Special Issue: Machine Learning for Complex Systems Modelling and Control
Guest Editors
Prof. Antonello Rizzi
Information Engineering, Electronics and Telecommunications Department University of Rome "La Sapienza", Italy
Email: antonello.rizzi@uniroma1.it
Dr. Alessio Martino
Institute of Cognitive Sciences and Technologies (ISTC-CNR) - Italian National Research Council, Italy
Email: alessio.martino@istc.cnr.it
Manuscript Topics
Complex systems are everywhere in nature and are by far more common than ‘simple’ ones, which are the true outliers in our world.
In short, a complex system can be characterized by a network of many atomic interacting elements, each of which performs a (usually non-linear) functional role. Dually, a complex system can be viewed as a multi-layered structure where each layer is composed by some sort of aggregation of previous level entities. For example, proteins do interact each other to give rise to an organized metabolism, while at the same time each protein (a single node of such interaction network) is itself a network of interacting amino-acid residues.
Building predictive models of such complex systems is a challenging task, yet of utmost importance for advancements in natural sciences (e.g., physics, biology, neurosciences), as well for achieving technological goals (e.g., cybersecurity, natural language processing, clinical diagnostic systems, precision medicine, smart grids, intelligent transportation systems).
In the last decade, machine learning and computational intelligence techniques emerged as powerful tools in order to model input-output relationships derived from (usually non-linear) processes that are not known in closed form. However, since modelling complexity demands complexity, naïve approaches such as a mere sampling of real-valued measurements and their concatenation in a feature vector are often ineffective as this would lead to a huge information loss which, in turn, might compromise the modelling capabilities.
In this Special Issue, we would like to collect research papers and focused reviews, as well as application-oriented works, on advanced pattern recognition techniques, mostly relying on computational intelligence and machine learning tools, able to deal with complex systems.
Topics of interest include (but are not limited to):
• Machine learning and computational intelligence
• Advanced pattern recognition systems
• Granular Computing
• Machine Learning techniques for structured data (such as graphs, sequences, trees)
• Recurrence Quantification Analysis
• Topological Data Analysis
• Multi-agent Systems and Agent-based Modelling
• Complex dynamical and hierarchical systems
Focused applications can include:
• Bioinformatics and bioengineering
• Precision medicine and clinical diagnostic systems
• Smart grids, micro grids and Intelligent Energy Management
• Intelligent transportation systems
• Natural language processing
• Cybersecurity
• Condition based maintenance
Instructions for authors
http://www.aimspress.com/mbe/news/solo-detail/instructionsforauthors
Please submit your manuscript to online submission system
https://aimspress.jams.pub/
Paper Submission
All manuscripts will be peer-reviewed before their acceptance for publication. The deadline for manuscript submission is 31 October 2021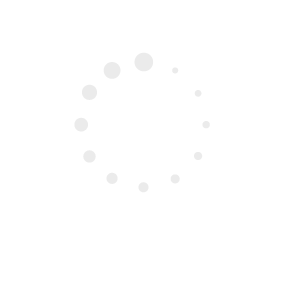