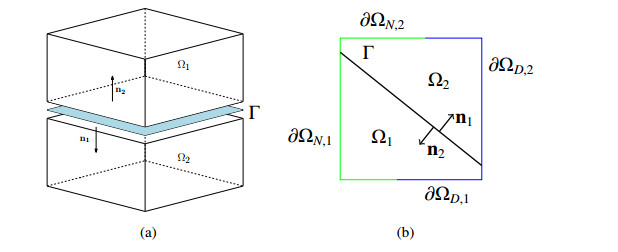
Biofilms are aggregates of bacteria, in most cases, which are resistant usually to broad-spectrum antibiotics in their typical concentrations or even in higher doses. A trend of increasing multi-drug resistance in biofilms, which are responsible for emerging life-threatening nosocomial infections, is becoming a serious problem. Biofilms, however, are at various sensitivity levels to environmental factors and are versatile in infectivity depending on virulence factors. This review presents the fundamental information about biofilms: formation, antibiotic resistance, impacts on public health and alternatives to conventional approaches. Novel developments in micro-biosystems that help reveal the new treatment tools by sensing and characterization of biofilms will also be discussed. Understanding the formation, structure, physiology and properties of biofilms better helps eliminate them by the usage of appropriate antibiotics or their control by novel therapy approaches, such as anti-biofilm molecules, effective gene editing, drug-delivery systems and probiotics.
Citation: Ruba Mirghani, Tania Saba, Hebba Khaliq, Jennifer Mitchell, Lan Do, Liz Chambi, Kelly Diaz, Taylor Kennedy, Katia Alkassab, Thuhue Huynh, Mohamed Elmi, Jennifer Martinez, Suad Sawan, Girdhari Rijal. Biofilms: Formation, drug resistance and alternatives to conventional approaches[J]. AIMS Microbiology, 2022, 8(3): 239-277. doi: 10.3934/microbiol.2022019
[1] | Wenhui Feng, Xingfa Zhang, Yanshan Chen, Zefang Song . Linear regression estimation using intraday high frequency data. AIMS Mathematics, 2023, 8(6): 13123-13133. doi: 10.3934/math.2023662 |
[2] | Zubair Ahmad, Zahra Almaspoor, Faridoon Khan, Sharifah E. Alhazmi, M. El-Morshedy, O. Y. Ababneh, Amer Ibrahim Al-Omari . On fitting and forecasting the log-returns of cryptocurrency exchange rates using a new logistic model and machine learning algorithms. AIMS Mathematics, 2022, 7(10): 18031-18049. doi: 10.3934/math.2022993 |
[3] | Xin Liang, Xingfa Zhang, Yuan Li, Chunliang Deng . Daily nonparametric ARCH(1) model estimation using intraday high frequency data. AIMS Mathematics, 2021, 6(4): 3455-3464. doi: 10.3934/math.2021206 |
[4] | Carey Caginalp, Gunduz Caginalp . Establishing cryptocurrency equilibria through game theory. AIMS Mathematics, 2019, 4(3): 420-436. doi: 10.3934/math.2019.3.420 |
[5] | Carey Caginalp . A dynamical systems approach to cryptocurrency stability. AIMS Mathematics, 2019, 4(4): 1065-1077. doi: 10.3934/math.2019.4.1065 |
[6] | Kai Xiao . Risk-seeking insider trading with partial observation in continuous time. AIMS Mathematics, 2023, 8(11): 28143-28152. doi: 10.3934/math.20231440 |
[7] | Kai Xiao, Yonghui Zhou . Linear Bayesian equilibrium in insider trading with a random time under partial observations. AIMS Mathematics, 2021, 6(12): 13347-13357. doi: 10.3934/math.2021772 |
[8] | Huayu Sun, Fanqi Zou, Bin Mo . Does FinTech drive asymmetric risk spillover in the traditional finance?. AIMS Mathematics, 2022, 7(12): 20850-20872. doi: 10.3934/math.20221143 |
[9] | Erlin Guo, Cuixia Li, Patrick Ling, Fengqin Tang . Convergence rate for integrated self-weighted volatility by using intraday high-frequency data with noise. AIMS Mathematics, 2023, 8(12): 31070-31091. doi: 10.3934/math.20231590 |
[10] | Stelios Arvanitis, Michalis Detsis . Mild explocivity, persistent homology and cryptocurrencies' bubbles: An empirical exercise. AIMS Mathematics, 2024, 9(1): 896-917. doi: 10.3934/math.2024045 |
Biofilms are aggregates of bacteria, in most cases, which are resistant usually to broad-spectrum antibiotics in their typical concentrations or even in higher doses. A trend of increasing multi-drug resistance in biofilms, which are responsible for emerging life-threatening nosocomial infections, is becoming a serious problem. Biofilms, however, are at various sensitivity levels to environmental factors and are versatile in infectivity depending on virulence factors. This review presents the fundamental information about biofilms: formation, antibiotic resistance, impacts on public health and alternatives to conventional approaches. Novel developments in micro-biosystems that help reveal the new treatment tools by sensing and characterization of biofilms will also be discussed. Understanding the formation, structure, physiology and properties of biofilms better helps eliminate them by the usage of appropriate antibiotics or their control by novel therapy approaches, such as anti-biofilm molecules, effective gene editing, drug-delivery systems and probiotics.
Many geophysical and engineering applications, including, for example, fluid-structure interaction, crack and wave propagation problems, and flow in fractured porous media, are characterized by a strong complexity of the physical domain, possibly involving thousands of fault/fractures, heterogeneous media, moving geometries/interfaces and complex topographies. Whenever classical Finite-Element-based approaches are employed to discretize the underlying differential model, the process of mesh generation can be the bottleneck of the whole simulation, as classical finite elements only support computational grids composed by tetrahedral/hexahedral/prismatic elements. To overcome this limitation, in the last decade a wide strand of literature focused on the design of numerical methods that support computational meshes composed of general polygonal and polyhedral (polytopic, for short) elements. In the conforming setting, we mention for example the composite finite element method [1,2], the mimetic finite difference method [3,4,5,6,7,8], the polygonal finite element method [9], the extended finite element method [10,11,12], the virtual element method [13,14,15,16,17] and the hybrid high-order method [18,19,20,21]. In the setting of non-conforming/discontinuos polygonal methods, we mention, for example, hybridizable discontinuous Galerkin methods [22,23,24,25], composite discontinuous finite element methods [26,27], non-conforming VEM [28,29,30], gradient schemes [31] and the polytopic discontinuous Galerkin method [32,33,34,35,36,37,38,39,40,41,42,43,44].
Within this framework, we focus our attention on the problem of modelling the flow in a fractured porous medium, which is fundamental in many energy or environmental engineering applications, such as tracing oil migration, isolation of radioactive waste, groundwater contamination, etc. Fractures are regions of the porous medium that are typically characterized both by a different porous structure and by a very small width. The first feature implies that fractures have a very strong impact on the flow, since they can possibly act as barriers or as preferential paths for the fluid. The second feature entails the need for a very large number of elements for the discretization of the fracture layer and, consequently, a high computational cost. For this reason, one popular modelling choice consists in a reduction strategy, so that fractures are treated as (d−1)-dimensional interfaces between d-dimensional porous matrices, d=2,3. In particular, we refer to the model for single-phase flow developed in [45,46,47,48]. Here, the flow in the porous medium (bulk) is assumed to be governed by Darcy's law and a suitable reduced version of the law is formulated on the surface modelling the single fracture. The two problems are then coupled through physically consistent conditions to account for the exchange of fluid between them. We remark that this model is able to handle both fractures with low and large permeability. Moreover, its extension to the case of two-phase flows has been addressed in [49,50], while the case of a totally immersed fracture has been considered e.g., in [51].
Even if the use of this kind of dimensionally reduced models avoids the need for extremely refined grids inside the fracture domains, in realistic cases, the construction of a computational grid aligned with the fractures is still a major issue. For example, a fractured oil reservoir can be cut by several thousands of fractures, which often intersect, create small angles or are nearly coincident [52]. In line with the previous discussion, in order to avoid the limitations imposed by standard finite element methods, various numerical methods supporting polytopic elements have been employed in the literature for the approximation of the coupled bulk-fracture problem. For example, in [8,52] a mixed approximation based on the use of mimetic finite difference method has been explored; in [53,54] a framework for treating flows in discrete fracture networks based on the virtual element method has been introduced, and in [55] the hybrid high-order method has been employed. We also mention that an alternative strategy consists in the use of non-conforming discretizations, where fractures are allowed to arbitrarily cut the bulk grid, which can then be chosen fairly regular. In particular, we refer to [49,56,57], where an approximation employing extended finite element method (XFEM) has been proposed and to the recent work [58], where the use of the cut finite element method (CUTFEM) has been explored.
Recently in [59], in the setting of conforming discretizations, we developed a numerical approximation of the coupled bulk-fracture model based on polytopic discountinuous Galerkin (PolyDG) methods. In particular, the intrinsic "discontinuous" nature of DG methods allows very general polytopic elements because of the freedom in representing the underlying (local) polynomial space. Indeed the degrees of freedom are not "attached" to any geometric quantity, (vertexes, edges, etcc), so that mesh elements with edges/faces that may be in arbitrary number and whose measure may be arbitrarily small compared to the diameter of the corresponding element are naturally supported with a solid theoretical background. This approach is then very well suited to tame the geometrical complexity featured by most of applications in the computational geoscience field. Moreover, since the interface conditions between the bulk and fracture problem can be naturally formulated using jump and average operators, the coupling of the two problems can be naturally embedded in the variational formulation.
The goal of this paper is to extend the results obtained in [59], where the proposed discretization based on PolyDG was in a primal-primal setting. Indeed, when dealing with the approximation of Darcy's flow there are two possible approaches: primal and mixed. The primal approach considers a single-field formulation with the pressure field of the fluid as only unknown. The mixed approach describes the flow not only through the pressure field, but also through an additional unknown representing Darcy's velocity, which is of primary interest in many engineering applications. The primal setting has the advantage of featuring less degrees of freedom and leads to a symmetric positive definite algebraic system of equations that can be efficiently solved based on employing, for example, multigrid techniques [39,44,60]. In this case, Darcy's velocity is afterwards reconstructed taking the gradient of the computed pressure and multiplying it by the permeability tensor. However, this process usually entails a loss of accuracy and does not guarantee mass conservation, see for example [61,62]. For this reason, the mixed setting is sometimes preferred. In this case Darcy's velocity is directly computed, so that a higher degree of accuracy is achieved, together with local and global mass conservation. However, the drawback of this approach is the complexity of the resulting scheme, which leads to a generalized saddle point algebraic system of equations. From the above discussion we may infer that, according to the desired approximation properties of the model, one may resort to either a primal or mixed approximation for the problem in the bulk, as well as to a primal or mixed approximation for the problem in the fracture. Our aim is then to design and analyze, in the unified framework of [63] based on the flux-formulation, all the possible combinations of primal-primal, mixed-primal, primal-mixed and mixed-mixed formulations for the bulk and fracture problems, respectively. In particular, the primal discretizations are obtained using the symmetric interior penalty discontinuous Galerkin method [64,65], whereas the mixed discretizations are based on employing the local DG (LDG) method of [66], both in their generalization to polytopic grids [36,37,38,40,41]. Moreover, the coupling conditions between bulk and fracture are imposed through a suitable definition of the numerical fluxes on the fracture faces. Such an abstract setting allows to analyse theoretically at the same time all the possible formulations. We perform a unified analysis of all the derived combinations of DG discretizations for the bulk-fracture problem. We prove their well-posedness and derive a priori hp-version error estimates in a suitable (mesh-dependent) energy norm. Finally, we present preliminary numerical experiments assessing the validity of the theoretical error estimates and comparing the accuracy of the proposed formulations on simplified geometries with manufactured solutions.
The rest of the paper is organized as follows. In Section 2 we introduce the model problem; its weak formulation is discussed in Section 3. The discretization based on employing PolyDG methods is presented, in the unified setting of [63], in Section 4. In Section 5, we address the problem of stability and prove that all formulations, namely primal-primal (PP), mixed-primal (MP), primal-mixed (PM) and mixed-mixed (MM) are well-posed. The corresponding error analysis is presented in Section 6. Several numerical tests, focusing, for the sake of brevity, on the primal-primal (PP) and mixed-primal (MP) cases, are presented in Section 7 to confirm the theoretical bounds. Moreover, we assess the capability of the method of handling more complicated geometries, presenting some test cases featuring networks of partially immersed fractures.
For simplicity, we consider the case where the porous medium is cut by a single, non immersed fracture. The extension to the case of a network of disjoint fractures can be treated analogously. The case where the fracture is partially or totally immersed in the domain is more complex to analyze, and we refer to [51,52] for its discussion. Nevertheless, the capability of our method to deal with networks of partially immersed fractures will be explored via numerical experiments in Section 7.4 in the mixed-primal setting (MP). Finally, the case of a network of interecting fractures will be the object of a future work and we refer to [59] for preliminary numerical results (in the primal-primal setting) showing that our method is able to handle also such cases.
The porous matrix is represented by the domain Ω⊂Rd, d=2,3, which we assume to be open, bounded, convex and polygonal/polyhedral. Moreover, following the strategy of [47], we suppose that the fracture may be described by the (d−1)-dimensional C∞ manifold (with no curvature) Γ⊂Rd−1, d=2,3. This approach is justified by the fact that the thickness of the fracture domain is typically some orders of magnitude smaller than the size of the domain. Since we are assuming that Γ is not immersed, it separates Ω into two connected disjoint subdomains, Ω∖Γ=Ω1∪Ω2 with Ω1∩Ω2=∅. We decompose the boundary of Ω into two disjoint subsets ∂ΩD and ∂ΩN, i.e., ∂Ω=∂ΩD∪∂ΩN, with ∂ΩD∩∂ΩN=∅, and we define ∂ΩD,i=∂ΩD∩∂Ωi and ∂ΩN,i=∂ΩN∩∂Ωi, for i=1,2. Finally, we denote by ni, i=1,2 the unit normal vector to Γ pointing outwards from Ωi and, for a (regular enough) scalar-valued function q and a (regular enough) vector-valued function v, we define the classical jump and average operators across the fracture Γ as
{q}=12(q1+q2)[[q]]=q1n1+q2n2,{v}=12(v1+v2)[[v]]=v1⋅n1+v2⋅n2, | (2.1) |
where the subscript i=1,2 denotes the restriction to the subdomain Ωi. Note that, since we are assuming that the fracture is continuously differentiable, it holds n1=−n2. We also denote by nΓ the normal unit vector on Γ with a fixed orientation from Ω1 to Ω2, so that we have nΓ=n1=−n2. In Figure 1 we report an example of domain cut by a single fracture.
We can now introduce the governing equations for our model. In the bulk, we suppose that the flow is governed by Darcy's law. The motion of an incompressible fluid in each domain Ωi, i=1,2, with pressure pi and velocity ui may then be described by:
{ui=νi∇piinΩi,−∇⋅ui=fiinΩi,pi=gD,ion∂ΩD,i,ui⋅ni=0on∂ΩN,i, | (2.2) |
where f∈L2(Ω) represents a source term, gD∈H1/2(∂ΩD) is the Dirichlet boundary datum and ν=ν(x)∈Rd×d is the bulk permeability tensor, which we assume to be symmetric, positive definite, uniformly bounded from below and above and with entries that are bounded, piecewise continuous real-valued functions.
On the manifold Γ representing the fracture, we formulate a reduced version of Darcy's law in the tangential direction (we refer to [47] for a rigorous derivation of the model). To this aim we assume that the fracture permeability tensor νΓ, has a block-diagonal structure of the form
νΓ=[νnΓ00ντΓ], | (2.3) |
when written in its normal and tangential components. Here, ντΓ∈R(d−1)×(d−1) is a positive definite, uniformly bounded tensor (it reduces to a positive number for d=2). Moreover, we assume that νΓ satisfies the same regularity assumptions as those satisfied by the bulk permeability ν. Setting ∂Γ=Γ∩∂Ω, ∂Γ=∂ΓD∪∂ΓN, introducing the fracture thickness ℓΓ>0 and denoting by pΓ and uΓ the fracture pressure and velocity, the governing equations for the fracture flow are
{uΓ=ντΓℓΓ∇τpΓinΓ,−∇τ⋅uΓ=fΓ−[[u]]inΓ,pΓ=gΓon∂ΓD,uΓ⋅τ=0on∂ΓN, | (2.4) |
where fΓ∈L2(Γ), gΓ∈H1/2(∂Γ), τ is vector in the tangent plane of Γ normal to ∂Γ and ∇τ and ∇τ⋅ denote the tangential gradient and divergence operators, respectively.
Finally, following [47], we close the model providing the interface conditions to couple problems (2.2) and (2.4) along their interface. Given a positive real number ξ≠12 that will be chosen later on, the coupling conditions read as follows
−{u}⋅nΓ=βΓ[[p]]⋅nΓonΓ, | (2.5a) |
−[[u]]=αΓ({p}−pΓ)onΓ, | (2.5b) |
where βΓ=12ηΓ and αΓ=2ηΓ(2ξ−1) and ηΓ=ℓΓνnΓ, νnΓ being the normal component of the fracture permeability tensor, see (2.3).
In conclusion, the coupled bulk-fracture model problem is the following:
{ui=νi∇piinΩi,−∇⋅ui=fiinΩi,pi=gD,ionγD,i,ui⋅ni=0onγN,iuΓ=ντΓℓΓ∇τpΓinΓ,−∇τ⋅uΓ=fΓ−[[u]]inΓ,pΓ=gΓon∂ΓD,uΓ⋅τ=0on∂ΓN,−{u}⋅nΓ=βΓ[[p]]⋅nΓonΓ,−[[u]]=αΓ({p}−pΓ)onΓ. | (2.6) |
In this section we introduce the weak formulation of our model problem (2.6) and prove its well-posedness. We start with the introduction of the functional setting.
We will employ the following notation. For an open, bounded domain D⊂Rd, d=2,3, we denote by Hs(D) the standard Sobolev space of order s, for a real number s≥0. For s=0, we write L2(D) in place of H0(D). The usual norm on Hs(D) is denoted by ||⋅||s,D and the usual seminorm by |⋅|s,D. We also introduce the standard space Hdiv(D)={v:D→Rd:||v||0,D+||∇⋅v||0,D<∞}. Given a decomposition of the domain into elements Th, we will denote by Hs(Th) the standard broken Sobolev space, equipped with the broken norm ||⋅||s,Th. Furthermore, we will denote by Pk(D) the space of polynomials of total degree less than or equal to k≥1 on D. The symbol ≲ (and ≳) will signify that the inequalities hold up to multiplicative constants which are independent of the discretization parameters, but might depend on the physical parameters.
Next, we introduce the functional spaces for our weak formulation. For the bulk pressure and velocity, we introduce the spaces Mb=L2(Ω) and Vb={v∈Hdiv(Ω):[[v]]|Γ∈L2(Γ),{v}|Γ∈[L2(Γ)]d,v⋅n|∂ΩN=0}, and equip the space Vb with the norm ||v||2Vb=||v||20,Ω+||∇⋅v||20,Ω+||[[v]]||20,Γ+||{v}||20,Γ.
Similarly, for the fracture pressure and velocity we define the spaces MΓ=L2(Γ) and VΓ={vΓ∈Hdiv,τ(Γ):vΓ⋅τ|∂Γ=0}. The norm on VΓ is given by ||vΓ||2VΓ=||vΓ||20,Γ+||∇τ⋅vΓ||20,Γ. Finally, we define the global spaces for the pressure and the velocity as M=Mb×MΓ and W=Vb×VΓ, equipped with the canonical norms for product spaces. In order to deal with non-homogeneous boundary conditions, we also introduce the affine spaces Vbg=Lg+Vb and VΓg=LgΓ+VΓ, where Lg∈Hdiv(Ω) and LgΓ∈Hdiv,τ(Γ) are liftings of the boundary data g and gΓ, respectively. We can then define the global space Wg=Vbg×VΓg.
We can now formulate problem (2.6) in weak form as follows: Find (u,uΓ)∈Wg and (p,pΓ)∈M such that
{A((u,uΓ),(v,vΓ))+B((v,vΓ),(p,pΓ))=Fu(v,vΓ)−B((u,uΓ),(q,qΓ))=Fp(q,qΓ) | (3.1) |
where the bilinear form A(⋅,⋅):Wg×Wg→R is defined as A((u,uΓ),(v,vΓ))=a(u,v)+aΓ(uΓ,vΓ) with
a(u,v)=∫Ων−1u⋅v+∫Γ1αΓ[[u]][[v]]+∫Γ1βΓ{u}⋅{v},aΓ(uΓ,vΓ)=∫Γ(ντΓℓΓ)−1uΓ⋅vΓ, |
and the bilinear form B(⋅,⋅):Wg×M→R is defined as B((v,vΓ),(q,qΓ))=b(v,q)+bΓ(vΓ,qΓ)+d(v,qΓ), with
b(v,q)=∫Ω∇⋅vq,bΓ(vΓ,qΓ)=∫Γ∇τ⋅vΓqΓ,d(v,qΓ)=−∫Γ[[v]]qΓ. |
Finally the linear operators Fu(⋅):Wg→R and Fp(⋅):M→R are defined as
Fu(v,vΓ)=∫∂Ωgv⋅n+∫∂ΓgΓvΓ⋅τ,Fp(q,qΓ)=∫Ωfq+∫ΓfΓqΓ. |
Next, we prove that formulation (3.1) is well-posed. For the sake of simplicity, we will assume that homogeneous Dirichlet boundary conditions are imposed for both the bulk and fracture problems, i.e., gD,i=0, i=1;2, and gΓ=0 and that the domain and fracture are smooth enough. The extension to the general non-homogeneous case is straightforward. Note that the existence and uniqueness of the problem can be proven only under the condition that the parameter ξ>1/2.
Theorem 3.1. Suppose that ξ>1/2. Then problem (3.1) admits a unique solution.
Proof. For the proof we follow the technique of [47]. First, we define the subspace of W, ˆW={(v,vΓ)∈W:B((v,vΓ),(q,qΓ))=0∀(q,qΓ)∈M}. To show existence and uniqueness of the solution of (3.1), we only need to show that A(⋅,⋅) is ˆW-elliptic and that B(⋅,⋅) satisfies the inf-sup condition, that is
inf(v,vΓ)∈ˆWA((v,vΓ),(v,vΓ))||(v,vΓ)||2W≳1,inf(q,qΓ)∈Msup(v,vΓ)∈WB((v,vΓ),(q,qΓ))||(q,qΓ)||M||(v,vΓ)||W≳1. |
First, we prove that A(⋅,⋅) is ˆW-elliptic. Since for elements in (v,vΓ)∈ˆW we have ∇⋅v=0 in L2(Ω) and ∇τ⋅vΓ=[[v]]|Γ in L2(Γ), the norm ||(v,vΓ)||W is equivalent to ||v||20,Ω+||vΓ||20,Γ+||[[v]]||20,Γ+||{v}||20,Γ. Owing to the regularity properties of the permeability tensors ν and νΓ, this implies that
A((v,vΓ),(v,vΓ))≳||(v,vΓ)||2W. |
Note that the hidden constant also depends on the parameter αΓ, and that we need to assume αΓ>0, or, equivalently, ξ>1/2, for the inequality to hold true.
To show that B satisfies the inf-sup condition, given (q,qΓ)∈M, we construct, exploiting the adjoint problem, (v,vΓ)∈W such that B((v,vΓ),(q,qΓ))=||(q,qΓ)||2M and ||(v,vΓ)||W≲||(q,qΓ)||M. Given (q,qΓ)∈M, let (ϕ,ϕΓ) be the solution of
{−Δϕ=q,on Ωϕ=0,on ∂Ω and {−ΔτϕΓ=qΓ,on ΓϕΓ=0,on ∂Γ. |
If we set v=(v1,v2) with vi=−∇ϕ|Ωi, i=1,2, and vΓ=−∇τϕΓ, we obtain ∇⋅v=q, ∇τ⋅vΓ=qΓ and [[v]]|Γ=0, since v∈H1(Ω). This implies that (v,vΓ)∈W and B((v,vΓ),(q,qΓ))=||q||20,Ω+||qΓ||20,Γ=||(q,qΓ)||2M. Finally, from elliptic regularity, we have ||(v,vΓ)||2W=||∇ϕ||20,Ω+||∇τϕΓ||20,Γ+||q||20,Ω+||qΓ||20,Γ+||{∇ϕ}||20,Γ≲||q||20,Ω+||qΓ||20,Γ, and this concludes the proof.
In this section we present a family of discrete formulations for the coupled bulk-fracture problem (3.1), which are based on discontinuous Galerkin methods on polytopic grids. In particular, since we can choose to discretize the problem in the bulk and the one in the fracture either in their mixed or in their primal form, we derive four formulations that embrace all the possible combinations of primal-primal, mixed-primal, primal-mixed and mixed-mixed discretizations. The mixed discretizations will be based on the local discontinuous Galerkin method (LDG) [66,67,68], while the primal discretizations on the Symmetric Interior Penalty method (SIPDG) [64,65], all supporting polytopic grids [36,37,40,41]. The derivation of our discrete formulations will be carried out following the same strategy as in [63], so that it will be based on the introduction of the numerical fluxes, which approximate the trace of the solutions on the boundary of each mesh element. In particular, the imposition of the coupling conditions (2.5a)-(2.5b) will be achieved through a proper definition of the numerical fluxes on the faces belonging to the fracture.
First, we introduce the notation related to the discretization of the domains by means of polytopic meshes. For the problem in the bulk, we consider a family of meshes Th made of disjoint open polygonal/polyhedral elements. Following [36,37,40], we introduce the concept of mesh interface, defined as the intersection of the (d−1)-dimensional facets of two neighbouring elements. We need now to distinguish between the case when d=3 and d=2:
● when d=3, each interface consists of a general polygon, which we assume may be decomposed into a set of co-planar triangles. We assume that a sub-triangulation of each interface is provided and we denote the set of all these triangles by Fh. We then use the terminology face to refer to one of the triangular elements in Fh;
● when d=2, each interface simply consists of a line segment, so that the concepts of face and interface are in this case coincident. We still denote by Fh the set of all faces.
Note that Fh is always defined as a set of (d−1)-dimensional simplices (triangles or line segments). As in [36,37,40], no limitation on either the number of faces of each polygon E∈Th or on the relative size of the faces compared to the diameter of the element is imposed.
We consider meshes Th that are aligned with the fracture Γ, so that any element E∈Th cannot be cut by Γ and it belongs exactly to one of the two disjoint subdomains Ω1 or Ω2. This implies that each mesh Th induces a subdivision of the fracture Γ into faces, which we denote by Γh. It follows that we can write
Fh=FIh∪FBh∪Γh, |
where FBh is the set of faces lying on the boundary of the domain ∂Ω and FIh is the set of interior faces not belonging to the fracture. In addition, we write FBh=FDh∪FNh, where FDh and FNh are the boundary faces contained in ∂ΩD and ∂ΩN, respectively (we assume the decomposition to be matching with the partition of ∂Ω into ∂ΩD and ∂ΩN).
The induced discretization of the fracture Γh consists of the faces of the elements of Th that share part of their boundary with the fracture, so that Γh is made up of line segments when d=2 and of triangles when d=3. Note that, in the 3D case, the triangles are not necessarily shape-regular and they may present hanging nodes, due to the fact that the sub-triangulations of each elemental interface is chosen independently from the others. For this reason, we extend the concept of interface also to the (d−2)-dimensional facets of elements in Γh, defined again as intersection of boundaries of two neighbouring elements. When d=2, the interfaces reduce to points, while when d=3 they consists of line segments. We denote by EΓ,h the set of all the interfaces (that we will also call edges) of the elements in Γh, and we write, accordingly to the previous notation, EΓ,h=EIΓ,h∪EBΓ,h, with EBΓ,h=EDΓ,h∪ENΓ,h.
For each element E∈Th, we denote by |E| its measure, by hE its diameter and we set h=maxE∈ThhE. Given an element E∈Th, for any face F⊂∂E, with F∈Fh, we define nF as the unit normal vector on F that points outward of E. We can then define the standard jump and average operators across a face F∈Fh∖FBh for (regular enough) scalar and vector-valued functions similarly to (2.1). We also recall a well-known identity [65] for scalar and vector-valued functions q and v that are piecewise smooth on Th:
∑E∈Th∫∂Eqv⋅nE=∫Fh{v}⋅[[q]]+∫Fh∖FBh[[v]]{q}, | (4.1) |
where we have used the compact notation ∫Fh⋅=∑F∈Fh∫F⋅ and jump and average operators on a boundary face F∈FBh are defined as [[q]]=qnF and {v}=v.
Analogous definitions may be also set up on the fracture. In particular, given an element F∈Γh, with measure |F| and diameter hF, for any edge e⊂∂F, with e∈EΓ,h, we define ne as the unit normal vector on e pointing outward of F (it reduces to ±1 when d=2). Finally, standard jump and average operators across every edge e can be defined for (regular enough) scalar and vector-valued functions and an analogous version of formula (4.1) can be stated for piecewise smooth function on the fracture mesh Γh.
We have now all the ingredients to introduce the discrete formulation of model problem (3.1).
For simplicity in the forthcoming analysis, we will suppose that the permeability tensors ν and νΓ are piecewise constant on mesh elements, i.e., ν|E∈[P0(E)]d×d for all E∈Th, and νΓ|F∈[P0(F)](d−1)×(d−1) for all F∈Γh. First, we introduce the finite-dimensional spaces where we will set our discrete problem. We set
Qbh={q∈L2(Ω):q|E∈PkE(E)∀E∈Th}Wbh={v∈[L2(Ω)]d:v|E∈[PkE(E)]d∀E∈Th}QΓh={qΓ∈L2(Γ):qΓ|F∈PkF(F)∀F∈Γh}WΓh={vΓ∈[L2(Γ)]d−1:vΓ|F∈[PkF(F)]d−1∀F∈Γh}. |
Note that, to each element E∈Th is associated the polynomial degree kE≥1, as well as to each face F∈Γh is associated the degree kF≥1. We remark that the polynomial degrees in the bulk and fracture discrete spaces just defined are chosen independently of each other.
We first focus on the problem in the bulk. Multiplying the first and second equations in (2.2) by test functions v∈Wbh and q∈Qbh, respectively, and integrating by parts over an element E∈Th, we obtain
∫Eν−1u⋅v=−∫Ep∇⋅v+∫∂Epv⋅nE,∫Eu⋅∇q=∫∂Equ⋅nE+∫Efq. |
In the spirit of [63], we start the derivation of our DG discretization from these equations. Adding over the elements E∈Th, the general discrete formulation for the problem in the bulk will then be: Find ph∈Qbh and uh∈Wbh, such that for all E∈Th we have
∑E∈Th∫Eν−1uh⋅v=−∑E∈Th∫Eph∇⋅v+∑E∈Th∫∂EˆpEv⋅nE∑E∈Th∫Euh⋅∇q=∑E∈Th∫∂EqˆuE⋅nE+∑E∈Th∫Efq, |
where the numerical fluxes ˆpE and ˆuE are approximations to the exact solutions u and p, respectively, on the boundary of E. The definition of the numerical fluxes in terms of ph, uh, of the boundary data and of the coupling conditions (2.5a)-(2.5b) will determine the method. Using identity (4.1), we get
∫Thν−1uh⋅v=−∫Thph∇⋅v+∫FIh∪Γh{ˆp}[[v]]+∫FIh∪FBh∪Γh[[ˆp]]⋅{v}, | (4.2) |
∫Thuh⋅∇q−∫FIh∪FBh∪Γh{ˆu}⋅[[q]]−∫FIh∪Γh[[ˆu]]{q}=∫Thfq, | (4.3) |
where we have introduced ˆp=(ˆpE)E∈Th and ˆu=(ˆuE)E∈Th. The numerical fluxes ˆp and ˆu must be interpreted as linear functionals taking values in the spaces ΠE∈ThL2(∂E) and [ΠE∈ThL2(∂E)]d, respectively. In particular, this means that they are, in general, double-valued on FIh∪Γh and single-valued on FBh. We also observe for future use that, after integrating by parts and using again identity (4.1), Eq. (4.2) may also be rewritten as
∫Thν−1uh⋅v=∫Th∇ph⋅v+∫FIh∪Γh{ˆp−ph}[[v]]+∫FIh∪FBh∪Γh[[ˆp−ph]]⋅{v}. | (4.4) |
We now reason analogously for the fracture. Multiplying the first and second equations in (2.4) by test functions vΓ and qΓ, respectively, integrating by parts over an element F∈Γh and summing over all the elements in Γh we obtain the following problem: Find pΓ,h∈QΓh and uΓ,h∈WΓh such that for all F∈Γh we have
∑F∈Γh∫F(ντΓℓΓ)−1uΓ,h⋅vΓ=−∑F∈Γh∫FpΓ,h∇⋅vΓ+∑F∈Γh∫∂FˆpΓ,Fv⋅nF,∑F∈Γh∫FuΓ,h⋅∇qΓ−∑F∈Γh∫∂FqΓˆuΓ,F⋅nF=∑F∈Γh∫FfΓqΓ−∑F∈Γh∫F[[ˆu]]qΓ. |
Here, we have introduced the numerical fluxes ˆpΓ,F and ˆuΓ,F. Again, the idea is that they represent approximations on the boundary of the fracture face F of the exact solutions pΓ and uΓ, respectively. Note also that here ˆu is the numerical flux approximating the bulk velocity on Γh. Using identity (4.1), we get
∫Γh(ντΓℓΓ)−1uΓ,h⋅vΓ=−∑F∈Γh∫FpΓ,h∇⋅vΓ+∫EIΓ,h{ˆpΓ}[[vΓ]]+∫EΓ,h{vΓ}⋅[[ˆpΓ]] | (4.5) |
∫ΓhuΓ,h⋅∇qΓ−∫EIΓ,h{qΓ}[[ˆuΓ]]−∫EΓ,h{ˆuΓ}⋅[[qΓ]]=∫ΓhfΓqΓ−∫Γh[[ˆu]]qΓ | (4.6) |
We point out that, in all previous equations, the gradient and divergence operators are actually tangent operators. Here, we have dropped the subscript τ in order to simplify the notation.
In the following, we explore all possible combinations of primal-primal, mixed-primal, primal mixed and mixed-mixed formulations for the bulk and fracture, respectively.
In order to obtain the primal-primal formulation, we need to eliminate the velocities uh and uΓ,h from equations (4.2)-(4.3) and (4.5)-(4.6). To do so, we need to express uh solely in terms of ph (and pΓ,h), and uΓ,h solely in terms of pΓ,h. As in [63] this will be achieved via the definition of proper lifting operators.
We start by focusing on the problem in the bulk. In order to complete the specification of the DG method that we want to use for the approximation, we need to give an expression to the numerical fluxes. We choose the classic symmetric interior penalty method (SIPDG). Moreover, coupling conditions (2.5a)-(2.5b) are imposed through a suitable definition of the numerical fluxes on the fracture faces. Since we want a primal formulation, the definition of ˆp and ˆu will not contain uh. The numerical fluxes are defined as follows:
ˆp=ˆp(ph)={{ph}onFIhgDonFDhphonFNhphonΓh |
ˆu=ˆu(ph,pΓ,h)={{ν∇ph}−σF[[ph]]onFIhν∇ph−σF(ph−gD)nFonFDh0onFNh−[αΓ({ph}−pΓ,h)nF2+βΓ[[ph]]]onΓh | (4.7) |
Here, we have introduced the discontinuity penalization parameter σ. In particular, σ is a non-negative bounded function, i.e., σ∈L∞(FIh∪FDh) and its precise definition will be given in Definition 5.2 below. Moreover, we have used the notation σF=σ|F, for F∈FIh∪FDh. We remark that, with this choice, the numerical flux ˆp is doubled valued on Γh and single valued on FIh∪FBh.
Using the definition of the numerical fluxes, it follows that
{ˆp−ph}=0,[[ˆu]]=0onFIh,{ˆp−ph}=0,[[ˆu]]=−αΓ({ph}−pΓ,h)onΓh,[[ˆp−ph]]=−[[ph]],{ˆu}={ν∇ph}−σF[[ph]]onFIh,[[ˆp−ph]]=(gD−ph)nF,{ˆu}=ν∇ph−σF(ph−gD)nFonFDh,[[ˆp−ph]]=0,{ˆu}=0onFNh,[[ˆp−ph]]=0,{ˆu}=−βΓ[[ph]]onΓh, |
so we rewrite (4.4) as
∫Thν−1uh⋅v=∫Th∇ph⋅v−∫FIh∪FDh[[ph]]⋅{v}+∫FDhgDv⋅n. | (4.8) |
At this point, we proceed with the elimination of the auxiliary variable uh from our equations. To this aim, we introduce the lifting operator LSIPb:[L1(FIh∪FDh)]d→Wbh defined by
∫ThLSIPb(ξ)⋅v=−∫FIh∪FDh{v}⋅ξ∀v∈Wbh. | (4.9) |
Similarly, we define the lifting Gb(gD)∈Wbh of the Dirichlet boundary datum gD as
∫ThGb⋅v=∫FDhgDv⋅n∀v∈Wbh. | (4.10) |
Thanks to the introduction of the lifting operators, Eq. (4.8) may be rewritten as
∫Th(uh−ν[∇ph+LSIPb([[ph]])+Gb])⋅v=0. |
Since ∇Qbh⊆Wbh, we can write
uh=ν[∇ph+LSIPb([[ph]])+Gb], | (4.11) |
where ∇ph+LSIPb([[ph]])+Gb can be seen as a discrete approximation of the gradient ∇p.
We can then rewrite Eq. (4.3) as
∫Thν[∇ph+LSIPb([[ph]])+Gb]⋅∇q−∫FIh∪FBh∪Γh{ˆu}⋅[[q]]−∫FIh∪Γh[[ˆu]]{q}=∫Thfq. |
Using the definition of the discrete gradient (4.11), of the lifting operators (4.9) and (4.10) and of the numerical flux ˆu (4.7), we have
∫Thν∇ph⋅∇q+∫ThνLSIPb([[ph]])⋅∇q+∫ThνLSIPb([[q]])⋅∇ph+∫FIh∪FDhσF[[ph]]⋅[[q]]+∫ΓhβΓ[[ph]]⋅[[q]]+∫ΓhαΓ({ph}−pΓ,h){q}=∫Thfq+∫FDhgDσFq−∫ThνGb⋅∇q. | (4.12) |
Now we move our attention to the problem in the fracture. We define the numerical fluxes ˆpΓ and ˆuΓ in order to obtain a symmetric interior penalty approximation as follows:
ˆpΓ=ˆpΓ(pΓ,h)={{pΓ,h}onEIΓ,hgΓonEDΓ,hpΓ,honENΓ,h, |
ˆuΓ=ˆuΓ(pΓ,h)={{ντΓℓΓ∇pΓ,h}−σe[[pΓ,h]]onEIΓ,hντΓℓΓ∇pΓ,h−σe(pΓ,h−gΓ)neonEDΓ,h0onENΓ,h. | (4.13) |
Again, we have introduced the discontinuity penalization parameter σΓ∈L∞(EIΓ,h∪EDΓ,h) and we set σe=σΓ|e for e∈EIΓ,h∪EDΓ,h. Its precise definition will be given in Definition 5.3 below. Next, as before, we introduce the lifting operator LSIPΓ:[L1(EIΓ,h∪EDΓ,h)]d−1→WΓh and the lifting of the boundary datum GΓ(gΓ,D)∈WΓh defined by
∫ΓhLSIPΓ(ξΓ)⋅vΓ=−∫EIΓ,h∪EDΓ,hξΓ⋅{vΓ}∀vΓ∈WΓh, | (4.14) |
∫ΓhGΓ⋅vΓ=∫EDΓ,hgΓ,DvΓ⋅nτ∀vΓ∈WΓh. | (4.15) |
Integrating by parts and using (4.1), we can rewrite Eq. (4.5) as
∫Γh(uΓ,h−ντΓℓΓ[∇pΓ,h+LSIPΓ([[pΓ,h]])+GΓ])⋅vΓ=0. |
Again, since ∇QΓh⊆WΓh elementwise, we can write
uΓ,h=ντΓℓΓ[∇pΓ,h+LSIPΓ([[pΓ,h]])+GΓ]. |
Plugging this last identity and the definition of the numerical fluxes ˆu (see (4.7)) and ˆuΓ (see (4.13)) into Eq. (4.6), we obtain
∫ΓhντΓℓΓ∇pΓ,h⋅∇qΓ+∫ΓhντΓℓΓLSIPΓ([[pΓ,h]])⋅∇qΓ+∫ΓhντΓℓΓLSIPΓ([[qΓ]])⋅∇pΓ,h+∫EIΓ,h∪EDΓ,hσe[[pΓ,h]]⋅[[qΓ]]+∫ΓhαΓpΓ,hqΓ−∫ΓhαΓ{ph}qΓ=∫ΓhfΓqΓ+∫EDΓ,hgΓσeqΓ−∫ΓhντΓℓΓGΓ⋅∇qΓ. | (4.16) |
In conclusion, summing Eqs. (4.12) and (4.16) we obtain the following discrete formulation: Find (ph,pΓh)∈Qbh×QΓh such that
APPh((ph,pΓh),(q,qΓ))=LPPh(q,qΓ)∀(q,qΓ)∈Qbh×QΓh, | (4.17) |
where PP stands for primal-primal and where Lh:Qbh×QΓh→R is defined as LPPh(q,qΓ)=LPb(q)+LPΓ(qΓ) and APPh:(Qbh×QΓh)×(Qbh×QΓh)→R is defined as
APPh((ph,pΓh),(q,qΓ))=APb(ph,q)+APΓ(pΓ,h,qΓ)+I((ph,pΓ,h),(q,qΓ)), |
with
APb(ph,q)=∫Thν∇ph⋅∇q+∫ThνLSIPb([[ph]])⋅∇q |
+∫ThνLSIPb([[q]])⋅∇ph+∫FIh∪FDhσF[[ph]]⋅[[q]], | (4.18) |
APΓ(pΓ,h,qΓ)=∫ΓhντΓℓΓ∇pΓ,h⋅∇qΓ+∫ΓhντΓℓΓLPΓ([[pΓ,h]])⋅∇qΓ |
+∫ΓhντΓℓΓLSIPΓ([[qΓ]])⋅∇pΓ,h+∫EIΓ,h∪EDΓ,hσe[[pΓ,h]]⋅[[qΓ]], | (4.19) |
I((ph,pΓ,h),(q,qΓ))=∫ΓhβΓ[[ph]]⋅[[q]]+∫ΓhαΓ({ph}−pΓ,h)({q}−qΓ,h), | (4.20) |
and
LPb(q)=∫Thfq+∫FDhgDσFq−∫ThνGb⋅∇q, | (4.21) |
LPΓ(qΓ)=∫ΓhfΓqΓ+∫EDΓ,hgΓσeqΓ−∫ΓhντΓℓΓGΓ⋅∇qΓ. | (4.22) |
We remark that we have recovered the formulation already obtained in [59] (in its not strongly consistent version), the only difference being that the bilinear form for the problem in the fracture is in the shape of SIPDG method, instead of classical conforming finite elements.
In this section, we discretize the problem in the bulk in its mixed form. To this aim, we use the local discontinuous Galerkin (LDG) method [66,67,68,69]. The LDG method is a particular DG method that can be included in the class of mixed finite element methods. However, the variable uh can be locally solved in terms of ph and then eliminated from the equations, giving rise to a primal formulation with ph as only unknown.
In what follows, we first derive the formulation of our method in a mixed setting. After that, we recast it in a primal setting, in order to perform the analysis in the framework of [63,69]. However, we remark that the mixed formulation is the one that will actually be implemented for the numerical experiments of Section 7. As far as the problem in the fracture is concerned, we work again in a primal setting, using the SIPDG method for the discretization.
In the bulk, we define the numerical fluxes as
ˆp=ˆp(ph)={{ph}+b⋅[[ph]]onFIhgDonFDhphonFNhphonΓh |
ˆu=ˆu(uh,ph,pΓ,h)={{uh}−b[[uh]]−σF[[ph]]onFIhuh−σF(phnF−gDnF)onFDh0onFNh−[αΓ({ph}−pΓ,h)nF2+βΓ[[ph]]]onΓh |
Here, b∈[L∞(FIh)]d is a (possibly null) vector-valued function which is constant on each face. It is chosen such that
||b||∞,FIh≤B, | (4.23) |
with B≥0 independent if the discretization parameters. Moreover, σ is the penalization parameter introduced in (4.7), whose precise definition will be given in (5.2) below. Note that the numerical flux ˆp does not depend on uh. This will allow for an element-by-element elimination of the variable uh, generating a primal formulation of the problem. We also point out that the definition of the numerical fluxes on the fracture faces is the same as in the primal SIPDG setting.
With this definition of the numerical fluxes, and after integration by parts as in (4.4), Eq. (4.2) becomes
∫Thν−1uh⋅v−∫Th∇ph⋅v+∫FIh[[ph]]⋅({v}−b[[v]])+∫FDhphv⋅nF=∫FDhgDv⋅nF, | (4.24) |
while Eq. (4.3) turns into
∫Thuh⋅∇q−∫FIh({uh}−b[[uh]])⋅[[q]]+∫FIh∪FDhσF[[ph]]⋅[[q]]−∫FDhquh⋅nF+∫ΓhβΓ[[ph]]⋅[[q]]+∫ΓhαΓ({ph}−pΓ,h){q}=∫Thfq+∫FDhσFgDq. | (4.25) |
If we discretize the problem in the fracture with the SIPDG method, we obtain the following discrete mixed problem: Find ((ph,uh),pΓh)∈Qbh×Wbh×QΓh such that
Mb(uh,v)+Bb(ph,v)=Fb(v)∀v∈Wbh, |
−Bb(q,uh)+Sb(ph,q)+I1(ph,q,pΓ,h)=Gb(q)∀q∈Qbh, | (4.26) |
APΓ(pΓ,h,qΓ)+I2(ph,pΓ,h,qΓ)=LPΓ(qΓ)∀qΓ∈QΓh, |
where
Mb(uh,v)=∫Thν−1uh⋅v, | (4.27) |
Bb(ph,v)=−∫Th∇ph⋅v+∫FIh[[ph]]⋅({v}−b[[v]])+∫FDhphv⋅nF, |
Sb(ph,q)=∫FIh∪FDhσF[[ph]]⋅[[q]], |
I1(ph,q,pΓ,h)=∫ΓhβΓ[[ph]]⋅[[q]]+∫ΓhαΓ({ph}−pΓ,h){q}, |
I2(ph,pΓ,h,qΓ)=∫ΓhαΓ(pΓ,h−{ph})qΓ, |
Fb(v)=∫FDhgDv⋅nF, |
Gb(q)=∫Thfq+∫FDhσFgDq, |
and APΓ(⋅,⋅) and LPΓ(⋅) are defined as in (4.19) and (4.22), respectively. Also note that we have I((ph,pΓ,h),(q,qΓ))=I1(ph,q,pΓ,h)+I2(ph,pΓ,h,qΓ).
We now focus on rewriting the problem in the bulk in a primal form, taking advantage of the local solvability of LDG method. We proceed as in the SIPDG case and introduce an appropriate lifting operator, LLDGb:[L1(FIh∪FDh)]d→Wbh, defined by
∫ThLLDGb(ξ)⋅v=−∫FIh({v}−b[[v]])⋅ξ−∫FDhξ⋅v∀v∈Wbh | (4.28) |
From Eq. (4.24) we obtain
uh=ν(∇ph+LLDGb([[ph]])+Gb), | (4.29) |
where Gb is the lifting of the Dirichlet boundary datum defined in (4.10). Eq. (4.25) now becomes
∫Thν∇ph⋅∇q+∫ThνLLDGb([[ph]])⋅∇qh+∫ThνGb⋅∇q−∫FIh({uh}+b[[uh]])⋅[[q]]−∫FDhquh⋅nF+∫FIh∪FDhσF[[ph]]⋅[[q]]+∫ΓhβΓ[[ph]]⋅[[q]]+∫ΓhαΓ({ph}−pΓ,h){q}=∫Thfq+∫FDhσFqgD. |
Using again the definition of the lifting LLDGb and the identity (4.29), we obtain
∫Thν(∇ph+LLDGb([[ph]]))⋅(∇q+LLDGb([[q]]))+∫FIh∪FDhσF[[ph]]⋅[[q]]+∫ΓhβΓ[[ph]]⋅[[q]]+∫ΓhαΓ({ph}−pΓ){q}=∫Thfq+∫FDhσFqgD−∫ThνGb⋅(∇q+LLDGb([[q]])). | (4.30) |
Summing Eqs. (4.30) and (4.16) we obtain the following discrete formulation: Find (ph,pΓh)∈Qbh×QΓh such that
AMPh((ph,pΓh),(q,qΓ))=LMPh(q,qΓ)∀(q,qΓ)∈Qbh×QΓh, | (4.31) |
where MP stands for mixed-primal and where AMPh:(Qbh×QΓh)×(Qbh×QΓh)→R is defined as
AMPh((ph,pΓh),(q,qΓ))=AMb(ph,q)+APΓ(pΓ,h,qΓ)+I((ph,pΓ,h),(q,qΓ)), |
and LMPh:Qbh×QΓh→R is defined as
LMPh(q,qΓ)=LMb(q)+LPΓ(qΓ) |
with
AMb(ph,q)=∫Thν(∇ph+LLDGb([[ph]]))⋅(∇q+LLDGb([[q]]))+∫FIh∪FDhσF[[ph]]⋅[[q]] |
+∫ΓhβΓ[[ph]]⋅[[q]]+∫ΓhαΓ({ph}−pΓ){q}, | (4.32) |
LMb(q)=∫Thfq+∫FDhσFqgD−∫ThνGb⋅(∇q+LLDGb([[q]])). |
Note that the mixed formulation (4.26) is equivalent to the primal formulation (4.31) together with the definition of the lifting operator (4.28) and Eq. (4.29).
We now want to approximate the problem in the fracture in mixed form, employing the LDG method and the problem in the bulk using the SIPDG method. We define the numerical fluxes as follows
ˆpΓ=ˆpΓ(pΓ,h)={{pΓ,h}+bΓ⋅[[pΓ,h]]onEIΓ,hgΓonEDΓ,hpΓ,honENΓ,h |
ˆuΓ=ˆuΓ(uΓ,h,pΓ,h)={{uΓ,h}−bΓ[[uΓ,h]]−σe[[pΓ,h]]onEIΓ,huΓ,h−σe(pΓ,hne−gΓne)onEDΓ,h0onENΓ,h |
Here, bΓ∈[L∞(EIΓ,h)]d−1 is a vector-valued function that is constant on each edge and it is chosen such that ||bΓ||∞,EIΓ,h≤BΓ, with BΓ≤0 independent of the discretization parameters. Eqs. (4.5) and (4.6) now become
∫Γh(ντΓℓΓ)−1uΓ,h⋅vΓ−∫ΓhvΓ⋅∇pΓ,h+∫EIΓ,h[[pΓ,h]]⋅({vΓ}−bΓ[[vΓ]])+∫EDΓ,hpΓ,hvΓ⋅ne=∫EDΓ,hgΓvΓ⋅ne | (4.33) |
∫ΓhuΓ,h⋅∇qΓ−∫EIΓ,h[[qΓ]]⋅({uΓ,h}−bΓ[[uΓ,h]])+∫EΓ,hσe[[pΓ,h]]⋅[[qΓ]]−∫EDΓ,hqΓuΓ,h⋅ne=∫ΓhfΓqΓ+∫ΓhαΓ({ph−pΓ,h})qΓ+∫EΓ,hσegΓqΓ, | (4.34) |
where we have also used the definition of the numerical flux ˆu on Γh (see (4.7)) to rewrite −[[ˆu]]=αΓ({ph}−pΓ,h). For the bulk we proceed as in the primal-primal section using for the discretization the SIPDG method. We then obtain the following primal-mixed problem: Find (ph,(pΓh,uΓ,h))∈Qbh×QΓh×WΓh such that
3APb(ph,q)+I1((ph,q),pΓ,h)=LPb(q)∀q∈Qbh |
MΓ(uΓ,h,vΓ)+BΓ(pΓ,h,vΓ)=FΓ(vΓ)∀vΓ∈WΓh, | (4.35) |
−BΓ(qΓ,uΓ,h)+SΓ(pΓ,h,qΓ)+I2(ph,(pΓ,h,qΓ))=GΓ(qΓ)∀qΓ∈QΓh, |
where
MΓ(uΓ,h,vΓ)=∫Γh(ντΓℓΓ)−1uΓ,h⋅vΓ,BΓ(pΓ,h,vΓ)=−∫ΓhvΓ⋅∇pΓ,h+∫EIΓ,h[[pΓ,h]]⋅({vΓ}−bΓ[[vΓ]])+∫EDΓ,hpΓ,hvΓ⋅ne,Sb(pΓ,h,qΓ)=∫EΓ,hσe[[pΓ,h]]⋅[[qΓ]],FΓ(vΓ)=∫EDΓ,hgΓvΓ⋅ne,GΓ(qΓ)=∫ΓhfΓqΓ+∫EΓ,hσegΓqΓ, |
and APb(ph,q) and LPb(q) are defined as in (4.18) and (4.21), respectively.
Aiming at rewriting the problem in the fracture in primal form, we introduce the lifting operator, LLDGΓ:[L1(EIh∪EDh)]d→WΓh, defined by
∫ΓhLLDGΓ(ξΓ)⋅vΓ=−∫EIΓ,h({vΓ}−bΓ[[vΓ]])⋅ξΓ−∫EDΓ,hξΓ⋅vΓ∀vΓ∈WΓh | (4.36) |
From Eq. (4.33) we obtain
uΓ,h=ντΓℓΓ[∇pΓ,h+LLDGΓ([[pΓ,h]])+GΓ] | (4.37) |
where GΓ is the lifting of the Dirichlet boundary datum defined in (4.15). Eq. (4.34) now becomes
∫ΓhντΓℓΓ(∇pΓ,h+LLDGΓ([[pΓ,h]]))⋅(∇qΓ+LLDGΓ([[qΓ]]))+∫EIΓ,h∪EDΓ,hσe[[pΓ,h]]⋅[[qΓ]]+∫ΓhαΓ(pΓ,h)−{ph})=∫ΓhfΓqΓ+∫EDΓ,hσeqΓgΓ−∫ΓhντΓℓΓGΓ⋅(∇qΓ+LLDGΓ([[qΓ]])). |
We obtain the following primal formulation: Find (ph,pΓh)∈Qbh×QΓh such that
APMh((ph,pΓh),(q,qΓ))=LPMh(q,qΓ)∀(q,qΓ)∈Qbh×QΓh, | (4.38) |
where PM stands for primal-mixed and where APMh:(Qbh×QΓh)×(Qbh×QΓh)→R is defined as
APMh((ph,pΓh),(q,qΓ))=APb(ph,q)+AMΓ(pΓ,h,qΓ)+I((ph,pΓ,h),(q,qΓ)), |
and LPMh:Qbh×QΓh→R is defined as LPMh(q,qΓ)=LPb(q)+LMΓ(qΓ) with
AMΓ(pΓ,h,qΓ)=∫ΓhντΓℓΓ(∇pΓ,h+LLDGΓ([[pΓ,h]]))⋅(∇qΓ+LLDGΓ([[qΓ]])) |
+∫EIΓ,h∪EDΓ,hσe[[pΓ,h]]⋅[[qΓ]], | (4.39) |
LMΓ(qΓ)=∫ΓhfΓqΓ+∫EDΓ,hσeqΓgΓ−∫ΓhντΓℓΓGΓ⋅(∇qΓ+LLDGΓ([[qΓ]])). |
Finally, if we approximate both the problem in the bulk and in the fracture with the LDG method, we obtain the following formulation: Find (ph,pΓ,h)∈Qbh×QΓh and (uh,uΓ,h)∈Wbh×WΓh such that
3Mb(uh,v)+Bb(ph,v)=Fb(v)∀v∈Wbh,−Bb(q,uh)+Sb(ph,q)+I1(ph,q,pΓ,h)=Gb(q)∀q∈Qbh,MΓ(uΓ,h,vΓ)+BΓ(pΓ,h,vΓ)=FΓ(vΓ)∀vΓ∈WΓh,−BΓ(qΓ,uΓ,h)+SΓ(pΓ,h,qΓ)+I2(ph,(pΓ,h,qΓ))=GΓ(qΓ)∀qΓ∈QΓh, | (4.40) |
This formulation, together with the definition of the lifting operators (4.28) and (4.36) and of the discrete gradients (4.29) and (4.37) is equivalent to the following: Find (ph,pΓ,h)∈Qbh×QΓh such that
AMMh((ph,pΓh),(q,qΓ))=LMMh(q,qΓ)∀(q,qΓ)∈Qbh×QΓh, | (4.41) |
where MM stands for mixed-mixed and where AMMh:(Qbh×QΓh)×(Qbh×QΓh)→R is defined as
AMMh((ph,pΓh),(q,qΓ))=AMb(ph,q)+AMΓ(pΓ,h,qΓ)+I((ph,pΓ,h),(q,qΓ)), |
and LMMh:Qbh×QΓh→R is defined as LMMh(q,qΓ)=LMb(q)+LMΓ(qΓ).
Next, we perform a unified analysis of all of the derived DG discretizations for the fully-coupled bulk-fracture problem. We remark that the analysis will be performed considering the mixed LDG discretizations recast in their primal form, following [69]. For clarity, in Table 1 we summarize the bilinear forms for all the four approaches.
Method | Primal bilinear form |
Primal-Primal (PP) | APb(p,q)+APΓ(pΓ,qΓ)+I((p,q),(pΓ,qΓ)) |
Mixed-Primal (MP) | AMb(p,q)+APΓ(pΓ,qΓ)+I((p,q),(pΓ,qΓ)) |
Primal-Mixed (PM) | APb(p,q)+AMΓ(pΓ,qΓ)+I((p,q),(pΓ,qΓ)) |
Mixed-Mixed (MM) | AMb(p,q)+AMΓ(pΓ,qΓ)+I((p,q),(pΓ,qΓ)) |
The bulk, fracture and interface bilinear forms are defined in:
APb(p,q):(4.18) APΓ(pΓ,qΓ):(4.19) I((p,q),(pΓ,qΓ)):(4.20)AMΓ(pΓ,qΓ):(4.32) AMb(p,q):(4.39) |
In this section, we address the problem of stability. We prove that the primal-primal (PP) (4.17), mixed-primal (MP) (4.31), primal-mixed (PM) (4.38) and mixed-mixed (MM)(4.41) formulations are well-posed. We remark that all these formulations are not strongly consistent, due to the presence of the lifting operators. This implies that the analysis will be based on Strang's second Lemma, [70].
We recall that, for simplicity in the analysis, we are assuming the permeability tensors ν and ντΓ to be piecewise constant. We will employ the following notation ˉνE=|√ν|E|22 and ˉντF=|√ντΓ|F|22, where |⋅|2 denotes the l2-norm.
To consider the boundedness and stability of our primal bilinear forms, we introduce the spaces Qb(h)=Qbh+˜Qb and QΓ(h)=QΓh+˜QΓ where ˜Qb={q=(q1,q2)∈H1(Ω1)×H1(Ω2)}∩H2(Th) and ˜QΓ=H1(Γ)∩H2(Γh). We remark that all the bilinear forms A∗∗h(⋅,⋅) are also well-defined on the extended space Qb(h)×QΓ(h).
Further, we introduce the following energy norm on the discrete space Qbh×QΓh
|||(q,qΓ)|||2=||q||2DG+||qΓ||2DG+||(q,qΓ)||2I |
where
||q||2DG=||ν1/2∇q||20,Th+||σ1/2F[[q]]||20,FIh∪FDh||qΓ||2DG=||(ντΓℓΓ)1/2∇qΓ||20,Γh+||σ1/2e[[qΓ]]||20,EIΓ,h∪EDΓ,h||(q,qΓ)||2I=||β1/2Γ[[q]]||20,Γh+||α1/2Γ({q}−qΓ)||20,Γh |
Note that |||⋅||| is also well defined on the extended space Qb(h)×QΓ(h).
Since our discretization employ general polytopic grids, we need introduce some technical instruments to work in this framework [36,37,38,40,41]. In particular, we need trace inverse estimates to bound the norm of a polynomial on a polytope's face/edge by the norm on the element itself. To this aim, we give the following
Definition 5.1. A mesh Th is said to be polytopic-regular if, for any E∈Th, there exists a set of non-overlapping (not necessarily shape-regular) d-dimensional simplices {SiE}nEi=1 contained in E, such that ˉF=∂ˉE∩¯SiE, for any face F⊆∂E, and
hE≲d|SiE||F|,i=1,…,nE, |
with the hidden constant independent of the discretization parameters, the number of faces of the element nE, and the face measure.
We remark that this definition does not give any restriction on the number of faces per element, nor on their measure relative to the diameter of the element the face belongs to.
Assumption 5.1. We assume that Th and Γh are polytopic-regular meshes.
With this hypothesis, we can state the following inverse-trace estimate that is valid for polytopic elements [38,41].
Lemma 5.2. Let E be a polygon/polyhedron belonging to a mesh satisfying Definition 5.1 and let v∈PkE(E). Then, we have
||v||2L2(∂E)≲k2EhE||v||2L2(E), | (5.1) |
where the hidden constant depends on the dimension d, but it is independent of the discretization parameters, of the number of faces of the element and of the relative size of the face compared to the diameter kE of E.
The second fundamental tool to deal with polytopic discretizations, is an appropriate definition of the discontinuity penalization parameter, which allows for the use of elements with arbitrarily small faces. Taking as a reference [36,37,38,40,41], we give the following two definitions for the bulk and fracture penalty functions:
Definition 5.2. The discontinuity-penalization parameter σ:Fh∖Γh→R+ for the bulk problem is defined facewise as
σ(x)=σ0{maxE∈{E+,E−}ˉνEk2EhEif x⊂F∈FIh,ˉF=∂ˉE+∩∂ˉE−,ˉνEk2EhEif x⊂F∈FDh,ˉF=∂ˉE∩∂ˉΩ, | (5.2) |
with σ0>0 independent of kE, |E| and |F|.
Definition 5.3. The discontinuity-penalization parameter σΓ:EΓ,h→R+ for the fracture problem is defined edgewise as
σΓ(x)=σ0,Γ{maxF∈{F+,F−}ˉντFk2FhFif x⊂e∈EIΓ,h,ˉe=∂ˉF+∩∂ˉF−,ˉντFk2FhF,if x⊂e∈EDΓ,h,ˉe=∂ˉF∩∂ˉΓ, | (5.3) |
with σ0,Γ>0 independent of kF, |F| and |e|.
Now we have all the technical tools to work in a polytopic framework. Next, we will state and prove some estimates that will be instrumental for the proof of the well-posedness of our discrete formulations. We start deriving some bounds on the lifting operators, with arguments similar to those of [68,69,71]. Note that all the results hold true on the extended spaces Qb(h) and QΓ(h).
Lemma 5.3. Let LSIPb(⋅) be the lifting operator defined in (4.9). Then, for every q∈Qb(h) it holds
||ν1/2LSIPb([[q]])||0,Ω≲1σ1/20||σ1/2F[[q]]||0,FIh∪FDh. | (5.4) |
Proof. Denoting by ΠWbh the L2-projection onto Wbh, by definition of the lifting operator LSIPb and Cauchy-Schwarz inequality, we have
||ν1/2LSIPb([[q]])||0,Ω=supz∈[L2(Ω)]d∫Ων1/2LSIPb([[q]])⋅z||z||0,Ω=supz∈[L2(Ω)]d∫ΩLSIPb([[q]])⋅ΠWbh(ν1/2z)||z||0,Ω=−supz∈[L2(Ω)]d∫FIh∪FDhσ1/2F[[q]]⋅σ−1/2F{ΠWbh(ν1/2z)}||z||0,Ω≤supz∈[L2(Ω)]d||σ1/2F[[q]]||0,FIh∪FDh||σ−1/2F{ΠWbh(ν1/2z)}||0,FIh∪FDh||z||0,Ω. |
Using the triangular inequality, the definition of the penalization coefficient σF (5.2), the inverse inequality (5.1), the assumptions on the permeability tensor ν and the continuity property of the L2-projector we have
||σ−1/2F{ΠWbh(ν1/2z)}||20,FIh∪FDh≲∑E∈Th1σ0hEˉνEk2E||ΠWbh(ν1/2z)||20,∂E≲∑E∈Th1ˉνE1σ0||ΠWbh(ν1/2z)||20,E | (5.5) |
≲1σ0∑E∈Th||z||20,E=1σ0||z||20,Ω. |
This proves the desired estimate.
Lemma 5.4. Let LSIPΓ(⋅) be the lifting operator defined in (4.14). Then, for every qΓ∈QΓ(h) it holds
||(ντΓℓΓ)1/2LSIPΓ([[qΓ]])||0,Γ≲1σ1/20,Γ||σ1/2e[[qΓ]]||0,EIΓ,h∪EDΓ,h. |
Proof. Same arguments as in in the proof of Lemma 5.3.
Lemma 5.5. Let LLDGb(⋅) be the lifting operator defined in (4.28). Then, for every q∈Qb(h) it holds
||ν1/2LLDGb([[q]])||0,Ω≲1+Bσ1/20||σ1/2F[[q]]||0,FIh∪FDh. | (5.6) |
Proof. We proceed as in the proof of Lemma 5.3. By definition of the lifting operator LLDGb and Cauchy-Schwarz inequality, we have
||ν1/2LLDGb([[q]])||0,Ω=supz∈[L2(Ω)]d∫Ων1/2LLDGb([[q]])⋅z||z||0,Ω=supz∈[L2(Ω)]d∫ΩLLDGb([[q]])⋅ΠWbh(ν1/2z)||z||0,Ω≤supz∈[L2(Ω)]d|−∫FIhσ1/2F[[q]]⋅σ−1/2F({ΠWbh(ν1/2z)}−b[[ΠWbh(ν1/2z)]])|||z||0,Ω+supz∈[L2(Ω)]d|−∫FDhσ1/2F[[q]]⋅σ−1/2FΠWbh(ν1/2z)|||z||0,Ω≤supz∈[L2(Ω)]d||σ1/2F[[q]]||0,FIh∪FDh||σ−1/2F{ΠWbh(ν1/2z)}||0,FIh∪FDh||z||0,Ω+supz∈[L2(Ω)]d||σ1/2F[[q]]||0,FIh||σ−1/2Fb[[ΠWbh(ν1/2z)]]||0,FIh||z||0,Ω=(a)+(b) |
From (5.5) we know that (a)≲1σ1/20||σ1/2F[[q]]||0,FIh∪FDh, while using similar arguments and bound (4.23) on b, we can prove that
||σ−1/2Fb[[ΠWbh(ν1/2z)]]||20,FIh≲B2σ0||z||20,Ω, |
so that (b)≲Bσ1/20||σ1/2F[[q]]||0,FIh∪FDh. This concludes the proof.
Lemma 5.6. Let LLDGΓ(⋅) be the lifting operator defined in (4.36). Then, For every qΓ∈QΓ(h) it holds
||(ντΓℓΓ)1/2LLDGΓ([[qΓ]])||0,Γ≲1+BΓσ1/20,Γ||σ1/2e[[qΓ]]||0,EIΓ,h∪EDΓ,h. |
Proof. Same arguments as in in the proof of Lemma 5.5.
Using these results, we can now prove that the bilinear forms for the bulk problem APb(⋅,⋅) and AMb(⋅,⋅) are continuous on Qb(h) and coercive on Qbh, as well as the fracture bilinear forms APΓ(⋅,⋅) and AMΓ(⋅,⋅) are continuous on QΓ(h) and coercive on QΓh.
Lemma 5.7. APb(⋅,⋅) is coercive on Qbh×Qbh and continuous on Qb(h)×Qb(h), that is
APb(q,q)≳||q||2DG∀q∈Qbh, |
APb(p,q)≲||p||DG||q||DG∀p,q∈Qb(h), |
provided that σ0 is chosen big enough.
Proof. We start with coercivity. Taking p=q∈Qbh, we have
APb(q,q)=∑E∈Th[||ν1/2∇q||20,E+2∫EνLSIPb([[q]])⋅∇q]+∑F∈FIh∪FDh||σ1/2F[[q]]||20,F |
From Young inequality we have
2∫EνLSIPb([[q]])⋅∇q≥−2||ν1/2LSIPb([[q]])||0,E||ν1/2∇q||0,E≥−ε||ν1/2LSIPb([[q]])||20,E−1ε||ν1/2∇q||20,E, |
so that, using the bound on the lifting (5.4), we obtain
APb(q,q)≥∑E∈Th[(1−ε)‖ |
for big enough.
Continuity directly follows from Cauchy Schwarz inequality and the bound on the lifting (5.4).
Lemma 5.8. is coercive on and continuous on , that is
provided that is chosen big enough.
Proof. Analogous to the proof of Lemma 5.7.
Lemma 5.9. is coercive on and continuous on , that is
Proof. We start with coercivity. From Young's inequality and the bound on the lifting (5.10) we have, for every ,
with , so that is coercive for every choice of the parameters and *. Continuity is again a direct consequence of Cauchy Schwarz's inequality and the bound on the lifting (5.6).
* More in detail: we need , with . We obtain , that is , being for every possible choice of and .
Lemma 5.10. is coercive on and continuous on , that is
Proof. Analogous to the proof of Lemma 5.9.
Employing Lemmas 5.7, 5.9, 5.8 and 5.10, we can easily prove the well-posedness of all of our discrete problems, as stated in the following stability result.
Proposition 5.11. Let the penalization parameters for the problem in the bulk and in the fracture be defined as in (5.2) and (5.3), respectively. Then, the fully-coupled discrete problems PP (4.22), MP (4.31), PM (4.38) and MM (4.41) are well-posed provided that and are chosen big enough for the primal formulations.
Proof. In order to use Lax-Milgram Lemma, we prove that the bilinear forms , , and are continuous on and coercive on . We have, from Cauchy-Schwarz inequality
so that coercivity and continuity are a direct consequence of the definition of the norm and of Lemmas 5.7, 5.9, 5.8 and 5.10. The continuity of the linear operators , , and on can be easily proved by using Cauchy-Schwarz's inequality, thanks to the regularity assumptions on the forcing terms and and on the boundary data and .
In this section we derive error estimates for our discrete problems.
The tool at the base of DG-method error analysis are -interpolation estimates. Here, we summarize the results contained in [36,37,38,40,41], where standard estimates on simplices are extended to arbitrary polytopic elements.
First, we give the following definitions.
Definition 6.1. A covering related to the polytopic mesh is a set of shape-regular -dimensional simplices , such that for each , there exists a such that .
Assumption 6.1. [36,37,38,40,41] There exists a covering of (see Definition 6.1) and a positive constant , independent of the mesh parameters, such that
and for each pair and , with .
Moreover, there exists a covering of and a positive constant , independent of the mesh parameters, such that
and for each pair and , with .
We can now state the following approximation result:
Lemma 6.2. [36,37,38,40,41] Let and denote the corresponding simplex such that (see Definition 6.1). Suppose that is such that , for some . Then, if Assumption 5.1 and 6.1 are satisfied, there exists , such that , and the following bound holds
(6.1) |
Moreover, if ,
(6.2) |
Here, and the hidden constants depend on the shape-regularity of , but are independent of , , and the number of faces per element and is the continuous extension operator as defined in [72].
Proof. See [36] for a detailed proof of (6.1) and [38] for the proof of (6.2). Clearly, analogous approximation results can be stated on the fracture spaces, since Assumptions 5.1 and 6.1 are both satisfied.
For each subdomain , , we denote by the classical continuous extension operator (cf. [72], see also [59]) , for . Similarly, we denote by the continuous extension operator , for . We then make the following regularity assumptions for the exact solution of problem (3.1):
Assumption 6.3. Let and denote the associated coverings of and , respectively, of Definition 6.1. We assume that the exact solution is such that:
A1. for every , if , it holds , with and with ;
A2. for every , it holds , with and with .
Assumption 6.4. We assume that the normal components of the exact fluxes and are continuous across mesh interfaces, that is on and on .
From Proposition 5.11 and Strang's second Lemma the following abstract error bound directly follows.
Lemma 6.5. Assuming that the hypotheses of Proposition 5.11 are satisfied, for all the discrete problems PP (4.17), MP (4.31), MM (4.41) and PM (4.41) the following abstract error bound holds
where the residual is defined as
with .
Note that, irrespective of the numerical method chosen for the discretization (PP, MP, PM or MM), the residual can always be split into two contributions, one deriving from the approximation of the problem in the bulk and one deriving from the approximation of the problem in the fracture, i.e.,
(6.3) |
It follows that, to derive a bound for the global residual, we can bound each of the two contributions separately. With this in mind, we state and prove the next two lemmas.
Lemma 6.6. Let be the exact solution of problem (3.1) satisfying the regularity Assumptions 6.4 and 6.3. Then, for every and , it holds
(6.4) |
(6.5) |
Proof. First, we prove (6.4). Let be the -orthogonal projector onto , then, integrating by parts elementwise, using the fact that satisfies (2.2) and recalling that, from Assumption 6.4, vanishes on , we obtain the following expression for the residual :
Employing the Cauchy-Schwarz's inequality and the definition of the norm , we then obtain
If we still denote by the vector-valued generalization of the projection operator defined in Lemma 6.2, we observe that
To bound the term (1), we employ the approximation result stated in Lemma 6.2. We obtain
Exploiting, the boundedness of the permeability tensor , the inverse inequality (5.1), the -stability of the projector and the approximation results stated in Lemma 6.2, we can bound term (2) as follows:
which concludes the proof of (6.4).
Proceeding as above we obtain the following expression for the residual :
Estimate (6.5) can then be proven with analogous arguments.
Lemma 6.7. Let be the exact solution of problem (3.1) satisfying the regularity Assumptions 6.4 and 6.3. Then, for every and , it holds
(6.6) |
(6.7) |
Proof. We focus on the proof of (6.6), estimate (6.7) can be obtained likewise. Recalling that denotes the -orthogonal projector onto , the residual has the following expression:
where we have used the identity and the continuity of across internal faces (Assumption 6.4). From Cauchy-Schwarz and triangular inequalities and the bound on the coefficient (4.23), we have
where we recall that, with a slight abuse of notation, still denotes the vector-valued generalization of the projection operator defined in Lemma 6.2. The thesis now follows from the boundedness of the permeability tensor , the inverse inequality (5.1), the -stability of the projector and the approximation results stated in Lemma 6.2.
Theorem 6.8. Let and denote the associated coverings of and , respectively, consisting of shape-regular simplexes as in Definition 4, satisfying Assumptions 6.1. Let be the solution of problem (3.1) and be its approximation obtained with the method PP, MP, MM or PM, with the penalization parameters given by (5.2) and (5.3) and and sufficiently large for the primal formulations. Moreover, suppose that the exact solution satisfies the regularity Assumptions 6.4 and 6.3. Then, the following error bound holds:
where the is to be interpreted as when or as when . Here, and , and the constants are defined according to the chosen approximation method as follows:
Proof. From Lemma 6.5 we know that the error satisfies the following bound
(6.8) |
We estimate the two terms on the right-hand side of (6.8) separately. We can rewrite term as
Again we consider each of the three terms separately. To bound term (), we exploit the two approximation results stated in Lemma 6.2 and obtain
Using analogous interpolation estimates on the fracture we can bound term () as follows:
Finally, for term (), we have
Exploiting the approximation result (6.2), we obtain
Similarly, we have
Finally, using the interpolation estimates for the fracture, we deduce that
In conclusion, combining all the previous estimates, we can bound the term on the right-hand side of (6.8) as follows:
(6.9) |
Finally, the desired estimates follow from the combination of (6.9), together with the bound on Term deriving from what observed in (6.3) and Lemmas 6.6 and 6.7.
Finally, from the above result we can derive some error estimates also for the velocities and .
Theorem 6.9. Let all the hypotheses of Theorem 6.8 hold. Let and be the solution of problem (3.1). Then:
● if is its approximation obtained with the MP method (4.26), it holds
● if is its approximation obtained with the PM method (4.35), it holds
● if is its approximation obtained with the MM method (4.40), it holds
where the constants , , and are defined as in Theorem 6.8.
Proof. Let and be the discrete solutions obtained with the MP method and with the MM method, respectively. Then, using identity (4.29) and the fact that , we can rewrite
From the uniform boundedness of , the triangular inequality, the bound on the lifting (5.6) and the definition of the norm it follows that
In particular, this implies that . Similarly, one can prove that, if and are the discrete solutions obtained with the PM method and with the MM method, respectively, it holds The thesis is now a direct consequence of Theorem 6.8.
In this section we present some two-dimensional numerical experiments with the aim of validating the obtained theoretical convergence results and of comparing the accuracy of the proposed formulations. In particular, we will focus on the paradigmatic primal-primal and mixed-primal settings. This means that, for the approximation of the problem in the bulk, we will employ the SIPDG method in the first setting and the LDG method in the second setting, while, for the problem in the fracture, we will employ the SIPDG method in both settings. All the numerical tests have been implemented in . For the generation of polygonal meshes conforming to the fractures we have suitably modified the code [73].
In particular, we present three sets of numerical experiments. The first set (Sections 7.1 and 7.2) is obtained assuming that analytical solutions are known and aims at verifying the a-priori error estimates obtained in Theorems 6.8 and 6.9. The second set (Section 7.3) is derived from physical considerations and aims at testing how different values of the fracture permeability may influence the flow in the bulk. Finally, the last set of experiments (Section 7.4) aims at showing how the method is capable of handling more complicated geometries, specifically networks of partially immersed fractures.
In this first test case we take and choose as exact solutions in the bulk and in the fracture
It is easy to prove that , and satisfy the coupling conditions (2.5a)-(2.5b) with , and . Note that in this case since the solution in the fracture is constant and .
Figure 2 shows three successive levels of refinements for the polygonal mesh employed in this set of experiments. In order to test the behaviour of the energy norm of the error, thus validating the -convergence properties of our methods proved in Theorem 6.8, we compute the quantity (Figure 3(a) and 3(b)). We also want to validate the results of Theorem 6.9 for the velocity, computing (Figure 3(e)). In addition, we test the behaviour of the -norm of the error for the primal unknowns, i.e., (Figure 3(c) and 3(d)). All the plots in Figure 3 show the computed errors as a function of the inverse of the mesh size (loglog scale). Each plot consists of four lines: Every line shows the behaviour of the computed error for a different polynomial degree in the bulk (we consider uniform degree for all ). For the fracture problem we always choose uniform quadratic polynomial degree, i.e., for all . On the left we report the results obtained with the (MP) approximation scheme and on the right with the (PP) scheme. We observe that, for both methods, the convergence rates are superoptimal with respect to the expected rate of (they coincide with the bulk polynomial degree ). This is probably due to the constant nature of the solution in the fracture. Indeed this behaviour will not be observed in the next set of experiments, cf. Section 7.2, where the solution in the fracture is trigonometric. Moreover, Figure 3(c) and 3(d)) show that one order of convergence is gained for the -norm. Finally, one can clearly see that the level of accuracy achieved by the (PP) and (MP) schemes is the same.
Next, we consider the domain and the fracture . We reproduce the numerical test already proposed in [59] for the primal-primal setting, choosing the exact solutions in the bulk and in the fracture as follows
We impose Dirichlet boundary conditions on the whole and also on . Notice that coupling conditions (2.5a)-(2.5b) are satisfied provided that we take , and , that is . The source terms are then chosen accordingly as
In the experiments, we set the components of the fracture permeability tensor and , we set the fracture thickness and the closure parameter . As before, in order to test the -convergence properties of the primal-primal and mixed-primal schemes, we compute the quantity . In Figure 4 we show the computed errors as a function of the inverse of the mesh size (loglog scale), together with the expected convergence rates. As before, we fix the polynomial degree for the fracture problem , and we vary the polynomial degree for the problem in the bulk taking . Figure 4(a) encloses the results obtained with the mixed-primal approximation, while Figure 4(b) with the primal-primal approximation. In each plot, the four lines describe the behaviour of the energy norm of the error for a different polynomial degree in the bulk. Notice that in this case, for both the (PP) and (MP) method, the theoretical convergence rates are clearly obtained, coinciding with (no superconvergence is observed). In particular, the convergence rate is equal to when the approximation in the bulk is linear, i.e., when and it is equal to in all the other cases, since we always choose a quadratic approximation for the problem in the fracture. Moreover, we remark that also in this case the (PP) and (MP) methods achieve the same level of accuracy.
Next, we reproduce some numerical experiments first presented in [47]. We examine two test cases with bulk domain and fracture domain . In the first case, we consider a fracture with constant permeability, while in the second case we consider a fracture with lower permeability in its middle part, thus presenting a discontinuity. In particular:
(a) Case 1: constant permeability: The permeability tensor in the fracture is given by . The bulk permeability is chosen to be constant and isotropic, i.e., . We impose Dirichlet boundary conditions on the left and right side of the bulk domain and homogeneous Neumann conditions on the top and bottom sides. On the fracture boundaries we impose Dirichlet boundary conditions.
(b) Case 2: discontinous permeabilty: The fracture is subdivided into two areas having different values for the permeability tensor: In the initial and ending part of the fracture the permeability tensor is defined as , while in the middle part the permeability is low and is defined as . The bulk permeability tensor is chosen again equal to the identity matrix, i.e., . In the bulk, we impose the same boundary conditions as in the previous test case, while at the fracture extremities we impose homogeneous Neumann conditions.
The two geometrical configurations are shown in Figures 5(a)-5(b), together with the boundary conditions. For both test cases we take the fracture thickness and the model parameter . Moreover, we discretize the problem in the bulk taking as polynomial degree and the problem in the fracture taking .
The obtained results are shown in Figure 6. For both cases (constant at the top, discontinuous at the bottom) we report the pressure field and Darcy velocity in the bulk (here the grid is very coarse only for visualization purposes) and the value of the pressure along the fracture. In the first case, since we have taken , we can observe that the fluid has the tendency to flow along the fracture. In the second case, one can see that the part of the fracture with low (normal) permeability acts as a geological barrier, so that the fluid tends to avoid it and we can observe a jump of the bulk pressure across it. Our results are in agreement with those obtained in [47].
With this last set of numerical experiments we investigate the capability of our discretization method to deal with more complicated geometrical configurations, considering a network of partially immersed fractures. Our reference is, in this case, [51], where the mathematical model developed in [47] has been extended to fully immersed fractures. In [59] we showed that our method in a primal-primal setting is capable of efficiently handling the configuration. Here, we reproduce the same numerical experiments to demonstrate that this holds true also in a mixed-primal setting.
In order to deal with immersed fractures, we need to supplement our model (2.6) with an equation describing the behaviour of the fracture pressure at the immersed tips. Following [51], we impose a homogeneous Neumann condition, thus assuming that the mass transfer across the immersed tips can be neglected, i.e., on . At the extremities of the fractures that are non-immersed, i.e., , we impose boundary conditions that are consistent with those imposed on in that point.
We consider the bulk domain cut by a network made of four partially immersed fractures: , , and . We perform two numerical experiments. In both of them, the fractures and are impermeable (), while and are partially permeable. In the first configuration, we consider for and the permeabilities and , while in the second, we consider and . Moreover, we vary the imposed boundary conditions as illustrated in Figure 7.
In both the experiments we consider an isotropic bulk permeability tensor i.e., and we assume that all the fractures have aperture . The flow is only generated by boundary conditions, since we take all the forcing terms . Finally, we choose as model parameter .
To obtain our results, we employed cartesian grids featuring approximately the same number of elements as those employed in [51] and such that the immersed tips of the fractures coincide with one of the mesh vertices. For the approximation of the problem in the bulk and in the fracture we chose the polynomial degrees . In Figure 8, we show the results obtained for the two test cases with a mesh of elements. In particular, we report the pressure field in the bulk with the streamlines of the velocity (left), the value of the bulk pressure along the line (middle) and the pressure field inside the four fractures (right). Our results are in perfect agreement with those obtained in [51] and in [59], thus showing that, also in a mixed-primal setting, our method is able to efficiently handle this configuration.
In this paper we have proposed a formulation based on discontinuous Galerkin methods on polygonal/polyhedral grids for the simulation of flows in fractured porous media. In particular, we have designed and analysed, in the unified framework of [63] based on the flux-formulation, a polyDG approximation for all the possible combinations of primal-primal, mixed-primal, primal-mixed and mixed-mixed formulations for the bulk and fracture problems, respectively. The novelty of our method relies on the imposition of coupling conditions between bulk and fracture through a suitable definition of the numerical fluxes on the fracture faces. We have proved in an unified setting the well-posedness of all the formulations and we have derived a priori -version error estimates in a suitable (mesh-dependent) energy norm, whose validity has been assessed performing some preliminary numerical experiments, focusing on the paradigmatic primal-primal and mixed-primal methods. In our test cases we have also compared, in a simplified setting, the performance of our approximation schemes. In particular, we have shown that the same level of accuracy may be obtained irrespective of the chosen method. The other the factors that should be taken into account when choosing which one between the (PP), (MP), (PM), and (MM) setting to employ, are summarized in the following.
● The desired accuracy in the approximation of the physical quantities (pressure and velocity) according to the application at hand. The primal approach considers a single-field formulation with the pressure field of the fluid as only unknown, so that velocity may only be afterwards reconstructed taking the gradient of the computed pressure and multiplying it by the permeability tensor. This process usually entails a loss of accuracy and does not guarantee mass conservation, see for example [61,62], so it may not be suitable for those Engineering applications (such as oil recovery or groundwater pollution modeling) where the simulation of the phenomenon requires very accurate approximation of the velocities of the involved fluids in order to be effective. In such cases, the mixed approach should be preferred, so that Darcy's velocity can be directly computed and a higher degree of accuracy can be achieved, together with local and global mass conservation.
● The numerical linear algebra resulting from the discretization. The primal setting has the advantage of featuring less degrees of freedom and leads to a symmetric positive definite algebraic system of equations, which can be efficiently solved based on employing, for example, multigrid techniques [39,44,60]. The mixed approach leads to a so-called generalized saddle point system of equations. For example, in the MP setting, problem (4.26) translates into an algebraic system of the form
where, referring to Eq. (4.27):
- is the mass matrix related to the bilinear form ;
- is related to the bilinear form ;
- is related to the bilinear form ;
- is related to the terms involving only bulk unknowns contained in the interface bilinear forms and ;
- is related to the terms involving both bulk and fracture unknowns in and ;
- is related to the fracture primal bilinear form ;
- is related to the terms involving only fracture unknowns contained in the interface bilinear forms and , which result in a reaction term for the fracture problem.
In this case the Schur complement of , i.e. the matrix , with , can be computed explicitly, thanks to the fact that, in the DG setting, either has a block-diagonal or a diagonal structure, depending on whether a modal or nodal expansion is chosen to span the discrete space. We also point out that the mixed case involves a (three-time) larger number of unknowns, so that efficient memory handling may be needed.
● The conditioning of the resulting systems. The behaviour of the condition number and the possibility of building efficient preconditioners is certainly of fundamental importance in the choice between the primal or mixed approach, especially for three-dimensional problems. We did not address this issue in the present work. However, one may refer to [76], where preconditioners for the system stemming from the discretization of problem (2.6) in mixed-mixed form, employing mimetic finite differences, are constructed. In the DG setting, for the -preconditioning of systems stemming from the discretization of diffusion problems, the reader may refer to [71] for standard grids and to [39,44,78] for the extension to the polytopic setting.
Finally, we mention that our method may be extended in order to deal with network of intersecting fractures. To this aim, the mathematical model needs to be complemented with some suitable physical conditions at the intersections, prescribing the behaviour of the fluid. One possible choice is to impose pressure continuity and flux conservation as in [52,74]. From the DG-discretization point of view, the key point to deal with this case is the generalization of the concepts of jump and average at the intersections. We refer to [75] for a rigorous analysis of the method in the primal-primal setting and to [59] for a preliminary numerical test case involving a totally immersed network of fractures.
Paola F. Antonietti and Chiara Facciolà have been partially supported by SIR Starting grant n. RBSI14VT0S "PolyPDEs: Non-conforming polyhedral finite element methods for the approximation of partial differential equations" funded by MIUR. Paola F. Antonietti and Marco Verani have been partially funded by the Italian research project PRIN n. 201744KLJL. All the authors have been partially supported by INdAM-GNCS.
The authors declare no conflict of interest.
[1] |
Silva VO, Soares LO, Silva Júnior A, et al. (2014) Biofilm formation on biotic and abiotic surfaces in the presence of antimicrobials by Escherichia coli isolates from cases of bovine mastitis. Appl Environ Microbiol 80: 6136-6145. https://doi.org/10.1128/aem.01953-14 ![]() |
[2] |
Donlan RM (2002) Biofilms: microbial life on surfaces. Emerging Infect Dis 8: 881-890. https://doi.org/10.3201/eid0809.020063 ![]() |
[3] |
Yin W, Wang Y, Liu L, et al. (2019) Biofilms: The microbial “protective clothing” in extreme environments. Int J Mol Sci 20: 3423. https://doi.org/10.3390/ijms20143423 ![]() |
[4] |
Koerdt A, Gödeke J, Berger J, et al. (2010) Crenarchaeal biofilm formation under extreme conditions. PLoS One 5: e14104. https://doi.org/10.1371/journal.pone.0014104 ![]() |
[5] |
Chen Y, Yan F, Chai Y, et al. (2013) Biocontrol of tomato wilt disease by Bacillus subtilis isolates from natural environments depends on conserved genes mediating biofilm formation. Environ Microbiol 15: 848-864. https://doi.org/10.1111/j.1462-2920.2012.02860.x ![]() |
[6] |
Van Houdt R, Michiels CW (2010) Biofilm formation and the food industry, a focus on the bacterial outer surface. J Appl Microbiol 109: 1117-1131. https://doi.org/10.1111/j.1365-2672.2010.04756.x ![]() |
[7] |
Di Martino P (2018) Extracellular polymeric substances, a key element in understanding biofilm phenotype. AIMS Microbiol 4: 274-288. https://doi.org/10.3934/microbiol.2018.2.274 ![]() |
[8] |
Decho AW, Gutierrez T (2017) Microbial extracellular polymeric substances (EPSs) in ocean systems. Front Microbiol 8. https://doi.org/10.3389/fmicb.2017.00922 ![]() |
[9] |
Flemming HC (2016) EPS-Then and Now. Microorganisms 4: 41. https://doi.org/10.3390/microorganisms4040041 ![]() |
[10] |
Kragh KN, Hutchison JB, Melaugh G, et al. (2016) Role of multicellular aggregates in biofilm formation. mBio 7: e00237-00216. https://doi.org/10.1128/mBio.00237-16 ![]() |
[11] |
Taylor M, Ross K, Bentham R (2009) Legionella, Protozoa and Biofilms: Interactions within complex microbial systems. Microbiol Ecol 58: 538-547. https://doi.org/10.1007/s00248-009-9514-z ![]() |
[12] |
de Alexandre Sebastião F, Pilarski F, Lemos MVF (2013) Composition of extracellular polymeric substances (EPS) produced by flavobacterium columnare isolated from tropical fish in Brazil. Braz J Microbiol 44: 861-864. https://doi.org/10.1590/S1517-83822013005000058 ![]() |
[13] |
McSwain BS, Irvine RL, Hausner M, et al. (2005) Composition and distribution of extracellular polymeric substances in aerobic flocs and granular sludge. Appl Environ Microbiol 71: 1051. https://doi.org/10.1128/AEM.71.2.1051-1057.2005 ![]() |
[14] |
López D, Vlamakis H, Kolter R (2010) Biofilms. Cold Spring Harbor Perspect Biol 2: a000398. https://doi.org/10.1101/cshperspect.a000398 ![]() |
[15] |
Nadell CD, Drescher K, Wingreen NS, et al. (2015) Extracellular matrix structure governs invasion resistance in bacterial biofilms. ISME J 9: 1700-1709. https://doi.org/10.1038/ismej.2014.246 ![]() |
[16] |
Davenport EK, Call DR, Beyenal H (2014) Differential protection from tobramycin by extracellular polymeric substances from Acinetobacter baumannii and Staphylococcus aureus biofilms. Antimicrob Agents Chemother 58: 4755-4761. https://doi.org/10.1128/AAC.03071-14 ![]() |
[17] |
Donlan RM (2001) Biofilm formation: A clinically relevant microbiological process. Clin Infect Dis 33: 1387-1392. https://doi.org/10.1086/322972 ![]() |
[18] |
Lewis K (2010) Persister cells. Annu Rev Microbiol 64: 357-372. https://doi.org/10.1146/annurev.micro.112408.134306 ![]() |
[19] |
Rumbaugh KP, Sauer K (2020) Biofilm dispersion. Nat Rev Microbiol 18: 571-586. https://doi.org/10.1038/s41579-020-0385-0 ![]() |
[20] |
Rice SA, Tan CH, Mikkelsen PJ, et al. (2009) The biofilm life cycle and virulence of Pseudomonas aeruginosa are dependent on a filamentous prophage. ISME J 3: 271-282. https://doi.org/10.1038/ismej.2008.109 ![]() |
[21] |
Garrett TR, Bhakoo M, Zhang Z (2008) Bacterial adhesion and biofilms on surfaces. Prog Nat Sci 18: 1049-1056. https://doi.org/10.1016/j.pnsc.2008.04.001 ![]() |
[22] |
Donlan RM (2001) Biofilms and device-associated infections. Emerg Infect Dis 7: 277-281. https://doi.org/10.3201/eid0702.010226 ![]() |
[23] |
Donlan RM, Costerton JW (2002) Biofilms: survival mechanisms of clinically relevant microorganisms. Clin Microbiol Rev 15: 167-193. https://doi.org/10.1128/cmr.15.2.167-193.2002 ![]() |
[24] |
Armbruster CR, Parsek MR (2018) New insight into the early stages of biofilm formation. Proc Natl Acad Sci 115: 4317. https://doi.org/10.1073/pnas.1804084115 ![]() |
[25] |
Da Cunda P, Iribarnegaray V, Papa-Ezdra R, et al. (2019) Characterization of the different stages of biofilm formation and antibiotic susceptibility in a clinical acinetobacter baumannii Strain. Microb Drug Resist 26: 569-575. https://doi.org/10.1089/mdr.2019.0145 ![]() |
[26] |
Rasamiravaka T, Labtani Q, Duez P, et al. (2015) The formation of biofilms by Pseudomonas aeruginosa: a review of the natural and synthetic compounds interfering with control mechanisms. Biomed Res Int 2015: 759348. https://doi.org/10.1155/2015/759348 ![]() |
[27] |
Koczan JM, Lenneman BR, McGrath MJ, et al. (2011) Cell surface attachment structures contribute to biofilm formation and xylem colonization by Erwinia amylovora. Appl Environ Microbiol 77: 7031-7039. https://doi.org/10.1128/AEM.05138-11 ![]() |
[28] |
Mandlik A, Swierczynski A, Das A, et al. (2008) Pili in Gram-positive bacteria: assembly, involvement in colonization and biofilm development. Trends Microbiol 16: 33-40. https://doi.org/10.1016/j.tim.2007.10.010 ![]() |
[29] |
Berne C, Ducret A, Hardy GG, et al. (2015) Adhesins involved in attachment to abiotic surfaces by Gram-Negative bacteria. Microbiol Spectrum 3: 10. https://doi.org/10.1128/microbiolspec.MB-0018-2015 ![]() |
[30] |
Dunne WM (2002) Bacterial adhesion: seen any good biofilms lately?. Clin Microbiol Rev 15: 155-166. https://doi.org/10.1128/cmr.15.2.155-166.2002 ![]() |
[31] |
Renner LD, Weibel DB (2011) Physicochemical regulation of biofilm formation. MRS Bull 36: 347-355. https://doi.org/10.1557/mrs.2011.65 ![]() |
[32] |
Hinsa SM, Espinosa-Urgel M, Ramos JL, et al. (2003) Transition from reversible to irreversible attachment during biofilm formation by Pseudomonas fluorescens WCS365 requires an ABC transporter and a large secreted protein. Mol Microbiol 49: 905-918. https://doi.org/10.1046/j.1365-2958.2003.03615.x ![]() |
[33] |
Petrova OE, Sauer K (2012) Sticky situations: key components that control bacterial surface attachment. J Bacteriol Res 194: 2413-2425. https://doi.org/10.1128/JB.00003-12 ![]() |
[34] |
Miller JK, Badawy HT, Clemons C, et al. (2012) Development of the Pseudomonas aeruginosa mushroom morphology and cavity formation by iron-starvation: a mathematical modeling study. J Theor Biol 308: 68-78. https://doi.org/10.1016/j.jtbi.2012.05.029 ![]() |
[35] |
Abebe GM (2020) The role of bacterial biofilm in antibiotic resistance and food contamination. Int J Microbiol 2020: 1705814. https://doi.org/10.1155/2020/1705814 ![]() |
[36] |
Jefferson KK (2004) What drives bacteria to produce a biofilm?. FEMS Microbiol Lett 236: 163-173. https://doi.org/10.1111/j.1574-6968.2004.tb09643.x ![]() |
[37] |
Kurmoo Y, Hook AL, Harvey D, et al. (2020) Real time monitoring of biofilm formation on coated medical devices for the reduction and interception of bacterial infections. Biomater Sci 8: 1464-1477. https://doi.org/10.1039/c9bm00875f ![]() |
[38] |
Salgar-Chaparro SJ, Lepkova K, Pojtanabuntoeng T, et al. (2020) Nutrient level determines biofilm characteristics and subsequent impact on microbial corrosion and biocide effectiveness. Appl Environ Microbiol 86: e02885-02819. https://doi.org/10.1128/AEM.02885-19 ![]() |
[39] | Wilson C, Lukowicz R, Merchant S, et al. (2017) Quantitative and qualitative assessment methods for biofilm growth: A mini-review. Res Rev J Eng Technol 6. Available from: http://www.rroij.com/open-access/quantitative-and-qualitative-assessment-methods-for-biofilm-growth-a-minireview-.pdf |
[40] |
Haney EF, Trimble MJ, Cheng JT, et al. (2018) Critical assessment of methods to quantify biofilm growth and evaluate antibiofilm activity of host defence peptides. Biomolecules 8: 29. https://doi.org/10.3390/biom8020029 ![]() |
[41] |
Kaplan JB (2010) Biofilm dispersal: mechanisms, clinical implications, and potential therapeutic uses. J Dent Res 89: 205-218. https://doi.org/10.1177/0022034509359403 ![]() |
[42] |
Rabin N, Zheng Y, Opoku-Temeng C, et al. (2015) Biofilm formation mechanisms and targets for developing antibiofilm agents. Future Med Chem 7: 493-512. https://doi.org/10.4155/fmc.15.6 ![]() |
[43] |
Sharpton TJ, Combrink L, Arnold HK, et al. (2021) Erratum to “Harnessing the gut microbiome in the fight against anthelminthic drug resistance”. Curr Opin Microbiol 53: 26-34. https://doi.org/10.1016/j.mib.2021.01.003 ![]() |
[44] |
Seth AK, Geringer MR, Hong SJ, et al. (2012) Comparative analysis of single-species and polybacterial wound biofilms using a quantitative, in vivo, rabbit ear model. PLoS One 7: e42897. https://doi.org/10.1371/journal.pone.0042897 ![]() |
[45] |
Yang L, Liu Y, Wu H, et al. (2011) Current understanding of multi-species biofilms. Int J Oral Sci 3: 74-81. https://doi.org/10.4248/IJOS11027 ![]() |
[46] |
Lohse MB, Gulati M, Johnson AD, et al. (2018) Development and regulation of single- and multi-species Candida albicans biofilms. Nat Rev Microbiol 16: 19-31. https://doi.org/10.1038/nrmicro.2017.107 ![]() |
[47] |
Lynch AS, Robertson GT (2008) Bacterial and fungal biofilm infections. Annu Rev Med 59: 415-428. https://doi.org/10.1146/annurev.med.59.110106.132000 ![]() |
[48] |
Seneviratne G, Zavahir JS, Bandara WMMS, et al. (2007) Fungal-bacterial biofilms: their development for novel biotechnological applications. World J Microbiol Biotechnol 24: 739. https://doi.org/10.1007/s11274-007-9539-8 ![]() |
[49] | Guo YS, Furrer JM, Kadilak AL, et al. (2018) Bacterial extracellular polymeric substances amplify water content variability at the pore scale. Front Environ Sci 6. https://doi.org/10.3389/fenvs.2018.00093 |
[50] | Wingender J, Neu TR, Flemming HC, et al. (1999) What are bacterial extracellular polymeric substances?. Microbial extracellular polymeric substances: Characterization, structure and function . Berlin: Springer Berlin Heidelberg 1-19. https://doi.org/10.1007/978-3-642-60147-7_1 |
[51] |
Wilking JN, Zaburdaev V, De Volder M, et al. (2013) Liquid transport facilitated by channels in Bacillus subtilis biofilms. Proc Natl Acad Sci U S A 110: 848-852. https://doi.org/10.1073/pnas.1216376110 ![]() |
[52] |
Costa OYA, Raaijmakers JM, Kuramae EE (2018) Microbial extracellular polymeric substances: ecological function and impact on soil aggregation. Front Microbiol 9: 1636-1636. https://doi.org/10.3389/fmicb.2018.01636 ![]() |
[53] |
Navarre WW, Schneewind O (1999) Surface proteins of gram-positive bacteria and mechanisms of their targeting to the cell wall envelope. Microbiol Mol Biol Rev 63: 174. https://doi.org/10.1128/MMBR.63.1.174-229.1999 ![]() |
[54] |
Davey ME, O'Toole GA (2000) Microbial biofilms: from ecology to molecular genetics. Microbiol Mol Biol Rev: MMBR 64: 847-867. https://doi.org/10.1128/mmbr.64.4.847-867.2000 ![]() |
[55] |
Hibiya K, Tsuneda S, Hirata A (2000) Formation and characteristics of nitrifying biofilm on a membrane modified with positively-charged polymer chains. Colloids Surf, B 18: 105-112. https://doi.org/10.1016/S0927-7765(99)00141-1 ![]() |
[56] |
Navada S, Knutsen MF, Bakke I, et al. (2020) Nitrifying biofilms deprived of organic carbon show higher functional resilience to increases in carbon supply. Sci Rep 10: 7121. https://doi.org/10.1038/s41598-020-64027-y ![]() |
[57] |
Hunt SM, Werner EM, Huang B, et al. (2004) Hypothesis for the role of nutrient starvation in biofilm detachment. Appl Environ Microbiol 70: 7418-7425. https://doi.org/10.1128/AEM.70.12.7418-7425.2004 ![]() |
[58] |
Maddela NR, Zhou Z, Yu Z, et al. (2018) Functional determinants of extracellular polymeric substances in membrane biofouling: Experimental evidence from pure-cultured sludge bacteria. Appl Environ Microbiol 84: e00756-00718. https://doi.org/10.1128/AEM.00756-18 ![]() |
[59] |
Stoodley P, Sauer K, Davies DG, et al. (2002) Biofilms as complex differentiated communities. Annu Rev Microbiol 56: 187-209. https://doi.org/10.1146/annurev.micro.56.012302.160705 ![]() |
[60] |
Limoli DH, Jones CJ, Wozniak DJ (2015) Bacterial extracellular polysaccharides in biofilm formation and function. Microbiol Spectrum 3: 10. https://doi.org/10.1128/microbiolspec.MB-0011-2014 ![]() |
[61] |
Magana M, Sereti C, Ioannidis A, et al. (2018) Options and limitations in clinical investigation of bacterial biofilms. Clin Microbiol Rev 31: e00084-16. https://doi.org/10.1128/CMR.00084-16 ![]() |
[62] |
Denef VJ, Mueller RS, Banfield JF (2010) AMD biofilms: using model communities to study microbial evolution and ecological complexity in nature. ISME J 4: 599-610. https://doi.org/10.1038/ismej.2009.158 ![]() |
[63] |
Martinez-Gil M, Goh KGK, Rackaityte E, et al. (2017) YeeJ is an inverse autotransporter from Escherichia coli that binds to peptidoglycan and promotes biofilm formation. Sci Rep 7: 11326. https://doi.org/10.1038/s41598-017-10902-0 ![]() |
[64] | Beloin C, Roux A, Ghigo JM (2008) Escherichia coli biofilms. Curr Top Microbio Immunol 322: 249-289. https://doi.org/10.1007/978-3-540-75418-3_12 |
[65] |
Klemm P, Hjerrild L, Gjermansen M, et al. (2004) Structure-function analysis of the self-recognizing Antigen 43 autotransporter protein from Escherichia coli. Mol Microbiol 51: 283-296. https://doi.org/10.1046/j.1365-2958.2003.03833.x ![]() |
[66] |
Müller D, Benz I, Tapadar D, et al. (2005) Arrangement of the translocator of the autotransporter adhesin involved in diffuse adherence on the bacterial surface. Infect Immun 73: 3851-3859. https://doi.org/10.1128/IAI.73.7.3851-3859.2005 ![]() |
[67] |
Barnhart MM, Chapman MR (2006) Curli biogenesis and function. Annu Rev Microbiol 60: 131-147. https://doi.org/10.1146/annurev.micro.60.080805.142106 ![]() |
[68] |
Tursi SA, Tükel Ç (2018) Curli-Containing enteric biofilms inside and out: Matrix composition, immune recognition, and disease implications. Microbiol Mol Biol Rev 82: e00028-18. https://doi.org/10.1128/MMBR.00028-18 ![]() |
[69] |
Hobley L, Harkins C, MacPhee CE, et al. (2015) Giving structure to the biofilm matrix: an overview of individual strategies and emerging common themes. FEMS Microbiol Rev 39: 649-669. https://doi.org/10.1093/femsre/fuv015 ![]() |
[70] |
Chen XD, Zhang CK, Zhou Z, et al. (2017) Stabilizing effects of bacterial biofilms: EPS penetration and redistribution of bed stability down the sediment profile. J Geophys Res: Biogeosci 122: 3113-3125. https://doi.org/10.1002/2017JG004050 ![]() |
[71] |
Ibáñez de Aldecoa AL, Zafra O, González-Pastor JE (2017) Mechanisms and regulation of extracellular DNA release and its biological roles in microbial communities. Front Microbiol 8: 1390-1390. https://doi.org/10.3389/fmicb.2017.01390 ![]() |
[72] |
Jamal M, Ahmad W, Andleeb S, et al. (2018) Bacterial biofilm and associated infections. J Chin Med Assoc 81: 7-11. https://doi.org/10.1016/j.jcma.2017.07.012 ![]() |
[73] |
Toyofuku M, Inaba T, Kiyokawa T, et al. (2016) Environmental factors that shape biofilm formation. Biosci Biotechnol Biochem 80: 7-12. https://doi.org/10.1080/09168451.2015.1058701 ![]() |
[74] |
Vu B, Chen M, Crawford RJ, et al. (2009) Bacterial extracellular polysaccharides involved in biofilm formation. Molecules (Basel,Switz) 14: 2535-2554. https://doi.org/10.3390/molecules14072535 ![]() |
[75] |
Skariyachan S, Sridhar VS, Packirisamy S, et al. (2018) Recent perspectives on the molecular basis of biofilm formation by Pseudomonas aeruginosa and approaches for treatment and biofilm dispersal. Folia Microbiol 63: 413-432. https://doi.org/10.1007/s12223-018-0585-4 ![]() |
[76] |
Mann EE, Wozniak DJ (2012) Pseudomonas biofilm matrix composition and niche biology. FEMS Microbiol Rev 36: 893-916. https://doi.org/10.1111/j.1574-6976.2011.00322.x ![]() |
[77] |
Zambrano MM, Kolter R (2005) Mycobacterial biofilms: A greasy way to hold it together. Cell 123: 762-764. https://doi.org/10.1016/j.cell.2005.11.011 ![]() |
[78] |
Li YH, Tian X (2012) Quorum sensing and bacterial social interactions in biofilms. Sensors (Basel,Switz) 12: 2519-2538. https://doi.org/10.3390/s120302519 ![]() |
[79] |
Sharma D, Misba L, Khan AU (2019) Antibiotics versus biofilm: an emerging battleground in microbial communities. Antimicrob Resist Infect Control 8: 76. https://doi.org/10.1186/s13756-019-0533-3 ![]() |
[80] |
Shrout JD, Chopp DL, Just CL, et al. (2006) The impact of quorum sensing and swarming motility on Pseudomonas aeruginosa biofilm formation is nutritionally conditional. Mol Microbiol 62: 1264-1277. https://doi.org/10.1111/j.1365-2958.2006.05421.x ![]() |
[81] |
Saygin H, Baysal A (2020) Biofilm formation of clinically important bacteria on bio-based and conventional micro/submicron-sized plastics. Bull Environ Contam Toxicol 105: 18-25. https://doi.org/10.1007/s00128-020-02876-z ![]() |
[82] |
Fulaz S, Vitale S, Quinn L, et al. (2019) Nanoparticle-Biofilm interactions: The role of the EPS matrix. Trends Microbiol 27: 915-926. https://doi.org/10.1016/j.tim.2019.07.004 ![]() |
[83] |
Chakraborty P, Kumar A (2019) The extracellular matrix of mycobacterial biofilms: could we shorten the treatment of mycobacterial infections?. Microb Cell (Graz,Austria) 6: 105-122. https://doi.org/10.15698/mic2019.02.667 ![]() |
[84] |
Yawata Y, Uchiyama H, Nomura N (2010) Visualizing the effects of biofilm structures on the influx of fluorescent material using combined confocal reflection and fluorescent microscopy. Microbes Environ 25: 49-52. https://doi.org/10.1264/jsme2.me09169 ![]() |
[85] |
Holman HY, Miles R, Hao Z, et al. (2009) Real-time chemical imaging of bacterial activity in biofilms using open-channel microfluidics and synchrotron FTIR spectromicroscopy. Anal Chem 81: 8564-8570. https://doi.org/10.1021/ac9015424 ![]() |
[86] |
Mattana S, Alunni Cardinali M, Caponi S, et al. (2017) High-contrast brillouin and raman micro-spectroscopy for simultaneous mechanical and chemical investigation of microbial biofilms. Biophys Chem 229: 123-129. https://doi.org/10.1016/j.bpc.2017.06.008 ![]() |
[87] |
Abadian PN, Tandogan N, Jamieson JJ, et al. (2014) Using surface plasmon resonance imaging to study bacterial biofilms. Biomicrofluidics 8: 021804. https://doi.org/10.1063/1.4867739 ![]() |
[88] |
McGoverin C, Vanholsbeeck F, Dawes JM, et al. (2020) Asia-pacific optical sensors conference: focus issue introduction. Opt Express 28: 21745-21748. https://doi.org/10.1364/OE.401277 ![]() |
[89] |
Yuan Y, Guo T, Qiu X, et al. (2016) Electrochemical surface plasmon resonance fiber-optic sensor: In situ detection of electroactive biofilms. Anal Chem 88: 7609-7616. https://doi.org/10.1021/acs.analchem.6b01314 ![]() |
[90] |
Subramanian S, Huiszoon RC, Chu S, et al. (2019) Microsystems for biofilm characterization and sensing-A review. Biofilm 2: 100015. https://doi.org/10.1016/j.bioflm.2019.100015 ![]() |
[91] |
Stöckl M, Schlegel C, Sydow A, et al. (2016) Membrane separated flow cell for parallelized electrochemical impedance spectroscopy and confocal laser scanning microscopy to characterize electro-active microorganisms. Electrochim Acta 220: 444-452. https://doi.org/10.1016/j.electacta.2016.10.057 ![]() |
[92] |
Estrada-Leypon O, Moya A, Guimera A, et al. (2015) Simultaneous monitoring of Staphylococcus aureus growth in a multi-parametric microfluidic platform using microscopy and impedance spectroscopy. Bioelectrochemistry 105: 56-64. https://doi.org/10.1016/j.bioelechem.2015.05.006 ![]() |
[93] |
Huiszoon RC, Subramanian S, Ramiah Rajasekaran P, et al. (2019) Flexible platform for in situ impedimetric detection and bioelectric effect treatment of Escherichia coli biofilms. IEEE Trans Biomed Eng 66: 1337-1345. https://doi.org/10.1109/tbme.2018.2872896 ![]() |
[94] |
Bayoudh S, Othmane A, Ponsonnet L, et al. (2008) Electrical detection and characterization of bacterial adhesion using electrochemical impedance spectroscopy-based flow chamber. Colloids Surf, A 318: 291-300. https://doi.org/10.1016/j.colsurfa.2008.01.005 ![]() |
[95] |
Bellin DL, Sakhtah H, Zhang Y, et al. (2016) Electrochemical camera chip for simultaneous imaging of multiple metabolites in biofilms. Nat Commun 7: 10535. https://doi.org/10.1038/ncomms10535 ![]() |
[96] |
Marcus IM, Herzberg M, Walker SL, et al. (2012) Pseudomonas aeruginosa attachment on QCM-D sensors: the role of cell and surface hydrophobicities. Langmuir 28: 6396-6402. https://doi.org/10.1021/la300333c ![]() |
[97] |
Olsson AL, van der Mei HC, Busscher HJ, et al. (2009) Influence of cell surface appendages on the bacterium-substratum interface measured real-time using QCM-D. Langmuir 25: 1627-1632. https://doi.org/10.1021/la803301q ![]() |
[98] |
Piasecki T, Guła G, Markwitz P, et al. (2016) Autonomous system for in situ assay of antibiotic activity on bacterial biofilms using viscosity and density sensing quartz tuning forks. Procedia Eng 168: 745-748. https://doi.org/10.1016/j.proeng.2016.11.267 ![]() |
[99] |
Sfaelou S, Karapanagioti HK, Vakros J (2015) Studying the formation of biofilms on supports with different polarity and their efficiency to treat wastewater. J Chem 2015: 734384. https://doi.org/10.1155/2015/734384 ![]() |
[100] |
Khatoon Z, McTiernan CD, Suuronen EJ, et al. (2018) Bacterial biofilm formation on implantable devices and approaches to its treatment and prevention. Heliyon 4: e01067. https://doi.org/10.1016/j.heliyon.2018.e01067 ![]() |
[101] | Revdiwala S, Rajdev BM, Mulla S (2012) Characterization of bacterial etiologic agents of biofilm formation in medical devices in critical care setup. Crit Care Res Pract 2012: 945805. https://doi.org/10.1155/2012/945805 |
[102] |
Trautner BW, Darouiche RO (2004) Role of biofilm in catheter-associated urinary tract infection. Am J Infect Control 32: 177-183. https://doi.org/10.1016/j.ajic.2003.08.005 ![]() |
[103] |
Liu S, Gunawan C, Barraud N, et al. (2016) Understanding, monitoring, and controlling biofilm growth in drinking water distribution systems. Environ Sci Technol 50: 8954-8976. https://doi.org/10.1021/acs.est.6b00835 ![]() |
[104] |
Maes S, Vackier T, Nguyen Huu S, et al. (2019) Occurrence and characterisation of biofilms in drinking water systems of broiler houses. BMC Microbiol 19: 77. https://doi.org/10.1186/s12866-019-1451-5 ![]() |
[105] |
Muhammad MH, Idris AL, Fan X, et al. (2020) Beyond risk: bacterial biofilms and their regulating approaches. Front Microbiol 11: 928-928. https://doi.org/10.3389/fmicb.2020.00928 ![]() |
[106] |
Ashbolt NJ (2015) Microbial contamination of drinking water and human health from community water systems. Curr Environ Health Rep 2: 95-106. https://doi.org/10.1007/s40572-014-0037-5 ![]() |
[107] |
Archer NK, Mazaitis MJ, Costerton JW, et al. (2011) Staphylococcus aureus biofilms: properties, regulation, and roles in human disease. Virulence 2: 445-459. https://doi.org/10.4161/viru.2.5.17724 ![]() |
[108] |
Arciola CR, Campoccia D, Montanaro L (2018) Implant infections: adhesion, biofilm formation and immune evasion. Nat Rev Microbiol 16: 397-409. https://doi.org/10.1038/s41579-018-0019-y ![]() |
[109] |
Boisvert AA, Cheng MP, Sheppard DC, et al. (2016) Microbial biofilms in pulmonary and critical care diseases. Ann Am Thorac Soc 13: 1615-1623. https://doi.org/10.1513/AnnalsATS.201603-194FR ![]() |
[110] |
Gnanadhas DP, Elango M, Datey A, et al. (2015) Chronic lung infection by Pseudomonas aeruginosa biofilm is cured by L-Methionine in combination with antibiotic therapy. Sci Rep 5: 16043. https://doi.org/10.1038/srep16043 ![]() |
[111] |
Minasyan H (2019) Sepsis: mechanisms of bacterial injury to the patient. Scand J Trauma Resusc Emerg Med 27: 19. https://doi.org/10.1186/s13049-019-0596-4 ![]() |
[112] |
VanEpps JS, Younger JG (2016) Implantable device-related infection. Shock (Augusta,Ga) 46: 597-608. https://doi.org/10.1097/SHK.0000000000000692 ![]() |
[113] |
Stewart PS (2002) Mechanisms of antibiotic resistance in bacterial biofilms. Int J Med Microbiol 292: 107-113. https://doi.org/10.1078/1438-4221-00196 ![]() |
[114] |
Vestby LK, Grønseth T, Simm R, et al. (2020) Bacterial biofilm and its role in the pathogenesis of disease. Antibiotics (Basel,Switz) 9: 59. https://doi.org/10.3390/antibiotics9020059 ![]() |
[115] |
González JF, Hahn MM, Gunn JS (2018) Chronic biofilm-based infections: skewing of the immune response. Pathog Dis 76: fty023. https://doi.org/10.1093/femspd/fty023 ![]() |
[116] |
Elias S, Banin E (2012) Multi-species biofilms: living with friendly neighbors. FEMS Microbiol Rev 36: 990-1004. https://doi.org/10.1111/j.1574-6976.2012.00325.x ![]() |
[117] | Hu X, Kang F, Yang B, et al. (2019) Extracellular polymeric substances acting as a permeable barrier hinder the lateral transfer of antibiotic resistance genes. Front Microbiol 10. https://doi.org/10.3389/fmicb.2019.00736 |
[118] |
Singh S, Singh SK, Chowdhury I, et al. (2017) Understanding the mechanism of bacterial biofilms resistance to antimicrobial agents. Open Microbiol J 11: 53-62. https://doi.org/10.2174/1874285801711010053 ![]() |
[119] |
Roy R, Tiwari M, Donelli G, et al. (2018) Strategies for combating bacterial biofilms: A focus on anti-biofilm agents and their mechanisms of action. Virulence 9: 522-554. https://doi.org/10.1080/21505594.2017.1313372 ![]() |
[120] |
Koo H, Allan RN, Howlin RP, et al. (2017) Targeting microbial biofilms: current and prospective therapeutic strategies. Nat Rev Microbiol 15: 740-755. https://doi.org/10.1038/nrmicro.2017.99 ![]() |
[121] |
Igiri BE, Okoduwa SIR, Idoko GO, et al. (2018) Toxicity and bioremediation of heavy metals contaminated ecosystem from tannery wastewater: A review. J Toxicol 2018: 2568038. https://doi.org/10.1155/2018/2568038 ![]() |
[122] |
Singh R, Paul D, Jain RK (2006) Biofilms: implications in bioremediation. Trends Microbiol 14: 389-397. https://doi.org/10.1016/j.tim.2006.07.001 ![]() |
[123] | Bispo PJM, Haas W, Gilmore MS (2015) Biofilms in infections of the eye. Pathogens (Basel,Switz) 4: 111-136. https://doi.org/10.3390/pathogens4010111 |
[124] | Nickel JC, Costerton JW (1992) Bacterial biofilms and catheters: A key to understanding bacterial strategies in catheter-associated urinary tract infection. Can J Infect Dis 3: 261-267. https://doi.org/10.1155/1992/517456 |
[125] | Guynn JB, Poretz DM, Duma RJ (1973) Growth of various bacteria in a variety of intravenous fluids. Am J Hosp Pharm 30: 321-325. https://doi.org/10.1093/ajhp/30.4.321 |
[126] |
Ferrières L, Hancock V, Klemm P (2007) Specific selection for virulent urinary tract infectious Escherichia coli strains during catheter-associated biofilm formation. FEMS Immunol Med Microbiol 51: 212-219. https://doi.org/10.1111/j.1574-695X.2007.00296.x ![]() |
[127] | Delcaru C, Alexandru I, Podgoreanu P, et al. (2016) Microbial biofilms in urinary tract infections and prostatitis: Etiology, pathogenicity, and combating strategies. Pathogens (Basel,Switz) 5: 65. https://doi.org/10.3390/pathogens5040065 |
[128] |
Wiley L, Bridge DR, Wiley LA, et al. (2012) Bacterial biofilm diversity in contact lens-related disease: Emerging role of achromobacter, stenotrophomonas, and delftia. Invest Ophthalmol Visual Sci 53: 3896-3905. https://doi.org/10.1167/iovs.11-8762 ![]() |
[129] |
Robertson DM, Parks QM, Young RL, et al. (2011) Disruption of contact lens-associated Pseudomonas aeruginosa biofilms formed in the presence of neutrophils. Invest Ophthalmol Visual Sci 52: 2844-2850. https://doi.org/10.1167/iovs.10-6469 ![]() |
[130] |
Litzler PY, Benard L, Barbier-Frebourg N, et al. (2007) Biofilm formation on pyrolytic carbon heart valves: influence of surface free energy, roughness, and bacterial species. J Thorac Cardiovasc Surg 134: 1025-1032. https://doi.org/10.1016/j.jtcvs.2007.06.013 ![]() |
[131] | Piper C, Körfer R, Horstkotte D (2001) Prosthetic valve endocarditis. Heart 85: 590. https://doi.org/10.1136/heart.85.5.590 |
[132] |
Schaudinn C, Gorur A, Keller D, et al. (2009) Periodontitis: an archetypical biofilm disease. J Am Dent Assoc 140: 978-986. https://doi.org/10.14219/jada.archive.2009.0307 ![]() |
[133] |
Naginyte M, Do T, Meade J, et al. (2019) Enrichment of periodontal pathogens from the biofilms of healthy adults. Sci Rep 9: 5491. https://doi.org/10.1038/s41598-019-41882-y ![]() |
[134] |
Chandki R, Banthia P, Banthia R (2011) Biofilms: A microbial home. J Indian Soc Periodontol 15: 111-114. https://doi.org/10.4103/0972-124X.84377 ![]() |
[135] |
Brady RA, Leid JG, Calhoun JH, et al. (2008) Osteomyelitis and the role of biofilms in chronic infection. FEMS Immunol Med Microbiol 52: 13-22. https://doi.org/10.1111/j.1574-695X.2007.00357.x ![]() |
[136] |
Masters EA, Trombetta RP, de Mesy Bentley KL, et al. (2019) Evolving concepts in bone infection: redefining “biofilm”, “acute vs. chronic osteomyelitis”, “the immune proteome” and “local antibiotic therapy”. Bone Res 7: 20. https://doi.org/10.1038/s41413-019-0061-z ![]() |
[137] |
Rochford ETJ, Sabaté Brescó M, Zeiter S, et al. (2016) Monitoring immune responses in a mouse model of fracture fixation with and without Staphylococcus aureus osteomyelitis. Bone 83: 82-92. https://doi.org/10.1016/j.bone.2015.10.014 ![]() |
[138] |
Oates A, Bowling FL, Boulton AJM, et al. (2014) The visualization of biofilms in chronic diabetic foot wounds using routine diagnostic microscopy methods. J Diabetes Res 2014: 153586. https://doi.org/10.1155/2014/153586 ![]() |
[139] |
Clinton A, Carter T (2015) Chronic wound biofilms: Pathogenesis and potential therapies. Lab Med 46: 277-284. https://doi.org/10.1309/lmbnswkui4jpn7so ![]() |
[140] |
Bjarnsholt T (2013) The role of bacterial biofilms in chronic infections. APMIS Suppl : 1-51. https://doi.org/10.1111/apm.12099 ![]() |
[141] |
Burmølle M, Thomsen TR, Fazli M, et al. (2010) Biofilms in chronic infections-a matter of opportunity-monospecies biofilms in multispecies infections. FEMS Immunol Med Microbiol 59: 324-336. https://doi.org/10.1111/j.1574-695X.2010.00714.x ![]() |
[142] |
Percival SL, McCarty SM, Lipsky B (2015) Biofilms and wounds: An overview of the evidence. Adv Wound Care 4: 373-381. https://doi.org/10.1089/wound.2014.0557 ![]() |
[143] |
Abdel-Nour M, Duncan C, Low DE, et al. (2013) Biofilms: the stronghold of Legionella pneumophila. Int J Mol Sci 14: 21660-21675. https://doi.org/10.3390/ijms141121660 ![]() |
[144] |
Høiby N, Ciofu O, Bjarnsholt T (2010) Pseudomonas aeruginosa biofilms in cystic fibrosis. Future Microbiol 5: 1663-1674. https://doi.org/10.2217/fmb.10.125 ![]() |
[145] |
Moreau-Marquis S, Stanton BA, O'Toole GA (2008) Pseudomonas aeruginosa biofilm formation in the cystic fibrosis airway. Pulm Pharmacol Ther 21: 595-599. https://doi.org/10.1016/j.pupt.2007.12.001 ![]() |
[146] |
Lalani T, Kanafani ZA, Chu VH, et al. (2006) Prosthetic valve endocarditis due to coagulase-negative staphylococci: findings from the international collaboration on endocarditis merged database. Eur J Clin Microbiol Infect Dis 25: 365-368. https://doi.org/10.1007/s10096-006-0141-z ![]() |
[147] |
van Steenbergen TJM, van Winkelhoff AJ, de Graaff J (1984) Pathogenic synergy: mixed infections in the oral cavity. Antonie van Leeuwenhoek 50: 789-798. https://doi.org/10.1007/BF02386241 ![]() |
[148] |
Chen L, Deng H, Cui H, et al. (2017) Inflammatory responses and inflammation-associated diseases in organs. Oncotarget 9: 7204-7218. https://doi.org/10.18632/oncotarget.23208 ![]() |
[149] |
Kany S, Vollrath JT, Relja B (2019) Cytokines in inflammatory disease. Int J Mol Sci 20: 6008. https://doi.org/10.3390/ijms20236008 ![]() |
[150] |
Hall-Stoodley L, Stoodley P, Kathju S, et al. (2012) Towards diagnostic guidelines for biofilm-associated infections. FEMS Immunol Med Microbiol 65: 127-145. https://doi.org/10.1111/j.1574-695X.2012.00968.x ![]() |
[151] | Olson ME, Ceri H, Morck DW, et al. (2002) Biofilm bacteria: formation and comparative susceptibility to antibiotics. Can J Vet Res 66: 86-92. |
[152] |
Mosaddad SA, Tahmasebi E, Yazdanian A, et al. (2019) Oral microbial biofilms: an update. Eur J Clin Microbiol Infect Dis 38: 2005-2019. https://doi.org/10.1007/s10096-019-03641-9 ![]() |
[153] | Fair RJ, Tor Y (2014) Antibiotics and bacterial resistance in the 21st century. Perspect Med Chem 6: 25-64. https://doi.org/10.4137/PMC.S14459 |
[154] |
Hedberg M, Nord CE (1996) Beta-lactam resistance in anaerobic bacteria: a review. J Chemother 8: 3-16. https://doi.org/10.1179/joc.1996.8.1.3 ![]() |
[155] |
Macià MD, Rojo-Molinero E, Oliver A (2014) Antimicrobial susceptibility testing in biofilm-growing bacteria. Clin Microbiol Infect 20: 981-990. https://doi.org/10.1111/1469-0691.12651 ![]() |
[156] |
Lewis K (2001) Riddle of biofilm resistance. Antimicrob Agents Chemother 45: 999. https://doi.org/10.1128/AAC.45.4.999-1007.2001 ![]() |
[157] |
Kaplan JB, Mlynek KD, Hettiarachchi H, et al. (2018) Extracellular polymeric substance (EPS)-degrading enzymes reduce Staphylococcal surface attachment and biocide resistance on pig skin in vivo. PLoS One 13: e0205526. https://doi.org/10.1371/journal.pone.0205526 ![]() |
[158] |
Powell LC, Pritchard MF, Ferguson EL, et al. (2018) Targeted disruption of the extracellular polymeric network of Pseudomonas aeruginosa biofilms by alginate oligosaccharides. npj Biofilms Microbiomes 4: 13. https://doi.org/10.1038/s41522-018-0056-3 ![]() |
[159] |
Wozniak DJ, Wyckoff TJ, Starkey M, et al. (2003) Alginate is not a significant component of the extracellular polysaccharide matrix of PA14 and PAO1 Pseudomonas aeruginosa biofilms. Proc Natl Acad Sci U S A 100: 7907-7912. https://doi.org/10.1073/pnas.1231792100 ![]() |
[160] |
Madsen JS, Burmølle M, Hansen LH, et al. (2012) The interconnection between biofilm formation and horizontal gene transfer. FEMS Immunol Med Microbiol 65: 183-195. https://doi.org/10.1111/j.1574-695X.2012.00960.x ![]() |
[161] |
Lister PD, Wolter DJ, Hanson ND (2009) Antibacterial-resistant Pseudomonas aeruginosa: clinical impact and complex regulation of chromosomally encoded resistance mechanisms. Clin Microbiol Rev 22: 582-610. https://doi.org/10.1128/CMR.00040-09 ![]() |
[162] |
Wilton M, Charron-Mazenod L, Moore R, et al. (2015) Extracellular DNA acidifies biofilms and induces aminoglycoside resistance in Pseudomonas aeruginosa. Antimicrob Agents Chemother 60: 544-553. https://doi.org/10.1128/AAC.01650-15 ![]() |
[163] |
Mulcahy H, Charron-Mazenod L, Lewenza S (2008) Extracellular DNA chelates cations and induces antibiotic resistance in Pseudomonas aeruginosa biofilms. PLoS Pathog 4: e1000213. https://doi.org/10.1371/journal.ppat.1000213 ![]() |
[164] |
Heilmann C, Hartleib J, Hussain MS, et al. (2005) The multifunctional Staphylococcus aureus autolysin aaa mediates adherence to immobilized fibrinogen and fibronectin. Infect Immunol 73: 4793-4802. https://doi.org/10.1128/iai.73.8.4793-4802.2005 ![]() |
[165] |
Heilmann C, Hussain M, Peters G, et al. (1997) Evidence for autolysin-mediated primary attachment of Staphylococcus epidermidis to a polystyrene surface. Mol Microbiol 24: 1013-1024. https://doi.org/10.1046/j.1365-2958.1997.4101774.x ![]() |
[166] |
Ch'ng JH, Chong KKL, Lam LN, et al. (2019) Biofilm-associated infection by enterococci. Nat Rev Microbiol 17: 82-94. https://doi.org/10.1038/s41579-018-0107-z ![]() |
[167] |
Dale JL, Nilson JL, Barnes AMT, et al. (2017) Restructuring of Enterococcus faecalis biofilm architecture in response to antibiotic-induced stress. npj Biofilms Microbiomes 3: 15. https://doi.org/10.1038/s41522-017-0023-4 ![]() |
[168] |
Balaban NQ, Helaine S, Lewis K, et al. (2019) Definitions and guidelines for research on antibiotic persistence. Nat Rev Microbiol 17: 441-448. https://doi.org/10.1038/s41579-019-0196-3 ![]() |
[169] |
Schilcher K, Horswill AR (2020) Staphylococcal biofilm development: Structure, regulation, and treatment strategies. Microbiol Mol Biol Rev 84: e00026-19. https://doi.org/doi:10.1128/MMBR.00026-19 ![]() |
[170] |
Waters EM, Rowe SE, O'Gara JP, et al. (2016) Convergence of Staphylococcus aureus persister and biofilm research: Can biofilms be defined as communities of adherent persister cells?. PLoS Pathog 12: e1006012. https://doi.org/10.1371/journal.ppat.1006012 ![]() |
[171] |
Lister JL, Horswill AR (2014) Staphylococcus aureus biofilms: recent developments in biofilm dispersal. Front Cell Infect Microbiol 4. https://doi.org/10.3389/fcimb.2014.00178 ![]() |
[172] |
Vuotto C, Longo F, Balice MP, et al. (2014) Antibiotic resistance related to biofilm formation in Klebsiella pneumoniae. Pathogens 3: 743-758. https://doi.org/10.3390/pathogens3030743 ![]() |
[173] |
Nirwati H, Sinanjung K, Fahrunissa F, et al. (2019) Biofilm formation and antibiotic resistance of Klebsiella pneumoniae isolated from clinical samples in a tertiary care hospital, Klaten, Indonesia. BMC Proc 13: 20. https://doi.org/10.1186/s12919-019-0176-7 ![]() |
[174] |
Ramos-Vivas J, Chapartegui-González I, Fernández-Martínez M, et al. (2019) Biofilm formation by multidrug resistant Enterobacteriaceae strains isolated from solid organ transplant recipients. Sci Rep 9: 8928. https://doi.org/10.1038/s41598-019-45060-y ![]() |
[175] |
Yang CH, Su PW, Moi SH, et al. (2019) Biofilm formation in acinetobacter baumannii: genotype-phenotype correlation. Molecules (Basel,Switz) 24: 1849. https://doi.org/10.3390/molecules24101849 ![]() |
[176] |
Smani Y, McConnell MJ, Pachón J, et al. (2012) Role of fibronectin in the adhesion of Acinetobacter baumannii to host cells. PLoS One 7: e33073. https://doi.org/10.1371/journal.pone.0033073 ![]() |
[177] |
Gaddy JA, Tomaras AP, Actis LA (2009) The Acinetobacter baumannii 19606 OmpA protein plays a role in biofilm formation on abiotic surfaces and in the interaction of this pathogen with eukaryotic cells. Infect Immun 77: 3150-3160. https://doi.org/10.1128/iai.00096-09 ![]() |
[178] |
Dumaru R, Baral R, Shrestha LB (2019) Study of biofilm formation and antibiotic resistance pattern of gram-negative Bacilli among the clinical isolates at BPKIHS, Dharan. BMC Res Notes 12: 38. https://doi.org/10.1186/s13104-019-4084-8 ![]() |
[179] |
Poulsen LK, Ballard G, Stahl DA (1993) Use of rRNA fluorescence in situ hybridization for measuring the activity of single cells in young and established biofilms. Appl Environ Microbiol 59: 1354-1360. https://doi.org/10.1128/aem.59.5.1354-1360.1993 ![]() |
[180] |
Sternberg C, Christensen BB, Johansen T, et al. (1999) Distribution of bacterial growth activity in flow-chamber biofilms. Appl Environ Microbiol 65: 4108-4117. https://doi.org/10.1128/aem.65.9.4108-4117.1999 ![]() |
[181] |
Mitchison JM (1969) Enzyme synthesis in synchronous cultures. Science 165: 657-663. https://doi.org/10.1126/science.165.3894.657 ![]() |
[182] | Gilbert P, Maira-Litran T, McBain AJ, et al. (2002) The physiology and collective recalcitrance of microbial biofilm communities. Adv Microb Physiol 46: 202-256. https://doi.org/10.1016/S0065-2911(02)46005-5 |
[183] |
Walters MC, Roe F, Bugnicourt A, et al. (2003) Contributions of antibiotic penetration, oxygen limitation, and low metabolic activity to tolerance of Pseudomonas aeruginosa biofilms to ciprofloxacin and tobramycin. Antimicrob Agents Chemother 47: 317-323. https://doi.org/10.1128/aac.47.1.317-323.2003 ![]() |
[184] |
Tresse O, Jouenne T, Junter GA (1995) The role of oxygen limitation in the resistance of agar-entrapped, sessile-like Escherichia coli to aminoglycoside and beta-lactam antibiotics. J Antimicrob Chemother 36: 521-526. https://doi.org/10.1093/jac/36.3.521 ![]() |
[185] |
Hall CW, Mah TF (2017) Molecular mechanisms of biofilm-based antibiotic resistance and tolerance in pathogenic bacteria. FEMS Microbiol Rev 41: 276-301. https://doi.org/10.1093/femsre/fux010 ![]() |
[186] |
Zheng Z, Stewart PS (2004) Growth limitation of Staphylococcus epidermidis in biofilms contributes to rifampin tolerance. Biofilms 1: 31-35. https://doi.org/10.1017/S1479050503001042 ![]() |
[187] |
Mah TFC, O'Toole GA (2001) Mechanisms of biofilm resistance to antimicrobial agents. Trends Microbiol 9: 34-39. https://doi.org/10.1016/S0966-842X(00)01913-2 ![]() |
[188] |
Zhao X, Yu Z, Ding T (2020) Quorum-Sensing regulation of antimicrobial resistance in bacteria. Microorganisms 8: 425. https://doi.org/10.3390/microorganisms8030425 ![]() |
[189] |
Shih PC, Huang CT (2002) Effects of quorum-sensing deficiency on Pseudomonas aeruginosa biofilm formation and antibiotic resistance. J Antimicrob Chemother 49: 309-314. https://doi.org/10.1093/jac/49.2.309 ![]() |
[190] | Algburi A, Comito N, Kashtanov D, et al. (2017) Control of biofilm formation: Antibiotics and beyond. Appl Environ Microbiol 83: e02508-02516. https://doi.org/10.1128/AEM.02508-16 |
[191] |
Jiao Y, Tay FR, Niu Ln, et al. (2019) Advancing antimicrobial strategies for managing oral biofilm infections. Int J Oral Sci 11: 28. https://doi.org/10.1038/s41368-019-0062-1 ![]() |
[192] |
Klemm P, Vejborg RM, Hancock V (2010) Prevention of bacterial adhesion. Appl Microbiol Biotechnol 88: 451-459. https://doi.org/10.1007/s00253-010-2805-y ![]() |
[193] |
Veerachamy S, Yarlagadda T, Manivasagam G, et al. (2014) Bacterial adherence and biofilm formation on medical implants: A review. Proc Inst Mech Eng, Part H 228: 1083-1099. https://doi.org/10.1177/0954411914556137 ![]() |
[194] |
Chow JY, Yang Y, Tay SB, et al. (2014) Disruption of biofilm formation by the human pathogen Acinetobacter baumannii using engineered quorum-quenching lactonases. Antimicrob Agents Chemother 58: 1802-1805. https://doi.org/10.1128/AAC.02410-13 ![]() |
[195] |
Brown HL, Reuter M, Hanman K, et al. (2015) Prevention of biofilm formation and removal of existing biofilms by extracellular DNases of campylobacter jejuni. PLoS One 10: e0121680. https://doi.org/10.1371/journal.pone.0121680 ![]() |
[196] | Jiang Y, Geng M, Bai L (2020) Targeting biofilms therapy: Current research strategies and development hurdles. Microorganisms 8. https://doi.org/10.3390/microorganisms8081222 |
[197] |
Gil C, Solano C, Burgui S, et al. (2014) Biofilm matrix exoproteins induce a protective immune response against Staphylococcus aureus biofilm infection. Infect Immun 82: 1017-1029. https://doi.org/10.1128/IAI.01419-13 ![]() |
[198] | Jiang Q, Jin Z, Sun B (2018) MgrA negatively regulates biofilm formation and detachment by repressing the expression of psm operons in Staphylococcus aureus. Appl Environ Microbiol 84. https://doi.org/10.1128/aem.01008-18 |
[199] |
Fleming D, Rumbaugh KP (2017) Approaches to dispersing medical biofilms. Microorganisms 5: 15. https://doi.org/10.3390/microorganisms5020015 ![]() |
[200] |
Gallant CV, Daniels C, Leung JM, et al. (2005) Common beta-lactamases inhibit bacterial biofilm formation. Mol Microbiol 58: 1012-1024. https://doi.org/10.1111/j.1365-2958.2005.04892.x ![]() |
[201] |
Reza A, Sutton JM, Rahman KM (2019) Effectiveness of efflux pump inhibitors as biofilm disruptors and resistance breakers in Gram-Negative (ESKAPEE) bacteria. Antibiotics (Basel,Switz) 8: 229. https://doi.org/10.3390/antibiotics8040229 ![]() |
[202] |
Ciofu O, Rojo-Molinero E, Macià MD, et al. (2017) Antibiotic treatment of biofilm infections. APMIS 125: 304-319. https://doi.org/10.1111/apm.12673 ![]() |
[203] |
Brooun A, Liu S, Lewis K (2000) A dose-response study of antibiotic resistance in Pseudomonas aeruginosa biofilms. Antimicrob Agents Chemother 44: 640-646. https://doi.org/10.1128/aac.44.3.640-646.2000 ![]() |
[204] |
Danese PN (2002) Antibiofilm Approaches: Prevention of Catheter Colonization. Chem Biol 9: 873-880. https://doi.org/10.1016/S1074-5521(02)00192-8 ![]() |
[205] |
Flemming HC, Ridgway H (2009) Biofilm control: Conventional and alternative approaches. Marine and Industrial Biofouling . Heidelberg: Springer Berlin Heidelberg 103-117. https://doi.org/10.1007/978-3-540-69796-1_5 ![]() |
[206] |
Sambanthamoorthy K, Gokhale AA, Lao W, et al. (2011) Identification of a novel benzimidazole that inhibits bacterial biofilm formation in a broad-spectrum manner. Antimicrob Agents Chemother 55: 4369. https://doi.org/10.1128/AAC.00583-11 ![]() |
[207] | Dinicola S, De Grazia S, Carlomagno G, et al. (2014) N-acetylcysteine as powerful molecule to destroy bacterial biofilms. A systematic review. Eur Rev Med Pharmacol Sci 18: 2942-2948. Available from: https://europepmc.org/article/MED/25339490 |
[208] |
Abraham NM, Lamlertthon S, Fowler VG, et al. (2012) Chelating agents exert distinct effects on biofilm formation in Staphylococcus aureus depending on strain background: role for clumping factor B. J Med Microbiol 61: 1062-1070. https://doi.org/10.1099/jmm.0.040758-0 ![]() |
[209] |
Antoci V, Adams CS, Parvizi J, et al. (2008) The inhibition of Staphylococcus epidermidis biofilm formation by vancomycin-modified titanium alloy and implications for the treatment of periprosthetic infection. Biomaterials 29: 4684-4690. https://doi.org/10.1016/j.biomaterials.2008.08.016 ![]() |
[210] |
Ma Y, Chen M, Jones JE, et al. (2012) Inhibition of Staphylococcus epidermidis biofilm by trimethylsilane plasma coating. Antimicrob Agents Chemother 56: 5923-5937. https://doi.org/10.1128/aac.01739-12 ![]() |
[211] |
Sanyasi S, Majhi RK, Kumar S, et al. (2016) Polysaccharide-capped silver nanoparticles inhibit biofilm formation and eliminate multi-drug-resistant bacteria by disrupting bacterial cytoskeleton with reduced cytotoxicity towards mammalian cells. Sci Rep 6: 24929. https://doi.org/10.1038/srep24929 ![]() |
[212] |
AshaRani PV, Low Kah Mun G, Hande MP, et al. (2009) Cytotoxicity and genotoxicity of silver nanoparticles in human cells. ACS Nano 3: 279-290. https://doi.org/10.1021/nn800596w ![]() |
[213] |
Raie DS, Mhatre E, Thiele M, et al. (2017) Application of quercetin and its bio-inspired nanoparticles as anti-adhesive agents against Bacillus subtilis attachment to surface. Mater Sci Eng, C 70: 753-762. https://doi.org/10.1016/j.msec.2016.09.038 ![]() |
[214] |
Hume EB, Baveja J, Muir B, et al. (2004) The control of Staphylococcus epidermidis biofilm formation and in vivo infection rates by covalently bound furanones. Biomaterials 25: 5023-5030. https://doi.org/10.1016/j.biomaterials.2004.01.048 ![]() |
[215] |
Li H, Bao H, Bok KX, et al. (2016) High durability and low toxicity antimicrobial coatings fabricated by quaternary ammonium silane copolymers. Biomater Sci 4: 299-309. https://doi.org/10.1039/C5BM00353A ![]() |
[216] |
Trentin DS, Silva DB, Frasson AP, et al. (2015) Natural green coating inhibits adhesion of clinically important bacteria. Sci Rep 5: 8287. https://doi.org/10.1038/srep08287 ![]() |
[217] |
Chen M, Yu Q, Sun H (2013) Novel strategies for the prevention and treatment of biofilm related infections. Int J Mol Sci 14: 18488-18501. https://doi.org/10.3390/ijms140918488 ![]() |
[218] |
Tran PL, Lowry N, Campbell T, et al. (2012) An organoselenium compound inhibits Staphylococcus aureus biofilms on hemodialysis catheters in vivo. Antimicrob Agents Chemother 56: 972-978. https://doi.org/10.1128/aac.05680-11 ![]() |
[219] |
Zhao X, Zhao F, Wang J, et al. (2017) Biofilm formation and control strategies of foodborne pathogens: food safety perspectives. RSC Adv 7: 36670-36683. https://doi.org/10.1039/C7RA02497E ![]() |
[220] |
de Sousa DG, Harvey LA, Dorsch S, et al. (2018) Interventions involving repetitive practice improve strength after stroke: a systematic review. J Physiother 64: 210-221. https://doi.org/10.1016/j.jphys.2018.08.004 ![]() |
[221] |
Chappell TC, Nair NU (2020) Engineered lactobacilli display anti-biofilm and growth suppressing activities against Pseudomonas aeruginosa. npj Biofilms Microbiomes 6: 48. https://doi.org/10.1038/s41522-020-00156-6 ![]() |
[222] |
Lu L, Hu W, Tian Z, et al. (2019) Developing natural products as potential anti-biofilm agents. Chin Med 14: 11. https://doi.org/10.1186/s13020-019-0232-2 ![]() |
[223] |
Bendaoud M, Vinogradov E, Balashova NV, et al. (2011) Broad-spectrum biofilm inhibition by Kingella kingae exopolysaccharide. J Bacteriol Res 193: 3879-3886. https://doi.org/10.1128/JB.00311-11 ![]() |
[224] |
Mi L, Licina GA, Jiang S (2014) Nonantibiotic-Based Pseudomonas aeruginosa biofilm inhibition with osmoprotectant analogues. ACS Sustainable Chem Eng 2: 2448-2453. https://doi.org/10.1021/sc500468a ![]() |
[225] |
Kuang X, Chen V, Xu X (2018) Novel approaches to the control of oral microbial biofilms. BioMed Res Int 2018: 6498932. https://doi.org/10.1155/2018/6498932 ![]() |
[226] |
Chen H, Zhang B, Weir MD, et al. (2020) S. mutans gene-modification and antibacterial resin composite as dual strategy to suppress biofilm acid production and inhibit caries. J Dent 93: 103278. https://doi.org/10.1016/j.jdent.2020.103278 ![]() |
[227] |
Pires DP, Melo LDR, Vilas Boas D, et al. (2017) Phage therapy as an alternative or complementary strategy to prevent and control biofilm-related infections. Curr Opin Microbiol 39: 48-56. https://doi.org/10.1016/j.mib.2017.09.004 ![]() |
[228] |
Dickey J, Perrot V (2019) Adjunct phage treatment enhances the effectiveness of low antibiotic concentration against Staphylococcus aureus biofilms in vitro. PLoS One 14: e0209390. https://doi.org/10.1371/journal.pone.0209390 ![]() |
[229] |
Pires DP, Vilas Boas D, Sillankorva S, et al. (2015) Phage therapy: a step forward in the treatment of Pseudomonas aeruginosa infections. J Virol 89: 7449-7456. https://doi.org/10.1128/jvi.00385-15 ![]() |
[230] |
Fu W, Forster T, Mayer O, et al. (2010) Bacteriophage cocktail for the prevention of biofilm formation by Pseudomonas aeruginosa on catheters in an in vitro model system. Antimicrob Agents Chemother 54: 397-404. https://doi.org/10.1128/aac.00669-09 ![]() |
[231] |
Paluch E, Rewak-Soroczyńska J, Jędrusik I, et al. (2020) Prevention of biofilm formation by quorum quenching. Appl Microbiol Biotechnol 104: 1871-1881. https://doi.org/10.1007/s00253-020-10349-w ![]() |
[232] | Singh VK, Mishra A, Jha B (2017) Anti-quorum sensing and anti-biofilm activity of delftia tsuruhatensis extract by attenuating the quorum sensing-controlled virulence factor production in Pseudomonas aeruginosa. Front Cell Infect Microbiol 7. https://doi.org/10.3389/fcimb.2017.00337 |
[233] |
Brackman G, Defoirdt T, Miyamoto C, et al. (2008) Cinnamaldehyde and cinnamaldehyde derivatives reduce virulence in Vibrio spp. by decreasing the DNA-binding activity of the quorum sensing response regulator LuxR. BMC Microbiol 8: 149. https://doi.org/10.1186/1471-2180-8-149 ![]() |
[234] |
Kalaiarasan E, Thirumalaswamy K, Harish BN, et al. (2017) Inhibition of quorum sensing-controlled biofilm formation in Pseudomonas aeruginosa by quorum-sensing inhibitors. Microb Pathog 111: 99-107. https://doi.org/10.1016/j.micpath.2017.08.017 ![]() |
[235] | Bulman ZP, Ly NS, Lenhard JR, et al. (2017) Influence of rhlR and lasR on polymyxin pharmacodynamics in Pseudomonas aeruginosa and implications for quorum sensing inhibition with azithromycin. Antimicrob Agents Chemother 61. https://doi.org/10.1128/aac.00096-16 |
[236] |
Maura D, Rahme LG (2017) Pharmacological inhibition of the Pseudomonas aeruginosa MvfR quorum-sensing system interferes with biofilm formation and potentiates antibiotic-mediated biofilm disruption. Antimicrob Agents Chemother 61. https://doi.org/10.1128/aac.01362-17 ![]() |
[237] |
Stenvang M, Dueholm MS, Vad BS, et al. (2016) Epigallocatechin gallate remodels overexpressed functional amyloids in Pseudomonas aeruginosa and increases biofilm susceptibility to antibiotic treatment*. J Biol Chem 291: 26540-26553. https://doi.org/10.1074/jbc.M116.739953 ![]() |
[238] |
Guo Q, Wei Y, Xia B, et al. (2016) Identification of a small molecule that simultaneously suppresses virulence and antibiotic resistance of Pseudomonas aeruginosa. Sci Rep 6: 19141. https://doi.org/10.1038/srep19141 ![]() |
[239] |
Freire MO, Devaraj A, Young A, et al. (2017) A bacterial-biofilm-induced oral osteolytic infection can be successfully treated by immuno-targeting an extracellular nucleoid-associated protein. Mol Oral Microbiol 32: 74-88. https://doi.org/10.1111/omi.12155 ![]() |
[240] | Sun D, Accavitti MA, Bryers JD (2005) Inhibition of biofilm formation by monoclonal antibodies against Staphylococcus epidermidis RP62A accumulation-associated protein. Clin Diagn Lab Immunol 12: 93-100. https://doi.org/10.1128/CDLI.12.1.93-100.2005 |
[241] |
Estellés A, Woischnig AK, Liu K, et al. (2016) A high-affinity native human antibody disrupts biofilm from Staphylococcus aureus bacteria and potentiates antibiotic efficacy in a mouse implant infection model. Antimicrob Agents Chemother 60: 2292-2301. https://doi.org/10.1128/aac.02588-15 ![]() |
[242] |
Hall AE, Domanski PJ, Patel PR, et al. (2003) Characterization of a protective monoclonal antibody recognizing Staphylococcus aureus MSCRAMM protein clumping factor A. Infect Immun 71: 6864-6870. https://doi.org/10.1128/iai.71.12.6864-6870.2003 ![]() |
[243] |
Visai L, Xu Y, Casolini F, et al. (2000) Monoclonal antibodies to CNA, a collagen-binding microbial surface component recognizing adhesive matrix molecules, detach Staphylococcus aureus from a collagen substrate. J Biol Chem 275: 39837-39845. https://doi.org/10.1074/jbc.M005297200 ![]() |
[244] |
Rennermalm A, Li YH, Bohaufs L, et al. (2001) Antibodies against a truncated Staphylococcus aureus fibronectin-binding protein protect against dissemination of infection in the rat. Vaccine 19: 3376-3383. https://doi.org/10.1016/s0264-410x(01)00080-9 ![]() |
[245] |
Belyi Y, Rybolovlev I, Polyakov N, et al. (2018) Staphylococcus aureus surface protein G is an immunodominant protein and a possible target in an anti-biofilm drug development. Open Microbiol J 12: 94-106. https://doi.org/10.2174/1874285801812010094 ![]() |
[246] |
Haghighat S, Siadat SD, Sorkhabadi SM, et al. (2017) Cloning, expression and purification of autolysin from methicillin-resistant Staphylococcus aureus: potency and challenge study in Balb/c mice. Mol Immunol 82: 10-18. https://doi.org/10.1016/j.molimm.2016.12.013 ![]() |
[247] |
Nair N, Vinod V, Suresh MK, et al. (2015) Amidase, a cell wall hydrolase, elicits protective immunity against Staphylococcus aureus and S. epidermidis. Int J Biol Macromol 77: 314-321. https://doi.org/10.1016/j.ijbiomac.2015.03.047 ![]() |
[248] |
Varrone JJ, de Mesy Bentley KL, Bello-Irizarry SN, et al. (2014) Passive immunization with anti-glucosaminidase monoclonal antibodies protects mice from implant-associated osteomyelitis by mediating opsonophagocytosis of Staphylococcus aureus megaclusters. J Orthop Res 32: 1389-1396. https://doi.org/10.1002/jor.22672 ![]() |
[249] |
van den Berg S, Bonarius HP, van Kessel KP, et al. (2015) A human monoclonal antibody targeting the conserved Staphylococcal antigen IsaA protects mice against Staphylococcus aureus bacteremia. Int J Med Microbiol 305: 55-64. https://doi.org/10.1016/j.ijmm.2014.11.002 ![]() |
[250] |
Liu B, Park S, Thompson CD, et al. (2017) Antibodies to Staphylococcus aureus capsular polysaccharides 5 and 8 perform similarly in vitro but are functionally distinct in vivo. Virulence 8: 859-874. https://doi.org/10.1080/21505594.2016.1270494 ![]() |
[251] |
Weisman LE (2007) Antibody for the prevention of neonatal noscocomial Staphylococcal infection: a review of the literature. Arch Pediatr 14 Suppl 1: S31-34. https://doi.org/10.1016/s0929-693x(07)80008-x ![]() |
[252] |
Raafat D, Otto M, Reppschläger K, et al. (2019) Fighting Staphylococcus aureus biofilms with monoclonal antibodies. Trends Microbiol 27: 303-322. https://doi.org/10.1016/j.tim.2018.12.009 ![]() |
[253] |
Freire-Moran L, Aronsson B, Manz C, et al. (2011) Critical shortage of new antibiotics in development against multidrug-resistant bacteria-time to react is now. Drug Resist Updat 14: 118-124. https://doi.org/10.1016/j.drup.2011.02.003 ![]() |
[254] |
Banerjee M, Moulick S, Bhattacharya KK, et al. (2017) Attenuation of Pseudomonas aeruginosa quorum sensing, virulence and biofilm formation by extracts of Andrographis paniculata. Microb Pathog 113: 85-93. https://doi.org/10.1016/j.micpath.2017.10.023 ![]() |
[255] |
Zhang L, Bao M, Liu B, et al. (2020) Effect of andrographolide and its analogs on bacterial infection: A review. Pharmacology 105: 123-134. https://doi.org/10.1159/000503410 ![]() |
[256] |
Rasool U, SP, Parveen A, et al. (2018) Efficacy of andrographis paniculata against extended spectrum β-lactamase (ESBL) producing E. coli. BMC Complementary Altern Med 18: 244-244. https://doi.org/10.1186/s12906-018-2312-8 ![]() |
[257] |
Carrol DH, Chassagne F, Dettweiler M, et al. (2020) Antibacterial activity of plant species used for oral health against Porphyromonas gingivalis. PLoS One 15: e0239316. https://doi.org/10.1371/journal.pone.0239316 ![]() |
[258] |
Gerits E, Verstraeten N, Michiels J (2017) New approaches to combat Porphyromonas gingivalis biofilms. J Oral Microbiol 9: 1300366. https://doi.org/10.1080/20002297.2017.1300366 ![]() |
[259] |
Liu G, Xiang H, Tang X, et al. (2011) Transcriptional and functional analysis shows sodium houttuyfonate-mediated inhibition of autolysis in Staphylococcus aureus. Molecules (Basel,Switz) 16: 8848-8865. https://doi.org/10.3390/molecules16108848 ![]() |
[260] |
Zhou J, Bi S, Chen H, et al. (2017) Anti-Biofilm and antivirulence activities of metabolites from plectosphaerella cucumerina against Pseudomonas aeruginosa. Front Microbiol 8: 769. https://doi.org/10.3389/fmicb.2017.00769 ![]() |
[261] |
Rabin N, Zheng Y, Opoku-Temeng C, et al. (2015) Agents that inhibit bacterial biofilm formation. Future Med Chem 7: 647-671. https://doi.org/10.4155/fmc.15.7 ![]() |
[262] |
Xiang H, Cao F, Ming D, et al. (2017) Aloe-emodin inhibits Staphylococcus aureus biofilms and extracellular protein production at the initial adhesion stage of biofilm development. Appl Microbiol Biotechnol 101: 6671-6681. https://doi.org/10.1007/s00253-017-8403-5 ![]() |
[263] |
Jakobsen TH, van Gennip M, Phipps RK, et al. (2012) Ajoene, a sulfur-rich molecule from garlic, inhibits genes controlled by quorum sensing. Antimicrob Agents Chemother 56: 2314-2325. https://doi.org/10.1128/AAC.05919-11 ![]() |
[264] |
Balcázar JL, Vendrell D, de Blas I, et al. (2008) Characterization of probiotic properties of lactic acid bacteria isolated from intestinal microbiota of fish. Aquaculture 278: 188-191. https://doi.org/10.1016/j.aquaculture.2008.03.014 ![]() |
[265] |
Didinen BI, Onuk EE, Metin S, et al. (2018) Identification and characterization of lactic acid bacteria isolated from rainbow trout (Oncorhynchus mykiss, Walbaum 1792), with inhibitory activity against Vagococcus salmoninarum and Lactococcus garvieae. Aquacult Nutr 24: 400-407. https://doi.org/10.1111/anu.12571 ![]() |
[266] |
Ben Taheur F, Kouidhi B, Fdhila K, et al. (2016) Anti-bacterial and anti-biofilm activity of probiotic bacteria against oral pathogens. Microb Pathog 97: 213-220. https://doi.org/10.1016/j.micpath.2016.06.018 ![]() |
[267] |
Lee DK, Park SY, An HM, et al. (2011) Antimicrobial activity of Bifidobacterium spp. isolated from healthy adult Koreans against cariogenic microflora. Arch Oral Biol 56: 1047-1054. https://doi.org/10.1016/j.archoralbio.2011.03.002 ![]() |
[268] |
Schwendicke F, Horb K, Kneist S, et al. (2014) Effects of heat-inactivated Bifidobacterium BB12 on cariogenicity of Streptococcus mutans in vitro. Arch Oral Biol 59: 1384-1390. https://doi.org/10.1016/j.archoralbio.2014.08.012 ![]() |
[269] |
Suzuki N, Yoneda M, Hatano Y, et al. (2011) Enterococcus faecium WB2000 inhibits biofilm formation by oral cariogenic Streptococci. Int J Dent 2011: 834151. https://doi.org/10.1155/2011/834151 ![]() |
[270] |
Sassone-Corsi M, Raffatellu M (2015) No vacancy: how beneficial microbes cooperate with immunity to provide colonization resistance to pathogens. J immunol 194: 4081-4087. https://doi.org/10.4049/jimmunol.1403169 ![]() |
[271] |
Tuomola EM, Ouwehand AC, Salminen SJ (1999) The effect of probiotic bacteria on the adhesion of pathogens to human intestinal mucus. FEMS Immunol Med Microbiol 26: 137-142. https://doi.org/10.1111/j.1574-695X.1999.tb01381.x ![]() |
[272] |
Yan F, Polk DB (2011) Probiotics and immune health. Curr Opin Gastroenterol 27: 496-501. https://doi.org/10.1097/MOG.0b013e32834baa4d ![]() |
[273] |
Hegarty JW, Guinane CM, Ross RP, et al. (2016) Bacteriocin production: a relatively unharnessed probiotic trait?. F1000Res 5: 2587. https://doi.org/10.12688/f1000research.9615.1 ![]() |
[274] |
Corcoran BM, Stanton C, Fitzgerald GF, et al. (2005) Survival of probiotic lactobacilli in acidic environments is enhanced in the presence of metabolizable sugars. Appl Environ Microbiol 71: 3060-3067. https://doi.org/10.1128/AEM.71.6.3060-3067.2005 ![]() |
[275] |
Vuotto C, Longo F, Donelli G (2014) Probiotics to counteract biofilm-associated infections: promising and conflicting data. Int J Oral Sci 6: 189-194. https://doi.org/10.1038/ijos.2014.52 ![]() |
[276] |
Fang K, Jin X, Hong SH (2018) Probiotic Escherichia coli inhibits biofilm formation of pathogenic E. coli via extracellular activity of DegP. Sci Rep 8: 4939. https://doi.org/10.1038/s41598-018-23180-1 ![]() |
[277] |
Jaffar N, Ishikawa Y, Mizuno K, et al. (2016) Mature biofilm degradation by potential probiotics: Aggregatibacter actinomycetemcomitans versus LactoBacillus spp. PLoS One 11: e0159466. https://doi.org/10.1371/journal.pone.0159466 ![]() |
[278] |
Mathur H, Field D, Rea MC, et al. (2018) Fighting biofilms with lantibiotics and other groups of bacteriocins. npj Biofilms Microbiomes 4: 9. https://doi.org/10.1038/s41522-018-0053-6 ![]() |
[279] |
Hertzberger R, Arents J, Dekker Henk L, et al. (2014) H2O2 production in species of the LactoBacillus acidophilus group: a central role for a novel NADH-Dependent flavin reductase. Appl Environ Microbiol 80: 2229-2239. https://doi.org/10.1128/AEM.04272-13 ![]() |
[280] |
Salas-Jara MJ, Ilabaca A, Vega M, et al. (2016) Biofilm forming LactoBacillus: New challenges for the development of probiotics. Microorganisms 4: 35. https://doi.org/10.3390/microorganisms4030035 ![]() |
[281] |
Jones SE, Versalovic J (2009) Probiotic LactoBacillus reuteribiofilms produce antimicrobial and anti-inflammatory factors. BMC Microbiol 9: 35. https://doi.org/10.1186/1471-2180-9-35 ![]() |
[282] |
Barzegari A, Kheyrolahzadeh K, Hosseiniyan Khatibi SM, et al. (2020) The battle of probiotics and their derivatives against biofilms. Infect Drug Resist 13: 659-672. https://doi.org/10.2147/IDR.S232982 ![]() |
[283] |
Miquel S, Lagrafeuille R, Souweine B, et al. (2016) Anti-biofilm activity as a health issue. Front Microbiol 7: 592-592. https://doi.org/10.3389/fmicb.2016.00592 ![]() |
[284] |
Tan L, Fu J, Feng F, et al. (2020) Engineered probiotics biofilm enhances osseointegration via immunoregulation and anti-infection. Sci Adv 6: eaba5723. https://doi.org/10.1126/sciadv.aba5723 ![]() |
[285] |
Carvalho FM, Teixeira-Santos R, Mergulhão FJM, et al. (2020) The use of probiotics to fight biofilms in medical devices: A systematic review and meta-analysis. Microorganisms 9. https://doi.org/10.3390/microorganisms9010027 ![]() |
[286] |
Gholizadeh P, Aghazadeh M, Asgharzadeh M, et al. (2017) Suppressing the CRISPR/Cas adaptive immune system in bacterial infections. Eur J Clin Microbiol Infect Dis 36: 2043-2051. https://doi.org/10.1007/s10096-017-3036-2 ![]() |
[287] |
Yao R, Liu D, Jia X, et al. (2018) CRISPR-Cas9/Cas12a biotechnology and application in bacteria. Synth Syst Biotechnol 3: 135-149. https://doi.org/10.1016/j.synbio.2018.09.004 ![]() |
[288] |
Jiang W, Bikard D, Cox D, et al. (2013) RNA-guided editing of bacterial genomes using CRISPR-Cas systems. Nat Biotechnol 31: 233-239. https://doi.org/10.1038/nbt.2508 ![]() |
[289] |
Goren M, Yosef I, Qimron U (2017) Sensitizing pathogens to antibiotics using the CRISPR-Cas system. Drug Resist Updat 30: 1-6. https://doi.org/10.1016/j.drup.2016.11.001 ![]() |
[290] |
Touchon M, Charpentier S, Pognard D, et al. (2012) Antibiotic resistance plasmids spread among natural isolates of Escherichia coli in spite of CRISPR elements. Microbiology (Reading) 158: 2997-3004. https://doi.org/10.1099/mic.0.060814-0 ![]() |
[291] |
Hale CR, Majumdar S, Elmore J, et al. (2012) Essential features and rational design of CRISPR RNAs that function with the Cas RAMP module complex to cleave RNAs. Mol Cell 45: 292-302. https://doi.org/10.1016/j.molcel.2011.10.023 ![]() |
[292] |
Bikard D, Euler CW, Jiang W, et al. (2014) Exploiting CRISPR-Cas nucleases to produce sequence-specific antimicrobials. Nat Biotechnol 32: 1146-1150. https://doi.org/10.1038/nbt.3043 ![]() |
[293] |
Vercoe RB, Chang JT, Dy RL, et al. (2013) Cytotoxic chromosomal targeting by CRISPR/Cas systems can reshape bacterial genomes and expel or remodel pathogenicity islands. PLoS Genet 9: e1003454. https://doi.org/10.1371/journal.pgen.1003454 ![]() |
[294] |
Gholizadeh P, Köse Ş, Dao S, et al. (2020) How CRISPR-Cas system could be used to combat antimicrobial resistance. Infect Drug Resist 13: 1111-1121. https://doi.org/10.2147/IDR.S247271 ![]() |
[295] |
Zuberi A, Misba L, Khan AU (2017) CRISPR interference (CRISPRi) inhibition of luxS gene expression in E. coli: An approach to inhibit biofilm. Front Cell Infect Microbiol 7. https://doi.org/10.3389/fcimb.2017.00214 ![]() |
[296] |
Gong T, Tang B, Zhou X, et al. (2018) Genome editing in Streptococcus mutans through self-targeting CRISPR arrays. Mol Oral Microbiol 33: 440-449. https://doi.org/10.1111/omi.12247 ![]() |
[297] |
Garrido V, Piñero-Lambea C, Rodriguez-Arce I, et al. (2021) Engineering a genome-reduced bacterium to eliminate Staphylococcus aureus biofilms in vivo. Mol Syst Biol 17: e10145. https://doi.org/10.15252/msb.202010145 ![]() |
1. | Mirinal Kapri, Tushar Dimri, Pushkar Singh, Anurag Maletha, Dibyahash Bordoloi, Manisha Aeri, 2024, Quantitative Insights into Breakout and Reversal Trading Strategies: A Comprehensive Analysis for Algorithmic Trading in Financial Markets, 979-8-3503-7105-5, 524, 10.1109/ICDT61202.2024.10488993 | |
2. | Om Mengshetti, Kanishk Gupta, Nilima Zade, Ketan Kotecha, Siddhanth Mutha, Gayatri Joshi, Synergizing quantitative finance models and market microstructure analysis for enhanced algorithmic trading strategies, 2024, 10, 21998531, 100334, 10.1016/j.joitmc.2024.100334 | |
3. | Ebenezer Fiifi Emire Atta Mills, Yuexin Liao, Zihui Deng, Data-Driven Price Trends Prediction of Ethereum: A Hybrid Machine Learning and Signal Processing Approach, 2024, 20967209, 100231, 10.1016/j.bcra.2024.100231 | |
4. | Saurabh Singh, Anil Pise, Byungun Yoon, Prediction of bitcoin stock price using feature subset optimization, 2024, 10, 24058440, e28415, 10.1016/j.heliyon.2024.e28415 | |
5. | Yu. V. Bezsmolnyi, M. M. Seniv, A decision support software system for cryptocurrency traders on the Trading View platform, 2024, 6, 27071898, 9, 10.23939/ujit2024.01.009 | |
6. | Gil Cohen, Avishay Aiche, Intelligent forecasting in bitcoin markets, 2025, 71, 15446123, 106487, 10.1016/j.frl.2024.106487 |
Method | Primal bilinear form |
Primal-Primal (PP) | |
Mixed-Primal (MP) | |
Primal-Mixed (PM) | |
Mixed-Mixed (MM) |