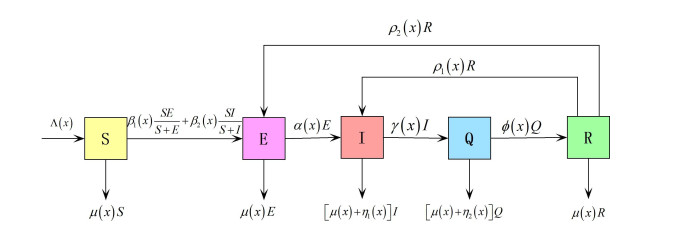
In this paper we introduce a method of global exponential attractor in the reaction-diffusion epidemic model in spatial heterogeneous environment to study the spread trend and long-term dynamic behavior of the COVID-19 epidemic. First, we prove the existence of the global exponential attractor of general dissipative evolution systems. Then, by using the existence theorem, the global asymptotic stability and the persistence of epidemic are discussed. Finally, combine with the official data of the COVID-19 and the national control strategy, some numerical simulations on the stability and global exponential attractiveness of the COVID-19 epidemic are given. Simulations show that the spread trend of the epidemic is in line with our theoretical results, and the preventive measures taken by the Chinese government are effective.
Citation: Cheng-Cheng Zhu, Jiang Zhu. Spread trend of COVID-19 epidemic outbreak in China: using exponential attractor method in a spatial heterogeneous SEIQR model[J]. Mathematical Biosciences and Engineering, 2020, 17(4): 3062-3087. doi: 10.3934/mbe.2020174
[1] | Cheng-Cheng Zhu, Jiang Zhu, Xiao-Lan Liu . Influence of spatial heterogeneous environment on long-term dynamics of a reaction-diffusion SVIR epidemic model with relaps. Mathematical Biosciences and Engineering, 2019, 16(5): 5897-5922. doi: 10.3934/mbe.2019295 |
[2] | Fen-fen Zhang, Zhen Jin . Effect of travel restrictions, contact tracing and vaccination on control of emerging infectious diseases: transmission of COVID-19 as a case study. Mathematical Biosciences and Engineering, 2022, 19(3): 3177-3201. doi: 10.3934/mbe.2022147 |
[3] | Ahmed Alshehri, Saif Ullah . A numerical study of COVID-19 epidemic model with vaccination and diffusion. Mathematical Biosciences and Engineering, 2023, 20(3): 4643-4672. doi: 10.3934/mbe.2023215 |
[4] | Ziqiang Cheng, Jin Wang . Modeling epidemic flow with fluid dynamics. Mathematical Biosciences and Engineering, 2022, 19(8): 8334-8360. doi: 10.3934/mbe.2022388 |
[5] | Hamdy M. Youssef, Najat A. Alghamdi, Magdy A. Ezzat, Alaa A. El-Bary, Ahmed M. Shawky . A new dynamical modeling SEIR with global analysis applied to the real data of spreading COVID-19 in Saudi Arabia. Mathematical Biosciences and Engineering, 2020, 17(6): 7018-7044. doi: 10.3934/mbe.2020362 |
[6] | Tao Chen, Zhiming Li, Ge Zhang . Analysis of a COVID-19 model with media coverage and limited resources. Mathematical Biosciences and Engineering, 2024, 21(4): 5283-5307. doi: 10.3934/mbe.2024233 |
[7] | Yongli Cai, Yun Kang, Weiming Wang . Global stability of the steady states of an epidemic model incorporating intervention strategies. Mathematical Biosciences and Engineering, 2017, 14(5&6): 1071-1089. doi: 10.3934/mbe.2017056 |
[8] | Jiangbo Hao, Lirong Huang, Maoxing Liu, Yangjun Ma . Analysis of the COVID-19 model with self-protection and isolation measures affected by the environment. Mathematical Biosciences and Engineering, 2024, 21(4): 4835-4852. doi: 10.3934/mbe.2024213 |
[9] | Pengfei Liu, Yantao Luo, Zhidong Teng . Role of media coverage in a SVEIR-I epidemic model with nonlinear incidence and spatial heterogeneous environment. Mathematical Biosciences and Engineering, 2023, 20(9): 15641-15671. doi: 10.3934/mbe.2023698 |
[10] | Ping Yan, Gerardo Chowell . Modeling sub-exponential epidemic growth dynamics through unobserved individual heterogeneity: a frailty model approach. Mathematical Biosciences and Engineering, 2024, 21(10): 7278-7296. doi: 10.3934/mbe.2024321 |
In this paper we introduce a method of global exponential attractor in the reaction-diffusion epidemic model in spatial heterogeneous environment to study the spread trend and long-term dynamic behavior of the COVID-19 epidemic. First, we prove the existence of the global exponential attractor of general dissipative evolution systems. Then, by using the existence theorem, the global asymptotic stability and the persistence of epidemic are discussed. Finally, combine with the official data of the COVID-19 and the national control strategy, some numerical simulations on the stability and global exponential attractiveness of the COVID-19 epidemic are given. Simulations show that the spread trend of the epidemic is in line with our theoretical results, and the preventive measures taken by the Chinese government are effective.
Many practical problems in real life such as epidemiology [1,2,3,4,5,6,7,8,9] and biology [10,11,12,13] can be solved by mathematical modeling. Among them, epidemiology is an important research area. The AIDS, the recent SARS, MERS, Ebola virus and outbursts of diseases such as the Zika virus are events of concern and interest to many people. During the Spring Festival of 2020, COVID-19 epidemic has spread rapidly in China, centered on Wuhan. The diffusive speed of the epidemic was unexpected, and the Chinese government quickly blocked the traffic of Wuhan to control the spread of the epidemic.
Researchers have established many types of epidemic model with diffusion. Some authors specifically to discuss the global stability of the spread of diseases [14,15,16,17], others study the traveling wave solutions about reaction-diffusion models [7,8,9,18].
The spread and diffusion of epidemic disease are geographically dependent. The extent of disease transmission is different under different conditions such as altitudes, humidity and temperature. Therefore, it is very necessary to consider spatial heterogeneity in epidemiological models. In 2008, Allen et al. [19] proposed a diffusive susceptible–infected–susceptible (SIS) model with space-dependent, studied the existence, uniqueness, stability of the disease-free equilibrium and discussed the asymptotic behavior of the unique endemic equilibrium as dS approaches zero. More precisely, their results implied that if the spatial environment can be modified to include low-risk sites and the movement of susceptible individuals can be restricted, then it may be possible to eliminate the infectious disease. In some subsequent work, Peng et al. [20,21,22] studied the asymptotic profiles, global stability, the effects of epidemic risk and population movement for the Allen's model. In 2018, Li et al. [23] and Tong et al. [24] concerned different reaction–diffusion SIS epidemic models in a similar method, they showed the global stability of the positive constant equilibria. For spatial heterogeneous systems, the authors discussed the existence of non-constant endemic equilibrium and studied the asymptotic profile of endemic equilibria which is determined if the diffusion rate of the susceptible or infected population at 0 or ∞. In 2019, Song et al. [25] proposed an SEIRS (Susceptible-Exposed-Infected-Recovery-Susceptible) reaction-diffusion model, where the disease transmission and recovery rates are spatially heterogeneous. They investigated the asymptotic profile of the endemic equilibrium when the diffusion rate of the susceptible individuals is small. However, we found that in the above literature, the authors have not considered the global asymptotic stability of the endemic equilibrium in the spatial heterogeneous environment.
Many diseases such as typhoid, malaria, rabies, hepatitis, AIDS, etc. cannot be completely cured at one time. These diseases will recur or relapse after treatment. Relapse, as the name implies, is a disease in the initial healing or remission phase, due to certain inducements to cause the disease to recur or relapse. Therefore, the effect of spatial factors on relapse should be taken into account. In [26], we discussed a class of reaction-diffusion SVIR (Susceptible-Vaccinated-Infected-Recovery) model with relapse and a varying external source in spatial heterogeneous environment as follows:
{∂S∂t=dSΔS+Λ(x)−β1(x)SIS+I−[μ(x)+α(x)]S, x∈Ω,t>0,∂V∂t=dVΔV+α(x)S−β2(x)VIV+I−μ(x)V, x∈Ω,t>0,∂I∂t=dIΔI+β1(x)SIS+I+β2(x)VIV+I+ρ(x)R−[μ(x)+η1(x)+ϕ(x)]I, x∈Ω,t>0,∂R∂t=dRΔR+ϕ(x)I−[ρ(x)+μ(x)+η2(x)]R, x∈Ω,t>0,∂S∂n=∂V∂n=∂I∂n=∂R∂n=0, x∈∂Ω,t>0,S(x,0)=S0(x),V(x,0)=V0(x),I(x,0)=I0(x),R(x,0)=R0(x), x∈Ω. | (1.1) |
We studied the long-term dynamic behavior of this model by means of global exponential attractor theory and gradient flow method, but the diffusion coefficient dS,dV,dI and dR are constant number.
The density of the population can also lead to the differences in the diffusion rate of disease. For example, the recent spread of the novel coronavirus pneumonia epidemic is closely related to population density and large-scale population movement during the Spring Festival. Hence, the diffusion coefficient should be dependent on the spatial position. In this article we focus on the effects of spatial heterogeneity on diffusion. We will apply the exponential attractor method, operator semigroup theory and combine the gradient flow method to study the long-term dynamic behavior of the dissipative evolution equation. Exponential attractors can be constructed for dissipative systems which possesses a certain kind of smoothing property. And the authors in [27] discuss the robustness of exponentially k-dissipative dynamical systems with perturbed parameters. In this manuscript, we construct an SEIQR (Susceptible-Exposed-Infected-Quarantined-Recovery) epidemic reaction-diffusion model with relapse and a varying external source in spatially heterogeneous environment. All coefficients of this model are spatially heterogeneous, including the diffusion coefficient. Since this model is difficult to construct Lyapunov function, we will introduce the global attractor method, and give the existence condition and existence theorem of the global exponential attractor for the general dissipative evolution equation. Apply this existence theorem of the global exponential attractor and gradient flow method, the global stability of the disease-free equilibrium of the model and the persistence of the endemic equilibrium are discussed. Due to the role of spatial heterogeneity, especially the effect of relapse in spatial heterogeneity, the usual basic reproduction number R0 has not been able to fully describe the spread of disease, but rather needs to be described by a new threshold such as eigenvalues. Finally, with the daily data on the COVID-19 published by the Chinese Health and Welfare Commission, we find that the actual spread and our theoretical results are moderate by conducting a large number of numerical simulations. Through numerical simulation, we find that the most effective way to control the spread of the epidemic is to control the contact between susceptible persons and exposed patients. Limiting contact between susceptible and infected people can only slightly reduce the number of infected people and delay the onset of time.
The organization of this paper is as follows. In Section 2, we present the existence theorem of global exponential attractor for the general dissipative evolution equation, it plays an important role in studying the global exponential attractor method of epidemic model in spatially heterogeneous environment. In Section 3, we first construct a reaction-diffusion SEIQR model with relapse and spatial heterogeneous under some assumption and prove the existence of the positive solutions. Secondly, we give the uniform boundedness and the existence of global solutions to our system. Third, we discuss the global stability and the persistence of the infection disease by using the existence theorem of global exponential attractor in Section 2. That is, we prove that the non-constant disease-free equilibrium is globally asymptotically stable when λ∗<0 and the epidemic disease is persisting uniformly when λ∗>0, where λ∗ is a threshold. In Section 4, we simulate the spread trend and the impact on the spatial heterogeneous environment of the COVID-19 epidemic by the official data. In Section 5, we give our conclusions and some discussions.
To study the global asymptotic stability of complex models which Lyapunov functions are difficult to construct, in this Section, we will introduce the global exponential attractor method. Hence, before discussing the global dynamics of the reaction-diffusion SEIQR model with relapse and spatial heterogeneous in detail, we need to recall some concept and existing results of exponential attractor.
Definition 2.1. ([27,Definition 1.1]) Let X be a Banach space, {Q(t)}t≥0 be a continuous semigroup on X. A compact set A∗⊂X is a global exponential attractor for Q(t) if
(1) it has finite fractal dimension,
(2) it is positive invariant, i.e. for arbitrary t≥0, Q(t)A∗⊂A∗,
(3) it attracts exponentially any bounded subsets of X, i.e., for any bounded subset B⊂X, there exist constant C(‖B‖X)>0 and α>0, such that
dist(Q(t)B,A∗)≤C(‖B‖X)e−αt, ∀t≥0. |
If A∗ only satisfies condition (2) and condition (3), we call it the global exponential attract set.
Definition 2.2. ([28]) Let X be a compact, connected subset of a Hilbert space H. Let Qs:X→X be a Lipschitz continous map. We call that Qs has squeezing property in X, if for some δ in (0,14) there exists an orthogonal projection P=P(δ) of rank equal to N0(δ) such that: for every u and v in X, either
‖Qsu−Qsv‖H≤δ‖u−v‖H, |
or
‖(I−P)(Qsu−Qsv)‖H≤‖P(Qsu−Qsv)‖H. |
Theorem 2.3. ([28]) Let Qs:X→X be a Lipschitz continous map and Qs have squeezing property in X, then Qs has an exponential fractal attractor A∗, moreover
dF(A∗)≤max{α(X),N0} |
where α(X)=logK0log1θ, θ∈(2δ,1) and K0 is a constant with respect to X.
In the following, we prove the existence of global exponential attractor for a class of nonlinear evolution equations.
{∂u∂t=Lu+G(u),u(0)=φ. | (2.1) |
Let H1,H are Hilbert space, H1⊂H is dense and H1↪H is compact. L:H1→H is a compact symmetrical sectorial operator and all eigenvalues of L are
0>λ1≥λ2≥...≥λk>..., λk→−∞ (k→∞), | (2.2) |
G:H1→H is continuous.
Definition 2.4. ([29,Definition A.2.1]) A mapping L+G:H1→H is called a gradient-type operator if there exists a C1 functional F:H1→R1 and for some constant C0>0 such that DF(u)⊂H for every u∈Hβ for some β≤1, and
⟨DF(u),Lu+G(u)⟩H≤−C0‖DF(u)‖2H, ∀u∈H1, |
DF(u0)=0⇔Lu0+G(u0)=0. |
In this case, F is called the energy functional of L+G, where Hβ is the fractional power subspace generated by the sectorial operator L.
Lemma 2.5. Assume that condition (2.2) holds, and there exists C1,C2>0, such that ∀φ∈H1, the solution u=u(t,φ) of system (2.1) satisfies condition
⟨Lu+G(u),u⟩H≤−C1‖u‖2H12+C2, | (2.3) |
where H12 is the fractional dimension subspace generated by the sectorial operator L. G(u) is locally Lipschitz with respect to u. Then the system (2.1) has a global solution and exists a invariant set which exponential attracts any bounded set under the H-norm.
Proof. By the assumption of Lemma 2.5 and G is locally Lipschitz with respect to u, if ∀φ∈H1, it follows from [30,Theorem 11.3.5] that the system (2.1) has a global solution. It means that system (2.1) generates a operator semigroup Q(t) and Q(t)φ=u(t,φ).
We prove that for any bounded set B⊂H1, there exists a invariant set BR⊂H for the operator semigroup Q(t). It follows from the inner product of Eq (2.1) in H with u and by using the condition (2.3), we have that
12ddt⟨u,u⟩H=⟨ut,u⟩H=⟨Lu+G(u),u⟩H≤−C1‖u‖2H12+C2, |
since H12↪H, so there exists C3>0, such that
‖u‖H12≥C3‖u‖H, ∀u∈H12. |
Hence,
12ddt‖u‖2H≤−C1C23‖u‖2H+C2, |
By Gronwall's inequality in differential form [26,Lemma 2.2], we obtain that
‖u‖2H≤e−αt‖φ‖2H+2C2α(1−e−αt), |
where α=2C1C23. It implies if R2>2C2α,φ∈BR, then
‖u‖2H≤e−αtR2+2C2α(1−e−αt)<R2. |
Therefore, for any t≥0, there has u(t,φ)⊂BR. Then, we have that BR⊂H is the invariant set.
Lemma 2.6. Assume H is a Hilbert space, L:H1→H is a injective and compact symmetrical sectorial operator and G(u) is Lipschitz with respect to u, there exists a time t∗>0, such that the operator Q∗=Q(t∗) satisfies the squeezing property on δ<18 in BR, and there exists a exponential attractor A∗ satisfies dF(A∗)≤max{α(BR),N0}.
Proof. Since L:H1→H is a injective and compact symmetrical sectorial operator, so the eigenvectors {ej}j∈N corresponding to the eigenvalues {λj}j∈N are the complete orthonormal basis of H, that is for any u,v∈H, u,v can be represented as follows
u=∞∑k=1akek,‖u‖2H=∞∑k=1a2k,v=∞∑k=1bkek,‖v‖2H=∞∑k=1b2k. |
Then, for any ˜θ∈(0,12], there exists a constant c>0, such that c|λ1|˜θ>1, hence,
‖u−v‖H=(∑k(ak−bk)2)12≤c|λ1|˜θ(∑k(ak−bk)2)12=c(∑kλ2˜θ1(ak−bk)2)12≤c(∑kλ2˜θk(ak−bk)2)12≤c‖L˜θ(u−v)‖H. |
This imples that
‖G(t,u)−G(t,v)‖H≤ˆL⋅‖u−v‖H≤cˆL⋅‖L˜θ(u−v)‖H. | (2.4) |
Since H1⊂H is dense and H1↪H is compact, according to the properties of the fractional dimension space, BR is compact in H. Combine Lemma 2.5, conditions (2.4) and applying [28,Proposition 3.1] on BR, we can get that there exists a time t∗>0, such that the operator Q∗=Q(t∗) satisfies the squeezing property on δ<18 in BR. And there exists a exponential attractor A∗ satisfies dF(A∗)≤max{α(BR),N0}.
Next we give the existence theorem of the global exponential attractor for the general dissipative evolution equation.
Theorem 2.7. Under the hypothesis of Lemma 2.5 and Lemma 2.5 holds, then the flow {Q(t)}t≥0 generated by (2.1) admits an global exponential attractor A∗=∪0≤t≤t∗Q(t)A∗, and its fractional dimension can be estimated as
dF(A∗)≤dF(A∗)+1. |
Proof. For any T>0, let F(t,u)=Q(t)u:[0,T]×A∗. We verify that F(t,u) is Lipschitz continuous. Since L is the infinitesimal generator of T(t)=etL and A∗ is compact, we have that
limt→0T(t)φ−φt=Lφ, ∀φ∈A∗. |
This shows that T(t)φ−φt−Lφ is continuous on the compact set [0,T]×A∗, thus, it is bounded on [0,T]×A∗, that is, there exists a constant ϵ>0, such that
‖T(t)φ−φt−Lφ‖H≤ϵ, ∀(t,φ)∈(0,T]×A∗. |
This implies that
‖T(t)φ−φ‖H≤(ϵ+‖Lφ‖H)t, ∀(t,φ)∈[0,T]×A∗. |
Since A∗ is compact and L is continuous, then there exists a constant ~C1>0, such that
ϵ+‖Lφ‖H≤~C1, ∀φ∈A∗, |
thereby,
‖T(t)φ−φ‖H≤~C1t, ∀(t,φ)∈[0,T]×A∗. | (2.5) |
For any (t1,φ),(t2,φ)∈[0,T]×A∗, without loss of generality, we assume that t1>t2, then
‖Q(t1)φ1−Q(t2)φ2‖H≤‖Q(t1)φ1−Q(t2)φ1‖H+‖Q(t2)φ1−Q(t2)φ2‖H, |
where Q(t)φ=etLφ+∫t0e(t−s)LG(Q(s)φ)ds. Then we can get that
Q(t1)φ1−Q(t2)φ1=et2L(e(t1−t2)L−I)φ1+∫t20e(t2−s)L(e(t1−t2)L−I)G(Q(s)φ1)ds+∫t1t2e(t1−s)LG(Q(s)φ1)ds. |
By the boundedness of solution of system (2.1), we know that G(Q(s)φ) is bounded. Hence, there exists a constant ~C2>0, such that
‖G(Q(s)φ1)‖H≤~C2, ∀φ1∈A∗. |
Since T(t)=etL is a continuous semigroup, then there exists a constant M>0, such that
‖etL‖≤M, t∈[0,T]. |
Therefore, by using the inequality (2.5), there has
‖Q(t1)φ1−Q(t2)φ1‖H≤‖et2L‖‖(e(t1−t2)L−I)φ1‖H+∫t20‖e(t2−s)L‖‖(e(t1−t2)L−I)G(Q(s)φ1)‖Hds+∫t1t2‖e(t1−s)L‖‖G(Q(s)φ1)‖Hds≤M‖(e(t1−t2)L−I)φ1‖H+∫t20M‖(e(t1−t2)L−I)G(Q(s)φ1)‖Hds+∫t1t2M‖G(Q(s)φ1)‖Hds≤M~C1|t1−t2|+M~C1~C2T|t1−t2|+M~C2|t1−t2|≤~C3|t1−t2|, |
where ~C3=max{M~C1,M~C1~C2T,M~C2}. Similarly, we can calculate that
‖Q(t2)φ1−Q(t2)φ2‖H=‖et2L(φ1−φ2)+∫t20M[G(Q(s)φ1)−G(Q(s)φ2)]ds‖H≤M‖φ1−φ2‖H+∫t20MˆL‖Q(s)φ1−Q(s)φ2‖Hds. |
By Gronwall's inequality, there exists a constant ~C4>0, such that
‖Q(t2)φ1−Q(t2)φ2‖H≤~C4‖φ1−φ2‖H. |
Let C=max{~C3,~C4}, for any t1,t2∈[0,T] and φ1,φ2∈A∗, we have
‖Q(t1)φ1−Q(t2)φ2‖H≤C(|t1−t2|+‖φ1−φ2‖H). |
Therefore, F(t,u)=Q(t)u:[0,T]×A∗ is Lipschitz continuous.
By [28,Theorem 3.1], we can obtain that the flow {Q(t)}t≥0 generated by (2.1) has an exponential attractor A∗=∪0≤t≤t∗Q(t)A∗, and its fractional dimension can be estimated as
dF(A∗)≤dF(A∗)+1. |
In this section, we construct a reaction-diffusion SEIQR (Susceptible-Exposed-Infected-Quarantined-Recovery) model with relapse in spatially heterogeneous environment. Our model is divided into five compartments, namely susceptible individuals (S), exposed individuals (E), infected individuals (I), quarantined individuals (Q) and temporary restorers (R). The parameters description and transfer diagram as shown below:
From Figure 1, the following system with the initial-boundary-value conditions is constructed by:
{∂S∂t=∇⋅(dS(x)∇S)+Λ(x)−β1(x)SES+E−β2(x)SIS+I−μ(x)S, x∈Ω,t>0,∂E∂t=∇⋅(dE(x)∇E)+β1(x)SES+E+β2(x)SIS+I+ρ2(x)R−[μ(x)+α(x)]E, x∈Ω,t>0,∂I∂t=∇⋅(dI(x)∇I)+α(x)E+ρ1(x)R−[μ(x)+η1(x)+γ(x)]I, x∈Ω,t>0,∂Q∂t=γ(x)I−[μ(x)+η2(x)+ϕ(x)]Q, x∈Ω,t>0,∂R∂t=∇⋅(dR(x)∇R)+ϕ(x)Q−[μ(x)+ρ1(x)+ρ2(x)]R, x∈Ω,t>0,∂S∂n=∂E∂n=∂I∂n=∂Q∂n=∂R∂n=0, x∈∂Ω,t>0,S(x,0)=S0(x),E(x,0)=E0(x),I(x,0)=I0(x),Q(x,0)=Q0(x),R(x,0)=R0(x), x∈Ω. | (3.1) |
Here, Ω is a bounded domain in Rm(m≥1) with smooth boundary ∂Ω (when m>1), dS(x),dE(x),dI(x),dR(x)∈C1(Ω) are positive, continuous and uniformly bounded diffusion coefficients depend on space and Λ(x),β1(x),β2(x),ρ1(x),ρ2(x),α(x),γ(x),ϕ(x),μ(x),η1(x) and η2(x) are positive Hölder continuous functions on accounting for the total recruitment scale, rates of contact, relapse, incidence, quarantined, recovery, natural death and disease-related death respectively. Neumann boundary conditions ∂S∂n=∂E∂n=∂I∂n=∂Q∂n=∂R∂n=0 denotes that the change rate on the boundary of the region Ω is equal to 0. It is straightforward to verify that SIS+I(SES+E) is a Lipschitz continuous function of S and I(E) in the open first quadrant. Therefore, we can extend it to the entire first quadrant by defining it to be zero whenever S=0 or I=0(E=0). Throughout the paper, we assume that the initial value S0,E0,I0,Q0 and R0 are nonnegative continuous functions on ¯Ω, and the number of infected individuals is positive, i.e., ∫ΩI0(x)dx>0. Specific parameters described in Table 1.
Parameter | Description |
S(x,t) | Density of susceptible individuals at location x and time t. |
E(x,t) | Density of exposed individuals at location x and time t. |
I(x,t) | Density of infected individuals at location x and time t. |
Q(x,t) | Density of quarantined individuals at location x and time t. |
R(x,t) | Density of temporary restorers at location x and time t. |
Λ(x) | Total recruitment scale into this homogeneous social mixing community at location x. |
βi(x),i=1,2 | Contact rate at location x. |
α(x) | Incidence rate at location x. |
γ(x) | Quarantined rate at location x. |
ρi(x),i=1,2 | Relapse rate at location x. |
ϕ(x) | Per-capita recovery (treatment) rate at location x. |
μ(x) | Natural mortality rate at location x. |
ηi(x),i=1,2 | Disease-related death rate at location x. |
dS(x),dI(x),dR(x) | Diffusion rate at location x. |
From now on, we denote that H=L2(Ω), H1=H10(Ω)∩C2,1(Ω), and for any given continuous function f on ¯Ω,
f∗=maxx∈¯Ωf(x) and f∗=minx∈¯Ωf(x). |
Theorem 3.1. For each (S0(x),E0(x),I0(x),Q0(x),R0(x))∈C(¯Ω), system (3.1) exists a positive and bounded global solution (S(x,t),E(x,t),I(x,t),Q(x,t),R(x,t))∈C2,1(Ω×(0,∞)).
Proof. Since L=(∇⋅(dS(x)∇),∇⋅(dE(x)∇),∇⋅(dI(x)∇),0,∇⋅(dR(x)∇)) is a symmetrical sectorial operator and all eigenvalues of L are
0>λ1≥λ2≥...≥λk>..., λk→−∞ (k→∞), |
G(S,E,I,Q,R):=(g1(S,E,I,Q,R),g2(S,E,I,Q,R),g3(S,E,I,Q,R),g4(S,E,I,Q,R),g5(S,E,I,Q,R))T, |
where
g1(S,E,I,Q,R)=Λ(x)−β1(x)SES+E−β2(x)SIS+I−μ(x)S,g2(S,E,I,Q,R)=β1(x)SES+E+β2(x)SIS+I+ρ2(x)R−[μ(x)+α(x)]E,g3(S,E,I,Q,R)=α(x)E+ρ1(x)R−[μ(x)+η1(x)+γ(x)]I,g4(S,E,I,Q,R)=γ(x)I−[μ(x)+η2(x)+ϕ(x)]Q,g5(S,E,I,Q,R)=ϕ(x)Q−[μ(x)+ρ1(x)+ρ2(x)]R |
be quasimonotone and satisfy the locally Lipschitz conditions, so by Lemma 2.5, we can guarantees that system (3.1) exists a global solution (S(x,t),E(x,t),I(x,t),Q(x,t),R(x,t))∈C2,1(Ω×(0,∞)). Similar to the method in [26,Lemma 2.1 and Theorem 2.2], we can easily prove that the global solution of the system (3.1) is positive. We next consider the following total population at time t. Define
U(t)=∫Ω[S(x,t)+E(x,t)+I(x,t)+Q(x,t)+R(x,t)]dx. |
From system (3.1), it is easy to see that
dU(t)dt=∫Ω[∂∂tS(x,t)+∂∂tE(x,t)+∂∂tI(x,t)+∂∂tQ(x,t)+∂∂tR(x,t)]dx=∫Ω{∇⋅(dS(x)∇S)+∇⋅(dE(x)∇E)+∇⋅(dI(x)∇I)+∇⋅(dR(x)∇R)+Λ(x)−μ(x)S−μ(x)E−[μ(x)+η1(x)]I−[μ(x)+η2(x)]Q−μ(x)R}dx≤∫Ω∇⋅(dS(x)∇S)dx+∫Ω∇⋅(dE(x)∇E)dx+∫Ω∇⋅(dI(x)∇I)dx+∫Ω∇⋅(dR(x)∇R)dx+∫Ω{Λ∗−μ∗[S+E+I+Q+R]}dx≤Λ∗|Ω|−μ∗U(t). |
By Gronwall's inequality in differential form [26,Lemma 2.2], we can obtain that
U(t)≤U(0)e−μ∗t+Λ∗|Ω|μ∗(1−e−μ∗t). |
So U(t)≤max{U(0),Λ∗|Ω|μ∗}, where
U(0)=∫Ω[S(x,0)+E(x,0)+I(x,0)+Q(x,0)+R(x,0)]dx≤∫Ω‖S(x,0)+E(x,0)+I(x,0)+Q(x,0)+R(x,0)‖L∞(Ω)dx=‖S(x,0)+E(x,0)+I(x,0)+Q(x,0)+R(x,0)‖L∞(Ω)|Ω|. |
This shows that U(t)=∫Ω(S+E+I+Q+R)dx is bounded. By the positivity of the solution of the system (3.1), we obtain that
‖S+E+I+Q+R‖L1(Ω)=∫Ω|S+E+I+Q+R|(x,t)dx=∫Ω(S+E+I+Q+R)(x,t)dx≤max{‖S(x,0)+E(x,0)+I(x,0)+Q(x,0)+R(x,0)‖L∞(Ω)|Ω|,Λ∗|Ω|μ∗}. |
We denote that K=max{‖S(x,0)+E(x,0)+I(x,0)+Q(x,0)+R(x,0)‖L∞(Ω)|Ω|,Λ∗|Ω|μ∗}, then we know
∫Ω(S+E+I+Q+R)dx≤K. |
In view of [31,Theorem 1 and Corollary 1], there exists a positive constant K∗ depending on K such that
‖S+E+I+Q+R‖L∞(Ω)≤K∗. |
Thus, we can obtain that S(x,t),E(x,t),I(x,t),Q(x,t),R(x,t) are uniformly bounded on ¯Ω. This implies the global solution of system (3.1) is positive and uniformly bounded.
In this section, we will analyze the qualitative behavior of system (3.1). It is clearly see that system (3.1) admits a disease-free equilibrium E0(x)=(S0(x),0,0,0,0).
In order to further study the long-term dynamic behavior of the diffusive SEIQR epidemic model with relapse in spatially heterogeneous environment, we need to prove the existence of principal eigenvalues of system (3.1). Linearizing the second, the third, the forth and the fifth equations of (3.1) at disease-free equilibrium, we get
{∂E∂t=∇⋅(dE(x)∇E)+β1(x)E+β2(x)I+ρ2(x)R−[μ(x)+α(x)]E, x∈Ω,t>0,∂I∂t=∇⋅(dI(x)∇I)+α(x)E+ρ1(x)R−[μ(x)+η1(x)+γ(x)]I, x∈Ω,t>0,∂Q∂t=γ(x)I−[μ(x)+η2(x)+ϕ(x)]Q, x∈Ω,t>0,∂R∂t=∇⋅(dR(x)∇R)+ϕ(x)Q−[μ(x)+ρ1(x)+ρ2(x)]R, x∈Ω,t>0,∂E∂n=∂I∂n=∂Q∂n=∂R∂n=0, x∈∂Ω. | (3.2) |
Let E=eλtχ(x),I=eλtφ(x),Q=eλtξ(x),R=eλtψ(x), equations (3.2) can be rewritten as
{∇⋅(dE(x)∇χ(x))+[β1(x)−(μ(x)+α(x))]χ(x)+β2(x)φ(x)+ρ2(x)ψ(x)=λχ(x),∇⋅(dI(x)∇φ(x))+α(x)χ(x)+ρ1(x)ψ(x)−[μ(x)+η1(x)+γ(x)]φ(x)=λφ(x),γ(x)φ(x)−[μ(x)+η2(x)+ϕ(x)]ξ(x)=λξ(x),∇⋅(dR(x)∇ψ(x))+ϕ(x)ξ(x)−[ρ(x)+μ(x)+η2(x)]ψ(x)=λψ(x), x∈Ω,∂χ∂n=∂φ∂n=∂ξ∂n=∂ψ∂n=0, x∈∂Ω. | (3.3) |
Denote Φ(x)=(χ(x),φ(x),ξ(x),ψ(x))T,D(x)=[dE(x)0000dI(x)000000000dR(x)] and
M(x)=(mij(x))=[m11(x)β2(x)0ρ2(x)α(x)m22(x)0ρ1(x)0γ(x)m33(x)000ϕ(x)m44(x)], |
where
m11(x)=β1(x)−(μ(x)+α(x)),m22(x)=−[μ(x)+η1(x)+γ(x)],m33(x)=−[μ(x)+η2(x)+ϕ(x)],m44(x)=−[μ(x)+ρ1(x)+ρ2(x)] |
and mij(x)≥0,i≠j,x∈¯Ω. Therefore, equations (3.3) can be rewritten as
{λΦ(x)=∇⋅(D(x)∇Φ(x))+M(x)Φ(x), x∈Ω,∂Φ∂n=0, x∈∂Ω | (3.4) |
By Krein–Rutman theorem, we can obtain that there exists a real eigenvalue λ∗ of Eq (3.4) and a corresponding eigenvector Φ∗(x)=(χ∗(x),φ∗(x),ξ∗(x),ψ∗(x)) satisfying Φ∗(x)>>0 for all x∈¯Ω in the case of Neumann boundary conditions.
In this section we use the global exponential attractor theory to discuss the long-term dynamic behavior of the reaction-diffusion SEIQR epidemic model with relapse in heterogeneous environment. Denote that H5=H×H×H×H×H and H51=H1×H1×H1×H1×H1. Note that H5 and H51 are Banach spaces equipped with norm
‖(S,E,I,Q,R)T‖H5:=‖S‖H+‖E‖H+‖I‖H+‖Q‖H+‖R‖H. |
and
‖(S,E,I,Q,R)T‖H51:=‖S‖H1+‖E‖H1+‖I‖H1+‖Q‖H1+‖R‖H1. |
In the following, we apply Lemma 2.5 and Theorem 2.7 to our system (3.1).
Theorem 3.2. System (3.1) exists a global exponential attractor A∗, it exponential attracts any bounded set in H5.
Proof. For the system (3.1), we first verify the condition (2.3). Since
⟨∇⋅(dS(x)∇S)+Λ(x)−β1(x)SES+E−β2(x)SIS+I−μ(x)S,S⟩H=∫Ω∇⋅(dS(x)∇S)⋅Sdx+∫ΩΛ(x)Sdx−∫Ωβ1(x)S2ES+Edx−∫Ωβ2(x)S2IS+Idx−∫Ωμ(x)S2dx=∫Ωn∑i=1S⋅∂∂xi(dS(x)∂S∂xi)dx+∫ΩΛ(x)Sdx−∫Ωβ1(x)S2ES+Edx−∫Ωβ2(x)S2IS+Idx−∫Ωμ(x)S2dx=n∑i=1∫ΩS⋅∂∂xi(dS(x)∂S∂xi)dx+∫ΩΛ(x)Sdx−∫Ωβ1(x)S2ES+Edx−∫Ωβ2(x)S2IS+Idx−∫Ωμ(x)S2dx=−n∑i=1∫ΩdS(x)(∂S∂xi)2dx+n∑i=1∫∂ΩS⋅(dS(x)∂S∂xi)⋅nxids+∫ΩΛ(x)Sdx−∫Ωβ1(x)S2ES+Edx−∫Ωβ2(x)S2IS+Idx−∫Ωμ(x)S2dx=−∫ΩdS(x)n∑i=1(∂S∂xi)2dx+∫∂ΩSdS(x)∂S∂nds+∫ΩΛ(x)Sdx−∫Ωβ1(x)S2ES+Edx−∫Ωβ2(x)S2IS+Idx−∫Ωμ(x)S2dx=−∫ΩdS(x)|∇S|2dx+∫ΩΛ(x)Sdx−∫Ωβ1(x)S2ES+Edx−∫Ωβ2(x)S2IS+Idx−∫Ωμ(x)S2dx≤−(dS)∗∫Ω|∇S|2dx+Λ∗∫ΩSdx=−(dS)∗‖S‖2H12+Λ∗∫ΩSdx, |
⟨∇⋅(dE(x)∇E)+β1(x)SES+E+β2(x)SIS+I+ρ2(x)R−[μ(x)+α(x)]E,E⟩H=∫Ω∇⋅(dE(x)∇E)⋅Edx+∫Ωβ1(x)SE2S+Edx+∫Ωβ2(x)SEIS+Idx+∫Ωρ2(x)ERdx−∫Ω[μ(x)+α(x)]E2dx≤−(dE)∗‖E‖2H12+∫Ωβ1(x)SE2S+Edx+∫Ωβ2(x)SEIS+Idx+∫Ωρ2(x)ERdx≤−(dE)∗‖E‖2H12+β∗1∫ΩE2dx+β∗2∫ΩEIdx+ρ∗2∫ΩERdx, |
⟨∇⋅(dI(x)∇I)+α(x)E+ρ1(x)R−[μ(x)+η1(x)+γ(x)]I,I⟩H=∫Ω∇⋅(dI(x)∇I)⋅Idx+∫Ωα(x)EIdx+∫Ωρ1(x)IRdx−∫Ω[μ(x)+η1(x)+γ(x)]I2dx≤−(dI)∗‖I‖2H12+α∗∫ΩEIdx+ρ∗1∫ΩIRdx, |
⟨γ(x)I−[μ(x)+η2(x)+ϕ(x)]Q,Q⟩H=∫Ωγ(x)IQdx−∫Ω[μ(x)+η2(x)+ϕ(x)]Q2dx≤γ∗∫ΩIQdx, |
⟨∇⋅(dR(x)∇R)+ϕ(x)Q−[μ(x)+ρ1(x)+ρ2(x)]R,R⟩H=∫Ω∇⋅(dR(x)∇R)⋅Rdx+∫Ωϕ(x)QRdx−∫Ω[μ(x)+ρ1(x)+ρ2(x)]R2dx≤−(dR)∗‖R‖2H12+ϕ∗∫ΩQRdx. |
From Theorem 3.1, we know (S(x,t),E(x,t),I(x,t),Q(x,t),R(x,t)) is uniformly bounded, hence, condition (2.3) holds. In addition, since SIS+I(SES+E) is a Lipschitz continuous function of S and I(E) in the open first quadrant, so it is not difficult for us to verify that
‖g1(S1,E1,I1,Q1,R1)−g1(S2,E2,I2,Q2,R2)‖H≤β∗1‖E1−E2‖H+β∗2‖I1−I2‖H+(β∗1+β∗2+μ∗)‖S1−S2‖H, |
‖g2(S1,E1,I1,Q1,R1)−g2(S2,E2,I2,Q2,R2)‖H≤(β∗1+β∗2)‖S1−S2‖H(β∗1+μ∗+α∗)‖E1−E2‖H+β∗2‖I1−I2‖H+ρ∗2‖R1−R2‖H, |
‖g3(S1,E1,I1,Q1,R1)−g3(S2,E2,I2,Q2,R2)‖H≤α∗‖E1−E2‖H+(μ∗+η∗1+γ∗)‖I1−I2‖H+ρ∗1‖R1−R2‖H, |
‖g4(S1,E1,I1,Q1,R1)−g4(S2,E2,I2,Q2,R2)‖H≤γ∗‖I1−I2‖H+(μ∗+η∗2+ϕ∗)‖Q1−Q2‖H, |
‖g5(S1,E1,I1,Q1,R1)−g5(S2,E2,I2,Q2,R2)‖H≤ϕ∗‖Q1−Q2‖H+(μ∗+ρ∗1+ρ∗2)‖R1−R2‖H. |
If we choose ˆL=β∗1+β∗2+ρ∗1+ρ∗2+α∗+γ∗+ϕ∗+μ∗+η∗1+η∗2, and denote u=(S1,E1,I1,Q1,R1),v=(S2,E2,I2,Q2,R2), then
‖G(t,u)−G(t,v)‖H5=‖(g1(t,u)−g1(t,v)),(g2(t,u)−g2(t,v)),(g3(t,u)−g3(t,v)),(g4(t,u)−g4(t,v)),(g5(t,u)−g5(t,v)),‖H5=‖g1(t,u)−g1(t,v)‖H+‖g2(t,u)−g2(t,v)‖H+‖g3(t,u)−g3(t,v)‖H+‖g4(t,u)−g4(t,v)‖H+‖g5(t,u)−g5(t,v)‖H≤(β∗1+β∗2+ρ∗1+ρ∗2+α∗+γ∗+ϕ∗+μ∗+η∗1+η∗2)‖u−v‖H5=ˆL⋅‖u−v‖H5. |
Hence, Lipschitz condition is well verified. As we know that
L=(∇⋅(dS(x)∇),∇⋅(dE(x)∇),∇⋅(dI(x)∇),0,∇⋅(dR(x)∇)) is a symmetrical sectorial operator and all eigenvalues of L are
0>λ1≥λ2≥...≥λk>..., λk→−∞ (k→∞), |
therefore, by Lemma 2.5, system (3.1) exists a invariant set, it exponential attracts any bounded set in H5. By Theorem 2.7, we can obtain that system (3.1) has a global exponential attractor A∗ and dimF(A∗)=d0<∞.
After getting the global exponential attractor, we discuss the stability and persists uniformly of the epidemic disease.
Theorem 3.3. The following statements are valid.
(1) If λ∗<0, then
limt→∞S(x,t)=S0(x),limt→∞E(x,t)=0,limt→∞I(x,t)=0,limt→∞Q(x,t)=0,limt→∞R(x,t)=0 |
in H, and hence, the disease-free equilibrium is globally asymptotically stable.
(2) If λ∗>0, then there exists a function γ(x)>0 independent of the initial data, such that any solution (S,E,I,Q,R) satisfies
liminft→∞S(x,t)≥γ(x),liminft→∞E(x,t)≥γ(x),liminft→∞I(x,t)≥γ(x),liminft→∞Q(x,t)≥γ(x),liminft→∞R(x,t)≥γ(x) |
for x∈¯Ω, and hence, the disease persists uniformly
Proof. (1) Suppose λ∗<0. We will use the comparison principle to show that E(x,t)→0,I(x,t)→0,Q(x,t)→0,R(x,t)→0 as t→∞ for every x∈Ω. First, we observe from the system (3.1) that
{∂E∂t≤∇⋅(dE(x)∇E)+{β1(x)−[μ(x)+α(x)]}E+β2(x)I+ρ2(x)R,∂I∂t≤∇⋅(dI(x)∇I)+α(x)E+ρ1(x)R−[μ(x)+η1(x)+γ(x)]I,∂Q∂t≤γ(x)I−[μ(x)+η2(x)+ϕ(x)]Q,∂R∂t≤∇⋅(dR(x)∇R)+ϕ(x)Q−[μ(x)+ρ1(x)+ρ2(x)]R, x∈Ω,t>0. |
Next, let us define (˜E(x,t),˜I(x,t),˜Q(x,t),˜R(x,t))=(Meλ∗tχ∗(x),Meλ∗tφ∗(x),Meλ∗tξ∗(x),Meλ∗tψ∗(x)) where λ∗<0,χ∗(x)>>0,φ∗(x)>>0,ξ∗(x)>>0,ψ∗(x)>>0 are the eigenvalue and eigenvectors in equations (3.3) and M is chosen so large that E(x,0)≤˜E(x,0),I(x,0)≤˜I(x,0),Q(x,0)≤˜Q(x,0),R(x,0)≤˜R(x,0) for every x∈Ω. It can be shown that (˜E(x,t),˜I(x,t),˜Q(x,t),˜R(x,t)) satisfies
{∂˜E∂t=∇⋅(dE(x)∇˜E)+{β1(x)−[μ(x)+α(x)]}˜E+β2(x)˜I+ρ2(x)˜R,∂˜I∂t=∇⋅(dI(x)∇˜I)+α(x)˜E+ρ1(x)˜R−[μ(x)+η1(x)+γ(x)]˜I,∂˜Q∂t=γ(x)˜I−[μ(x)+η2(x)+ϕ(x)]˜Q,∂˜R∂t=∇⋅(dR(x)∇˜R)+ϕ(x)˜Q−[μ(x)+ρ1(x)+ρ2(x)]˜R, x∈Ω,t>0.∂˜E∂n=∂˜I∂n=∂˜Q∂n=∂˜R∂n=0, x∈∂Ω. |
By the comparison principle [26,Lemma 2.1], E(x,t)≤˜E(x,t),I(x,t)≤˜I(x,t),Q(x,t)≤˜Q(x,t),R(x,t)≤˜R(x,t) for every x∈Ω and t≥0. Since ˜E(x,t)→0,˜I(x,t)→0,˜Q(x,t)→0,˜R(x,t)→0 as t→∞ for every x∈Ω, we also have that E(x,t)→0,I(x,t)→0,Q(x,t)→0,R(x,t)→0 as t→∞ for every x∈Ω.
Next we claim S(⋅,t)→S0(x) uniformly on as t→∞. Given any small constant ε>0, there exists a large time T>0 such that 0≤E(x,t),I(x,t)≤ε for all x∈¯Ω,t≥T. From the first equation in system (3.1), it is observed that S is a super-solution to
{∂w∂t−∇⋅(dS(x)∇w)=Λ(x)−(β∗1+β∗2)ε−μ(x)w, x∈Ω,t≥T,∂w∂n=0, x∈∂Ω,w(x,T)=S(x,T), x∈Ω | (3.5) |
and a sub-solution to
{∂v∂t−∇⋅(dS(x)∇v)=Λ(x)−μ(x)v, x∈Ω,t≥T,∂v∂n=0, x∈∂Ω,v(x,T)=S(x,T), x∈Ω. | (3.6) |
Denote by w and v the solution of system (3.5) and system (3.6), respectively. The parabolic comparison principle gives
w(x,t)≤S(x,t)≤v(x,t) for all x∈¯Ω,t≥T. |
For system (3.5), we can verify that
⟨∇⋅(dS(x)∇w)+Λ(x)−(β∗1+β∗2)ε−μ(x)w,w⟩H=∫Ω∇⋅(dS(x)∇w)⋅wdx+∫ΩΛ(x)wdx−∫Ω(β∗1+β∗2)εwdx−∫Ωμ(x)w2dx≤−(dS)∗‖w‖2H12+Λ∗∫Ωwdx, |
this means that system (3.5) satisfies condition (2.3) for Lw+G(w)=∇⋅(dS(x)∇w)+Λ(x)−(β∗1+β∗2)ε−μ(x)w. It is similar to the proof of Theorem 3.2, we can obtain that system (3.5) exists a global exponential attractor Aw. In addition, system (3.5) has a variational structure, the corresponding functional of the variational structure is
F(w)=∫Ω[dS(x)2|∇w|2−g(x,w)]dx, |
where
g(x,w)=∫w0[Λ(x)−(β∗1+β∗2)ε−μ(x)u]du. |
Then
⟨DF(w),Lw+G(w)⟩H=⟨DF(w),∇⋅(dS(x)∇w)+Λ(x)−(β∗1+β∗2)ε−μ(x)w⟩H=−‖DF(w)‖2H, |
so L+G is a gradient type operator. From [29,Theorem A.2.2], we can prove that
limt→∞w(x,t)=S0−(ε,x) in H, |
where S0−(ε,x) is the unique positive steady state of problems (3.5). Similarly, for system (3.6), we can obtain
limt→∞v(x,t)=S0(x) in H, |
where S0(x) is the unique positive steady state of problems (3.6). Furthermore, due to the arbitrariness of ε, it is easily checked that
S0−(ε,x)→S0(x) in H, as ε→0. |
Thus, our analysis implies that the S(⋅,t)→S0(x) uniformly as t→∞.
(2) Since λ∗>0, it is observed that the solution of
{∂S−∂t−∇⋅(dS(x)∇S−)=Λ(x)−[β1(x)+β2(x)+μ(x)]S−, x∈Ω,t≥T,∂S−∂n=0, x∈∂Ω,S−(x,T)=S(x,T), x∈Ω | (3.7) |
is a sub-solution of the first equation in (3.1). Similar to the proof of conclusion (1), system (3.7) is also a gradient type equation. From [29,Theorem A.2.2], we can prove that
limt→∞S−(x,t)=S∗−(x) in H. |
By weak maximum principle, we know that S∗−(x)>0 for all x∈Ω. Next, let us define (E−(x,t),I−(x,t),Q−(x,t),R−(x,t))=(εχ∗(x),εφ∗(x),εξ∗(x),εψ∗(x)) where χ∗(x)>>0,φ∗(x)>>0,ξ∗(x)>>0,ψ∗(x)>>0 and ε>0 is a sufficiently small constant. Substituting εχ∗(x),εφ∗(x),εξ∗(x),εψ∗(x) into the second, the third, the forth and the fifth equations of system (3.1), we know
ε∇⋅(dE(x)∇χ∗)+β1(x)Sεχ∗S+εχ∗+β2(x)Sεφ∗S+εφ∗+ερ2(x)ψ∗−ε[μ(x)+α(x)]χ∗−∂(εχ∗)∂t=ε∇⋅(dE(x)∇χ∗)+β1(x)Sεχ∗S+εχ∗+β2(x)Sεφ∗S+εφ∗+ερ2(x)ψ∗−ε[μ(x)+α(x)]χ∗+εβ1(x)χ∗−εβ1(x)χ∗+εβ2(x)φ∗−εβ2(x)φ∗=ε{∇⋅(dE(x)∇χ∗)+β1(x)χ∗+β2(x)φ∗+ρ2(x)ψ∗−ε[μ(x)+α(x)]χ∗}+εβ1(x)χ∗[SS+εχ∗−1]+εβ2(x)φ∗[SS+εφ∗−1]=ελ∗χ∗+εβ1(x)χ∗[SS+εχ∗−1]+εβ2(x)φ∗[SS+εφ∗−1]>0 (ε>0 is a sufficiently small constant), |
ε∇⋅(dI(x)∇φ∗)+εα(x)χ∗+ερ1(x)ψ∗−ε[μ(x)+η1(x)+γ(x)]φ∗−∂(εφ∗)∂t=ε{∇⋅(dI(x)∇φ∗)+α(x)χ∗+ρ1(x)ψ∗−[μ(x)+η1(x)+γ(x)]φ∗}=ελ∗φ∗>0 (ε>0 is a sufficiently small constant), |
εγ(x)φ∗−ε[μ(x)+η2(x)+ϕ(x)]ξ∗−∂(εξ∗)∂t=ε{γ(x)φ∗−[μ(x)+η2(x)+ϕ(x)]ξ∗}=ελ∗ξ∗>0 (ε>0 is a sufficiently small constant) |
and
ε∇⋅(dR(x)∇ψ∗)+εϕ(x)ξ∗−ε[μ(x)+ρ1(x)+ρ2(x)]ψ∗−∂(εψ∗)∂t=ε{∇⋅(dR(x)∇ψ∗)+ϕ(x)ξ∗−[μ(x)+ρ1(x)+ρ2(x)]ψ∗}=ελ∗ψ∗>0 (ε>0 is a sufficiently small constant). |
Therefore, (εχ∗(x),εφ∗(x),εξ∗(x),εψ∗(x)) is the sub-solution of the second, the third, the forth and the fifth equations of system (3.1). We choose
0<γ(x)<min{S∗−(x),εχ∗(x),εφ∗(x),εξ∗(x),εψ∗(x)}, we can obtain that
liminft→∞S(x,t)≥γ(x),liminft→∞E(x,t)≥γ(x),liminft→∞I(x,t)≥γ(x),liminft→∞Q(x,t)≥γ(x) and liminft→∞R(x,t)≥γ(x) |
for x∈¯Ω, then it shows that the disease persists.
The synthesis of Theorem 3.2 and Theorem 3.3 can give the following results: if λ∗>0 and t→∞, then the positive solution of system (3.1) is globally exponential attractive and the attraction domain is A∗. Medically speaking, the disease persists in this situation. The epidemic is globally asymptotically stable or attracted by the global exponential attractor.
The COVID-19 was discovered because of Wuhan Viral Pneumonia cases in 2019. It was named by the World Health Organization on January 12, 2020. During the Spring Festival in 2020, the epidemic broke out in China with Wuhan as the center and began to diffuse. The Chinese government quickly adopted a strong response. Since January 23, 2020, Wuhan has implemented the strategy of closing the city, and various regions have adopted strategies such as restricting travel to control the spread of the epidemic. On January 30, 2020, WHO released the COVID-19 pneumonia epidemic as a public health emergency of international concern, emphasizing that travel and trade restrictions are not recommended, and reaffirming the Chinese government's control measures. While medical workers are fighting the epidemic, epidemiologists around the world have begun to use data analysis and modeling to study the spread of disease and find the fastest control methods [32,33,34,35].
The official website of the National Health Committee of the People's Republic of China has updated the relevant data of the COVID-19 epidemic [36] from January 24, 2020. The official website of the WHO can check the relevant data from January 21 to the present [37]. The specific data is shown in the Table 2.
Date | Suspected | Confirmed | Intensive care | Cured | Death |
2020.1.24 | 1965 | 1287 | 237 | 38 | 41 |
2020.1.25 | 2684 | 1975 | 324 | 49 | 56 |
2020.1.26 | 5794 | 2744 | 461 | 51 | 80 |
2020.1.27 | 6973 | 4515 | 976 | 60 | 106 |
2020.1.28 | 9239 | 5974 | 1239 | 103 | 132 |
2020.1.29 | 12167 | 7711 | 1370 | 124 | 170 |
2020.1.30 | 15238 | 9692 | 1527 | 171 | 213 |
2020.1.31 | 17988 | 11791 | 1795 | 243 | 259 |
2020.2.1 | 19544 | 14380 | 2110 | 328 | 304 |
2020.2.2 | 21558 | 17205 | 2296 | 475 | 361 |
2020.2.3 | 23214 | 20438 | 2788 | 632 | 425 |
2020.2.4 | 23260 | 24324 | 3219 | 892 | 490 |
2020.2.5 | 24702 | 28018 | 3859 | 1153 | 563 |
2020.2.6 | 26359 | 31161 | 4821 | 1504 | 636 |
2020.2.7 | 27657 | 34546 | 6101 | 2050 | 722 |
2020.2.8 | 28942 | 37198 | 6188 | 2649 | 811 |
2020.2.9 | 23589 | 40171 | 6484 | 3281 | 908 |
2020.2.10 | 21675 | 42638 | 7333 | 3996 | 1016 |
2020.2.11 | 16067 | 44653 | 8204 | 4780 | 1113 |
Judging from the existing data and various notifications of the epidemic, the COVID-19 is not yet relapse. Based on the data in Table 2, with the help of simple data analysis and calculation, we can get some important parameters in Table 3.
Parameter | Data estimated | Data sources |
Λ | 100000 | Estimate |
β1 | 0.7 | Estimate |
β2 | 0.5 | Estimate |
α | 0.423 | References[36] |
γ | 0.798 | References[36] |
ρ1 | 0 | References[36] |
ρ2 | 0 | References[36] |
ϕ | 0.0228 | References[36] |
μ | 0.1595 | References[38] |
η1 | 0.021 | References[36] |
η2 | 0.157 | References[36] |
dS | 2 | Estimate |
dE | 1 | Estimate |
dI | 0.3 | Estimate |
dR | 2 | Estimate |
According to the data in Table 3 and our system (3.1), we first simulate the spread of the COVID-19 epidemic (Figure 2).
From Figure 2, we can see that the numerical simulation basically accords with the current official data, especially the exposed individuals and the infected people. In addition, we also find that the COVID-19 is currently in a period of high incidence and extremely low cure rate. In such a period, the number of quarantines in the hospital will be large, and the sickbeds in the hospital will be very tight.
The Chinese government has tried to curb the spread of the epidemic by blocking cities and traffic. The essence is to control the exposure of the disease. If we choose β2=10−8 in Table 3, then we can get the image in Figure 3.
From the Figure 3 we can see that merely controlling the contact between susceptible and infected people can only delay the time of getting sick, and cannot obviously reduce the number of people infected. If we want a better control, we need to control the contact rate between the susceptible and the exposed individuals, because there are more people in the exposed period and their activities are more frequent. After several numerical simulations, we found that when β1<0.38, the stability of the model's endemic equilibrium began to be destroyed. If we choose β1=0.35 in Table 3, then we can get a simulated image of the disease-free equilibrium of constant coefficient model (Figure 4).
We can see from the Figure 4 that if people's travel is restricted and the contact rate between susceptible and incubation period patients is controlled, the epidemic will be effectively controlled and the disease will eventually die out. At this time, the disease-free equilibrium of the COVID-19 epidemic is globally asymptotically stable. Therefore, it is very correct for the Chinese government to restrict people's access through policies such as traffic blocking and forced quarantine, and these policies may only be implemented so smoothly in China.
Next, we discuss the dynamics of the epidemic model with a spatial heterogeneous environment and relapse, so we then simulate the stability and persistence of the spatial system (3.1). From the system (3.1) we can see that all the parameters are x−related functions, so we choose different functions will directly lead to different stability results. As of the end of February, a small number of relapse cases of the COVID-19 epidemic have appeared. If we choose β1(x)=0.4|sinx|,β2(x)=0.31+x,ρ1(x)=0.4e−x,ρ2(x)=0.32+x,η1(x)=0.021e−x,η2(x)=0.157e−2x and select other parameters from Table 3, then we can clearly see that the disease-free equilibrium of the spatial heterogeneity epidemic is globally asymptotically stable (Figure 5).
According to our discussion in the previous Section, the diffusion coefficient is highly dependent on space. The spread of viruses and human activities are subject to conditions such as climate and temperature. From this perspective, the diffusion coefficient should have periodic characteristics. Hence, from now on, we choose dS(x)=e30sinx,dE(x)=e15sinx,dI(x)=e5sinx,dR(x)=e10sinx. If we then choose ρ1(x)=0.03e−x,ρ2(x)=0.022+x,η1(x)=0.021e−x and select other parameters from Table 3, then we can clearly see that the spatial heterogeneity epidemic is persists uniformly (Figure 6).
In Figure 6, we find that the fluctuation of the image shows an irregular and unsmooth state. This irregular and unsmooth phenomenon is caused by the spatial heterogeneity of the diffusion coefficient. However, this irregular fluctuation is still in a controllable range. And this range is also the scope of the global exponential attractor.
If we choose β2(x)=0.05sinx,ρ1(x)=0.03e−x,ρ2(x)=0.022+x,η1(x)=0.021e−x and select other parameters from Table 3, then we can clearly see that the spatial heterogeneity epidemic is persists uniformly (Figure 7), and the disease has obvious almost periodic characteristics.
From all above simulation we can clearly see that both the disease-free equilibrium and endemic equilibrium of the constant-coefficient model are global asymptotic stability. For spatial heterogeneous models, the stability of the model is dependent on the spatial parameters and diffusion coefficients. In addition, we also see that the image whether rising or falling is very fast in the initial stage, and then fast tend to stability. It is also confirms that the solutions of system (3.1) is global exponentially attractive.
We first prove the existence theorem of the global exponential attractor for the general dissipative evolution system. Then we formulate a reaction-diffusion SEIQR model with relapse in the spatially heterogeneous environment and prove the positivity, uniform boundedness and existence of solution of the epidemiological model. Applying the general results of global exponential attractor for dissipative evolution system in Section 2 to the model in Section 3, we can get the existence of the attractor of the epidemic model. In addition, we prove that the disease-free equilibrium is global asymptotically stable and the endemic is persisting uniformly. Due to the influence of spatial heterogeneity relapse rate, it is no longer possible to rely on the basic reproductive number to describe the spread of epidemics. Therefore, we describe the phenomenon of disease transmission through the main eigenvalue of the system. From our proof, we can find that λ∗ has threshold characteristics. Finally, we perform numerical simulations of the spread trend of the COVID-19 epidemic in China recently. With these authoritative data, we also simulate the diffusion of the epidemic in the spatially heterogeneous environment. Simulation results show that the best way to eliminate the disease is to control the contact rate. Choosing different spatial coefficients can produce a fundamental change of the global dynamics of the system.
This work was supported by the Natural Science Foundation of Jiangsu Province, China (Grant No. BK20190578), the Fundamental Research Funds for the Central Universities, China (Grant No. JUSRP11949), the National Natural Science Foundation of China (Grant Nos. 11671180, 11671181).
The authors declare there is no conflict of interest.
[1] |
S. B. Hsu, F. B. Wang, X. Q. Zhao, Mathematical modeling and analysis of harmful algal blooms in flowing habitats, Math. Biosci. Eng., 16 (2019), 6728-6752. doi: 10.3934/mbe.2019336
![]() |
[2] |
Y. D. Zhang, H. F. Huo, H. Xiang, Dynamics of tuberculosis with fast and slow progression and media coverage, Math. Biosci. Eng., 16 (2019), 1150-1170. doi: 10.3934/mbe.2019055
![]() |
[3] |
F. Li, X. Q. Zhao, Dynamics of a periodic bluetongue model with a temperature-dependent incubation period, SIAM J. Appl. Math., 79 (2019), 2479-2505. doi: 10.1137/18M1218364
![]() |
[4] |
F. Li, X. Q. Zhao, A periodic SEIRS epidemic model with a time-dependent latent period, J. Math. Biol., 78 (2019), 1553-1579. doi: 10.1007/s00285-018-1319-6
![]() |
[5] |
F. Li, J. Liu, X. Q. Zhao, A West Nile Virus model with vertical transmission and periodic time delays, J. Nonlinear Sci., 30 (2020), 449-486. doi: 10.1007/s00332-019-09579-8
![]() |
[6] | Y. Xing, L. Zhang, X. Wang, Modelling and stability of epidemic model with free-living pathogens growing in the environment, J. Appl. Anal. Comput., 10 (2020), 55-70. |
[7] |
Z. Xu, C. Ai, Traveling waves in a diffusive influenza epidemic model with vaccination, Appl. Math. Model., 40 (2016), 7265-7280. doi: 10.1016/j.apm.2016.03.021
![]() |
[8] | C. C. Zhu, W. T. Li, F. Y. Yang, Traveling waves of a reaction-diffusion SIRQ epidemic model with relapse, J. Appl. Anal. Comput., 7 (2017), 147-171. |
[9] |
C. C. Zhu, J. Zhu, Stability of a reaction-diffusion alcohol model with the impact of tax policy, Comput. Math. Appl., 74 (2017), 613-633. doi: 10.1016/j.camwa.2017.05.005
![]() |
[10] |
B. S. Han, Y. Yang, An integro-PDE model with variable motility, Nonlinear Anal. Real World Appl., 45 (2019), 186-199. doi: 10.1016/j.nonrwa.2018.07.004
![]() |
[11] |
H. F. Huo, S. L. Jing, X. Y. Wang, H. Xiang, Modelling and analysis of an alcoholism model with treatment and effect of twitter, Math. Biosci. Eng., 16 (2019), 3595-3622. doi: 10.3934/mbe.2019179
![]() |
[12] |
Y. Jin, R. Peng, J. Shi, Population dynamics in river networks, J. Nonlinear Sci., 29 (2019), 2501-2545. doi: 10.1007/s00332-019-09551-6
![]() |
[13] |
Z. P. Ma, Spatiotemporal dynamics of a diffusive Leslie-Gower prey-predator model with strong Allee effect, Nonlinear Anal. Real World Appl., 50 (2019), 651-674. doi: 10.1016/j.nonrwa.2019.06.008
![]() |
[14] |
Z. G. Guo, L. P. Song, G. Q. Sun, C. Li, Z. Jin, Pattern dynamics of an SIS epidemic model with nonlocal delay, Int. J. Bifurcat. Chaos, 29 (2019), 1950027. doi: 10.1142/S0218127419500275
![]() |
[15] |
R. Peng, X. Q. Zhao, A reaction-diffusion SIS epidemic model in a time-periodic environment, Nonlinearity, 25 (2012), 1451-1471. doi: 10.1088/0951-7715/25/5/1451
![]() |
[16] |
R. Wu, X. Q. Zhao, A Reaction-Diffusion Model of Vector-Borne Disease with Periodic Delays, J. Nonlinear Sci., 29 (2019), 29-64. doi: 10.1007/s00332-018-9475-9
![]() |
[17] |
F. Y. Yang, W. T. Li, S. Ruan, Dynamics of a nonlocal dispersal SIS epidemic model with Neumann boundary conditions, J. Differential Equations, 267 (2019), 2011-2051. doi: 10.1016/j.jde.2019.03.001
![]() |
[18] |
C. C. Zhu, W. T. Li, F. Y. Yang, Traveling waves in a nonlocal dispersal SIRH model with relapse, Comput. Math. Appl., 73 (2017), 1707-1723. doi: 10.1016/j.camwa.2017.02.014
![]() |
[19] |
L. J. S. Allen, B. M. Bolker, Y. Lou, A. L. Nevai, Asymptotic profiles of the steady states for an SIS epidemic reaction-diffusion model, Discrete Contin. Dyn. Syst., 21 (2008), 1-20. doi: 10.3934/dcds.2008.21.1
![]() |
[20] |
R. Peng, Asymptotic profiles of the positive steady state for an SIS epidemic reaction-diffusion model. Part Ⅰ, J. Differ. Equ., 247 (2009), 1096-1119. doi: 10.1016/j.jde.2009.05.002
![]() |
[21] |
R. Peng, S. Liu, Global stability of the steady states of an SIS epidemic reaction-diffusion model, Nonlinear Anal., 71 (2009), 239-247. doi: 10.1016/j.na.2008.10.043
![]() |
[22] |
R. Peng, F. Yi, Asymptotic profile of the positive steady state for an SIS epidemic reactiondiffusion model: effects of epidemic risk and population movement, Phys. D, 259 (2013), 8-25. doi: 10.1016/j.physd.2013.05.006
![]() |
[23] |
H. Li, R. Peng, Z. A. Wang, On a diffusive SIS epidemic model with mass action mechanism and birth-death effect: analysis, simulations and comparison with other mechanisms, SIAM J. Appl. Math., 78 (2018), 2129-2153. doi: 10.1137/18M1167863
![]() |
[24] |
Y. Tong, C. Lei, An SIS epidemic reaction-diffusion model with spontaneous infection in a spatially heterogeneous environment, Nonlinear Anal. Real World Appl., 41 (2018), 443-460. doi: 10.1016/j.nonrwa.2017.11.002
![]() |
[25] |
P. Song, Y. Lou, Y. Xiao, A spatial SEIRS reaction-diffusion model in heterogeneous environment, J. Differ. Equ., 267 (2019), 5084-5114. doi: 10.1016/j.jde.2019.05.022
![]() |
[26] |
C. C. Zhu, J. Zhu, X. L. Liu, Influence of spatial heterogeneous environment on long-term dynamics of a reaction-diffusion SVIR epidemic model with relapse, Math. Biosci. Eng., 16 (2019), 5897-5922. doi: 10.3934/mbe.2019295
![]() |
[27] | J. Zhang, Y. Wang, C. K. Zhong, Robustness of exponentially κ-dissipative dynamical systems with perturbation, Discrete Contin. Dyn. Syst. Ser. B, 22 (2017), 3875-3890. |
[28] | A. Eden, C. Foias, B. Nicolaenko, R. Temam, Exponential attractors for dissipative evolution equations, Masson, Paris, 1994. |
[29] | T. Ma, S. Wang, Phase transition dynamics, Springer Science+Business Media, LLC 2014. |
[30] | I. I. Vrabie, C0 semigroups and application, Elsevier Science B.V., New York, 2003. |
[31] |
D. Le, Dissipativity and global attractors for a class of quasilinear parabolic systems, Commun. Partial Differ. Equ., 22 (1997), 413-433. doi: 10.1080/03605309708821269
![]() |
[32] |
S. He, S. Tang, L. Rong, A discrete stochastic model of the COVID-19 outbreak: Forecast and control, Math. Biosci. Eng., 17 (2020), 2792-2804. doi: 10.3934/mbe.2020153
![]() |
[33] | B. Tang, N. L. Bragazzi, Q. Li, S. Tang, Y. Xiao, J. Wu, An updated estimation of the risk of transmission of the novel coronavirus (COVID-19), Infect. Dis. Model, 5 (2020), 248-255. |
[34] | Z. Yang, Z. Zeng, K. Wang, S. S. Wong, W. Liang, M. Zanin, et al., Modified SEIR and AI prediction of the epidemics trend of COVID-19 in China under public health interventions, J. Thorac. Dis., (2020), doi: 10.21037/jtd.2020.02.64. |
[35] |
N. Zhu, D. Zhang, W. Wang, X. Li, B. Yang, J. Song, et al., A novel coronavirus from patients with pneumonia in China, 2019, N. Engl. J. Med., 382 (2020), 727-733. doi: 10.1056/NEJMoa2001017
![]() |
[36] | Notification of pneumonia outbreak of new coronavirus infection. Available from: http://www.nhc.gov.cn or http://en.nhc.gov.cn. |
[37] | Coronavirus disease (COVID-2019) situation reports. Available from: https://www.who.int/emergencies/diseases/novel-coronavirus-2019/situation-reports. |
[38] | World Health Statistics, 2013. Available from: http://www.who.int. |
1. | Cheng-Cheng Zhu, Jiang Zhu, Dynamic analysis of a delayed COVID-19 epidemic with home quarantine in temporal-spatial heterogeneous via global exponential attractor method, 2021, 143, 09600779, 110546, 10.1016/j.chaos.2020.110546 | |
2. | Arunodaya Raj Mishra, Pratibha Rani, R. Krishankumar, K.S. Ravichandran, Samarjit Kar, An extended fuzzy decision-making framework using hesitant fuzzy sets for the drug selection to treat the mild symptoms of Coronavirus Disease 2019 (COVID-19), 2021, 103, 15684946, 107155, 10.1016/j.asoc.2021.107155 | |
3. | M A A Pratama, A K Supriatna, N Anggriani, A mathematical model to study the effect of travel between two regions on the COVID-19 infections, 2021, 1722, 1742-6588, 012044, 10.1088/1742-6596/1722/1/012044 | |
4. | Zahra Dehghan Shabani, Rouhollah Shahnazi, Spatial distribution dynamics and prediction of COVID‐19 in Asian countries: spatial Markov chain approach, 2020, 12, 1757-7802, 1005, 10.1111/rsp3.12372 | |
5. | R. Prem Kumar, Sanjoy Basu, Dipankar Ghosh, Prasun Kumar Santra, G. S. Mahapatra, Dynamical analysis of novel COVID ‐19 epidemic model with non‐monotonic incidence function , 2022, 22, 1472-3891, 10.1002/pa.2754 | |
6. | Xiao-Lan Liu, Cheng-Cheng Zhu, A Non-Standard Finite Difference Scheme for a Diffusive HIV-1 Infection Model with Immune Response and Intracellular Delay, 2022, 11, 2075-1680, 129, 10.3390/axioms11030129 | |
7. | Jerzy J. Parysek, Lidia Mierzejewska, Cities in the epidemic, the epidemic in cities: Reconstruction of COVID-19 development in Polish cities, 2022, 125, 02642751, 103676, 10.1016/j.cities.2022.103676 | |
8. | S. A. Alblowi, M. El Sayed, M. A. El Safty, Decision Making Based on Fuzzy Soft Sets and Its Application in COVID-19, 2021, 30, 1079-8587, 961, 10.32604/iasc.2021.018242 | |
9. | Cheng-Cheng Zhu, Jiang Zhu, The effect of self-limiting on the prevention and control of the diffuse COVID-19 epidemic with delayed and temporal-spatial heterogeneous, 2021, 21, 1471-2334, 10.1186/s12879-021-06670-y | |
10. | Sanjoy Basu, R. Prem Kumar, P.K. Santra, G.S. Mahapatra, A.A. Elsadany, Preventive control strategy on second wave of Covid-19 pandemic model incorporating lock-down effect, 2022, 61, 11100168, 7265, 10.1016/j.aej.2021.12.066 | |
11. | Fernando Córdova-Lepe, Juan Pablo Gutiérrez-Jara, Katia Vogt-Geisse, A Kinematic Approach to the Classical SIR Model, 2024, 13, 2075-1680, 718, 10.3390/axioms13100718 | |
12. | Cheng-Cheng Zhu, Jiang Zhu, Jie Shao, Epidemiological Investigation: Important Measures for the Prevention and Control of COVID-19 Epidemic in China, 2023, 11, 2227-7390, 3027, 10.3390/math11133027 | |
13. | Paras Soomro, Israr Ahmed, Faraz Ahmed Soomro, Darshan Mal, Numerical Simulation Model of the Infectious Diseases by Comparing Backward Euler Method and Adams-Bash forth 2-Step Method, 2024, 12, 2309-0022, 402, 10.21015/vtm.v12i1.1881 | |
14. | Cheng-Cheng Zhu, Jiang Zhu, The existence conditions for global exponential attractor of non-autonomous evolution equations and applications, 2023, 173, 09600779, 113711, 10.1016/j.chaos.2023.113711 |
Parameter | Description |
S(x,t) | Density of susceptible individuals at location x and time t. |
E(x,t) | Density of exposed individuals at location x and time t. |
I(x,t) | Density of infected individuals at location x and time t. |
Q(x,t) | Density of quarantined individuals at location x and time t. |
R(x,t) | Density of temporary restorers at location x and time t. |
Λ(x) | Total recruitment scale into this homogeneous social mixing community at location x. |
βi(x),i=1,2 | Contact rate at location x. |
α(x) | Incidence rate at location x. |
γ(x) | Quarantined rate at location x. |
ρi(x),i=1,2 | Relapse rate at location x. |
ϕ(x) | Per-capita recovery (treatment) rate at location x. |
μ(x) | Natural mortality rate at location x. |
ηi(x),i=1,2 | Disease-related death rate at location x. |
dS(x),dI(x),dR(x) | Diffusion rate at location x. |
Date | Suspected | Confirmed | Intensive care | Cured | Death |
2020.1.24 | 1965 | 1287 | 237 | 38 | 41 |
2020.1.25 | 2684 | 1975 | 324 | 49 | 56 |
2020.1.26 | 5794 | 2744 | 461 | 51 | 80 |
2020.1.27 | 6973 | 4515 | 976 | 60 | 106 |
2020.1.28 | 9239 | 5974 | 1239 | 103 | 132 |
2020.1.29 | 12167 | 7711 | 1370 | 124 | 170 |
2020.1.30 | 15238 | 9692 | 1527 | 171 | 213 |
2020.1.31 | 17988 | 11791 | 1795 | 243 | 259 |
2020.2.1 | 19544 | 14380 | 2110 | 328 | 304 |
2020.2.2 | 21558 | 17205 | 2296 | 475 | 361 |
2020.2.3 | 23214 | 20438 | 2788 | 632 | 425 |
2020.2.4 | 23260 | 24324 | 3219 | 892 | 490 |
2020.2.5 | 24702 | 28018 | 3859 | 1153 | 563 |
2020.2.6 | 26359 | 31161 | 4821 | 1504 | 636 |
2020.2.7 | 27657 | 34546 | 6101 | 2050 | 722 |
2020.2.8 | 28942 | 37198 | 6188 | 2649 | 811 |
2020.2.9 | 23589 | 40171 | 6484 | 3281 | 908 |
2020.2.10 | 21675 | 42638 | 7333 | 3996 | 1016 |
2020.2.11 | 16067 | 44653 | 8204 | 4780 | 1113 |
Parameter | Data estimated | Data sources |
Λ | 100000 | Estimate |
β1 | 0.7 | Estimate |
β2 | 0.5 | Estimate |
α | 0.423 | References[36] |
γ | 0.798 | References[36] |
ρ1 | 0 | References[36] |
ρ2 | 0 | References[36] |
ϕ | 0.0228 | References[36] |
μ | 0.1595 | References[38] |
η1 | 0.021 | References[36] |
η2 | 0.157 | References[36] |
dS | 2 | Estimate |
dE | 1 | Estimate |
dI | 0.3 | Estimate |
dR | 2 | Estimate |
Parameter | Description |
S(x,t) | Density of susceptible individuals at location x and time t. |
E(x,t) | Density of exposed individuals at location x and time t. |
I(x,t) | Density of infected individuals at location x and time t. |
Q(x,t) | Density of quarantined individuals at location x and time t. |
R(x,t) | Density of temporary restorers at location x and time t. |
Λ(x) | Total recruitment scale into this homogeneous social mixing community at location x. |
βi(x),i=1,2 | Contact rate at location x. |
α(x) | Incidence rate at location x. |
γ(x) | Quarantined rate at location x. |
ρi(x),i=1,2 | Relapse rate at location x. |
ϕ(x) | Per-capita recovery (treatment) rate at location x. |
μ(x) | Natural mortality rate at location x. |
ηi(x),i=1,2 | Disease-related death rate at location x. |
dS(x),dI(x),dR(x) | Diffusion rate at location x. |
Date | Suspected | Confirmed | Intensive care | Cured | Death |
2020.1.24 | 1965 | 1287 | 237 | 38 | 41 |
2020.1.25 | 2684 | 1975 | 324 | 49 | 56 |
2020.1.26 | 5794 | 2744 | 461 | 51 | 80 |
2020.1.27 | 6973 | 4515 | 976 | 60 | 106 |
2020.1.28 | 9239 | 5974 | 1239 | 103 | 132 |
2020.1.29 | 12167 | 7711 | 1370 | 124 | 170 |
2020.1.30 | 15238 | 9692 | 1527 | 171 | 213 |
2020.1.31 | 17988 | 11791 | 1795 | 243 | 259 |
2020.2.1 | 19544 | 14380 | 2110 | 328 | 304 |
2020.2.2 | 21558 | 17205 | 2296 | 475 | 361 |
2020.2.3 | 23214 | 20438 | 2788 | 632 | 425 |
2020.2.4 | 23260 | 24324 | 3219 | 892 | 490 |
2020.2.5 | 24702 | 28018 | 3859 | 1153 | 563 |
2020.2.6 | 26359 | 31161 | 4821 | 1504 | 636 |
2020.2.7 | 27657 | 34546 | 6101 | 2050 | 722 |
2020.2.8 | 28942 | 37198 | 6188 | 2649 | 811 |
2020.2.9 | 23589 | 40171 | 6484 | 3281 | 908 |
2020.2.10 | 21675 | 42638 | 7333 | 3996 | 1016 |
2020.2.11 | 16067 | 44653 | 8204 | 4780 | 1113 |
Parameter | Data estimated | Data sources |
Λ | 100000 | Estimate |
β1 | 0.7 | Estimate |
β2 | 0.5 | Estimate |
α | 0.423 | References[36] |
γ | 0.798 | References[36] |
ρ1 | 0 | References[36] |
ρ2 | 0 | References[36] |
ϕ | 0.0228 | References[36] |
μ | 0.1595 | References[38] |
η1 | 0.021 | References[36] |
η2 | 0.157 | References[36] |
dS | 2 | Estimate |
dE | 1 | Estimate |
dI | 0.3 | Estimate |
dR | 2 | Estimate |