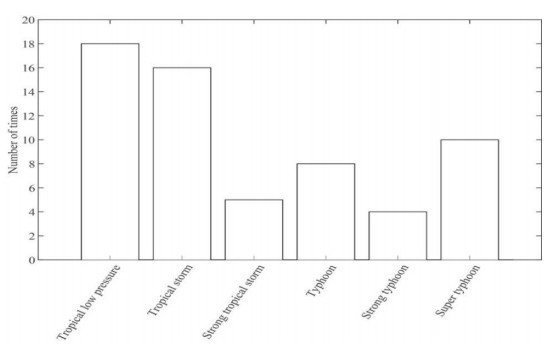
Most of the offshore projects will be impacted by storm surge, especially in the sea area where typhoons are frequent, and storm surge disasters are inevitable. The establishment of a theoretical distribution model of the number of typhoon occurrences and random waves is of great theoretical and practical significance for the study of the number of storm surge impacts and damage to marine engineering as well as the safety evaluation of offshore engineering. Based on stochastic process theory, this paper discusses the effects of the number of typhoon occurrences and typhoon intensity on wave displacement and water level by constructing a compound Poisson process model of typhoon frequency and typhoon intensity. The limitations of using typhoon intensity and wave height as random variables in the marine environment are improved. The average cumulative damage, reliability, and average service life of marine works were analyzed by marine works reliability analysis and a compound Poisson process of the number of storm surge impacts on marine works and the damage caused to marine works by each impact. The results show that the statistical theoretical model and reliability analysis of the storm surge hazard factors based on the stochastic process covers the original extreme value statistical distribution model, and can determine the parameters in the model according to different thresholds in line with the objective facts, and then deduce the design return level, while the deduced return level still has a certain degree of reliability in the case of short or missing data. Therefore, the stochastic process-based model of the imputed level of design parameters for the marine environment provides a new option for marine engineering design and risk management.
Citation: Baiyu Chen, Yi Kou, Yufeng Wang, Daniel Zhao, Shaoxun Liu, Guilin Liu, Liping Wang, Xuefeng Han. Analysis of storm surge characteristics based on stochastic process[J]. AIMS Mathematics, 2021, 6(2): 1177-1190. doi: 10.3934/math.2021072
[1] | Antonio Di Crescenzo, Alessandra Meoli . On a fractional alternating Poisson process. AIMS Mathematics, 2016, 1(3): 212-224. doi: 10.3934/Math.2016.3.212 |
[2] | Noorah Mshary, Hamdy M. Ahmed, Ahmed S. Ghanem . Existence and controllability of nonlinear evolution equation involving Hilfer fractional derivative with noise and impulsive effect via Rosenblatt process and Poisson jumps. AIMS Mathematics, 2024, 9(4): 9746-9769. doi: 10.3934/math.2024477 |
[3] | Leonid Shaikhet . About one unsolved problem in asymptotic $ p $-stability of stochastic systems with delay. AIMS Mathematics, 2024, 9(11): 32571-32577. doi: 10.3934/math.20241560 |
[4] | Vladica S. Stojanović, Hassan S. Bakouch, Radica Bojičić, Gadir Alomair, Shuhrah A. Alghamdi . Poisson-Lindley minification INAR process with application to financial data. AIMS Mathematics, 2024, 9(8): 22627-22654. doi: 10.3934/math.20241102 |
[5] | Waqar Afzal, Sayed M. Eldin, Waqas Nazeer, Ahmed M. Galal . Some integral inequalities for harmonical $ cr $-$ h $-Godunova-Levin stochastic processes. AIMS Mathematics, 2023, 8(6): 13473-13491. doi: 10.3934/math.2023683 |
[6] | Xiuxian Chen, Zhongyang Sun, Dan Zhu . Mean-variance investment and risk control strategies for a dynamic contagion process with diffusion. AIMS Mathematics, 2024, 9(11): 33062-33086. doi: 10.3934/math.20241580 |
[7] | Fan Yang, Xiaohui Ai . Exponential stability of stochastic complex networks with multi-weights driven by second-order process based on graph theory. AIMS Mathematics, 2024, 9(4): 9847-9866. doi: 10.3934/math.2024482 |
[8] | Adel Fahad Alrasheedi, Jungeun Kim, Rukhsana Kausar . q-Rung orthopair fuzzy information aggregation and their application towards material selection. AIMS Mathematics, 2023, 8(8): 18780-18808. doi: 10.3934/math.2023956 |
[9] | Thanin Sitthiwirattham, Muhammad Aamir Ali, Hüseyin Budak, Saowaluck Chasreechai . Quantum Hermite-Hadamard type integral inequalities for convex stochastic processes. AIMS Mathematics, 2021, 6(11): 11989-12010. doi: 10.3934/math.2021695 |
[10] | Safa' Alsheyab, Mohammed K. Shakhatreh . A new stochastic diffusion process based on generalized Gamma-like curve: inference, computation, with applications. AIMS Mathematics, 2024, 9(10): 27687-27703. doi: 10.3934/math.20241344 |
Most of the offshore projects will be impacted by storm surge, especially in the sea area where typhoons are frequent, and storm surge disasters are inevitable. The establishment of a theoretical distribution model of the number of typhoon occurrences and random waves is of great theoretical and practical significance for the study of the number of storm surge impacts and damage to marine engineering as well as the safety evaluation of offshore engineering. Based on stochastic process theory, this paper discusses the effects of the number of typhoon occurrences and typhoon intensity on wave displacement and water level by constructing a compound Poisson process model of typhoon frequency and typhoon intensity. The limitations of using typhoon intensity and wave height as random variables in the marine environment are improved. The average cumulative damage, reliability, and average service life of marine works were analyzed by marine works reliability analysis and a compound Poisson process of the number of storm surge impacts on marine works and the damage caused to marine works by each impact. The results show that the statistical theoretical model and reliability analysis of the storm surge hazard factors based on the stochastic process covers the original extreme value statistical distribution model, and can determine the parameters in the model according to different thresholds in line with the objective facts, and then deduce the design return level, while the deduced return level still has a certain degree of reliability in the case of short or missing data. Therefore, the stochastic process-based model of the imputed level of design parameters for the marine environment provides a new option for marine engineering design and risk management.
Storm surges are one of the more serious hydro-meteorological hazards, accounting for about 94% of all marine hazards [1,2]. Storm surges can pose a serious threat to industrial and agricultural production, as well as the safety of individual lives and property, especially in coastal areas. Typhoons are an important factor in storm surge hazard analysis, and the extremely high-water levels and wave heights resulting from their occurrence can cause tremendous damage to the coast of the affected sea areas. Strong winds pose a very serious threat to the economic construction of our coastal areas, and according to the World Meteorological Organization, the number of people who die from typhoons worldwide each year is about 2000–3000, and the average annual economic loss due to typhoons in the countries along the western Pacific Ocean is about US$4 billion [3,4]. The Marine Early Warning and Monitoring Department of the Ministry of Natural Resources released the 2019 China Marine Disasters Bulletin, which shows that in 2019, China's marine disasters were dominated by storm surges, waves and red tides, and compared to the average conditions of the past 10 years (2010–2019), the direct economic losses from marine disasters in 2019 were higher than the average (10.016 billion Yuan) [5,6]. The disaster with the heaviest direct economic loss is still the storm surge disaster, accounting for 99% of the total direct economic loss. On August the 10th 2019 at around 01:45, the super typhoon "Lekima" landed for the first time on the coast of Chengnan Town, Wenling City, Zhejiang Province. The maximum wind speed near the landing center was 52 m/s, and the maximum wind force near the center was grade 16 when it landed [7]. Under the combined influence of the storm surge and inshore waves, eight coastal provinces (municipalities directly under the central government) from north of Fujian to Liaoning suffered certain losses, with direct economic losses totaling to 10.288 billion yuan [8]. Thus, it can be seen that objective statistical description of the number and intensity of typhoons and random waves, which are the disaster-causing factors of storm surge, and the establishment of theoretical distribution models will be of great theoretical and practical significance for storm surge forecasting, early warning, risk assessment and safety analysis of marine engineering [9].
The development and progress of observation technology has provided researchers with plenty of data, and various efficient observation means have been used in ocean observation, such as ocean section monitoring, wave-measuring radar, remote sensing aircraft, ocean satellites, meteorological satellites and others [10]. While different observation means have been maturely applied to ocean observation, the analysis of such much data has also brought great challenges to researchers. The development of professional technology in many industries leads to many methods for data processing [11,12]. The parameters control by improving the algorithm WMSDE of wavelet basis function de- and accelerating convergence to avoid premature convergence by Deng et al. [13,14,15], engineering optimization solution by Xu et al. that based on data characteristics [16,17,18], and Chen's method of extracting data through equivalence evaluation [19,20], all provide reference for processing a variety of abnormal data. Effective processing of abnormal data caused by hurricanes, how to make more objective statistical inferences on storm surge causative factors based on both temporal and spatial dimensions is necessary to improve the marine disaster monitoring, forecasting and warning systems in each country around the world.
The spatial two-dimensional numerical models of typhoon and storm surge include the open coastal typhoon and storm surge graphic projections, the semi-closed sea typhoon and storm surge graphic projections, and the China Sea typhoon and storm surge projections. All these can simulate extreme sea conditions according to the climate and coastal hydrographic features of different countries [21], considering both the finite amplitude effect and the variation of the Coriolis parameter with dimensionality, but the elements of the marine environment corresponding to these models are usually based on random variables when the relevant boundary and initial conditions are entered [22,23]. Based on the principle of maximum entropy, Wang et al. established a joint distribution function of double entropy considering the frequency of typhoon occurrence to calculate the wave height of typhoon [24]. It is well known that storm surge leads to dramatic changes in water level and wave front displacement, which are stochastic phenomena with strong uncertainty [25,26], and the number of typhoon occurrences, water level, and wave front displacement also vary with time, and it is not sufficient to consider only the variation of these marine environmental elements in the space of values, but also their variation with time. At present, in the analysis of storm surge hazards, wave height, water level and typhoon intensity are used as random variables in a probability space, and the distribution function and some statistical properties can be inferred according to the different observation samples, which is a common analysis method used by many scientists [27]. For example, in the estimation of the design wave height and the design water level for a multi-year event, the annual extreme value of each year is taken as a random variable, and then the distribution function is estimated using the observations of the samples in different years [28].
Obviously, the number of occurrence of typhoons, the wave front displacement, wave height and water level are all time-dependent and can be described as stochastic processes with time as the indicator set. They are a random variable when the time is fixed and a function about time when values are determined. Based on the stochastic process theory, Liu and Chen studied the local statistical characteristics of typhoon frequency, and proposed a random process model which can describe the local occurrences of typhoon in given time domain [29,30,31]. The theory of stochastic process to analyze storm surge disasters has obvious advantages for describing uncertainty phenomena, not only the number of typhoons, water level, wave displacement and other changes in the value space, but also consider their changes over time, the statistical characteristics will be more comprehensive. Extending the current study of storm surge hazards based on random variables to stochastic processes would be a major methodological advance in disaster prevention and mitigation.
In this paper, the theoretical models of the storm surge-causing factors such as the number of typhoons, the wave height, and the variation of marine environmental factors over time are presented based on stochastic process theory. Based on the measured data of Chaolian Island, the reliability analysis of the marine project made easier by the compound Poisson process of the number of storm surge impacts and the damage caused to the marine project by each impact, which gives two important indicators, the average cumulative damage suffered by the marine project in time and the probability of the project failing to reach the danger threshold in cumulative damage in time.
Firstly, it is shown that the waiting time interval distributions of the typhoon Poisson process are mutually independent exponential distributions, and the waiting time interval distributions are used to derive a maximum likelihood estimate of the Poisson process intensity, which is the basis for specific engineering applications of the Poisson process. These serve as the basis for the specific application of the Poisson process. Using Nt and λ as the number of typhoon occurrences in the time interval (0,t) and the arrival rate or strength respectively. Nt is both a counting process and a Poisson process [22]. Thus, the typhoon process gives a random number of events in each bounded time interval or in each bounded region of a certain space.
Definition 1 We call the counting process {Nt,t≥0} that meets the following conditions as a Poisson process with the strength of λ:
(1) P(N0=0)=1;
(2) It has independent increment, that is, the number of events is independent on any finite number of disjoint time intervals;
(3) Independent increment, that is, the number of occurrences of events that is independent of each other in a finite number of mutually disjoint time intervals. For 0≤s<t, Ns,t=Nt−Ns is a Poisson distribution with the parameter λ(t−s):
P(Ns,t=n)=[λ(t−s)]nexp(−λ(t−s))/n!,n=0,1,2,… | (1) |
Definition 2. Assume Yt=∑Ntn=1ξn, {Nt,t≥0} is a Poisson process. {ξn,n≥1} is the sequence of independent and identically distributed random variables {Nt,t≥0} is mutually independent with {ξn,n≥1}, then we call {Yt=∑Ntn=1ξn,t≥0} a compound Poisson process.
Compound Poisson processes are often used for risk control and reliability of ocean engineering. Nt represents the risk counts during the time 0,t, {ξn,n≥1} represents the loss from the risk.
Theorem 1. If {Yt=∑Ntn=1ξn,t≥0} is the compound Poisson process, then we have:
(1) Yt is the independent incremental process.
(2) For any t, the characteristic function of Yt is ψ(s)=exp{λt(φ(s)−1)}, in which φ(s) is the characteristic function of ξn.
(3) If Eξ12<∞, then there is EYt=λtEξ1, Var(Yt)=λtEξ12.
Proof: (1) For any 0≤t0<t1<⋯<tk, there is Yt0=∑Nt0n=1ξn, Ytm−Ytm−1=∑Ntmn=Ntm−1+1ξn, m can take from 1,2,…,k. Yt is independent and incremental based on the definitions of {Nt,t≥0} and {ξn,n≥1}.
(2) ψ(s)=Eexp(isYt)=∑∞n=0E(exp(isYt)|Nt=n)P(Nt=n)
=∑∞n=0E(exp(is∑nk=1ξk)|Nt=n)e−λt(λt)nn!=∑∞n=0E(exp(is∑nk=1ξk))e−λt(λt)nn! |
=∑∞n=0(Eeisξ1)ne−λt(λt)nn!=∑∞n=0(φ(s))ne−λt(λt)nn!=exp(λt(φ(s)−1)) | (2) |
(3) EYt=E(E(Yt|Nt))=E(NtEξ1)=λtEξ1
Var(Yt)=E(Var(Yt|Nt))+Var(E(Yt|Nt))
When Nt=n, Var(Yt|Nt=n)=Var(∑nk=1ξk|Nt=n)=Var(∑nk=1ξk)=nVar(ξ1)
So Var(Yt|Nt)=NtVar(ξ1), the variance of Yt is
Var(Yt)=E(Nt)Var(ξ1)+Var(NtEξ1)=λtVar(ξ1)+λt(Eξ1)2=λtEξ12 | (3) |
By establishing a compound Poisson process model of the number and intensity of typhoon occurrences, we can obtain the cumulative impact of the average typhoon and its variance, as well as the cumulative damage that does not exceed the upper limit, from which we can obtain the service life of the ocean engineering.
Theorem 2. A is set to a constant, then P(Yt<A)=e−λt+∑∞n=1Fn(A)e−λt(λt)nn!, where Fn is the distribution function convolution of a random variable ξ1,…,ξn.
Proof: from the full probability formula, we can have
P(Yt<A)=∑∞n=0P(∑nk=1ξk<A|Nt=k)P(Nt=k) | (4) |
Since {Nt,t≥0} and {ξn,n≥1} are independent, so there is
P(Yt<A)=P(Nt=0)+∑∞n=1P(∑nk=1ξk<A)P(Nt=k) | (5) |
Then ξ1,…,ξn is independent and identically distributed, and the distribution function of the sum of which is the convolution of the distribution function ξ, which can be recorded as. Then there is:
P(Yt<A)=e−λt+∑∞n=1Fn(A)e−λt(λt)nn! | (6) |
In particular, if {ξn,n≥1} is an exponential distribution that is independent and identically distributed and subject to the mean μ, then ∑nk=1ξk is subject to the Gamma distribution with the parameters 1μ and n. Thus there is:
P(Yt<A)=e−λt+∑∞n=11μn(n−1)!∫A0xn−1e−xμdxe−λt(λt)nn! | (7) |
From Eq 7, it is clear that the design parameters of the marine environment can be described by the set of time indicators, and this dynamic description provides better stability in obtaining calculations in the case of data shortage or missing. The occurrence of extreme events plays a very important role in risk analysis. Although the number of extreme events is small, the harm caused by each occurrence is huge. The occurrence time interval of extreme event also reflects its harmfulness to some extent [32]. Therefore, studying the time interval of each extreme event is of great significance for disaster analysis. The typhoon arrival time interval distribution model is given below, and it can be proved that it obeys the exponential distribution, and the different time intervals are independent of each other.
Theorem 3 Let τ0=0, τk indicates the moment when the kth event occurs, let Tk=τk−τk−1 means the time interval from the k−1th occurrence of the event to the kth occurrence. {Tk,k≥1} is an independent and identically distributed sequence of random variables, subject to an exponential distribution of the parameter λ.
Proof: The event {T1>t} indicates that the first event occurred after the time, which is equivalent to no events occurring during the time interval 0,t, that is P(T1>t)=P(Nt=0)=e−λt. Since the Poisson process has a smooth independent increment property, it is easy to know:
P(T2>t|T1=s)=P(Ns+t−Ns=0|T1=s)=P(Nt=0)=e−λt | (8) |
Then T1 and T2 are both independent and subject to the exponential distribution of the parameter λ. Thus, the theorem can be proven.
The maximum likelihood estimation of the parameter λ is obtained below. The parameter in the Poisson process is a very important parameter λ. In general, if we start from time 0, what we observe is the time when the Poisson process {Nt,t≥0} occurs at each time. When the sample size is n, it is a list of time observations: t1<t2<⋯<tn.
Theorem 4 The maximum likelihood estimate of the parameter in λ the Poisson process is λ=nτn.
Proof: from the theorem 2.1 we know that T1,…,Tn is a sequence of independent and identically distributed random variables whose set of observations is t1,t2−t1,…,tn−tn−1. The likelihood function of t1,t2−t1,…,tn−tn−1 is:
L(τ1,…,τn)=λnexp(−λ∑ni=1(τi−τi−1))=λne−λτn | (9) |
When ∂L∂λ=0, it is easy to find the maximum likelihood estimate of the parameter λ as ˆλ=nτn, thus when the sample is t1<t2<⋯<tn, the maximum likelihood estimate of the parameter λ is ˆλ=nτn.
The maximum wind speed is selected as the index to describe the typhoon intensity. It is used as a disaster causing factor of storm surge and is a continuous random variable. The maximum wind speed of each typhoon is an independent and identically distributed random variable sequence {ξn,n≥1}. The typhoon influence {Yt=∑Ntn=1ξn,t≥0} is a compound Poisson process. The statistical distribution of maximum wind speed is discussed below.
Let the maximum wind speed at the time of each typhoon be a series of independent and identically distributed random variables, recorded as {ξn,n≥1}. The number of times a typhoon occurred during the time 0,t is recorded as Nt, where {Nt,t≥0} is a counting process. If we assume that the number of typhoons occurring in t,t+s is subject to Poisson distribution with λs, then Yt=∑Ntn=1ξn is a compound Poisson process, which can be used as a variable to measure the impact of a typhoon. We term it as typhoon influence. It is much more reasonable and comprehensive than a single consideration of the number of typhoons and the intensity of typhoons. The influence of typhoons is a variable that has an important influence on wave front displacement and water level.
The occurrence of typhoon surge will produce huge waves. The effect of a typhoon on the wave front displacement is discussed below. Let T=0,∞) (time). The wave front displacement ξ(t) is a stochastic process with time T as a set of indicators. Considering the variation of the wave front displacement in a sufficiently small period of time Δt, there are only three variations in the wave front displacement: First, the probability p1 rises to μξ; second, the probability p2 remains the same; third, the probability p3 drops to dξ, with p1+p2+p3=1. The three probabilities are related to the typhoon influence and time interval. Since the time interval can be sufficiently small, it is justifiable to consider that they are linear.
Let
p1=αEYtΔt+o((Δt)2) | (10) |
p3=βEYtΔt+o((Δt)2) | (11) |
then, p2=1−p1−p3, Where α and β are constants, o(t) is expressed as higher order infinitesimal quantities of t.
Because Yt=∑Ntn=1ξn is a compound Poisson process, there is,
p1=αλEξ1(Δt)2+o((Δt)2) | (12) |
p3=βλEξ1(Δt)2+o((Δt)2) | (13) |
With the development of observation technology, we can obtain rich observation data. From these data, we can determine or approximate the values of two parameters α and β based on the probability distribution of the sample statistics, and they determine the increase of wave front displacement.
In actual engineering, marine engineering works are often affected by disasters caused by storm surges such as waves and typhoons in its working environment. It is generally considered that the damage caused by the impact of ocean engineering each time is an independent and identically distributed sequence of random variables. Based on Poisson process, with the discussion of reliability of marine engineering, the superimposed engineering damage caused by each impact during a storm surge event can be calculated.
Assume in the time [0,t], the number of times the ocean engineering is hit by the storm surge Nt is a Poisson process with parameter λ, and the damage suffered by the nth impact is a random variable ξn, and they form independent and identical distribution sequences with the change. Then at the moment, the cumulative damage suffered by ocean engineering structure Yt=∑Ntn=1ξn is consistent with the Poisson process over time.
In actual engineering, the average cumulative damage suffered by marine engineering EYt during the time t is an important indicator for evaluating the reliability of marine engineering. When the damage ξn suffered by the impact for the n th time obeys the exponential distribution with the mean of μ, we have EYt=λtEξ1=λtμ.
Another reliability indicator for ocean engineering is the probability that the cumulative damage of ocean engineering at the moment has not reached the critical threshold A, i.e. reliability: R(t)=P(Yt<A). We can use the reliability of the corresponding ocean engineering as an indicator to evaluate the safety of offshore engineering.
Typhoons are the main hazard factor for storm surge disaster analysis. Accompanying intensity, wind, rain, and its landing location are all important factors to consider when studying typhoon disasters. The maximum wind force, maximum wind speed, and minimum center air pressure when the typhoon lands are the three main indicators describing the strength of the landing typhoon. Feng Lihua et al. believe that the observation accuracy of the central air pressure is relatively high, so the central air pressure is used to describe the strength of the landing typhoon, and the typhoon intensity obeys the exponential distribution based on the principle of maximum entropy. Since the data obtained from Chaolian Island in this paper are typhoon wind speed data, the maximum wind speed of the typhoon is chosen as an indicator to describe the intensity of the typhoon. According to the new national standard t="Tropical Cyclone Rating" implemented on May 15, 2006, the typhoon intensity is divided into several categories according to wind speed: tropical low pressure: 10.8–17.1 m/s (grade 6–7); tropical storm: 17.2–24.4 m/s. (grade 8–9); strong tropical storm: 24.5–32.6 m/s (grade 10–11); typhoon: 32.7v36.9 m/s (grade 12), 37.1–41.4 m/s (grade 13); strong typhoon: 41.5–46.1 m/s (grade 14), 46.2–50.9 m/s (grade 15); super typhoon: 51.0–56.0 m/s (grade 16), greater than or equal to 56.1 m/s (grade 17). The bar chart of the number of occurrences of the strong typhoon, tropical low pressure and typhoon are shown in Figure 1, and the corresponding maximum wind speed is shown in Figure 2.
From Figures 1 and 2, it can be seen that the maximum wind speed of typhoons between 1970 and 1972 showed an increasing trend. 76.77% of the first 30 typhoons had maximum wind speeds between 10 m/s and 30 m/s, while 74.19% of the last 31 typhoons had maximum wind speeds between 10 m/s and 50 m/s. There was a tendency for a strong typhoon to appear with increased wind speed when it occurs every 11 to 14 times.
The number of typhoon occurrences in the waters of the Chaolian Island obeys the Poisson distribution with the expectation λs that the parameter value of the Poisson process can be calculated as 0.07 based on the maximum likelihood estimation ˆλ=ntn from the sample observations, as shown in Theorem 4. Therefore, we can use the Poisson process with a parameter value of 0.07 to describe the number of typhoons occurring in the sea area of the island.
The maximum wind speed observations at the time of the typhoon in the Chaolian Island (120.881488, 35.893816) were fitted with the truncated Gumbel distribution, the truncated Weibull distribution, and the truncated Pearson-III distribution. As shown in Figure 3, the corresponding Kolmogorov Smirnov test results are shown in Table 1. It can be seen from Figure 3 and table 1 that Pearson-Ⅲ distribution is more suitable to describe the statistical distribution of wind speed in ChaoLian island sea area.
Distribution pattern | p−value | Statistical value |
Truncated Gumbel | 0.94 | 0.15 |
Truncated Weibull | 0.86 | 0.17 |
Truncated Pearson-Ⅲ | 0.98 | 0.19 |
According to the probability density function formula (14) of Pearson-Ⅲ distribution, the corresponding expectation EX=βα and characteristic function (15) can be obtained. The expected maximum wind speed is 25.2 m/s, and the characteristic function is φ(t)=(1−i0.48t)−52.5:
f(x)=αβΓ(β)xβ−1e−αx1(0,∞)(x) | (14) |
φ(t)=(1−itα)−β | (15) |
respectively.
Based on the above analysis, a compound Poisson process for the occurrence and intensity of typhoons can be established in the sea area of Chaolian Island: {Yt=∑Ntn=1ξn,t≥0}.
From Theorem 1, the expected formula (16) and the distribution function formula (17) of the wave surface displacement in response to typhoon can be obtained:
EYt=λtEξ1=λtβα | (16) |
ψ(s)=exp{λt((1−isα)−β−1)} | (17) |
In sufficiently small period of time (Δt)2, here are only three variations in the wave front displacement: The first, the probability p1 rises to μξ; the second, the probability p2 remains the same; the third, the probability p3 drops to dξ, and we also have p1+p2+p3=1 with p1=a1λtβαΔt+o((Δt)2),p3=a3λtβαtΔt+o((Δt)2).
The extreme wave height data of the Chaotian Island sea area are shown in Figure 4.
The threshold of wave height data is 6.5 m, then the cumulative graph exceeding the threshold and year is shown in Figure 5.
The Kolmogorov Smirnov test of the annual extreme wave height over the threshold is shown in Table 2. From the extreme value distribution of the annual extreme wave height and the statistical characteristics of the composite Poisson process, the recurrence levels of Gumbel distribution, Weibull distribution and Pearson-III distribution, as well as the average cumulative damage EYt (m) and reliability Rt of offshore engineering under different return periods can be obtained. See Tables 3–5.
Distribution pattern | p−value | Statistical value |
Truncated Gumbel | 0.19 | 0.17 |
Truncated Weibull | 0.87 | 0.14 |
Truncated Pearson-Ⅲ | 0.96 | 0.20 |
Return period | Return period level | EYt | Rt |
100 | 5.24 | 0.76 | 0.82 |
200 | 5.32 | 0.84 | 0.79 |
500 | 5.89 | 0.92 | 0.78 |
Return period | Return period level | EYt | Rt |
100 | 5.20 | 0.71 | 0.83 |
200 | 5.41 | 0.87 | 0.81 |
500 | 5.93 | 0.93 | 0.76 |
Return period | Return period level | EYt | Rt |
100 | 5.29 | 0.69 | 0.86 |
200 | 5.43 | 0.82 | 0.83 |
500 | 5.90 | 0.91 | 0.81 |
In the ocean, there are uncertainties everywhere. Their time-dependent versatility makes them very appropriate to be described in a stochastic process. This paper attempts to apply basic theories in the stochastic process to the analysis of storm surge disasters calculates the actual engineering models and compares the model results with the measured data. Specifically, this study has made the following achievements:
According to the tropical cyclone yearbook, the typhoon occurrence time is established, and the Poisson process of the number of typhoon occurrences in a certain period of time is established. The maximum likelihood estimation is applied to estimate the strength parameters.
Taking the extreme wave height of Chaolian Island as an example, the distribution of annual extreme wave height is described by different censored distributions. The distribution function, empirical distribution function, and Kolmogorov-Smirnov test results are analyzed.
Maximum wind speed during the typhoon landing has been selected as an indicator to describe its intensity, and a compound Poisson process model of the typhoon intensity and the number of typhoon occurrences is established.
Based on the stochastic process theory, a theoretical model of the time-dependent variation of the frequency of typhoon and wave height of the storm surge is given. The recurrence levels of Gumbel distribution, Weibull distribution and Pearson-Ⅲ distribution, and the average cumulative damage EYt and reliability Rt of marine engineering are obtained under different return periods.
Based on the statistical distribution of the maximum wind speed, the compound Poisson process model of typhoon intensity and the number of typhoon occurrences is determined and used to analyze the influence of typhoon intensity and the number of typhoon occurrences on the wave front displacement.
While reflecting the spatial factors, how to further study the self-correlation and inter-correlation of environmental factors at different time scales to better demonstrate the influence of time factors on storm surge hazards is what we will study in the future.
This research was funded by the National Natural Science Foundation of China (No. 52071306 and No.51379195), the Natural Science Foundation of Shandong Province (No. ZR2019MEE050), the 111 Project (No. B14028), and the Graduate Education Foundation (No. HDYA19006), which the authors show their gratitude.
The authors declare no conflict of interest.
[1] |
L. P. Wang, B. Y. Chen, C. Chen, Z. S. Chen, G. L. Liu, Application of linear mean-square estimation in ocean engineering, China Ocean Eng., 30 (2016), 149-160. doi: 10.1007/s13344-016-0007-9
![]() |
[2] |
L. P. Wang, B. Y. Chen, J. F. Zhang, Z. Chen, A new model for calculating the design wave height in typhoon-affected sea areas, Nat. Hazards, 67 (2013), 129-143. doi: 10.1007/s11069-012-0266-6
![]() |
[3] | W. P. Huang, X. Y. Han, S. Dong, Bivariate copula modelling of successive wave periods in combined sea states, Estuarine, Coastal and Shelf Science, ISSN 0272-7714,242 (2020), 10686. Available from: https://doi.org/10.1016/j.ecss.2020.106860. |
[4] | G. L. Liu, X. Li, J. Wang, Y. Kou, X. Wang, Research on the statistical characteristics of typhoon frequency, Ocean Eng., 209 (2020). |
[5] | L. Feng, P. Zhang, Typhoon statistical forecast based on the principle of maximum entropy, 27 (2003), 47. |
[6] | Y. Zhao, S. Dong, F. Jiang, Reliability analysis of mooring lines for floating structures using ANN-BN inference, Proceedings of the Institution of Mechanical Engineers. J. Eng. Maritime Environ., (2020), Available from: https://doi.org/10.1177/1475090220925200. |
[7] | G. L. Liu, Z. K. Gao, B. Y. Chen, H. Fu, S. Jiang, L. P. Wang, et al. Study on threshold selection methods in calculation of ocean environmental design parameters, IEEE Access, 7 (2019), 39515-39527. Available from: https://doi.org/10.1109/access.2019.2906935. |
[8] | B. Y. Chen, S. Escalera, I. Guyon, V. Ponce-López, N. Shah, M. O. Simón, Overcoming Calibration Problems in Pattern Labeling with Pairwise Ratings: Application to Personality Traits, Computer Vision-ECCV 2016 Workshops, (2016), 419-432. |
[9] |
B. Y. Chen, B. Wang, Location selection of logistics center in e-commerce network environments, Am. J. Neural Networks Appl., 3 (2017), 40. doi: 10.11648/j.ajnna.20170304.11
![]() |
[10] | H. J. Escalante, V. Ponce-López, J. Wan, M. A. Riegler, B. Chen, A. Clapés, et al. ChaLearn Joint Contest on Multimedia Challenges Beyond Visual Analysis: An overview. 2016 23rd International Conference on Pattern Recognition (ICPR), (2016), 67-73. |
[11] | S. Jiang, M. Lian, C. Lu, S. Ruan, Z. Wang, B. Chen, SVM-DS fusion based soft fault detection and diagnosis in solar water heaters, Energy Explor. Exploit., 37 (2019), 1125-1146. Available from: https://doi.org/10.1177/0144598718816604. |
[12] |
Y. Zeng, Z. Xie, J. Zou, Hydrologic and climatic responses to global anthropogenic groundwater extraction, Climate, 30 (2017), 71-90. doi: 10.1175/JCLI-D-16-0209.1
![]() |
[13] | W. Deng, H. Zhao, X. Yang, J. Xiong, M. Sun, B. Li, Study on an improved adaptive PSO algorithm for solving multi-objective gate assignment, Appl. Soft Comput., 59 (2017), 288-302. Available from: https://doi.org/10.1016/j.asoc.2017.06.004. |
[14] | W. Deng, J. J. Xu, Y. J. Song, H. M. Zhao, Differential evolution algorithm with wavelet basis function and optimal mutation strategy for complex optimization problem, Appl. Soft Comput., (2020), Doi: 10.1016/j.asoc.2020.106724. |
[15] | W. Deng, J. J. Xu, Y. J. Song, A novel gate resource allocation method using improved PSO-based QEA. IEEE Transactions on Intelligent Transportation Systems, (2020), Doi: 10.1109/TITS.2020.3025796. |
[16] |
J. Xu, B. Lei, Data Interpretation technology of GPR survey based on variational mode decomposition, Appl. Sci., 9 (2019), 2017. doi: 10.3390/app9102017
![]() |
[17] | J. Xu, H. Wei. Ultrasonic testing analysis of concrete structure based on S transform, Shock Vibration, (2019), 2019. |
[18] |
J. Xu, Z. Shen, S. Yang, X. Xie, Z. Yang, Finite element simulation of prevention thermal cracking in mass concrete, Int. J. Comput. Sci. Math., 10 (2019), 327-339. doi: 10.1504/IJCSM.2019.102691
![]() |
[19] | B. Chen, G. L. Liu, L. Wang, K. Zhang, S. Zhang, Determination of water level design for an estuarine city, J. Oceanol. Limnol., 37 (2019), 1186-1196. Available from: https://doi.org/10.1007/s00343-019-8107-z. |
[20] | B. Chen, Y. Kou, F. Wu, Study on evaluation standard of uncertainty of design wave height calculation model, J. Oceanol. Limnol., (2020), Doi: 10.1007/s00343-020-0327-8. |
[21] | V. Ponce-López, B. Y. Chen, M. Oliu, C. Corneanu, A. Clapés, I. Guyon, et al. ChaLearn LAP 2016: First Round Challenge on First Impressions-Dataset and Results, Computer Vision-ECCV 2016 Workshops, (2016), 400-418. |
[22] | J. Song, Q. Feng, X. Wang, H. Fu, W. Jiang, B. Y. Chen, Spatial Association and Effect Evaluation of CO2 Emission in the Chengdu-Chongqing Urban Agglomeration: Quantitative Evidence from Social Network Analysis, Sustainability, 11 (2018), 1. Available from: https://doi.org/10.3390/su11010001. |
[23] |
Y. Zeng, Z. Xie, Liu S, J. Xie, B. Jia, J. Gao, Global land surface modeling including lateral groundwater flow, J. Adv. Model. Earth. Sy., 10 (2018), 1882-1990. doi: 10.1029/2018MS001304
![]() |
[24] |
L. Wang, X. Xu, G. Liu, B. Chen, Z. Chen, A new method to estimate wave height of specified return period, Chin. J. Ocean. Limnol., 35 (2017), 1002-1009. doi: 10.1007/s00343-017-6056-y
![]() |
[25] | S. F. Zhang, W. Shen, D. S. Li, X. W. Zhang, B. Y. Chen, Nondestructive ultrasonic testing in rod structure with a novel numerical Laplace based wavelet finite element method, Latin Am. J. Solids Struct., 15 (2018), e48. Available from: https://doi.org/10.1590/1679-78254522. |
[26] | Y. Zeng, Z. Xie, Y. Yu, S. Liu, L. Wang, B. Jia, et al. Ecohydrological effects of stream-aquifer water interaction: A case study of the Heihe River basin, northwestern China, Hydrol. Earth Syst. Sci., 20 (2016), 2333-2352. |
[27] | D. Xu, Stochastic Theory of Sea Wave (Book), Higher Education Press, (2001), 111-145. |
[28] | X. Liu, Y. He, H. Fu, B. Chen, M. Wang, Z. Wang, How environmental protection motivation influences on residents' recycled water reuse behaviors: A case study in Xi'an city, Water, 10 (2018), 1282. Available from: https://doi.org/10.3390/w10091282. |
[29] | G. L. Liu, B. Y. Chen, L. P. Wang, S. Zhang, K. Zhang, X. Lei, Wave height statistical characteristic analyss, J. Oceanol. Limnol., 37 (2019), 448-460. Available from: https://doi.org/10.1007/s00343-019-8006-3. |
[30] | B. Y. Chen, K. Y. Zhang, L. P. Wang, S. Jiang, G. L. Liu, Generalized extreme value-pareto distribution function and its applications in ocean engineering, China Ocean Eng., 3 (2019), 127-136. Available from: https://doi.org/10.1007/s13344-019-0013-9. |
[31] | G. L. Liu, B. Y. Chen, Z. K. Gao, H. Fu, S. Jiang, L. P. Wang, et al. Calculation of joint return period for connected edge data, Water, 11 (2019), 300. Available from: https://doi.org/10.3390/w11020300. |
[32] | B. Chen, Y. Kou, D. Zhao, F. Wu, S. Liu, A. Chia, et al. Calculations on stopping time and return period, Nat. Hazards, 101 (2020), 537-550. Available from: https://doi.org/10.1007/s11069-020-03884-2. |
1. | Jun Liu, Jingpan Bai, Huahua Li, Bo Sun, Improved LSTM-Based Abnormal Stream Data Detection and Correction System for Internet of Things, 2022, 18, 1551-3203, 1282, 10.1109/TII.2021.3079504 | |
2. | Guilin Liu, Xinsheng Zhou, Yi Kou, Fang Wu, Daniel Zhao, Zongbing Yu, Analysis of extreme sea states under the impact of typhoon in different periods: A nested stochastic compound distribution applied in the South China Sea, 2022, 127, 01411187, 103298, 10.1016/j.apor.2022.103298 | |
3. | Guilin Liu, Pengfei Xu, Yi Kou, Fang Wu, Yi Yang, Daniel Zhao, Zaijin You, Design Wave Height Parameter Estimation Model Reflecting the Influence of Typhoon Time and Space, 2021, 9, 2077-1312, 950, 10.3390/jmse9090950 | |
4. | Guilin Liu, Xiuxiu Nong, Yi Kou, Fang Wu, Daniel Zhao, Zongbing Yu, Assessment of typhoon storm surge disaster scale based on expansion model, 2023, 2096-5508, 10.1007/s00343-022-2051-z | |
5. | Guilin Liu, Bokai Yang, Xiuxiu Nong, Yi Kou, Fang Wu, Daniel Zhao, Pubing Yu, Risk Level Assessment of Typhoon Hazard Based on Loss Utility, 2023, 11, 2077-1312, 2177, 10.3390/jmse11112177 | |
6. | Youjun Sun, Huajun Zhang, Shulin Hu, Jun Shi, Jianning Geng, Yixin Su, ConvGRU-RMWP: A Regional Multi-Step Model for Wave Height Prediction, 2023, 11, 2227-7390, 2013, 10.3390/math11092013 | |
7. | Guilin Liu, Xinsheng Zhou, Yi Kou, Fang Wu, Daniel Zhao, Yu Xu, Uncertainty analysis for the calculation of marine environmental design parameters in the South China Sea, 2023, 41, 2096-5508, 427, 10.1007/s00343-022-2052-y | |
8. | Chengwang Lin, Degradation and life prediction of mechanical equipment based on multivariate stochastic process, 2024, 10, 2297-3079, 10.3389/fmech.2024.1418137 | |
9. | Guilin Liu, Jingyi Yin, Shichun Song, Wenjin Yang, Yuhang Tian, Liping Wang, Yu Xu, Risk Estimation of Typhoon Disaster Based on Three-Dimensional Information Diffusion Method, 2023, 11, 2077-1312, 1080, 10.3390/jmse11051080 | |
10. | L. Cortese, X. Zhang, Marc Simard, S. Fagherazzi, Storm Impacts on Mineral Mass Accumulation Rates of Coastal Marshes, 2024, 129, 2169-9003, 10.1029/2023JF007065 |
Distribution pattern | p−value | Statistical value |
Truncated Gumbel | 0.94 | 0.15 |
Truncated Weibull | 0.86 | 0.17 |
Truncated Pearson-Ⅲ | 0.98 | 0.19 |
Distribution pattern | p−value | Statistical value |
Truncated Gumbel | 0.19 | 0.17 |
Truncated Weibull | 0.87 | 0.14 |
Truncated Pearson-Ⅲ | 0.96 | 0.20 |
Return period | Return period level | EYt | Rt |
100 | 5.24 | 0.76 | 0.82 |
200 | 5.32 | 0.84 | 0.79 |
500 | 5.89 | 0.92 | 0.78 |
Return period | Return period level | EYt | Rt |
100 | 5.20 | 0.71 | 0.83 |
200 | 5.41 | 0.87 | 0.81 |
500 | 5.93 | 0.93 | 0.76 |
Return period | Return period level | EYt | Rt |
100 | 5.29 | 0.69 | 0.86 |
200 | 5.43 | 0.82 | 0.83 |
500 | 5.90 | 0.91 | 0.81 |
Distribution pattern | p−value | Statistical value |
Truncated Gumbel | 0.94 | 0.15 |
Truncated Weibull | 0.86 | 0.17 |
Truncated Pearson-Ⅲ | 0.98 | 0.19 |
Distribution pattern | p−value | Statistical value |
Truncated Gumbel | 0.19 | 0.17 |
Truncated Weibull | 0.87 | 0.14 |
Truncated Pearson-Ⅲ | 0.96 | 0.20 |
Return period | Return period level | EYt | Rt |
100 | 5.24 | 0.76 | 0.82 |
200 | 5.32 | 0.84 | 0.79 |
500 | 5.89 | 0.92 | 0.78 |
Return period | Return period level | EYt | Rt |
100 | 5.20 | 0.71 | 0.83 |
200 | 5.41 | 0.87 | 0.81 |
500 | 5.93 | 0.93 | 0.76 |
Return period | Return period level | EYt | Rt |
100 | 5.29 | 0.69 | 0.86 |
200 | 5.43 | 0.82 | 0.83 |
500 | 5.90 | 0.91 | 0.81 |