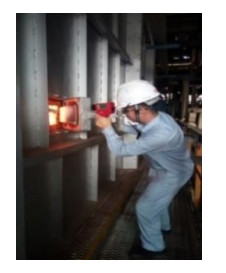
Citation: Timothy Jan Bergmann, Giorgia Brambilla Pisoni, Maurizio Molinari. Quality control mechanisms of protein biogenesis: proteostasis dies hard[J]. AIMS Biophysics, 2016, 3(4): 456-478. doi: 10.3934/biophy.2016.4.456
[1] | Qirui Li, Baikun Zhang, Delong Cui, Zhiping Peng, Jieguang He . The research of recognition of peep door open state of ethylene cracking furnace based on deep learning. Mathematical Biosciences and Engineering, 2022, 19(4): 3472-3486. doi: 10.3934/mbe.2022160 |
[2] | Liang Yu, Zhengkuan Zhang, Yangbing Lai, Yang Zhao, Fu Mo . Edge computing-based intelligent monitoring system for manhole cover. Mathematical Biosciences and Engineering, 2023, 20(10): 18792-18819. doi: 10.3934/mbe.2023833 |
[3] | Jiang Zhao, Dan Wu . The risk assessment on the security of industrial internet infrastructure under intelligent convergence with the case of G.E.'s intellectual transformation. Mathematical Biosciences and Engineering, 2022, 19(3): 2896-2912. doi: 10.3934/mbe.2022133 |
[4] | Yinhua Su . Visualization design of health detection products based on human-computer interaction experience in intelligent decision support systems. Mathematical Biosciences and Engineering, 2023, 20(9): 16725-16743. doi: 10.3934/mbe.2023745 |
[5] | Lingmin Lin, Kailai Liu, Huan Feng, Jing Li, Hengle Chen, Tao Zhang, Boyun Xue, Jiarui Si . Glucose trajectory prediction by deep learning for personal home care of type 2 diabetes mellitus: modelling and applying. Mathematical Biosciences and Engineering, 2022, 19(10): 10096-10107. doi: 10.3934/mbe.2022472 |
[6] | Shufen Niu, Wei Liu, Sen Yan, Qi Liu . Message sharing scheme based on edge computing in IoV. Mathematical Biosciences and Engineering, 2023, 20(12): 20809-20827. doi: 10.3934/mbe.2023921 |
[7] | Xiaoyan Zhao, Sanqing Ding . A multi-dimension information fusion-based intelligent prediction approach for health literacy. Mathematical Biosciences and Engineering, 2023, 20(10): 18104-18122. doi: 10.3934/mbe.2023804 |
[8] | Jiaming Ding, Peigang Jiao, Kangning Li, Weibo Du . Road surface crack detection based on improved YOLOv5s. Mathematical Biosciences and Engineering, 2024, 21(3): 4269-4285. doi: 10.3934/mbe.2024188 |
[9] | Shahab Shamshirband, Javad Hassannataj Joloudari, Sahar Khanjani Shirkharkolaie, Sanaz Mojrian, Fatemeh Rahmani, Seyedakbar Mostafavi, Zulkefli Mansor . Game theory and evolutionary optimization approaches applied to resource allocation problems in computing environments: A survey. Mathematical Biosciences and Engineering, 2021, 18(6): 9190-9232. doi: 10.3934/mbe.2021453 |
[10] | Muhammad Zia Ur Rahman, Ali Hassan Raza, Abeer Abdulaziz AlSanad, Muhammad Azeem Akbar, Rabia Liaquat, Muhammad Tanveer Riaz, Lulwah AlSuwaidan, Halah Abdulaziz Al-Alshaikh, Hatoon S Alsagri . Real-time artificial intelligence based health monitoring, diagnosing and environmental control system for COVID-19 patients. Mathematical Biosciences and Engineering, 2022, 19(8): 7586-7605. doi: 10.3934/mbe.2022357 |
Manufacturing industries play a key role at the national level and even in the whole human society [1]. Intelligent manufacturing is a major trend and the core content of the current manufacturing development. It is also an important initiative to accelerate the change in the development mode and promote the industry to the middle and high ends [2]. However, in intelligent manufacturing, the real-time communication between cloud platform and factory production facilities, information interaction between massive sensors and artificial intelligence platform, and efficient interaction between human and machine have diverse demands on communication networks and performance requirements, necessitating the introduction of highly reliable wireless communication technologies [3]. 5G network can provide extremely low time delay, high reliability, and massive connectivity, causing a new change to intelligent manufacturing systems [4,5].
In the petrochemical industry, the ethylene industry is particularly important. The industry chain covers a wide range. The current global production of ethylene continues to increase at a high rate of 7–8% per year [6]. The ethylene cracking furnace is the most important device in the ethylene industry. Further, the tube is the most critical part of the ethylene cracking furnace and also the reactor of steam cracking [7]. When the raw materials undergo cracking reactions at high temperatures, coke particles or coke bodies are generated and attached to the surface of tubes and equipment due to the special characteristics of hydrocarbons. Therefore, coke is inevitably generated during the cracking of hydrocarbon feedstocks, and the thickness of the coke layer increases further with the increase in the cracking time. The coke formed under such high-temperature conditions is a poor conductor of heat, which reduces the heat transfer coefficient, increases energy consumption, increases the thermal resistance of the tube wall, leads to a smaller inner diameter of the tube and a higher outer-surface temperature, and even blocks the tube, thus affecting the stable operation of the device. A more negative effect is the carburization of the tube, which causes material deterioration and reduces the mechanical strength of the tube [8]. Slagging leads to frequent coke cleaning of the cracking furnace, shortening of the cracking furnace operation and the effective production time of the device, an increase in energy consumption and equipment damage, and a decrease in tube life. Further, tubes are usually made of the nickel-chromium alloy and are expensive. Therefore, tube slagging causes a sharp increase in the production cost of ethylene devices. Additionally, severe slagging can lead to tube blockage and even tube explosion, forcing the ethylene production device to stop. If the local overtemperature caused by tube fire and internal slagging is not detected in time, it may result in a great safety hazard. In serious cases, it may even burn through the tube and cause a fire in the furnace, resulting in serious economic losses, endangering operators' lives, and bringing huge economic losses and safety risks to the petrochemical industry.
It is of great significance to conduct the diagnosis and prediction of tube slagging degree accurately and extend the effective operating cycle of the tube safely. These are effective technical means to ensure the safety of cracking furnace operation and improve the production efficiency of cracking furnace tubes.
Cracking overtemperature is the most important cause of slagging in cracking furnace tubes, which, in turn, leads to mechanical failure of the tube [9]. Therefore, monitoring of the cracking furnace tube temperature during the ethylene production process, especially the outer-surface temperature monitoring, is of great importance, and then the tube slagging situation needs to be examined and judged [10,11,12].
At present, the outer-surface temperature of the cracking furnace tube is mainly measured by two methods [13,14]. First, the thermocouple measurement is performed in different parts of the tube. Second, the outside operator is arranged for tube positioning and measurement by aiming at the tube with the thermoprobe through the cracking furnace peephole regularly. The tube outer-surface temperature is measured using an artificial thermoprobe as shown in Figure 1 [15]. However, both methods have serious limitations: the thermocouple method is readily exposed to a temperature shift owing to the high-temperature environment in the furnace, resulting in extreme challenges in the process operation and control. Also, the portable thermoprobe has the following drawbacks that hinder ethylene production: 1) the large cracking furnace smoke and fire, high temperature, and large heat radiation result in high temperature in the surrounding environment. This is coupled with the cracking furnace constantly vibrating and generating noise, harsh temperature measurement environment, and high labor intensity. Especially, the current cracking furnace external operators are significantly reduced. Reducing their labor intensity is highly important to improve the quality of labor and labor enthusiasm [16]. 2) Cracking furnace is usually equipped with several hundreds of tubes. However, the manpower and time are limited due to the low efficiency of manual temperature measurement. Hence, the external operator can usually only randomly select a small number of tubes for temperature measurement. Further, the measured temperature data are not uniform, incomplete, and therefore not representative [17]. 3) The infrared beam angle of incidence has a greater impact on the infrared temperature measurement accuracy. Usually, the vertical irradiation accuracy is the highest, but the handheld approach is more arbitrary. Therefore, the manual temperature measurement accuracy is low, coupled with different operating habits, resulting in the same tube having 20–30℃ deviation in the measurement results [18]. 4) Cracking furnaces often shake in the production process. The visual inspection of the tube position and then hand aiming greatly affect the temperature measurement accuracy of the tube [19]. A few ethylene production enterprises use an infrared thermal imager and a multispectral monitoring probe for temperature measurement on the outer surface, as shown in Figure 2. Nevertheless, both methods are costly and uncontrollable, limiting their wide application. Therefore, many ethylene production enterprises urgently need a cost-effective, real-time online automatic monitoring of the outer-surface temperature of the cracking furnace tube with accurate diagnosis and prediction of the tube slagging platform.
Based on the aforementioned common needs of ethylene production enterprises, this study developed an intelligent health-monitoring platform for ethylene cracking furnace tubes, which was successfully applied and achieved expected results [21,22]. Although the platform could effectively achieve the intelligent monitoring of cracking furnace tubes, tube monitoring became more difficult with the development of large, complex, and extreme operating parameters of cracking furnace equipment. The platform still had some technical difficulties that needed to be solved. The main aspects were as follows:
1) LoRa network is easy to build and deploy. It has the advantages of low power consumption, long transmission distance, license-free band nodes, and long battery life. However, it also has the disadvantages of spectrum interference, highly centralized technology, need for new networks, low transmission rate, and small transmission bandwidth. Also, it is not suitable for video transmission, making it difficult to expand the scale of monitoring. Further, it has limited transmission data volume and high site distribution environment requirements, which is not conducive to the promotion and application of this platform.
2) Temperature measurement using an intelligent temperature-measuring device still requires the external operator to open the peep door manually and control the temperature-measuring device by remote control to scan the outer surface of the tube and the inner wall of the furnace wall, resulting in manual intervention in the entire temperature measurement process and hence limiting its working efficiency. Therefore, intelligent modification of the peep door and temperature-measuring device is urgently required.
3) The platform uses a traditional central cloud structure, where the sampled data from devices are transmitted to a remote cloud for centralized analysis and processing. This has problems such as computing latency, congestion, low reliability, and security attacks.
4) The core indicators of the intelligent temperature-measuring device, such as intelligence, cost-effectiveness, accuracy, measurement time, equipment cost, installation and maintenance cost, and data transmission, have obvious advantages compared with similar products at home and abroad. However, the intelligent algorithms in the temperature-measuring device, such as overlapping tube identification, surface temperature and inner-wall temperature identification, and tube outer-surface temperature measurement, are cured in the embedded chip of the instrument, which lacks self-adaptation to the field monitoring environment. As a typical edge device, the intelligent temperature-measuring device does not take full advantage of the edge-cloud collaboration for self-directed learning.
Recently, modern communication technology represented by 5G; computer technology represented by edge computing, fog computing, and cloud computing; and artificial intelligence technology represented by deep learning have developed rapidly and are the hot spots for research and application in industry and academia in recent years. We used the interconnectivity and powerful communication capability of 5G and integrated edge-fog-cloud mixed computing and deep learning technology to build a 5G + mixed computing + deep learning technology system. The objectives were to upgrade and optimize the ethylene intelligent health-monitoring platform for the cracking furnace tube under cloud computing environment, solve the technical problems of the platform, improve the intelligence and automation of the platform, create the next-generation intelligent health-monitoring platform for ethylene cracking furnace tubes, further promote the quality and efficiency of the enterprise, and better protect the safe operation of the cracking furnace device.
Figure 3 illustrates the overall structure of the proposed 5G fusion-based next-generation intelligent health-monitoring platform for the ethylene cracking furnace tube. Studying the key common problems in the platform, such as 5G-based intelligent temperature-measuring device, 5G-based intelligent peep door gearing, 5G-based edge-fog-cloud collaboration mechanism, and mixed deep learning application, can improve the overall performance of the platform, enhance the automation and intelligence level of the platform, further promote the quality and efficiency of the enterprise, and better protect the safe operation of the cracking furnace device [23,24].
The 5G-based intelligent temperature-measuring device comprises an intelligent temperature-measuring device, a drive console, and a charging protection cabin.
1) Overall structure of the intelligent temperature-measuring device
The intelligent temperature-measuring device is mainly applied to the intelligent monitoring of tube outer-surface temperature, cracking furnace peep-hole initial operating parameters of adaptive matching, and cracking furnace peephole operating conditions of real-time video monitoring. It also aims to achieve remote automatic measurement of the cracking furnace tube and remote monitoring of the cracking furnace peep door, solve the measurement deviation caused by the operator's technical level and operation method in the manual temperature measurement process, reduce operator's labor intensity, and improve the accuracy and timeliness of data measurement and processing analysis. Figure 4 shows the overall structure of the intelligent temperature-measuring device. It comprises the main body control board, an infrared temperature sensor, a laser distance sensor, an HD binocular camera, the 5G wireless communication module, and the stepper motor drive module.
2) Overall structure of the drive console
The drive console is the main part of intelligent temperature-measuring device motion control, mainly used to realize intelligent motion control of the intelligent temperature-measuring device. Figure 5 shows the overall structure of a drive console, which comprises a high-precision electric turntable, two-phase hybrid stepper motors, magnetic charging modules, Hall sensors, and optoelectronic sensors. Through the cooperation of each module, the functions of starting position positioning, initial angle determination, horizontal linear motion, simultaneous rotation of the measurement angle during automatic temperature measurement using an intelligent temperature-measuring device, as well as the function of self-testing and automatic recharging of the instrument after the measurement, can be realized.
3) Overall structure of the charging protection cabin
The intelligent temperature-measuring device also comprises a charging protection cabin, as shown in Figure 6. It comprises an in-cabin inspection site, the power display section, and the magnetic charging module. During the automatic measurement process of the intelligent temperature-measuring device, when the power battery runs low, the automatic return to the charging protection cabin is initiated to realize the automatic charging of the temperature-measuring device. The in-cabin inspection site in the charging cabin is used to realize the in-cabin inspection function of the intelligent temperature-measuring device. Whenever the intelligent temperature-measuring device measurement task is completed, the instrument automatically returns to the cabin, the detection point detects that the instrument has entered the cabin completely, and the charging protection cabin automatically locks the protection cabin door to prevent accidental loss of the instrument.
The ethylene cracking furnace is closed all year-round to minimize the heat loss from the furnace. The only way to measure the temperature on the outside of the cracking furnace tube is to make the cracking furnace tube visible to the temperature-measuring device through a small peephole in the furnace wall. Owing to technical limitations and safety, peep doors are still used mechanically and manually, which directly affects the automation and intelligence of the tube temperature measurement.
The small peephole is the only way for the intelligent temperature-measuring device to peek into the cracking furnace and perform an outer-surface temperature measurement. The temperature-measuring device requires a high degree of a peep door opening. If the peep door is not open enough, some of the cracking furnace tubes may not be scanned and the detection data may be incomplete.
1) Opening and closing gearing of the peep door
The peephole of the ethylene cracking furnace is mechanically a vertical rotating single door. As shown in Figure 7, high-temperature and high-pressure flames are present in the ethylene cracking furnace chamber. When the peep door is opened, the high-temperature heat is radiated outward through the peephole due to the negative pressure effect. Therefore, the start/stop control of existing peep doors is opened by conventional manual operation. The inspection workers must wear high-temperature protective clothing, goggles, gloves, and other facilities before they can peep inside the furnace. The high-temperature and harsh operating environment bring great labor intensity to inspection workers and even causes injuries.
Automatic opening/closing control of peep doors is achieved using a chain-type electrical system/drive. The peep door gearing consists of a stepper motor, a bi-directional drive telescopic chain, a connecting bracket, and a peephole door connector.
The peep door gearing is located under the peep door, suspending the connection with the cracking furnace, reducing the direct contact area with the cracking furnace, effectively decreasing the operating environment temperature, reducing the high-temperature wear of the peep door gearing, and increasing its service life.
2) Peep door position detection device
The amplitude of the peep door opening is one of the main bases for determining the scanning position and scanning initialization angle of the intelligent temperature-measuring device. It is directly related to the accuracy of tube temperature measurement and the prediction of tube operation status. Meanwhile, when the automatic control of peep door opening and closing is realized, the telescopic chain of peep door gearing is beyond the working position and damaged if the position of peep door operation is not detected in real time. This is because the motor cannot be controlled and stops. Therefore, the accurate detection of the peep door position is one of the key issues to be solved.
The peep door position information is detected by the spring-press conversion method to ensure the accurate identification of the peep door position state. As shown in Figure 8, the spring is connected to the peep door through a bracket, and the other end of the spring is connected to the pressure sensor. When the position of the peep door changes, it drives the spring to deformation. The spring force is used to squeeze the pressure sensor to generate voltage change, the voltage change data are collected from the pressure sensor, the relationship between voltage and position is analyzed, and the position of the peep door is indirectly measured.
Edge computing, fog computing, and cloud computing are core supporting technologies of the intelligent monitoring platform structure [25]. Edge computing provides edge intelligent services nearby to meet the critical needs of industry digitization for agile connectivity, real-time business, data optimization, application intelligence, security, and privacy protection. Fog computing extends the services and tasks of the cloud to the network edge. Cloud computing, with its resource advantages, can provide large-scale data storage, analysis, working, and processing capabilities. At present, the cloud computing service model aggregates computation in the central cloud, which has shortcomings in location awareness, real-time content delivery, service latency, and mobility support [26,27].
1) Edge-fog-cloud layering mechanism
Based on the central cloud structure, a three-tier architecture system of mixed computing based on edge computing, fog computing, and cloud computing was built, as shown in Figure 9. The 5G fusion-based next-generation intelligent monitoring platform for the ethylene cracking furnace tube comprises an edge computing layer, a fog computing layer, and a cloud computing layer. The edge computing layer communicates with the fog computing layer over a 5G network, while the fog and cloud computing layers are connected over a dedicated high-speed wired network.
Edge computing layer: It mainly includes an intelligent temperature-measuring device and an intelligent peep door gearing, which can collect tube surface temperature data and peep door status data. The tube surface temperature data acquisition is complex, requiring optimization of the initial position and angle of temperature measurement, dual-phase synchronized control of horizontal motion and running rotation, overlapping tube identification, identification of outer-surface temperature and inner-wall temperature, outer-surface temperature measurement, and detection of abnormal temperatures. In the central cloud structure of the original platform, all these tasks run on the temperature-measuring device, making the device heavily burdened. With 5G and fog computing layer, the functions that are relatively simple and closely related to the temperature measurement instruments, such as the initial position and angle optimization of temperature measurement, dual-phase synchronous control of horizontal motion and running rotation, and identification of cracking furnace tube outer-surface temperature and inner-wall temperature, are still retained on the temperature-measuring device, while more complex functions, such as overlapping tube identification, outer-surface temperature measurement, and abnormal temperature detection, are moved to the fog computing layer. The peep door status data is mainly acquired using the HD camera integrated in the intelligent temperature-measuring device in the format of video. The base station server receives the video signal and identifies the status of the peep door, which is combined with the intelligent peep door control signal to achieve precise control of the peep door.
Fog computing layer: It mainly includes 5G base station, base station server, and other network devices, forming a mini-data center, which is responsible for two main tasks. One is to further process the data transmitted by the intelligent temperature-measuring device, such as the overlapping tube identification, outer-surface temperature measurement, abnormal temperature detection, and transfer of the effective data to the cloud computing layer to reduce the amount of data uploaded to the cloud computing layer. The second is that the received video data is used to discriminate the peep door status using deep learning, migration learning, and other methods for the synchronized control of the intelligent peep door with the intelligent temperature-measuring device.
Cloud computing layer: As the central cloud, it runs the slagging diagnosis and prediction system of the ethylene cracking furnace tube and other systems of the enterprise. It receives temperature measurement data from the fog computing layer, combines the data from the data collection system located in the same cloud, and includes cracking furnace tube outlet temperature, crossover section pressure, and venturi pressure so as to achieve real-time accurate diagnosis of the slagging degree of the ethylene cracking furnace tube and accurate prediction of slagging trends. Meanwhile, the cloud computing layer is responsible for the training of intelligent models in the monitoring platform, such as the peep door status identification model and the overlapping tube identification model.
2) Edge-fog-cloud collaboration mechanism
The divide-and-conquer and north-south collaboration methods were adopted. The edge computing layer is the site work node, which consists of edge devices such as an intelligent temperature-measuring device and an intelligent peep door control device. It is responsible for transferring the collected outer-surface temperature data of the cracking furnace tube, video data monitoring of the peep door and edge device working-state data northward through 5G to the fog computing layer, and receiving the operation commands and trained intelligent models from the fog computing layer. The fog computing layer is an intermediate node consisting of the 5G base station, base station server, and other network devices. It further cleans and processes the data in the south direction and exchanges data and control signals with the edge computing layer. The north direction exchanges data with the cloud computing layer and provides data support for the cloud computing layer. The cloud computing layer obtains valuable data from the fog computing layer for mass storage. On the one hand, intelligent models are trained and the automatic intelligent model update of the relevant edge devices is conducted to the fog computing layer server or through the fog computing layer. On the other hand, the data from other systems in the central cloud are combined to perform large data analysis, diagnose and predict the slagging of the cracking furnace tube, and provide decision support for production. The three layers of the edge-fog-cloud operate efficiently in a complementary manner through data collaboration, intelligence collaboration, and control collaboration.
The proposed edge-fog-cloud collaboration mechanism uses knowledge migration, embedded machine/deep learning, and other methods and techniques to optimize data collection, cleaning, transmission, storage, and application. The purpose is to achieve the collaboration of global optimization based on cloud computing and local optimization based on fog/edge computing through data collaboration, intelligence collaboration, and control collaboration. The working process of the next-generation platform involves three levels: data flow, control flow, and business flow. The whole platform's edge-fog-cloud collaborative capability and content include data collaboration, intelligence collaboration, and control collaboration, as shown in Figure 10.
Data collaboration: It consists of two data flows of tube temperature and peep door status. First, the intelligent temperature-measuring device in the edge computing layer initially processes and analyzes the collected data about the cracking furnace tube according to the rules or data model, and uploads the processing results to the fog computing layer through the 5G network. The fog computing layer further processes the received data and uploads it to the cloud computing terminal via a dedicated high-speed wired network. The cloud continuously receives data from the edge nodes and carries out a large data statistical analysis based on the massive operational status data (e.g., abnormal status data) to diagnose and predict the slagging degree of the cracking furnace tube. Second, the intelligent temperature-measuring device in the edge computing layer captures the video data of peep door status through the integrated camera and then uploads it to the fog computing layer through the 5G network. The fog computing layer uses a deep learning model to process the video to identify the state of the peep door, and uploads the key video to the cloud computing layer via a dedicated high-speed network for permanent storage. The data collaboration of edge, fog, and cloud supports the controlled and orderly flow of data between edge, fog, and cloud, forming a complete data flow path for efficient and low-cost data lifecycle management and value mining.
Intelligence collaboration: The temperature identification intelligent models are embedded in the intelligent temperature-measuring device for edge devices, while the fog computing layer integrates intelligent models for overlapping tube identification, abnormal temperature detection, and video identification. The operating environment of the cracking furnace tube is complex and variable. These models need to learn continuously to improve the self-adaptability of the temperature-measuring device and fog computing layer server, but learning training is a computationally intensive and time-consuming process. Therefore, the training task can only be placed in the cloud computing layer. The edge computing layer and the fog computing layer provide data input for the training of the model, and are responsible for the execution of edge inference. Considering the limited resource constraints of edge nodes, deploying AI chips or modules enables intelligence collaboration more efficiently. The cloud computing layer performs large data analysis by combining auxiliary data provided by other systems, continuously optimizes the model training, and updates the trained model to the fog computing layer server or to the intelligent temperature-measuring device via the fog computing layer. Through intelligence collaboration, the self-directed learning of the fog computing server and the intelligent temperature-measuring device can be achieved.
Control collaboration: The peep door must be opened and closed automatically to achieve full automation of the temperature measurement process. As the temperature-measuring device measures the temperature of the cracking furnace tube through the peephole, the opening angle of the peep door is strict during the temperature measurement. The whole control process is divided into seven steps:
Step 1: The fog computing layer server sends an open command to the intelligent peep door gearing according to a predefined policy.
Step 2: The fog computing layer server sends a video capture command to the temperature-measuring device.
Step 3: The fog computing layer server receives video signals from the edge computing layer and identifies the intelligent peep door status (e.g., whether it is open or closed).
Step 4: If the peep door has been opened at a sufficient angle, the temperature-measuring device is started for temperature measurement.
Step 5: When the temperature measurement is completed, a completion signal is sent to the fog computing layer server.
Step 6: The fog computing layer server sends a close command to the intelligent peep door gearing.
Step 7: Steps 2 and 3 are executed, if the peep door is closed; then the task is completed.
The identification of peep door status via the peep door surveillance video involves complex timing information. In the basic multilayer long short-term memory (LSTM) modeling sequential characteristic, the deep LSTM networks take the output of the previous layer of LSTM networks as the input at this moment. Not only do the deep LSTM networks lose the original video information, but also the training and information transfer of the networks may encounter bottlenecks. A time-domain multiscale space–time fusion model based on the multiscale feature in image processing was proposed to solve this problem, as shown in Figure 11. As observed, the video was sampled at different intervals, and the space–time fusion features of the video frame were used as the input of the network to obtain the time-domain features of the video content at different sampling intervals. The first-layer LSTM extracted 16 video frames, that is, video frame sequences {v1,v2,…,v16} as the input. The second-layer LSTM halved the input of the first layer, that is, eight video frames {v1,v2,…,v8} as the input. The input of the third-layer LSTM was halved from the second-layer input, that is, four video frames {v1,v2,…,v4} were extracted as input to the LSTM networks. The detail technical details and experimental results can be seen in [20].
1) Data set description
In terms of figure collection method, 150 pictures were taken by mobile phone. The size of the image after shooting is 2328 × 4656 pixels, and the format is JPG format. The default RGB color display standard is adopted, and three color channels are included. View of the shooting angle, for most images of fire door is not at the same level with the lens, lens is slightly higher than the view on the location of the fire door view fire door, most image medium fire door in a look down at the point of view of another part of the pictures were taken in view of the fire door side, and there are four without fire door airtight cover images. Parts of peep door data set are shown in the Figure 12.
2) Network structure and arameters
The keras framework in Tensorflow is used to construct the entire model structure. The structure of the convolutional neural network and parameters are shown in Table 1.
Layer (type) | Output Shape | Param |
conv2d_input (InputLayer) | [(n, 200,200, 3)] | 0 |
conv2d (Conv2D) | (n, 198,198, 16) | 448 |
max_pooling2d (MaxPooling2D) | (n, 99, 99, 16) | 0 |
conv2d_1 (Conv2D) | (n, 97, 97, 32) | 4640 |
max_pooling2d_1 (MaxPooling2) | (n, 48, 48, 32) | 0 |
conv2d_2 (Conv2D) | (n, 46, 46, 64) | 18,496 |
max_pooling2d_2 (MaxPooling2) | (n, 23, 23, 64) | 0 |
flatten (Flatten) | (n, 33856) | 0 |
dense (Dense) | (n, 512) | 17,334,784 |
dense_1 (Dense) | (n, 1) | 513 |
3) Model performance test
Figure 13 shows the accuracy and loss values of the training set and verification set respectively in the training process. A total of 10 epochs have been performed, and excellent recognition rate has been achieved in the first training.
Densenet-121 network is used for transfer learning, the results are shown in Figure 14. Results on the training set and the validation set is shown in Figure 14. The recognition model requires multiple epochs of training until the accuracy rate meets the re quirements. It is glad to see that the accuracy on the training set already reaches 95% after the fifth epoch. The accuracy on the validation set reaches 100%. After training four epochs, the proposed model has a 100% recognition rate on the test set.
The overlapping tubes in the cracking furnace meant that the tubes were caused to block each other and the blocked tubes were only partially visible through the peephole during the production process due to the tube shift. Therefore, the intelligent temperature-measuring device required an efficient and accurate overlapping tube identification method during the measurement process to correctly distinguish the measured outer-surface temperature. Empirically, the intelligent temperature-measuring device could be used for overlapping tube identification using the point-counting method and the adjacent point distance jump method, but the identification rate was low with a tendency for misjudgment situations and the most serious overlapping tubes were completely blocked, as shown in Figure 15, which led to the serious consequence of mismeasurement of the tube outer-surface temperature.
Based on the difference between normal and overlapping tubes in the measured temperature and distance 2D data and the advantage of DCNN in identifying 2D image features, DCNN was transplanted to the embedded chip of the edge device (intelligent temperature-measuring device), and the compressed DCNN was used on the edge side to identify overlapping tubes and normal tubes with high accuracy.
The DCNN for determining overlapping tubes is shown in Figure 16. It consisted of one input layer, three convolutional layers, two pooling layers, one fully connected layer, and one output layer. The input layer was used to input the 2D data features of normal and overlapping tubes, and the output layer was used to output the probability that the 2D data features belonged to normal and overlapping tubes.
During feature extraction of tube distance data in the 2D map, the temperature data of tubes and furnace walls in the 2D map were removed first, and then the distance data of furnace walls in the 2D map were removed. Further, the invalid threshold data of tube distance data having low feature correlation with overlapping tubes were removed so that the feature maps of overlapping and nonoverlapping tubes were extracted to form the dataset for DCNN network model training. After extracting the tube features, the DCNN overlapping tube identification model was trained and validated in cloud.
For realizing the overlapping tube identification function of the intelligent temperature-measuring device, the proposed steps to implement the DCNN overlapping tube identification inside the intelligent temperature-measuring device with an embedded processor as the core were as follows:
Step 1: DCNN training weights were compressed, transformed, and ported. In the cloud server, the DCNN network model for identifying normal and overlapping tubes was first trained, and then the trained network model was compressed using the NCS-based method. Further, the compressed DCNN node weights were transformed into data types that could be stored and computed inside the ARM Cortex-M7 embedded processor. Finally, the transformed weights were embedded in the embedded processor.
The ARM Cortex-M7 embedded processor only received 8-bit fixed-point numbers; therefore, the DCNN node weights data type needed to be compressed and transformed. The compression conversion process to be adopted was as follows: a generalized fixed-point number was represented as [QI: QF], where QI and QF corresponded to the integer and fractional parts, respectively. The fixed-point number usually included a sign bit to indicate the positive or negative of the number. The relationship between the integer length (IL), fractional length (FL), and length of sign bits of the fixed-point number, and bit-width (B) of the bit number of fixed-point number was as follows:
B=FL+IL+1 | (1) |
When converting floating-point numbers into fixed-point numbers, a specific rule was proposed to automatically determine the number of integer bits required. Specifically, enough bits were selected to avoid saturation. Therefore, for a given set of numbers S, the required length of the integer part was as follows:
ILS=⌈log2(maxSx+1)⌉ | (2) |
where ⌈x⌉ denotes the rounding up for x.
For conversion into fixed-point numbers with specified bit-width N, the length of the integer part IL was determined by:
IL={ILS,ILS<N−1N−1,ILS≥N−1 | (3) |
The length of the fractional part could be calculated by:
FL=N−IL−1 | (4) |
The minimum positive number of the fixed-point number representation was defined as ε, which was the representation precision of the fixed-point number as ε. The formula was as follows:
ε=2−FL | (5) |
Therefore, a given floating-point number could be converted into an approximation of the specified bit-width according to the following equation, which facilitated the subsequent fixed-point conversion of the value:
fixed(x)={⌊x⌋,⌊x⌋≤x≤⌊x⌋+ε2⌊x⌋+ε,⌊x⌋+ε2<x≤⌊x⌋+ε | (6) |
where ⌊x⌋ is defined as a value less than or equal to x and is the largest integer multiple with respect to ε.
For a fixed-point number given bit-width, the numerical approximation took the range:
{−2IL−1,x≤−2I−12I−1−2−FL,x≥2IL−1−2−FLfixed(x),otherwise | (7) |
After the approximation of the original values, the fixed point of approximate value was implemented, and the fixed-point formula was as follows:
value=(−1)s⋅2−FL⋅B−2∑i=02i⋅xi | (8) |
where x represents the binary complement form of the approximation.
Once the fixed point was complete, the fixed-point weights were finally ported to the embedded processor.
Step 2: DCNN reconstruction. The implementation of DCNN reconstruction within the embedded processor applied the ported compressed fixed-point weights for network computation and relied on CMSIS-NN, an optimization software kernel proposed by ARM specifically for deploying neural networks on Cortex-M CPUs. The CMSIS-NN library contained two parts: NNFunction and NNSupportFunctions. The NNFunction contained functions that implemented common neural network layer types, such as convolution, full connectivity, pooling, and activation functions, which were used by the application code to implement neural network inference applications. The NNSupportFunctions included different utility functions, such as data conversion and activation menu functions, which could be used by the application code to construct more complex neural network modules.
Step 3: The raw data collected were converted. The original one-dimensional data collected by the temperature-measuring device were converted into two-dimensional feature data, which were fed into the embedded processor reconstructed DCNN network and combined with the compressed and transformed weights to realize the function of DCNN to identify normal and overlapping tubes. The 2D data were obtained by dimensionally transforming the one-dimensional tube feature data obtained from the temperature-measuring device, which was used as the input to the embedded DCNN network.
The parameter settings of the CNN network are shown in Table 3, and the sample composition is shown in Table 4.
Parameter | Value | Parameter | Value |
test_iter | 40 | gamma | 0.0001 |
base_lr | 0.001 | power | 0.75 |
momentum | 0.9 | display | 500 |
weight_decay | 0.0005 | max_iter | 10,000 |
Sample | Normal | Overlapped | Total |
Training | 1,456 | 608 | 2064 |
Test | 1,028 | 412 | 1440 |
1) Model Validity Performance Test
The main steps and model performance test are as follows, and the detail technical details and experimental results can be see in [22].
Step 1: The furnace wall distance was removed from the original collection distance data, as shown in Figure 17 below:
Step 2: Extract the features of each normal tube and overlap tube, as shown in Figure 18:
Step 3: The training set is used to train the CNN network, and the validation set is used to judge the effect of the training model and optimize network parameters during the training process, the recognition results are shown in the Figure 19, the results show the output accuracy is 100%.
2) Model performance comparative analysis
In the experiment, six different learning strategies such as Fixed, STEP, EXP, INV, Poly and SigmoID were used to train the model. The change curve of model accuracy during training is shown in Figure 20. It can be seen from Figure 20 that the final accuracy of the overlapping furnace tube identification model trained by other methods except step and EXP is 99.85%.
The intelligent temperature-measuring device was a typical mobile edge terminal device, which ran monitoring tasks in a remote data center through a high-speed and highly reliable wireless interface. The tasks were transmitted through the wireless access network and then through the core network to the data center. Nevertheless, this model had an inherent drawback due to the long-distance propagation from the end device to the data center. The message delivery needed to go through the wireless access network, backhaul network, where the network routing and management operations might add additional time delay overhead. For this reason, the industry has proposed mobile edge computing (MEC). MEC refers to the deployment of computing and storage resources at the mobile network edge to provide IT service environment and computing power for mobile networks, thus providing users with ultra-low time delay and high-bandwidth network service solutions, while enhancing the privacy and security of mobile applications. Although a certain amount of computing resource is placed at the access network edge, MEC can reduce the application time delay through computational offloading. However, with limited communication and computing resources, designing a reasonable offloading strategy and resource allocation scheme is still an issue to be explored.
For the limited computing resource, communication resource, and local computing resource, an effective computation offloading and resource allocation strategy was designed based on the deep reinforcement learning method in combination with 5G communication technology so that all tasks could be executed with minimum total time delay.
The objective of uninstall decisions of edge devices, such as multiple intelligent temperature-measuring devices in the system and the allocation of network and computing resources, was to minimize the total time delay of all tasks, and the problem was represented as follows:
mincn,texen,toffnN∑n=1cnTln+(1−cn)Ton | (9) |
s.t.cn∈{0,1} |
texen≥toffn+Ttn |
where Cn represents the uninstall decision variable. As the task was nonseparable, the uninstall decision variable for edge device n is an integral 0, 1 variable. The second constraint indicated that the task needed to be uploaded to the MEC server first before it could be executed.
To solve this problem, we needed to determine three decision variables, which were the uninstall decision variable, the upload data time of the uninstall task, and the execution time. As the number of edge devices increased, the solution space size of the problem increased rapidly. Meanwhile, because the three decision variables were integer variables, the problem was not a convex optimization problem, but an NP-hard problem. Hence, the problem was solved using deep reinforcement learning methods.
It is first necessary to translate the problem into a representation of the basic elements of reinforcement learning to find the optimal strategy using the deep reinforcement learning approach. Second, the parameters of the deep learning network structure needed to be determined because deep learning was used as a reinforcement learning agent. Figure 21 shows the MEC resource scheduling model based on deep reinforcement learning.
Reinforcement learning comprised four components: state, action, reward, and agent. The agent in deep reinforcement learning was a neural network, that is, the strategy was represented by a deep learning network. The state, action, and reward were designed as follows.
Status: In the MEC resource scheduling problem of this project, the status should include the network resource usage of the base station, the MEC server computing resource usage, and the tasks waiting for a decision in the mobile device. Figure 22 shows the network resources and computing resource occupancy from the current time slice to the next few time slices. The vertical direction represented the time slices to be allocated, and each small square represented a time slice resource. The horizontal direction represented the resource category, that is, computing resource or network resource. When the edge device had a task to perform, it first sent a request to the base station. The base station stored the corresponding task information, that is, network resource consumption, computing resource consumption, and channel status, in the backlog. When the job slot queue was empty, the base station scheduled the task from the backlog to the job slot and waited for the base station's decision on the task scheduling. In the job slot queue, tasks were represented as the number of time slices of network resources to be consumed and the number of computing resource time slices.
Action: It used deep learning as an agent, requiring a fixed input and output form. Therefore, the action space could not change as the number of tasks in the state changed. The action space should contain all uninstall possibilities. As the tasks waiting to be scheduled could choose some tasks uninstalled to the base station and others were executed on the local side, the agent could choose any part of the tasks waiting to be scheduled as an execute decision uninstalled to a base station and other tasks were executed on the local device. The relationship between the size of action space and the number n of tasks to be scheduled was 2n, that is, any task could be scheduled to the base station and also be executed on the local device. As the number of tasks to be scheduled increased, the action space increased drastically, which did not meet the requirement of fixed action space for the neural network output. Therefore, the following method was used to reduce the action space size.
In each time slice, the base station could execute more than one task in a row. In the state, the tasks to be scheduled included tasks in the job slot and backlog queue. It was assumed that the size of the job slot was k and the action space was {0,1,2,⋯,2k}, where a=0 meant no task to be scheduled and it was an empty action.a∈{0,1,2,⋯,k} meant that the base station chose to uninstall the task on the ath job slot to the base station side. a∈{k+1,k+2,⋯,2k} meant that the base station chose the task on the a−kth job slot to execute in the edge device.
Reward: Agents in reinforcement learning were designed to maximize the cumulative discounted rewards over time, that is, the value function:
R=n∑t=0γnrt | (10) |
where γ is the discount factor, and rt represents the reward at state t. The goal of this problem was to minimize the total time delay of all tasks. Specifically, the reward function was set as follows:
rt=∑j∈J−1 | (11) |
where j represents all the tasks in the state and the tasks performed by the local device. As reinforcement learning had a reward for each action selection, the reward was 0 when the action was a valid action defined in the action space, and the reward was the value of reward function in Eq (11) when the action was an invalid action defined in the action space. In other words, the evaluation of the agent uninstall policy in this time slice needed to wait until the invalid action was generated, at which point the reward of Eq (11) was given, while the valid action indicated that the task uninstall was not finished in this time slice and returned to 0. After a task was executed on the edge device or base station side, it no longer belonged to J. The discount factor was set to 1, so that the –1 accumulation of all time slices occupied by a task from the time the uninstall request was sent until it was executed represented a negative time delay in execution. The optimization of deep learning parameters used the gradient descent method, that is, minimized the loss function. While reinforcement learning maximized the long-term reward value, in deep reinforcement learning, the long-term reward was the loss function. Therefore, the reward of the aforementioned design was a negative time delay sum, and maximizing the negative time delay sum meant minimizing the total time delay.
The train model parameter settings of the DQN are shown in Table 5, and the network parameters is shown in Table 6.
Parameter | Value | Parameter | Value |
Train time Learning rate Discount factor Target network update interval Experience pool size |
1000 0.001 0.95 100 30000 |
Greedy factor ɛ initial value Greedy factor ɛ max value Ɛ amplification Round number Mini-batch |
0.5 0.9 0.001 20 32 |
Net layer | Convolution layer | Maximum pooling layer | Full connection layer |
Input size Convolution kernel size Step size Number fo convolution kernel Activation function Output size |
180 * 20 2 × 2 (1, 1) 8 ReLU 178*18 |
178 * 18 2 × 2 (2, 2) -- -- 89*9 |
89 * 9 -- -- -- -- 21 |
1) Convergence verification
Compared with classical heuristic short-job priority SJF algorithm, Teris* algorithm and strategy gradient algorithm DeepRM, the results of the experiment are shown in Figure 23. Compared with DeepRM, DQN algorithm shows faster convergence and more stable curve. Compared with DeepRM algorithm, the average completion time of the final convergent job is reduced by 5.2%. During the whole training process, the DQN and DeepRM curves were unstable and oscillating in the first 100 iterations, and the average completion time was longer than that of the heuristic SJF algorithm and Teris* algorithm. After 200 rounds of training, the curve gradually tends to be stable, and the completion time is significantly lower than SJF algorithm and Teris* algorithm, and finally tends to converge. And the detail technical details and experimental results can be seen in [28,29,30].
2) Validity verification
Figure 24 shows the change trend of the average operation sabotage of the four comparison algorithms under different loads. As shown in the figure, under the condition of low load, there is little difference in the change of different algorithms. When the load reaches or exceeds 90%, it can be clearly observed that the average operation sabotage change rate of DeepRM and DQN algorithms is small. The results were significantly lower than those of the heuristic algorithms SJF and Tetris*. The results also shows that when the load reaches 130% and the training times reaches 200 rounds, DQN and DeepRM algorithms begin to converge, and the convergence result is smaller than SJF and Tetris*. At the same time, DQN converges faster than DeepRM and achieves smaller average operation slowdowns.
Compared with the previous platform, the 5G-based next-generation intelligent health-monitoring platform for ethylene cracking furnace tubes had a clear advantage (Table 7). The application site of this platform in a large petrochemical company is shown in Figure 25.
Function or technology | Next-generation platform | Previous platform |
Network transmission technology | 5G-based | LoRa-based |
Temperature measurement method | Remote automated control in the workshop |
Manual control at the cracking furnace site |
Peep hole operational condition monitoring | Real-time video remote monitoring | Manual on-site visual inspection |
Peep hole initial operation parameter setting | Fully adaptive | Manual measurement |
Self-learning of temperature-measuring device | Strong | Weak |
Peep door | Intelligent type, automatic open and close |
Mechanical type, manual open and close |
Identification of overlapping tubes | ≥ 99.99% | About 80% |
Charging of temperature-measuring device | Automatic | Manual |
Deep network model | Compressed | Not compressed |
Platform structure | Edge-fog-cloud three-layer structure | Central cloud structure |
Computing resource scheduling | Based on deep reinforcement learning, resource utilization is higher | Based on reinforcement learning, resource utilization needs to be improved |
Temperature measurement time per cycle | ≤ 7 min | ~10 min |
Considering the technology demand from ethylene production industry and market, a next-generation intelligent health-monitoring platform was built based on 5G + mixed computing + deep learning for ethylene cracking furnace tubes deriving from the previously developed platform and its successful application. The key common problems in the platform, such as 5G-based intelligent temperature-measuring device, 5G-based intelligent peep door gearing, 5G-based edge-fog-cloud collaboration mechanism, and mixed deep learning application, were also examined. The aim was to improve the overall performance of the platform, enhance the automation and intelligence level of the platform, further promote the quality and efficiency of the enterprise, better protect the safe operation of the cracking furnace device, and also promote the industry-scientific and technological progress, transformation, and upgrading through the application of the platform.
This study was supported by the National Natural Science Foundation of China (61772145, 61672174), the Guangdong Basic and Applied Basic Research Foundation (2021A1515012252, 2020A1515010727, 2022A1515012022), the Key Field Special Project of the Department of Education of Guangdong Province (2020ZDZX3053), the Key Realm R & D Program of Guangdong Province (2021B0707010003), and the Maoming Science and Technology Project (210429094551175, mmkj2020008, and mmkj2020033), 2022 Guangdong Province Science and Technology Innovation Strategy Special Project (pdjh2022b0349).
The authors declare there is no conflict of interest.
[1] | Crick FHC (1956) Ideas on protein synthesis. Wellcome Library for the History and Understanding of Medicine. Avaiable from: http://archives.wellcome.ac.uk/. |
[2] |
Crick FHC (1970) Central Dogma of Molecular Biology. Nature 227: 561–563. doi: 10.1038/227561a0
![]() |
[3] |
Baltimore D (1970) RNA-dependent DNA polymerase in virions of RNA tumour viruses. Nature 226: 1209–1211. doi: 10.1038/2261209a0
![]() |
[4] |
Temin HM, Mizutani S (1970) RNA-dependent DNA polymerase in virions of Rous sarcoma virus. Nature 226: 1211–1213. doi: 10.1038/2261211a0
![]() |
[5] |
Koonin EV (2012) Does the central dogma still stand? Biol Direct 7: 27. doi: 10.1186/1745-6150-7-27
![]() |
[6] |
Melnikov S, Ben-Shem A, Garreau de Loubresse N, et al. (2012) One core, two shells: bacterial and eukaryotic ribosomes. Nat Struct Mol Biol 19: 560–567. doi: 10.1038/nsmb.2313
![]() |
[7] |
Khatter H, Myasnikov AG, Natchiar SK, et al. (2015) Structure of the human 80S ribosome. Nature 520: 640–645. doi: 10.1038/nature14427
![]() |
[8] |
Kolitz SE, Lorsch JR (2010) Eukaryotic initiator tRNA: finely tuned and ready for action. FEBS Lett 584: 396–404. doi: 10.1016/j.febslet.2009.11.047
![]() |
[9] |
Rodnina MV (2016) The ribosome in action: Tuning of translational efficiency and protein folding. Protein Sci 25: 1390–1406. doi: 10.1002/pro.2950
![]() |
[10] |
Dabrowski M, Bukowy-Bieryllo Z, Zietkiewicz E (2015) Translational readthrough potential of natural termination codons in eucaryotes--The impact of RNA sequence. RNA Biol 12: 950–958. doi: 10.1080/15476286.2015.1068497
![]() |
[11] |
Karpinets TV, Greenwood DJ, Sams CE, et al. (2006) RNA:protein ratio of the unicellular organism as a characteristic of phosphorous and nitrogen stoichiometry and of the cellular requirement of ribosomes for protein synthesis. BMC Biol 4: 1–10. doi: 10.1186/1741-7007-4-1
![]() |
[12] |
Ingolia NT, Lareau LF, Weissman JS (2011) Ribosome profiling of mouse embryonic stem cells reveals the complexity and dynamics of mammalian proteomes. Cell 147: 789–802. doi: 10.1016/j.cell.2011.10.002
![]() |
[13] |
Dennis PP, Bremer H (1974) Differential Rate of Ribosomal Protein Synthesis in Escherichia coli B/r. J Mol Biol 84: 407–422. doi: 10.1016/0022-2836(74)90449-5
![]() |
[14] |
Dennis PP, Nomura M (1974) Stringent Control of Ribosomal Protein Gene Expression in Escherichia coli. Proc Nat Acad Sci USA 71: 3819–3823. doi: 10.1073/pnas.71.10.3819
![]() |
[15] |
Young R, Bremer H (1976) Polypeptide-Chain-Elongation Rate in Escherichia coli B/r as a Function ofGrowth Rate. Biochem J 160: 185–194. doi: 10.1042/bj1600185
![]() |
[16] | Schaaper RM (1993) Base selection, proofreading, and mismatch repair during DNA replication in Escherichia coli. J Biol Chem 268: 23762–23765. |
[17] | Drake JW, Charlesworth B, Charlesworth D, et al. (1998) Rates of spontaneous mutation. Genetics 148: 1667–1686. |
[18] |
Kunkel TA (2004) DNA replication fidelity. J Biol Chem 279: 16895–16898. doi: 10.1074/jbc.R400006200
![]() |
[19] |
Bebenek K, Kunkel TA (2004) Functions of DNA polymerases. Adv Protein Chem 69: 137–165. doi: 10.1016/S0065-3233(04)69005-X
![]() |
[20] |
Kunkel TA (2009) Evolving Views of DNA Replication (In)Fidelity. Cold Spring Harb Sym 74: 91–101. doi: 10.1101/sqb.2009.74.027
![]() |
[21] |
Sainsbury S, Bernecky C, Cramer P (2015) Structural basis of transcription initiation by RNA polymerase II. Nat Rev Mol Cell Biol 16: 129–143. doi: 10.1038/nrm3952
![]() |
[22] |
Sharma N (2016) Regulation of RNA polymerase II-mediated transcriptional elongation: Implications in human disease. IUBMB Life 68: 709–716. doi: 10.1002/iub.1538
![]() |
[23] | Loya TJ, Reines D (2016) Recent advances in understanding transcription termination by RNA polymerase II. F1000 Res 5: 1478. |
[24] |
Schwanhausser B, Busse D, Li N, et al. (2011) Global quantification of mammalian gene expression control. Nature 473: 337–342. doi: 10.1038/nature10098
![]() |
[25] |
Imashimizu M, Oshima T, Lubkowska L, et al. (2013) Direct assessment of transcription fidelity by high-resolution RNA sequencing. Nucleic Acids Res 41: 9090–9104. doi: 10.1093/nar/gkt698
![]() |
[26] |
Ninio J (1991) Connections between translation, transcription and replication error-rates. Biochimie 73: 1517–1523. doi: 10.1016/0300-9084(91)90186-5
![]() |
[27] |
Gouta JF, Thomasb WK, Smithc Z, et al. (2013) Large-scale detection of in vivo transcription errors. PNAS 110: 18584–18589. doi: 10.1073/pnas.1309843110
![]() |
[28] |
Cochella L, Green R (2005) Fidelity in protein synthesis. Curr Biol 15: 536–540. doi: 10.1016/j.cub.2005.02.019
![]() |
[29] | Kramer EB, Farabaugh PJ (2007) The frequency of translational misreading errors in E. coli is largely determined by tRNA competition. RNA 13: 87–96. |
[30] |
Zaher HS, Green R (2009) Fidelity at the molecular level: lessons from protein synthesis. Cell 136: 746–762. doi: 10.1016/j.cell.2009.01.036
![]() |
[31] | Gingold H, Pilpel Y (2011) Determinants of translation efficiency and accuracy. Mol Syst Biol 7: 141–150. |
[32] |
Ribas de Pouplana L, Santos MA, Zhu JH, et al. (2014) Protein mistranslation: friend or foe? Trends Biochem Sci 39: 355–362. doi: 10.1016/j.tibs.2014.06.002
![]() |
[33] |
Anfinsen CB (1973) Principles that govern the folding of protein chains. Science 181: 223–230. doi: 10.1126/science.181.4096.223
![]() |
[34] |
Bulik S, Peters B, Holzhutter HG (2005) Quantifying the Contribution of Defective Ribosomal Products to Antigen Production: A Model-Based Computational Analysis. J Immunol 175: 7957–7964. doi: 10.4049/jimmunol.175.12.7957
![]() |
[35] |
Vabulas RM, Hartl FU (2005) Protein synthesis upon acute nutrient restriction relies on proteasome function. Science 310: 1960–1963. doi: 10.1126/science.1121925
![]() |
[36] |
Schubert U, Antón LC, Gibbs J, et al. (2000) Rapid degradation of a large fraction of newly synthesized proteins by proteasomes. Nature 404: 770–774. doi: 10.1038/35008096
![]() |
[37] |
Vabulas RM, Hartl UF (2005) Protein Synthesis upon Acute Nutrient Restriction Relies on Proteasome Function. Science 310: 1960–1963. doi: 10.1126/science.1121925
![]() |
[38] | Hung MC, Link W (2011) Protein localization in disease and therapy. J Cell Sci 124: 3381–3392. |
[39] |
Chacinska A, Koehler CM, Milenkovic D, et al. (2009) Importing mitochondrial proteins: machineries and mechanisms. Cell 138: 628–644. doi: 10.1016/j.cell.2009.08.005
![]() |
[40] |
Rapoport TA (2007) Protein translocation across the eukaryotic endoplasmic reticulum and bacterial plasma membranes. Nature 450: 663–669. doi: 10.1038/nature06384
![]() |
[41] |
Geva Y, Schuldiner M (2014) The back and forth of cargo exit from the endoplasmic reticulum. Curr Biol 24: 130–136. doi: 10.1016/j.cub.2013.12.008
![]() |
[42] |
Barlowe C, Helenius A (2016) Cargo Capture and Bulk Flow in the Early Secretory Pathway. Annu Rev Cell Dev Biol 32: 197–222. doi: 10.1146/annurev-cellbio-111315-125016
![]() |
[43] |
Herrmann JM, Neupert W (2000) Protein transport into mitochondria. Curr Opin Microbiol 3: 210–214. doi: 10.1016/S1369-5274(00)00077-1
![]() |
[44] |
Zimmermann R, Eyrisch S, Ahmad M, et al. (2011) Protein translocation across the ER membrane. Biochim Biophys Acta 1808: 912–924. doi: 10.1016/j.bbamem.2010.06.015
![]() |
[45] |
Freitas N, Cunha C (2009) Mechanisms and signals for the nuclear import of proteins. Curr Genomics 10: 550–557. doi: 10.2174/138920209789503941
![]() |
[46] |
Nichols WC, Seligsohn U, Zivelin A, et al. (1998) Mutations in the ER-Golgi intermediate compartment protein ERGIC-53 cause combined deficiency of coagulation factors V and VIII. Cell 93: 61–70. doi: 10.1016/S0092-8674(00)81146-0
![]() |
[47] |
Spreafico M, Peyvandi F (2009) Combined Factor V and Factor VIII Deficiency. Semin Thromb Hemost 35: 390–399. doi: 10.1055/s-0029-1225761
![]() |
[48] |
Rock KL, Gramm C, Rothstein L, et al. (1994) Inhibitors of the proteasome block the degradation of most cell proteins and the generation of peptides presented on MHC class I molecules. Cell 78: 761–771. doi: 10.1016/S0092-8674(94)90462-6
![]() |
[49] |
Goldberg AL (2003) Protein degradation and protection against misfolded or damaged proteins. Nature 426: 895–899. doi: 10.1038/nature02263
![]() |
[50] |
Finley D (2009) Recognition and processing of ubiquitin-protein conjugates by the proteasome. Annu Rev Biochem 78: 477–513. doi: 10.1146/annurev.biochem.78.081507.101607
![]() |
[51] |
Rock KL, Farfan-Arribas DJ, Colbert JD, et al. (2014) Re-examining class-I presentation and the DRiP hypothesis. Trends Immunol 35: 144–152. doi: 10.1016/j.it.2014.01.002
![]() |
[52] | Cohen-Kaplan V, Livneh I, Avni N, et al. (2016) The ubiquitin-proteasome system and autophagy: Coordinated and independent activities. Int J Biochem Cell Biol: In Press. |
[53] |
Ravikumar B, Sarkar S, Davies JE, et al. (2010) Regulation of mammalian autophagy in physiology and pathophysiology. Physiol Rev 90: 1383–1435. doi: 10.1152/physrev.00030.2009
![]() |
[54] |
Mariappan M, Li X, Stefanovic S, et al. (2010) A ribosome-associating factor chaperones tail-anchored membrane proteins. Nature 466: 1120–1124. doi: 10.1038/nature09296
![]() |
[55] |
Brandman O, Stewart-Ornstein J, Wong D, et al. (2012) A ribosome-bound quality control complex triggers degradation of nascent peptides and signals translation stress. Cell 151: 1042–1054. doi: 10.1016/j.cell.2012.10.044
![]() |
[56] |
Defenouillère Q, Yao Y, Mouaikel J, et al. (2013) Cdc48 associated complex bound to 60s particles is required for the clearance of aberrant translation products. PNAS 110: 5046–5051. doi: 10.1073/pnas.1221724110
![]() |
[57] |
Shao S, von der Malsburg K, Hegde RS (2013) Listerin-dependent nascent protein ubiquitination relies on ribosome subunit dissociation. Mol Cell 50: 637–648. doi: 10.1016/j.molcel.2013.04.015
![]() |
[58] | Verma R, Oania RS, Kolawa1 NJ, et al. (2013) Cdc48/p97 promotes degradation of aberrant nascent polypeptides bound to the ribosome. eLife 2: e00308. |
[59] | Shen PS, Park J, Qin Y, et al. (2016) Rqc2p and 60S ribosomal subunits mediate mRNA-independent elongation of nascent chains. Science 347: 75–78. |
[60] |
Ghaemmaghami S, Huh WK, Bower K, et al. (2003) Global analysis of protein expression in yeast. Nature 425: 737–741. doi: 10.1038/nature02046
![]() |
[61] |
Schwarz F, Aebi M (2011) Mechanisms and principles of N-linked protein glycosylation. Curr Opin Struct Biol 21: 576–582. doi: 10.1016/j.sbi.2011.08.005
![]() |
[62] |
Tannous A, Pisoni GB, Hebert DN, et al. (2015) N-linked sugar-regulated protein folding and quality control in the ER. Semin Cell Dev Biol 41: 79–89. doi: 10.1016/j.semcdb.2014.12.001
![]() |
[63] |
Ellgaard L, Molinari M, Helenius A (1999) Setting the standards: quality control in the secretory pathway. Science 286: 1882–1888. doi: 10.1126/science.286.5446.1882
![]() |
[64] |
Aebi M, Bernasconi R, Clerc S, et al. (2010) N-glycan structures: recognition and processing in the ER. Trends Biochem Sci 35: 74–82. doi: 10.1016/j.tibs.2009.10.001
![]() |
[65] |
Schallus T, Feher K, Sternberg U, et al. (2010) Analysis of the specific interactions between the lectin domain of malectin and diglucosides. Glycobiology 20: 1010–1020. doi: 10.1093/glycob/cwq059
![]() |
[66] |
Galli C, Bernasconi R, Solda T, et al. (2011) Malectin participates in a backup glycoprotein quality control pathway in the mammalian ER. PLoS One 6: e16304. doi: 10.1371/journal.pone.0016304
![]() |
[67] |
Pisoni GB, Ruddock LW, Bulleid N, et al. (2015) Division of labor among oxidoreductases: TMX1 preferentially acts on transmembrane polypeptides. Mol Biol Cell 26: 3390–3400. doi: 10.1091/mbc.E15-05-0321
![]() |
[68] |
Lamriben L, Graham JB, Adams BM, et al. (2016) N-Glycan-based ER Molecular Chaperone and Protein Quality Control System: The Calnexin Binding Cycle. Traffic 17: 308–326. doi: 10.1111/tra.12358
![]() |
[69] |
Cabral CM, Choudhury P, Liu Y, et al. (2000) Processing by endoplasmic reticulum mannosidases partitions a secretion-impaired glycoprotein into distinct disposal pathways. J Biol Chem 275: 25015–25022. doi: 10.1074/jbc.M910172199
![]() |
[70] |
Olivari S, Cali T, Salo KE, et al. (2006) EDEM1 regulates ER-associated degradation by accelerating de-mannosylation of folding-defective polypeptides and by inhibiting their covalent aggregation. Biochem Biophys Res Commun 349: 1278–1284. doi: 10.1016/j.bbrc.2006.08.186
![]() |
[71] |
Ninagawa S, Okada T, Sumitomo Y, et al. (2014) EDEM2 initiates mammalian glycoprotein ERAD by catalyzing the first mannose trimming step. J Cell Biol 206: 347–356. doi: 10.1083/jcb.201404075
![]() |
[72] |
Hirao K, Natsuka Y, Tamura T, et al. (2006) EDEM3, a soluble EDEM homolog, enhances glycoprotein endoplasmic reticulum-associated degradation and mannose trimming. J Biol Chem 281: 9650–9658. doi: 10.1074/jbc.M512191200
![]() |
[73] |
Olivari S, Molinari M (2007) Glycoprotein folding and the role of EDEM1, EDEM2 and EDEM3 in degradation of folding-defective glycoproteins. FEBS Lett 581: 3658–3664. doi: 10.1016/j.febslet.2007.04.070
![]() |
[74] |
Christianson JC, Shaler TA, Tyler RE, et al. (2008) OS-9 and GRP94 deliver mutant alpha1-antitrypsin to the Hrd1-SEL1L ubiquitin ligase complex for ERAD. Nat Cell Biol 10: 272–282. doi: 10.1038/ncb1689
![]() |
[75] |
Bernasconi R, Galli C, Calanca V, et al. (2010) Stringent requirement for HRD1, SEL1L, and OS-9/XTP3-B for disposal of ERAD-LS substrates. J Cell Biol 188: 223–235. doi: 10.1083/jcb.200910042
![]() |
[76] |
Vembar SS, Brodsky JL (2008) One step at a time: endoplasmic reticulum-associated degradation. Nat Rev Mol Cell Biol 9: 944–957. doi: 10.1038/nrm2546
![]() |
[77] |
Merulla J, Solda T, Molinari M (2015) A novel UGGT1 and p97-dependent checkpoint for native ectodomains with ionizable intramembrane residue. Mol Biol Cell 26: 1532–1542. doi: 10.1091/mbc.E14-12-1615
![]() |
[78] |
Merulla J, Fasana E, Solda T, et al. (2013) Specificity and regulation of the endoplasmic reticulum-associated degradation machinery. Traffic 14: 767–777. doi: 10.1111/tra.12068
![]() |
[79] |
Bernasconi R, Molinari M (2011) ERAD and ERAD tuning: disposal of cargo and of ERAD regulators from the mammalian ER. Curr Opin Cell Biol 23: 176–183. doi: 10.1016/j.ceb.2010.10.002
![]() |
[80] |
Koenig PA, Nicholls PK, Schmidt FI, et al. (2014) The E2 ubiquitin-conjugating enzyme UBE2J1 is required for spermiogenesis in mice. J Biol Chem 289: 34490–34502. doi: 10.1074/jbc.M114.604132
![]() |
[81] |
Hagiwara M, Ling J, Koenig PA, et al. (2016) Posttranscriptional Regulation of Glycoprotein Quality Control in the Endoplasmic Reticulum Is Controlled by the E2 Ub-Conjugating Enzyme UBC6e. Mol Cell 63: 753–767. doi: 10.1016/j.molcel.2016.07.014
![]() |
[82] |
Glick D, Barth S, Macleod KF (2010) Autophagy: cellular and molecular mechanisms. J Pathol 221: 3–12. doi: 10.1002/path.2697
![]() |
[83] |
Mizushima N, Ohsumi Y, Yoshimori T (2002) Autophagosome Formation in Mammalian Cells. Cell Struct Funct 27: 421–429. doi: 10.1247/csf.27.421
![]() |
[84] |
Ohsumi Y (2014) Historical landmarks of autophagy research. Cell Res 24: 9–23. doi: 10.1038/cr.2013.169
![]() |
[85] | Ariosa AR, Klionsky DJ (2016) Autophagy core machinery: overcoming spatial barriers in neurons. J Mol Med (Berl): In Press. |
[86] |
Ryter SW, Cloonan SM, Choi AM (2013) Autophagy: a critical regulator of cellular metabolism and homeostasis. Mol Cells 36: 7–16. doi: 10.1007/s10059-013-0140-8
![]() |
[87] |
Choi AM, Ryter SW, Levine B (2013) Autophagy in human health and disease. N Engl J Med 368: 1845–1846. doi: 10.1056/NEJMc1303158
![]() |
[88] | Lin F, Qin ZH (2013) Degradation of misfolded proteins by autophagy: is it a strategy for Huntington's disease treatment? J Huntingtons Dis 2: 149–157. |
[89] |
Webb JL, Ravikumar B, Atkins J, et al. (2003) Alpha-Synuclein is degraded by both autophagy and the proteasome. J Biol Chem 278: 25009–25013. doi: 10.1074/jbc.M300227200
![]() |
[90] | Pickford F, Masliah E, Britschgi M, et al. (2008) The autophagy-related protein beclin 1 shows reduced expression in early Alzheimer disease and regulates amyloid beta accumulation in mice. J Clin Invest 118: 2190–2199. |
[91] |
Lee MJ, Lee JH, Rubinsztein DC (2013) Tau degradation: the ubiquitin-proteasome system versus the autophagy-lysosome system. Prog Neurobiol 105: 49–59. doi: 10.1016/j.pneurobio.2013.03.001
![]() |
[92] |
Perlmutter DH (2011) Alpha-1-antitrypsin deficiency: importance of proteasomal and autophagic degradative pathways in disposal of liver disease-associated protein aggregates. Annu Rev Med 62: 333–345. doi: 10.1146/annurev-med-042409-151920
![]() |
[93] |
Fu L, Sztul E (2009) ER-associated complexes (ERACs) containing aggregated cystic fibrosis transmembrane conductance regulator (CFTR) are degraded by autophagy. Eur J Cell Biol 88: 215–226. doi: 10.1016/j.ejcb.2008.11.003
![]() |
[94] | Farre JC, Subramani S (2016) Mechanistic insights into selective autophagy pathways: lessons from yeast. Nat Rev Mol Cell Biol 17: 537–552. |
[95] |
Khaminets A, Heinrich T, Mari M, et al. (2015) Regulation of endoplasmic reticulum turnover by selective autophagy. Nature 522: 354–358. doi: 10.1038/nature14498
![]() |
[96] | Fumagalli FNJ, Bergmann TJ, Cebollero E, et al. (2016) Translocon component Sec62 acts in endoplasmic reticulum turnover during stress recovery. Nat Cell Biol: In press. |
[97] |
Inoue T, Tsai B (2013) How viruses use the endoplasmic reticulum for entry, replication, and assembly. Cold Spring Harb Perspect Biol 5: a013250. doi: 10.1101/cshperspect.a013250
![]() |
[98] |
van den Boomen DJ, Lehner PJ (2015) Identifying the ERAD ubiquitin E3 ligases for viral and cellular targeting of MHC class I. Mol Immunol 68: 106–111. doi: 10.1016/j.molimm.2015.07.005
![]() |
[99] | Gardner BM, Pincus D, Gotthardt K, et al. (2013) Endoplasmic reticulum stress sensing in the unfolded protein response. Cold Spring Harb Perspect Biol 5: a013169. |
[100] |
Mori K (2009) Signalling pathways in the unfolded protein response: development from yeast to mammals. J Biochem 146: 743–750. doi: 10.1093/jb/mvp166
![]() |
[101] |
Zhang L, Zhang C, Wang A (2016) Divergence and Conservation of the Major UPR Branch IRE1-bZIP Signaling Pathway across Eukaryotes. Sci Rep 6: 27362. doi: 10.1038/srep27362
![]() |
[102] |
Lee AH, Iwakoshi NN, Glimcher LH (2003) XBP-1 regulates a subset of endoplasmic reticulum resident chaperone genes in the unfolded protein response. Mol Cell Biol 23: 7448–7459. doi: 10.1128/MCB.23.21.7448-7459.2003
![]() |
[103] |
Hollien J, Weissman JS (2006) Decay of endoplasmic reticulum-localized mRNAs during the unfolded protein response. Science 313: 104–107. doi: 10.1126/science.1129631
![]() |
[104] |
Haze K, Yoshida H, Yanagi H, et al. (1999) Mammalian transcription factor ATF6 is synthesized as a transmembrane protein and activated by proteolysis in response to endoplasmic reticulum stress. Mol Biol Cell 10: 3787–3799. doi: 10.1091/mbc.10.11.3787
![]() |
[105] |
Shoulders MD, Ryno LM, Genereux JC, et al. (2013) Stress-independent activation of XBP1s and/or ATF6 reveals three functionally diverse ER proteostasis environments. Cell Rep 3: 1279–1292. doi: 10.1016/j.celrep.2013.03.024
![]() |
[106] |
Bertolotti A, Zhang Y, Hendershot LM, et al. (2000) Dynamic interaction of BiP and ER stress transducers in the unfolded-protein response. Nat Cell Biol 2: 326–332. doi: 10.1038/35014014
![]() |
[107] |
Harding HP, Zhang Y, Ron D (1999) Protein translation and folding are coupled by an endoplasmic-reticulum-resident kinase. Nature 397: 271–274. doi: 10.1038/16729
![]() |
[108] |
Vattem KM, Wek RC (2004) Reinitiation involving upstream ORFs regulates ATF4 mRNA translation in mammalian cells. Proc Natl Acad Sci U S A 101: 11269–11274. doi: 10.1073/pnas.0400541101
![]() |
[109] |
Lu PD, Harding HP, Ron D (2004) Translation reinitiation at alternative open reading frames regulates gene expression in an integrated stress response. J Cell Biol 167: 27–33. doi: 10.1083/jcb.200408003
![]() |
[110] |
Jiang HY, Wek SA, McGrath BC, et al. (2004) Activating transcription factor 3 is integral to the eukaryotic initiation factor 2 kinase stress response. Mol Cell Biol 24: 1365–1377. doi: 10.1128/MCB.24.3.1365-1377.2004
![]() |
[111] |
Schroder M, Kaufman RJ (2005) ER stress and the unfolded protein response. Mutat Res 569: 29–63. doi: 10.1016/j.mrfmmm.2004.06.056
![]() |
[112] |
Redler RL, Das J, Diaz JR, et al. (2016) Protein Destabilization as a Common Factor in Diverse Inherited Disorders. J Mol Evol 82: 11–16. doi: 10.1007/s00239-015-9717-5
![]() |
[113] |
Sitia R, Braakman I (2003) Quality control in the endoplasmic reticulum protein factory. Nature 426: 891–894. doi: 10.1038/nature02262
![]() |
[114] |
Bernier V, Lagace M, Bichet DG, et al. (2004) Pharmacological chaperones: potential treatment for conformational diseases. Trends Endocrinol Metab 15: 222–228. doi: 10.1016/j.tem.2004.05.003
![]() |
[115] |
Molinari M (2007) N-glycan structure dictates extension of protein folding or onset of disposal. Nat Chem Biol 3: 313–320. doi: 10.1038/nchembio880
![]() |
[116] |
Kopito RR, Ron D (2000) Conformational disease. Nat Cell Biol 2: 207–209. doi: 10.1038/35041139
![]() |
[117] |
Gidalevitz T, Ben-Zvi A, Ho KH, et al. (2006) Progressive disruption of cellular protein folding in models of polyglutamine diseases. Science 311: 1471–1474. doi: 10.1126/science.1124514
![]() |
[118] |
Powers ET, Morimoto RI, Dillin A, et al. (2009) Biological and chemical approaches to diseases of proteostasis deficiency. Annu Rev Biochem 78: 959–991. doi: 10.1146/annurev.biochem.052308.114844
![]() |
[119] |
Morello JP, Petaja-Repo UE, Bichet DG, et al. (2000) Pharmacological chaperones: a new twist on receptor folding. Trends Pharmacol Sci 21: 466–469. doi: 10.1016/S0165-6147(00)01575-3
![]() |
[120] |
Cohen FE, Kelly JW (2003) Therapeutic approaches to protein-misfolding diseases. Nature 426: 905–909. doi: 10.1038/nature02265
![]() |
[121] |
Convertino M, Das J, Dokholyan NV (2016) Pharmacological Chaperones: Design and Development of New Therapeutic Strategies for the Treatment of Conformational Diseases. ACS Chem Biol 11: 1471–1489. doi: 10.1021/acschembio.6b00195
![]() |
[122] |
Ma Y, Hendershot LM (2004) The role of the unfolded protein response in tumour development: friend or foe? Nat Rev Cancer 4: 966–977. doi: 10.1038/nrc1505
![]() |
[123] |
Fernandez PM, Tabbara SO, Jacobs LK, et al. (2000) Overexpression of the glucose-regulated stress gene GRP78 in malignant but not benign human breast lesions. Breast Cancer Res Treat 59: 15–26. doi: 10.1023/A:1006332011207
![]() |
[124] | Shuda M, Kondoh N, Imazeki N, et al. (2003) Activation of the ATF6, XBP1 and grp78 genes in human hepatocellular carcinoma: a possible involvement of the ER stress pathway in hepatocarcinogenesis. J Hepatol 38: 605–614. |
[125] | Song MS, Park YK, Lee JH, et al. (2001) Induction of glucose-regulated protein 78 by chronic hypoxia in human gastric tumor cells through a protein kinase C-epsilon/ERK/AP-1 signaling cascade. Cancer Res 61: 8322–8330. |
[126] |
Gazit G, Lu J, Lee AS (1999) De-regulation of GRP stress protein expression in human breast cancer cell lines. Breast Cancer Res Treat 54: 135–146. doi: 10.1023/A:1006102411439
![]() |
[127] | Plate L, Paxman RJ, Wiseman RL, et al. (2016) Modulating protein quality control. Elife 5: e18431. |
[128] |
Wang X, Venable J, LaPointe P, et al. (2006) Hsp90 cochaperone Aha1 downregulation rescues misfolding of CFTR in cystic fibrosis. Cell 127: 803–815. doi: 10.1016/j.cell.2006.09.043
![]() |
[129] |
Mu TW, Ong DS, Wang YJ, et al. (2008) Chemical and biological approaches synergize to ameliorate protein-folding diseases. Cell 134: 769–781. doi: 10.1016/j.cell.2008.06.037
![]() |
[130] |
Chiang WC, Hiramatsu N, Messah C, et al. (2012) Selective activation of ATF6 and PERK endoplasmic reticulum stress signaling pathways prevent mutant rhodopsin accumulation. Invest Ophthalmol Vis Sci 53: 7159–7166. doi: 10.1167/iovs.12-10222
![]() |
[131] |
Luheshi LM, Dobson CM (2009) Bridging the gap: from protein misfolding to protein misfolding diseases. FEBS Lett 583: 2581–2586. doi: 10.1016/j.febslet.2009.06.030
![]() |
[132] |
Braakman I, Bulleid NJ (2011) Protein folding and modification in the mammalian endoplasmic reticulum. Annu Rev Biochem 80: 71–99. doi: 10.1146/annurev-biochem-062209-093836
![]() |
[133] |
Brodsky JL, Skach WR (2011) Protein folding and quality control in the endoplasmic reticulum: Recent lessons from yeast and mammalian cell systems. Curr Opin Cell Biol 23: 464–475. doi: 10.1016/j.ceb.2011.05.004
![]() |
[134] |
Papa FR, Zhang C, Shokat K, et al. (2003) Bypassing a kinase activity with an ATP-competitive drug. Science 302: 1533–1537. doi: 10.1126/science.1090031
![]() |
[135] |
Wiseman RL, Zhang Y, Lee KP, et al. (2010) Flavonol activation defines an unanticipated ligand-binding site in the kinase-RNase domain of IRE1. Mol Cell 38: 291–304. doi: 10.1016/j.molcel.2010.04.001
![]() |
[136] |
Wang L, Perera BG, Hari SB, et al. (2012) Divergent allosteric control of the IRE1alpha endoribonuclease using kinase inhibitors. Nat Chem Biol 8: 982–989. doi: 10.1038/nchembio.1094
![]() |
[137] | Sidrauski C, Tsai JC, Kampmann M, et al. (2015) Pharmacological dimerization and activation of the exchange factor eIF2B antagonizes the integrated stress response. Elife 4: e07314. |
[138] |
Robblee MM, Kim CC, Porter Abate J, et al. (2016) Saturated Fatty Acids Engage an IRE1alpha-Dependent Pathway to Activate the NLRP3 Inflammasome in Myeloid Cells. Cell Rep 14: 2611–2623. doi: 10.1016/j.celrep.2016.02.053
![]() |
[139] | Gallagher CM, Walter P (2016) Ceapins inhibit ATF6alpha signaling by selectively preventing transport of ATF6alpha to the Golgi apparatus during ER stress. Elife 5: e11880. |
[140] | Gallagher CM, Garri C, Cain EL, et al. (2016) Ceapins are a new class of unfolded protein response inhibitors, selectively targeting the ATF6alpha branch. Elife 5: e11880. |
[141] | Plate L, Cooley CB, Chen JJ, et al. (2016) Small molecule proteostasis regulators that reprogram the ER to reduce extracellular protein aggregation. Elife 5: e15550. |
[142] |
Hsu PD, Lander ES, Zhang F (2014) Development and applications of CRISPR-Cas9 for genome engineering. Cell 157: 1262–1278. doi: 10.1016/j.cell.2014.05.010
![]() |
1. | Tielin Lu, yue lei, Xiaojing liu, yujia shang, xiwei feng, jie gao, Xuejun Zhang, Xiaoyong Wang, Yifan Dai, Lihua Li, Lingbao Kong, Dawei Zhang, Feng Gong, 2023, Research on unattended vision inspection system for petrochemical industry based on 5G, 9781510672673, 42, 10.1117/12.3008807 | |
2. | Xintian Wang, Zhiqiang Geng, Liangchao Chen, Yongming Han, Improved dragonfly optimization algorithm based on quantum behavior for multi-objective optimization of ethylene cracking furnace, 2024, 88, 22106502, 101607, 10.1016/j.swevo.2024.101607 | |
3. | Chanin Panjapornpon, Chutithep Rochpuang, Santi Bardeeniz, Mohamed Azlan Hussain, Machine learning approach with a posteriori-based feature to predict service life of a thermal cracking furnace with coking deposition, 2024, 22, 25901230, 102349, 10.1016/j.rineng.2024.102349 | |
4. | Zhiqiang Geng, Weikang Kong, Xintian Wang, Ling Wang, Yongming Han, Adaptive search based Grey Wolf optimization algorithm for multi-objective optimization of ethylene cracking furnace, 2025, 92, 22106502, 101810, 10.1016/j.swevo.2024.101810 |
Layer (type) | Output Shape | Param |
conv2d_input (InputLayer) | [(n, 200,200, 3)] | 0 |
conv2d (Conv2D) | (n, 198,198, 16) | 448 |
max_pooling2d (MaxPooling2D) | (n, 99, 99, 16) | 0 |
conv2d_1 (Conv2D) | (n, 97, 97, 32) | 4640 |
max_pooling2d_1 (MaxPooling2) | (n, 48, 48, 32) | 0 |
conv2d_2 (Conv2D) | (n, 46, 46, 64) | 18,496 |
max_pooling2d_2 (MaxPooling2) | (n, 23, 23, 64) | 0 |
flatten (Flatten) | (n, 33856) | 0 |
dense (Dense) | (n, 512) | 17,334,784 |
dense_1 (Dense) | (n, 1) | 513 |
Parameter | Value | Parameter | Value |
test_iter | 40 | gamma | 0.0001 |
base_lr | 0.001 | power | 0.75 |
momentum | 0.9 | display | 500 |
weight_decay | 0.0005 | max_iter | 10,000 |
Sample | Normal | Overlapped | Total |
Training | 1,456 | 608 | 2064 |
Test | 1,028 | 412 | 1440 |
Parameter | Value | Parameter | Value |
Train time Learning rate Discount factor Target network update interval Experience pool size |
1000 0.001 0.95 100 30000 |
Greedy factor ɛ initial value Greedy factor ɛ max value Ɛ amplification Round number Mini-batch |
0.5 0.9 0.001 20 32 |
Net layer | Convolution layer | Maximum pooling layer | Full connection layer |
Input size Convolution kernel size Step size Number fo convolution kernel Activation function Output size |
180 * 20 2 × 2 (1, 1) 8 ReLU 178*18 |
178 * 18 2 × 2 (2, 2) -- -- 89*9 |
89 * 9 -- -- -- -- 21 |
Function or technology | Next-generation platform | Previous platform |
Network transmission technology | 5G-based | LoRa-based |
Temperature measurement method | Remote automated control in the workshop |
Manual control at the cracking furnace site |
Peep hole operational condition monitoring | Real-time video remote monitoring | Manual on-site visual inspection |
Peep hole initial operation parameter setting | Fully adaptive | Manual measurement |
Self-learning of temperature-measuring device | Strong | Weak |
Peep door | Intelligent type, automatic open and close |
Mechanical type, manual open and close |
Identification of overlapping tubes | ≥ 99.99% | About 80% |
Charging of temperature-measuring device | Automatic | Manual |
Deep network model | Compressed | Not compressed |
Platform structure | Edge-fog-cloud three-layer structure | Central cloud structure |
Computing resource scheduling | Based on deep reinforcement learning, resource utilization is higher | Based on reinforcement learning, resource utilization needs to be improved |
Temperature measurement time per cycle | ≤ 7 min | ~10 min |
Layer (type) | Output Shape | Param |
conv2d_input (InputLayer) | [(n, 200,200, 3)] | 0 |
conv2d (Conv2D) | (n, 198,198, 16) | 448 |
max_pooling2d (MaxPooling2D) | (n, 99, 99, 16) | 0 |
conv2d_1 (Conv2D) | (n, 97, 97, 32) | 4640 |
max_pooling2d_1 (MaxPooling2) | (n, 48, 48, 32) | 0 |
conv2d_2 (Conv2D) | (n, 46, 46, 64) | 18,496 |
max_pooling2d_2 (MaxPooling2) | (n, 23, 23, 64) | 0 |
flatten (Flatten) | (n, 33856) | 0 |
dense (Dense) | (n, 512) | 17,334,784 |
dense_1 (Dense) | (n, 1) | 513 |
Parameter | Value | Parameter | Value |
test_iter | 40 | gamma | 0.0001 |
base_lr | 0.001 | power | 0.75 |
momentum | 0.9 | display | 500 |
weight_decay | 0.0005 | max_iter | 10,000 |
Sample | Normal | Overlapped | Total |
Training | 1,456 | 608 | 2064 |
Test | 1,028 | 412 | 1440 |
Parameter | Value | Parameter | Value |
Train time Learning rate Discount factor Target network update interval Experience pool size |
1000 0.001 0.95 100 30000 |
Greedy factor ɛ initial value Greedy factor ɛ max value Ɛ amplification Round number Mini-batch |
0.5 0.9 0.001 20 32 |
Net layer | Convolution layer | Maximum pooling layer | Full connection layer |
Input size Convolution kernel size Step size Number fo convolution kernel Activation function Output size |
180 * 20 2 × 2 (1, 1) 8 ReLU 178*18 |
178 * 18 2 × 2 (2, 2) -- -- 89*9 |
89 * 9 -- -- -- -- 21 |
Function or technology | Next-generation platform | Previous platform |
Network transmission technology | 5G-based | LoRa-based |
Temperature measurement method | Remote automated control in the workshop |
Manual control at the cracking furnace site |
Peep hole operational condition monitoring | Real-time video remote monitoring | Manual on-site visual inspection |
Peep hole initial operation parameter setting | Fully adaptive | Manual measurement |
Self-learning of temperature-measuring device | Strong | Weak |
Peep door | Intelligent type, automatic open and close |
Mechanical type, manual open and close |
Identification of overlapping tubes | ≥ 99.99% | About 80% |
Charging of temperature-measuring device | Automatic | Manual |
Deep network model | Compressed | Not compressed |
Platform structure | Edge-fog-cloud three-layer structure | Central cloud structure |
Computing resource scheduling | Based on deep reinforcement learning, resource utilization is higher | Based on reinforcement learning, resource utilization needs to be improved |
Temperature measurement time per cycle | ≤ 7 min | ~10 min |