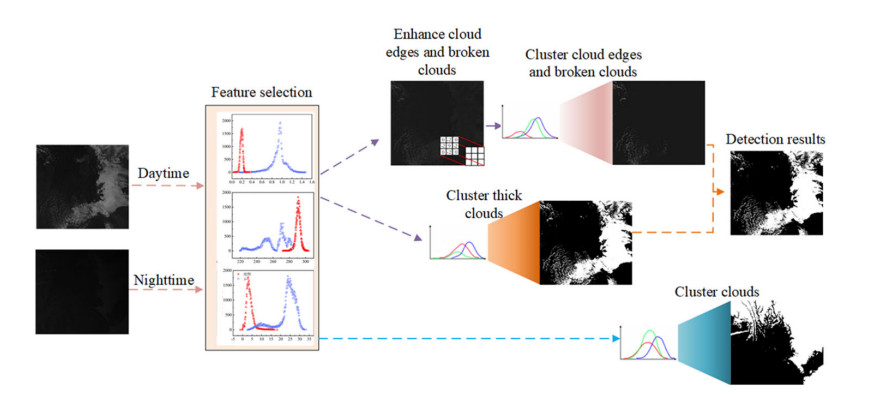
This article reports known and emerging adverse health effects associated with the administration of gadolinium-based contrast agents. It focuses on the issue of the incomplete excretion of these drugs leading to the deposition of gadolinium in the tissues of the patients. The evidence of deposition is reviewed. The analysis presents gaps in our knowledge but also suggests neglected or still poorly considered parameters to possibly explain discrepancies among studies (e.g. off-label use; rate of administration; gadolinium concentration in the pharmaceutical formulation, cumulative metal toxicity). The article also presents a critical assessment of some aspects reported in the literature as well as future needs. Potential biases in the investigation and evaluation of the health/clinical implications associated with gadolinium deposition are pointed out. The analysis emphasizes that the vast majority of the clinical studies conducted up to date on gadolinium-based contrast agents were designed to assess acute toxicity and diagnostic efficacy of the agents, not to identify long-term health effects.
Citation: Silvia Maria Lattanzio. Toxicity associated with gadolinium-based contrast-enhanced examinations[J]. AIMS Biophysics, 2021, 8(2): 198-220. doi: 10.3934/biophy.2021015
[1] | Jinyun Jiang, Jianchen Cai, Qile Zhang, Kun Lan, Xiaoliang Jiang, Jun Wu . Group theoretic particle swarm optimization for gray-level medical image enhancement. Mathematical Biosciences and Engineering, 2023, 20(6): 10479-10494. doi: 10.3934/mbe.2023462 |
[2] | Kunpeng Li, Zepeng Wang, Yu Zhou, Sihai Li . Lung adenocarcinoma identification based on hybrid feature selections and attentional convolutional neural networks. Mathematical Biosciences and Engineering, 2024, 21(2): 2991-3015. doi: 10.3934/mbe.2024133 |
[3] | Jun Gao, Qian Jiang, Bo Zhou, Daozheng Chen . Convolutional neural networks for computer-aided detection or diagnosis in medical image analysis: An overview. Mathematical Biosciences and Engineering, 2019, 16(6): 6536-6561. doi: 10.3934/mbe.2019326 |
[4] | Yufeng Li, Chengcheng Liu, Weiping Zhao, Yufeng Huang . Multi-spectral remote sensing images feature coverage classification based on improved convolutional neural network. Mathematical Biosciences and Engineering, 2020, 17(5): 4443-4456. doi: 10.3934/mbe.2020245 |
[5] | Hong Yu, Wenhuan Lu, Qilong Sun, Haiqiang Shi, Jianguo Wei, Zhe Wang, Xiaoman Wang, Naixue Xiong . Design and analysis of a robust breast cancer diagnostic system based on multimode MR images. Mathematical Biosciences and Engineering, 2021, 18(4): 3578-3597. doi: 10.3934/mbe.2021180 |
[6] | Binju Saju, Neethu Tressa, Rajesh Kumar Dhanaraj, Sumegh Tharewal, Jincy Chundamannil Mathew, Danilo Pelusi . Effective multi-class lungdisease classification using the hybridfeature engineering mechanism. Mathematical Biosciences and Engineering, 2023, 20(11): 20245-20273. doi: 10.3934/mbe.2023896 |
[7] | Sushovan Chaudhury, Kartik Sau, Muhammad Attique Khan, Mohammad Shabaz . Deep transfer learning for IDC breast cancer detection using fast AI technique and Sqeezenet architecture. Mathematical Biosciences and Engineering, 2023, 20(6): 10404-10427. doi: 10.3934/mbe.2023457 |
[8] | S. Dinesh Krishnan, Danilo Pelusi, A. Daniel, V. Suresh, Balamurugan Balusamy . Improved graph neural network-based green anaconda optimization for segmenting and classifying the lung cancer. Mathematical Biosciences and Engineering, 2023, 20(9): 17138-17157. doi: 10.3934/mbe.2023764 |
[9] | Yingjian Yang, Wei Li, Yingwei Guo, Nanrong Zeng, Shicong Wang, Ziran Chen, Yang Liu, Huai Chen, Wenxin Duan, Xian Li, Wei Zhao, Rongchang Chen, Yan Kang . Lung radiomics features for characterizing and classifying COPD stage based on feature combination strategy and multi-layer perceptron classifier. Mathematical Biosciences and Engineering, 2022, 19(8): 7826-7855. doi: 10.3934/mbe.2022366 |
[10] | Haiyan Song, Cuihong Liu, Shengnan Li, Peixiao Zhang . TS-GCN: A novel tumor segmentation method integrating transformer and GCN. Mathematical Biosciences and Engineering, 2023, 20(10): 18173-18190. doi: 10.3934/mbe.2023807 |
This article reports known and emerging adverse health effects associated with the administration of gadolinium-based contrast agents. It focuses on the issue of the incomplete excretion of these drugs leading to the deposition of gadolinium in the tissues of the patients. The evidence of deposition is reviewed. The analysis presents gaps in our knowledge but also suggests neglected or still poorly considered parameters to possibly explain discrepancies among studies (e.g. off-label use; rate of administration; gadolinium concentration in the pharmaceutical formulation, cumulative metal toxicity). The article also presents a critical assessment of some aspects reported in the literature as well as future needs. Potential biases in the investigation and evaluation of the health/clinical implications associated with gadolinium deposition are pointed out. The analysis emphasizes that the vast majority of the clinical studies conducted up to date on gadolinium-based contrast agents were designed to assess acute toxicity and diagnostic efficacy of the agents, not to identify long-term health effects.
In recent years, remote sensing (RS) imagery has been widely applied in various fields, with the continuous development of RS technology. The fields include environmental monitoring [1], natural disaster monitoring [2,3,4], mineral resource development [5] and cartography [6]. However, a large part of Earth's surface is covered by clouds [7], which results in a significant amount of ground information being inaccessible to satellites. Additionally, the texture and spectral information of images are inconstant. There are substantial challenges in subsequent object detection and analysis tasks. Therefore, accurate and effective cloud detection is crucial due to being a preprocessing step for getting over the challenges before the analysis of satellite images [8].
Currently, cloud detection methods can be categorized into multi-spectral threshold methods and machine learning-based methods. Multi-spectral threshold methods use spectral differences between clouds and the underlying surface in different wavelength bands to select thresholds for cloud detection. Rossow et al. used spectral thresholding based on the 11 μm brightness temperature data of cloud tops in the international satellite cloud climatology project [9]. Xiong et al. proposed a cloud detection approach based on hybrid multi-spectral features with dynamic thresholds [10]. The multi-spectral threshold methods have a simple structure and are easy to implement. However, it is challenging for them to distinguish between clouds and the underlying surface for them when the ground is covered with ice, snow, desert or a cloud cover that is thin.
Many cloud detection algorithms based on machine learning have emerged with the rapid development of computer science, including the support vector machine (SVM) [11,12,13], random forest (RF) [14,15], convolutional neural network (CNN) [16,17] and others. Li et al. [11] utilized brightness temperature and texture features, employing SVM as a classifier for cloud detection. Zhang et al. proposed a cloud detection method based on the multi-feature embedded learning SVM [12]. Fu et al. used the results of the ensemble threshold as training samples for the RF classifier [15]. Zhang et al. designed a cascaded feature attention module based on the encoder-decoder structure to obtain and enhance useful feature information [16]. Xie et al. proposed a CNN with two branches to detect different types of clouds [17]. However, all the machine learning methods used by them encounter the following challenges:
(1) Cloud edges are often semi-transparent, making it difficult for them to separate the underlying surface based solely on visual features. Therefore, it is essential to make full use of the multi-channel information from RS satellites.
(2) These machine learning methods require a sufficient amount of training data to achieve reliable performance [18]. However, fragmented cloud samples are often sparse and scattered, demanding a significant amount of effort and time for manual labeling.
The GMM, as an unsupervised learning method, does not require manual sample labeling. Therefore, it requires less preparation work before detection [19]. Furthermore, visual features can have difficulty in distinguishing between cloud edges and the underlying surface during manual labeling, and incorrect labeling may result in model inaccuracies. In contrast, the GMM algorithm use satellite multi-channel reflectance and brightness temperature as clustering features and can effectively leverage the spectral differences between clouds and the underlying surface for classification.
In summary, we propose a cloud detection algorithm based on a feature enhancement module and GMM clustering. The proposed method utilizes clustering-based feature analysis to select appropriate feature schemes for different time periods, enabling cloud detection throughout the day. Additionally, we employ the Laplacian operator to enhance the spectral characteristics of the cloud edges and broken cloud regions and improve the accuracy of the GMM classifier for cloud detection.
The paper is structured as follows: Section 2 presents the various applications of cloud detection. Section 3 discusses data preprocessing, with a focus on the selection of clustering features. Section 4 presents a modular description of the proposed algorithm, highlighting its key components and functionalities. Section 5 provides a comprehensive account of all experiments conducted and their corresponding observations, shedding light on the algorithm's performance and efficacy. Section 6 discusses the ethical considerations and potential societal impacts of enhanced cloud detection. Finally, Section 7 offers a summary of the paper, summarizing the major contributions.
(1) Wildfire detection
When utilizing satellite data for wildfire monitoring, clouds' mid-infrared reflectance might cause them to be mistakenly identified as fire. Hence, cloud detection is crucial. Zhang et al. set different thresholds during daytime and nighttime to detect clouds [20]. Kang et al. employed an algorithm utilizing Imager's reflectance and BT to eliminate clouds in aerosol optical depth retrieval [21].
(2) Cartography in agriculture
To reduce spectral redundancy in images and improve method efficiency, cloud detection is often a crucial step in data preprocessing during agricultural mapping. Xia et al. utilized cloud masks generated by the C Function of Mask algorithm [22]. Li et al. calculated cloud scores according to the spectral data from six bands to achieve cloud removal [23].
(3) Vegetation change analysis
Vegetation change analysis is particularly sensitive to cloud contamination because unmarked clouds above the vegetation can potentially be mistaken for change. Even minor errors in cloud detection can lead to significant inaccuracies in downstream vegetation change analysis. Huang et al. used clear view forest pixels as references to define cloud boundaries, separating clouds from the clear view surface in the spectral-temperature space [24]. Long et al. employed cloud identification algorithms provided by Google Earth Engine, utilizing the QA band to identify clouds and shadows in the images [25].
The cloud detection algorithm in this paper is based on multi-spectral data from the Himawari-8 satellite. As shown in Figure 1, the first step involves conducting a statistical analysis using probability density functions (PDFs) of pixel spectral data from clouds and underlying surfaces to select feature schemes for different time periods. The second step involves cloud detection based on the different feature schemes selected. During nighttime, the raw data of BT15 and BTD7−14 are directly subjected to GMM clustering to obtain the detection results. During the daytime, the detection process is divided into three parts. First, a feature enhancement module is applied to R3+4, and the enhanced data is clustered to detect cloud edges and broken clouds. Furthermore, the raw data is clustered to detect thick clouds. Finally, the results from the dual branches of clustering are combined to obtain the detection results.
The definition of clouds is crucial, and many scholars currently use a subjective approach to define different types of clouds. In this paper, cloud transparency and distribution are used as criteria for subjective definition.
(1) Thick clouds are opaque and typically appear over large areas.
(2) Cloud edges refer to clouds with transparency at the cloud boundaries. Cloud edges do not abruptly separate but gradually transition between the cloud layer and the non-cloud layer.
(3) Broken clouds exhibit varying degrees of transparency, with their most noticeable characteristic being their distribution in small patches across the sky.
Figure 2 illustrates various cloud types, with some cloud edges highlighted using red boxes.
The synchronous meteorological satellite Himawari-8 was officially launched in July 2015. The satellite is equipped with the advanced Himawari imager (AHI), which has 16 observation channels covering visible, near-infrared and thermal infrared ranges [26]. The central wavelengths of these channels for detecting various objects are shown in Table 1. Additionally, AHI can provide high-resolution observation data for the entire disk at intervals of 10 minutes, and its high temporal resolution data is advantageous for target detection and analysis [27]. The open accessibility of Himawari-8/AHI data, provided by the Japan Aerospace Exploration Agency, empowers researchers to extensively leverage this data for scientific inquiry and innovation. This ease of access and the widespread availability of this data have propelled its applications across the fields of Earth sciences, meteorology and environmental studies. For instance, it plays a pivotal role in monitoring natural disasters such as storm tracking, wildfire detection and climate change observations. Moreover, these datasets offer rich information for fields like agriculture, water resource management and urban planning.
Channel | Central wavelength/μm | Detection targets |
1 | 0.46 | aerosol, coastline |
2 | 0.51 | phytoplankton |
3 | 0.64 | vegetation, aerosol |
4 | 0.86 | stratus cloud |
5 | 1.6 | cloud top phase, snow |
6 | 2.3 | surface, cloud, snow |
7 | 3.9 | surface, wildfire |
8 | 6.2 | upper atmospheric water vapor, rainfall |
9 | 7.0 | mid-level atmospheric water vapor, rainfall |
10 | 7.3 | sulfur dioxide |
11 | 8.6 | water, sulfur dioxide, rainfall |
12 | 9.6 | ozone, airflow, wind |
13 | 10.4 | surface, cloud |
14 | 11.2 | surface, cloud |
15 | 12.4 | water, sea surface temperature |
16 | 13.3 | temperature, cloud altitude, cloudiness |
As shown in Figure 3, the data retrieved from the Himawari-8 satellite comes in the network common data form (NetCDF) format, requiring preprocessing before utilization. Initially, the NetCDF format will undergo conversion into physical variables. Channels 1 to 6 will denote reflectance data, while channels 7 to 16 will contain brightness temperature data. Following this, a sequence of geometric corrections will be applied, opting for the Geographic WGS84 Projection, succeeded by cropping procedures.
Moreover, different features may have varying measurement units or numerical ranges. Normalization can alleviate these effects, enabling the model to treat each feature more evenly. The normalization formula is as follows:
Y=X−XmeanXstd | (1) |
Where the mean and standard deviation of X are indicated by Xmean and Xstd, respectively. X and Y represent the initial and the normalized feature values, respectively.
The spectral data from the Himawari-8 satellite is analyzed using PDF to generate PDF distributions for reflectance, brightness temperature and brightness temperature difference of pixels corresponding to both clouds and the underlying surface. The selection of cluster features is completed based on the analysis of these PDF distributions. As shown in Figure 4, a random sample of cloud and underlying surface pixels from a series of RS images is used for statistical analysis.
Figure 5 shows the PDF distributions of different cluster features for cloud and underlying surface pixels. The PDF distributions for three cluster features, R3+4, BT15 and BTD7−14, exhibit some overlap for both cloud and underlying surface pixels. As shown in Figure 5(a), the PDF distribution of R3+4 for both cloud and underlying surface pixels roughly follows a normal distribution. Cloud PDF mainly ranges from 0.8 to 1.3, with a peak around 1.2, while the underlying surface PDF predominantly ranges from 0.2 to 0.35, with a peak around 0.3.
Figure 5(b) displays the PDF distribution of BT15 for clouds and underlying surface pixels. Cloud BT15 PDF is mainly distributed between 220 K and 285 K, displaying two peaks near 250 K and 270 K, respectively. In contrast, the underlying surface BT15 PDF mainly ranges from 280 K to 300 K, with a peak of around 290 K.
Figure 5(c) demonstrates that the PDF distributions of BTD7−14 for both cloud and underlying surface pixels also roughly follow a normal distribution. Cloud BTD7−14 PDF mainly ranges from 20 K to 30 K, with a peak around 23 K, while the underlying surface BTD7−14 PDF mainly ranges from 0 K to 7 K, with a peak around 2 K.
Although there are significant differences in the R3+4 PDF distributions between clouds and the underlying surface, during nighttime periods, the visible light band of AHI struggles to capture clear visible light images. Therefore, in this work, we use different cluster feature schemes for cloud detection during day and night periods: R3+4 during the day and BT15 and BTD7−14 at night.
During the process of cloud detection, cloud edges (the overlapping parts of PDFs for various cluster features in Figure 5) are prone to being misclassified as non-cloud pixels. This is primarily due to the minimal spectral feature differences between cloud edges and the underlying surface. Additionally, broken clouds are often sparse and scattered, causing their detection to be challenging. Therefore, as shown in Figure 6, to enhance the algorithm's efficiency in detecting cloud edges and broken clouds, convolution operations are employed to enhance the spectral features of cloud edges and broken clouds. The convolution operation involves sliding the sharpening convolution kernel over each pixel of the original image and computing the weighted sum of the convolution kernel with the pixel values. The formula for this enhancement is as follows:
g(x,y)=f(x,y)∗w(i,j) | (2) |
In the equation, f(x, y) represents the pixel value at (x, y), and w(i, j) represents the convolution kernel.
The Laplacian operator, often used in image processing, plays a crucial role in extracting gradient information from images [28,29]. It accentuates abrupt changes in pixel values, making it particularly useful in highlighting boundaries and fine details, such as edges of clouds or broken clouds. It is essentially a filter that amplifies high-frequency components, aiding in the detection and emphasis of subtle variations in the image. Compare the sharpening effects of the Laplacian operator with commonly used operators. As depicted in Figure 7, the sharpening results obtained using Roberts, Prewitt and Sobel operators exhibit missing cloud edges, whereas the sharpening results at the edges using the Laplacian operator demonstrate continuity. Therefore, the Laplacian operator is chosen to enhance the features of cloud edges and broken clouds. The definition of the Laplacian operator is as follows:
∇2f=∂2f∂2x+∂2f∂2y | (3) |
By separately taking the second derivatives of the Laplacian operator in the x and y directions through differencing, we obtain:
∇2f=f(x+1,y)+f(x−1,y)+f(x,y+1)+f(x,y−1)−4f(x,y) | (4) |
The above formulas can be transformed into the form of convolution kernels:
(0101−41010) | (5) |
After the Laplacian operator processing, the spectral features of cloud edges and broken clouds are enhanced. However, the extensive areas of thick clouds that manifest as low-frequency signals are removed. This can lead to sample imbalance and affect clustering results. Therefore, overlaying the image processed with the Laplacian operator onto the original image is crucial. The resulting feature-enhanced image can highlight cloud edges and broken cloud details while preserving the thick cloud portions from the original image. The overlay formula is as follows:
g(x,y)=f(x,y)∗w(i,j)=f(x,y)−[∇2f] | (6) |
Finally, based on Eq (6), we obtain the convolution kernel used in this paper:
w(i,j)=(0 - 10 - 15 - 10 - 10) | (7) |
Figure 8 provides a comparison of the results before and after feature enhancement. From Figure 8(a), (b), a noticeable improvement in the clarity of cloud edges and broken clouds that is within the RS image is observed after the feature enhancement. Additionally, the R3+4 values for cloud edges and broken clouds are extracted from the image for PDF comparison. Figure 8(c) shows that feature enhancement significantly increases the R3+4 values for cloud edges and broken clouds. This will effectively reduce the occurrence of false negatives in the algorithm for cloud edge and broken cloud pixels.
During detection, the diversity of cloud and the underlying surface results in complex distributions of reflectance and brightness temperature. GMM can represent the PDF of data by the way of using a convex combination of multiple Gaussian functions (a linear combination of multiple Gaussian functions). Given a sufficient number of samples, GMM can model distributions of any shape [30]. Therefore, GMM can accurately describe the probability distributions of reflectance and brightness temperature for both clouds and the underlying surface. Additionally, some spectral characteristics of clouds and underlying surfaces may overlap (as shown in Figure 5). The advantage of GMM compared to clustering algorithms like K-means and mean-shift lies in its more flexible modeling capability. Unlike K-means and other hard clustering methods, GMM has the ability to perform soft clustering which allows data points to belong to different clusters with certain probabilities. This flexibility helps in better understanding and representing relationships between data points, making the model more adaptable to clusters that exhibit overlap or have fuzzy boundaries. Additionally, GMM uses probability distributions to describe clusters, which makes it better suited for handling complex data distributions, especially when dealing with non-spherical distributions where GMM often outperforms other algorithms [31]. Its PDF formula is as follows:
P(x|θ)=K∑j=1βjf(x|μj,σj2) | (8) |
In the equation, x represents a vector X = {x1, x2, x3, ..., xn} consisting of n sample values, where X contains K categories. The weight of the jth class is denoted by βj, and the function f(x) is the PDF of a single Gaussian model with mean μj and variance σj for the jth class.
The set of data for the study area, R3+4, BT15 and BTD7-14, is denoted as X = {x1, x2, x3, ..., xn}, where xi is a sample drawn from the set X. The elements in the set are independent of each other and follow a GMM. As shown in Eq (7), the GMM density function contains a parameter set θ = {β, μ, σ2} and this parameter set is defined as θ. For a single Gaussian model, the parameters θ can be estimated using the Maximum Likelihood (ML) method, where the likelihood function is given by the PDF:
L(θ)=n∏i=1P(x|θ)=n∏i=11√2πσ2exp(−(xi−μ)22σ2) | (9) |
Similarly, the likelihood function can be derived from the GMM PDF as follows:
L(θ)=n∏i=1P(x|θ)=n∑i=1log[K∑j=1βjf(x|μj,σj2)] | (10) |
In the equation, there is a complex nonlinear relationship between the likelihood function L (θ) and the parameter set θ, making it impractical to directly maximize the likelihood function through differentiation. The Expectation-Maximization (EM) algorithm is a common method for maximum likelihood estimation and is suitable for data with hidden, unknown variables. It can appropriately estimate the optimal parameters of probability models [32,33]. The specific steps of the EM algorithm are as follows:
Step 1: Initialize the parameter set θ = {β, μ, σ2} for each category (hidden variables) based on prior knowledge.
Step 2: Calculate the probability that the data is generated by a Gaussian distribution as follows:
γ(i,j)=βjP(xi|μj,σj2)K∑j=1βjP(xi|μj,σj2) | (11) |
Step 3: Update the μ and σ2 for each Gaussian model as follows:
μj=n∑i=1γ(i,j)−xin∑i=1γ(i,j) | (12) |
σj2=n∑i=1γ(i,j)(xi−μj)(xi−μj)Tn∑i=1γ(i,j) | (13) |
Step 4: Update the β for each Gaussian model as follows:
βj=n∑n=1γ(i,j)n | (14) |
Repeat Steps 2 through Step 4 until the maximum likelihood value L (θ) approaches stability. Once this condition is met, the algorithm is complete.
Before applying the GMM algorithm for clustering, the value of K needs to be determined. In practical situations, the types of distributions contained in the data samples are unknown. As the number of sub-models in the observed samples increases, the mixture model better reflects the characteristics of the data samples. However, this also leads to a more complex model. A balance point should be established to maintain the simplicity of the mixture model and ensure the accuracy of the model fit. Therefore, how to determine the value of parameter K to ensure that the mixture model is both accurate and concise is a primary goal to optimize the model. We use the AIC and BIC criterions from information statistics to determine the parameter K and their expressions are as follows:
VAIC=2m−lnL(x|K,β,μ,σ2)VBIC=mlnn−lnL(x|K,β,μ,σ2) | (15) |
In the equations, m is the number of parameters in the mixture model, n is the number of samples, and lnL (x|K, β, μ, σ2) represents the maximum likelihood value. The minimum value of VAIC and VBIC is taken as the criteria for selecting the optimal model.
First, four sets of RS images of R3+4 are selected as sample data, and the range for selecting K is set between 1 and 14. VAIC and VBIC are calculated for different values of K. As shown in Figure 9, with the increase of K, both VAIC and VBIC show an overall decreasing trend, and the trend gradually stabilizes when K > 8. To further determine the specific value of K, values of K are set to 5, 6, 7 and 8, and the GMM cloud detection results for different K values are compared to determine the appropriate value of K. Figure 10 shows the comparison of cloud pixel omissions for K = 5, 6 and 7. As the increase in the value of K, the number of omitted cloud pixels gradually decreases. When K = 7, the algorithm produces detection results for various types of clouds that closely align with the actual cloud coverage. Furthermore, as shown in Figure 11, there is tiny little difference in the detection results of cloud edge details between K = 7 and K = 8. Therefore, K = 7 ensures that the mixture model is both accurate and concise.
The Himawari-8 data was provided by the Japan Aerospace Exploration Agency (https://www.eorc.jaxa.jp/ptree/). In this study, a dataset was constructed for AHI, encompassing partial data from 2021 and 2022. This dataset includes both full disk images and study area images, with over 1,400,000 pixels annotated.
The computer used for the experiments has an AMD R5 5600G processor with a base frequency of 2900 MHz, a 1 TB solid-state drive and 16 GB of RAM. The experiment uses the OpenCV and Scikit-Learn packages to implement the proposed algorithm.
The GMM algorithm selected in this study requires pre-determination of the number of clusters, similar to the K-means algorithm. Therefore, to demonstrate the advantage of GMM's soft clustering capability over K-means, a comparison was made. The selected images were taken during early morning or evening when the reflectance of clouds is lower compared to daytime, posing a significant challenge to the clustering algorithms. As shown in Figure 12 within the red box, K-means wrongly classified many low-reflectance clouds as underlying surfaces. This is because K-means tends to rigidly assign data points to the nearest cluster center when dealing with non-convex shapes or overlapping regions. Consequently, some low-reflectance clouds were erroneously categorized as land features by K-means. On the other hand, GMM is capable of better reflecting the probability of data points belonging to different clusters. Therefore, in such scenarios, GMM might more accurately identify areas with ambiguous boundaries. It excels in capturing complex data distributions and delineating regions with unclear boundaries, which might lead to a more precise identification of low-reflectance clouds compared to K-means.
The proposed algorithm uses a feature enhancement module to enhance the features of cloud edges and broken clouds, and it integrates the detection results of cloud edges and broken clouds in the final detection results, thereby improving the overall accuracy of the algorithm. Figure 13 shows the heatmap of the proposed algorithm, which reflects the aggregation of broken clouds and cloud edges with their respective categories. In Figure 13(a), (b), the model's attention to broken clouds is evident (highlighted in yellow boxes). Figure 13(b), (c) depict the attention to cloud edges, and it can be observed that the cloud edges of thin clouds also receive significant attention (highlighted in yellow boxes).
Traditional methods such as the multi-spectral threshold method are widely used, and machine learning methods like RF, KNN and GMM have efficient classification capabilities. Therefore, this paper implemented the proposed algorithm and the aforementioned algorithm and implemented cloud detection experiments on Himawari-8 images from different time phases and regions to validate the applicability of the proposed algorithm.
Figure 14 presents the comparison of cloud detection results using the multi-spectral threshold method [10], RF [15], KNN [34], GMM and the proposed algorithm. In the figure, the true labels obtained through visual interpretation are depicted in yellow, while the detection results are shown in white rendering. Professionally trained experts or analysts, following the cloud definition method outlined in Section 3.1, utilized Photoshop software to meticulously annotate the cloud's location pixel by pixel.
In Figure 14(a), the detection results of GMM and the proposed algorithm are the most accurate, while the multi-spectral threshold method and RF exhibit a slight underestimation of cloud pixels, primarily at the cloud edges. KNN shows the highest number of cloud pixel omissions (highlighted within the green bounding boxes in the images). In Figure 14(b), GMM and the proposed algorithm provide the best detection results, with the multi-spectral threshold method and RF exhibit the greatest number of cloud pixels omission. KNN exhibits a large area of omissions in the lower right corner of the image and it also misclassifies some non-cloud pixels as clouds at the cloud edges (highlighted within the purple bounding boxes for omissions and the yellow bounding boxes for misclassifications). In Figure 14(c), (d), the cloud edges in the upper right corners of the images are somewhat blurred. Upon comparison, it is evident that the proposed algorithm produces the most accurate detection results. Moreover, the multi-spectral threshold method, RF and GMM all exhibit varying degrees of omissions at the cloud edges. Multi-spectral threshold method has the most pronounced omission phenomenon, followed by RF (highlighted within the blue bounding boxes for omissions and the yellow bounding boxes for misclassifications).
For the detection of broken clouds, as depicted within the red box in Figure 14(d), the multi-spectral threshold method exhibits the highest number of missed broken cloud pixels, followed by RF and GMM. Additionally, KNN misclassifies numerous pixels as clouds. Analysis of the above comparison results reveals that in sufficient sunlight conditions during the day, the reflectance of clouds in the visible light spectrum is relatively high. During this time, the multi-spectral threshold method is more suitable. However, as it approaches evening, decreasing light levels can lead to higher threshold values, which is not conducive to cloud detection. RF and KNN, as supervised learning algorithms, have high requirements for sample size and the balance of samples among different categories. However, the small and dispersed nature of scattered clouds poses challenges in creating training samples for RF and KNN, making it difficult for these algorithms to learn the necessary features effectively.
In contrast, as an unsupervised learning algorithm, GMM can automatically learn the inherent relationships between different categories and perform classification without the need for an extensive set of training samples. Because the spectral characteristics of cloud edges are somewhat similar to those of underlying surfaces, the threshold set by the multi-spectral threshold method is relatively high for cloud edge detection. While GMM has several advantages in detecting large-area thick clouds, it does not consider the spectral feature similarity between cloud edges and underlying surfaces. Therefore, the proposed algorithm achieves the best detection results for various cloud types.
Additionally, as shown in Figure 15, full disk RS data from September 24, 2022, at UTC 03:00 are selected for cloud detection. Among the five algorithms, the multi-spectral threshold method and GMM achieve relatively good detection results, but there are instances of cloud underestimation in some regions (highlighted within the green boxes). RF and KNN show varying degrees of cloud false positives (highlighted within the red boxes). The proposed algorithm, an enhancement of GMM, reduces the underestimation of cloud pixels, resulting in the best detection performance among the five algorithms, demonstrating that this algorithm provides reliable results even for large-scale areas.
To quantitatively evaluate these methods, this study uses two evaluation metrics, the hit rate (HR) and the Hansen-Kuiper skill score (KSS), to analyze 15 RS images from different times and regions. The HR represents the proportion of correctly identified cloud and clear-sky pixels to the total number of pixels. The KSS score is used to provide a comprehensive overall measure. The formulas for calculating HR and KSS are as follows:
HR=TC+TUPA | (16) |
KSS=TC×TU−TF×FT(TC+FT)(TU+TF) | (17) |
In the formulas, TC represents the number of pixels correctly detected as clouds, TU is the number of pixels that are actually non-cloud but are detected as clouds, FT is the number of pixels that are actually clouds but are detected as non-cloud and PA is the total number of pixels in the image.
Figure 16 shows the evaluation results of different algorithms for detecting thick clouds, cloud edges and broken clouds. The values of HR and KSS approaching 1 indicate superior algorithm performance. For thick cloud detection, the HR values of the multi-spectral threshold method, RF, KNN, GMM and the proposed algorithm are 0.793, 0.832, 0.799, 0.974 and 0.976, respectively, and the KSS values are 0.692, 0.763, 0.642, 0.973 and 0.974, respectively. It is evident that GMM and the proposed algorithm outperform the other three algorithms in thick cloud detection. For cloud edge and broken cloud detection, the HR values of the five algorithms are 0.619, 0.687, 0.624, 0.963 and 0.974, respectively, and the KSS values are 0.458, 0.561, 0.563, 0.947 and 0.973, respectively. Compared to thick cloud detection, the HR and KSS values of the multi-spectral threshold method, RF and KNN significantly decrease in cloud edge and broken cloud detection, while those of GMM and the proposed algorithm remain above 0.96 and 0.94, respectively. Moreover, the proposed algorithm, which incorporates the feature enhancement module, shows an improvement of 0.011 in HR and 0.026 in KSS compared to GMM. This indicates that the feature enhancement module aids the classification model in extracting deeper cloud edge and broken cloud features. Additionally, as shown in Table 2, 20 images sized at 256 × 256 were selected for quantitative analysis to demonstrate the overall performance of the algorithm. Compared to GMM, the proposed algorithm shows an improvement of 0.002 in HR and 0.015 in KSS.
Algorithm | HR | KSS |
Multi-spectral threshold method | 0.763 | 0.572 |
RF | 0.811 | 0.65 |
KNN | 0.753 | 0.606 |
GMM | 0.973 | 0.958 |
Proposed algorithm | 0.975 | 0.973 |
When performing target detection and task analysis, the vast amount of RS data meant that the runtime of cloud detection algorithms is an important concern. To address this, RS images of sizes 1000 × 1100, 2401 × 2401 and 60016001 are selected to compare the runtime differences between different algorithms. As shown in Figure 17, when the image sizes are 1000 × 1100 and 2401 × 2401, the runtime differences among the four machine learning algorithms does not vary significantly, with GMM having the shortest runtime, followed by the proposed algorithm, RF and KNN. However, when the image size is 6001 × 6001, KNN's runtime is significantly longer than the other three algorithms (approximately 3.9 times longer than GMM and 3.65 times longer than RF), while the proposed algorithm with the feature enhancement module has a runtime only 7.45 seconds longer than GMM. Therefore, for cloud detection in large-sized RS images, the algorithm proposed in this study can maintain high accuracy while having a relatively short runtime.
While the proposed algorithm generally achieves satisfactory cloud detection results in most scenarios, it encounters errors, particularly when dealing with cloud overlap. This defect is especially obvious when dealing with cloud shadows. These shadows, appearing as dark regions in visible light images, share spectral characteristics similar to the underlying surfaces. The similarity poses a challenge in distinguishing clouds within these shadowed areas, leading to errors in identification. Figure 18 illustrates examples of cloud detection errors specifically within shadowed regions, highlighting numerous omitted pixels in the detection results. In addition, the proposed algorithm is tailored for satellites capable of providing 2 km resolution images. With higher image resolution, the diversity of surface types becomes more intricate, leading to fluctuations in the optimal K value. In such scenarios, extensive experimental analysis becomes crucial for re-evaluating the selection of the K value. Even then, pinpointing a stable K value can be challenging. For instance, in an image observed at 2 km resolution, a pixel m (x, y) might initially be identified as a cloud. However, as the resolution increases, the area represented by m (x, y) encompasses more pixels. Among these newly added pixels, some might reveal underlying surfaces that were previously unobservable at the 2 km resolution.
The future research goal is to combine thermal infrared spectroscopy with neural network algorithms to enhance the algorithm's transferability. Moreover, by exploring the imaging correlation between clouds and their shadows, there is potential to calculate the orientation and distance of shadows concerning cloud areas. This computational approach could be helpful in effectively filtering out these shadows from the detection results.
The improved cloud detection technology holds significant potential in disaster management. Accurate cloud coverage data is meaningful to monitoring and early warning systems for natural disasters such as storms and floods, aiding in the proactive implementation of response measures and minimizing potential losses. However, when applying these technologies, the critical importance of data accuracy and timeliness in decision-making must be considered to prevent the potential risks of misinformation or delays.
Furthermore, advancements in cloud detection technology may raise concerns regarding personal privacy, particularly in the monitoring and analysis of extensive areas. Therefore, safeguarding personal information and data privacy should be a crucial consideration when utilizing these algorithms, necessitating the establishment of stringent guidelines for data processing and sharing.
In this paper, we propose a multi-spectral data cloud detection algorithm based on a feature enhancement module and Gaussian mixture model (GMM) clustering. First, the spectral data of clouds and underlying surfaces are statistically analyzed. Based on the analysis results, an appropriate feature scheme is selected to achieve cloud detection throughout the day. Second, the Laplacian operator is used to enhance the spectral features of cloud edges and broken clouds. The enhanced data is then input into a GMM classifier for cloud edge and broken cloud detection. The original RS data is simultaneously subjected to GMM clustering to obtain thick cloud detection results. Finally, the results from the both branches are combined to obtain the final cloud detection result.
Comparing the results of the multi-spectral threshold methods, RF, KNN, GMM and the proposed algorithm, the following conclusions are drawn: The proposed algorithm achieves the highest accuracy with a hit rate (HR) of 0.976 for the case of thick cloud detection and a Hansen-Kuiper skill score (KSS) of 0.974 for the case of cloud edges and broken cloud detection. This means the proposed algorithm demonstrates significant advantages over other algorithms, with an HR and KSS improvement of 0.011 and 0.026, respectively, compared to GMM. Furthermore, the inclusion of the feature enhancement module in the proposed algorithm maintains a relatively short runtime. This further demonstrates that the proposed algorithm can achieve fast and high-precision cloud detection for different types of clouds. Therefore, the proposed algorithm can provide real-time cloud detection results for various tasks such as vegetation change analysis, disaster monitoring and cartography, offering a viable solution to alleviate the challenges posed by cloud interference.
The authors declare that they have not used Artificial Intelligence (AI) tools in the creation of this article.
This work is supported by the Major Scientific and Technological Projects of Yunnan Province: Research on Key Technologies of Ecological Environment Monitoring and Intelligent Management of Natural Resources in Yunnan (202202AD080010), the National Natural Science Foundation of China (52107017) and the Fundamental Research Fund of Science and Technology Department of Yunnan Province (202201AU070172).
The authors declare that there are no conflicts of interest.
[1] |
Tamburrini O, Aprile I, Falcone C, et al. (2011) Off-label use of intravascular iodinated organic and MR contrast media. Radiol Med 116: 1-14. doi: 10.1007/s11547-010-0601-5
![]() |
[2] |
Meloni MM, Barton S, Xu L, et al. (2017) Contrast agents for cardiovascular magnetic resonance imaging: an overview. J Mater Chem B 5: 5714-5725. doi: 10.1039/C7TB01241A
![]() |
[3] |
Lauffer RB (1987) Paramagnetic metal complexes as water proton relaxation agents for NMR imaging: theory and design. Chem Rev 87: 901-927. doi: 10.1021/cr00081a003
![]() |
[4] |
Schörner W, Kazner E, Laniado M, et al. (1984) Magnetic resonance tomography (MRT) of intracranial tumours: Initial experience with the use of the contrast medium gadolinium-DTPA. Neurosurg Rev 7: 303-312. doi: 10.1007/BF01892910
![]() |
[5] |
Essig M, Anzalone N, Combs SE, et al. (2012) MR imaging of neoplastic central nervous system lesions: review and recommendations for current practice. Am J Neuroradiol 33: 803-817. doi: 10.3174/ajnr.A2640
![]() |
[6] |
Anzalone N, Gerevini S, Scotti R, et al. (2009) Detection of cerebral metastases on magnetic resonance imaging: intraindividual comparison of gadobutrol with gadopentetate dimeglumine. Acta Radiol 50: 933-940. doi: 10.1080/02841850903095385
![]() |
[7] |
Malayeri AA, Brooks KM, Bryant LH, et al. (2016) National Institutes of health perspective on reports of gadolinium deposition in the brain. J Am Coll Radiol 13: 237-241. doi: 10.1016/j.jacr.2015.11.009
![]() |
[8] |
Weinmann HJ, Brasch RC, Press WR, et al. (1984) Characteristics of gadolinium-DTPA complex: a potential NMR contrast agent. Am J Roentgenol 142: 619-624. doi: 10.2214/ajr.142.3.619
![]() |
[9] |
Lin SP, Brown JJ (2007) MR contrast agents: Physical and pharmacologic basics. J Magn Reson Imaging 25: 884-899. doi: 10.1002/jmri.20955
![]() |
[10] |
Ersoy H, Rybicki FJ (2007) Biochemical safety profiles of gadolinium-based extracellular contrast agents and nephrogenic systemic fibrosis. J Magn Reson Imaging 26: 1190-1197. doi: 10.1002/jmri.21135
![]() |
[11] |
Bellin MF, Van Der Molen AJ (2008) Extracellular gadolinium-based contrast media: an overview. Eur J Radiol 66: 160-167. doi: 10.1016/j.ejrad.2008.01.023
![]() |
[12] |
Evans CH (1990) Biochemistry of the Lanthanides New York: Plenum Press. doi: 10.1007/978-1-4684-8748-0
![]() |
[13] |
De León-Rodríguez LM, Martins AF, Pinho MC, et al. (2015) Basic MR relaxation mechanisms and contrast agent design. J Magn Reson Imaging 42: 545-565. doi: 10.1002/jmri.24787
![]() |
[14] |
Levine D, McDonald RJ, Kressel HY (2018) Gadolinium retention after contrast-enhanced MRI. JAMA 320: 1853-1854. doi: 10.1001/jama.2018.13362
![]() |
[15] | Shepherd M, Lata S, Mani S, et al. (2009) Anaphylaxis to gadolinium radiocontrast: a case report and review of the literature. J La State Med Soc 161: 282-284. |
[16] |
Raisch DW, Garg V, Arabyat R, et al. (2014) Anaphylaxis associated with gadolinium-based contrast agents: Data from the food and drug administration's adverse event reporting system and review of case reports in the literature. Expert Opin Drug Saf 13: 15-23. doi: 10.1517/14740338.2013.832752
![]() |
[17] |
Franckenberg S, Berger F, Schaerli S, et al. (2018) Fatal anaphylactic reaction to intravenous gadobutrol, a gadolinium-based MRI contrast agent. Radiol Case Rep 13: 299-301. doi: 10.1016/j.radcr.2017.09.012
![]() |
[18] |
Jung JW, Kangand HR, Kim MH, et al. (2012) Immediate hypersensitivity reaction to gadolinium-based MR contrast media. Radiology 264: 414-422. doi: 10.1148/radiol.12112025
![]() |
[19] |
Morzycki A, Bhatia A, Murphy KJ (2017) Adverse reactions to contrast material: a Canadian update. Can Assoc Radiol J 68: 187-193. doi: 10.1016/j.carj.2016.05.006
![]() |
[20] |
Behzadi AH, Farooq Z, Newhouse JH, et al. (2018) MRI and CT contrast media extravasation a systematic review. Medicine 97: e0055. doi: 10.1097/MD.0000000000010055
![]() |
[21] |
Varela DC, Sepulveda P, Prieto J, et al. (2015) Extravasation of intravenous contrast media: What every radiologist should know. Rev Chil Radiol 21: 151-157. doi: 10.4067/S0717-93082015000400006
![]() |
[22] |
Blasco-Perrin H, Glaser B, Pienkowski M, et al. (2013) Gadolinium induced recurrent acute pancreatitis. Pancreatology 13: 88-89. doi: 10.1016/j.pan.2012.12.002
![]() |
[23] |
Unal O, Arslan H (1999) Cardiac arrest caused by IV gadopentetate dimeglumine. Am J Roentgenol 172: 1141. doi: 10.2214/ajr.172.4.10587169
![]() |
[24] | US Food and Drug AdministrationMedical Imaging Drugs Advisory Committee Meeting, FDA briefing document: Gadolinium retention after gadolinium based contrast magnetic resonance imaging in patients with normal renal function. (2017) . |
[25] |
Maramattom BV, Manno EM, Wijdicks EFM, et al. (2005) Gadolinium encephalopathy in a patient with renal failure. Neurology 64: 1276-1278. doi: 10.1212/01.WNL.0000156805.45547.6E
![]() |
[26] |
Hui FK, Mullins M (2009) Persistence of gadolinium contrast enhancement in CSF: A possible harbinger of gadolinium neurotoxicity? Am J Neuroradiol 30: e1. doi: 10.3174/ajnr.A1205
![]() |
[27] |
Kim SH, Jo EJ, Kim MY, et al. (2013) Clinical value of radiocontrast media skin tests as a prescreening and diagnostic tool in hypersensitivity reactions. Ann Allergy Asthma Immunol 110: 258-262. doi: 10.1016/j.anai.2013.01.004
![]() |
[28] |
Shellock FG, Kanal E (1999) Safety of magnetic resonance imaging contrast agents. J Magn Res Im 10: 477-484. doi: 10.1002/(SICI)1522-2586(199909)10:3<477::AID-JMRI33>3.0.CO;2-E
![]() |
[29] |
Grobner T (2006) Gadolinium-a specific trigger for the development of nephrogenic fibrosing dermopathy and nephrogenic systemic fibrosis? Nephrol Dial Transpl 21: 1104-1108. doi: 10.1093/ndt/gfk062
![]() |
[30] |
Thomson LK, Thomson PC, Kingsmore DB, et al. (2015) Diagnosing nephrogenic systemic fibrosis in the post-FDA restriction era. J Magn Reson Imaging 41: 1268-1271. doi: 10.1002/jmri.24664
![]() |
[31] |
Larson KN, Gagnon AL, Darling MD, et al. (2015) Nephrogenic systemic fibrosis manifesting a decade after exposure to gadolinium. JAMA Dermatol 151: 1117-1120. doi: 10.1001/jamadermatol.2015.0976
![]() |
[32] |
Bernstein EJ, Schmidt-Lauber C, Kay J (2012) Nephrogenic systemic fibrosis: a systemic fibrosing disease resulting from gadolinium exposure. Best Pract Res Cl Rheumatol 26: 489-503. doi: 10.1016/j.berh.2012.07.008
![]() |
[33] |
Sanyal S, Marckmann P, Scherer S, et al. (2011) Multiorgan gadolinium (Gd) deposition and fibrosis ina patient with nephrogenic systemic fibrosis-an autopsy-based review. Nephrol Dial Transplant 26: 3616-3626. doi: 10.1093/ndt/gfr085
![]() |
[34] |
Kay J, Bazari H, Avery LL, et al. (2008) Case 6-2008: a 46-year-old woman with renal failure and stiffness of the joints and skin. New Engl J Med 358: 827-838. doi: 10.1056/NEJMcpc0708697
![]() |
[35] |
Bhave G, Lewis JB, Chang SS (2008) Association of gadolinium based magnetic resonance imaging contrast agents and nephrogenic systemic fibrosis. J Urol 180: 830-835. doi: 10.1016/j.juro.2008.05.005
![]() |
[36] |
Cowper SE, Su LD, Bhawan J, et al. (2001) Nephrogenic fibrosing dermopathy. Am J Dermatopath 23: 383-393. doi: 10.1097/00000372-200110000-00001
![]() |
[37] |
Cowper SE, Robin HS, Steinberg SM, et al. (2000) Scleromyxedema-like cutaneous disease in renal dialysis patients. Lancet 356: 1000-1001. doi: 10.1016/S0140-6736(00)02694-5
![]() |
[38] |
Weigle JP, Broome DR (2008) Nephrogenic systemic fibrosis: chronic imaging findings and review of the medical literature. Skeletal Radiol 37: 457-464. doi: 10.1007/s00256-008-0464-1
![]() |
[39] |
Zou Z, Zhang HL, Roditi GH, et al. (2011) Nephrogenic systemic fibrosis: review of 370 biopsy-confirmed cases. JACC: Cardiovasc Imag 4: 1206-1216. doi: 10.1016/j.jcmg.2011.08.013
![]() |
[40] |
Morris MF, Zhang Y, Zhang H, et al. (2009) Features of nephrogenic systemic fibrosis on radiology examinations. Am J Roentgenol 193: 61-69. doi: 10.2214/AJR.08.1352
![]() |
[41] |
Tsushima Y, Kanal E, Thomsen HS (2010) Nephrogenic systemic fibrosis: risk factors suggested from Japanese published cases. Brit J Radiol 83: 590-595. doi: 10.1259/bjr/17689538
![]() |
[42] |
Thomsen HS, Morcos SK, Almén T, et al. (2013) Nephrogenic systemic fibrosis and gadolinium-based contrast media: updated ESUR contrast medium safety committee guidelines. Eur Radiol 23: 307-318. doi: 10.1007/s00330-012-2597-9
![]() |
[43] |
Mazhar SM, Shiehmorteza M, Kohl CA, et al. (2009) Nephrogenic systemic fibrosis in liver disease: a systematic review. J Magn Reson Imaging 30: 1313-1322. doi: 10.1002/jmri.21983
![]() |
[44] | Elmholdt TR, Jørgensen B, Ramsing M, et al. (2010) Two cases of nephrogenic systemic fibrosis after exposure to the macrocyclic compound gadobutrol. NDT Plus 3: 285-287. |
[45] |
Kay J (2008) Gadolinium and nephrogenic systemic fibrosis: The evidence of things not seen. Clev Clin J Med 75: 112. doi: 10.3949/ccjm.75.2.112
![]() |
[46] |
Todd DJ, Kay J (2008) Nephrogenic systemic fibrosis: an epidemic of gadolinium toxicity. Curr Rheumatol Rep 10: 195-204. doi: 10.1007/s11926-008-0033-6
![]() |
[47] |
Sieber MA, Lengsfeld P, Frenzel T, et al. (2008) Preclinical investigation to compare different gadolinium-based contrast agents regarding their propensity to release gadolinium in vivo and to trigger nephrogenic systemic fibrosis-like lesions. Eur Radiol 18: 2164-2173. doi: 10.1007/s00330-008-0977-y
![]() |
[48] |
Semelka RC, Prybylskib JP, Ramalho M (2019) Influence of excess ligand on nephrogenic systemic fibrosis associated with nonionic, linear gadolinium-based contrast agents. Magn Res Imaging 58: 174-178. doi: 10.1016/j.mri.2018.11.015
![]() |
[49] | US Food and Drug Administration FDA request boxes warning for contrast agents used to improve MRI images (2007) .Available from: http://wayback.archive-it.org/7993/20170112033008/http://www.fda.gov/NewsEvents/Newsroom/PressAnnouncements/2007/ucm108919.htm. |
[50] |
Khawaja AZ, Cassidy DB, Al Shakarchi J, et al. (2015) Revisiting the risks of MRI with Gadolinium based contrast agents: review of literature and guidelines. Insights Imaging 6: 553-558. doi: 10.1007/s13244-015-0420-2
![]() |
[51] | Canga A, Kislikova M, Martínez-Gálvez M, et al. (2014) Renal function, nephrogenic systemic fibrosis and other adverse reactions associated with gadolinium-based contrast media. Nefrologia 34: 428-438. |
[52] |
Martin DR, Krishnamoorthy SK, Kalb B, et al. (2010) Decreased incidence of NSF in patients on dialysis after changing gadolinium contrast-enhanced MRI protocols. J Magn Reson Imaging 31: 440-446. doi: 10.1002/jmri.22024
![]() |
[53] |
Altun E, Martin DR, Wertman R, et al. (2009) Nephrogenic systemic fibrosis: Change in incidence following a switch in gadolinium agents and adoption of a gadolinium policy—report from two US universities. Radiology 253: 689-696. doi: 10.1148/radiol.2533090649
![]() |
[54] |
Xia D, Davis RL, Crawford JA, et al. (2010) Gadolinium released from MR contrast agents is deposited in brain tumors: in situ demonstration using scanning electron microscopy with energy dispersive X-ray spectroscopy. Acta Radiol 51: 1126-1136. doi: 10.3109/02841851.2010.515614
![]() |
[55] |
Kanda T, Ishii K, Kawaguchi H, et al. (2014) High signal intensity in the dentate nucleus and globus pallidus on unenhanced T1-weighted MR images: Relationship with increasing cumulative dose of a gadolinium based contrast material. Radiology 270: 834-841. doi: 10.1148/radiol.13131669
![]() |
[56] |
McDonald RJ, McDonald JS, Kallmes DF, et al. (2015) Intracranial gadolinium deposition after contrast-enhanced MR imaging. Radiology 275: 772-782. doi: 10.1148/radiol.15150025
![]() |
[57] |
Olchowy C, Cebulski K, Łasecki M, et al. (2017) The presence of the gadolinium-based contrast agent depositions in the brain and symptoms of gadolinium neurotoxicity-a systematic review. PLoS One 12: e0171704. doi: 10.1371/journal.pone.0171704
![]() |
[58] |
Pullicino R, Radon M, Biswas S, et al. (2018) A review of the current evidence on gadolinium deposition in the brain. Clin Neuroradiol 28: 159-169. doi: 10.1007/s00062-018-0678-0
![]() |
[59] |
Gianolio E, Gregorio ED, Aime S (2019) Chemical insights into the issues of Gd retention in the brain andother tissues upon the administration of Gd-containing MRI contrast agents. Eur J Inorg Chem 2019: 137-151. doi: 10.1002/ejic.201801220
![]() |
[60] |
Kanda T, Osawa M, Oba H, et al. (2015) High signal intensity in dentate nucleus on unenhanced T1-weightedMR images: association with linear versus macrocyclic gadolinium chelate administration. Radiology 275: 803-809. doi: 10.1148/radiol.14140364
![]() |
[61] |
Weberling LD, Kieslich PJ, Kickingereder P, et al. (2015) Increased signal intensity in the dentate nucleus on unenhanced T1-weighted images after gadobenate dimeglumine administration. Invest Radiol 50: 743-748. doi: 10.1097/RLI.0000000000000206
![]() |
[62] |
Errante Y, Cirimele V, Mallio CA, et al. (2014) Progressive increase of T1 signal intensity of the dentate nucleus on unenhanced magnetic resonance images is associated with cumulative doses of intravenously administered gadodiamide in patients with normal renal function, suggesting dechelation. Invest Radiol 49: 685-690. doi: 10.1097/RLI.0000000000000072
![]() |
[63] |
Zhang Y, Cao Y, Shih GL, et al. (2017) Extent of signal hyperintensity on unenhanced T1-weighted brain MR images after more than 35 administrations of linear gadolinium-based contrast agents. Radiology 282: 516-525. doi: 10.1148/radiol.2016152864
![]() |
[64] |
Radbruch A, Weberling LD, Kieslich PJ, et al. (2015) Gadolinium retention in the dentate nucleus and globus pallidus is dependent on the class of contrast agent. Radiology 275: 783-791. doi: 10.1148/radiol.2015150337
![]() |
[65] |
Malhotra A, LeSar B, Wu X, et al. (2018) Progressive T1 shortening of the dentate nucleus in patients with multiple sclerosis: Result of multiple administrations of linear gadolinium contrast agents versus intrinsic disease. Am J Roentgenol 211: 1099-1105. doi: 10.2214/AJR.17.19155
![]() |
[66] |
Flood TF, Stence NV, Maloney JA, et al. (2017) Pediatric brain: Repeated exposure to linear gadolinium-based contrast material is associated with increased signal intensity at unenhanced T1-weighted MR imaging. Radiology 282: 222-228. doi: 10.1148/radiol.2016160356
![]() |
[67] |
Adin ME, Kleinberg L, Vaidya D, et al. (2015) Hyperintense dentate nuclei on T1-weighted MRI: relation to repeat gadolinium administration. Am J Neuroradiol 36: 1859-1865. doi: 10.3174/ajnr.A4378
![]() |
[68] |
Miller JH, Hu HH, Pokorney A, et al. (2015) MRI brain signal intensity changes of a child during the course of 35 gadolinium contrast examinations. Pediatrics 136: e1637-e1640. doi: 10.1542/peds.2015-2222
![]() |
[69] |
Hu HH, Pokorney A, Towbin RB, et al. (2016) Increased signal intensities in the dentate nucleus and globus pallidus on unenhanced T1-weighted images: evidence in children undergoing multiple gadolinium MRI exams. Pediatr Radiol 46: 1590-1598. doi: 10.1007/s00247-016-3646-3
![]() |
[70] |
Bae S, Lee H, Han K, et al. (2017) Gadolinium deposition in the brain: association with various GBCAs using a generalized additive model. Eur Radiol 27: 3353-3361. doi: 10.1007/s00330-016-4724-5
![]() |
[71] |
Quattrocchi CC, Mallio CA, Errante Y, et al. (2015) Gadodiamide and dentate nucleus T1 hyperintensity in patients with meningioma evaluated by multiple follow-up contrast-enhanced magnetic resonance examinations with no systemic interval therapy. Invest Radiol 50: 470-472. doi: 10.1097/RLI.0000000000000154
![]() |
[72] |
McDonald JS, McDonald RJ, Jentoft ME, et al. (2017) Intracranial gadolinium deposition following gadodiamide-enhanced magnetic resonance imaging in pediatric patients: a case-control study. JAMA Pediatr 171: 705-707. doi: 10.1001/jamapediatrics.2017.0264
![]() |
[73] |
Mallio CA, Vullo GL, Messina L, et al. (2020) Increased T1 signal intensity of the anterior pituitary gland on unenhanced magnetic resonance images after chronic exposure to gadodiamide. Invest Radiol 55: 25-29. doi: 10.1097/RLI.0000000000000604
![]() |
[74] |
Gianolio E, Bardini P, Arena F, et al. (2017) Gadolinium retention in the rat brain: Assessment of the amounts of insoluble gadolinium-containing species and intact gadolinium complexes after repeated administration of gadolinium-based contrast agents. Radiology 285: 839-849. doi: 10.1148/radiol.2017162857
![]() |
[75] |
Rasschaert M, Schroeder JA, Wu TD, et al. (2018) Multimodal imaging study of gadolinium presence in rat cerebellum: differences between Gd chelates, presence in the Virchow-Robin space, association with lipofuscin, and hypotheses about distribution pathway. Invest Radiol 53: 518. doi: 10.1097/RLI.0000000000000490
![]() |
[76] |
Radbruch A, Richter H, Fingerhu S, et al. (2019) Gadolinium deposition in the brain in a large animal model. Comparison of linear and macrocyclic gadolinium-based contrast agents. Invest Radiol 54: 531-536. doi: 10.1097/RLI.0000000000000575
![]() |
[77] |
Boyken J, Frenzel T, Lohrke J, et al. (2018) Gadolinium accumulation in the deep cerebellar nuclei and globus pallidus after exposure to linear but not macrocyclic gadolinium-based contrast agents in a retrospective pig study with high similarity to clinical conditions. Invest Radiol 53: 278-285. doi: 10.1097/RLI.0000000000000440
![]() |
[78] |
Robert P, Violas X, Grand S, et al. (2016) Linear gadolinium-based contrast agents are associated with brain gadolinium retention in healthy rats. Invest Radiol 51: 73-82. doi: 10.1097/RLI.0000000000000241
![]() |
[79] |
Strzeminska I, Factor C, Robert P, et al. (2020) Long-term evaluation of gadolinium retention in rat brain after single injection of a clinically relevant dose of gadolinium-based contrast agents. Invest Radiol 55: 138-143. doi: 10.1097/RLI.0000000000000623
![]() |
[80] |
Radbruch A, Haase R, Kieslich PJ, et al. (2017) No signal intensity increase in the dentate nucleus on unenhanced T1-weighted MR images after more than 20 serial injections of macrocyclic gadolinium-based contrast agents. Radiology 282: 699-707. doi: 10.1148/radiol.2016162241
![]() |
[81] |
Radbruch A, Haase R, Kickingereder P, et al. (2017) Pediatric brain: no increased signal intensity in the dentate nucleus on unenhanced T1-weighted MR images after consecutive exposure to a macrocyclic gadolinium-based contrast agent. Radiology 283: 828-836. doi: 10.1148/radiol.2017162980
![]() |
[82] |
Tibussek D, Rademacher C, Caspers J, et al. (2007) Gadolinium brain deposition after macrocyclic gadolinium administration: a pediatric case-control study. Radiology 285: 223-230. doi: 10.1148/radiol.2017161151
![]() |
[83] |
Schneider GK, Stroeder J, Roditi G, et al. (2017) T1 signal measurements in pediatric brain: findings after multiple exposures to gadobenate dimeglumine for imaging of non neurologic disease. Am J Neuroradiol 38: 1799-1806. doi: 10.3174/ajnr.A5270
![]() |
[84] |
Conte G, Preda L, Cocorocchio E, et al. (2017) Signal intensity change on unenhanced T1-weighted images in dentate nucleus and globus pallidus after multiple administrations of gadoxetate disodium: an intraindividual comparative study. Eur Radiol 27: 4372-4378. doi: 10.1007/s00330-017-4810-3
![]() |
[85] |
Ryu YJ, Choi YH, Cheon J, et al. (2018) Pediatric brain: Gadolinium deposition in dentate nucleus and globus pallidus on unenhanced T1-weighted images is dependent on the type of contrast agent. Invest Radiol 53: 246-255. doi: 10.1097/RLI.0000000000000436
![]() |
[86] |
Stanescu AL, Shaw DW, Murata N, et al. (2020) Brain tissue gadolinium retention in pediatric patients after contrast-enhanced magnetic resonance exams: pathological confirmation. Pediatr Radiol 50: 388-396. doi: 10.1007/s00247-019-04535-w
![]() |
[87] |
Bjørnerud A, Vatnehol SAS, Larsson C, et al. (2017) Signal enhancement of the dentate nucleus at unenhanced MR imaging after very high cumulative doses of the macrocyclic gadolinium-based contrast agent gadobutrol: an observational study. Radiology 285: 434-444. doi: 10.1148/radiol.2017170391
![]() |
[88] |
Splendiani A, Perri M, Marsecano C, et al. (2018) Effects of serial macrocyclic based contrast materials gadoterate meglumine and gadobutrol administrations on gadolinium related dentate nuclei signal increases in unenhanced t1-weighted brain: a retrospective study in 158 multiple sclerosis (MS) patients. Radiol Med 123: 125-134. doi: 10.1007/s11547-017-0816-9
![]() |
[89] |
Stojanov DA, Aracki-Trenkic A, Vojinovic S, et al. (2016) Increasing signal intensity within the dentate nucleus and globus pallidus on unenhanced T1W magnetic resonance images in patients with relapsing remitting multiple sclerosis: Correlation with cumulative dose of a macrocyclic gadolinium-based contrast agent, gadobutrol. Eur Radiol 26: 807-815. doi: 10.1007/s00330-015-3879-9
![]() |
[90] |
Tedeschi E, Palma G, Canna A, et al. (2016) In vivo dentate nucleus MRI relaxometry correlates with previous administration of gadolinium-based contrast agents. Eur Radiol 26: 4577-4584. doi: 10.1007/s00330-016-4245-2
![]() |
[91] |
Lattanzio SM, Imbesi F (2020) Fibromyalgia associated with repeated gadolinium contrast-enhanced MRI examinations. Radiol Case Rep 15: 534-541. doi: 10.1016/j.radcr.2020.02.002
![]() |
[92] |
Roberts DR, Welsh CA, LeBel DP, et al. (2017) Distribution map of gadolinium deposition within the cerebellum following GBCA administration. Neurology 88: 1206-1208. doi: 10.1212/WNL.0000000000003735
![]() |
[93] |
Gibby WA, Gibby KA, Gibby WA (2004) Comparison of Gd-DTPA-BMA (Omniscan) versus Gd-HPDO3A (ProHance) retention in human bone tissue by inductively coupled plasma atomic emission spectroscopy. Invest Radiol 39: 138-142. doi: 10.1097/01.rli.0000112789.57341.01
![]() |
[94] |
White GW, Gibby WA, Tweedle MF (2006) Comparison of Gd(DTPA-BMA)(Omniscan) versus Gd(HPDO3A)(ProHance) relative to gadolinium retention in human bone tissue by inductively coupled mass spectroscopy. Invest Radiol 41: 272-278. doi: 10.1097/01.rli.0000186569.32408.95
![]() |
[95] |
Darrah TH, Prutsman-Pfeiffer JJ, Poreda RJ, et al. (2009) Incorporation of excess gadolinium into human bone from medical contrast agents. Metallomics 1: 479-488. doi: 10.1039/b905145g
![]() |
[96] |
Murata N, Gonzalez-Cuyar LF, Murata K, et al. (2016) Macrocyclic and other non–group 1 gadolinium contrast agents deposit low levels of gadolinium in brain and bone tissue: Preliminary results from 9 patients with normal renal function. Invest Radiol 51: 447-53. doi: 10.1097/RLI.0000000000000252
![]() |
[97] |
Lord ML, Chettle DR, Gräfe JL, et al. (2018) Observed deposition of gadolinium in bone using a new noninvasive in vivo biomedical device: Results of a small pilot feasibility study. Radiology 287: 96-103. doi: 10.1148/radiol.2017171161
![]() |
[98] |
Turyanskaya A, Rauwol M, Pichler V, et al. (2020) Detection and imaging of gadolinium accumulation inhuman bone tissue by micro- and submicro-XRF. Sci Rep 10: 6301. doi: 10.1038/s41598-020-63325-9
![]() |
[99] |
Vidaud C, Bourgeois D, Meyer D (2012) Bone as target organ for metals: the case of f-elements. Chem Res Toxicol 25: 1161-1175. doi: 10.1021/tx300064m
![]() |
[100] |
Gräfe JL, McNeill FE (2018) Measurement of gadolinium retention: current status and review from an applied radiation physics perspective. Physiol Meas 39: 06TR01. doi: 10.1088/1361-6579/aacc16
![]() |
[101] |
Hasegawa M, Duncan BR, Marshall DA, et al. (2020) Human hair as a possible surrogate marker of retained tissue gadolinium. A pilot autopsy study correlating gadolinium concentrations in hair with brain and other tissues among decedents who received gadolinium-based contrast agents. Invest Radiol 55: 636-642. doi: 10.1097/RLI.0000000000000681
![]() |
[102] |
Saussereau E, Lacroix C, Cattaneo A, et al. (2008) Hair and fingernail gadolinium ICP-MS contents in an overdose case associated with nephrogenic systemic fibrosis. Forensic Sci Int 176: 54-57. doi: 10.1016/j.forsciint.2007.06.026
![]() |
[103] | US Food and Drug Administration, 5-18, 2015 Available from: https://www.fda.gov/drugs/drug-safety-and-availability/fda-drug-safety-communication-fda-evaluating-risk-brain-deposits-repeated-use-gadolinium-based. |
[104] | EMA/625317/2017. EMA's final opinion confirms restrictions on use of linear gadolinium agents in body scans Available at: http://www.ema.europa.eu/docs/en _ GB/document _ library/ Referrals _ document/gadolinium _ contrast _ agents _ 31/ European _ Commission _ final _ decision/WC500240575.pdf. |
[105] |
Lancelot E, Desché P (2020) Gadolinium retention as a safety signal: experience of a manufacturer. Invest Radiol 55: 20-24. doi: 10.1097/RLI.0000000000000605
![]() |
[106] | PMDA, Revision of Precautions, Gadodiamide hydrate Meglumine gadopentetate, 2017 Available from: http://www.pmda.go.jp/files/000221377.pdf. |
[107] | PMDA, Revision of Precautions, Gadoxetate sodium, Gadoteridol, Meglumine gadoterate, Gadobutrol, 2017 Available from: http://www.pmda.go.jp/files/000221376.pdf. |
[108] |
Kanda T, Nakai Y, Hagiwara A, et al. (2017) Distribution and chemical forms of gadolinium in the brain: a review. Br J Radiol 90: 20170115. doi: 10.1259/bjr.20170115
![]() |
[109] | Bracco Diagnostics Bayer, Guerbet GE Healthcare Important drug warning for all gadolinium-based contrast agents [dear health care provider letter] (2018) .Available from: https://www.guerbet.com/media/uh4h4kon/dhcp-letter-05-02-2018-signed.pdf. |
[110] |
Harvey HB, Gowda V, Cheng G (2019) Gadolinium deposition disease: a new risk management threat. J Am Coll Radiol 17: 546-550. doi: 10.1016/j.jacr.2019.11.009
![]() |
[111] |
Semelka RC, Commander CW, Jay M, et al. (2016) Presumed gadolinium toxicity in subjects with normal renal function a report of 4 cases. Invest Radiol 51: 661-665. doi: 10.1097/RLI.0000000000000318
![]() |
[112] |
Semelka RC, Ramalho M, Jay M (2016) Summary of special issue on gadolinium bioeffects and toxicity with a look to the future. Magn Reson Imaging 34: 1399-1401. doi: 10.1016/j.mri.2016.09.002
![]() |
[113] | US Food and Drug AdministrationMedical Imaging Drugs Advisory Committee Meeting, FDA briefing document: Gadolinium retention after gadolinium-based contrast magnetic resonance imaging in patients with normal renal function, 27–28. (2017) . |
[114] |
Burke LMB, Ramalho M, AlObaidy M, et al. (2016) Self-reported gadolinium toxicity: A survey of patients with chronic symptoms. Magn Reson Imaging 34: 1078-1080. doi: 10.1016/j.mri.2016.05.005
![]() |
[115] |
Semelka RC, Ramalho J, Vakharia A, et al. (2016) Gadolinium deposition disease: initial description of adisease that has been around for a while: a family of disorders. Magn Res Imaging 34: 1383-1390. doi: 10.1016/j.mri.2016.07.016
![]() |
[116] |
Roberts DR, Lindhorst SM, Welsh CT, et al. (2016) High levels of gadolinium deposition in the skin of a patient with normal renal function. Invest Radiol 51: 280-289. doi: 10.1097/RLI.0000000000000266
![]() |
[117] |
Barbieri S, Schroeder C, Froehlich JM, et al. (2016) High signal intensity in dentate nucleus and globus pallidus on unenhanced T1-weighted MR images in three patients with impaired renal function and vascular calcification. Contrast Media Mol Imaging 11: 245-250. doi: 10.1002/cmmi.1683
![]() |
[118] |
Swaminathan S (2016) Gadolinium toxicity: iron and ferroportin as central targets. Magnet Reson Imaging 34: 1373-1376. doi: 10.1016/j.mri.2016.08.016
![]() |
[119] |
Di Gregorio ED, Furlan C, Atlante S, et al. (2020) Gadolinium retention in erithrocytes and leukocytes from human and murine blood upon treatment with gadolinium-based contrast agents for magnetic resonance imaging. Invest Radiol 55: 30-37. doi: 10.1097/RLI.0000000000000608
![]() |
[120] |
Di Gregorio E, Ferrauto G, Furlan C, et al. (2018) The issue of gadolinium retained in tissue. Invest Radiol 53: 167-172. doi: 10.1097/RLI.0000000000000423
![]() |
[121] |
Kartamihardja AAP, Hanaoka H, Andriana P, et al. (2019) Quantitative analysis of Gd in the protein content of the brain following single injection of gadolinium-based contrast agents (GBCAs) by size exclusion chromatography. Br J Radiol 92: 20190062. doi: 10.1259/bjr.20190062
![]() |
[122] |
Newton BB, Jimenez SA (2009) Mechanism of NSF: New evidence challenging the prevailing theory. J Magn Reson Imaging 30: 1277-1283. doi: 10.1002/jmri.21980
![]() |
[123] |
Taoka T, Jost G, Frenzel T, et al. (2018) Impact of the glymphatic system on the kinetic and distribution of gadodiamide in rat brain. Invest Radiol 53: 529-534. doi: 10.1097/RLI.0000000000000473
![]() |
[124] |
Nehra AK, McDonald RJ, Bluhm AM, et al. (2018) Accumulation of gadolinium in human cerebrospinal fluid after gadobutrol-enhanced MR imaging: a prospective observational cohort study. Radiology 288: 416-423. doi: 10.1148/radiol.2018171105
![]() |
[125] |
McDonald RJ, Levine D, Weinreb J, et al. (2018) Gadolinium retention: A research roadmap from the 2018 NIH/ACR/RSNA workshop on gadolinium chelates. Radiology 289: 517-534. doi: 10.1148/radiol.2018181151
![]() |
[126] |
Le Fur M, Caravan P (2019) The biological fate of gadolinium-based MRI contrast agents: a call to action for bioinorganic chemists. Metallomics 11: 240-254. doi: 10.1039/C8MT00302E
![]() |
[127] |
Tweedle MF (2016) Gadolinium deposition: Is it chelated or dissociated gadolinium? How can we tell? Magn Res Imaging 34: 1377-1382. doi: 10.1016/j.mri.2016.09.003
![]() |
[128] |
Kiviniemi A, Gardberg M, Ek P, et al. (2019) Gadolinium retention in gliomas and adjacent normal brain tissue: association with tumor contrast enhancement and linear/macrocyclic agents. Neuroradiology 61: 535-544. doi: 10.1007/s00234-019-02172-6
![]() |
[129] |
Kanda T, Fukusato T, Matsuda M, et al. (2015) Gadolinium-based contrast agent accumulates in the brain even in subjects without severe renal dysfunction: evaluation of autopsy brain specimens with inductively coupled plasma mass spectroscopy. Radiology 276: 228-232. doi: 10.1148/radiol.2015142690
![]() |
[130] |
Herculano-Houzel S (2009) The human brain in numbers: a linearly scaled-up primate brain. Front Hum Neurosci 3: 31. doi: 10.3389/neuro.09.031.2009
![]() |
[131] |
Popescu BFG, Robinson CA, Rajput A, et al. (2009) Iron, copper, and zinc distribution of the cerebellum. Cerebellum 8: 74-79. doi: 10.1007/s12311-008-0091-3
![]() |
[132] |
Kromrey ML, Liedtke KR, Ittermann T, et al. (2017) Intravenous injection of gadobutrol in an epidemiological study group did not lead to a difference in relative signal intensities of certain brain structures after 5years. Eur Radiol 27: 772-777. doi: 10.1007/s00330-016-4418-z
![]() |
[133] |
Staks T, Schuhmann-Giampieri G, Frenzel T, et al. (1994) Pharmacokinetics, dose proportionality and tolerability of gadobutrol after single intravenous injection in healthy volunteers. Invest Radiol 29: 709-715. doi: 10.1097/00004424-199407000-00008
![]() |
[134] |
Gutierrez JE, Rosenberg M, Duhaney M, et al. (2015) Phase 3 efficacy and safety trial of gadobutrol, a 1.0 molar macrocyclic MR imaging contrast agent, in patients referred for contrast-enhanced MR imagingof the central nervous system. J Magn Reson Imaging 41: 788-796. doi: 10.1002/jmri.24583
![]() |
[135] |
Kuwatsuru R, Takahashi S, Umeoka S, et al. (2015) A multicenter, randomized, controlled, single-blind comparison phase III study to determine the efficacy and safety of gadobutrol 1.0 M versus gadopentetate dimeglumine following single injection in patients referred for contrast-enhanced MRI of the body regions or extremities. J Magn Reson Imaging 41: 404-413. doi: 10.1002/jmri.24566
![]() |
[136] | Liang Z, Ma L, Wang D, et al. (2012) Efficacy and safety of gadobutrol (1.0 M) versus gadopentetate dimeglumine (0.5 M) for enhanced MRI of CNS lesions: A phase III, multicenter, single-blind, randomized study in Chinese patients. Mag Res Insights 5: MRI-S9348. |
[137] |
Naito S, Tazaki H, Okamoto T, et al. (2017) Comparison of nephrotoxicity between two gadolinium-contrasts, gadodiamide and gadopentetate in patients with mildly diminished renal failure. J Toxicol Sci 42: 379-384. doi: 10.2131/jts.42.379
![]() |
[138] |
Semelka RC, Hernandes MA, Stallings CG, et al. (2013) Objective evaluation of acute adverse events andimage quality of gadolinium-based contrast agents (gadobutrol and gadobenate dimeglumine) by blinded evaluation. Pilot study. Magn Reson Imaging 31: 96-101. doi: 10.1016/j.mri.2012.06.025
![]() |
[139] |
Tanaka A, Masumoto T, Yamada H, et al. (2016) A Japanese, multicenter, open-label, phase 3 study to investigate the safety and efficacy of gadobutrol for contrast-enhanced MR imaging of the central nervous system. Magn Reson Med Sci 15: 227-236. doi: 10.2463/mrms.mp.2015-0083
![]() |
[140] | Zech CJ, Schwenke C, Endrikat J (2019) Diagnostic efficacy and safety of gadoxetate disodium vs gadobenate dimeglumine in patients with known or suspected focal liver lesions: Results of a clinical phase III study. Magn Reson Insight 12: 1178623X19827976. |
[141] |
Tweedle MF, Wedeking P, Kumar K (1995) Biodistribution of radiolabeled, formulated gadopentetate, gadoteridol, gadoterate, and gadodiamide in mice and rats. Invest Radiol 30: 372-380. doi: 10.1097/00004424-199506000-00008
![]() |
[142] |
Rocklage SM, Worah D, Kim SH (1991) Metal ion release from paramagnetic chelates: what is tolerable? Magn Reson Med 22: 216-221. doi: 10.1002/mrm.1910220211
![]() |
[143] |
Khairinisa MA, Takatsuru Y, Amano I, et al. (2018) The effect of perinatal gadolinium-based contrast agents. Invest Radiol 53: 110-118. doi: 10.1097/RLI.0000000000000417
![]() |
[144] |
Ray JG, Vermeulen MJ, Bharatha A, et al. (2016) Association between MRI exposure during pregnancy and fetal and childhood outcomes. JAMA 316: 952-961. doi: 10.1001/jama.2016.12126
![]() |
[145] |
Runge VM, Kuehl TJ, Jackson CB, et al. (2005) Subchronic toxicity of the gadolinium chelates. Acad Radiol 12: S6-S9. doi: 10.1016/j.acra.2005.02.015
![]() |
[146] |
Alkhunizi SM, Fakhoury M, Abou-Kheir W, et al. (2020) Gadolinium retention in the central and peripheral nervous system: implications for pain, cognition, and neurogenesis. Radiology 297: 407-416. doi: 10.1148/radiol.2020192645
![]() |
[147] |
Wang S, Hesse B, Roman M, et al. (2019) Increased retention of gadolinium in the inflamed brain after repeated administration of gadopentetate dimeglumine. Invest Radiol 54: 617-626. doi: 10.1097/RLI.0000000000000571
![]() |
[148] |
Reimer P, Vosshenrich R (2008) Off-label use of contrast agents. Eur Radiol 18: 1096-1101. doi: 10.1007/s00330-008-0886-0
![]() |
[149] |
Essig M, Shiroishi MS, Nguyen TB, et al. (2013) Perfusion MRI: The five most frequently asked technical questions. Am J Roentgenol 200: 24-34. doi: 10.2214/AJR.12.9543
![]() |
[150] |
Wolansky LJ, Cadavid D, Punia V, et al. (2015) Hypophosphatemia is associated with the serial administration of triple-dose gadolinium to patients for brain MRI. J Neuroimaging 25: 379-383. doi: 10.1111/jon.12241
![]() |
[151] |
Essig M, Giesel E, Le-Huu M, et al. (2004) Perfusion MRI in CNS disease: current concepts. Neuroradiology 46: S201-S207. doi: 10.1007/s00234-004-1331-y
![]() |
[152] |
Lee JY, Park JE, Kim HS, et al. (2017) Up to 52 administrations of macrocyclic ionic MR contrast agent are not associated with intracranial gadolinium deposition: multifactorial analysis in 385 patients. PloS One 12: e0183916. doi: 10.1371/journal.pone.0183916
![]() |
[153] | Ng KH, Ahmad AC, Nizam M, et al.Magnetic resonance imaging: Health effects and safety, Proceedings of the international conference on non-ionizing radiation at UNITEN, Electromagnetic Fields and our Health. (2003) . |
[154] |
Cho S, Lee Y, Choi YJ, et al. (2014) Enhanced cytotoxic and genotoxic effects of gadolinium following ELF-EMF irradiation in human lymphocytes. Drug Chem Toxicol 37: 440-447. doi: 10.3109/01480545.2013.879662
![]() |
[155] |
Sadiq S, Ghazala Z, Chowdhury A, et al. (2012) Metal toxicity at the synapse: presynaptic, postsynaptic, and long-term effects. J Toxicol 2012: 132671. doi: 10.1155/2012/132671
![]() |
[156] | Food U S, Drug Administration, Safety Announcement 2017 Available from: https://www.fda.gov/media/109825/download. |
[157] |
Veiga M, Mattiazzi P, de Goisc JS, et al. (2020) Presence of other rare earth metals in gadolinium-based contrast agents. Talanta 216: 120940. doi: 10.1016/j.talanta.2020.120940
![]() |
[158] |
Parfrey P (2005) The clinical epidemiology of contrast-induced nephropathy. Cardiovasc Intervent Radiol 28: S3-S11. doi: 10.1007/s00270-005-0196-8
![]() |
[159] |
Karcaaltincaba M, Oguz B, Haliloglu M (2009) Current status of contrast-induced nephropathy and nephrogenic systemic fibrosis in children. Pediatr Radiol 39: S382-S384. doi: 10.1007/s00247-009-1236-3
![]() |
[160] |
Kulaksiz S, Bau M (2011) Anthropogenic gadolinium as a micro-contaminant in tap water used as drinking water in urban areas and megacities. Appl Geochem 26: 1877-1885. doi: 10.1016/j.apgeochem.2011.06.011
![]() |
[161] |
Hatje V, Bruland KW, Flegal AR (2016) Increases in anthropogenic gadolinium anomalies and rare earth element concentrations in San Francisco bay over a 20 year record. Environ Sci Technol 50: 4159-4168. doi: 10.1021/acs.est.5b04322
![]() |
[162] |
Rabiet M, Brissaud F, Seidel JL, et al. (2009) Positive gadolinium anomalies in wastewater treatment plant effluents and aquatic environment in the Hérault watershed (South France). Chemosphere 75: 1057-1064. doi: 10.1016/j.chemosphere.2009.01.036
![]() |
[163] |
Chen Y, Cao XD, Lu Y, et al. (2000) Effects of rare earth metal ions and their EDTA complexes on antioxidant enzymes of fish liver. B Environ Contam Tox 65: 357-365. doi: 10.1007/s001280000136
![]() |
[164] |
Henriques B, Coppola F, Monteiro R, et al. (2019) Toxicological assessment of anthropogenic gadolinium in seawater: Biochemical effects in mussels mytilus galloprovincialis. Sci Total Environ 664: 626-634. doi: 10.1016/j.scitotenv.2019.01.341
![]() |
[165] |
Hanana H, Turcotte P, André C, et al. (2017) Comparative study of the effects of gadolinium chloride and gadolinium-based magnetic resonance imaging contrast agent on freshwater mussel, dreissena polymorpha. Chemosphere 181: 197-207. doi: 10.1016/j.chemosphere.2017.04.073
![]() |
[166] |
Martino C, Costa C, Roccheria MC, et al. (2018) Gadolinium perturbs expression of skeletogenic genes, calcium uptake and larval development in phylogenetically distant sea urchin species. Aquat Toxicol 194: 57-66. doi: 10.1016/j.aquatox.2017.11.004
![]() |
[167] |
Schmidt K, Bau M, Merschel G, et al. (2019) Anthropogenic gadolinium in tap water and in tap-based beverages from fast-food franchises in six major cities in Germany. Sci Total Environ 687: 1401-1408. doi: 10.1016/j.scitotenv.2019.07.075
![]() |
Channel | Central wavelength/μm | Detection targets |
1 | 0.46 | aerosol, coastline |
2 | 0.51 | phytoplankton |
3 | 0.64 | vegetation, aerosol |
4 | 0.86 | stratus cloud |
5 | 1.6 | cloud top phase, snow |
6 | 2.3 | surface, cloud, snow |
7 | 3.9 | surface, wildfire |
8 | 6.2 | upper atmospheric water vapor, rainfall |
9 | 7.0 | mid-level atmospheric water vapor, rainfall |
10 | 7.3 | sulfur dioxide |
11 | 8.6 | water, sulfur dioxide, rainfall |
12 | 9.6 | ozone, airflow, wind |
13 | 10.4 | surface, cloud |
14 | 11.2 | surface, cloud |
15 | 12.4 | water, sea surface temperature |
16 | 13.3 | temperature, cloud altitude, cloudiness |
Algorithm | HR | KSS |
Multi-spectral threshold method | 0.763 | 0.572 |
RF | 0.811 | 0.65 |
KNN | 0.753 | 0.606 |
GMM | 0.973 | 0.958 |
Proposed algorithm | 0.975 | 0.973 |
Channel | Central wavelength/μm | Detection targets |
1 | 0.46 | aerosol, coastline |
2 | 0.51 | phytoplankton |
3 | 0.64 | vegetation, aerosol |
4 | 0.86 | stratus cloud |
5 | 1.6 | cloud top phase, snow |
6 | 2.3 | surface, cloud, snow |
7 | 3.9 | surface, wildfire |
8 | 6.2 | upper atmospheric water vapor, rainfall |
9 | 7.0 | mid-level atmospheric water vapor, rainfall |
10 | 7.3 | sulfur dioxide |
11 | 8.6 | water, sulfur dioxide, rainfall |
12 | 9.6 | ozone, airflow, wind |
13 | 10.4 | surface, cloud |
14 | 11.2 | surface, cloud |
15 | 12.4 | water, sea surface temperature |
16 | 13.3 | temperature, cloud altitude, cloudiness |
Algorithm | HR | KSS |
Multi-spectral threshold method | 0.763 | 0.572 |
RF | 0.811 | 0.65 |
KNN | 0.753 | 0.606 |
GMM | 0.973 | 0.958 |
Proposed algorithm | 0.975 | 0.973 |