE matrix is analyzed. For each TE matrix, the information feature of each channel is calculated by the method in Section 2.5, and its mean value is taken as the evaluation of the brain network. In this way, we would get a total evaluation value of 20 brain networks. If the brain network has an evaluation value larger than its average value, it is considered an active network; otherwise, it is an inactive one. All results of the active networks are shown in Table 1. Here we continue to use the labels given to each brain network in Section 3.2.
Subject | Video | Arousal | Labels of active networks | Number of active networks |
S1 | 18 | high | 3 5 7 9 10 12 14 15 16 | 9 |
24 | high | 2 4 5 6 7 8 10 12 14 16 19 20 | 12 | |
7 | low | 4 5 7 8 9 11 12 13 14 15 | 10 | |
22 | low | 2 5 6 7 8 9 12 15 16 | 9 | |
S5 | 2 | high | 1 3 6 9 10 11 12 13 14 16 17 19 | 12 |
6 | high | 1 2 4 5 6 9 10 13 16 18 19 20 | 12 | |
26 | low | 1 2 7 8 9 12 14 18 19 20 | 10 | |
27 | low | 6 8 9 10 13 14 17 19 20 | 9 | |
S6 | 5 | high | 1 2 4 5 6 8 10 11 12 16 18 20 | 12 |
7 | high | 2 3 4 5 6 7 8 9 10 14 | 10 | |
8 | low | 4 6 7 8 9 11 13 15 16 17 19 | 11 | |
12 | low | 1 7 10 12 13 17 19 20 | 8 | |
S7 | 7 | high | 2 3 4 8 10 11 13 14 15 17 18 19 | 12 |
10 | high | 1 2 4 5 6 10 13 15 18 19 20 | 11 | |
17 | low | 1 4 6 10 12 13 16 18 20 | 9 | |
29 | low | 4 7 8 9 10 12 14 16 17 20 | 10 |
From the result of Table 1, it could be observed that there are more active brain networks under high arousal conditions than under low arousal conditions, indicating that subjects have more brain activities under high arousal conditions. Among these active brain networks, more consecutive networks show high activities under the condition of high arousal.
Furthermore, the features are statistically analyzed under high and low arousal. Non-parametric test was applied on the eigenvalue arrangement (Figure 10) of each subject under the two conditions of high and low arousal to determine whether the features of high and low arousal were significantly different. As can be seen from the results in Table 2, there is a significant difference between high and low arousal(P < 0.05). In addition, we counted the number of active brain networks of each subject based on Table 1, and the results were listed in Table 2. The number of all subjects under different arousal conditions was counted to form a test with different arousal conditions. The significance test results showed that P = 0.0091 < 0.01, and the results showed significant differences.
Subject | Video | Arousal | Number of active networks | P |
S1 | 18 | high | 9 | 0.021 |
24 | high | 12 | ||
7 | low | 10 | ||
22 | low | 9 | ||
S5 | 2 | high | 12 | 0.045 |
6 | high | 12 | ||
26 | low | 10 | ||
27 | low | 9 | ||
S6 | 5 | high | 12 | 0.037 |
7 | high | 10 | ||
8 | low | 11 | ||
12 | low | 8 | ||
S7 | 7 | high | 12 | 0.041 |
10 | high | 11 | ||
17 | low | 9 | ||
29 | low | 10 |
After observing the dynamics of the dynamic brain network, the specific changes of the relevant brain regions and channels are further analyzed in the study. We focus on these highly active networks and the activities of their specific channels.The mean values of channel norm information of input and output are used to evaluate the importance of the channel. The higher the mean value, the more information is involved in the process, the more active and important the channel is. After normalization, we use 0.6 as the threshold of the mean values of channel norm information. The active channel is defined with a mean value of channel norm information larger than the threshold.
For example, in the high arousal experiment of subject S1, from the results in Table 1, its active segments are 3, 5, 7, 9, 10, 12, 14, 15, 16, a total of 9 segments. They are renumbered as network 1 to network 9.
After sorting out the active distribution of the nine-segment high-state brain network channels, to make the difference more obvious, normalization is applied in Figure 11.
Finally, the active channels selected are F4, FC2, FC5, T7, C3, Cz, T8, CP6, CP2, P7, PZ, P8, PO3. Combining the experiments of all the subjects, it reflected the active situation of different numbers of channels in different subjects. The result of active channels of all subjects that we chose is shown in Table 3. The results in the table show the active channels of different subjects. It can be seen that different subjects have different numbers of active channels. For example, subject S1 has more than 6 active channels under high arousal conditions, but subject S6 has 3. Moreover, FC and CP channels are frequently found between subjects, which indicates the activity of the frontal and parietal regions. A more detailed analysis of the results will be carried out in the discussion section.
Subject | Video (high arousal) | Active channels | Video (low arousal) | Active channels |
S1 | 18 | F4, FC2, FC5, T7, C3, Cz, T8, CP6, CP2, P7, PZ, P8, PO3 | 7 | FC2, FC1, FC5, T7, CZ, T8, CP6, CP1, P8 |
24 | F7, FC1, FC5, T7, CZ, CP2 | 22 | F4, T7, CZ, CP6, CP2, CP1 | |
S5 | 2 | AF3, CZ, C4, CP1, O1, OZ | 26 | AF3, AF4, CP1, O1 |
6 | AF3, C4, T8, CP1, O1, OZ. | 27 | AF3, C4, CP1, O1 | |
S6 | 5 | Fz, T7, P8 | 8 | Fz, T7, P8 |
7 | Fz, T7, P8 | 12 | Fz, T7, P7, P8 | |
S7 | 7 | AF3, F7, FC6, FC5, CP1, O1 | 17 | AF3, F7, FC6, FC2, FC5, CP1 |
10 | AF3, F7, FC6, FC2, FC5, CP2, CP1, O1 | 29 | F7, F3, FC6, FC5, T8, CP1 |
In this study, the dynamic brain network of each subject is constructed, which shows the brain states in different time periods during the whole experimental stimulation process. The dynamics of consecutive brain networks are verified by channel norm information. It is found that high active states are not always maintained even in high arousal conditions. However, compared with low arousal, subjects have more consecutive active networks under high arousal conditions. The results of statistical analysis of the features show that there are significant differences. And in these active networks, the frontal lobe, temporal lobe, and parietal lobe are highly active regions.
The differences between different brain regions could be observed intuitively from the brain network topology of high arousal and low arousal conditions, as in Figure7 and Figure9. Compared with low arousal, there are more connections (more red nodes) in the occipital lobe in the brain network with high arousal. It indicates that the information transfer in this region is more intensive.
In the visual perception experiment, Sutherland et al. [32] indicated that the degree of arousal is the key factor in enhancing the visual perception of significant targets. The experiment showed that sounds rated as more emotional arousal would result in more recognition of highly significant letters, and the sound's valence rating did not affect the significance bias. Furthermore, combined with the results in Section 3.2, the channel color of the occipital region in the early stage of the experiment is mostly dark, showing that functional areas such as vision have a better activation effect under high arousal. In other words, the high arousal stimulation in the experiment triggered the enhancement of occipital lobe function.
In addition, a similar phenomenon can be found in the prefrontal lobe between high and low arousal. Dolcos et al. [33] investigated the activity of the prefrontal cortex (PFC) related to emotional assessment and subsequent memory through event-related functional MRI (fMRI). The results have shown that the dorsal area of the prefrontal cortex is sensitive to arousal. Nielen et al. [34] studied the brain mechanism of arousal by FMRI data and found that high arousal images significantly activated brain regions in the middle temporal region, hippocampus, and ventral prefrontal cortex. Therefore, the prefrontal lobe is more significantly activated under the condition of high arousal.
However, it is noted that although the occipital lobe function of the brain under high arousal is slightly stronger than that under low arousal, the occipital lobe is not activated as significantly as other regions, such as the parietal lobe, for all brain regions.
In these dynamic brain networks, these active brain networks are of interest because the highly active states are not always maintained. The active networks are calculated through the channel norm information. Different active brain networks occupy different time segments, and they constitute active time segments under a sample. According to the results of the significance analysis of features, the distribution of features of high and low arousal has significant difference. Indicates a tendency for different brain states under different arousal conditions.
The number of active brain networks (Section 3.3) proves that the number of active brain networks under high arousal is larger than that under low arousal. This means the subjects have more brain activities under the stimulation of the high arousal experiment. Many active brain networks are consecutive under high arousal. Leite et al. [35] used pictures with shock stimuli in the ERP experiment; the subjects' response to high stimuli might indicate an increase in motivational attention. According to their experimental results, the amplitude of pleasant high arousal pictures (M = 6.051, SE = 0.868) is greater than that of pleasant low arousal pictures (M = 2.533, SE = 0.598). We also found in our results that, for example, S5 subjects got 12 active brain networks under high arousal conditions. Therefore, the increase in arousal might increase the subjects' attention. BRADLEY and LANG [36] found in the study of high attention and low attention motivational stimuli that different levels of arousal represent different levels of motivation. For example, high attention stimuli generally induce high levels of arousal and arouse strong arousal. Therefore, the stimulation caused by some irritating pictures and videos is related to the high arousal state.
In the active brain networks, we further study the active channels. Even under the same conditions (high arousal or low arousal), some subjects have more active channels than others due to individual differences. For example, for subject S1, there are more active channels. But for subject S6, even if the threshold for determining active channels is lower, the number of active channels is still small.
In general, under high arousal conditions, the frontal lobe, temporal lobe, and parietal lobe are highly active regions. Aydın [37] used the wavelet correlation (WC) method is used to estimate the non-linear inter-hemispheric synchrony level of emotional dysfunction. In its classification experiment, gamma has the highest classification accuracy rate, the highest is 88.06%. Their results show the fronto-central lobes are the most activated brain regions during emotional stimu-lation. Similarly, a large number of channels in fronto-central also appeared in our results like FC2, FC5 (in Table 3). Zheng [38] applied machine learning methods to study the stable EEG patterns of emotion recognition over time and found that the lateral temporal area activates more positive emotions than negative emotions. In our experimental results, except for the temporal lobe, the channels related to the parietal lobe also occupy a large proportion, and their importance cannot be ignored.
According to the active channel results (Section 3.5), the active brain regions tend to be in the left hemisphere in both high arousal (25 active channels in the left, 15 in the right) and low arousal (24 active channels in the left, 15 in the right). The value specificity theory holds a different division of labor in processing emotion information with different values in the brain's left and right hemispheres. The right hemisphere is mainly responsible for processing unpleasant emotions, while the left hemisphere is mainly responsible for processing pleasant emotions [39]. According to this theory, the result of active brain regions in the hemisphere corresponds to the pleasant emotion sample we choose.
Through the results of channels and networks, it can also be found that in the whole brain network, the functional connection between nodes dynamic at different time scales. During the experiment, the brain showed dynamic changes in various task states. This dynamic brain network has also been widely used in the fields of normal people's cognition [14], the common cognitive model of working memory [40], and emotional perception [41].
Among the studies that use the DEAP dataset for emotion recognition and classification, many select the best data segments based on trials [2]. There is no guideline to select the date segments. Some choose the whole 60-second data; the others intercept partial data at the beginning, the end, or even in the middle. For instance, Zhang et al. [42] removed the first 33 seconds and the last 21 seconds of each EEG signal and select 9-second signals (from 34 s to 42 s) for feature extraction and performance evaluation.
In this paper, after dividing the 60s data into 3s segments, some segments that are in a high active state in stimulation are obtained. High active brain network may be more suitable for representing brain states, so it is more conducive to classification and recognition. We can choose the appropriate segments to analyze according to the needs. On the whole, our approach provides some insights on data selection.
In the stimulation of some subjects (both high and low arousal), the brain network activity is low in the last few seconds, which may indicate that the subjects gradually enter the inactive state, as shown in Figure12. Also, in some subjects, brain activity in the latter part of the stimulation experiment is not as active as before, to some extent, indicating a possible state of tiredness. It is reasonable for some authors to give up the latter part of the data because of subjects' fatigue.
In this paper, dynamic brain networks are constructed by calculating the transfer entropy from EEG signal segments and applying channel norm information for emotion analysis on the arousal dimension. It adds both time scope and arousal dimension in emotion research. Therefore, the dynamics of the dynamic brain networks under emotional stimulation show the changes in brain states. In addition, the transfer entropy test ensure the reliability of connection. The features and significance analysis based on the transfer entropy matrix also show the differences of different arousal conditions. This method complements the role of arousal in emotional processing, and observes the dynamic changes of the whole and local details of the brain.
The dynamic changes of brain networks for different subjects under high and low arousal conditions over time are observed. In the whole process of stimulation, the corresponding stimulation state is not maintained at all times. The number of active brain networks under high arousal conditions is generally higher than those under low arousal conditions. More consecutive networks show high activity under high arousal conditions among these active brain networks. We evaluated and analyzed the channels in highly active brain regions of the subject's areas in more detail, so as to understand the whole emotional processing process. In general, this method can quantify and dynamically analyze the brain state of the subjects to get the fragments we want. In addition, it further explores the emotional dimension model to provide reference for emotional analysis.
In order to explore the changes of the brain state over time under different arousal conditions, this paper uses dynamic brain networks to analyze the state changes of some subjects' brains in the emotional arousal dimension. On the one hand, it describes the details of the subject's status in more detail. This provides some explanations for the processing of the emotional arousal dimension. On the other hand, the features information quantifies the state of the brain, which makes the changes in the state of brain more intuitive.Although it provides more detailed status information, it inevitably increases the difficulty and cost of analysis. And this article provides some preliminary observation methods under the set conditions, and there is still some work to be further studied for its related research and analysis. In the future, we will work on emotion classification and recognition built on the current results to verify further the valid time period of emotion classification and recognition.
This work was supported by the National Nature Science Foundation of China (Grant No.61971168, 61871427), the Open Research Projects of Zhejiang Lab (No. 2021MC0AB04).
[1] | B. Farahi, Emotional intelligence: Affective computing in architecture and design, Architectural Intelligence: Selected Papers from the 1st International Conference on Computational Design and Robotic Fabrication (CDRF 2019), 2020,235-251. |
[2] |
Z. H. Zeng, M. Pantic, G. I. Roisman, T. S. Huang, A survey of affect recognition methods: Audio, visual, and spontaneous expressions, IEEE Trans. Pattern Anal. Mach. Intell., 31 (2009), 39-58. doi: 10.1109/TPAMI.2008.52
![]() |
[3] | J. Zhang, Y. Zhou, Y. Liu, EEG-based emotion recognition using an improved radial basis function neural network, J. Amb. Intell. Human. Comput., (2020). |
[4] |
A. Momennezhad, EEG-based emotion recognition utilizing wavelet coefficients, Mult. Tools Appl., 77 (2018), 27089-27106. doi: 10.1007/s11042-018-5906-8
![]() |
[5] | J. Posner, J. Russell, B. Peterson, The circumplex model of affect: An integrative approach to affective neuroscience, cognitive development, and psychopathology, Development Psychopathol., 17 (2005), 715-734. |
[6] |
F. Citron, Neural correlates of written emotion word processing: A review of recent electrophysiological and hemodynamic neuroimaging studies, Brain Language, 122 (2012), 211-226. doi: 10.1016/j.bandl.2011.12.007
![]() |
[7] | A. Haag, S. Goronzy, P. Schaich, J. Williams, Emotion recognition using bio-sensors: First steps towards an automatic system, Affective Dialogue Systems, Springer Berlin Heidelberg, Berlin, Heidelberg, 2004, 36-48. |
[8] |
A. Keil, M. M. Müller, T. Gruber, C. Wienbruch, M. Stolarova, T. Elbert, Effects of emotional arousal in the cerebral hemispheres: A study of oscillatory brain activity and event, Clin. Neurophysiol., 112 (2001), 2057-2068. doi: 10.1016/S1388-2457(01)00654-X
![]() |
[9] |
J. H. Kang, H. M. Ahn, J. W. Jeong, I. Hwang, H. T. Kim, S. H. Kim, et al., The modulation of parietal gamma oscillations in the human electroencephalogram with cognitive reappraisal, Neuroreport, 23 (2012), 995. doi: 10.1097/WNR.0b013e32835a6475
![]() |
[10] | Y. Tang, Y. Li, J. Wang, S. Tong, Y. Jing, Induced gamma activity in eeg represents cognitive control during detecting emotional expressions, 33rd Annual International Conference of the IEEE Engineering-in-Medicine-and-Biology-Society (EMBS), (2011). |
[11] |
D. J. Oathes, W. J. Ray, A. S.Yamasaki, T. D. Borkovec, L. G. Castonguay, M. G. Newman, et al., Worry, generalized anxiety disorder, and emotion: Evidence from the EEG gamma band, Biol. Psychol., 79 (2008), 165-170. doi: 10.1016/j.biopsycho.2008.04.005
![]() |
[12] |
Y. X. Yang, Z. K. Gao, X. Wang, Y. L. Li, J. W. Han, A recurrence quantification analysis-based channel-frequency convolutional neural network for emotion recognition from EEG, Chaos Interdiscipl. J. Nonlinear Sci., 28 (2018), 085724. doi: 10.1063/1.5023857
![]() |
[13] | M. Li, B. L. L. S. Member, Emotion classification based on gamma-band EEG, 2009 Annual International Conference of the IEEE Engineering in Medicine and Biology Society, 2009. |
[14] |
V. Calhoun, R. Miller, G. Pearlson, T. J. N. Adal, The chronnectome: Time-varying connectivity networks as the next frontier in fMRI data discovery, Neuron, 84 (2014), 262-274. doi: 10.1016/j.neuron.2014.10.015
![]() |
[15] |
P. Balsam, H. V. J. T. Volkinburg, T. Perception, Effects of emotional valence and arousal on time perception, Tim. Time Percept., 2 (2014), 360. doi: 10.1163/22134468-00002034
![]() |
[16] |
S. G. S. S. Droit-Voletsupb/Sup, S. J. C. Emot, Emotional time distortions: The fundamental role of arousal, Cogn. Emot., 26 (2012), 847-862. doi: 10.1080/02699931.2011.625401
![]() |
[17] |
M. Kaiser, A tutorial in connectome analysis: Topological and spatial features of brain networks, NeuroImage, 57 (2011), 892-907. doi: 10.1016/j.neuroimage.2011.05.025
![]() |
[18] | V. Gonuguntla, R. Mallipeddi, K. C. Veluvolu, Identification of emotion associated brain functional network with phase locking value, Eng. Med. Biol. Society, (2016), 4515-4518. |
[19] |
F. Bartolomei, A. Trébuchon, M. Gavaret, J. Régis, F. Wendling, P. J. C. N. Chauvel, Acute alteration of emotional behaviour in epileptic seizures is related to transient desynchrony in emotion-regulation networks, Clin. Neurophysiol., 116 (2005), 2473-2479. doi: 10.1016/j.clinph.2005.05.013
![]() |
[20] | F. Hou, C. Liu, Z. Yu, X. Xu, J. Zhang, C. K. Peng, et al., Age-related alterations in electroencephalography connectivity and network topology during n-back working memory task, Front. Human Neurosci., 12 (2018), 484. |
[21] |
M. Bola, B. A. Sabel, Dynamic reorganization of brain functional networks during cognition, Neuroimage, 114 (2015), 398-413. doi: 10.1016/j.neuroimage.2015.03.057
![]() |
[22] | A. B. Eder, L. Hartmut, R. Klaus, S. Schweinberger, Automatic response activation in sequential affective priming: an ERP study, Social Cognit. Affect. Neurosci., (2012), 436-445. |
[23] |
K. Schmidt, P. Patnaik, E. A. Kensigner, Emotion's influence on memory for spatial and temporal context, Cognit. Emot., 25 (2011), 229-243. doi: 10.1080/02699931.2010.483123
![]() |
[24] | M. Batashvili, P. A. Staples, I. Baker, D. Sheffield, Exploring the relationship between gamma-band activity and maths anxiety, Cognit. Emot., (2019), 1-11. |
[25] | M. Yan, H. Shihui, M. Gelfand, The role of gamma interbrain synchrony in social coordination when humans face territorial threats, Social Cognit. Affect. Neurosci., (2017), 1614-1623. |
[26] | S. Shao, C. Guo, W. Luk, S. Weston, Accelerating transfer entropy computation, 2014 International Conference on Field-Programmable Technology (FPT), 2014, 60-67. |
[27] |
R. Vicente, M. Wibral, M. Lindner, G. Pipa, Transfer entropy—a model-free measure of effective connectivity for the neurosciences, J. Comput. Neurosci., 30 (2011), 45-67. doi: 10.1007/s10827-010-0262-3
![]() |
[28] | M. Wibral, R. Vicente, M. Lindner, Transfer Entropy in Neuroscience, Understanding Complex Systems, 2014. |
[29] |
E. Maris, R. Oostenveld, Nonparametric statistical testing of EEG- and MEG-data, J. Neurosci. Methods, 164 (2007), 177-190. doi: 10.1016/j.jneumeth.2007.03.024
![]() |
[30] |
S. Koelstra, C. Muhl, M. Soleymani, J. S. Lee, A. Yazdani, T. Ebrahimi, et al., DEAP: A database for emotion analysis using physiological signals, IEEE Transact. Affect. Comput., 3 (2012), 18-31. doi: 10.1109/T-AFFC.2011.15
![]() |
[31] | H. Kuai, H. Xu, J. Yan, Emotion recognition from EEG using rhythm synchronization patterns with joint time-frequency-space correlation, International Conference on Brain Informatics, 2017,159-168. |
[32] | M. R. Sutherland, M. J. C. Mather, Emotion, Arousal (but not valence) amplifies the impact of salience, Cognit. Emotion, (2017). |
[33] |
F. Dolcos, K. S. LaBar, R. Cabeza, Dissociable effects of arousal and valence on prefrontal activity indexing emotional evaluation and subsequent memory: an event-related fMRI study, Neuroimage, 23 (2004), 64-74. doi: 10.1016/j.neuroimage.2004.05.015
![]() |
[34] |
M. Nielen, D. J. Heslenfeld, K. Heinen, J. Strien, M. P. Witter, C. Jonker, et al., Distinct brain systems underlie the processing of valence and arousal of affective pictures, Brain Cogn., 71 (2009), 387-396. doi: 10.1016/j.bandc.2009.05.007
![]() |
[35] | J. Leite, S. Carvalho, S. Galdo-Alvarez, J. Alves, A. Sampaio, Ó. F. Gonçalves, Affective picture modulation: Valence, arousal, attention allocation and motivational significance, Int. J. Psychophysiol., 83 (2012), 375-381. |
[36] | J. T. Cacioppo, L. G. Tassinary, G. G. Berntson, Handbook of Psychophysiology, Cambridge University Press, 2017. |
[37] |
S. Aydın, S. Demirtaş, S. Yetkin, Cortical correlations in wavelet domain for estimation of emotional dysfunctions, Neural Comput. Appl., 30 (2018), 1085-1094. doi: 10.1007/s00521-016-2731-8
![]() |
[38] | W. L. Zheng, J. Y. Zhu, B.L. Lu, Identifying stable patterns over time for emotion recognition from EEG, Affect. Comput. IEEE Transact., 2016. |
[39] |
G. P. Lee, K. J. Meador, D. W. Loring, J. D. Allison, W. S. Brown, L. K. Paul, et al., Neural substrates of emotion as revealed by functional magnetic resonance imaging, Cognit. Behav. Neurol. Offic. J. Society Behav. Cognit. Neurol., 17 (2004), 9. doi: 10.1097/00146965-200403000-00002
![]() |
[40] | J. E. Chen, C. Chang, M. Greicius, G. Glover, Introducing co-activation pattern metrics to quantify spontaneous brain network dynamics, NeuroImage, 111 (2015). |
[41] |
C. Kuhbandner, P. Spachtholz, B. PastÖTter, Bad things come easier to the mind but harder to the body: Evidence from brain oscillations, Cognit. Affect. Behav. Neurosci., 16 (2016), 768-778. doi: 10.3758/s13415-016-0429-0
![]() |
[42] | Y. Zhang, S. Zhang, X. Ji, Tools, Applications, EEG-based classification of emotions using empirical mode decomposition and autoregressive model, Mult. Tools Appl., 2018. |
1. | Wei Liu, Kebin Jia, Zhuozheng Wang, Zhuo Ma, A Depression Prediction Algorithm Based on Spatiotemporal Feature of EEG Signal, 2022, 12, 2076-3425, 630, 10.3390/brainsci12050630 | |
2. | Zhuozheng Wang, Zhuo Ma, Wei Liu, Zhefeng An, Fubiao Huang, A Depression Diagnosis Method Based on the Hybrid Neural Network and Attention Mechanism, 2022, 12, 2076-3425, 834, 10.3390/brainsci12070834 | |
3. | Yi Ding, Neethu Robinson, Su Zhang, Qiuhao Zeng, Cuntai Guan, TSception: Capturing Temporal Dynamics and Spatial Asymmetry From EEG for Emotion Recognition, 2023, 14, 1949-3045, 2238, 10.1109/TAFFC.2022.3169001 | |
4. | Mahfuza Akter Maria, M. A. H. Akhand, A. B. M. Aowlad Hossain, Md. Abdus Samad Kamal, Kou Yamada, A Comparative Study on Prominent Connectivity Features for Emotion Recognition From EEG, 2023, 11, 2169-3536, 37809, 10.1109/ACCESS.2023.3264845 | |
5. | Wei Liu, Kebin Jia, Zhuozheng Wang, Graph-based EEG approach for depression prediction: integrating time-frequency complexity and spatial topology, 2024, 18, 1662-453X, 10.3389/fnins.2024.1367212 | |
6. | Keyi Lu, Zhihao Gu, Fei Qi, Chengfeng Sun, Hao Guo, Lining Sun, CMLP-Net: A convolution-multilayer perceptron network for EEG-based emotion recognition, 2024, 96, 17468094, 106620, 10.1016/j.bspc.2024.106620 | |
7. | Kaiyang Cheng, Hua Bo, Lei Ren, Wei Kong, 2023, Emotion Recognition from EEG Signals Based on GoogleNet and Multi-Dimensional Fusion, 979-8-3503-9414-6, 76, 10.1109/IMSE61332.2023.00022 | |
8. | Mina Lv, Guoyou Shi, Weifeng Li, Xiaofei Ma, Seafarer Fatigue Identification Based on PSO-ACLSTM, 2025, 11, 2376-7642, 10.1061/AJRUA6.RUENG-1447 | |
9. | Yi Ding, Chengxuan Tong, Shuailei Zhang, Muyun Jiang, Yong Li, Kevin JunLiang Lim, Cuntai Guan, EmT: A Novel Transformer for Generalized Cross-Subject EEG Emotion Recognition, 2025, 36, 2162-237X, 10381, 10.1109/TNNLS.2025.3552603 |
Subject | Video | Arousal | Labels of active networks | Number of active networks |
S1 | 18 | high | 3 5 7 9 10 12 14 15 16 | 9 |
24 | high | 2 4 5 6 7 8 10 12 14 16 19 20 | 12 | |
7 | low | 4 5 7 8 9 11 12 13 14 15 | 10 | |
22 | low | 2 5 6 7 8 9 12 15 16 | 9 | |
S5 | 2 | high | 1 3 6 9 10 11 12 13 14 16 17 19 | 12 |
6 | high | 1 2 4 5 6 9 10 13 16 18 19 20 | 12 | |
26 | low | 1 2 7 8 9 12 14 18 19 20 | 10 | |
27 | low | 6 8 9 10 13 14 17 19 20 | 9 | |
S6 | 5 | high | 1 2 4 5 6 8 10 11 12 16 18 20 | 12 |
7 | high | 2 3 4 5 6 7 8 9 10 14 | 10 | |
8 | low | 4 6 7 8 9 11 13 15 16 17 19 | 11 | |
12 | low | 1 7 10 12 13 17 19 20 | 8 | |
S7 | 7 | high | 2 3 4 8 10 11 13 14 15 17 18 19 | 12 |
10 | high | 1 2 4 5 6 10 13 15 18 19 20 | 11 | |
17 | low | 1 4 6 10 12 13 16 18 20 | 9 | |
29 | low | 4 7 8 9 10 12 14 16 17 20 | 10 |
Subject | Video | Arousal | Number of active networks | P |
S1 | 18 | high | 9 | 0.021 |
24 | high | 12 | ||
7 | low | 10 | ||
22 | low | 9 | ||
S5 | 2 | high | 12 | 0.045 |
6 | high | 12 | ||
26 | low | 10 | ||
27 | low | 9 | ||
S6 | 5 | high | 12 | 0.037 |
7 | high | 10 | ||
8 | low | 11 | ||
12 | low | 8 | ||
S7 | 7 | high | 12 | 0.041 |
10 | high | 11 | ||
17 | low | 9 | ||
29 | low | 10 |
Subject | Video (high arousal) | Active channels | Video (low arousal) | Active channels |
S1 | 18 | F4, FC2, FC5, T7, C3, Cz, T8, CP6, CP2, P7, PZ, P8, PO3 | 7 | FC2, FC1, FC5, T7, CZ, T8, CP6, CP1, P8 |
24 | F7, FC1, FC5, T7, CZ, CP2 | 22 | F4, T7, CZ, CP6, CP2, CP1 | |
S5 | 2 | AF3, CZ, C4, CP1, O1, OZ | 26 | AF3, AF4, CP1, O1 |
6 | AF3, C4, T8, CP1, O1, OZ. | 27 | AF3, C4, CP1, O1 | |
S6 | 5 | Fz, T7, P8 | 8 | Fz, T7, P8 |
7 | Fz, T7, P8 | 12 | Fz, T7, P7, P8 | |
S7 | 7 | AF3, F7, FC6, FC5, CP1, O1 | 17 | AF3, F7, FC6, FC2, FC5, CP1 |
10 | AF3, F7, FC6, FC2, FC5, CP2, CP1, O1 | 29 | F7, F3, FC6, FC5, T8, CP1 |
Subject | Video | Arousal | Labels of active networks | Number of active networks |
S1 | 18 | high | 3 5 7 9 10 12 14 15 16 | 9 |
24 | high | 2 4 5 6 7 8 10 12 14 16 19 20 | 12 | |
7 | low | 4 5 7 8 9 11 12 13 14 15 | 10 | |
22 | low | 2 5 6 7 8 9 12 15 16 | 9 | |
S5 | 2 | high | 1 3 6 9 10 11 12 13 14 16 17 19 | 12 |
6 | high | 1 2 4 5 6 9 10 13 16 18 19 20 | 12 | |
26 | low | 1 2 7 8 9 12 14 18 19 20 | 10 | |
27 | low | 6 8 9 10 13 14 17 19 20 | 9 | |
S6 | 5 | high | 1 2 4 5 6 8 10 11 12 16 18 20 | 12 |
7 | high | 2 3 4 5 6 7 8 9 10 14 | 10 | |
8 | low | 4 6 7 8 9 11 13 15 16 17 19 | 11 | |
12 | low | 1 7 10 12 13 17 19 20 | 8 | |
S7 | 7 | high | 2 3 4 8 10 11 13 14 15 17 18 19 | 12 |
10 | high | 1 2 4 5 6 10 13 15 18 19 20 | 11 | |
17 | low | 1 4 6 10 12 13 16 18 20 | 9 | |
29 | low | 4 7 8 9 10 12 14 16 17 20 | 10 |
Subject | Video | Arousal | Number of active networks | P |
S1 | 18 | high | 9 | 0.021 |
24 | high | 12 | ||
7 | low | 10 | ||
22 | low | 9 | ||
S5 | 2 | high | 12 | 0.045 |
6 | high | 12 | ||
26 | low | 10 | ||
27 | low | 9 | ||
S6 | 5 | high | 12 | 0.037 |
7 | high | 10 | ||
8 | low | 11 | ||
12 | low | 8 | ||
S7 | 7 | high | 12 | 0.041 |
10 | high | 11 | ||
17 | low | 9 | ||
29 | low | 10 |
Subject | Video (high arousal) | Active channels | Video (low arousal) | Active channels |
S1 | 18 | F4, FC2, FC5, T7, C3, Cz, T8, CP6, CP2, P7, PZ, P8, PO3 | 7 | FC2, FC1, FC5, T7, CZ, T8, CP6, CP1, P8 |
24 | F7, FC1, FC5, T7, CZ, CP2 | 22 | F4, T7, CZ, CP6, CP2, CP1 | |
S5 | 2 | AF3, CZ, C4, CP1, O1, OZ | 26 | AF3, AF4, CP1, O1 |
6 | AF3, C4, T8, CP1, O1, OZ. | 27 | AF3, C4, CP1, O1 | |
S6 | 5 | Fz, T7, P8 | 8 | Fz, T7, P8 |
7 | Fz, T7, P8 | 12 | Fz, T7, P7, P8 | |
S7 | 7 | AF3, F7, FC6, FC5, CP1, O1 | 17 | AF3, F7, FC6, FC2, FC5, CP1 |
10 | AF3, F7, FC6, FC2, FC5, CP2, CP1, O1 | 29 | F7, F3, FC6, FC5, T8, CP1 |