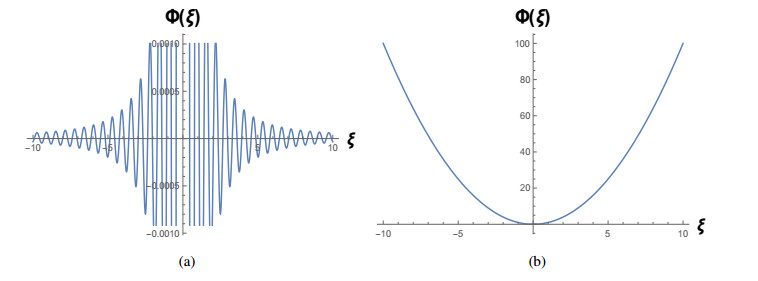
Citation: Antonella Lupica, Piero Manfredi, Vitaly Volpert, Annunziata Palumbo, Alberto d'Onofrio. Spatio-temporal games of voluntary vaccination in the absence of the infection: the interplay of local versus non-local information about vaccine adverse events[J]. Mathematical Biosciences and Engineering, 2020, 17(2): 1090-1131. doi: 10.3934/mbe.2020058
[1] | Ahmed Alshehri, Saif Ullah . A numerical study of COVID-19 epidemic model with vaccination and diffusion. Mathematical Biosciences and Engineering, 2023, 20(3): 4643-4672. doi: 10.3934/mbe.2023215 |
[2] | Maddalena Donà, Pieter Trapman . Possible counter-intuitive impact of local vaccine mandates for vaccine-preventable infectious diseases. Mathematical Biosciences and Engineering, 2024, 21(7): 6521-6538. doi: 10.3934/mbe.2024284 |
[3] | Kelu Li, Junyuan Yang, Xuezhi Li . Effects of co-infection on vaccination behavior and disease propagation. Mathematical Biosciences and Engineering, 2022, 19(10): 10022-10036. doi: 10.3934/mbe.2022468 |
[4] | Roy Curtiss III . The impact of vaccines and vaccinations: Challenges and opportunities for modelers. Mathematical Biosciences and Engineering, 2011, 8(1): 77-93. doi: 10.3934/mbe.2011.8.77 |
[5] | Pride Duve, Samuel Charles, Justin Munyakazi, Renke Lühken, Peter Witbooi . A mathematical model for malaria disease dynamics with vaccination and infected immigrants. Mathematical Biosciences and Engineering, 2024, 21(1): 1082-1109. doi: 10.3934/mbe.2024045 |
[6] | Anthony Morciglio, R. K. P. Zia, James M. Hyman, Yi Jiang . Understanding the oscillations of an epidemic due to vaccine hesitancy. Mathematical Biosciences and Engineering, 2024, 21(8): 6829-6846. doi: 10.3934/mbe.2024299 |
[7] | Xiaojing Wang, Yu Liang, Jiahui Li, Maoxing Liu . Modeling COVID-19 transmission dynamics incorporating media coverage and vaccination. Mathematical Biosciences and Engineering, 2023, 20(6): 10392-10403. doi: 10.3934/mbe.2023456 |
[8] | Lili Han, Mingfeng He, Xiao He, Qiuhui Pan . Synergistic effects of vaccination and virus testing on the transmission of an infectious disease. Mathematical Biosciences and Engineering, 2023, 20(9): 16114-16130. doi: 10.3934/mbe.2023719 |
[9] | Qianqian Cui, Zhipeng Qiu, Ling Ding . An SIR epidemic model with vaccination in a patchy environment. Mathematical Biosciences and Engineering, 2017, 14(5&6): 1141-1157. doi: 10.3934/mbe.2017059 |
[10] | Eunha Shim, Beth Kochin, Alison Galvani . Insights from epidemiological game theory into gender-specific vaccination against rubella. Mathematical Biosciences and Engineering, 2009, 6(4): 839-854. doi: 10.3934/mbe.2009.6.839 |
Mathematical epidemiology (ME) of infectious disease is one of the oldest and richest areas of mathematical biology [1,2], but it is also the area that probably had the largest impact on actual public health policies. Indeed, mathematical models of infections are nowadays routinely applied by international and national public health institutions: from the design of immunization programs up to the preparedness plans against possible future pandemics [3].
A limitation of classical ME is that its key assumptions are the legacy of classical Statistical mechanics. As a consequence, the social contacts between agents at risk of spreading or acquiring the infection are treated as 'encounters of particles' of a perfect gas. Pairwise, the contagion process is abstracted as it were a chemical reaction. As a consequence, transmission processes have been traditionally modelled by means of the mass action law.
Treating human beings as molecules implies that the spread of infectious diseases is totally unaffected by the agents' behavior, and vice-versa, simply because behavior is absent from the models of classical ME. This means that e.g., models used to evaluate the impact and cost-effectiveness of vaccination programs under voluntary immunization did not include neither the individuals risk perceptions about the disease nor those about vaccine-related adverse events (VAEs). Similarly, ME models treat the social contact process between individuals as a physical constant, implying that individuals continue contacting each other at the same rate independently of the magnitude they perceived of the risk of contracting the infection.
Nowadays we know well that the above assumption are quite coarse, possible useful to depict 'normal' situations but totally inadequate to describe scenarios such as vaccine scares, where the perceived risk of VAEs blows up for a while, or the course of an epidemics with high mortality [4,5].
The pioneering work that first included the human behavior in ME was [6], which extended the classical Kermack and McKendrick's ODE epidemic model to account for behavioral responses. Unfortunately, this paper remained relatively isolated until the need of embedding human behavior in ME models became increasingly urgent. This need arose due to the onset of a range of new phenomena such as the increasing mistrust and opposition towards vaccines [7,8,9,10]. In [4,5] it was argued that such phenomena are characteristic of the current landscape of infection and public health in modern industrialised countries. Notably, mistrust towards vaccination can be considered as part of the more global phenomenon known as post-trust society [11].
This led in the last two decades to the birth of a new branch of ME: the behavioral Epidemiology of infectious diseases (BEID) [4,5]. The main aim of BEID is to properly model the role of human behavior in the transmission and control of infectious diseases. This is done by integrating the classical ME tools with models and ideas from disciplines ranging from psychology to neural sciences, and from economics to sociology.
Most BEID models of vaccination behavior include the strategic behavior of agents, and therefore extensively use Game Theory. In particular, Bauch [12] pioneered the applications of evolutionary games to describe the dynamics of the vaccine propensity in a population by using an Imitation Game Dynamics (IGD) i.e., a model where the strategy perceived as better at a given time spreads in the population through imitation. In [12] the perceived risk of infection is taken as linearly increasing with the infection prevalence, whereas the perceived risk of VAE is assumed constant. Instead, in [13] the perceived risk of suffering a vaccine side effect is modelled as an increasing function of the information of the present and past incidence of VAEs.
As expected, in BEID a key role is played by the information that agents can access and use to evaluate risks, which modulates their vaccine–related decisions. In [14], the IGD vaccination model was further extended by including the effect of public interventions in communicating actual risks from vaccines and infection.
Most previously cited BEID models [12,13,14,15], and other works in the same line, are based on ordinary differential equation (ODE) models, and disregard any type of structural heterogeneity. In particular, they are 'spatially homogeneous', which is a crude approximation from at least two standpoints: (ⅰ) the wide and complicate population mobility patterns; (ⅱ) the complicate role played by the spatial information network for behavior in relation to health.
In relation to this, a still unexplored area in the BEID literature regards the incorporation of behavioral hypotheses within classical PDE models of spatial dynamics of the reaction-diffusion type. In our opinion this represent a worthwhile effort for two main reasons. The first one is substantive and relates to the critical role played by information in behavioral epidemiology models. From this standpoint it is fundamental to subdivide the type of information that can be accessed by vaccination decision makers into a few sharply distinct types namely, 'local' vs 'global' vs 'non-local' based on simple hypotheses on the underlying spatial information kernels. The second reason relates to the robust analytic techniques that classical diffusion mathematics makes it available for the understanding of real world processes. Indeed, since the pioneering works of Kendall [16] and of Bailey [1,17] published in sixties, mathematical reaction-diffusion theory was extensively applied in ME models for the spread of human [2,18,19,20,21,22] and animal [23] infectious diseases. A paradigmatic example is the well–known pioneering paper by Noble [24] on European plague epidemics in the 14th century. Recently, Ducrot and Giletti [25] showed that, under Fickian diffusion hypothesis, that the Kermack– McKendrick epidemic model with non-diffusive susceptible population can have pulsating traveling wave solutions. Very recently Magal and coworkers [26] adopted Fickian diffusion with anisotropic diffusion coefficients to model the spread of infectious diseases, with focus on the impact of diffusion on the basic reproduction number. They adopted a similar approach [27] to model the spread of influenza in Puerto Rico, including also behavioral effects. They obtained a good match between their simulations and available spatiotemporal data. As far as vector-borne infectious diseases are concerned, recently Fitzgibbon et al. [28] proposed a model, where the diffusion of hosts was described by Fickian diffusion. Zhao and colleagues [29] again adopted Fickian diffusion to model the spread of a two–groups infectious diseases of SIR type, focusing in particular on the onset of traveling waves.
As stressed in the review paper [30], in its pioneering period, an important chapter of the spatial modelling of the spread of infectious disease was represented by the modelling of the contagion as a non–local process, as in [1,2,16,17,18,19,20].
In the light of the above background, our aim in this article is to investigate the interplay between vaccination dynamics, human decisions, spatial mobility and information. To do so we extended to a continuous spatially structured setting the behavioral epidemiology models for the impact of vaccine adverse events (VAEs) on vaccination choices that were first introduced in [13,14] by a classical economy–oriented game theory approach and reformulated as a process of double contagion of ideas in [5,15,31]. We note here that the first phenomenological approach is partially remindful of the phenomenological theory of innovation diffusion (TID) by Mahajan [32,33,34] (see also [36]). However, taking into the account the second approach, which is more mechanistic, important differences emerge with TID, which are stressed in Section 12.
In particular, we will consider voluntary immunization decisions and focus on the simple but important case where the disease is absent in the population under study. This case is not special at all. On the contrary, it is of central relevance since, e.g., it represents the case of a population where a previously endemic vaccine preventable infectious disease of childhood has been successfully eliminated, so that prevalence is equal to zero, but there is the need to maintain a high-coverage immunization policy in the post-elimination period to prevent the risk of infection re-emergence. A major instance is that of poliomyelitis in industrialized countries. In such a context, where the absence of the infection will remarkably reduce the incentive to immunize thereby weakening the probability of switching from the 'non-vaccinator' to the 'vaccinator' strategy, the dynamics of VAEs will arguably become the key determinant of vaccination decision and collective coverage. To investigate the interplay with spatial mobility the resulting vaccination dynamics is set into the simplest possible framework for spatial mobility, namely classical diffusion based on Fick's law.
The consideration of the spatial effects i.e., the spatial distribution of VAEs, requires to carefully take into account the information on VAEs that is handled by parents of children eligible for vaccination while forming their perceptions of risk, that they will subsequently use to take their immunization decisions.
In relation to this 'information issue' we considered three main scenarios. In the first scenario, the information that individuals access and use uniquely concerns VAEs occurred locally. In the second case, the information used is both the local one and a global, nation-wide, average. Roughly speaking, this scenario corresponds to the case where agents take their decisions also based on national media such as national news or the internet. The third scenario is an intermediate one and aims at taking into the account both the known phenomenon of information attenuation [37], and the reasonable assumption that agents give less weight to events occurred at far distant locations. We represented the latter scenario, where the information used is not purely local but it is also not global, by resorting to a range of different spatial information kernels.
Of the resulting wide collection of problems we have investigated (by also distinguishing the nature of space as bounded vs non-bounded) (ⅰ) the presence and stability of space homogeneous equilibria, focusing on the nontrivial behavioral equilibrium; (ⅱ) conditions for bifurcations; (ⅲ) existence of classical and generalized traveling waves; (ⅳ) effects of awareness campaigns enacted by the Public Health System to effectively sustain vaccine uptake, as first proposed by [14].
To model the impact of human responses to VAEs on the overall dynamics of vaccine uptake in the new-born babies, we follow [12,13,14] and assume that vaccination is a binary decision problem, i.e., one for which only two, mutually exclusive, strategies are played by parents: "'vaccinator"' (strategy 1), and "'non-vaccinator"' (strategy 2). Let us consequently denote by P(x,t) and A(x,t) the fractions of vaccination decision makers (e.g., parents of children eligible for vaccination) at location x and time t that follow, respectively, strategy 1 and 2. The previously cited models of IGD [12,13,14] all relied on the concept of payoff. However, many evolutionary game models belong to the class of urn models [38], a remarkably wide family that includes both chemical kinetics models as well as classical ME models. Thus, we follow here [5,15,31], where the vaccination IGD was derived as a model of 'double contagion' of ideas between the two involved groups namely, the group playing the 'vaccinator' strategy and the one playing the 'non-vaccinator' strategy).
Thus, by extending to the present spatio-temporal setting the IGD in [5,31], we obtain the following family of models:
∂tP=D∇2P+ϑ(Mi)AP−α(Mse)AP,∂tA=D∇2A−ϑ(Mi)AP+α(Mse)AP, | (2.1) |
where t>0, x∈Ω, with Ω a bounded subset of Rn, n=1,2. If n=1, then we assume that Ω=[−L,L], L>0, while, if n=2, then we assume that its boundary is sufficiently smooth.
In particular, ϑ(Mi) and α(Mse) represent the strategy-specific 'transmission rates' following social contacts with individuals playing the other strategy. They are assumed to be non-decreasing functions of the variables Mi and Mse. The latter are information indices summarizing the available information about the (current or past) state of the infection (Mi) and of VAEs (Mse), respectively, that are used by parents to formulate evaluations of related risks.
Assuming a stationary population and normalizing w.r.t. its steady state, since A=1−P, system (2.1) yields:
∂tP=D∇2P+P(1−P)(ϑ(Mi)−α(Mse)). | (2.2) |
As for the key quantities Mi and Mse, given our focus on situations where the infection has been eliminated, we assume that the perceived risk related to infection is constant:
ϑ(Mi(I))=ϑ0 | (2.3) |
The previous formulation mimics the situation where the infection is absent, so the corresponding perceived risk is prevalence–independent. We assume that ϑ0>0 to reflect a non–null perceived risk of infection even in the absence of infection. This can be justified by the continued activity of an active public health system that aims to keep a high degree of population awareness on the risk of reintroduction which is perceived as homogeneous throughout the entire space. Moreover, we assumed that the perceived risk of VAEs depends on information on vaccine side effects occurring both locally but also non-locally, as follows:
α(Mse(P))=α0+α1P+α2J(P), | (2.4) |
where
J(P)=∫Ωϕ(x−y)P(y,t)dy, | (2.5) |
where function ϕ(x) is non-negative with
∫Ωϕ(x)dx=1. | (2.6) |
and even:
ϕ(x)=ϕ(−x), for all x∈Ω. | (2.7) |
To sum up, the perceived risk of VAEs α(Mse), has three components: (ⅰ) a baseline value α0 possibly constant over space, mirroring a true underlying risk of VAEs, (ⅱ) a strictly local component α1P, possibly reflecting local deviations in vaccine coverage and ensuing deviations in the local number of VAEs, and (ⅲ) a non-local component α2J(P) tuning risk perceptions arising from differences in VAEs at different geographic sites, according to a suitable spatial information kernel. In particular, the functional J(P) describes two mathematically equivalent scenarios, where individuals located at position x can: ⅰ) receive information concerning the value of P at the space point y with a weight/attenuation ϕ(x−y); ⅱ) receive full spatial information but, in taking their decisions, they assign a weight ϕ(x−y) to P(y,t).
Setting ϑ∗=ϑ0−α0>0, brings to the following model
∂tP=D∇2P+P(1−P)(ϑ∗−α1P−α2J(P)), | (2.8) |
with x∈Ω and t>0.
Let l be a characteristic length of the domain Ω; to mathematically simplify the analysis, the model is reformulated in terms of the following variables:
t∗=ϑ∗t, x∗=xl−1, D∗=Dϑ−1∗l−2, |
α∗1=α1ϑ−1∗, α∗2=α2ϑ−1∗, | (2.9) |
where we assumed 1/ϑ∗ is time unit. The set derived from Ω by adimensionalization will be denoted as Ω∗.
Thus, the model (2.8) becomes (for the sake of simplicity we omit the stars):
∂tP=D∇2P+P(1−P)(1−α1P−α2J(P)), | (2.10) |
with x∈Ω and t>0.
Moreover, by imposing Neumann boundary conditions:
∂xP=0, on ∂Ω, if Ω⊂R, or ∂nP=0, on ∂Ω,if Ω⊂R2, | (2.11) |
where n is the outer normal vector with respect to ∂Ω.
In the case in which the characteristic size of Ω is very large (for example in one dimension, L≫1), then we can redefine Ω as Ω=(−L,L) and (as if we operated the limit L→+∞) assume that the domain is unbounded, i.e., Ω=Rn, n=1,2.
In the special case where people do not take into account the spatial variation in VAEs, i.e., α(Mse(P))=α0, model (2.10) reduces to the well-known Fisher-Kolmogorov equation
∂tP=D∇2P+P(1−P), | (3.1) |
introduced in [39,41]. The FK equation (3.1) in our case describes the spread (via traveling waves [22]) of the idealized case P=1, where all people is in favor of the vaccination and the 'recession' of the case P=0, where no subjects are in favor of vaccination.
Arguably, the behavior of (2.10) critically depends on the specific functional form of the spatial information kernel ϕ(x). We list a number of relevant forms.
The simplest case is when the available information is purely local, that is
ϕ(x)=δ(x), |
where δ(x) is the Dirac function. Then, Eq (2.10) reads as:
∂tP=D∇2P+P(1−P)(1−αP), | (4.1) |
where, with slight abuse of notation, we set
α=α1+α2. |
As argued in the introduction, by far the most important specific form for ϕ(x) is the constant one, namely
ϕ(x)=1μ(Ω), |
where μ(Ω) is the measure of Ω; thus, the non–local term (2.5) yields:
J(P)=1μ(Ω)∫ΩP(y,t)dt. | (4.2) |
This specific form models the noteworthy scenario where people are exposed to a global (average) information e.g., by the media (including the internet) and the Public Health system, about the current state of vaccine-side effects coming from the whole domain Ω. The resulting model is:
∂tP=D∇2P+P(1−P)(1−α1P−α21μ(Ω)∫ΩP(y,t)dt). | (4.3) |
Another important scenario is when the information about VAEs comes from the whole Ω but it is either attenuated with the distance (this was possibly true for diseases in historical epochs) or it is the individual receiving the information that pays lesser and lesser weight depending on the distance.
Two reasonable functional forms that might well represent this scenario are:
ⅰ) Gaussian decay
ϕ1(x)=Cge−ax2, |
where a>0 and 1/√a is the characteristic attenuation length. In Rn (n=1,2) the normalization constant Cg is:
Cg=(aπ)n2. |
In this case, model (2.10) becomes:
∂tP=D∇2P+P(1−P)(1−α1P−α2∫ΩCge−a(x−y)2P(y,t)dy). |
ⅱ) Exponential decay,
ϕ2(x)=Cee−a|x|, |
where a>0 and in Rn (n=1,2) the constant Ce reads as follows
Ce=a2, in R, and Ce=a22π, in R2. |
In this case, model (2.10) becomes:
∂tP=D∇2P+P(1−P)(1−α1P−α2∫ΩCee−a|x−y|P(y,t)dy). |
Another important class of spatial kernels is represented by non–local kernels with bounded support, i.e., that are null beyond a given distance. An example is:
ϕ3(x)=CN(1−|xN|2)Hev(N−|x|) |
where Hev(.) is the Heaviside function and
CN=34N, in R, and CN=2πN2, in R2. |
In this case, model (2.10) becomes:
∂tP=D∇2P+P(1−P)(1−α1P−α2∫ΩCN(1−|x−yN|2)Hev(N−|x−y|)P(y,t)dy). |
Another example in R is
ϕ4(x)=12hHev(h−|x|), |
and in R2
ϕ4(x)=(1/(πh2))Hev(h−|x|). |
In this case, model (2.10) becomes:
∂tP=D∇2P+P(1−P)(1−α1P−α2∫Ω(1/(πh2))Hev(h−|x−y|)P(y,t)dy). |
As it is to be expected, the Fourier transform will be important in the mathematical analysis of the problem in study.
We use the following definition of Fourier transform for a generic function f(x):Rn⟶R (n=1,2):
˜f(ξ)=∫Rnf(x)e−iξxdx, | (4.4) |
where ξ is the Fourier's vector, ξx=ξ1x in R or ξx=ξ1x1+ξ2x2 in R2. Here and further down ξ2=ξ21 in R or ξ2=ξ21+ξ22 in R2.
We have that:
˜ϕ1(ξ)=e−ξ24a, in Rn, n=1,2; |
˜ϕ2(ξ)=(a2a2+ξ2)(n+1)/2, in Rn, n=1,2; |
˜ϕ3(ξ)=3N3ξ3(sin[Nξ]−Nξcos[Nξ]), in R; |
˜ϕ4(ξ)=1hξsin(hξ), in R. |
We briefly summarize the results in [13], concerning the behavior of the spatially homogenous model corresponding to (4.1). This is described by the ordinary differential equation (ODE)
P′(t)=P(1−P)(1−αP). | (5.1) |
A first equilibrium P0=0 corresponds to the no vaccination scenario where no parent is favorable to immunization. This non-vaccinator equilibrium, NVE is always unstable. If 1<α, then there exists a non-trivial behavioral equilibrium
P2=1α, | (5.2) |
that is globally attractive in (0, 1). We term P2 a behavioral equilibrium (BE) because its level is tuned by the balance between the parents' perceptions of risks based on the handling of available information. Finally, there is a pure vaccinator equilibrium, PVE P1=1 [12,13], corresponding to the unrealistic scenario where all parents are favorable to immunization. The PVE is unstable if 1<α, and globally attractive if 0≤α≤1.
Arguably, in the case of an infection that has been eliminated one expects that at least in an initial phase it holds P2>pC where pC=1−1/R0 is the critical vaccination threshold allowing infection elimination, and R0 is the basic reproduction number of infection. However, this does not need to be true at subsequent times, and this is why it is important for the public health system to monitor the evolution of VAEs and related information. Finally, if α=0 then the model reduces to the well-known logistic model (see also next section).
The equilibria of the ODE model (5.1) described in Section 5 are also homogenous equilibria of the spatially structured model (4.1). In this section we will investigate their stability, focusing primarily on the behavioral equilibrium P2 defined in (5.2), since the other two equilibria (P0=0, i.e., no vaccinators, and P1=1, i.e., all vaccinators) do not correspond to realistic situations.
We will explore multiple relevant cases depending on: ⅰ) the nature of the information used to make the vaccination decision: local, global or non–local i.e., on the structure of the spatial information kernel; ⅱ) the nature of Ω: bounded vs non-bounded.
Under purely local information i.e., Eq (4.1) with boundary condition (2.11), the structure of the local stability of the homogeneous equilibria is similar to the one characteristic of the ODE model (5.1). We proved the following:
Theorem 1. The non-vaccinator equilibrium P0 is always unstable.
If α>1, the behavioral equilibrium P2 is locally asymptotically stable (LAS) and the PVE P1 is unstable. Moreover, if Ω is bounded then P2 is also globally asymptotically stable (GAS).
If 0≤α≤1, P2 is not an admissible equilibrium and the PVE P1 is LAS. Moreover, if Ωis bounded then P1 is also globally asymptotically stable (GAS).
We report the proof for the non-trivial parts. If Ω is bounded, from the linearized equation around the behavioral equilibrium P2=1/α,
∂tw=D∇2w−(1−P2)w, | (6.1) |
it follows that the the m–th eigenvalue is given by:
λm=−Dξ2m−(1−P2)<0, | (6.2) |
where νm=−ξ2m is the m–th eigenvalue of the heat equation with Neumann conditions (2.11) in Ω. For example, if Ω=[−L,L], then ξm=mπ/L, m∈N.
Similarly, if Ω is unbounded, then by applying the Fourier's Transform (4.4), we obtain the following expression for the spectrum:
λ(ξ)=−Dξ2−(1−P2)<0. | (6.3) |
Formulas (6.2) and (6.3) will be useful to assess the impact of the local vs non-local vs global structure of information on our system.
The global stability of P2 and P1, respectively, can both be demonstrated by adopting as Liapunov functional the free energy:
Li(t)=∫Ω(D|∇P|2+U(P))dx, | (6.4) |
where U(P) is the 'potential' (associated to the 'force' P(1−P)(1−αP)), given by:
U(P)=−∫(P(1−P)(1−αP))dP. |
from which the claim easily follows.
Under global information i.e., when people are exposed to the average information on VAEs coming from the whole domain (note this scenario only concerns the case where Ω is bounded):
J(P)=⟨P(x,t)⟩=1μ(Ω)∫ΩP(y,t)dy. |
the model (2.10) becomes:
∂tP=D∇2P+P(1−P)(1−α1P−α21μ(Ω)∫ΩP(y,t)dy), | (6.5) |
with Neumann conditions (2.11).
The linearized equation at P2=1/α reads as follows
∂tw=D∇2w−P2(1−P2)(α1w+α21μ(Ω)∫Ωw(y,t)dy). | (6.6) |
Setting w(x)=cos(ξmx) as eigenfunction, it is straightforward to show that the m–th corresponding eigenvalue is given by
˜λ0=−(1−P2)<0, if m=0, ˜λm=−Dξ2m−α1P2(1−P2)<0, if m≤1. | (6.7) |
Thus P2 is LAS.
Interestingly, if m=0, referring to (6.2) and (6.7), then λ0=˜λ0. On the contrary, if m≥1, comparing eigenvalues in (6.2) and (6.7), we can deduce that ˜λm>λm: therefore eigenvalues in (6.7) are 'less negative' than their counterparts for the local information model in (6.2). This indicates a slower convergence to P2 in Eq (6.5) compared to Eq (4.1) for all modes m≥1.
As for the NVE P0=0, the eigenvalues obey
˜λm=−Dξ2m+1, |
i.e., at least the mode 0 is unstable. Moreover, the linearized equation of (6.5) around the PVE P1=1 gives the eigenvalues:
˜λm=−Dξ2m+α−1. |
Thus P1 is unstable, unless the unlikely event 0≤α≤1 holds, implyig the LAS of P1.
At variance with the global information scenario, under non-local information it is necessary to distinguish the case where Ω is unbounded from the one where it is bounded.
As noted above, assuming that L≫1, we can study the following equation deduced from (2.10)
∂tP=D∇2P+P(1−P)(1−α1P−α2∫Ωϕ(x−y)P(y,t)dy) | (6.8) |
with x∈Ω=Rn, n=1,2, t>0 and Neumann condition (2.11) on the boundary (i.e., at infinity).
The associated linearized equation at a generic homogeneous steady state P∗ is:
∂tw=D∇2w+[(1−2P∗)(1−αP∗)−α1P∗(1−P∗)]w−α2P∗(1−P∗)∫Ωϕ(x−y)w(y,t)dy. | (6.9) |
The associated eigenvalue problem has the form:
D∇2W+[(1−2P∗)(1−αP∗)−α1P∗(1−P∗)]W−α2P∗(1−P∗)∫Ωϕ(x−y)W(y)dy=λW. | (6.10) |
Let us now focus on the case of primary interest here namely when P∗ is given by the behavioral equilibrium i.e., P2 defined in (5.2). This yields:
D∇2W−P2(1−P2)(α1W+α2J(W))=λW. | (6.11) |
We consider the linearized operator L on L2(Rn) (n = 1, 2), such that
LW:=−D∇2W+P2(1−P2)(α1W+α2J(W)). | (6.12) |
The spectrum of this operator is denoted by σ(L). The eigenvalue problem
LW=λW |
is called spectrally stable if σ(L)⊂[0,+∞) and spectrally unstable if there exists λ<0 | λ∈σ(L). Moreover, σ(L) is spectrally stable iff the operator L is positive, i.e., (LW,W)>0 for any W. Let us introduce the function
Φ(ξ)=Dξ2+P2(1−P2)(α1+α2˜ϕ(ξ)). |
where ˜ϕ(ξ) is the Fourier transform (defined in formula (4.4)) of ϕ(x), which is real since we assumed symmetric ϕ(x).
Noting that
˜ϕ(0)=∫Ωϕ(y)dy=1, | (6.13) |
we can state the following:
ⅰ) function Φ(ξ) evaluated at ξ=0 is always positive, i.e.,
Φ(0)=P2(1−P2)(α1+α2)=1−P2>0; |
ⅱ) for any given continuous and bounded function ˜ϕ, Φ(ξ) becomes strictly positive if D is sufficiently large. Thus, for sufficiently large values of the diffusion coefficient the behavioral equilibrium P2 will be stable irrespective of the shape of the spatial information kernel.
On the contrary, if ϕ(x) has bounded support, then its Fourier transform can take negative values that can destabilize P2.
We now consider the special cases of the spatial information kernel ϕ(x) considered in Section 4.
If ϕ(x)=ϕ1(x)=Cge−ax2 then function Φ takes the form:
Φ(ξ)=Dξ2+1α(1−1α)(α1+α2e−ξ24a). | (6.14) |
As the latter expression is strictly positive, the behavioral equilibrium P2 is always locally stable.
Similarly, under the exponential tent kernel ϕ(x)=ϕ2(x)=Cee−a|x|, x∈Rn (n=1 or 2):
Φ(ξ)=Dξ2+1α(1−1α)(α1+α2(a2a2+ξ2)(n+1)/2). | (6.15) |
Therefore, also in this case function Φ(ξ) is strictly positive and P2 is locally asymptotically stable.
These two examples suggest that kernels that are non-null over the whole space, and have a realistic shape (i.e., are decreasing in the distance from the current site), always promote the local stability of the behavioral equilibrium P2.
To analyse the effects of the two proposed kernels with bounded support, we will only consider, for sake of notation simplicity, the case Ω=R.
Under the kernel ϕ(x)=ϕ3(x)=CN(N2−x2)Hev(N−|x|), with N>0, function Φ is as follows:
Φ(ξ)=Dξ2+1α(1−1α)(α1+α23N3ξ3(sin[Nξ]−Nξcos[Nξ])). | (6.16) |
Depending on N and on the other parameters, Φ(ξ) can be strictly positive or can change sign. As noted before, Φ(0)=1−1/α>0. Also in this case, if ξ is large enough, the function Φ(ξ) is positive and the equilibrium P2 is locally stable. When the diffusion coefficient is large enough, the local stability in ensured. The instability can appear if D is small enough and if the ratio ρ=α2/α1 is large enough.
This type of behavior is illustrated in the Figure 1 in the particular case in which α1=0.
We finally consider ϕ(x)=ϕ4(x)=1/(2h)Hev(h−|x|), with h>0. The function Φ(ξ) reads as follows:
Φ(ξ)=Dξ2+1α(1−1α)(α1+α2sin[hξ]hξ). | (6.17) |
Depending on h and the other parameters, this function can be strictly positive or can change sign. Moreover, Φ(ξ) is positive for |ξ| sufficiently large. If the diffusion coefficient is large, then Φ(ξ) is positive for all ξ, and the behavioral equilibrium P2 is locally stable. The instability can appear if D is small enough and ρ=α2/α1 is large enough.
A possible form of function Φ(ξ) is given in Figure 2, in the special case in which α1=0.
So we may say that in the examined examples, the stability of P2 depends on whether or not the support of ϕ(x) is bounded. If it is bounded, then P2 can become unstable depending on the parameters characterizing the kernel ϕ(x), i.e., on the features of the kernel. In this case some spatially heterogeneous solutions can appear.
Proceeding as above, it is an easy matter to show that replacing P∗=P0=0 (the 'no-vaccinators' scenario) in eigenvalue problem (6.10), we obtain instability (since Φ(ξ)=Dξ2−1); moreover if α>1 (that correspond to biological existence of P2), also P1=1 is unstable:
Φ(ξ)=Dξ2+1−α. | (6.18) |
In the unlikely event that α≤1, instead, the all-vaccinator homogeneous equilibrium P1=1 is LAS.
In Figure 3 an illustration of stability analysis of equilibrium P2 is given for Eq (6.8). The Eq (2.10) is simulated with the step function ϕ4(x)=1/(2h)Hev(h−|x|) as kernel. Choosing a small perturbation as initial data, in Figure 3(a), the solution evolves in time approaching to the stable equilibrium P2; while in Figure 3(b) the loss of stability of the constant equilibrium point P2 and the convergence to a periodic spatial structure is shown. The numerical results confirm the analytical ones: in the case where D is sufficiently large and the step h small, P2 is LAS, if D is small and h increases, we see convergence to non-constant structures.
Assuming now that Ω is bounded, we obtain the following equation from (2.10)
∂tP=D∇2P+P(1−P)(1−α1P−α2∫Ωϕ(x−y)P(y,t)dy), | (6.19) |
with t>0, x∈Ω and Neumann conditions (2.11).
For the sake of notation simplicity we will consider here Ω=[−L,L]. The eigenvalues problem obtained as a result of the linearization about a generic steady state P∗ is given by the equality
DW′′+[(1−2P∗)(1−αP∗)−α1P∗(1−P∗)]W−α2P∗(1−P∗)∫L−Lϕ(x−y)W(y)dy=λW. | (6.20) |
Since for both the NVE and the PVE equilibria P∗=P0=0 and P∗=P1=1 the non–local term disappears, the resulting local stability analysis is straightforward and it is omitted. Therefore, we will only focus on the behavioral equilibrium P2=1/α.
Here, we make some assumptions. We will suppose that the function ϕ(x) is defined on the whole axis and that it is periodic with the period 2L:
ϕ(x+2Lm)=ϕ(x),−L≤x≤L,m=±1,±2,... | (6.21) |
Taking P∗=P2, brings to the eigenvalue problem
DW′′−P2(1−P2)(α1W+α2∫L−Lϕ(x−y)W(y)dy)=λW, |
in the form
w(x)=cos(ξmx), ξm=mπL, m=0,1,2,... |
Note that the boundary conditions (2.11) are satisfied. Taking into account that function ϕ is periodic and even, we obtain
∫L−Lϕ(x−y)cos(ξmy)dy=cos(ξmx)∫L−Lϕ(z)cos(ξmz)dz. |
Thus, we have that the m–th eigenvalue reads as follows:
λ∗m=−Dξ2m−P2(1−P2)(α1+α2ϕm), | (6.22) |
where
ϕm=∫L−Lϕ(z)cos(ξmz)dz. | (6.23) |
We note that λ∗m is negative for m=0 and for m sufficiently large, while it can be positive for some intermediate values of m depending on the function ϕ and on the values of parameters, similarly to what we observed in the continuous spectrum analysis.
Let us now consider ϕ(x)=ϕ3(x)=(1/(2h))Hev(h−|x|), 0<h<L. Then
ϕm=1ξmhsin(ξmh). | (6.24) |
Consider the functions
Φm(h)=−Dξ2m−P2(1−P2)(α1+α21ξmhsin(ξmh)), m=1,2,... |
If Φm(h) is a positive function, then the corresponding eigenvalue λ∗m is also positive. Let us find the conditions on parameters when the maximal eigenvalue is zero (stability boundary). From the conditions
Φm(h)=0,Φ′m(h)=0, |
where prime denotes the derivative with respect to h, we obtain
ν=tanν,m2P2(1−P2)(α1+α2νsinν)=0, | (6.25) |
where ν=ξmh. The first relation in (6.25) allows us to find ν, and the second relation determines the stability boundary.
In the case of nonlocal information and bounded one-dimensional Ω, it is possible to carry out the bifurcation analysis (a.k.a. weakly nonlinear analysis) for Eq (6.19) under the assumption that D is the bifurcation parameter [40].
The stationary non-homogenous solutions of the model solve
DP′′+P(1−P)(1−α1P−α2∫L−Lϕ(x−y)P(y,t)dy)=0, | (7.1) |
with P′(−L)=P′(L)=0 and assuming that conditions (6.21) for the kernel ϕ(x) hold.
The equilibrium state P(x)=P2=1/α is a solution of (7.1), independently from the values assumed by D.
If D crosses a bifurcation value D0, a simple real eigenvalue of the linearized problem crosses zero. In order to study this bifurcation, we look for solutions of (7.1) in the form of the expansion
P(x)=P2+εp1(x)+ε2p2(x)+... |
where ε is a small parameter. We set
D=D0+εD1+ε2D2+... |
Substituting these expansions into Eq (7.1) and equating the terms in ε1, we get
D0p′′1(x)−α1P2(1−P2)p1(x)−α2P2(1−P2)∫L−Lϕ(x−y)p1(y)dy=0, | (7.2) |
with p1(−L)=p1(L)=0. This problem coincides with eigenvalue problem (6.20) with P∗=P2 and λ=0. Hence the value D0 should be chosen in such a way that this eigenvalue problem has a zero eigenvalue, p1(x)=cos(ξmx) is the corresponding eigenfunction, with m an integer, m≠0.
Next, we equate the terms with ε2:
D0p′′2(x)−α1P2(1−P2)p2(x)−α2P2(1−P2)∫L−Lϕ(x−y)p2(y)dy=f, | (7.3) |
where p2(−L)=p2(L)=0 and
f=−D1p′′1(x)+(1+α1−3α1P2−α2P2)p1(x)2+α2(1−2P2)p1(x)∫L−Lϕ(x−y)p1(y)dy==(−D1+1−2P2P2(1−P2)D0p1(x))p′′1(x)+(1+α1−3α1P2−α2P2+α1(2P2−1))p21(x) |
In order to obtain solvability conditions for problem (7.3), let us note that problem (7.2) is self-adjoint since the kernel ϕ is an even function. Indeed, it can be directly verified that
∫L−Lv(x)(LP)(x)dx=∫L−LP(x)(Lv)(x)dx, |
where L is the operator which corresponds to the left-hand side of (7.2) and which acts on C2 functions satisfying the boundary conditions. Hence problem (7.3) is solvable if and only if
∫L−Lf(x)p1(x)dx=0. |
Therefore
D1=D0(1−2P2)P2(1−P2)∫L−Lp31(x)dx∫L−Lp21(x)dx=0, |
and
p2(x)=A(1+Bcos(2ξmx)), |
where
A=D0ξ2m(2P2−1)2P2(P2−1)2,B=(1−P2)4D0ξ2m+P2(1−P2)(α1+α2ϕ2m). |
The terms with ε3 give the problem
D0p′′3(x)−α1P2(1−P2)p3(x)−α2P2(1−P2)∫L−Lϕ(x−y)p3(y)dy=f1, | (7.4) |
where
f1=−D2p′′1−α1p31+(2+2α1−6α1P2−2α2P2)p1(x)p2(x)+α2[(1−2P2)p2(x)−p21(x)]∫L−Lϕ(x−y)p1(y)dy+α2(1−2P2)p1(x)∫L−Lϕ(x−y)p2(y)dy=(−D2+D0(1−2P2)p2(x)P2(1−P2)−D03P22−3P2+1P22(1−P2)2p1(x)2)p″1+D01−2P2P2(1−P2)p1(x)p″2. |
From the solvability condition
∫−LLf1(x)p1(x)dx=0, |
we obtain
D2=D04A(2+5B)P32−3[3+2A(2+5B)]P22+[9+2A(2+5B)]P2−34P22(1−P2)2. |
If D2≠0, then from the expansion for D we obtain
ε=±√D−D0D2, |
and up to the second-order terms,
P(x)=P2+εP1(x)+ε2P2(x). | (7.5) |
The bifurcation is supercritical (D>D0) for D2>0 and P2>12. The bifurcation is subcritical (D<D0) if the ratio (2P2−1)/D2 is negative.
Preliminarily, we recall that in Section 2 we pointed out that in case of constant rate of transfer from the strategy 'vaccine' to the strategy 'no–vaccine' (α(Mse)=α0) our model is equivalent to the most prototypical equation generating traveling waves, the Fisher-Kolmogorov equation (3.1). However, this case can more be considered as pathological than a realistic scenario.
In this section, we look in non-pathological cases (from the epidemiological viewpoint) for the onset of possible traveling waves [22,43], i.e., heteroclinic connections between two equilibria.
In this section, we restrict the analysis to the case in which the domain Ω is one-dimensional and unbounded, i.e., Ω=R.
Wave solutions for Eq (4.1)
∂tP=∇2P+P(1−P)(1−αP), |
are function of the form P(x−ct)=p(z) bounded on the whole axis and twice continuously differentiable. The constant c is a speed of the wave, z=x−ct is the moving coordinate frame and
limx→±∞P(x)=P± | (8.1) |
with P− unstable and P+ stable equilibrium point. After substitution of variable z in Eq (4.1), we obtain the following ordinary differential equation:
Dp″+cp′+p(1−p)(1−αp)=0. | (8.2) |
It is known that, a necessary condition for the existence of the solution is that the P− must have an unstable (departing) manifold and P+ a stable (incoming) manifold. After stability analysis, and following [44], we obtain the following result:
Theorem 2. If α>1, there exists a constant c(1)=2√D such that ∀ c∈[c(1),+∞[, there exists a traveling wave solution of velocity c connecting equilibrium P2=1/α and equilibrium P0=0, i.e., a function P(x−ct), solution of Eq (8.2) on the real line ]−∞,+∞[ and satisfying Eq (8.1) with P−=P2 and P+=P0. This solution is monotone decreasing and the derivatives P″(z) and P′(z) tend to zero as x→±∞. Moreover, a traveling wave solution of velocity c connecting equilibria P1 and P0 cannot exist.
We recall that for bounded Ω we had shown that the spatially homogenous equilibrium P2 is GAS. In the above theorem it is shown, roughly speaking, that the TW are such that the scenario where the value P=P2 spreads until it invades all the space.
The case 0<α<1 has here some further mathematical interest, since the equation can be read as a modification of the Fisher-Kolmogorov equation.
Theorem 3. If 0<α<1, there exists a constant c(1) such that ∀ c∈[c(1),+∞[, there exists a traveling wave solution of velocity c connecting equilibrium P1=1 and equilibrium P0=0, i.e., a function P(x−ct), solution of Eq (8.2) on the real line ]−∞,+∞[ and satisfying Eq (8.1) with P−=P1 and P+=P0. This solution is monotone decreasing and the derivatives P″(z) and P′(z) tend to zero as x→±∞.
Traveling wave solutions for the Eq (4.1) are shown in Figure 4 in the case in which α>1. They connect the constant state P2 at −∞ and the constant state P0 at +∞, with the minimal speed c=c(1)=2√D.
In previous sections, we proved that, in the case of non–local information, the spatially homogeneous equilibrium solution P2=1/α can lose its stability. Then some spatial structures can bifurcate from it. Therefore, instead of traveling waves connecting P0 and P2, we can expect the existence of some other solutions connecting P0 at +∞ with some structures at −∞. Such solutions are called generalized traveling waves (GTW) and were first introduced in [45] for reaction-diffusion systems. Such solutions can be characterized by two main properties:
(1) They exist for all t∈R. Moreover, under some conditions, such solution can be unique and stable.
(2) They are propagating solutions, which can be explained as follows: let q be a constant, P+<q<P−. For each t fixed consider the equation P(x,t)=q with respect to x. Denote by m+q(t) its maximal solution (if it exists) and by m−q(t) its minimal solution. If m±q(t)t→c as t→∞, then we say that this solution propagates with the speed c. Thus, GTW are global propagating solutions.
Thus, we want to study GTW solution for the equation
∂tP=D∇2P+P(1−P)(1−α1P−α2∫∞−∞ϕ(x−y)P(y,t)dy). | (8.3) |
where Ω is unbounded.
Consider the Cauchy problem for the equation
∂tP=D∇2P+c∂P∂x+P(1−P)(1−α1P−α2∫∞−∞ϕ(x−y)P(y,t)dy), | (8.4) |
with c≥2√D a given constant. Assume that the initial condition P(x,0)=P0(x) is non-negative and less than 1. Then the solution P(x,t) exists and is also non-negative and less than 1 for all t≥0. Consider
J(x,t)≡∫∞−∞ϕ(x−y)P(y,t)dy≥0. |
Hence
d(x,t)≡1−α1P−α2J(x,t)≤1. |
Equation (8.4) becomes
∂tP=D∇2P+c∂P∂x+P(1−P)d(x,t). | (8.5) |
Consider also the equation
∂tv=D∇2v+c∂v∂x+v(1−v). | (8.6) |
The following result holds:
Lemma 4. If c≥2√D, there exists a stationary solution vc(x) of (8.6) such that
P(x,0)<vc(x), x∈R ⇒ P(x,t)<vc(x), x∈R, t>0. | (8.7) |
Proof. Denoting z=v−P and taking the difference of Eqs (8.6) and (8.5), we obtain
∂tz=D∇2z+c∂z∂x+z(1−v−P)+P(1−P)(1−d(x,t)). | (8.8) |
Since the last term in the right-hand side of Eq (8.8) is non-negative, then from the inequality z(x,0)>0 for all x∈R, it follows that z(x,t)>0 for all t>0 and x∈R. Hence,
P(x,0)<v(x,0), x∈R ⇒ P(x,t)<v(x,t), x∈R, t>0. | (8.9) |
Stationary solutions of Eq (8.6) correspond to traveling waves for the KPP-equation [39,41]. If c≥2√D, then they are monotone in space and stable, while for 0<c<2√D they are non-monotone and unstable [41,44]. These waves have limits at infinity: v(−∞)=1, v(+∞)=0.
Denoting by vc(x) a stationary solution of Eq (8.6) for c≥2√D, the claim is proved.
Thus, we obtain an estimate from above of the solution of the Cauchy problem associated to (8.5). We now estimate it from below. From the inequality (8.9),
J(x,t)≤∫+∞−∞ϕ(x−y)vc(y)dy≡K(x). | (8.10) |
We assume w<1: consider the equation
∂tw=D∇2w+c∂w∂x+w(1−w)(1−α1vc−α2K(x)). | (8.11) |
Denoting s=P−w and taking the difference between Eqs (8.5) and (8.11), we obtain
∂ts=D∇2s+c∂s∂x+s(1−P−w)(1−α1vc−α2J(x))+α1P(1−P)(vc−P)+α2P(1−P)(K(x)−J(x,t)). | (8.12) |
Since the last two terms in the right-hand side of this equation are non-negative, then
w(x,0)≤P(x,0), x∈R, ⇒ w(x,t)≤P(x,t), x∈R, t>0. | (8.13) |
The following result holds:
Lemma 5. If c≥2√D, there exists a stationary solution wc(x) of (8.11) such that wc(x0)=0 for some x0, wc(x)>0 for x>x0, wc(x)<0 for x<x0 and wc(x)∼vc(x), when x→∞
Proof. We look for stationary solution of Eq (8.11), i.e.,
Dw″+cw′+w(1−w)(1−α1vc−α2K(x))=0. | (8.14) |
Since vc and K(x) tend to zero when x→∞, then 1−α1vc−α2K(x) is close to 1 in some right half-axis. Then, for c≥2√D, solutions of (8.14) are close to functions vc(x) when x→∞.
We prove the existence of a solution which has a zero. Denote g(x)=1−α1vc−α2K(x) and let I=(−∞,ω) be the interval where g(x)<0: then any non-zero solution of (8.14) has at most one zero in I. Indeed, let w(x) be solution of (8.14) and x0 one of its zeros. Put
W(x)=ecD(x−x0)w(x)w′(x), x∈I. | (8.15) |
Taking into account Eq (8.14), we have
W′(x)=ecD(x−x0)((w′)2−w2D(1−w)g(x))≥0. | (8.16) |
So W is non-decreasing on I. If W has another zero x1∈I, then W(x)=0 on [x0,x1]. Thus w is constant on [x0,x1], namely w=0 (from (8.14)) on [x0,x1] and consequently on I. The contradiction shows that w has at most one zero in I.
Since w(x) converges to zero when x→∞, there exists x∗ large enough such that we can assume that Eq (8.14) becomes:
Dw″+cw′+g(x)w=0. | (8.17) |
Let c>2√D. Since g(x)→1 at infinity, there exist two linearly independent solutions of Eq (8.17) given by [42]
w1(x)∼e−λ1x,w2(x)∼e−λ2x, | (8.18) |
where λ1 and λ2 are solution of the algebraic equation
Dλ2−cλ+1=0. |
If λ1>λ2, then the general solution of (8.17) can be written as
w(x)=k1w1(x)+k2w2(x), x∈R, |
where k1 and k2 are real constants. We can choose k1<0 and k2>0 such that w(x) has an only zero x0 and w(x)<0 for x<x0, w(x)>0 for x>x0. In addition, w(x) behaves as vc(x) if c>2√D: so that, we can have the estimation w(x)≤P(x,0)≤vc(x) for the initial condition.
If c=2√D, then λ1=λ2. Then the qualitative behavior of the solutions of Eq (8.17) as x→∞ is determined by
w(x)=w1(x)(k1+k2x), | (8.19) |
with k1 and k2 real constants. We can choose k1<0 and k2>0 such that w(x) has an only zero x0 and w(x)<0 for x<x0, w(x)>0 for x>x0. This result can be achieved choosing both the constants k1 and k2 positive. In addition, w(x) behaves as vc(x) if c=2√D: so that, we can have the estimation w(x)≤P(x,0)≤vc(x) for the initial condition.
Lemma 6. Let z1(x)=max(0,wc(x)), and z2(x)=vc(x). If
z1(x)<P0(x)<z2(x), x∈R |
then the solution of the Cauchy problem for Eq (8.4) with the initial condition P0(x) satisfies the estimate
z1(x)<P(x,t)<z2(x), x∈R |
for all t>0.
Theorem 7. There exist positive GTW solutions of Eq (8.3) for all c≥2√D. Positive GTW converging to zero as x→∞ do not exist for c<2√D.
Proof. The existence of GTWs for all c≥2√D follows from the previous lemma. Indeed, consider solution of Eq (8.3) in the form P(x,t)=P(x−ct,t). Then
∂tP=D∇2P+c∇P+P(1−P)(1−α1P−α2∫∞−∞ϕ(x−y)P(y,t)dy). | (8.20) |
It follows from Lemma 3 that there exists an ω-limit solution Pc(x,t) of Eq (8.20) such that
z1(x)≤Pc(x,t)≤z2(x), | (8.21) |
for all t∈R. In order to construct this solution, consider the solution P(x,t) of Eq (8.20) with an initial condition P0(x) which satisfies the inequality z1(x)≤P0(x)≤z2(x) for all x. Let tn→∞ as n→∞. Consider next solutions Pn(x,t) with the initial conditions P0n=P(x,tn). Obviously, each of them is defined for t>−tn. A locally convergent subsequence of the sequence of functions Pn(x,t) is a solution of Eq (8.20) defined for all t∈R. It satisfies inequality (8.21). It can be easily verified that it is a GTW with the speed c.
Suppose now that there exists a positive GTW Pc(x,t), converging to 0 as x→∞, with a speed c<2√D. Then Pc(x−ct,t) satisfies Eq (8.20). Let us take c<c0<2√D and consider the equation
DP″+c0P′+P(1−P)=0. | (8.22) |
It has a solution P0(x) that is non-monotone and unstable [41]. Moreover, when x tends to infinity, then P0(x)∼exp(−c0x/2)sin(ax), where a=√D|c20/4−1|. Therefore, equation
∂tP=D∇2P+c∇P+P(1−P), | (8.23) |
has a solution P∗(x,t)=εP0(x−(c0−c)t), where ε is a positive constant. Let x=N1 and x=N2 be two consecutive zeros of the function P0(x) such that P0(x) is positive between them. Then P∗(x,t) is a solution of the boundary value problem for Eq (8.23) in the domain
N1+(c0−c)t≤x≤N2+(c0−c)t |
with the zero boundary conditions. For ε small enough, similarly to (8.9) we can obtain the inequality
P∗(x,t)<Pc(x−ct,t),N1+(c0−c)t≤x≤N2+(c0−c)t. | (8.24) |
If ma(t) is the maximal solution of the equation
Pc(x,t)=a,0<a<maxN1+(c0−c)t≤x≤N2+(c0−c)tP∗(x,t), | (8.25) |
then
¯limt→∞ma(t)t≥c0. | (8.26) |
Since c0>c and Pc(x,t) converges to zero as x→∞, then the last inequality contradicts the assumption that Pc(x,t) is a GTW with the speed c.
This contradiction proves the theorem.
In Figure 5 we present the results of numerical simulations of Eq (2.10) in one space dimensions. The kernel is the function ϕ4(x). If the support of ϕ is sufficiently small, then there is a classical traveling wave propagating with a constant speed. Figure 5(a) shows the solution P(x,t) of Eq (2.10) with the initial condition which has a bounded support. The solution represents two waves propagating in the opposite directions. It is interesting to note that the wave is not monotone with respect to x. If we increase the support of the function ϕ, then the homogeneous in space stationary solution P2 loses its stability and a periodic in space structure appears. In this case we observe propagation of a periodic wave (Figure 5(b)-(c)). Figure 5(d) shows the solution P(x,t) with the exponentially decaying initial conditions. Therefore, depending on the values of parameters equation (2.10) can have solutions of different types. For all other parameters fixed, usual traveling waves (with a constant speed and profile) are observed for sufficiently small values of h. Periodic traveling waves exist for sufficiently large h. Transition from simple to periodic waves occurs due to the essential spectrum crossing the imaginary axis. The stationary solution homogeneous in space P2 loses its stability resulting in the appearance of a stationary periodic solution. The traveling wave connects the constant value P0 for x=+∞ with this periodic solution for x⟶−∞ . The waves in (a)-(b)-(c) (in which the initial data has a bounded support) move at their minimal speed c=2√D=2 and this speed does not depends on h; while, the speed of the wave (d) is greater than the minimal speed c>2√D and depends on h. Though we consider in numerical simulations a finite interval, if it is sufficiently large, then the solution can approach the corresponding GTW.
Here we assume that the information is not only spatially and but also temporally non–local: the subjects take their decisions not only on the information on the current state of the system but also on the past states. In other words, we include the memory of the subjects concerning the past information about vaccine side-effects. We consider in this section the 1D unbounded domain, i.e., Ω=R.
These assumptions yield:
α(Mse(P))=α0+α1∫+∞0W(τ)P(x,t−τ)dτ+α2∫Ω∫+∞0ϕ(x−y)W(τ)P(y,t−τ)dτdy, | (9.1) |
with W(τ) a delaying kernel, i.e., a positive function such that ∫+∞0W(τ)dτ=1. Using scaling (2.9), we obtain:
∂tP=D∇2P+P(1−P)(1−α1∫+∞0W(τ)P(x,t−τ)dτ−α2∫Ω∫+∞0ϕ(x−y)W(τ)P(y,t−τ)dτdy), | (9.2) |
with t>0, x∈Ω.
Here we will focus on the so called acquisition–fading kernel (AFK) [46]:
W(τ)=bdd−b(e−bτ−e−dτ), | (9.3) |
with 0<b<d. This memory kernel, which is such that W(0)=0 (i.e., absence of information of the current state of the process), models the process of temporal acquisition of information (with a rapid timescale 1/d) followed by a fading of the memory (with a 'slow' timescale 1/b).
By applying the linear chain trick (see the Appendix) one gets the following equivalent system:
∂tP=D∇2P+P(1−P)(1−α1M−α2J(M)),∂tZ=b(P−Z),∂tM=d(Z−M). | (9.4) |
Model (9.4) is remindful of systems generating complex self-organized patterns in biochemistry and in population biology [22,47], since only one of the involved state densities is endowed of diffusion. The aim of this section is to investigate the possible bifurcation from the homogenous equilibria of (9.4) of such self-organized structures.
It is easy to verify that model (9.4) admits the following homogeneous in space stationary solutions
E0=(0,0,0),E1=(1,1,1), |
E2=(1α,1α,1α). |
Also here we will focus on E2. Considering the case where Ω is unbounded and linearizing (9.4) at E2 yields the following eigenvalue problem:
D∇2P−1α(1−1α)(α1M+α2J(M))=λP, | (9.5) |
b(P−Z)=λZ, | (9.6) |
d(Z−M)=λM, | (9.7) |
whose essential spectrum is given by:
−Dξ2˜P−1α(1−1α)(α1˜M+α2˜M˜ϕ(ξ))−λ˜P=0, | (9.8) |
˜M=bd(λ+b)(λ+d)˜P | (9.9) |
Thus, one obtains the following ξ-family of λ polynomials
q(λ,ξ)=λ3+a2(ξ)λ2+a1(ξ)λ+a0(ξ)=0, | (9.10) |
where
a2(ξ)=b+d+Dξ2>0, |
a1(ξ)=bd+Dξ2(b+d)>0, |
a0(ξ)=bdα2[Dξ2α2+(α−1)(α1+α2˜ϕ)]. |
Thus E2 is stable iff a0(ξ)>0 and
H1(ξ)=a2(ξ)a1(ξ)−a0(ξ)>0, |
that is:
H1(ξ)=1α2{(d+b)D2ξ4α2+(b+d)2Dξ2α2+bd[(b+d)α2−(α−1)(α1+α2˜ϕ)]}>0. | (9.11) |
We recall that a change of sign of H1 in non-spatial systems is associated to the onset of a Hopf bifurcation, a change of sign in a spatiotemporal context give rise to a Hopf-Turing bifurcation [48] and finally the only change of sign of a0(ξ) would give rise to a 'pure' Turing bifurcation [48,49].
In this context it is important to distinguish instabilities that genuinely involve the spatio–temporal model form those that would have been also found in the non–spatial model (NSM). As regards the NSM, we observe that
a0|D=0,˜ϕ=1=bdα(α−1)>0. |
Thus, for Routh-Hurwitz criterion to be satisfied by the NSM, it must be:
HNSM1=H1|D=0,˜ϕ=1=bdα[α(b+d−1)+1]>0. |
Thus:
ⅰ) if b+d>1−1/α, then HNSM1>0 and the equilibrium of the the NSM is LAS;
ⅱ) if b+d<1−1/α then HNSM1<0 and the equilibrium of the NSM is unstable via Hopf bifurcation.
The above result on HNSM1 actually implies that H1(ξ)>0. Indeed from (9.11), we have that H1(ξ) is composed by three addenda: (d+b)D2ξ4>0, (b+d)2Dξ2>0 and
bdα2[(b+d)α2−(α−1)(α1+α2˜ϕ)]= |
=bdα2[(b+d−1)α2+α−(α−1)α2(−1+˜ϕ)]= |
=bdα2(α−1)[αα−1(1+(b+d−1)α)+α2(1−˜ϕ)]>0. |
So no Turing-Hopf bifurcation can be generated if the equilibrium of the NSM is LAS.
Thus, apparently the only route to instability at E2 is via changes of sign of a0(ξ). However it is easy matter to verify that the condition a0(ξ)>0 is nothing else that the LAS condition of the intermediate equilibrium P2=1/α defined in (5.2) of our model (2.10) without memory.
Summarizing, we may state that the introduction of temporal non-locality via the AFK does not make E2 unstable unless:
ⅰ) b+d≥1−1/α, i.e., the NSM is unstabilized at E2 by the memory effect;
ⅱ) there exist ξ such that a0(ξ)<0, i.e., the original model without memory is unstable.
The above analysis, with simple changes, can also be repeated in the case of local and of global information.
In the Figure 6 we give an illustration of the stability character of the state of equilibrium E2 for the system (9.4), with ϕ(x)=ϕ4(x), choosing a small perturbation as initial data. In Figure 6(a) we see that the solutions evolve in time to reach the stable equilibrium E2, while in Figure 6(b) the stability is lost and we see the convergence to a periodic spatial structure. This depends on the diffusion coefficient D, the ratio ρ=α2/α1 and the parameter h.
Even if the presence of GTW has not been proved analytically for the system (9.4), in Figure 7 we provide an example of possible GTW in the case of system (9.4). The kernel ϕ(x) is the function ϕ4(x) and the initial data decays exponentially. We focus our attention on delay parameters b and d. If b and d are small enough, then spatial periodic structures appear, while increasing the value of b and d, this kind of structure disappear.
Now, we describe wave solutions for Eq (9.4), setting the Dirac delta δ(x) as kernel, i.e., solutions of the following system
∂tP=D∇2P+P(1−P)(1−αM),∂tZ=b(P−Z),∂tM=d(Z−M). | (9.12) |
Let E=(P,Z,M)T be the variable vector of system (9.12) such that
limx→±∞E(x)=E± | (9.13) |
with E− unstable and E+ stable equilibrium state. After substitution of the wave variable z=x−ct in model (9.12), we obtain the following system of ordinary differential equations:
Dp″+cp′+p(1−p)(1−αm)=0,cω′+b(p−ω)=0,cm′+d(ω−m)=0, | (9.14) |
where P(x−ct)=p(z), Z(x−ct)=ω(z) and M(x−ct)=m(z). When α<1, recall that E0 is unstable and E1 is stable with respect to the system (9.4). Thus, the following result holds [44]:
Theorem 8. If 0<α<1, there exists a traveling wave solution of velocity c ∀ c∈[c(1),+∞[, connecting equilibrium E1 and equilibrium E0, i.e., a vector function E(x−ct), solution of system (9.14) on the real line ]−∞,+∞[ and satisfying Eq (9.13) with E−=E1 and E+=E0.
If α>1, then equilibrium E1 becomes unstable while equilibrium E2 is stable with respect to model (9.4). Therefore, it can affirmed that [44]:
Theorem 9. If α>1, there exists a traveling wave solution of velocity c ∀ c∈[c(1),+∞[, connecting equilibrium E2 and equilibrium E0, i.e., a vector function E(x−ct), solution of system (9.14) on the real line ]−∞,+∞[ and satisfying Eq (9.13) with E−=E2 and E+=E0; moreover, traveling wave solution between E1 to E0 cannot exist.
In Figure 8, we show traveling wave solution for system (9.4) with minimal speed c=c(1)=2√D, connecting the equilibrium E2 at −∞ and equilibrium E0 at +∞.
A relevant task of a Public Health System (PHS) is that of investing to continuously sustain the vaccine uptake in the population. In the scenario we considered, namely prevention of an infection which has been eliminated, this is particularly important. Indeed, in such a case parents are scarcely motivated to vaccinate due to the low perceived risk, which is mirrored by the fact that ϑ(Mi)=ϑ0.
The action of the PHS in enacting vaccine awareness campaigns was modelled in [14] by amending the basic imitation dynamics of strategies. Briefly, they assumed that the action by the PHS allows a steadily positive flux γA switching per time unit from the Non-vaccinator to the Vaccinator strategy.
Extending the above ideas [5,14,15,31] to the present spatiotemporal setting yields the model:
∂tP=D∇2P+ϑ(Mi)AP−α(Mse)AP+γA,∂tA=D∇2A−ϑ(Mi)AP+α(Mse)AP−γA, | (10.1) |
where γ is a positive constant.
Keeping expressions (2.3) and (2.4), using A=1−P and scaling as (2.9), from (10.1) we obtain:
∂tP=D∇2P+P(1−P)(1−α1P−α2J(P))+γ(1−P), | (10.2) |
with t>0, x∈Ω and Neumann condition (2.11).
A first effect of public action can immediately be seen: the NVE P0=0 is no more an equilibrium point.
Moreover, system (10.2) has two spatially homogenous equilibria, namely the PVE P1=1 and the equilibrium Pγ∈(1/α,1) where Pγ is the solution of:
γ=αP2−P, |
i.e.,
Pγ=1+√1+4αγ2α. | (10.3) |
It is easy to verify that:
ⅰ) if 0<γ<(α−1) then Pγ∈(1/α,1); i.e., Pγ is epidemiologically meaningful;
ⅱ) it is d/dγPγ>0;
ⅲ) it is Pγ=P2=1/α, if γ=0. The previous results show that the new behavioral equilibrium Pγ, which replaces the 'old' equilibrium P2, allows a higher vaccine uptake than P2 thanks to the univocal effect of public intervention.
Before investigating the effects of the awareness intervention by the PHS on the stability of the equilibria of (10.2) (and its dependence on the distinct hypotheses on the spatial information kernel), here we illustrate, by means of a simulation, how a Public Health System intervention to favour vaccination can impact on the behavior of the system. We assume that subjects make their decisions by taking into the account their memory. In particular we choose the scenario depicted in the in the panel (a) of Figure 7 a GTW whose features are deeply influenced by the temporal non–locality. We assume that initially there is no public health intervention, which starts at about two third of the simulation interval and grows up to a maximal value γMax:
γ(t)=γMax50Hev(t−100). | (11.1) |
Thus, we simulate the equation:
∂tP=D∇2P+P(1−P)(1−α1∫+∞0W(τ)P(x,t−τ)dτ−α2∫Ω∫+∞0ϕ(x−y)W(τ)P(y,t−τ)dτdy)+γ(t)(1−P), | (11.2) |
where W(τ) is the delay function defined in (9.3) and γ(t) the function defined in (11.1). Figures 9 and 10 (view from the top) show that initially the GTW pattern coexists with a rapidly increasing uniform pattern. This pattern relatively soon destroys the GTW and, any case, it immediately increases the minimum value attained by the GTW.
Under purely local information (i.e., ϕ(x)=δ(x)), Eq (10.2) becomes:
∂tP=∇2P+P(1−P)(1−αP)+γ(1−P). | (11.3) |
Proceeding as in the case without vaccine awareness campaign, the following global stability results hold:
Theorem 10. If α>1 and γ∈(0,α−1) then Pγ is globally stable (and P1 is unstable).
If α>1 and γ≥α−1 then P1 is globally stable.
In the trivial case where 0≤α≤1 then P1 is globally stable independently of γ≥0.
The previous results straightforwardly extend to the present spatially structured case the findings in [14], showing that the behavioral equilibrium Pγ is always GAS whenever it exists, while when it disappears the PVE inherits its global stability. As for possible heteroclinic connections between Pγ and P1, introducing the traveling variable z=x−ct, the following negative result is established:
Theorem 11. There are no monotone decreasing traveling wave solutions for Eq (11.3) connecting equilibrium P1 at equilibrium Pγ with positive speed.
As noted above, assuming that L≫1, we have the following equation
∂tP=D∇2P+P(1−P)(1−α1P−α2∫∞−∞ϕ(x−y)P(y,t)dy)+γ(1−P) | (11.4) |
with x∈Ω=R, t>0 and Neumann condition (2.11).
The associated linearized equation at a generic homogeneous steady state P∗ reads
∂tw=D∇2w+[(1−2P∗)(1−αP∗)−α1P∗(1−P∗)−γ]w−α2P∗(1−P∗)∫∞−∞ϕ(x−y)w(y,t)dy | (11.5) |
and the related eigenvalue problem has the form:
D∇2W+[(1−2P∗)(1−αP∗)−α1P∗(1−P∗)−γ]W−α2P∗(1−P∗)∫∞−∞ϕ(x−y)W(y)dy=λW. | (11.6) |
If P∗=Pγ, then we obtain the following eigenvalue
ν(ξ)=−Dξ2+(1−2Pγ)(1−αPγ)−α1Pγ(1−Pγ)−γ−α2Pγ(1−Pγ)˜ϕ(ξ). | (11.7) |
To determine the sign of
χγ=(1−2Pγ)(1−αPγ)−γ, |
it is useful to take into the account that
αP2γ=γ+Pγ, |
yielding
χγ=1−αPγ+γ, |
i.e.,
χγ=1+2γ−√1+4αγ2. |
It is easy to show that if γ<α−1 then χ<0. Thus, eigenvalues (11.7) become
ν(ξ)=−Dξ2+χγ−Pγ(1−Pγ)(α1+α2˜ϕ(ξ)). | (11.8) |
Therefore, we have demonstrated the following results:
ⅰ) since χγ<0, for both ϕ(x)=ϕ1(x)=Cge−ax2 and ϕ(x)=ϕ2(x)=Cee−a|x| it is
ν(ξ)<0, |
so that Pγ is LAS;
ⅱ) for ϕ(ξ)=ϕ3(x)=CN(1−(|x|/N)2)Hev(N−|x|) and ϕ(ξ)=ϕ4(x)=(1/2h)Hev(h−|x|) the equilibrium Pγ can be unstable.
Moreover, if P∗=P1=1, consider the eigenvalue problem (11.6), then we obtain
ν(ξ)=−Dξ2+α−1−γ. |
If γ>α−1, then ν(ξ) is negative for any ξ and equilibrium P1 is LAS.
Assuming that the set Ω is bounded, we obtain the following equation from (10.2):
∂tP=D∇2P+P(1−P)(1−α1P−α2∫Ωϕ(x−y)P(y,t)dy)+γ(1−P) | (11.9) |
and Neumann conditions (2.11).
We will consider here Ω=[−L,L].
The eigenvalues problem associated at the linearization about a generic steady state P∗ is given by
DW′′+[(1−2P∗)(1−αP∗)−α1P∗(1−P∗)−γ]W−α2P∗(1−P∗)∫L−Lϕ(x−y)W(y)dy=λW. | (11.10) |
Since for the equilibrium P1=1 the nonlocal term disappears, the ensuing local stability analisys is straightforward and it is omitted. Thus we will only focus on the equilibrium Pγ.
Assuming that the kernel ϕ(x) satisfy conditions (6.21) and (2.7) i.e., function ϕ is periodic with period 2L, even and normalized, if P∗=Pγ, we look for solution of the following eigenvalue problem
DW′′+[χγ−α1Pγ(1−Pγ)]W−α2Pγ(1−Pγ)∫L−Lϕ(x−y)W(y)dy=λW, |
in the form
w(x)=cos(ξmx), ξm=mπL, m=0,1,2,... |
Then the boundary conditions are satisfied. Taking into account that the function ϕ is periodic and even, the m–th eigenvalue reads as follows:
νm=−Dξ2m+[χγ−α1P2(1−P2)]−α2Pγ(1−Pγ)ϕm, | (11.11) |
where ϕm is defined in (6.23). We note that νm is negative for m=0 and for m sufficiently large.
If ϕm is a positive function, then the corresponding eigenvalue νm is also positive. If the kernel ϕ has a bounded support, then, depending on the parameters, the corresponding eigenvalues can be negative and stability is lost.
In case of global information,
∂tP=D∇2P+P(1−P)(1−α1P−α21μ(Ω)∫ΩP(y,t)dy)+γ(1−P) | (11.12) |
with x∈Ω=R, t>0 and Neumann condition (2.11), it is trivial to show that Pγ is LAS. Indeed, setting W(x)=cos(ξmx) as eigenfunction of the problem:
DW′′+[χγ−α1Pγ(1−Pγ)]W−α2Pγ(1−Pγ)1μ(Ω)∫ΩW(y)dy=λW, |
we get the following eigenvalues:
˜ν0=χγ−αPγ(1−Pγ)<0, |
˜νm=−Dξ2m+χγ−α1Pγ(1−Pγ)<0. |
In this section we aim at highlighting similarities and key differences between our models (2.1) and (10.1) and the Theory of Innovation Diffusion (TID) by Mahajan and others [34,32]. For the sake of the notation simplicity we mainly consider non-spatial models.
The TID models focus on the dynamics of the adoption of innovation. Defining Y(t) as the fraction of adopters of the innovation at time t, and U(t)=1−Y(t) the fraction of 'non–adopters' the basic family of models is the following:
Y′=g(t)(1−Y), | (12.1) |
where g(t)>0, which was initially interpreted as an 'hazard of adoption' [36], has later been compared by Capasso and Zonno to the force of infection of epidemic models [33], with whom it has striking analogies. Thus, we will call it 'Force of Innovation Adoption' (FIA).
The positivity of the FIA g(t) has an important consequence for our comparison: all specific models belonging to the family (12.1) are such that Y(t)→1−, i.e., there is a unique equilibrium point, which can be termed 'all adopters'. As a consequence, assuming Y=P, all possible analogies with our family of models is limited to the unrealistic cases where the 'all vaccinators' equilibrium is GAS. For example, the family of models (12.1) for g(t)=γ corresponds to our model in the trivial case where there is no contagion of ideas. The positivity of g(t) and its interpretation as a 'force' of infection also stress the key difference between the family of models we investigated here and the family of model (12.1): in the scenario we investigated there is a double contagion of ideas, i.e., a double flux: ⅰ) from A to P; ⅱ) from P to A. On the contrary, the family (12.1) implies an uni–directional contagion of ideas. This is a direct consequence of the deeply different nature of the underlying 'social processes'. However, it is of interest to investigate formal analogies between the two theories. The specific instances of imitation game-like equations of TID [32,34] are obtained phenomenologically by assuming that g(t) is an analytical function of Y [34]:
g(t)=a0+a1Y(t)++∞∑j=2ajYj(t) | (12.2) |
where ak≥0 can also be function of time [33,35]. For example, assuming that the non–linear terms are null, g(t)=a0+a1Y(t), yields the most popular popular model of the TID: the Bass model [34,36], which reads as follows:
Y′=a1Y(1−Y)+a0(1−Y). | (12.3) |
The non–negativity of the coefficients ak would preclude formal analogies with our models. However, maintaining the constraint of positivity of the FIA g(t)>0 but relaxing for k≥2 the hypothesis that ak≥0 yields the following g(t) that allows a formal analogy with some models of the family of models considered in this work:
g(t)=Y(t)(θ0−α(Y(t))) | (12.4) |
with, however, the following sharp constraint (α(Y) is non–decreasing):
θ0−α(1)≥0 |
that again leads to the global stability of the 'all adopters' ('all vaccinators') model.
The important case of linear–affine α(y) can be expressed within the frame of the TDI family of models as follows:
g(t)=γ+(θ0−α0)Y−α1Y2 | (12.5) |
Note that this generalized framework is such that: ⅰ) can be framed with the epidemiological theory by Capasso where forces of infection can also be non–monotone [6], which can also be applied to diffusion of information [33]; ⅱ) it remains a formal model of a uni-dimensional flow.
Namely, a FIA g(t) of the type (12.4) can be read in terms of TID as an initially beneficial effect of the product qualities, possibly followed, for larger Y, by a partial mitigation of the 'enthusiasm' for the product due to the spread of information of its possible (real of presumed) defects. Thus, a reduced FIA is observed.
As far as the space is concerned, let us first consider the Fisher-Kolgomorov–like model (3.1), for which the equilibrium 'all vaccinators' is GAS. If we further assume α0=0 then we have a unidirectional flux from the group 'non-vaccinators' to the group 'vaccinators'. In such a case our model (3.1) is equivalent to the Mahajan spatiotemporal model of Innovation Diffusion [34].
More interestingly, in [35,33] Capasso proposed, in the framework of an SIR-like diffusion of innovation model, a family of non-local models of the FIA. This family of models is non-locally depending on the adopters, again in the context of a uni-directional flux. In our case, instead, we have two fluxes and it is the force of infections from the adopters towards non–adopters that is non–local.
The role played by the spatial structure of information used by vaccination decision makers is a main topic of behavioral epidemiology. In this work we have investigated, within a spatial framework based on classical diffusion, the effects of three different structures of information ((ⅰ) purely 'local' information, 'local' plus a 'global', country-wide, average information, (ⅲ) a mix of local and non-local information) on the dynamics of vaccine uptake in absence of the infection. As a consequence, given the background of low incentive to immunization, the dynamics of VAEs emerge as the key determinants of vaccination decisions and collective coverage. This possibly represents a main case of the current public health landscape in modern industrialized countries, where a number of vaccine preventable infectious disease were successfully eliminated 'locally' (think e.g., to polio which was locally eliminated long ago in most Europe), but infection re-emergence must be prevented by means of large vaccine coverage.
We focused our analyses on the pattern and properties - namely stability, bifurcation, existence of classical and generalized traveling waves, effects of temporal delays, and the effects of awareness campaigns by the Public Health System - of the key space-homogeneous equilibrium solutions, that we termed the 'behavioral equilibria'.
Our main results were as follows.
As regards the stability properties of the behavioral equilibrium, these show a nice interplay between the form of the spatial information kernel and the nature of the spatial domain (bounded vs unbounded). Under “purely local information” the BE is always LAS (and GAS) whenever it exists. Under 'local and global' information the BE is always LAS whenever it exists. However, convergence is slower than in the case of purely local information. Under 'mixed' information results are more articulated. For unbounded domains stability will prevails independently of the shape of the spatial information kernel under large values of the diffusion coefficients. In particular kernels that are strictly decreasing in the distance from the local position are always stabilising independently of the spatial kernel. However, bounded-support kernels (e.g., strictly positive up to some threshold distance and zero thereafter) can yield instability at low levels of the diffusion coefficient when the strength of non-local information tend to prevail on local one.
Interestingly, the instability caused by the presence of non–local information can generate generalized traveling waves characterised by more or less pronounced oscillations as well as other not static spatial patterns. The onset of these traveling waves depends on the interplay between behavioral parameters and the structure of the spatial kernel. This means that the fraction of individuals favourable to vaccination can show oscillations. Then we investigated the case where agents also use past - and not only current - information about VAEs in forming their perceptions of risk. We showed that in the realistic case where this temporal non-locality is modelled by the acquisition–fading kernel no further specific instability can arise w.r.t. the non–spatial scenario.
However, the presence of information memories can remarkably impact on the 'shape' of the generalised traveling waves. Indeed, for certain combination of the characteristic times of the memory kernel, the spatial oscillations internal to the propagating front are so that, at each time, there are large zones where the proportion favorable to vaccination is close to zero. This can compromise the herd immunity of the population.
Remarkably, instabilities and emergence of generalised traveling waves are cleared out soon after the start of vaccine awareness campaigns enacted by the public health system, in line with the intuitions supplied in [14].
We briefly mention that the above illustrated mathematical behaviors could also be read, from theoretical physics viewpoint, as phase changes and phase transitions (PTs), an increasingly important concept in ME [50,51]. Indeed, on the one hand in general the kinetic of PTs is most often characterized by traveling waves [52,53,54]. On the other hand, all imitation games (as our model) where individual switch between two mutually exclusive strategies have some analogy with the well-known Ising model [55]. In our case, one can 'read' P(x,t)≈1 as a phase rich in pro–vaccine subjects (an 'ordered' phase) and P(x,t)≈0 as a phase poor in pro–vaccine subjects (a 'disordered phase').
Finally, we highlighted fundamental differences and some analogies between the phenomena in study and those investigated by the Theory of Innovation Diffusion. Basically in classical TID models, there is a uni–directional contagion of ideas between 'non-adopters' and 'adopters'. As a consequence all classical models of TID predicts global stability of the full adoption of the innovation. This correspond to the pathological case where P=1 is Globally Asymptotically Stable.
This work obviously has a number of limitations.
A key limitation of this study is the hypothesis that at the macroscale the agents' mobility can be approximated by classical diffusion i.e., by random walk around geographic space. This is a coarse approximation, thus our model is essentially a benchmark for providing clear-cut baseline results. They will have to be validated against more robust hypotheses. Indeed, real patterns of mobility of human individuals are complex [56] and can introduce remarkable implications for infection patterns, such as unexpected phase transitions [57]. A promising recent route is to model human mobility as a superdiffusive process [58,59,60,61]. Superdiffusion allows a potentially more realistic and flexible representation of human spatial mobility while, at the same time, remaining analytically tractable. Thus superdiffusion is a natural candidate for future extensions of the present work. In particular, Brockmann and Hufnagel [62] have modelled a double chemical reaction leading to equations similar to a classical imitation game in case of superdiffusive mobility of molecules and we plan to follow their approach in a follow-up work.
A second limitation of this study lies in the specific case-study, namely a vaccination scenario in the absence of the infection. As mentioned in the introduction, this case is a central. However, it is equally important the scenario where the disease is continuously re–introduced. This, for example, is the case of measles, which has caused sizable epidemics in recent years [8,9,63]. Further forthcoming work will therefore be devoted to the study of the spatio-temporal interplay between the vaccine opinion dynamics and infection spread.
We thank the four anonymous referees that with their important comments helped us to significantly improve this manuscript. The publication has been prepared with the support of the RUDN University Program 5-100, and the French-Russian project PRC2307. The publication was also supported by LYSM-INDAM and GNFM-INdAM.
The authors declare there is no conflict of interest.
Here, it is shown that the integro-differential information model (9.2) is equivalent to the differential system (9.4) with the delaying kernel W(τ) defined in (9.3). First, consider the function
Z(x,t)=be−bt∫t−∞ebτP(x,τ)dτ, | (A-1) |
which is clearly the solution of the following linear differential equation:
∂tZ+bZ=bP. | (A-2) |
Given W(τ) defined in (9.3), consider
M(x,t)=∫t−∞W(t−τ)P(x,τ)dτ, | (A-3) |
i.e.,
M(x,t)=bdd−b(e−bt∫t−∞ebτP(x,τ)dτ−e−dt∫t−∞edτP(x,τ)dτ). | (A-4) |
Therefore,
∂tM+dM=dbe−bt∫t−∞ebτP(x,τ)dτ, | (A-5) |
i.e.,
∂tM+dM=dZ. | (A-6) |
[1] | N. T. J. Bailey, The mathematical theory of infectious diseases and its applications (2nd edition), 2nd edition, Charles Griffin & Company Ltd, 1975. |
[2] | V. Capasso and V. Capasso, Mathematical structures of epidemic systems, Springer, 1993. |
[3] | C. Metcalf, E. Jessica, W. J. Edmunds, et al., Six challenges in modelling for public health policy, Epidemics, 10 (2015), 93-96. |
[4] | P. Manfredi and A. d'Onofrio, Modeling the Interplay Between Human Behavior and the Spread of Infectious Diseases, Springer, 2013. |
[5] | Z. Wang, C. T. Bauch, S. Bhattacharyya, et al., Statistical physics of vaccination, Phys. Rep., 664 (2016), 1-113. |
[6] | V. Capasso and G. Serio, A generalization of the Kermack-McKendrick deterministic epidemic model, Math. Biosci., 42 (1978), 43-61. |
[7] | R. Casiday, T. Cresswell, D. Wilson, et al., A survey of UK parental attitudes to the MMR vaccine and trust in medical authority, Vaccine, 24 (2006), 177-184. |
[8] | E. Dubé, C. Laberge, M. Guay, et al., Vaccine hesitancy: an overview, Human Vaccin. Immunother., 9 (2013), 1763-1773. |
[9] |
V. A. A. Jansen, N. Stollenwerk, H. J. Jensen, et al., Measles outbreaks in a population with declining vaccine uptake, Science, 301 (2003), 804-804. doi: 10.1126/science.1086726
![]() |
[10] | S. B. Omer, D. A. Salmon, W. A. Orenstein, et al., Vaccine refusal, mandatory immunization, and the risks of vaccine-preventable diseases, N. Engl. J. Med., 360 (2009), 1981-1988. |
[11] | R. Löfstedt, Risk management in post-trust societies, Palgrave-McMillan, 2005. |
[12] | C. T. Bauch, Imitation dynamics predict vaccinating behaviour, Proc. R. Soc. Lond. B Biol. Sci., 272 (2005), 1669-1675. |
[13] | A. d'Onofrio, P. Manfredi and P. Poletti, The impact of vaccine side effects on the natural history of immunization programmes: an imitation-game approach, J. Theor. Biol., 273 (2011), 63-71. |
[14] | A. d'Onofrio, P. Manfredi and P. Poletti, The interplay of public intervention and private choices in determining the outcome of vaccination programmes, PLoS ONE, 7 (2012), e45653. |
[15] | B. Buonomo, G. Carbone and A. d'Onofrio, Effect of seasonality on the dynamics of an imitation- based vaccination model with public health intervention, Math. Biosci. Eng., 15 (2018), 299-321. |
[16] | D. G. Kendall, Mathematical models of the spread of infection, Math. Comput. Sci. Biol. Med. (1965), 213-225. |
[17] | N. T. J. Bailey, The simulation of stochastic epidemics in two dimensions, Proc. Fifth Berkeley Symp. Math. Statist. Prob., 4 (1967), 237-257. |
[18] | D. G. Aronson, The asymptotic speed of propagation of a simple epidemic, Nonlinear Diffusion, 14 (1977), 1-23. |
[19] | V. Capasso, Global solution for a diffusive nonlinear deterministic epidemic model, SIAM J. Appl. Math., 35 (1978), 274-284. |
[20] | F. Hoppensteadt, Mathematical Theories of Populations: Deomgraphics, Genetics, and Epidemics, Siam, 1975. |
[21] | H. Malchow, S. V. Petrovskii and E. Venturino, Spatiotemporal patterns in ecology and epidemiology: theory, models, and simulation, Chapman and Hall/CRC, 2007. |
[22] | J. D. Murray, Mathematical biology II. Spatial models and biomedical applications, SpringerVerlag New York Incorporated New York, 2001. |
[23] | S. Ruan and J. Wu, Modeling spatial spread of communicable diseases involving animal hosts, Spatial Ecol., (2009), 293-316. |
[24] | J. V. Noble, Geographic and temporal development of plagues, Nature, 250 (1974), 726. |
[25] | A. Ducrot and T. Giletti, Convergence to a pulsating travelling wave for an epidemic reactiondiffusion system with non-diffusive susceptible population, J. Math. Biol., 69 (2014), 533-552. |
[26] | P. Magal, G. F. Webb and Y. Wu, On the Basic Reproduction Number of Reaction-Diffusion Epidemic Models, SIAM J. Appl. Math., 79 (2019), 284-304. |
[27] | P. Magal, G. F. Webb and Y. Wu, Spatial spread of epidemic diseases in geographical settings: seasonal influenza epidemics in Puerto Rico, arXiv preprint arXiv:1801.01856 (2018). |
[28] | W. E. Fitzgibbon, J. J. Morgan, G. F. Webb, et al., A vector-host epidemic model with spatial structure and age of infection, Nonlinear Anal-Real., 41 (2018), 692-705. |
[29] | L. Zhao, Z. C. Wang and S. Ruan, Traveling wave solutions in a two-group SIR epidemic model with constant recruitment, J. Math. Biol., 77 (2018), 1871-1915. |
[30] | S. Ruan, Spatial-temporal dynamics in nonlocal epidemiological models, Math. Life Sci. Med., (2007), 97-122. |
[31] | B. Buonomo, P. Manfredi and A. d'Onofrio, Optimal time-profiles of public health intervention to shape voluntary vaccination for childhood diseases, J. Math. Biol., 78 (2019), 1089-1113. |
[32] | R. Peres, E. Muller and V. Mahajan, Innovation diffusion and new product growth models: A critical review and research directions, Int. J. Res. Market., 27 (2010), 91-106. |
[33] | V. Capasso and M. Zonno, Mathematical Models for the Diffusion of Innovations, Proc. Fourth Eur. Conference Math. Industry, (1991), 225-233. |
[34] | V. Mahajan and R. A. Peterson, Models for innovation diffusion, Sage Publications Inc, 1985. |
[35] | V. Capasso, A. Di Liddo and L. Maddalena, A nonlinear model for the geographical spread of innovations, Dyn. Syst Appl., 3 (1994), 207-220. |
[36] | F. M. Bass, A new product growth for model consumer durables, Manag. Sci., 15 (1969), 215-227. |
[37] | S. Funk, E. Gilad, C. Watkins, et al., The spread of awareness and its impact on epidemic outbreaks, Proc. Natl. Acad. Sci., 106 (2009), 6872-6877. |
[38] | E. Frey, Evolutionary game theory: Theoretical concepts and applications to microbial communities, Physica A, 389 (2010), 4265-4298. |
[39] | R. A. Fisher, The wave of advance of advantageous genes, Annals Eugenics, 7 (1937), 355-369. |
[40] | V. Volpert, Elliptic Partial Differential Equations: Volume 2: Reaction-Diffusion Equations, 104, Springer, 2014. |
[41] | A. N. Kolmogorov, I. N. Petrovsky and N. S. Piskunov, Étude de l'équation de la diffusion avec croissance de la quantité de matière et son application à un problème biologique, Bull. Univ. Moskow, Ser. Internat., Sec. A, 1 (1937), 1-25. |
[42] | A. D. Polyanin and V. F. Zaitsev, Handbook of ordinary differential equations: exact solutions, methods, and problems, Chapman and Hall/CRC, 2017. |
[43] | J. D. Murray, Mathematical biology I: an introduction, Springer New York, 2002. |
[44] | A. I. Volpert, V. A. Volpert and V. A. Volpert, Traveling wave solutions of parabolic systems, 140 American Mathematical Soc., 1994. |
[45] | S. Vakulenko and V. Volpert, Generalized travelling waves for perturbed monotone reactiondiffusion systems, Nonlinear Analysis TMA, 6 (2001), 757-776. |
[46] | A. d'Onofrio, P. Manfredi and E. Salinelli, Vaccinating behaviour and the dynamics of vaccine preventable infections, Modeling the Interplay Between Human Behavior and the Spread of Infectious Diseases (2013), 267-287. |
[47] | M. Mincheva and M. R. Roussel, Turing-Hopf instability in biochemical reaction networks arising from pairs of subnetworks, Math. Biosci., 240 (2012), 1-11. |
[48] | B. Ermentrout and M. Lewis, Pattern formation in systems with one spatially distributed species, Bull. Math. Biol., 59 (1997), 533-549. |
[49] | A. M. Turing, The chemical basis of morphogenesis, Phil. Trans. R. Soc. Lond. B, 237 (1952), 37-72. |
[50] | N. Stollenwerk and V. Jansen, Population Biology and Criticality: From critical birth-death processes to self-organized criticality in mutation pathogen systems, World Scientific, 2011. |
[51] | N. Stollenwerk, S. van Noort, J. Martins, et al., A spatially stochastic epidemic model with partial immunization shows in mean field approximation the reinfection threshold, J. Biol. Dyn., 4 (2010), 634-649. |
[52] | A. M. Albano, N. B. Abraham, D. E. Chyba, et al., Bifurcations, propagating solutions, and phase transitions in a nonlinear chemical reaction with diffusion, Am. J. Phys., 52 (1984), 161-167. |
[53] | J. P. Eckmann and T. Gallay, Front solutions for the Ginzburg-Landau equation, Commun. Math. Phys., 152 (1993), 221-248. |
[54] | B. J. Matkowsky and V. A. Volpert, Stability of plane wave solutions of complex Ginzburg-Landau equations, Quart. Appl. Math., 51 (1993), 265-281. |
[55] | L. E. Reichl, A modern course in statistical physics, Wiley-VCH, 2016. |
[56] | M. C. Gonzalez, C. A. Hidalgo and A. L. Barabasi, Understanding individual human mobility patterns, Nature, 453 (2008), 779. |
[57] | D. Balcan and A. Vespignani, Phase transitions in contagion processes mediated by recurrent mobility patterns, Nature Phys., 7 (2011), 581. |
[58] | U. Skwara, J. Martins, P. Ghaffari, et al., Fractional calculus and superdiffusion in epidemiology: shift of critical thresholds, Proceedings of the 12th International Conference on Computational and Mathematical Methods in Science and Engineering, La Manga (2012). |
[59] | J. P. Boto and N. Stollenwerk, Fractional calculus and Levy flights: modelling spatial epidemic spreading, Computational and Mathematical Methods in Science and Engineering, (2009). |
[60] | U. Skwara, J. Martins, P. Ghaffari, et al., Applications of fractional calculus to epidemiological models, AIP Conf. Proc., 1479 (2012), 1339-1342. |
[61] | U. Skwara, L. Mateus, R. Filipe, et al., Superdiffusion and epidemiological spreading, Ecol. Complex., 36 (2018), 168-183. |
[62] | D. Brockmann and L. Hufnagel, Front propagation in reaction-superdiffusion dynamics: Taming Lévy flights with fluctuations, Phys. Rev. Lett., 98 (2009), 178301. |
[63] | D. R. Sinclair, J. J. Grefenstette, M. G. Krauland, et al., Forecasted Size of Measles Outbreaks Associated With Vaccination Exemptions for Schoolchildren, JAMA Network Open, 2 (2019), e199768-e199768. |
1. | M. Banerjee, M. Kuznetsov, O. Udovenko, V. Volpert, Nonlocal Reaction–Diffusion Equations in Biomedical Applications, 2022, 70, 0001-5342, 10.1007/s10441-022-09436-4 | |
2. | Rossella Della Marca, Nadia Loy, Marco Menale, Intransigent vs. volatile opinions in a kinetic epidemic model with imitation game dynamics, 2022, 1477-8599, 10.1093/imammb/dqac018 | |
3. | Bruno Buonomo, Alberto d’Onofrio, The Rionero’s special type of Lyapunov function and its application to a diffusive epidemic model with information, 2023, 0035-5038, 10.1007/s11587-023-00807-8 | |
4. | Rossella Della Marca, Marco Menale, Modelling the impact of opinion flexibility on the vaccination choices during epidemics, 2024, 0035-5038, 10.1007/s11587-023-00827-4 | |
5. | Tangjuan Li, Yanni Xiao, Jane Heffernan, Linking Spontaneous Behavioral Changes to Disease Transmission Dynamics: Behavior Change Includes Periodic Oscillation, 2024, 86, 0092-8240, 10.1007/s11538-024-01298-w | |
6. | V. Volpert, Mathematical model of repressive response to collective action and protest waves, 2024, 595, 00225193, 111970, 10.1016/j.jtbi.2024.111970 | |
7. | Félix Foutel-Rodier, Arthur Charpentier, Hélène Guérin, Optimal vaccination policy to prevent endemicity: a stochastic model, 2025, 90, 0303-6812, 10.1007/s00285-024-02171-z |