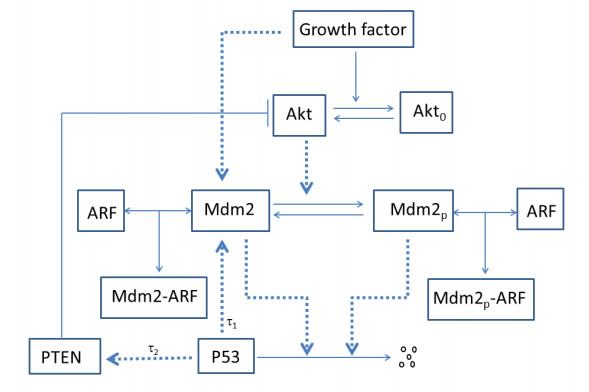
Citation: Zhong-Kai Guo, Hai-Feng Huo, Hong Xiang. Global dynamics of an age-structured malaria model with prevention[J]. Mathematical Biosciences and Engineering, 2019, 16(3): 1625-1653. doi: 10.3934/mbe.2019078
[1] | Juenu Yang, Fang Yan, Haihong Liu . Dynamic behavior of the p53-Mdm2 core module under the action of drug Nutlin and dual delays. Mathematical Biosciences and Engineering, 2021, 18(4): 3448-3468. doi: 10.3934/mbe.2021173 |
[2] | LanJiang Luo, Haihong Liu, Fang Yan . Dynamic behavior of P53-Mdm2-Wip1 gene regulatory network under the influence of time delay and noise. Mathematical Biosciences and Engineering, 2023, 20(2): 2321-2347. doi: 10.3934/mbe.2023109 |
[3] | Tingzhe Sun, Dan Mu . Multi-scale modeling identifies the role of p53-Gys2 negative feedback loop in cellular homeostasis. Mathematical Biosciences and Engineering, 2020, 17(4): 3260-3273. doi: 10.3934/mbe.2020186 |
[4] | Cicely K. Macnamara, Mark A. J. Chaplain . Spatio-temporal models of synthetic genetic oscillators. Mathematical Biosciences and Engineering, 2017, 14(1): 249-262. doi: 10.3934/mbe.2017016 |
[5] | Shunyi Li . Hopf bifurcation, stability switches and chaos in a prey-predator system with three stage structure and two time delays. Mathematical Biosciences and Engineering, 2019, 16(6): 6934-6961. doi: 10.3934/mbe.2019348 |
[6] | Qingwen Hu . A model of regulatory dynamics with threshold-type state-dependent delay. Mathematical Biosciences and Engineering, 2018, 15(4): 863-882. doi: 10.3934/mbe.2018039 |
[7] | Peng Feng, Menaka Navaratna . Modelling periodic oscillations during somitogenesis. Mathematical Biosciences and Engineering, 2007, 4(4): 661-673. doi: 10.3934/mbe.2007.4.661 |
[8] | Jie He, Zhenguo Bai . Global Hopf bifurcation of a cholera model with media coverage. Mathematical Biosciences and Engineering, 2023, 20(10): 18468-18490. doi: 10.3934/mbe.2023820 |
[9] | Yuan Ma, Yunxian Dai . Stability and Hopf bifurcation analysis of a fractional-order ring-hub structure neural network with delays under parameters delay feedback control. Mathematical Biosciences and Engineering, 2023, 20(11): 20093-20115. doi: 10.3934/mbe.2023890 |
[10] | Jinhu Xu, Yicang Zhou . Bifurcation analysis of HIV-1 infection model with cell-to-cell transmission and immune response delay. Mathematical Biosciences and Engineering, 2016, 13(2): 343-367. doi: 10.3934/mbe.2015006 |
Numerous studies have shown that P53 plays a key role in determining cell survival and death. More than 50 percent of cancer patients are detected with mutations in the P53 gene [1]. High levels of the mutant p53 protein were found in mouse tumors as early as 1983 [2]. In subsequent studies, abnormal expression of P53 protein was also found in a variety of human tumors [3,4,5]. In normal human cells, P53 is generally kept at a low level due to the downregulation of Mdm2 (murine double minute2) [6], which also helps cells avoid premature aging and apoptosis [7]. In general, with cells stimulated by hypoxia, DNA damage and impair of telomere function, P53 is rapidly produced and activated [8]. As a transcription factor, P53 prevents (or at least alleviates) damage caused by mutations, thereby regulating the expression of genes involved in various cellular functions, including cell cycle arrest, DNA damage repair, and apoptosis [9]. Previous investigation has proved that the specific function of P53 closely related to its level. When a weak stimulus is felt, P53 rapidly rises to a moderate level, thereby making cell cycle arrest and avoiding the inheritance of abnormal DNA replication to the next generation. Until the damage was eliminated, the expression level of P53 returned to the normal level [10,11,12]. Moreover, a large damage can cause the level of P53 rises to a higher state in order to induce apoptosis [13,14]. As we all know, P53 promotes transcription of its target gene Mdm2 (a P53-specific E3 ubiquitin ligase), which contributes to the degradation of P53 in return [15,16]. In fact, many cancer treatment programs are related to the network that is based on the P53-Mdm2 negative feedback loop [17,18]. Clinically, when the P53 protein is mutated, for example, mutations in the P53 protein make cells resistant to chemotherapy drugs [19,20]. However, when the P53 mutant protein is knocked out or interfered with, chemotherapy-induced apoptosis can be restored [21,22].
In recent years, many scholars dedicated to investigate the P53-Mdm2 feedback network. In 2000, the researchers theoretically modeled the P53 damped oscillations and also found that the negative feedback of P53-Mdm2 with time delay can led to the occurrence of P53 oscillation [23]. In 2005, Ma and his collaborators explored the mechanism of P53 pulse generation in theory at the IBM Watson Research Center in the United States [24]. In 2007, the Tysons team constructed a theoretical model of the P53 network response to DNA damage and explored the dynamics of P53 pulse-determining cell fate whose view is that the number of P53 pulses determining cell fate [25]. The more perfect result is that for mild lesions, a small amount of P53 pulses cause cell cycle arrest, and cells can return to normal after repair is completed. Whereas for severe damage, persistent P53 pulses kill the irreparable cell by activating downstream apoptosis program [25]. In 2009, Zhang et al. constructed a more comprehensive P53 network model and concluded that the fate of cells is closely related to the P53 pulse [26]. In 2011, the study of Zhang et al. shows that a sequential predominance of distinct feedback loops may elicit multiple-phase dynamical behaviors [27]. Only the research of Tingzhe Sun et al. shows that the kinetics of P53 under non-stressed conditions is triggered by an excitatory mechanism, and cells can only respond fully in the face of severe damage [28]. Although a large number of studies have been done, most of them were based on stress (DNAdamage, radiation, hypoxia, etc.). However, researches on non-stress situations are rarely involved. Moreover, as for these systems, the transcription, translation and integration into the polymer are usually seen as instant. In fact, the impact of the time delay of transcription and translation on the system in real biological processes is not negligible [29], especially multiple simultaneous time delays occur at the same time. Admittedly, there are several mathematical models of p53 that include time delays [7,28,30,31], which just illustrated that the objective existence of time delay is recognized. These studies have perfectly studied the dynamic mechanism of the p53 pulse from different aspects. But without exception, they did not specially focus on the role of time delay on the system. For example, Purvis et al. proposed a delayed computational model and identified a sequence of precisely timed drug additions that alter p53 pulses to instead produce a sustained p53 response that suggested that the drug is efficient and can directly influencing cellular fate decisions [7]. Even though there are three delays including Nutlin-3 activity delay, p53-induced expression of Mdm2 and Wip1 (Wild type p53-induced phosphatase 1) delays, the effect of time delay on the dynamic process is not received attention. Zhang et al. established a complex model without time delay to study the signal threshold to elicit cell death and the time delay between signal and response [30]. It should be noticed that the time delay there refers to the time required from the onset of external signal to the apoptosis triggered by the system. A mathematical model with two time delays that respectively represent the time consumed on the transcription of Mdm2 and wip1 activated by p53 is proposed by Sun et al. The model described the negative feedback loop Wip1 –∣ ATM (mutated in the disease ataxia telangiectasia) → P53 → Wip1 except to the core negative feedback loop formed by P53 and Mdm2 to study the excitability of p53 pulses and the effect of Wip on the p53 pulses [28]. Chong et al. developed a delayed mathematical model that incorporated the molecular interactions in the core regulation of p53 and the apoptosis initiation module involving Puma, Bcl2 and Bax and demonstrated how molecular interactions and stress signaling of the p53 network dictate cell fate decisions [31]. Although there are 10 time delays, their dynamic effects on the p53 pulse are also lacked. Here, we consider the following facts. P53 is regulated by ARF (ADP-ribosylation factor), which exerts an anti-cancer role by combining with Mdm2 (or Mdm2p), and is considered to be the main regulator of P53, so it is well known to promote the activation and stability of P53 [32]. Previous studies have shown that Growth factors in serum not only induce the transcriptional activation of Mdm2 [33], but the activation of Akt (protein kinase B) which phosphorylates Mdm2 promoting Mdm2p nuclear accumulation [34]. P53 trans-activates PTEN (gene of phosphate and tension homology deleted on chromsome ten), a tumor suppressor that dampens Akt activation by dephosphorylating PIP3 [34,35]. Especially, this paper places emphasis on the effect of positive feedback loop P53 → PTEN –∣ Akt → Mdm2 –∣ P53 and two time delays that respectively represent the p53-induced expression of Mdm2 and PTEN delays on the dynamic of the system. On the above interaction facts among biological molecules, we focus on the dynamics of P53 under non-stressed conditions based on the model which was given by Xinyu Tian, Bo Huang, Xiao-Peng Zhang et al. in 2017 [36].
The novelty of this paper is that most studies have considered the dynamic behavior of P53 under stress, while our study considered the non-stress state and studied the delay in detail. First of all, the effects of time delay on the P53 oscillation is analyzed in two different cases including there are one time delay and two time delays co-exist by using Hopf bifurcation theory. Previous studies have well demonstrated that negative feedback loop with time delay can produce oscillations and the network which includes coupled positive and negative feedback loops can also generate oscillations when negative feedback plays the main role [37,38]. It is quite important that we find the relative length of the double time delays is likely to control the dynamic properties of the system in the coupled positive and negative feedback loops. In addition, we consider the influences of several important chemical reaction rates that relating to P53 directly or indirectly.
This paper is organized as follows. In Section 2, we introduced the materials and method. In Section 3, we give numerical simulation results of the influences of time delays and key parameters on the P53 oscillation behaviors. Discussion is made in Section 4. Conclusion is drawn in Section 5. The theoretical derivations about the properties of Hopf bifurcations are presented in Supplementary.
As can be seen from Figure 1. Growth factors promote Mdm2 accumulation and Akt activation, while active Akt can promote the transfer of Mdm2 into the nucleus and phosphorylate Mdm2. The phosphorylated Mdm2 can be denoted by Mdm2p, which has a more pronounced ability to degrade P53 [34]. Mdm2p can be further phosphorylate to Mdm2pp. The latter ability of Mdm2pp to degrade P53 is very weak [39]. The increase of the total amount of Mdm2 in the nucleus indirectly leads to a decrease in the level of P53. In verse, P53 promotes the transcription and translation of Mdm2. Thus, a negative feedback loop of P53-Mdm2 is formed. On the other hand, PTEN can inhibit the activity of Akt and convert it to inactive Akt0 to reduce the conversion of Mdm2 to Mdm2p and thus indirectly promote the accumulation of P53. P53 can also promotes PTEN transcription. So the interations construct a positive feedback loop P53-PTEN-ATK-Mdm2. ARF binds to two forms of Mdm2 and inhibits the degradation of P53. Among these regulation process, it should be noticed that from DNA to protein, all transcription, transport and translation take time [40,41,42], which is ignored by the previous theoretical study [36]. We considered that both Mdm2 and PTEN could be upregulated by a means of a transcription activation from P53 [15,43,44]. Based on the related literatures mentioned above, we developed a delayed ODEs system to model the P53-PTEN-Akt-Mdm2 network, which is shown as (2.1).
Next, we mainly imply the stability and bifurcation theory to analyze the dynamic behavior of system (2.1). First, by selecting time delays as the bifurcation parameters and analyzing the distribution of the roots of the corresponding characteristic equation in detail, we mainly investigate the stability of positive equilibrium and the existence of Hopf bifurcation. Further, their direction and properties of the oscillatory dynamic are also researched by using normative theory and central manifold method. Moreover, the dynamic influences of four important parameters, namely S (concentration of serum), k3 (Rate constant for Mdm2p dephosphorylation), k10 (Basal expression rate of PTEN) and k14 (Rate constant for PTEN-induced Akt dephosphorylation) on the P53-PTEN-Akt-Mdm2 network are discussed. In addition, some numerical simulations are executed by using the software Mathematica 11 to verify the theoretical predictions. Finally, the discussion and conclusion are drawn.
{dxdt=k1[S]K1+[S]+k2[v(t−τ1)]n1Kn12+[v(t−τ1)]n1+D1z(t)+k3y(t)K3+y(t)−k4ax(t)−k5q(t)x(t)K4+x(t)−d1x(t),dydt=D2u(t)+k5q(t)x(t)K4+x−k6ay(t)−k3y(t)K3+y(t)−d2y(t),dzdt=k4ax(t)−D1z(t)−d3z(t),dudt=k6ay(t)−D2u(t)−d4u(t),dvdt=k7−k8x(t)v(t)K5+v(t)−k9y(t)v(t)K6+v(t)−d5v(t),dpdt=k10+k11[v(t−τ2)]n2Kn27+[v(t−τ2)]n2−d6p(t),dqdt=k12[S]K8+[S]A−q(t)K9+A−q(t)−k13q(t)K10+q(t)−k14p(t)q(t)K11+q(t). | (2.1) |
where τ1 and τ2 respectively represent transcriptional delays of Mdm2 and PTEN triggered by P53, and the meaning of all the variables are given in Table 1 and related parameters are given in Table 2.
Variable Symbol | Description | Reference |
x | The concentration of MDM2, which is evenly distributed in the nucleus and cytosol | [36] |
y | The concentration of phosphorylated MDM2, which is mainly distributed in the nucleus | [36] |
z | The concentration of MDM2-ARF complex | [36] |
u | The concentration of MDM2p-ARF complex | [36] |
v | The concentration of P53 in the nucleus | [36] |
p | The concentration of PTEN, which is a P53 target and inhibits Akt activation by dephosphorylating PIP3 | [36] |
q | The concentration of active Akt | [36] |
Parameter | Description | value | Reference |
S | serum concentration; it is proportional to the concentration of GFs; GFs induce mitogenic signals | 0.035 | [36] |
a | Free ARF protein(P14ARF) | 0.03 | [36] |
k1 | Rate constant of MDM2 expression induced by serum(is proportional to the concentration of GFs) | 0.15μM/h | [24,45] |
k2 | Rate cconstant of P53-mediated expression of Mdm2 | 0.9μM/h | [24] |
k3 | Rate constant for Mdm2p dephosphorylation | 12μM/h | [36] |
k4 | Rate constant for Mdm2/ARF ossociation | 43(μMh) | [36] |
k5 | Rate constant Mdm2 phosphorylation mediated by Akt | 56/h | [36] |
k6 | Rate constant for Mdm2p/ARF ossociation | 10/(μMh) | [36] |
k7 | Basal rate constant of P53 expression | 8μ/h | [25,44] |
k8 | Rate constant for Mdm2p-mediated P53 degradation | 5/h | [36] |
k9 | Rate constant for Mdm2p-mediated P53 degradation | 18/h | [36] |
k10 | Basal expression rate of PTEN | 0.05μM/h | [36] |
k11 | Rate constant of P53-dependent synthesis of PTEN | 0.7μM/h | [36] |
k12 | Rate constant for Akt phosphorylation induced by growth factors | 12.9μM/h | [36] |
k13 | Rate constant for Akt dephosphorylation | 9.4μM/h | [46,47] |
k14 | Rate constant for PTEN Cinduced Akt dephosphorylation; refering to the dephosphorylation of PIP3 by PTEN | 30/h | [36] |
K1 | Michaelis constant for Mdm2 expression triggered by growth factors | 0.0045 | [36] |
K2 | Hill constant for P53-induced expression of Mdm2 | 0.5μM | [36] |
K3 | Michaelis constant for Mdm2p dephosphorylation | 0.081μM | [36] |
K4 | Michaelis constant for Akt-mediated phosphorylation of Mdm2 | 0.5μM | [36] |
K5 | Michaelis constant for Mdm2-mediated P53 degradation | 0.5μM | [36] |
K6 | Michaelis constant for Mdm2p-mediated P53 degradation | 0.1μM | [36] |
K7 | Hill constant for P53-induced expression of PTEN greater than that of Mdm2 | 1μM | [36] |
K8 | Michaelis constant for Akt activation triggered by growth factor | 0.0147 | [36] |
K9 | Threshold of the total enzyme amount for Akt activation | 0.35μM | [36] |
K10 | Michaelis constant for Akt dephosphorylation | 0.2μM | [36] |
K11 | Michaelis constant for PTEN-induced Akt dephosphorylation | 0.6μM | [36] |
D1 | Rate constant for Mdm2-ARF disassociation | 6/h | [36] |
D2 | Rate constant for Mdm2p-ARF disassociation | 24/h | [36] |
d1 | Half-life of Mdm2 is about 90 min | 0.5/h | [36] |
d2 | Degradation rate of Mdm2p | 0.1/h | [36] |
d3 | Degradation rate of Mdm2-ARF complex | 0.6/h | [36] |
d4 | Degradation rate of Mdm2p-ARF complex | 0.6/h | [36] |
d5 | Half-life of P53 is about 5-20 min | 3.6/h | [36] |
d6 | Degradation rate of PTEN | 0.5/h | [36] |
A | total amount of Akt, which is much more than active Akt and is considered a constant | 2.5 | [36] |
n1 | Hill coefficient of P53-dependent expression of Mdm2 | 4 | [36] |
n2 | Hill coefficient of P53-dependent synthesis of PTEN | 3 | [36] |
τ1 | Time delay of P53 transcription for Mdm2 | 30–75min | estimate |
τ2 | Time delay of P53 transcription for PTEN | 10–15min | estimate |
In this section, we will focus on the effects of two time delays including τ1 and τ2 and four important parameters related to P53 including S, k3, k10, k14 on the system oscillations. All the numerical simulations are performed via Mathematical 11.
For the legibility of the text, we only give short explanation for the theoretical results and the expatiatory and tedious theoretical derivations and results are put in the Supplementary Section. Then numerical simulations of time delay τ1 on the system (2.1) are given when τ2=0.
There is a positive equilibrium solution E∗=(x∗,y∗,z∗,u∗,v∗,p∗,q∗) by setting the right-hand side of the equation equal to zero in (2.1). Then we can get the linearized equation near the equilibrium as follows
{dxdt=c1x(t)+c2y(t)+c3z(t)+c4e−λτ1v(t)+c5q(t),dydt=c6x(t)+c7y(t)+c8u(t)+c9q(t),dzdt=c10x(t)+c11z(t),dudt=c12y(t)+c13u(t),dvdt=c14x(t)+c15y(t)+c16v(t),dpdt=c17v(t)+c18p(t),dqdt=c19p(t)+c20q(t), | (3.1) |
where ci(i=1,...,20) are decided by the parameters in system (2.1) and the positive equilibrium solution E∗=(x∗,y∗,z∗,u∗,v∗,p∗,q∗) and the actual formulas are given in the Supplementary Section.
Furthermore, the characteristic equation of the system (3.1) is given as
m1+m3λ+m5λ2+m7λ3+m9λ4+m11λ5+m13λ6−λ7+e−λτ1(m2+m4λ+m6λ2+m8λ3+m10λ4+m12λ5+m14λ6)=0. | (3.2) |
where mi(i=1,...,14) are depended on the parameters ci(i=1,...,20) and the actual formulas can be found in the Supplementary Section.
Suppose ±iω(ω>0) is a pair of pure virtual roots of the characteristic equation (3.2), then ω satisfies the following condition
m1+im3w−m5w2−im7w3+m9w4+im11w5−m13w6+iw7+(m2+im4w −m6w2−im8w3+m10w4+im12w5−m14w6)(coswτ1−isinwτ1)=0. | (3.3) |
Through a large amount of calculation which is displayed in the Supplementary Section, it is found that there exists unique positive root w0 of equation (3.3). Moreover,
τ(j)1=1w0arccosEF+2πjw0,j=0,1,2,3,⋯. | (3.4) |
Define τ01=min{τ(j)1>0}+∞j=0. Thus, when τ=τ01 equation (3.2) has a pair of pure imaginary roots ±iw0.
Furthermore, it is verified that the condition
sgn{dRe(λ(τ1))dτ1|τ1=τ01}=sgn{Re[dλ(τ1)dτ1]−1|τ1=τ01}>0 | (3.5) |
is holds.
It indicates that the root of characteristic equation(3.2) changes though negative to positive that corresponds to the positive equilibrium of system (2.1) is changed from stability to instability as the time delay τ1 increases and crosses the threshold τ01. What is more, Hopf bifurcation of the positive equilibrium solution occurs at the threshold τ1=τ01. Concretely, the system is asymptotically stable when τ1∈[0,τ01), while it is unstable when τ1>τ01. Thus, τ01 is a vital critical value and represents the length of time delay need for the system arising oscillation behavior.
In addition, according to the normal form of functional differential equation, the following four index to depict the properties of the above Hopf bifurcation are obtained.
{C1(0)=i2w0τ01(g11g20−2|g11|2−|g02|23)+g212,μ2=−Re{C1(0)}Re{λ′(τ01)},T2=−Im{C1(0)}+μ2Im{λ′(τ01)}w0τ01,β2=2Re{C1(0)}. | (3.6) |
Among them, μ2 determines the direction of Hopf bifurcation. If μ2>0, then the Hopf bifurcation is supercritical and the bifurcating periodic solutions exist for τ>τ01, and while it is subcritical when μ2<0 and the bifurcating periodic solutions exist for τ<τ01. The stability of the bifurcating periodic solutions is depended on β2. Especially, the bifurcating periodic solutions in the center manifold are stable when β2<0, while they are unstable if β2>0. The period of the bifurcating periodic solutions is determined by T2. If T2>0, the period increases. When T2<0, the period decreases.
In this section, we will perform numerical simulation to study the affect of time delay τ1 on the system (2.1). On the one hand, it will further verify the correctness of the above theoretical derivation. On the other hand, it will give a more intuitively understand on the importance of time delay τ1. Except for the special parameter changes, all others are taken from Table 2. It is easy to calculated that there is a approximate positive equilibrium E∗(0.56548,0.37000,0.11052,0.00451,0.39694,0.18240,0.33258). The critical value of τ1 is calculated as τ01=0.70454767059 by the formula (3.4). The four important indexes are also calculated as follows according to the formulas (3.6).
{C1(0)=−36.9894−42.7627i,μ2=30.4674,T2=81.8789,β2=−73.9788. | (3.7) |
In order to vividly show the affect of τ1, we used different values to obtain a series of images of Mdm2+Mdm2p, P53, PTEN and Akt concentrations over time as shown in Figure 2. We can see that the system is stable when τ1=0. But as its increases, the system begins to change from stable to progressively stable. When τ1 reaches the value τ01≈0.70455, the system experiences Hopf bifurcation and changes from asymptotic stability to sustained oscillation, which is consistence with the above theoretical conclusion. As τ1 continues to increase, the system still keeps oscillating continuously. At the same time, the magnitude and period of the oscillation of the system become larger. As identified by the above calculated values μ2>0, T2>0 and β2>0, which suggested that the Hopf bifurcation is subcritical and the period and amplitude of the oscillations increases as τ1 increases. Therefore, the behavior of the system can be indirectly controlled by regulating the time delay τ1. Moreover, we plot their images by setting discrete time delays τ1=0.75, τ1=0.8, τ1=0.85, τ1=0.95, τ1=1.05 separately as shown in Figure 3. From Figure 3(a) and (b) we can see that both of the magnitude and period of P53 and Mdm2tot increases as time delay τ1 gradually varies from small to big. Figure 3(c) and (d) respectively shows that magnitude and period of PTEN and Akt are also added as time delay τ1 increases. Interestingly, the oscillation valleys of PTEN nearly remain unchanged. But for the the Akt, its oscillation peaks almost stayed the same. For more visual observation, the change in the period and amplitude are drawn in Figure 4, which illustrated that both of the period and amplitude of four molecule including P53, Mdm2tot, PTEN and Akt are increased as the time delay τ1 increases. The increase degrees of the period for the four molecules are almost the same as shown in Figure 4(a), which is coordinate to the experiments. However, the increase degrees of the amplitude for the four molecules are different as shown in Figure 4(b), which is Mdm2tot, P53, PTEN and Akt in the order from small to large and the biological meanings are mysterious.
In the Supplementary, we have only proved the existence of Hopf bifurcation when τ1 varies. According to the above subsection, we identified that system (2.1) undergoes a supercritical Hopf bifurcation at the positive equilibrium E∗ when τ1=τ01. However, we do not consider the time delay τ2 of P53-promoting transcription of PTEN for the convenience of the study. In fact, the time delay does exist. Therefore, it is necessary to study the co-regulation of τ1 and τ2. In this subsection, we take τ1 to satisfy the oscillation condition and then make the τ2 varies. By comparing Figure 5 (b) and (c), (a) and (e), (d) and (f), we get that the system oscillation is enhanced by τ1, which is agree with the above analysis. Intestinally, if we comparing Figure 5 (a) and (b), (c) and (d), (e) and (f), the inverse phenomenon occurs, which is he system oscillation is suppressed by τ2. Therefore, the relative sizes between τ1 and τ2 determines the degree of oscillation. To our knowledge, transcription and splicing of intron sequences increase the time required for the extension and splicing of genes to mature RNA [40,48,49]. Thus, an effective way to vary the time delay is changing the number of introns within the gene. Takashima et al. previously showed that deletion of all three introns within the Hes7 gene reduces the time delay by 19 min and completely abolishes oscillatory expression [50]. Based on conclusion that each consecutive intron splicing in mammalian cells required about 0.4 to 7.5 minutes [51,52], the number of introns within the genes of Mdm2 and PTEN can be calculated in order to achieve the desired length of time delay τ1 and τ2, which requires specific experimental verification. Time delays τ1 and τ2 can flexibly regulate the oscillation dynamics of P53. Experiments have shown that P53 oscillation dynamics is closely related to many diseases including cancer [9,25,26,27]. In particular, P53 oscillation can promote the cells to repair damage. And if the damage is too severe to repair, it can also promote the cell trigger the programmed cell death. In this way, the cells can avoid the wrong genetic information from being inherited to the next generation of cells. Therefore, the above research results provide a method for regulating P53 oscillation dynamics through time delays, which may provide a new insight for the treatment of cancer and other diseases.
In this subsection, in order to more comprehensive understand the P53 oscillation dynamic, a Sobol sensitivity analysis is performed to examine the influence of each parameter ki(i=1,2,...,14) on the P53 gene regulatory network using the ODE sensitivity package in R software. The evolution processes of the parameter sensitivity indices including the first order and total Sobol' indices for the P53 gene regulatory system (2.1) are shown in Figure 6. The first order Sobol' indices measures the pure interaction influence of the variables, while the total sensitivity index measures the influence of the variables including all interactions of any order and it reflects the individual influence of all factors plus every interaction effect [53]. Using the total Sobol' indices for the concentration of MDM2 denoted by x, a clear order of series parameters have been established: k3 has the largest total effect, followed by S, k7, k11, k5, k8, k2, k12, k14, k10 and so on. In addition, considering the influence of some parameters that have important biological significance and can cause the essential changes in the system, this subsection will focus on the four parameters S, k3, k10, and k14 to elaborate the cooperative effects among them and two time delays on the system dynamics. In concretely, growth factor S in serum not only induce the transcriptional activation of Mdm2, but the activation of Akt which, phosphorylates Mdm2, promoting its nuclear accumulation and further enhance inhibition to P53 [36]. Both of Mdm2 and Mdm2p have the ability to induce the P53 degradation. k3 represents the rate constant for Mdm2p dephosphorylation. Mdm2p has the stronger degradation ability on P53 than Mdm2 [24,54]. In other words, the greater k3, the greater dephosphorylation rate of Mdm2p, this will convert Mdm2p into Mdm2 and increase P53 indirectly. Thus, S and k3 can indirectly affect the kinetics of P53. k10 is the basal expression rate of PTEN and k14 refers to the rate constant for PTEN induced Akt dephosphorylation. Both of them reflect the strength of the positive feedback loop P53 → PTEN –∣ Akt → Mdm2 –∣ P53 including P53 and PTEN. The larger the k10, the more PTEN is produced, which in turn promotes the positive feedback loop of P53 leading to an increase in P53. The larger the k14, the less Akt is phosphorylated, the more P53 produced due to the weak repression of P53 from Mdm2. Obviously, both of the values k10 and k14 are important to the system dynamic behavior.
Now, we will discuss the the cooperative effects of S, k3, k10 and k14 with the time delays respectively, and the simulation results are obtained in Figure 7, 8, 9, and 10. Here, we set the time delay τ1=0.8 and only with τ2 varies. Especially, we consider three values τ2, which is 0, 0.1, and 0.15 in turn. On the one hand, if we horizontally observe the Figure 7, it is obviously that the parameter S can induce and block the oscillation of the four molecules in all three sets of the time delays. The oscillation range occurs at a neighborhood of S≈0.035. On the other hand, if we vertically observe the Figure 7, it can be find that the time delay τ2 has the inhibitory role on the system oscillation in all three sets of the time delays, which is in accordance with the above results. S represents the Serum concentration that promotes the concentration of Mdm2 so that S indirectly against the accumulation of P53 concentration. Too much or too little serum concentration will not cause the produce of system oscillation, only moderate serum concentration will induce it. For the parameter k3, k10 and k14, we can get the same result with S. First, observing the Figures 8, 9, and 10 horizontally, we will find that regardless which value set of the time delays, the three parameters k3, k10 and k14 can induce the oscillation of the four molecules or make it disappear. Then, looking at the Figures 8, 9, and 10 vertically, it can also be found that no matter which value of the parameters k3, k10 and k14 takes, the time delay τ2 has a suppressive effect on system oscillation. In particular, the parameter range of the system oscillation is k3≈11.2, k10≈0.04 and k14≈24 in sequence when other parameters keep fixed. In summary, under the influence of time delays, the system has strong sensitivity to four parameters and is also strictly controlled by the them. The k3, k10 and k14 are all indirectly enhance the P53 concentration. Similarly with S, neither too large nor too small parameters can cause system oscillations, and only appropriately large parameters can do it.
In order to obtain a more visual perspective on the cooperative effect of two time delays and four important parameters, bifurcation diagrams of P53 with respect to four important parameters under different cases of time delay are given in Figure 11. The existence of Hopf bifurcation indicates that the system oscillation occurs. It can be seen that there are three common characters among the Figures 11(a), (b), (c) and (d). One of them is that the Hopf bifurcation occurs only when τ1=0.8,τ2=0, while under other two cases including τ1=0,τ2=0 and τ1=0.8,τ2=0.1 the bifurcation phenomenon can not be observed. This led us to conclude that time delay τ1 has the opposite effect on the P53 oscillation production than τ2. Concretely, τ1 can prompt the P53 oscillation occur, whereas τ1 tends to hold back the oscillation. From a biological point of view, P53 oscillation means that the cell choices to repair the damage in response to stresses. The second common character is that bistability occurs only when τ1=0.8,τ2=0, while under other two cases including τ1=0,τ2=0 and τ1=0.8,τ2=0.1 the bistability phenomenon can not be found. The bistability of P53 is an important feature, which corresponding to the switch of cell fate decision. More specifically, the low steady-state means that the cell stays on a normal cell cycle state, while the high steady-state means that the cell triggers appoptosis when upon extra stimulations. Both of the DNA damage repair and appoptosis are the preventive measures of cells to avoid disease or cancers [8,9,10,11,12,13,14]. Therefore, τ1 provides two ways to prevent the occurrence of diseases. The third common character is that time delays τ1 and τ2 is helpful to accumulate or silent the activity of P53 as can be seen by comparing the pink and blue lines, which suggested that the time delays τ1 and τ2 have the ability to flexibly control the state of P53. On the other hand, there are also two main differences among the Figures 11(a), (b), (c) and (d). The first one is the parameter s has the opposite role than other three parameters. As s increases, the P53 concentration is declined. But as k3,k10 and k14 increases, the P53 concentration is raised. The second one is that the switch in the Figure 11(c) is irreversible, while others are reversible because the parameter k10 can not be negative. This indicates that the apoptosis triggered by the cooperation of k10 and τ1 is irredeemable death. The above analysis again shows that p53 oscillation is regulated by two time delays and four important parameters. The specific conclusion is a prediction, which needs further verification by subsequent biological experiments.
In this paper, we studied a mathematical model of the core regulatory network of cell fate decision that responds to growth factor instead of DNA damage. It contains seven major components and two key time delays. In our model, the dynamics of the P53-PTEN-Akt-Mdm2 network is studied using Hopf bifurcation theory and numerical simulation. The results help us understand how normal cells respond to growth factor stimuli, thereby preventing the occurrence and development of disease. Here, we just studied the effect of time delay on the P53-PTEN-Akt-Mdm2 network, whereas the downstream networks including survival and apoptosis module have not been considered. Beyond that, It has been reported that aberrant expression of miRNAs and a global decrease of their levels are often observed in multiple types of human cancer cells. In addition, the P53 pathway are often crosstalk with E2F and NF-kB signalling pathway. Therefore, more extensively network should be further investigated for comprehensive understand the potential mechanism of P53 related network in the future.
In this paper, we proposed a delayed mathematical model to describe the P53-PTEN-Akt-Mdm2 network and mainly focused on Hopf bifurcation and oscillation behavior of the system. Firstly, we analyse the role of time delays in the system and find that the time delay could cause Hopf bifurcation. In order to facilitate the research, we let τ2=0 and the threshold value τ01≈0.70455 for generating the Hopf bifurcation is obtained by calculation. Then, we obtain that the period and amplitude increase theoretically and numerically with the rising of τ1. Besides, for the first step of numerical simulation, we verify the existence of Hopf bifurcation consistent with theoretical derivation. Next, we take τ2 into account that τ1 and τ2 and infer that τ1 and τ2 can cause oscillation and suppressed oscillation respectively. More important is that we found that the relative size of τ2 and τ2 controls the pulse shape of P53. The dynamic properties of the whole system could be subverted for their minor change. Furthermore, we study the influences of four important parameters, namely S(concentration of serum), k3(Rate constant for Mdm2p dephosphorylation), k10(Basal expression rate of PTEN) and k14(Rate constant for PTEN-induced Akt dephosphorylation) in the system. The results indicate that we obtained is the stability and the amplitude of the periodic solution could also be destroyed by their minor changes. We mainly focus on the dynamic properties of P53 and its related components under non-stress conditions, therefore this research might be helpful to further understand the mechanism of P53 and its related components under the normal circumstances and provide a new perspective for preventing cancer.
The authors are grateful to the anonymous referees and editors for their valuable suggestions which helped us to improve the quality of this work. This research was funded by the National Natural Science Foundation of China (11762022) and the youth academic and technical leaders of Yunnan Province (2019HB015).
The authors declare that they have no conflict of interest.
For the convenience of presentation, we set x, y, z, u, v, p and q to represent Mdm2, Mdm2p, MA, MpA, P53, PTEN and Akt respectively. And parameters of the model are replaced by the form in Table 1, our system will be converted to
{dxdt=k1[S]K1+[S]+k2[v(t−τ1)]n1Kn12+[v(t−τ1)]n1+D1z(t)+k3y(t)K3+y(t)−k4ax(t)−k5q(t)x(t)K4+x(t)−d1x(t),dydt=D2u(t)+k5q(t)x(t)K4+x−k6ay(t)−k3y(t)K3+y(t)−d2y(t),dzdt=k4ax(t)−D1z(t)−d3z(t),dudt=k6ay(t)−D2u(t)−d4u(t),dvdt=k7−k8x(t)v(t)K5+v(t)−k9y(t)v(t)K6+v(t)−d5v(t),dpdt=k10+k11[v(t−τ2)]n2Kn27+[v(t−τ2)]n2−d6p(t),dqdt=k12[S]K8+[S]A−q(t)K9+A−q(t)−k13q(t)K10+q(t)−k14p(t)q(t)K11+q(t). | (5.1) |
Obviously, it is easy to get a positive equilibrium solution by setting the right-hand side of the equation equal to zero in (6.1), which is quite consistent with the actual biological background and significance. For the convenience of research, we set τ2=0 and E∗=(x∗,y∗,z∗,u∗,v∗,p∗,q∗) as a positive balance point of the system(6.1), and then we can get the linearization equation near the equilibrium as follows
{˙x(t)=c1x(t)+c2y(t)+c3z(t)+c4e−λv(t)+c5q(t),˙y(t)=c6x(t)+c7y(t)+c8u(t)+c9q(t),˙z(t)=c10x(t)+c11z(t),˙u(t)=c12y(t)+c13u(t),˙v(t)=c14x(t)+c15y(t)+c16v(t),˙p(t)=c17v(t)+c18p(t),˙q(t)=c19p(t)+c20q(t). | (5.2) |
where
c1=−K5K4q∗(K4+x∗)2−ak4−d1, c2=K3k3(K3+y∗)2, c3=D1, c4=4K42k2(v∗)3(K44+(v∗)4)2c5=−k5x∗K4+x∗, c6=K4k5q∗(K4+x∗)2, c7=−K3k3(k3+y∗)2−ak6−d2, c8=D2,c9=k5x∗K4+x∗, c10=ak4, c11=−D1−d3, c12=ak6, c13=−D2−d4,c14=−k8v∗K5+v∗, c15=−k9v∗K6+v∗, c16=−K5k8x∗(K5+v∗)2−K6k9y∗(K6+v∗)2−d5c17=3K37k11(v∗)2(K37+(v∗)3)2, c18=−d6, c19=−k14q∗(K11+q∗),c20=−k12SK9(K8+S)(K9+A−q∗)2−K10k13(K10+q∗)2−K11k14p∗(K11+q∗)2. |
Furthermore, we can get the characteristic equations of equations (6.2)
m1+m3λ+m5λ2+m7λ3+m9λ4+m11λ5+m13λ6−λ7+e−λτ1(m2+m4λ+m6λ2+m8λ3+m10λ4+m12λ5+m14λ6)=0. | (5.3) |
where
m1=c5c8c11c12c14c17c19−c5c7c11c13c14c17c19+c2c9c11c13c14c17c19+c3c9c10c13c15c17c19 +c5c6c11c13c15c17c19−c1c9c11c13c15c17c19+c3c8c10c12c16c18c20−c1c8c11c12c16c18c20 −c3c7c10c13c16c18c20−c2c6c11c13c16c18c20+c1c7c11c13c16c18c20, m2=c4c8c11c12c14c18c20−c4c7c11c13c14c18c20+c4c6c11c13c15c18c20 m3=−c3c8c10c12c16c18+c1c8c11c12c16c18+c3c7c10c13c16c18+c2c6c11c13c16c18 −c1c7c11c13c16c18−c3c8c10c12c20c18+c1c8c11c12c20c18+c3c7c10c13c20c18 +c2c6c11c13c20c18−c1c7c11c13c20c18+c3c7c10c16c20c18+c2c6c11c16c20c18 −c1c7c11c16c20c18+c1c8c12c16c20c18+c8c11c12c16c20c18+c2c6c13c16c20c18 −c1c7c13c16c20c18+c3c10c13c16c20c18−c1c11c13c16c20c18−c7c11c13c16c20c18 +c5c7c11c14c17c19−c2c9c11c14c17c19−c5c8c12c14c17c19+c5c7c13c14c17c19 −c2c9c13c14c17c19+c5c11c13c14c17c19−c3c9c10c15c17c19−c5c6c11c15c17c19 +c1c9c11c15c17c19−c5c6c13c15c17c19+c1c9c13c15c17c19+c9c11c13c15c17c19 −c3c8c10c12c16c20+c1c8c11c12c16c20+c3c7c10c13c16c20+c2c6c11c13c16c20 −c1c7c11c13c16c20, m4=−c4c8c11c12c14c18+c4c7c11c13c14c18−c4c6c11c13c15c18+c4c7c11c14c20c18 −c4c8c12c14c20c18+c4c7c13c14c20c18+c4c11c13c14c20c18−c4c6c11c15c20c18 −c4c6c13c15c20c18−c4c8c11c12c14c20+c4c7c11c13c14c20−c4c6c11c13c15c20, m5=c3c8c10c12c16−c1c8c11c12c16−c3c7c10c13c16−c2c6c11c13c16 +c1c7c11c13c16−c3c7c10c18c16−c2c6c11c18c16+c1c7c11c18c16 −c1c8c12c18c16−c8c11c12c18c16−c2c6c13c18c16+c1c7c13c18c16 −c3c10c13c18c16+c1c11c13c18c16+c7c11c13c18c16+c11c13c18c16 −c3c7c10c20c16−c2c6c11c20c16+c1c7c11c20c16−c1c8c12c20c16 −c8c11c12c20c16−c2c6c13c20c16+c1c7c13c20c16−c3c10c13c20c16 +c1c11c13c20c16+c7c11c13c20c16−c2c6c18c20c16+c1c7c18c20c16 −c3c10c18c20c16+c1c11c18c20c16+c7c11c18c20c16−c8c12c18c20c16 +c1c13c18c20c16+c7c13c18c20c16+c3c8c10c12c18−c1c8c11c12c18 −c3c7c10c13c18−c2c6c11c13c18+c1c7c11c13c18−c5c7c14c17c19 +c2c9c14c17c19−c5c11c14c17c19−c5c13c14c17c19+c5c6c15c17c19 −c1c9c15c17c19−c9c11c15c17c19−c9c13c15c17c19+c3c8c10c12c20 −c1c8c11c12c20−c3c7c10c13c20−c2c6c11c13c20+c1c7c11c13c20 −c3c7c10c18c20−c2c6c11c18c20+c1c7c11c18c20−c1c8c12c18c20 −c8c11c12c18c20−c2c6c13c18c20+c1c7c13c18c20−c3c10c13c18c20 +c1c11c13c18c20+c7c11c13c18c20, m6=c4c8c11c12c14−c4c7c11c13c14−c4c7c11c18c14+c4c8c12c18c14 −c4c7c13c18c14−c4c11c13c18c14−c4c7c11c20c14+c4c8c12c20c14 −c4c7c13c20c14−c4c11c13c20c14−c4c7c18c20c14−c4c11c18c20c14 −c4c13c18c20c14+c4c6c11c13c15+c4c6c11c15c18+c4c6c13c15c18 +c4c6c11c15c20+c4c6c13c15c20+c4c6c15c18c20, |
m7=−c3c8c10c12+c1c8c11c12+c1c8c16c12+c8c11c16c12 +c1c8c18c12+c8c11c18c12+c8c16c18c12+c1c8c20c12 +c8c11c20c12+c8c16c20c12+c8c18c20c12+c3c7c10c13 +c2c6c11c13−c1c7c11c13+c3c7c10c16+c2c6c11c16 −c1c7c11c16+c2c6c13c16−c1c7c13c16+c3c10c13c16 −c1c11c13c16−c7c11c13c16+c3c7c10c18+c2c6c11c18 −c1c7c11c18+c2c6c13c18−c1c7c13c18+c3c10c13c18 −c1c11c13c18−c7c11c13c18+c2c6c16c18−c1c7c16c18 +c3c10c16c18−c1c11c16c18−c7c11c16c18−c1c13c16c18 −c7c13c16c18−c11c13c16c18+c5c14c17c19+c9c15c17c19 +c3c7c10c20+c2c6c11c20−c1c7c11c20+c2c6c13c20 −c1c7c13c20+c3c10c13c20−c1c11c13c20−c7c11c13c20 +c2c6c16c20−c1c7c16c20+c3c10c16c20−c1c11c16c20 −c7c11c16c20−c1c13c16c20−c7c13c16c20−c11c13c16c20 +c2c6c18c20−c1c7c18c20+c3c10c18c20−c1c11c18c20 −c7c11c18c20−c1c13c18c20−c7c13c18c20−c11c13c18c20 −c1c16c18c20−c7c16c18c20−c11c16c18c20−c13c16c18c20, m8=c4c7c11c14−c4c8c12c14+c4c7c13c14+c4c11c13c14 +c4c7c18c14+c4c11c18c14+c4c13c18c14+c4c7c20c14 +c4c11c20c14+c4c13c20c14+c4c18c20c14−c4c6c11c15 −c4c6c13c15−c4c6c15c18−c4c6c15c20, m9=−c3c7c10−c3c13c10−c3c16c10−c3c18c10−c3c20c10 −c2c6c11+c1c7c11−c1c8c12−c8c11c12−c2c6c13 +c1c7c13+c1c11c13+c7c11c13−c2c6c16+c1c7c16 +c1c11c16+c7c11c16−c8c12c16+c1c13c16+c7c13c16 +c11c13c16−c2c6c18+c1c7c18+c1c11c18+c7c11c18 −c8c12c18+c1c13c18+c7c13c18+c11c13c18+c1c16c18 +c7c16c18+c11c16c18+c13c16c18−c2c6c20+c1c7c20 +c1c11c20+c7c11c20−c8c12c20+c1c13c20+c7c13c20 +c11c13c20+c1c16c20+c7c16c20+c11c16c20+c13c16c20 +c1c18c20+c7c18c20+c11c18c20+c13c18c20+c16c18c20, m10=−c4c7c14−c4c11c14−c4c13c14−c4c18c14−c4c20c14+c4c6c15, m11=c2c6−c1c7+c3c10−c1c11−c7c11+c8c12−c1c13−c7c13−c11c13 −c1c16−c7c16−c11c16−c13c16−c1c18−c7c18−c11c18−c13c18 −c16c18−c1c20−c7c20−c11c20−c13c20−c16c20−c18c20, m12=c4c14, m13=c1+c7+c11+c13+c16+c18+c20, m14=0. |
In order to theoretically analyze the sufficient conditions for generating oscillations, we assume that iw(w>0) is a root of equation (6.3). Then, bring iw into equation (6.3) to get:
m1+im3w−m5w2−im7w3+m9w4+im11w5−m13w6+iw7+(m2+im4w −m6w2−im8w3+m10w4+im12w5−m14w6)(coswτ1−isinwτ1)=0. | (5.4) |
Separating the real part and the imaginary part we can get
{ m1−m5w2+m9w4−m13w6=−(m2−m6w2+m10w4−m14w6)coswτ1 −(m4w−m8w3+m12w5)sinwτ1, m3w−m7w3+m11w5+w7=−(m4w−m8w3+m12w5)coswτ1 −(−m2+m6w2−m10w4+m14w6)sinwτ1. | (5.5) |
Then there will be
{ coswτ1=EF sinwτ1=M+NF, F=(−m4w+m8w3−m12w5)2−(m2−m6w2+m10w4−m14w6)× (−m2+m6w2−m10w4+m14w6) E=(−m1+m5w2−m9w4+m13w6)(m2−m6w2+m10w4−m14w6) +(−m4w+m8w3−m12w5)(m3w−m7w3+m11w5+w7) M=(m2m3−m1m4)w+(m4m5−m3m6−m2m7+m1m8)w3 +(−m1m12+m11m2+m10m3+m6m7−m5m8−m4m9)w5 N=(m2−m14m3+m13m4+m12m5−m11m6−m10m7+m8m9)w7 +(m10m11−m6+m14m7−m13m8−m12m9)w9 +(m10+m12m13−m11m14)w11−m14w13 | (5.6) |
The above equations are squared and then the equations of the equal sign are added separately. Then, there will be
w14+w12(2m11+m213)+w10(−2m7+m211+m212−2m9m13)+w8(2m3+m29−m210 −2m7m11−2m8m12+2m5m13)+w6(m27+m28−2m5m9+2m6m10+2m3m11+ 2m4m12−2m1m13)+w4(m25−m26−2m3m7−2m4m8+2m1m9−2m2m10)+w2(m23 +m24−2m1m5+2m2m6)+m21−m22=0. | (5.7) |
Moreover, it is easy to verify that
2m11+m213>0,−2m7+m211+m212−2m9m13>0,2m3+m29−m210−2m7m11−2m8m12+2m5m13>0,m27+m28−2m5m9+2m6m10+2m3m11+2m4m12−2m1m13>0,m25−m26−2m3m7−2m4m8+2m1m9−2m2m10>0m23+m24−2m1m5+2m2m6>0,m21−m22<0. |
Therefore, equation (6.7) exists unique positive root w0. From (6.6) we can get
τ(j)1=1w0arccosEF+2πjw0 |
where j = 0, 1, 2, 3⋯. And we define τ01=min{τ(j)1>0}+∞j=0. Thus, when τ=τ01 equation (6.3) has a pair of pure imaginary roots ±iw0. Next we will prove that the root of equation (6.3) has a strict negative real part at τ1∈[0,τ01), and there are at least two roots containing positive real parts when τ1∈(τ01,τm1), where m is the minimum positive integer satisfying for τ01<τm1.
Lemma 6.1 [55] For the exponential polynomial P(λ,e−λτ1,e−λτ2⋯e−λτm) in the process of (τ1,τ2,⋯,τm) changes, only when P(λ,e−λτ1,e−λτ2⋯e−λτm) has a zero point on the imaginary axis, or when it has a zero point through the imaginary axis, the sum of the zeros in the half-open plane is likely to change. Where
P(λ,e−λτ1,e−λτ2⋯e−λτm)=λn+p(0)1λn−1+⋯+p(0)n−1λ+p(0)n+(p(1)1λn−1 +⋯+p(1)n−1λ+p(1)n)e−λτ1+⋯+(p(m)1λn−1+⋯+p(m)n−1λ+p(m)n)e−λτm. | (5.8) |
It can be seen from the lemma that it is only necessary to prove that the root of the equation (6.3) has a strict negative real part at τ1=0. And then as τ1=τ01, a pair of pure imaginary roots ±iw0 are derived, therefore, for the second half of our results we only need to prove the following formula:dRe(λ(τ1))dτ1|τ1=τ01>0. The left and right ends of the second equation of equation (6.5) are derived for A respectively, we can get
m3−3m7w20+5m11w40+7w60+(2m5w0−4m9w30+6m13w50)i+ (cosw0τ01−isinw0τ01)[(m2−m6w20+m10w40−m14w60+(m4− m8w30+m12w50)i)(−iw0/λ′(τ01)−τ01)+m4−3m8w20+5m12w40 +(2m6w0−4m10w30+6m14w50)i]=0. | (5.9) |
Let A=m3−3m7w20+5m11w40+7w60, B=2m5w0−4m9w30+6m13w50, C=cosw0τ01−isinw0τ01, D=m2−m6w20+m10w40−m14w60, E=m4−m8w30+m12w50, F=m4−3m8w20+5m12w40, G=2m6w0−4m10w30+6m14w50. Furthermore, [dλ(τ1)dτ1]−1|τ1=τ01=iw0[−(A+B)−C(F+G)C(D+E)+τ01]. Then by calculating we can get sgn{dRe(λ(τ1))dτ1|τ1=τ01}=sgn{Re[dλ(τ1)dτ1]−1|τ1=τ01}>0.
Therefore, we prove that the root of characteristic equation(6.5) crosses the virtual axis from left to right as λ(τ01)=±iw0. Obviously, Hopf bifurcation of the positive equilibrium solution occurs at τ1=τ01. Besides, the system is progressively stable when τ1∈[0,τ01) while, if τ1>τ01 it is unstable. So for our system, τ01 is a vital quantity, it could predict the oscillation of P53 or not.
In the previous section, we have obtained that the system (6.2) undergoes a Hopf bifurcation or Turing-Hopf bifurcation at the positive constant (x∗,y∗,z∗,u∗,v∗,p∗,q∗) when τ1=τ01. In this section, we will study the properties of Hopf bifurcation and the stability of bifurcated periodic solutions by using the normal form theory and central manifold theory due to Hassard, Kazarinoff and Wan [56]. Throughout this section, we always assume that system (2.1) undergoes Hopf bifurcation when τ1=τ01 at the positive equilibrium (x∗,y∗,z∗,u∗,v∗,p∗,q∗), and the corresponding purely imaginary roots of the characteristic equation are ±iw0. Subsequently, we let ˉx=x(t)−x∗,ˉy=y(t)−x∗,ˉz=z(t)−z∗,ˉu=u(t)−u∗,ˉv=v(t)−v∗,ˉp=p(t)−p∗,ˉq=q(t)−q∗. And we still denote ˉx,ˉy,ˉz,ˉu,ˉv,ˉp,ˉq as x,y,z,u,v,pandq. Moreover, let τ1=τ01+γ normalizing the time delay τ1 by the time-scaling t→t/τ1, and the system (2) transform to
(˙x˙y˙z˙u˙v˙p˙q)=(τ01+γ)(c1c2c30c4e−λ0c5c6c70c800c9c100c1100000c120c13000c14c1500c16000000c17c18000000c19c20)(xyzuvpq)+(τ01+γ)(∞∑i=21i![f(i)11(v∗)v(t−1)i+f(i)12(y∗)yi+f(i)13(x∗)q∗xi+if(i−1)13(x∗)qxi−1]∞∑i=21i![−f(i)13(x∗)q∗xi−if(i−1)13(x∗)qxi−1+f(i)12(y∗)yi]00∞∑i=21i![f(i)51(v∗)x∗vi+if(i−1)51(v∗)xvi−1+f(i)52(v∗)y∗vi+if(i−1)52(v∗)yvi−1]∞∑i=21i!f(i)61(v∗)vi∞∑i=21i![f(i)71(q∗)qi+f(i)72(q∗)qi+f(i)73(q∗)q∗qi+if(i−1)73(q∗)pqi−1]) |
where c1,c2,⋯,c20 is consistent with the previously defined, and
f(2)11(v)=32k2v10(K42+v4)3−44k2v6(K42+v4)2+12k2v2K42+v4, f(3)11(v)=−384k2v13(K42+v4)4+672k2v9(K42+v4)3−312k2v5(K42+v4)2+24k2vK42+v4 f(2)12(y)=2k3y(K3+y)3−2k3(K3+y)2,f(3)12(y)=−6k3y(K3+y)4+6k3(K3+y)3, f(1)13(x)=k5x(K4+x)2−k5K4+x,f(2)13(x)=−2k5x(K4+x)3+2k5(K4+x)2 f(3)13(x)=6k5x(K4+x)4−6k5(K4+x)3,f(1)51(v)=k8v(K5+v)2−k8K5+v, f(2)51(v)=−2k8v(K5+v)3+2k8(K5+v)2,f(3)51(v)=6k8v(K5+v)4−6k8(K5+v)3, |
f(1)52(v)=k9v(K6+v)2−k9K6+v,f(2)52(v)=−2k9v(K6+v)3+2k9(K6+v)2, f(3)52(v)=6k9v(K6+v)4−6k9(K6+v)3,f(2)61(v)=18k11v7(K37+v3)3−24k11v4(K37+v3)2+6k11vK37+v3, f(3)61(v)=−162k11v9(K37+v3)4+270k11v6(K37+v3)3−114k11v3(K37+v3)2+6k11K37+v3, f(2)71(q)=2k12(A−q)S(A+K9−q)3(K8+S)−2k12S(A+K9−q)2(K8+S), f(3)71(q)=6k12(A−q)S(A+K9−q)4(K8+S)−6k12S(A+K9−q)3(K8+S), f(2)72(q)=−2k13q(K10+q)3+2k13(K10+q)2,f(3)72(q)=6k13q(K10+q)4−6k13(K10+q)3, f(1)73(q)=k14q(K11+q)2−k14K11+q,f(2)73(q)=−2k14q(K11+q)3+2k14(K11+q)2, f(3)73(q)=6k14q(K11+q)4−6k14(K11+q)3. |
Let
U =(u1(t),u2(t),u3(t),u4(t),u5(t),u6(t),u7(t))T =(x(t),y(t),z(t),u(t),v(t),p(t),q(t))T |
And define C=C([−1,0],R7), then (6.2) becomes to
˙U=Lγ(Ut)+f(γ,Ut) | (5.10) |
where Lγ:C→R7,f:R×C→R7 whose specific form is as follows
Lγ(ϕ)=(τ01+γ)(c1c2c3000c5c6c70c800c9c100c1100000c120c13000c14c1500c16000000c17c18000000c19c20)(ϕ1(0)ϕ2(0)ϕ3(0)ϕ4(0)ϕ5(0)ϕ6(0)ϕ7(0)) +(τ01+γ)(0000c400000000000000000000000000000000000000000000)(ϕ1(−1)ϕ2(−1)ϕ3(−1)ϕ4(−1)ϕ5(−1)ϕ6(−1)ϕ7(−1)) | (5.11) |
f(γ,ϕ)=(τ01+γ)(∞∑i=21i![f(i)11(v∗)ϕi5(−1)+f(i)12(y∗)ϕi2(0)+f(i)13(x∗)q∗ϕi1(0)+if(i−1)13(x∗)ϕ7(0)ϕi−11(0)]∞∑i=21i![−f(i)13(x∗)q∗ϕi1(0)−if(i−1)13(x∗)ϕ7(0)ϕi−11(0)+f(i)12(y∗)ϕi2(0)]00∞∑i=21i![f(i)51(v∗)x∗ϕi5(0)+if(i−1)51(v∗)ϕ1(0)ϕi−15(0)+f(i)52(v∗)y∗ϕi5(0)+if(i−1)52(v∗)ϕ2(0)ϕi−15(0)]∞∑i=21i!f(i)61(v∗)ϕi5(0)∞∑i=21i![f(i)71(q∗)ϕi7(0)+f(i)72(q∗)ϕi7(0)+f(i)73(q∗)p∗ϕi7(0)+if(i−1)73(q∗)ϕ6(0)ϕi−17(0)]) | (5.12) |
where ϕ=(ϕ1(t),ϕ2(t),ϕ3(t),ϕ4(t),ϕ5(t)ϕ6(t),ϕ7(t))T∈C. According to the Riesz representation theorem there exists a 7×7 matrix function η(θ,γ), which is bounded variogram on θ∈[−1,0] such that Lγϕ=∫0−1dη(θ,γ)ϕ(θ) for ϕ∈C([−1,0],R7).
In fact, we can choose
η(θ,γ)=(τ01+γ)(c1c2c3000c5c6c70c800c9c100c1100000c120c13000c14c1500c16000000c17c18000000c19c20)δ(θ)+(τ01+γ)(0000c400000000000000000000000000000000000000000000)δ(θ+1) | (5.13) |
where δ(θ) is Dirac delta function. And we define
Λγϕ={dϕ(θ)dθ,θ∈[−1,0),∫0−1dη(θ,γ)ϕ(θ),θ=0,and Rγϕ={0,θ∈[−1,0),f(γ,ϕ),θ=0. | (5.14) |
Obviously, we can transform (6.10) to the following form
˙U=ΛγUt+RγUt | (5.15) |
where Ut(θ)=U(t+θ). For ψ∈C1([−1,0],(R7)∗), we define
Λ∗γψ(s)={−dψ(s)ds,θ∈(0,−1],∫0−1dηT(t,γ)ψ(−t),s=0, | (5.16) |
and
⟨ψ(s),ϕ(θ)⟩=ˉψ(s)ϕ(0)−∫0−1∫θξ=0ˉψ(ξ−θ)dη(θ)ϕ(ξ)dξ, | (5.17) |
which is a bilinear inner product. Obviously, Λ0 and Λ∗0 are adjoint operators for each other. In addition, ±iw0τ01 are the eigenvalues of Λ0. Therefore, they are also eigenvalues of Λ∗0. We let q(θ) be the eigenvector of Λ0 corresponding to iw0τ01 and q∗(s) be the eigenvector of Λ∗0 corresponding to −iw0τ01, which meet the following conditions
q(θ)=eiw0τ01θ(1,v1,v2,v3,v4,v5,v6)T, q∗(s)=Geiw0τ01s(1,v∗1,v∗2,v∗3,v∗4,v∗5,v∗6). | (5.18) |
From Λ0q(0)=iw0τ01q(0) and Λ∗0q∗(0)=−iw0τ01q∗(0), it is easy to deduce
v1=−v11v12v11=(−c14c17c19c9+c6(c16−iw0)(c18−iw0)(c20−iw0))(c13−iw0)v12=(−c15c17c19c9+(c16−iw0)(c18−iw0)(c20−iw0)(c7−iw0))(c13−iw0)−c12c8(c16−iw0)(c18−iw0)(c20−iw0) |
v2=−v21v22v21=c10v22=c11−iw0 |
v3=−v31v32v31=c12(c9c14c17c19−c6c16c18c20)+c12(ic6c16c18+ic6c16c20+ic6c18c20)w0+c12(c6c16+c6c18+c6c20)w20−ic6c12w30v32=−c9c13c15c17c19−c8c12c16c18c20+c7c13c16c18c20+(ic8c12c16c18−ic7c13c16c18+ic9c15c17c19+ic8c12c16c20−ic7c13c16c20+ic8c12c18c20−ic7c13c18c20−ic7c16c18c20−ic13c16c18c20)w0+(c8c12c16−c7c13c16+c8c12c18−c7c13c18−c7c16c18−c13c16c18+c8c12c20−c7c13c20−c7c16c20−c13c16c20−c7c18c20−c13c18c20−c16c18c20)w20+(−ic8c12+ic7c13+ic7c16+ic13c16+ic7c18+ic13c18+ic16c18+ic7c20+ic13c20+ic16c20+ic18c20)w30+(c7+c13+c16+c18+c20)w40−iw50 |
v4=−v41v42v41=w40c14−c8c12c14c18c20+c7c13c14c18c20−c6c13c15c18c20+w20(c8c12c14−c7c13c14+c6c13c15−c7c14c18−c13c14c18+c6c15c18−c7c14c20−c13c14c20+c6c15c20−c14c18c20)+w30(c7c14+c13c14−c6c15+c14c18+c14c20)i+w0(c8c12c14c18−c7c13c14c18+c6c13c15c18+c8c12c14c20−c7c13c14c20+c6c13c15c20−c7c14c18c20−c13c14c18c20+c6c15c18c20)iv42=−iw50−c9c13c15c17c19−c8c12c16c18c20+c7c13c16c18c20+w40(c7+c13+c16+c18+c20)+w30(−c8c12+c7c13+c7c16+c13c16+c7c18+c13c18+c16c18+c7c20+c13c20+c16c20+c18c20)i+w20(c8c12c16−c7c13c16+c8c12c18−c7c13c18−c7c16c18−c13c16c18+c8c12c20−c7c13c20−c7c16c20−c13c16c20−c7c18c20−c13c18c20−c16c18c20)+w0(c8c12c16c18−c7c13c16c18+c9c15c17c19+c8c12c16c20−c7c13c16c20+c8c12c18c20−c7c13c18c20−c7c16c18c20−c13c16c18c20)i |
v5=−v51v61v51=−w30c14c17+(−c6c15c17c20+c7c13c14c17−c6c13c15c17+c13c14c17c20−c8c12c14c17)w0−c14c17c20+iw20(c6c15c17−c13c14c17−c14c17c20−c7c14c17)+i(c7c13c14c17c20−c8c12c14c17c20−c6c13c15c17c20)v52=−w50+w30(c7c13+c7c16−c8c12+c7c18+c7c20+c13c20+c16c20+c13c16+c13c18+c16c18+c18c20)+w0(c8c12c16c18−c7c13c16c18+c9c15c17c19+c8c12c16c20−c7c13c16c20+c8c12c18c20−c7c13c18c20−c7c16c18c20)−iw40(c7+c13+c16+c18+c20)+iw20(c7c13c16−c8c12c16−c8c12c18+c7c13c18+c7c16c18+c13c16c18+c7c16c20+c13c16c20+c7c18c20+c13c18c20+c16c18c20−c8c12c20+c7c13c20)+i(c8c12c16c18c20−c13c16c18c20−c7c13c16c18c20+c9c13c15c17c19) |
v61=w0(c6c15c17c19−c7c14c17c19−c13c14c17c19)+i(w20c14c17c19+c8c12c14c17c19−c7c13c14c17c19+c6c13c15c17c19)v62=−w50+w30(c13c16−c8c12+c7c13+c7c16+c7c18+c13c18+c16c18+c7c20+c13c20+c16c20+c18c20)+w0(c8c12c16c18−c7c13c16c18+c8c12c16c20+c9c15c17c19+c8c12c18c20−c7c13c16c20−c7c13c18c20−c7c16c18c20−c13c16c18c20)−iw40(c7+c13+c16+c18+c20)+iw20(c7c13c16−c8c12c16−c8c12c18+c7c13c18+c7c16c18+c13c16c18−c8c12c20+c7c13c20+c7c16c20+c13c16c20+c7c18c20+c13c18c20+c16c18c20)+i(c8c12c16c18c20−c7c13c16c18c20+c9c13c15c17c19) |
v∗1=−V11V12V11=(c13+iw0)(−c5c15c17c19−(c18+iw0)(c20+iw0)(c4c15eiτ01w0−c2(c16+iw0)))V12=(−c9c15c17c19+(c7+iw0)(c16+iw0)(c18+iw0)(c20+iw0))(c13+iw0)−c8c12(c16+iw0)(c18+iw0)(c20+iw0) |
v∗2=−c3c11+iw0 |
v∗3=−V31V32V31=c8(ic4c15w20eiτ01w0+c4c15c18w0eiτ01w0+c4c15c20w0eiτ01w0−ic4c15c18c20eiτ01w0+c2w30−ic2c16w20−ic2c18w20−ic2c20w20−c2c16c18w0−c2c16c20w0−c2c18c20w0−ic5c15c17c19+ic2c16c18c20)V32=−ic7w40−ic13w40−ic16w40−ic18w40−ic20w40+c8c12w30−c7c13w30−c7c16w30−c13c16w30−c7c18w30−c13c18w30−c16c18w30−c7c20w30−c13c20w30−c16c20w30−c18c20w30−ic8c12c16w20+ic7c13c16w20−ic8c12c18w20+ic7c13c18w20+ic7c16c18w20+ic13c16c18w20−ic8c12c20w20+ic7c13c20w20+ic7c16c20w20+ic13c16c20w20+ic7c18c20w20+ic13c18c20w20+ic16c18c20w20−c8c12c16c18w0+c7c13c16c18w0−c9c15c17c19w0−c8c12c16c20w0+c7c13c16c20w0−c8c12c18c20w0+c7c13c18c20w0+c7c16c18c20w0+c13c16c18c20w0+ic9c13c15c17c19+ic8c12c16c18c20−ic7c13c16c18c20+w50 |
v∗4=−V41V42,V41=ic4w40eiτ01w0+c4c7w30eiτ01w0+c4c13w30eiτ01w0+c4c18w30eiτ01w0+c4c20w30eiτ01w0+ic4c8c12w20eiτ01w0−ic4c7c13w20eiτ01w0−ic4c7c18w20eiτ01w0−ic4c13c18w20eiτ01w0−ic4c7c20w20eiτ01w0−ic4c13c20w20eiτ01w0−ic4c18c20w20eiτ01w0+c4c8c12c18w0eiτ01w0−c4c7c13c18w0eiτ01w0+c4c8c12c20w0eiτ01w0−c4c7c13c20w0eiτ01w0−c4c7c18c20w0eiτ01w0−c4c13c18c20w0eiτ01w0−ic4c8c12c18c20eiτ01w0+ic4c7c13c18c20eiτ01w0−ic5c17c19w20−c5c7c17c19w0+c2c9c17c19w0−c5c13c17c19w0−ic5c8c12c17c19+ic5c7c13c17c19−ic2c9c13c17c19,V42=ic7w40+ic13w40+ic16w40+ic18w40+ic20w40−c8c12w30+c7c13w30+c7c16w30+c13c16w30+c7c18w30+c13c18w30+c16c18w30+c7c20w30+c13c20w30+c16c20w30+c18c20w30+ic8c12c16w20−ic7c13c16w20+ic8c12c18w20−ic7c13c18w20−ic7c16c18w20−ic13c16c18w20+ic8c12c20w20−ic7c13c20w20−ic7c16c20w20−ic13c16c20w20−ic7c18c20w20−ic13c18c20w20−ic16c18c20w20+c8c12c16c18w0−c7c13c16c18w0+c9c15c17c19w0+c8c12c16c20w0−c7c13c16c20w0+c8c12c18c20w0−c7c13c18c20w0−c7c16c18c20w0−c13c16c18c20w0−ic9c13c15c17c19−ic8c12c16c18c20+ic7c13c16c18c20−w50, |
v∗5=−V51V52V51=c4c9c15c19w0eiτ01w0−ic4c9c13c15c19eiτ01w0−c5c19w30+ic5c7c19w20−ic2c9c19w20+ic5c13c19w20+ic5c16c19w20−c5c8c12c19w0+c5c7c13c19w0−c2c9c13c19w0+c5c7c16c19w0−c2c9c16c19w0+c5c13c16c19w0+ic5c8c12c16c19−ic5c7c13c16c19+ic2c9c13c16c19,V52=ic7w40+ic13w40+ic16w40+ic18w40+ic20w40−c8c12w30+c7c13w30+c7c16w30+c13c16w30+c7c18w30+c13c18w30+c16c18w30+c7c20w30+c13c20w30+c16c20w30+c18c20w30+ic8c12c16w20−ic7c13c16w20+ic8c12c18w20−ic7c13c18w20−ic7c16c18w20−ic13c16c18w20+ic8c12c20w20−ic7c13c20w20−ic7c16c20w20−ic13c16c20w20−ic7c18c20w20−ic13c18c20w20−ic16c18c20w20+c8c12c16c18w0−c7c13c16c18w0+c9c15c17c19w0+c8c12c16c20w0−c7c13c16c20w0+c8c12c18c20w0−c7c13c18c20w0−c7c16c18c20w0−c13c16c18c20w0−ic9c13c15c17c19−ic8c12c16c18c20+ic7c13c16c18c20−w50, |
v∗6=−V61V62V61=−c4c9c15w20eiτ01w0+ic4c9c13c15w0eiτ01w0+ic4c9c15c18w0eiτ01w0+c4c9c13c15c18eiτ01w0+c5w40−ic5c7w30+ic2c9w30−ic5c13w30−ic5c16w30−ic5c18w30+c5c8c12w20−c5c7c13w20+c2c9c13w20−c5c7c16w20+c2c9c16w20−c5c13c16w20−c5c7c18w20+c2c9c18w20−c5c13c18w20−c5c16c18w20−ic5c8c12c16w0+ic5c7c13c16w0−ic2c9c13c16w0−ic5c8c12c18w0+ic5c7c13c18w0−ic2c9c13c18w0+ic5c7c16c18w0−ic2c9c16c18w0+ic5c13c16c18w0−c5c8c12c16c18+c5c7c13c16c18−c2c9c13c16c18,V62=c7w40+c13w40+c16w40+c18w40+c20w40+ic8c12w30−ic7c13w30−ic7c16w30−ic13c16w30−ic7c18w30−ic13c18w30−ic16c18w30−ic7c20w30−ic13c20w30−ic16c20w30−ic18c20w30+c8c12c16w20−c7c13c16w20+c8c12c18w20−c7c13c18w20−c7c16c18w20−c13c16c18w20+c8c12c20w20−c7c13c20w20−c7c16c20w20−c13c16c20w20−c7c18c20w20−c13c18c20w20−c16c18c20w20−ic8c12c16c18w0+ic7c13c16c18w0−ic9c15c17c19w0−ic8c12c16c20w0+ic7c13c16c20w0−ic8c12c18c20w0+ic7c13c18c20w0+ic7c16c18c20w0+ic13c16c18c20w0−c9c13c15c17c19−c8c12c16c18c20+c7c13c16c18c20+iw50. |
From
⟨q∗,q⟩=ˉq∗(0)q(0)−∫0−1∫θξ=0ˉq∗(ξ−θ)dη(θ)q(ξ)dξ=ˉq∗(0)q(0)+ˉq∗(0)τ01(0000c400000000000000000000000000000000000000000000)e−iw0τ01q(0)=ˉG[(1+v1ˉv∗1+v2ˉv∗2+v3ˉv∗3+v4ˉv∗4+v5ˉv∗5+v6ˉv∗6)+τ01e−iw0τ01c4v4] |
to make ⟨q∗,q⟩=1, we let ˉG=1(1+v1ˉv∗1+v2ˉv∗2+v3ˉv∗3+v4ˉv∗4+v5ˉv∗5+v6ˉv∗6)+τ01e−iw0τ01c4v4. And then G=1(1+ˉv1v∗1+ˉv2v∗2+ˉv3v∗3+ˉv4v∗4+ˉv5v∗5+ˉv6v∗6)+τ01eiw0τ01c4v4.
The above ˉv1 and ˉv∗1 represent the conjugate plural of v1 and v∗1 respectively and the other analogy. Next we will compute the coordinate to describe the center manifold C0 at γ=0 using the way of Hassard et al [56].
We let Ut be the solution of (6.15) at at γ=0 and define
z(t)=⟨q∗,xt⟩ W(t,θ)=Ut(θ)−2Re{z(t)q(θ)}. | (5.19) |
On the center manifold C0, we can regard W(t,θ) as
W(z,ˉz,θ)=W20(θ)z22+W11(θ)zˉz+W02(θ)ˉz22+⋯ | (5.20) |
In fact, z(t) and ˉz(t) are local coordinates for center manifold C0 in the direction of q∗ and ˉq∗. It is easy to know that W(t,θ) is real only when Ut(θ) is real. We just consider it is a real solution of (6.10), and then
˙z(t)=⟨q∗,˙Ut⟩=⟨q∗,Λ0Ut+R0Ut⟩=⟨Λ∗0q∗,Ut⟩+⟨q∗,f(0,Ut)⟩=iw0τ01z+ˉq∗(0)f(0,W(z,ˉz,θ)+2Re{z(t)q(θ)})=iw0τ01z+ˉq∗(0)f0, | (5.21) |
f0=f(0,W(z,ˉz,θ)+2Re{z(t)q(θ)}) | (5.22) |
˙z(t)=iw0τ01z+g(z,ˉz), | (5.23) |
where
g(z,ˉz)=g20z22+g11zˉz+g02ˉz22+g21z2ˉz2+⋯. | (5.24) |
The following formula can be obtained from equations (6.19) and (6.20).
Ut=W(t,θ)+2Re{z(t)q(θ)}=W20(θ)z22+W11(θ)zˉz+W02(θ)ˉz22+zq+ˉzˉq⋯=W20(θ)z22+W11(θ)zˉz+W02(θ)ˉz22+eiw0τ01θ(1,v1,v2,v3,v4,v5,v6)Tz+e−iw0τ01θ(1,ˉv1,ˉv2,ˉv3,ˉv4,ˉv5,ˉv6)Tˉz+⋯. | (5.25) |
Combined with (6.12), (6.22), (6.25), we have
g(z,ˉz)=ˉq∗(0)f0=ˉq∗(0)f(0,Ut)=ˉGτ01(1,ˉv∗1,ˉv∗2,ˉv∗3,ˉv∗4,ˉv∗5,ˉv∗6)×(∞∑i=21i![f(i)11(v∗)ϕ5(−1)i+f(i)12(y∗)ϕi2(0)+f(i)13(x∗)q∗ϕi1(0)+if(i−1)13(x∗)ϕ7(0)ϕi−11(0)]∞∑i=21i![−f(i)13(x∗)q∗ϕi1(0)−if(i−1)13(x∗)ϕ7(0)ϕi−11(0)+f(i)12(y∗)ϕi2(0)]00∞∑i=21i![f(i)51(v∗)x∗ϕi5(0)+if(i−1)51(v∗)ϕ1(0)ϕi−15(0)+f(i)52(v∗)y∗ϕi5(0)+if(i−1)52(v∗)ϕ2(0)ϕi−15(0)]∞∑i=21i!f(i)61(v∗)ϕi5(0)∞∑i=21i![f(i)71(q∗)ϕi7(0)+f(i)72(q∗)ϕi7(0)+f(i)73(q∗)p∗ϕi7(0)+if(i−1)73(q∗)ϕ6(0)ϕi−17(0)]) | (5.26) |
Comparing the coefficients with equation (6.24), we obtain
g20=ˉGτ01[f(2)13(x∗)q∗+f(2)12(y∗)v21−f(2)13(x∗)q∗ˉv∗1−f(2)12(y∗)v21ˉv∗1+e−2iτ01w0f(2)11(v∗)v24+2f(1)51(v∗)v4ˉv∗4+2f(1)52(v∗)v1v4ˉv∗4+f(2)61(v∗)v24ˉv∗5+2f(1)13(x∗)v6−2f(1)13(x∗)ˉv∗1v6+2f(1)73(q∗)v5v6ˉv∗6+f(2)71(q∗)v26ˉv∗6+f(2)72(q∗)v26ˉv∗6+f(2)73(q∗)p∗v26ˉv∗6+f(2)51(v∗)v24ˉv∗4x∗+f(2)52(v∗)v24ˉv∗4y∗] |
g11=ˉGτ01[f(2)13(x∗)q∗+f(2)12(y∗)v1ˉv∗1−f(2)13(x∗)q∗ˉv∗1−f(2)12(y∗)v1ˉv1ˉv∗1+f(2)11(v∗)v4ˉv4+f(1)51(v∗)v4ˉv∗4+f(1)52(v∗)ˉv1v4ˉv∗4+f(1)51(v∗)ˉv4ˉv∗4+f(1)52(v∗)v1ˉv4ˉv∗4+f(2)61(v∗)v4ˉv4ˉv∗5+f(1)13(x∗)v6−f(1)13(x∗)ˉv∗1v6+f(1)13(x∗)ˉv6−f(1)13(x∗)ˉv∗1ˉv6+f(1)73(q∗)ˉv5v6ˉv∗6+f(1)73(q∗)v5ˉv6ˉv∗6+f(2)71(q∗)v6ˉv6ˉv∗6+f(2)72(q∗)v6ˉv6ˉv∗6+f(2)73(q∗)p∗v6ˉv6ˉv∗6+f(2)51(v∗)v4ˉv4ˉv∗4x∗+f(2)52(v∗)v4ˉv4ˉv∗4y∗] |
g02=ˉGτ01[f(2)13(x∗)q∗+f(2)12(y∗)ˉv21−f(2)13(x∗)q∗ˉv∗1−f(2)12(y∗)ˉv21ˉv∗1+e2iτ01w0f(2)11(v∗)ˉv24+2f(1)51(v∗)ˉv4ˉv∗4+2f(1)52(v∗)ˉv1ˉv4ˉv∗4+f(2)61(v∗)ˉv24ˉv∗5+2f(1)13(x∗)ˉv6−2f(1)13(x∗)ˉv∗1ˉv6+2f(1)73(q∗)ˉv5ˉv6ˉv∗6+f(2)71(q∗)ˉv26ˉv∗6+f(2)72(q∗)ˉv26ˉv∗6+f(2)73(q∗)p∗ˉv26ˉv∗6+f(2)51(v∗)ˉv24ˉv∗4x∗+f(2)52(v∗)ˉv24ˉv∗4y∗] |
g21=ˉGτ01[f(3)13(x∗)q∗+f(3)12(y∗)v21ˉv1−f(3)13(x∗)ˉv∗1q∗−f(3)12(y∗)v21ˉv1ˉv∗1+e−iτ01w0f(3)11(v∗)v24ˉv4+f(2)51(v∗)v24ˉv∗4+f(2)52(v∗)ˉv1v24ˉv∗4+2f(2)51(v∗)v4ˉv4ˉv∗4+2f(2)52(v∗)v1v4ˉv4ˉv∗4+f(3)61(v∗)v24ˉv4ˉv∗5+2f(2)13(x∗)v6−2f(2)13(x∗)ˉv∗1v6+f(2)13(x∗)ˉv6−f(2)13(x∗)ˉv6ˉv∗1+f(2)73(q∗)ˉv5v26ˉv∗6+2f(2)73(q∗)v5v6ˉv6ˉv∗6+f(3)71(q∗)v26ˉv6ˉv∗6+f(3)72(q∗)v26ˉv6ˉv∗6+f(3)73(q∗)p∗v26ˉv6ˉv∗6+2f(2)13(x∗)q∗W111(0)−2f(2)13(x∗)q∗ˉv∗1W111(0)+2f(1)51(v∗)v4ˉv∗4W111(0)+2f(1)13(x∗)v6W111(0)−2f(1)13(x∗)v6ˉv∗1W111(0)+2f(2)12(y∗)v1W211(0)−2f(2)12(y∗)v1ˉv∗1W211(0)+2f(1)52(v∗)v4ˉv∗4W211(0)+2f(1)51(v∗)ˉv∗4W511(0)+2f(1)52(v∗)v1ˉv∗4W511(0)+2f(2)61(v∗)v4ˉv∗5W511(0)+2e−iτ01w0f(2)11(v∗)v4W511(−1)+2f(1)73(q∗)v6ˉv∗6W611(0)+2f(1)13(x∗)W711(0)−2f(1)13(x∗)ˉv∗1W711(0)+2f(1)73(q∗)v5ˉv∗6W711(0)+2f(2)71(q∗)v6ˉv∗6W711(0)+2f(2)72(q∗)v6ˉv∗6W711(0)+2f(2)73(q∗)p∗v6ˉv∗6W711(0)+f(2)13(x∗)q∗W120(0)−f(2)13(x∗)q∗ˉv∗1W120(0)+f(1)51(v∗)ˉv4ˉv∗4W120(0)+f(1)13(x∗)ˉv6W120(0)−f(1)13(x∗)ˉv∗1ˉv6W120(0)+f(2)12(y∗)ˉv1W220(0)−f(2)12(y∗)ˉv1ˉv∗1W220(0)+f(1)52(v∗)ˉv4ˉv∗4W220(0)+f(1)51(v∗)ˉv∗4W520(0)+f(1)52(v∗)ˉv1ˉv∗4W520(0)+f(2)61(v∗)ˉv4ˉv∗5W520(0)+eiτ01w0f(2)11(v∗)ˉv4W520(−1)+f(1)73(q∗)ˉv6ˉv∗6W620(0)+f(1)13(x∗)W720(0)−f(1)13(x∗)ˉv∗1W720(0)+f(1)73(q∗)ˉv5ˉv∗6W720(0)+f(2)71(q∗)ˉv6ˉv∗6W720(0)+f(2)72(q∗)ˉv6ˉv∗6W720(0)+f(2)73(q∗)p∗ˉv6ˉv∗6W720(0)+f(3)51(v∗)v24ˉv4ˉv∗4x∗+2f(2)51(v∗)v4ˉv∗4W511(0)x∗+f(2)51(v∗)ˉv4ˉv∗4W520(0)x∗+f(3)52(v∗)v24ˉv4ˉv∗4y∗+2f(2)52(v∗)v4ˉv∗4W511(0)y∗+f(2)52(v∗)ˉv4ˉv∗4W520(0)y∗]. |
Since W20(θ) and W11(θ) are unknown in g21, we will continue to solve for W20(θ) and W11(θ).
From (6.15) and (6.21) we have
˙W=˙Ut−˙z(t)q(θ)−˙ˉzˉq(θ)=Λ0Ut+R0Ut−[iw0τ01z(t)+ˉq∗(0)f0(z,ˉz)]q(θ)−[−iw0τ01z(t)+q∗(0)ˉf0(z,ˉz)]ˉq(θ)={Λ0W−2ℜ(ˉq∗(0)f0(z,ˉz)),θ∈[−1,0)Λ0W−2ℜ(ˉq∗(0)f0(z,ˉz))+f0,θ=0=Λ0W+H(z,ˉz,θ) | (5.27) |
where
H(z,ˉz,θ)=H20(θ)z22+H11(θ)zˉz+H02(θ)ˉz22+⋯. | (5.28) |
Differentiating formula (6.20) for t we can have
˙W=Wz˙z+Wˉz˙ˉz=(W20(θ)z+W11(θ)ˉz⋯)(iw0τ01z+g(z,ˉz))+(W11(θ)z+W02(θ)ˉz⋯)(−iw0τ01ˉz+ˉg(z,ˉz)). | (5.29) |
Bring (6.27) into (6.29), we obtain
˙W=Λ0(W20(θ)z22+W11(θ)zˉz+W02(θ)ˉz22+⋯)+H20(θ)z22+H11(θ)zˉz+H02(θ)ˉz22+⋯=(Λ0W20(θ)+H20(θ))z22+(Λ0W11(θ)+H11(θ))zˉz+(Λ0W02(θ)+H02(θ))ˉz22+⋯. | (5.30) |
Compare the coefficients of z2 and zˉz in (6.29) and (6.30) to obtain the following equation
(Λ0−2iw0τ01I)W20(θ)=−H20(θ), | (5.31) |
and
Λ0W11(θ)=−H11(θ), | (5.32) |
where I is unit matrix or unit transformation. From (6.27) we can get
H(z,ˉz,θ)=−ˉq∗(0)f0q(θ)−q∗(0)ˉf0ˉq(θ)=−g(z,ˉz)q(θ)−ˉg(z,ˉz)ˉq(θ)=−(g20z22+g11zˉz+g02ˉz22+⋯)q(θ)−(ˉg20ˉz22+ˉg11zˉz+ˉg02z22+⋯)ˉq(θ) | (5.33) |
for θ∈[−1,0). Comparing the corresponding coefficients of (6.28), (6.33) we can get the following equation
H20(θ)=−g20q(θ)−ˉg02ˉq(θ) | (5.34) |
H11(θ)=−g11q(θ)−ˉg11ˉq(θ). | (5.35) |
From (6.31) we can obtain
Λ0W20(θ)=2iw0τ01W20(θ)−H20(θ). | (5.36) |
According the definition of Λ0 and q(θ) and combining (6.34), then
˙W20(θ)=2iw0τ01W20(θ)+g20q(0)eiw0τ01θ+ˉg02ˉq(0)e−iw0τ01θ | (5.37) |
W20(θ)=ig20w0τ01q(0)eiw0τ01θ+iˉg023w0τ01ˉq(0)e−iw0τ01θ+N1e2iw0τ01θ | (5.38) |
where N2=(N(1)2,N(2)2,N(3)2,N(4)2,N(5)2,N(6)2,N(7)2)T is also a constant vector. Next we just need to calculate N1 and N2. From (6.31), (6.32) and the define of Λ0 the following equations can be exported.
∫0−1dη(θ)W20(θ)=2iw0τ01W20(0)−H20(0) | (5.39) |
∫0−1dη(θ)W11(θ)=−H11(0) | (5.40) |
where η(θ)=η(0,θ). From (6.27) we have
H20(0)=−g20q(0)−ˉg02ˉq(0)+τ01(f(2)13(x∗)q∗+f(2)12(y∗)v21+e−2iw0τ01f(2)11(v∗)v24+2f(1)13(x∗)v6−f(2)13(x∗)q∗−f(2)12(y∗)v21−2f(1)13(x∗)v6002f(1)51(v∗)v4+2f(1)52(v∗)v1v4+f(2)51(v∗)v24x∗+f(2)52(v∗)v24y∗f(2)61(v∗)v242f(1)73(q∗)v5v6+f(2)71(q∗)v26+f(2)72(q∗)v26+f(2)73(q∗)p∗v26) | (5.41) |
H11(0)=−g11q(0)−ˉg11ˉq(0)+τ01(f(2)13(x∗)q∗+f(2)12(y∗)v1ˉv1+f(2)11(v∗)v4ˉv4+f(1)13(x∗)v6+f(1)13(x∗)ˉv6−f(2)13(x∗)q∗−f(2)12(y∗)v1ˉv1−f(1)13(x∗)v6−f(1)13(x∗)ˉv600f(1)51(v∗)v4+f(1)52(v∗)ˉv1v4+f(1)51(v∗)ˉv4+f(1)52(v∗)v1ˉv4+f(2)51(v∗)v4ˉv4x∗+f(2)52(v∗)v4ˉv4y∗f(2)61(v∗)v4ˉv4f(1)73(q∗)ˉv5v6+f(1)73(q∗)v5ˉv6+f(2)71(q∗)v6ˉv6+f(2)72(q∗)v6ˉv6+f(2)73(q∗)p∗v6ˉv6) | (5.42) |
Substituting (6.38) and (6.41) into (6.39), we obtain
∫0−1dη(θ)(ig20w0τ01q(0)eiw0τ01θ+iˉg023w0τ01ˉq(0)e−iw0τ01θ+N1e2iw0τ01θ)=2iw0τ01(ig20w0τ01q(0)+iˉg023w0τ01ˉq(0)+N1)−H20(0). | (5.43) |
And then since iw0τ01 is the eigenvalues of Λ0, which corresponding eigenvector q(0). Furthermore, From (6.43) to
(2iw0τ01I−∫0−1e2iw0τ01θdη(θ))N1=τ01(f(2)13(x∗)q∗+f(2)12(y∗)v21+e−2iw0τ01f(2)11(v∗)v24+2f(1)13(x∗)v6−f(2)13(x∗)q∗−f(2)12(y∗)v21−2f(1)13(x∗)v6002f(1)51(v∗)v4+2f(1)52(v∗)v1v4+f(2)51(v∗)v24x∗+f(2)52(v∗)v24y∗f(2)61(v∗)v242f(1)73(q∗)v5v6+f(2)71(q∗)v26+f(2)72(q∗)v26+f(2)73(q∗)p∗v26) | (5.44) |
Similarly, we can get
∫0−1dη(θ)N2=−τ01(f(2)13(x∗)q∗+f(2)12(y∗)v1ˉv1+f(2)11(v∗)v4ˉv4+f131v6zZ+f(1)13(x∗)ˉv6−f(2)13(x∗)q∗−f(2)12(y∗)v1ˉv1−f(1)13(x∗)v6−f(1)13(x∗)ˉv600f(1)51(v∗)v4+f(1)52(v∗)ˉv1v4+f(1)51(v∗)ˉv4+f(1)52(v∗)v1ˉv4+f(2)51(v∗)v4ˉv4x∗+f(2)52(v∗)v4ˉv4y∗f(2)61(v∗)v4ˉv4f(1)73(q∗)ˉv5v6+f(1)73(q∗)v5ˉv6+f(2)71(q∗)v6ˉv6+f(2)72(q∗)v6ˉv6+f(2)73(q∗)p∗v6ˉv6) | (5.45) |
Finally, according to the definition of η(θ), we can solve N1, N2 and g21. Besides we can also get the following values
C1(0)=i2w0τ01(g11g20−2|g11|2−|g02|23)+g212,μ2=−Re{C1(0)}Re{λ′(τ01)},T2=−Im{C1(0)}+μ2Im{λ′(τ01)}w0τ01,β2=2Re{C1(0)}. |
From the above discussion we can get the following results:
(1) The direction of Hopf bifurcation is determined by μ2 : if μ2>0 (resp.μ2<0), then the Hopf bifurcation is supercritical (resp. subcritical) and the bifurcating periodic solutions exist for τ>τ01 (resp.τ<τ01);
(2) The stability of the bifurcating periodic solutions is depended on β2, the bifurcating periodic solutions in the center manifold are stable (resp. unstable) for β2<0 (resp. β2>0);
(3) The period of the bifurcating periodic solutions is determined by T2 :the period increases if T2>0 (resp. decreases T2<0).
[1] | World Health Organization,World malaria report, 2016. Available from: http://www.who.int/ malaria/publications/world-malaria-report-2016/en/. |
[2] | CDC. malaria, malaria worldwide, 2018. Available from: https://www.cdc.gov/malaria/ about/biology/index.html. |
[3] | R. Ross, The Prevention of Malaria, 2nd edition, Murray, London, 1911. |
[4] | G. Macdonald, The epidemiology and control of malaria, Oxford University press, London, 1957. |
[5] | S. A. Gourley, R. Liu and J. Wu, Some vector borne diseases with structured host populations: Extinction and spatial spread, SIAM J. Appl. Math., 67 (2007), 408–433. |
[6] | Fatmawati and H. Tasman, An optimal control strategy to reduce the spread of malaria resistance, Math. Biosci., 262 (2015), 73–79. |
[7] | S. Ruan, D. Xiao and J. C. Beier, On the delayed Ross-Macdonald model for malaria transmission, B. Math. Biol., 70 (2008), 1098–1114. |
[8] | L. M. Cai, X. Z. Li, B. Fang, et al., Global properties of vector–host disease models with time delays, J. Math. Biol., 74 (2017), 1397–1423. |
[9] | J. Xu and Y. Zhou, Hopf bifurcation and its stability for a vector-borne disease model with delay and reinfection, Appl. Math. Model., 40 (2016), 1685–1702. |
[10] | Y. Lou and X. Q. Zhao, A climate-based malaria transmission model with structured vector population, SIAM J. Appl. Math., 70 (2010), 2023–2044. |
[11] | Y. X. Dang, Z. P. Qiu, X. Z. Li, et al., Global dynamics of a vector-host epidemic model with age of infection, Math. Biosci. Eng., 14 (2017), 1159–1186. |
[12] | D. Gao and S. Ruan, A multipatch malaria model with logistic growth populations, SIAM J. Appl. Math., 72 (2012), 819–841. |
[13] | J. Li, Y. Zhao and S. Li, Fast and slow dynamics of malaria model with relapse, Math. Biosci., 246 (2013), 94–104. |
[14] | B. Cao, H. F. Huo and H. Xiang, Global stability of an age-structure epidemic model with imperfect vaccination and relapse, Physica A, 486 (2017), 638–655. |
[15] | X. Duan, S. Yuan and X. Li, Global stability of an SVIR model with age of vaccination, Appl. Math. Comput., 226 (2014), 528–540. |
[16] | H. Inaba and H. Sekine, A mathematical model for chagas disease with infection-age-dependent infectivity, Math. Biosci., 190 (2004), 39–69. |
[17] | L.Wang, Z. Liu and X. Zhang, Global dynamics for an age-structured epidemic model with media impact and incomplete vaccination, Nonlinear Anal. RWA, 32 (2016), 136–158. |
[18] | H. Chen, W. Wang, R. Fu, et al., Global analysis of a mathematical model on malaria with competitive strains and immune responses, Appl. Math. Comput., 259 (2015), 132–152. |
[19] | H. Xiang, Y. L. Tang and H. F. Huo, A viral model with intracellular delay and humoral immunity, B. Malays. Math. Sci. So., 40 (2017), 1011–1023. |
[20] | H. F. Huo, F. F. Cui and H. Xiang, Dynamics of an SAITS alcoholism model on unweighted and weighted networks, Physica A, 496 (2018), 249–262. |
[21] | H. Xiang, Y. Y. Wang and H. F. Huo, Analysis of the binge drinking models with demographics and nonlinear infectivity on networks, J. Appl. Anal. Comput., 8 (2018), 1535–1554. |
[22] | Y. Cai, J. Jiao, Z. Gui, et al., Environmental variability in a stochastic epidemic model, Appl. Math. Comput., 329 (2018), 210–226. |
[23] | X. B. Zhang, Q. H. Shi, S. H. Ma, et al., Dynamic behavior of a stochastic SIQS epidemic model with Lévy jumps, Nonlinear Dynam., 93 (2018), 1481–1493. |
[24] | Z. Du and Z. Feng, Existence and asymptotic behaviors of traveling waves of a modified vectordisease model, Commun. pur. appl. anal., 17 (2018), 1899–1920. |
[25] | Malaria vaccine. Available from: https://en.wikipedia.org/wiki/Malaria_vaccine. |
[26] | G. Webb, Theory of nonlinear age-dependent population dynamics, marcel dekker, New York, 1985. |
[27] | M. Iannelli, Mathematical theory of age-structured population dynamics, Giadini Editori e Stampatori, Pisa, 1994. |
[28] | H. L. Smith and H. R. Thieme, Dynamical systems and population persistence, American Mathematical Society, Providence, 2011. |
[29] | P. Magal and X. Q. Zhao, Global attractors and steady states for uniformly persistent dynamical systems, SIAM J. Appl. Math., 37 (2005), 251–275. |
[30] | E. M. C. D'Agata, P. Magal, S. Ruan, et al., Asymptotic behavior in nosocomial epidemic models with antibiotic resistance, Differ. Integral Equ., 19 (2006), 573–600. |
[31] | C. J. Browne and S. S. Pilyugin, Global analysis of age-structured within-host virus model, Discrete Cont. Dyn. S. B, 18 (2013), 1999–2017. |
[32] | H. M. Wei, X. Z. Li and M. Martcheva, An epidemic model of a vector-borne disease with direct transmission and time delay, J. Math. Anal. Appl., 342 (2008), 895–908. |
[33] | J. Wang, J. Lang and X. Zou, Analysis of an age structured hiv infection model with virus-to-cell infection and cell-to-cell transmission, Nonlinear Anal. RWA, 34 (2017), 75–96. |
[34] | J. K. Hale and P. Waltman, Persistence in infinite-dimensional systems, SIAM J. Appl. Math., 20 (1989), 388–395. |
[35] | Z. K. Guo, H. F. Huo, X. Hong, Bifurcation analysis of an age-structured alcoholism model, J. Biol. Dynam., 12 (2018), 1009–1033. |
1. | Ján Eliaš, Cicely K. Macnamara, Mathematical Modelling of p53 Signalling during DNA Damage Response: A Survey, 2021, 22, 1422-0067, 10590, 10.3390/ijms221910590 |
Variable Symbol | Description | Reference |
x | The concentration of MDM2, which is evenly distributed in the nucleus and cytosol | [36] |
y | The concentration of phosphorylated MDM2, which is mainly distributed in the nucleus | [36] |
z | The concentration of MDM2-ARF complex | [36] |
u | The concentration of MDM2p-ARF complex | [36] |
v | The concentration of P53 in the nucleus | [36] |
p | The concentration of PTEN, which is a P53 target and inhibits Akt activation by dephosphorylating PIP3 | [36] |
q | The concentration of active Akt | [36] |
Parameter | Description | value | Reference |
S | serum concentration; it is proportional to the concentration of GFs; GFs induce mitogenic signals | 0.035 | [36] |
a | Free ARF protein(P14ARF) | 0.03 | [36] |
k1 | Rate constant of MDM2 expression induced by serum(is proportional to the concentration of GFs) | 0.15μM/h | [24,45] |
k2 | Rate cconstant of P53-mediated expression of Mdm2 | 0.9μM/h | [24] |
k3 | Rate constant for Mdm2p dephosphorylation | 12μM/h | [36] |
k4 | Rate constant for Mdm2/ARF ossociation | 43(μMh) | [36] |
k5 | Rate constant Mdm2 phosphorylation mediated by Akt | 56/h | [36] |
k6 | Rate constant for Mdm2p/ARF ossociation | 10/(μMh) | [36] |
k7 | Basal rate constant of P53 expression | 8μ/h | [25,44] |
k8 | Rate constant for Mdm2p-mediated P53 degradation | 5/h | [36] |
k9 | Rate constant for Mdm2p-mediated P53 degradation | 18/h | [36] |
k10 | Basal expression rate of PTEN | 0.05μM/h | [36] |
k11 | Rate constant of P53-dependent synthesis of PTEN | 0.7μM/h | [36] |
k12 | Rate constant for Akt phosphorylation induced by growth factors | 12.9μM/h | [36] |
k13 | Rate constant for Akt dephosphorylation | 9.4μM/h | [46,47] |
k14 | Rate constant for PTEN Cinduced Akt dephosphorylation; refering to the dephosphorylation of PIP3 by PTEN | 30/h | [36] |
K1 | Michaelis constant for Mdm2 expression triggered by growth factors | 0.0045 | [36] |
K2 | Hill constant for P53-induced expression of Mdm2 | 0.5μM | [36] |
K3 | Michaelis constant for Mdm2p dephosphorylation | 0.081μM | [36] |
K4 | Michaelis constant for Akt-mediated phosphorylation of Mdm2 | 0.5μM | [36] |
K5 | Michaelis constant for Mdm2-mediated P53 degradation | 0.5μM | [36] |
K6 | Michaelis constant for Mdm2p-mediated P53 degradation | 0.1μM | [36] |
K7 | Hill constant for P53-induced expression of PTEN greater than that of Mdm2 | 1μM | [36] |
K8 | Michaelis constant for Akt activation triggered by growth factor | 0.0147 | [36] |
K9 | Threshold of the total enzyme amount for Akt activation | 0.35μM | [36] |
K10 | Michaelis constant for Akt dephosphorylation | 0.2μM | [36] |
K11 | Michaelis constant for PTEN-induced Akt dephosphorylation | 0.6μM | [36] |
D1 | Rate constant for Mdm2-ARF disassociation | 6/h | [36] |
D2 | Rate constant for Mdm2p-ARF disassociation | 24/h | [36] |
d1 | Half-life of Mdm2 is about 90 min | 0.5/h | [36] |
d2 | Degradation rate of Mdm2p | 0.1/h | [36] |
d3 | Degradation rate of Mdm2-ARF complex | 0.6/h | [36] |
d4 | Degradation rate of Mdm2p-ARF complex | 0.6/h | [36] |
d5 | Half-life of P53 is about 5-20 min | 3.6/h | [36] |
d6 | Degradation rate of PTEN | 0.5/h | [36] |
A | total amount of Akt, which is much more than active Akt and is considered a constant | 2.5 | [36] |
n1 | Hill coefficient of P53-dependent expression of Mdm2 | 4 | [36] |
n2 | Hill coefficient of P53-dependent synthesis of PTEN | 3 | [36] |
τ1 | Time delay of P53 transcription for Mdm2 | 30–75min | estimate |
τ2 | Time delay of P53 transcription for PTEN | 10–15min | estimate |
Variable Symbol | Description | Reference |
x | The concentration of MDM2, which is evenly distributed in the nucleus and cytosol | [36] |
y | The concentration of phosphorylated MDM2, which is mainly distributed in the nucleus | [36] |
z | The concentration of MDM2-ARF complex | [36] |
u | The concentration of MDM2p-ARF complex | [36] |
v | The concentration of P53 in the nucleus | [36] |
p | The concentration of PTEN, which is a P53 target and inhibits Akt activation by dephosphorylating PIP3 | [36] |
q | The concentration of active Akt | [36] |
Parameter | Description | value | Reference |
S | serum concentration; it is proportional to the concentration of GFs; GFs induce mitogenic signals | 0.035 | [36] |
a | Free ARF protein(P14ARF) | 0.03 | [36] |
k1 | Rate constant of MDM2 expression induced by serum(is proportional to the concentration of GFs) | 0.15μM/h | [24,45] |
k2 | Rate cconstant of P53-mediated expression of Mdm2 | 0.9μM/h | [24] |
k3 | Rate constant for Mdm2p dephosphorylation | 12μM/h | [36] |
k4 | Rate constant for Mdm2/ARF ossociation | 43(μMh) | [36] |
k5 | Rate constant Mdm2 phosphorylation mediated by Akt | 56/h | [36] |
k6 | Rate constant for Mdm2p/ARF ossociation | 10/(μMh) | [36] |
k7 | Basal rate constant of P53 expression | 8μ/h | [25,44] |
k8 | Rate constant for Mdm2p-mediated P53 degradation | 5/h | [36] |
k9 | Rate constant for Mdm2p-mediated P53 degradation | 18/h | [36] |
k10 | Basal expression rate of PTEN | 0.05μM/h | [36] |
k11 | Rate constant of P53-dependent synthesis of PTEN | 0.7μM/h | [36] |
k12 | Rate constant for Akt phosphorylation induced by growth factors | 12.9μM/h | [36] |
k13 | Rate constant for Akt dephosphorylation | 9.4μM/h | [46,47] |
k14 | Rate constant for PTEN Cinduced Akt dephosphorylation; refering to the dephosphorylation of PIP3 by PTEN | 30/h | [36] |
K1 | Michaelis constant for Mdm2 expression triggered by growth factors | 0.0045 | [36] |
K2 | Hill constant for P53-induced expression of Mdm2 | 0.5μM | [36] |
K3 | Michaelis constant for Mdm2p dephosphorylation | 0.081μM | [36] |
K4 | Michaelis constant for Akt-mediated phosphorylation of Mdm2 | 0.5μM | [36] |
K5 | Michaelis constant for Mdm2-mediated P53 degradation | 0.5μM | [36] |
K6 | Michaelis constant for Mdm2p-mediated P53 degradation | 0.1μM | [36] |
K7 | Hill constant for P53-induced expression of PTEN greater than that of Mdm2 | 1μM | [36] |
K8 | Michaelis constant for Akt activation triggered by growth factor | 0.0147 | [36] |
K9 | Threshold of the total enzyme amount for Akt activation | 0.35μM | [36] |
K10 | Michaelis constant for Akt dephosphorylation | 0.2μM | [36] |
K11 | Michaelis constant for PTEN-induced Akt dephosphorylation | 0.6μM | [36] |
D1 | Rate constant for Mdm2-ARF disassociation | 6/h | [36] |
D2 | Rate constant for Mdm2p-ARF disassociation | 24/h | [36] |
d1 | Half-life of Mdm2 is about 90 min | 0.5/h | [36] |
d2 | Degradation rate of Mdm2p | 0.1/h | [36] |
d3 | Degradation rate of Mdm2-ARF complex | 0.6/h | [36] |
d4 | Degradation rate of Mdm2p-ARF complex | 0.6/h | [36] |
d5 | Half-life of P53 is about 5-20 min | 3.6/h | [36] |
d6 | Degradation rate of PTEN | 0.5/h | [36] |
A | total amount of Akt, which is much more than active Akt and is considered a constant | 2.5 | [36] |
n1 | Hill coefficient of P53-dependent expression of Mdm2 | 4 | [36] |
n2 | Hill coefficient of P53-dependent synthesis of PTEN | 3 | [36] |
τ1 | Time delay of P53 transcription for Mdm2 | 30–75min | estimate |
τ2 | Time delay of P53 transcription for PTEN | 10–15min | estimate |