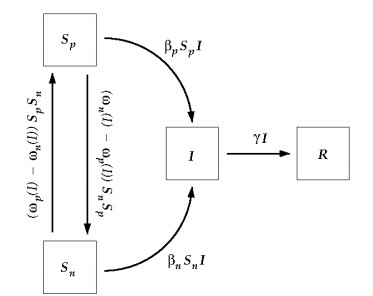
Public opinion and opinion dynamics can have a strong effect on the transmission rate of an infectious disease for which there is no vaccine. The coupling of disease and opinion dynamics however, creates a dynamical system that is complex and poorly understood. We present a simple model in which susceptible groups adopt or give up prophylactic behaviour in accordance with the influence related to pro- and con-prophylactic communication. This influence varies with disease prevalence. We observe how the speed of the opinion dynamics affects the total size and peak size of the epidemic. We find that more reactive populations will experience a lower peak epidemic size, but possibly a larger final size and more epidemic waves, and that an increase in polarization results in a larger epidemic.
Citation: Rebecca C. Tyson, Noah D. Marshall, Bert O. Baumgaertner. Transient prophylaxis and multiple epidemic waves[J]. AIMS Mathematics, 2022, 7(4): 5616-5633. doi: 10.3934/math.2022311
[1] | Tahir Khan, Fathalla A. Rihan, Muhammad Bilal Riaz, Mohamed Altanji, Abdullah A. Zaagan, Hijaz Ahmad . Stochastic epidemic model for the dynamics of novel coronavirus transmission. AIMS Mathematics, 2024, 9(5): 12433-12457. doi: 10.3934/math.2024608 |
[2] | Siriprapa Ritraksa, Chadaphim Photphanloet, Sherif Eneye Shuaib, Arthit Intarasit, Pakwan Riyapan . Mathematical modeling to study the interactions of two risk populations in COVID-19 spread in Thailand. AIMS Mathematics, 2023, 8(1): 2044-2061. doi: 10.3934/math.2023105 |
[3] | Urmee Maitra, Ashish R. Hota, Rohit Gupta, Alfred O. Hero . Optimal protection and vaccination against epidemics with reinfection risk. AIMS Mathematics, 2025, 10(4): 10140-10162. doi: 10.3934/math.2025462 |
[4] | Hany A. Hosham, Thoraya N. Alharthi . Bifurcation and chaos in simple discontinuous systems separated by a hypersurface. AIMS Mathematics, 2024, 9(7): 17025-17038. doi: 10.3934/math.2024826 |
[5] | Abdon Atangana, Mekkaoui Toufik . A piecewise heat equation with constant and variable order coefficients: A new approach to capture crossover behaviors in heat diffusion. AIMS Mathematics, 2022, 7(5): 8374-8389. doi: 10.3934/math.2022467 |
[6] | José Luis Díaz Palencia, Saeed ur Rahman, Antonio Naranjo Redondo . Analysis of travelling wave solutions for Eyring-Powell fluid formulated with a degenerate diffusivity and a Darcy-Forchheimer law. AIMS Mathematics, 2022, 7(8): 15212-15233. doi: 10.3934/math.2022834 |
[7] | Iftikhar Ahmad, Hira Ilyas, Muhammad Asif Zahoor Raja, Tahir Nawaz Cheema, Hasnain Sajid, Kottakkaran Sooppy Nisar, Muhammad Shoaib, Mohammed S. Alqahtani, C Ahamed Saleel, Mohamed Abbas . Intelligent computing based supervised learning for solving nonlinear system of malaria endemic model. AIMS Mathematics, 2022, 7(11): 20341-20369. doi: 10.3934/math.20221114 |
[8] | E. S. Aly, Mahmoud A. E. Abdelrahman, S. Bourazza, Abdullah Ali H. Ahmadini, Ahmed Hussein Msmali, Nadia A. Askar . New solutions for perturbed chiral nonlinear Schrödinger equation. AIMS Mathematics, 2022, 7(7): 12289-12302. doi: 10.3934/math.2022682 |
[9] | José L. Díaz . Existence, uniqueness and travelling waves to model an invasive specie interaction with heterogeneous reaction and non-linear diffusion. AIMS Mathematics, 2022, 7(4): 5768-5789. doi: 10.3934/math.2022319 |
[10] | Abdullahi Yusuf, Tukur A. Sulaiman, Mustafa Inc, Sayed Abdel-Khalek, K. H. Mahmoud . $ M- $truncated optical soliton and their characteristics to a nonlinear equation governing the certain instabilities of modulated wave trains. AIMS Mathematics, 2021, 6(9): 9207-9221. doi: 10.3934/math.2021535 |
Public opinion and opinion dynamics can have a strong effect on the transmission rate of an infectious disease for which there is no vaccine. The coupling of disease and opinion dynamics however, creates a dynamical system that is complex and poorly understood. We present a simple model in which susceptible groups adopt or give up prophylactic behaviour in accordance with the influence related to pro- and con-prophylactic communication. This influence varies with disease prevalence. We observe how the speed of the opinion dynamics affects the total size and peak size of the epidemic. We find that more reactive populations will experience a lower peak epidemic size, but possibly a larger final size and more epidemic waves, and that an increase in polarization results in a larger epidemic.
Infectious disease dynamics, as predicted by classical compartmental models, involve a single epidemic wave that can be prevented if the basic reproduction number R0 is less than 1 [25]. However, such models are of limited use in situations where knowledge of disease can alter behaviour that impacts disease spread. Behaviour-disease models (or disease-behaviour) are intended to address this limitation [18,19,29]. Depending on the model, the behaviour respresented could be vaccination, social distancing, face-mask wearing, or other activities related to prophylaxis.
Behaviour-disease models can be roughly divided into two types, those representing lasting prophylaxis, others transient prophylaxis. Vaccination is an example of lasting prophylaxis because of the long-term protection received from the choice to vaccinate (where "long-term" indicates that protection lasts much longer than the typical time course of the epidemic). Face-mask wearing is an example of transient prophylaxis because the term of protection is nearly identical with the period of choosing it.
For behaviour-disease models with lasting prophylaxis, as exemplified by [20], we expect that "every little bit helps": As we increase the fraction of the population that vaccinates, there is a monotonic decrease in various measures of epidemic severity. In this context there are important questions about how decision making undergirding behaviour affects various thresholds that need to be reached in order to achieve desired levels of lower disease severity.
However, the same monotonic and cumulative patterns do not seem to hold for transient prophylaxis. That is, merely increasing the fraction of the population that engages in prophylaxis for some time is not necessarily associated with decreases in severity, at least as measured by multiple waves of infection. Multiple epidemic waves, or recurrent epidemics, have been observed in models that include a periodic external forcing, e.g., seasonal changes in behaviour [5,8], seasonal variations in temperature or moisture [7], mutation in the pathogen or waning immunity [6], or government lockdowns [26,27] and other management approaches [1]. Indeed, in the current COVID-19 pandemic, several jurisdictions across the globe have experienced multiple epidemic waves [4], brought on, at least in part, by changes in behaviour as governments impose restrictions on movement, and then release them later, in a repeating pattern.
Prior to the COVID-19 pandemic, transient prophylaxis was shown to have non-monotonic effects on epidemic severity. For example, [21] developed models where transient behaviour like social distancing is changed as the result of infection data being spread through media. Transient prophylaxis can significantly reduce epidemic severity for short-term outbreaks, especially when the infectious disease has high morbidity and mortality rates. In scenarios such as these, [21] recommend a strategy where the relevant information is provided as early as possible. However, for long-term outbreaks, the reporting of infection data to the population can result in higher epidemic severity than no reporting at all, as measured by final size (the total number of individuals who contracted the disease during the epidemic).
Another example where transient prophylaxis has non-monotonic effects is given by [24]. They imposed an SIR model on a spatial network where individuals can choose to social distance by dropping connections based on information of infection rates in their 'awareness neighborhood'. They consider that there is a cost to prophylaxis (social distancing), which is weighed against the economic benefit of reducing the impact of the epidemic. They look specifically at parameters related to risk attitudes and size of the awareness neighbourhood. Their finding is that, where final size is concerned, unless control measures are instituted quickly and effectively, it is better to let an epidemic run its course - partial controls that do not succeed at suppressing the epidemic lead to worse outcomes (again, with respect to final size).
Given such non-monotonic effects and their complications on public health decision making, it is worth developing models involving decision-procedures that underly transient prophylaxis. To that end, [28] investigated the role of planning horizons in a decision-theoretic model of self-interested individuals deciding to engage in transient prophylactic behaviour. They found that depending on the frequency with which decisions are made and perceptions regarding the risk of infection, engaging in prophylaxis can prolong epidemics and produce multiple waves of infection. Similar results were obtained by [22], but in their model susceptible individuals decide whether or not to adopt prophylactic behaviour using imitation dynamics in an evolutionary game-theoretic framework. In such a framework, individuals evaluate their risk of infection (and associated costs) by interacting with susceptible, infectious, or removed individuals.
In short, these and related examples explore the non-monotonic effects on disease severity generated by transient prophylaxis and various mechanisms that contribute to the switching of behaviour, including risk perception and awareness of disease information. These mechanisms capture the response of individuals to actual (or perceived) disease metrics, to which they have access via news, social media, or interactions with "neighbours".
We are interested in understanding how multiple waves can arise simply as a consequence of human opinion dynamics interacting with disease dynamics. In such a model, individuals are less prescient than those in decision-theoretic or game-theoretic models, and instead change their behaviour (or not) in response to "conversations" with other individuals, where the influence of prophylactic voices increases with disease prevalence. We believe this is an important contribution to the literature. Modern technology makes the rate of communication of individual opinions extremely fast, making opinion dynamics a potentially significant factor in the time course of epidemics. Moreover, while it is still unknown just how large an impact social media has [11], it can lead to the intensification of "echo chambers", where people primarily listen to (or are presented with, via social media algorithms) opinions that are the same as their own. One of the consequences of this dynamic is that opinions on either side, in this case, prophylaxis or not, can lead to a polarized population that is less responsive to dynamic threats [12,13]. By approaching a behaviour-disease model from the perspective of opinion dynamics, we can capture some aspects of opinion polarization.
As we will show, we find that adding a very simple model for human opinion dynamics to a standard SIR-type compartmental model is enough to get complex epidemic behaviour. In particular, we find that multiple epidemic waves can arise simply as a result of the coupling between disease and opinion dynamics - we need not suppose outside interventions, delayed information, misperception, mutations of the disease, etc.
Our coupled model is both a simplification and an extension of an earlier disease and opinion dynamics model [30]. In this earlier model, susceptible individuals could adopt one of four levels of prophylaxis, with only the highest level reducing transmission sufficiently to make R0<1. The motivation for having multiple groups was to have prophylactic behaviour spread in a way akin to opinion dynamics where groups can exert influence on one another. We were interested in the case where opinions were spread across a spectrum between extremes on either side of an issue. In order to represent extreme and moderate opinions on both sides of the opinion spectrum, we needed a minimum of four groups. Each susceptible group had a transient behaviour associated with prophylaxis that provided some reduction in the spread rate of the disease; diligence in wearing a face-mask is an example of such behaviour. One group was very prophylactic (e.g., wearing a face-mask 100% of the time), another somewhat prophylactic (e.g., wearing a face-mask only indoors), a third somewhat non-prophylactic (e.g., only wearing a face-mask when directly asked to do so), and the fourth completely non-prophylactic (e.g., refusing to wear a face-mask). We assumed that there was no cost to prophylaxis. The challenge presented by this original model [30] is that the use of four susceptible groups leads to a 6th order system of ODEs for which a comprehensive characterization of model behaviour is exceedingly difficult. In the present work we reduce the complexity of the opinion dynamics portion of the model, allowing susceptible individuals only "prophylactic" or "non-prophylactic" states. The two groups still influence one another, allowing us to investigate opinion dynamics with disease dynamics in a simpler framework. In addition to this simplification, the new model has an extension that makes it more behaviourally compelling: We introduce a cost to prophylaxis. This cost means that the prophylactic group tends to revert to being non-prophylactic when disease incidence drops, as we would expect with the wearing of face-masks, for example.
In some respects the nuances of human behaviour are more consistent with the earlier model, e.g., it's unlikely that a population can be cleanly divided into the non-face-mask-wearers and the always-face-mask-wearers, but rather have some levels in between. In other respects, the nuances of human behaviour are more consistent with this new model. Prophylaxis has a cost - wearing a face mask is at the very least less comfortable than not wearing one - and such cost can be a relevant consideration in understanding the interaction between behaviour and disease spread [38]. Nuances regarding representation aside, this newer model is simpler and that simplification allows us a more exhaustive exploration of parameter space and a more thorough understanding of conditions leading to multi-wave epidemic behaviour.
Our model is a classic SIR (Susceptible-Infective-Removed) model with additional compartments for opinions. The compartmental diagram is shown in Figure 1. We assume that recovered individuals are permanently immune. We also assume that the rate at which susceptible individuals are persuaded to transition between the different behaviours (prophylaxis or non-prophylaxis) depends on the prevalence of infection (I) in a nonlinear way as defined by influence functions ωi(I) (discussed in more detail in Section 2.1). The model we present here is both a simplification and an extension of an earlier opinion and disease dynamics model by [30], in which the susceptibles were divided into four subgroups, with increasing degrees of prophylactic or non-prophylactic behaviour. The simplification we assume here is that the population is divided into just two subgroups. Our extension is to include the cost of prophylactic behaviour in the influence function (see Section 2.1).
The state variables in our model are the population proportions of prophylactic susceptibles, Sp, non-prophylactic susceptibles Sn, infectious, I, and removed (dead, or recovered and immune), R. The model equations are written
˙Sp=−βpISp+(ωp(I)−ωn(I))SpSn, | (2.1a) |
˙Sn=−βnISn−(ωp(I)−ωn(I))SpSn, | (2.1b) |
˙I=(βpSp+βnSn−γ)I, | (2.1c) |
˙R=γI. | (2.1d) |
Note that all populations are scaled by the total population, thus, Sp, Sn, I, R are all between 0 and 1, and Sp+Sn+I+R=1. For reference, the parameter and variable descriptions are listed in Table 1. These parameter values are chosen for illustration purposes.
Symbol | Definition | Default Value |
Si | Susceptible subpopulation with opinion i∈n,p | |
I | Infectious population | |
R | Recovered population | |
ωi(I) | Influence of susceptibles with opinion i∈n,p | |
ω0 | Baseline influence when I=0 | 0.1 |
β0 | Standard infection rate | 0.15 |
a−1 | Prophylactic reduction in infection rate | 0.5 |
γ | Recovery rate from infection | 0.1 |
c | Cost of prophylaxis | 0.01 |
k | Half saturation constant of influence functions | 0.1 |
Prophylactic behaviours such as mask wearing, increased hygiene, or social distancing has been shown to significantly reduce the spread of infectious diseases [35,36,37]. We thus assume that Sn and Sp individuals become infected at different rates, denoted βn and βp, respectively. Given that prophylaxis reduces the probability of contracting the disease, we assume
βn=β0,βp=β0a, | (2.2) |
where β0 is the baseline infection rate for the non-prophylactic population, and a>1 is the reduction in the infection rate associated with prophylaxis. Note that in our model we do not represent the mechanisms that cause this reduction.
Susceptible individuals may transition between prophylaxis and non-prophylaxis over time, as a consequence of meaningful interactions through encounters with other susceptible individuals (these are the SpSn terms in (2.1a) and (2.1b)). This updating of opinions and behaviour is moderated by influence. The influence, ωi(I), is a factor that increases the rate of interactions between opposite-minded individuals leading to the adoption of opinion i. For example, the overall rate at which Sj individuals adopt opinion i is given by ωi(I)SiSj, proportional to both the frequency (SiSj) and effectiveness (ωi(I)) of interactions between prophylactic and non-prophylactic individuals.
The influence functions we develop are based on two main properties of human behavioural responses to disease. First, research has shown that attitudes shift towards prophylaxis if the threat of disease is high [9]. Second, when disease prevalence drops, populations tend to revert to familiar, less costly, non-prophylactic behaviours, though the rate of reversion varies from one jurisdiction to another [14,39]. These two behaviours are evident in the [40] opinion poll, which asks Americans about their fear of the Coronavirus. This graph appears to be high when disease is high and low when disease is low. There was a dip in fear along with the dip in cases seen mid-summer 2020. We therefore assume that the influence exerted by Sp and Sn individuals, ωp and ωn respectively, is affected by the level of infection I. In particular, we take ωp(I) to be a monotonically increasing function of I, and ωn(I) to be a monotonically decreasing function of I. Second, we assume an 'influence cost of prophylaxis', ωn(0)−ωp(0)=c>0, which means that non-prophylactic influence dominates when there is no disease present, and so the population tends to revert to non-prophylaxis. This quantity, c, captures the cost of, for example, waiting in line for one's turn to enter a store, purchasing and washing masks, or giving up social activities involving large gatherings.
We use saturating influence functions developed in previous work [30], with the additional cost term c:
ωp(I)=ω0(1+Ik+I)−c, | (2.3a) |
ωn(I)=ω0(1Ik+1). | (2.3b) |
Note that in Equations 2.1, the influence functions do not appear separately but always as their difference, which is given by
ωp(I)−ωn(I)=ω0(1+Ik+I−1Ik+1)−c. | (2.4) |
Figure 2 shows the two individual influence functions and their difference for the default parameter values. From (2.4) we can infer that increasing ω0 will cause the positive section of the ωp−ωn curve (the portion above the axis) to increase, and the negative section to decrease. That is, the net rate of opinion change increases as ω0 increases.
We require c≤ω0 to prevent negative influence in the absence of disease. We assume that this influence cost of prophylaxis is fixed. Increasing ω0 increases the baseline reactivity of each subpopulation to the opposing opinion; the rate at which susceptibles become prophylactic in the presence of disease is also increased. The difference ωp(I)−ωn(I) determines the net rate at which susceptibles move into the prophylactic subpopulation. The disease level at which the net rate of adoption of prophylaxis becomes positive is given by
ωn(Icross)=ωp(Icross)⇔Icross=ck2ω0−c. | (2.5) |
Thus, for I<Icross, the prophylactic subpopulation tends to zero, and for I>Icross, the non-prophylactic subpopulation tends to zero. From (2.5) we see that that increasing ω0 decreases Icross, resulting in a lower threshold for prophylactic response. Similarly, increasing c or k increases Icross.
As the susceptible population in our model is divided into two groups with different infection rates, the transmissibility of the disease depends on the subdivision of susceptibles into prophylactic and non-prophylactic proportions. The effective reproductive number is thus
reff(t)=βnSn(t)+βpSp(t)γ. | (2.6) |
When reff>1 the disease will spread, and when reff<1 the disease will die out. By design, the influence cost of prophylaxis will cause the susceptible population to tend towards non-prophylaxis in the time limit. Herd immunity is achieved when the susceptible subpopulations are sufficiently small. Since we have assumed a closed population, this criterion is equivalent to stating that the removed population is sufficiently large. Since the susceptible population reverts to non-prophylaxis when disease prevalence is low, we determine the necessary size of R for herd immunity assuming the entire susceptible population is non-prophylactic. We thus arrive at the herd immunity threshold [25]
R∗=1−γβn. | (2.7) |
If R≥R∗ herd immunity will be achieved and any infectious individuals remaining in the population cannot initiate another epidemic wave.
To solve the system dynamics we use the ode45 solver in [32]. The population is initialized with I(0)=0.01, and a 99:1 non-prophylactic:prophylactic majority. The parameter values used are listed in Table 1. We explore how adjusting the timing and speed of the prophylactic response, via ω0, affect the course of the epidemic.
We find that multiple epidemic waves can arise simply as a consequence of the interaction between the disease and opinion dynamics, where the number of waves is determined by the relative rates of the disease and opinion dynamics. Figure 3 shows the nonlinear relationship between the number of epidemic waves and increasing values of the two basic rates, β0 and ω0. We see that for fast enough disease dynamics (β0 large enough), the opinion dynamics cannot respond quickly enough to change the course of the epidemic, and there is a single wave. For slow enough disease dynamics, there is no wave at all: This scenario corresponds to the situation where reff<1 even in the absence of opinion dynamics. For intermediate values of β0 however, opinion dynamics can respond to infection fast enough to alter the course of the epidemic. For our default parameter values we see up to four epidemic waves before the disease ultimately dies out.
Multiple waves occur when the reactivity of the population to increasing disease prevalence is fast enough to ensure that herd immunity is not achieved after the first wave. Figure 4 illustrates this behaviour. In the top plot, we see a simple epidemic with a single wave and herd immunity achieved (exactly, for the parameter values shown). In the middle and bottom plots, the speed of the opinion dynamics is increased relative to the disease dynamics by increasing ω0 and holding β0 at the default value. In other words, the susceptible population is more responsive to the increase in disease prevalence, and shifts more quickly to prophylaxis. In these scenarios, herd immunity is not achieved after the first wave, and so a second epidemic wave is observed.
New waves of disease are created as follows. First, the initial epidemic wave must infect some proportion of the population less than that required for herd immunity. During this initial infection, opinions in the susceptible population shift towards prophylaxis, reducing disease spread and decreasing reff(t). After infection and switching to prophylaxis have sufficiently reduced the size of the non-prophylactic susceptible population, Sn, reff<1 and the first wave dies out. Once I<Icross however, the susceptible population will eventually revert to non-prophylaxis. When a sufficiently large proportion of the population has become non-prophylactic, once again reff>1 (since herd immunity was not achieved through the first wave), and the infection grows again. The result is a second epidemic wave.
Two different types of second wave are possible, shown in the middle and bottom plots of Figure 4. In the case ω0=0.14, the second wave is small enough so that the Icross threshold is not crossed by the increasing I subpopulation, and the susceptible population remains largely non-prophylactic. In this case, there is no shift of opinions towards prophylaxis, and the epidemic dies out simply because the non-prophylactic population is reduced through transition to the infectious class. If the speed of the opinion dynamics is increased to ω0=0.2 however, the second epidemic wave is much larger, with I overshooting Icross and the reduction in the non-prophylactic subpopulation achieved through transition to both the prophylactic as well as the infectious subpopulations. In both cases (ω0=0.14 and 0.2), the removed population that remains after the second wave exceeds the herd immunity threshold, and so the second wave is the final one.
A key measure of an epidemic's impact is its final size. To determine final size, we ran simulations to steady state, that is, to the point where there were no more epidemic waves and the population values were constant. A comparison of the final size in the single and two-wave epidemics in Figure 4 shows that the final size of the epidemic increases with increasing responsiveness of the non-prophylactic subpopulation. Further investigation of this relationship, however, reveals that final size is not a monotonic function of responsiveness (Figure 5, top), but is instead almost periodic in nature. Final size decreases with increasing responsiveness (ω0) until the opinion dynamics are fast enough to conquer the epidemic before herd immunity is achieved. Then, as the population reverts to non-prophylaxis, a second epidemic wave occurs, resulting in an increase in the final size of the epidemic. Final size then initially increases with increasing responsiveness, as the size of the first wave is further reduced, leading to a larger second wave. Eventually, however, the speed of the opinion dynamics becomes fast enough to reduce the size of both waves to the point where even after two epidemic waves herd immunity has not been achieved. Then, a third wave occurs and final size increases yet again.
We point out here that the smallest possible epidemic is achieved with a single wave with the correct prophylactic response rate. That is, none of the subsequent minima in final size are less than the first one (Figure 5). Thus, the prophylaxis adoption rate that leads to the smallest total epidemic is the one that results in a single wave which is of a size just exactly large enough to induce herd immunity in the population. The reason it takes ever faster opinion dynamics to reduce the final size resulting from additional disease waves, is that the later waves are smaller in size. Consequently, the I population is smaller, and the non-prophylactic subpopulation needs to be sensitive to ever smaller I values in order for the switch to prophylaxis to occur quickly enough to stop the increasing, but small, disease prevalence.
If we investigate final epidemic size as a function of both responsiveness and infectiousness, that is, over the (ω0,β0) plane, we find that the observations made above continue to hold (Figure 6). For any particular opinion dynamics response rate ω0, increasing the speed of the disease dynamics increases the final size of the epidemic (except, perhaps, with the exception of the ω0=0.2 case). For fixed infectiousness (β0) however, increasing the speed of the opinion dynamics can result in an increase or a decrease in final size. This nonmonotonicity is most prevalent at the intermediate values of β0 we investigated in Figure 5. In contrast to final size, peak size (Figure 6) decreases monotonically with increased rates of prophylaxis in response to disease prevalence. It should be noted that peak size refers to the maximum value of the infectious population at any time and across waves. In our model, this peak will always occur in the first wave.
A strongly polarized population is one in which there are few individuals with centrist opinions, and the remaining population is divided fairly evenly between the two extremes of the attitude spectrum [17]. The advent of social media has increased polarization in societies [16] on many topics including ones related to disease transmission. Polarization on issues such as mask-wearing likely has strong effects on disease transmission, and so we ask here how polarization affects epidemic dynamics.
In our two-attitude model we do not have a measure of opinion intensity, and so do not have access to traditional distributional measures of polarization. Nevertheless, we can explore polarization as characterised by a very slow rate of change of opinions from one side to the other. In our model, decreasing the rate at which opinions change, from non-prophylaxis to prophylaxis, corresponds to either increasing the cost c of adopting prophylactic behaviour, or decreasing the rate of responsiveness to rising infection levels by increasing k. We investigate both approaches to slowing the rate of change of opinions.
We see in Figure 7 that peak and final size both increase with increasing polarization, under either mechanism. Both measures of epidemic severity can be significantly decreased by having a larger proportion of initially prophylactic individuals. The non-monotonic behaviour for final size in the Sp(0)=0.6 case is due to the development of a second epidemic wave: The Sp(0)=0.4 and 0.5 cases only have one epidemic wave.
The importance of human behaviour in shaping epidemics has been in evidence during the COVID-19 pandemic. The differences in patterns of disease prevalence across jurisdictions is at least partly due to differences in the rate at which non-pharmaceutical prophylactic behaviours are adopted [15]. We cannot use the data [39] to obtain fitted values for ω0, as there is the confounding effect of government regulations mandating masks in many places and at different times. Nonetheless, the data are useful to indicate that the timescales of infection and opinion dynamics can be similar.
The current COVID-19 pandemic confirms our prediction that opinion dynamics coupled with disease dynamics can result in multiple epidemic waves, a phenomenon observed in numerous countries around the world [4]. These waves reflect opinion dynamics of individual citizens, and of countries as a whole as represented by public health restrictions mandated by governments. We do not, however, observe decreasing peak size in the global data. A number of factors not included in our model could contribute to increasing peak size: pandemic fatigue, the advent of more contagious variants, and inconsistencies in the ways in which governments imposed restrictions on the movement of their citizens. The differences between our predictions and the COVID-19 data indicate that these additional factors have a strong effect.
In this paper we explored the range of epidemic behaviours that are possible with a simple model that couples opinion and disease dynamics, as well as some of the counter-intuitive implications of faster and slower opinion dynamics. One interesting example we find is a plausible range of parameter settings where there are multiple waves of infection. Roughly, if responsiveness to disease spread is too low and prophylactic behavior is adopted too slowly, we see a single large outbreak as one does in a typical model of epidemic curves. If responsiveness is sufficiently fast, however, we can see multiple outbreaks: the opinion dynamics that allow prophylactic behaviour to be adopted quickly also allow a rapid return to non-prophylactic behaviour, potentially leading to a feedback loop that seems characteristic of many behaviour disease models. The primary driver seems to be the timing of behavioural changes relative to disease spread. The basic idea is that multiple waves can occur when the coupled dynamics allow for a feedback process that has roughly the following steps: i) increase in disease spread, which leads to ii) adoption of prophylactic behavior, which leads to iii) decrease in disease spread, which leads to iv) reversion to non-prophylactic behavior, which, if enough susceptibles remain in the population, leads back to the conditions under which (i) can occur.
Our model and results are an important addition to other possible factors that can contribute to these timing effects, including external interventions [31], as well as internal considerations, such as risk perception and memory [23], planning horizons [28], and strategic decision-making [22]. In fact, the wave patterns we obtain are similar but simpler than the ones observed by [22]: Each epidemic peak is followed by an almost disease-free interval before emergence of the following peak.
Unlike these other examples, however, our model is also able to capture some aspects of opinion polarization. We do this by slowing the rate of opinion dynamics relative to disease dynamics. While some nuances are lost by representing opinions as binary (contra our previous model), the inclusion of such details are unlikely to change our qualitative finding that polarization means larger epidemics as measured by both peak and final sizes.
Reflecting more broadly, we know that different disease models can play different roles in informing public health. For one, they can be used to predict and forecast disease spread, without the need for physical interventions or observations [33]. For another, they can provide a bridge between patterns identified by theory and empirical measurement, as exemplified by the well known example of how rates of disease processes, like transmission and recovery, relate to the basic reproductive number, which roughly represents the average number of secondary infections per infectious individual [25,34].
In our case, the most relevant contribution is a better understanding of how (transient) behaviours can have differential non-monotonic effects across important epidemic measures. For example, consider the difference between peak size, final size, and the number of epidemic waves. From the perspective of hospitals that have constraints on how many beds they can provide at any given time, peak size will play a dominant role in sustaining health systems from collapse [2]. Even if the final size is relatively large, as long as hospitals can provide the relevant care during the peak(s) of an epidemic, hospitals can avoid triage and related disasters (e.g., having to turn away patients), so even partial controls are benefical from this perspective. From the perspective of government that is concerned with general welfare of its population, the final size of an epidemic can be a reflection of the efficacy of the government's ability to address a health disaster. Such considerations can be particularly important during election years, as sitting governments can be scrutinized in this regard by non-sitting candidates. Finally, multiple waves can be important to perspectives that focus on public health messaging related to health policies. Compliance to policies that require wearing face-masks, for example, is more easily achieved when norms and buy-in from the populace can develop. But multiple waves, in both disease and behavior, can lead to confusion and behavioural fatigue, which in turn can lead to lower levels of compliance. What behaviour-disease models like ours show is that interventions that can impact transient prophylaxis may not have simple downstream consequences, particularly in contrast to lasting prophylaxis. As a result, extra care has to be taken when assessing interventions when considering transient prophylaxis in comparison to the lasting prophylaxis counterparts.
A logical next step is to parameterize this model for specific diseases and specific populations (using, for example, user-initiated data streams [3]), and quantify its ability to predict or explain the disease dynamics. Just as the parameters characterising different diseases can vary widely, we expect that populations from different cultures around the world, and under different styles of leadership, will also respond very differently to the threat of disease. Being able to anticipate how a disease will progress in one country, could help its neighbours prepare more effectively. This work would also involve estimating the timing between epidemic waves. While we left this question unanalysed in the current study, our results in Figure 4 suggest that the inter-wave interval will vary with each subsequent wave.
Another direction to explore is the role of social media. In addition to the rapid communication of individual opinions across large geographic scales, and the intensification of polarization processes, it also facilitates the spread of misinformation [10]. This last feature is a factor that is worth investigating in future work.
Our model and approach is not without limitations. As an ODE it assumes a well mixed population. Real populations are not well mixed and not deterministic: The spatial configuration of a population and somewhat stochastic interactions alter the disease and opinion spread dynamics. So a natural direction for future work is to investigate how our results are impacted by the introduction of social structure and stochasticity. Our model is also only valid in the short term, as we ignore births, deaths, and waning immunity.
In summary, many epidemic models assume that human behaviour is fixed, or that it varies stepwise as a result of government intervention, and model the ensuing evolution of the epidemic. It is clear, however, that human behavioural responses to disease incidence vary continuously, especially when there is continuous media coverage of the epidemic, and that this constantly changing behaviour has a significant effect on the progress of the epidemic. On the other hand, if behaviour doesn't change, as in some polarized populations, there will be needless disease burden and, for a disease that can be fatal, needless deaths. A concerted effort to study the interaction of disease and opinion dynamics, and control efforts that take both into account, are badly needed.
This work was supported by (RCT) the NSERC Discovery Grant program (RGPIN-2016-05277). NDM had just finished his undergraduate degree when this work was done. RCT and BOB initiated the project, NDM did most of the code-writing and simulations, and wrote the first draft of the manuscript. RCT ran final simulations, and both BOB and RCT wrote the final draft.
All authors declare that there is no interests in this paper.
[1] |
S. Ghosh, J. Heffernan, Influenza Pandemic Waves under Various Mitigation Strategies with 2009 H1N1 as a Case Study, PLoS One, 5 (2010), e14307. http://dx.doi.org/10.1371/journal.pone.0014307 doi: 10.1371/journal.pone.0014307
![]() |
[2] | A. R. Tuite, D. N. Fisman, A. L. Greer, Mathematical modelling of COVID-19 transmission and mitigation strategies in the population of Ontario, Canada, Cmaj, 192 (2020), E497–E505. |
[3] |
B. M. Althouse, S. V. Scarpino, L. A. Meyers, J. W. Ayers, M. Bargsten, J. Baumbach, et al., Enhancing disease surveillance with novel data streams: challenges and opportunities, EPJ Data Sci., 4 (2015), 1–8. http://dx.doi.org/10.1140/epjds/s13688-015-0054-0 doi: 10.1140/epjds/s13688-015-0054-0
![]() |
[4] | M. Roser, H. Ritchie, E. Ortiz-Ospina, J. Hasell, Coronavirus Pandemic (COVID-19), Our World in Data, 2020. Available from: https://ourworldindata.org/covid-cases |
[5] |
T. Oraby, O. Vasilyeva, D. Krewski, F. Lutscher, Modeling seasonal behavior changes and disease transmission with application to chronic wasting disease, J. Theor. Biol., 340 (2014), 50–59. http://dx.doi.org/10.1016/j.jtbi.2013.09.003 doi: 10.1016/j.jtbi.2013.09.003
![]() |
[6] |
A. Mummert, H. Weiss, L.-P. Long, J. M. Amigo, X.-F. Wan, A Perspective on Multiple Waves of Influenza Pandemics, PLoS One, 8 (2013), e60343. http://dx.doi.org/10.1371/journal.pone.0060343 doi: 10.1371/journal.pone.0060343
![]() |
[7] |
N. C. Grassly, C. Fraser, Seasonal infectious disease epidemiology, P. Roy. Soc. B - Biol. Sci., 273 (2006), 2541–2550. http://dx.doi.org/10.1098/rspb.2006.3604 doi: 10.1098/rspb.2006.3604
![]() |
[8] |
W. P. London, J. A. Yorke, Recurrent outbreaks of measles, chickenpox, and mumps.1. Seasonal-variation in contact rates, Am. J. Epidemiol., 98 (1973), 453–468. http://dx.doi.org/10.1093/oxfordjournals.aje.a121575 doi: 10.1093/oxfordjournals.aje.a121575
![]() |
[9] |
D. Glabska, D. Skolmowska, D. Guzek, Population-based study of the influence of the COVID-19 pandemic on hand hygiene behaviors - Polish adolescents' COVID-19 experience (PLACE-19) study, Sustainability, 12 (2020), 4930. https://doi.org/10.3390/su12124930 doi: 10.3390/su12124930
![]() |
[10] | C. O'Connor, J. O. Weatherall, The misinformation age: How false beliefs spread, Yale University Press, 2019. https://doi.org/10.2307/j.ctv8jp0hk |
[11] |
E. Dubois, G. Blank, The echo chamber is overstated: the moderating effect of political interest and diverse media, Inf., commun. soc., 21 (2018), 729–745. https://doi.org/10.1080/1369118X.2018.1428656 doi: 10.1080/1369118X.2018.1428656
![]() |
[12] |
D. M. Kahan, A risky science communication environment for vaccines, Science, 342 (2013), 53–54. https://doi.org/10.1126/science.1245724 doi: 10.1126/science.1245724
![]() |
[13] |
B. Baumgaertner, B. J. Ridenhour, F. Justwan, J. E. Carlisle, C. R. Miller, Risk of disease and willingness to vaccinate in the United States: A population-based survey, PLoS med., 17 (2020), e1003354. https://doi.org/10.1371/journal.pmed.1003354 doi: 10.1371/journal.pmed.1003354
![]() |
[14] |
I. C.-H. Fung, S. Cairncross, How often do you wash your hands? A review of studies of hand-washing practices in the community during and after the SARS outbreak in 2003, Int. J. Environ. Heal. R., 17 (2007), 161–183. https://doi.org/10.1080/09603120701254276 doi: 10.1080/09603120701254276
![]() |
[15] |
S. Jamshidi, M. Baniasad, D. Niyogi, Global to USA country scale analysis of weather, urban density, mobility, homestay, and mask use on COVID-19, Int. J. Env. Res. Pub. Heal., 17 (2020), 7847. http://dx.doi.org/10.3390/ijerph17217847 doi: 10.3390/ijerph17217847
![]() |
[16] |
A. Matakos, E. Terzi, P. Tsaparas, Measuring and moderating opinion polarization in social networks, Data Min. Knowl. Disc., 31 (2017), 1480–1505. https://doi.org/10.1007/s10618-017-0527-9 doi: 10.1007/s10618-017-0527-9
![]() |
[17] |
J.-M. Esteban, D. Ray, On the mesaurement of polarization, econometrica, 62 (1994), 819–851. https://doi.org/10.2307/2951734 doi: 10.2307/2951734
![]() |
[18] | P. Manfredi, A. d'Onofrio, Modeling the Interplay Between Human Behavior and the Spread of Infectious Diseases, Springer, New York, 2017. http://dx.doi.org/10.1007/978-1-4614-5474-8 |
[19] |
N. Perra, D. Balcan, B. Gonçalves, A. Vespignani, Towards a characterization of behavior-disease models, PloS one, 6 (2011), e23084. https://doi.org/10.1371/journal.pone.0023084 doi: 10.1371/journal.pone.0023084
![]() |
[20] |
L. Mao, Predicting self-initiated preventive behavior against epidemics with an agent-based relative agreement model, J. Artif. Soc. Social S., 18 (2015), 6. https://doi.org/10.18564/jasss.2892 doi: 10.18564/jasss.2892
![]() |
[21] |
A. Mummert, H. Weiss, Get the news out loudly and quickly: the influence of the media on limiting emerging infectious disease outbreaks, PloS one, 8 (2013), e71692. https://doi.org/10.1371/journal.pone.0071692 doi: 10.1371/journal.pone.0071692
![]() |
[22] |
P. Poletti, B. Caprile, M. Ajelli, A. Pugliese, S. Merler, Spontaneous behavioural changes in response to epidemics, J. Theor. Biol., 260 (2009), 31–40. https://doi.org/10.1016/j.jtbi.2009.04.029 doi: 10.1016/j.jtbi.2009.04.029
![]() |
[23] |
P. Poletti, M. Ajelli, S. Merler, Risk perception and effectiveness of uncoordinated behavioral responses in an emerging epidemic, Math. Biosci., 238 (2012), 80–89. https://doi.org/10.1016/j.mbs.2012.04.003 doi: 10.1016/j.mbs.2012.04.003
![]() |
[24] |
S. Maharaj, A. Kleczkowski, Controlling epidemic spread by social distancing: Do it well or not at all, BMC Public Health, 12 (2012), 1–16. https://doi.org/10.1186/1471-2458-12-679 doi: 10.1186/1471-2458-12-679
![]() |
[25] | M. Martcheva, An Introduction to Mathematical Epidemiology, Vol. 61, Springer, 2015. https://doi.org/10.1007/978-1-4899-7612-3 |
[26] |
S. C. Anderson, A. M. Edwards, M. Yerlanov, N. Mulberry, J. E. Stockdale, S. A. Iyaniwura, et al., Estimating the impact of COVID-19 control measures using a Bayesian model of physical distancing, medRxiv, 2020. https://doi.org/10.1101/2020.04.17.20070086 doi: 10.1101/2020.04.17.20070086
![]() |
[27] |
N. M. Ferguson, D. Laydon, G. Nedjati-Gilani, N. Imai, K. Ainslie, M. Baguelin, et al., Impact of non-pharmaceutical interventions (NPIs) to reduce COVID-19 mortality and healthcare demand, Imperial College London, (2020). https://doi.org/10.25561/77482 doi: 10.25561/77482
![]() |
[28] |
L. G. Nardin, C. R. Miller, B. J. Ridenhour, S. M. Krone, P. Joyce, B. O. Baumgaertner, Planning horizon affects prophylactic decision-making and epidemic dynamics, PeerJ, 4 (2016), e2678. https://doi.org/10.7717/peerj.2678 doi: 10.7717/peerj.2678
![]() |
[29] |
D. Weston, K. Hauck, R. Amlôt, Infection prevention behaviour and infectious disease modelling: a review of the literature and recommendations for the future, BMC Public Health, 18 (2018), 336–351. https://doi.org/10.1186/s12889-018-5223-1 doi: 10.1186/s12889-018-5223-1
![]() |
[30] |
R. C. Tyson, S. D. Hamilton, A. S. Lo, B. O. Baumgaertner, S. M. Krone, The Timing and Nature of Behavioural Responses Affect the Course of an Epidemic, B. Math. Biol., 82 (2020), 1–28. https://doi.org/10.1007/s11538-019-00684-z doi: 10.1007/s11538-019-00684-z
![]() |
[31] |
A. K. Misra, R. K. Rai, Y. Takeuchi, Modeling the control of infectious diseases: Effects of TV and social media advertisements, Math. Biosci. Eng., 15 (2018), 1315–1343. https://doi.org/10.3934/mbe.2018061 doi: 10.3934/mbe.2018061
![]() |
[32] | MATLAB, 9.8.0.1323502 (R2020a), The MathWorks Inc., Natick, Massachusetts, 2020. |
[33] |
M. J. Keeling, L. Danon, Mathematical modelling of infectious diseases, Brit. Med. Bull., 92 (2009), 33–42. https://doi.org/10.1093/bmb/ldp038 doi: 10.1093/bmb/ldp038
![]() |
[34] |
A. Huppert, G. Katriel, Mathematical modelling and prediction in infectious disease epidemiology, Clin. Microbiol. Infec., 19 (2013), 999–1005. https://doi.org/10.1111/1469-0691.12308 doi: 10.1111/1469-0691.12308
![]() |
[35] |
M. W. Fong, H. Gao, J. Y. Wong, J. Xiao, E. Y. C. Shiu, S. Ryu, et al., Nonpharmaceutical Measures for Pandemic Influenza in Nonhealthcare Settings—Social Distancing Measures, Emerg. Infect. Dis., 26 (2020), 976–984. https://doi.org/10.3201/eid2605.190995 doi: 10.3201/eid2605.190995
![]() |
[36] |
A. E. Aiello, R. M. Coulborn, V. Perez, E. L. Larson, Effect of Hand Hygiene on Infectious Disease Risk in the Community Setting: A Meta-Analysis, Am. J. Public Health, 98 (2008), 1372–1381. https://doi.org/10.2105/ajph.2007.124610 doi: 10.2105/ajph.2007.124610
![]() |
[37] |
T. Jefferson, R. Foxlee, C. Del Mar, L. Dooley, E. Ferroni, B. Hewak, et al., Physical interventions to interrupt or reduce the spread of respiratory viruses: systematic review, BMJ, 336 (2007), 77–80. https://doi.org/10.1136/bmj.39393.510347.be doi: 10.1136/bmj.39393.510347.be
![]() |
[38] | F. Chen, A. Griffith, A. Cottrell, Y.-L. Wong, Behavioral Responses to Epidemics in an Online Experiment: Using Virtual Diseases to Study Human Behavior, PLoS ONE, 8 (2013), e52814. https://doi.org/10.1371/journal.pone.0052814 |
[39] | YouGov, Personal measures taken to avoid COVID-19, 2020. Available from: https://today.yougov.com/topics/international/articles-reports/2020/03/17/personal-measures-taken-avoid-covid-19 |
[40] | AP-NORC, Coronavirus Concerns on the Rise Again Amid Spike in New Cases, 2020. Available from: https://apnorc.org/projects/coronavirus-concerns-on-the-rise-again-amid-spike-in-new-cases/?doing_wp_cron=1598305841.2085039615631103515625 |
1. | Jeta Molla, Suzan Farhang-Sardroodi, Iain R. Moyles, Jane M. Heffernan, Pharmaceutical and non-pharmaceutical interventions for controlling the COVID-19 pandemic, 2023, 10, 2054-5703, 10.1098/rsos.230621 | |
2. | Chénangnon Frédéric Tovissodé, Bert Baumgaertner, Heterogeneous risk tolerance, in-groups, and epidemic waves, 2024, 10, 2297-4687, 10.3389/fams.2024.1360001 | |
3. | Ann Osi, Navid Ghaffarzadegan, A simultaneous simulation of human behavior dynamics and epidemic spread: A multi-country study amidst the COVID-19 pandemic, 2025, 380, 00255564, 109368, 10.1016/j.mbs.2024.109368 | |
4. | Avneet Kaur, Rebecca C. Tyson, Iain R. Moyles, The Impact of Fear and Behavior Response to Established and Novel Diseases, 2025, 85, 0036-1399, 687, 10.1137/24M1670718 |
Symbol | Definition | Default Value |
Si | Susceptible subpopulation with opinion i∈n,p | |
I | Infectious population | |
R | Recovered population | |
ωi(I) | Influence of susceptibles with opinion i∈n,p | |
ω0 | Baseline influence when I=0 | 0.1 |
β0 | Standard infection rate | 0.15 |
a−1 | Prophylactic reduction in infection rate | 0.5 |
γ | Recovery rate from infection | 0.1 |
c | Cost of prophylaxis | 0.01 |
k | Half saturation constant of influence functions | 0.1 |
Symbol | Definition | Default Value |
Si | Susceptible subpopulation with opinion i∈n,p | |
I | Infectious population | |
R | Recovered population | |
ωi(I) | Influence of susceptibles with opinion i∈n,p | |
ω0 | Baseline influence when I=0 | 0.1 |
β0 | Standard infection rate | 0.15 |
a−1 | Prophylactic reduction in infection rate | 0.5 |
γ | Recovery rate from infection | 0.1 |
c | Cost of prophylaxis | 0.01 |
k | Half saturation constant of influence functions | 0.1 |