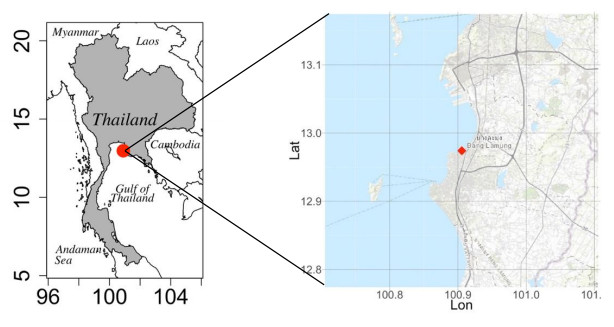
Short-term air quality monitoring in a coastal area, Naklua Subdistrict, Pattaya, Thailand is an activity to support the designated area under Thailand's sustainable tourism development. This study provided a short-term monitoring data analysis on time series and Bivariate Polar Plot (BVP) to provide the status of air quality and to determine the potential source area of air pollution. The result showed that NO2, SO2, CO and PM10 were not higher than the national air quality standards, while the 24-hour average of PM2.5 and the 8-hour average of O3 were slightly higher than the World Health Organization (WHO) air quality guideline values. The nighttime PM2.5 concentration was higher than the daytime concentration, and its potential source area is urban areas in the south. However, the daytime O3 concentration is higher than the nighttime concentration. Its potential source area is from the northwest, where Sichang island is located. This result could be used to support air pollution management by controlling and reducing emissions in the potential source areas as the first priority. Also, the study revealed that the BVP technique could be used to determine the source area of air pollution in the coastal area, where wind circulation is more complex than that over the land.
Citation: Suwimon Kanchanasuta, Sirapong Sooktawee, Natthaya Bunplod, Aduldech Patpai, Nirun Piemyai, Ratchatawan Ketwang. Analysis of short-term air quality monitoring data in a coastal area[J]. AIMS Environmental Science, 2021, 8(6): 517-531. doi: 10.3934/environsci.2021033
[1] | K. Wayne Forsythe, Cameron Hare, Amy J. Buckland, Richard R. Shaker, Joseph M. Aversa, Stephen J. Swales, Michael W. MacDonald . Assessing fine particulate matter concentrations and trends in southern Ontario, Canada, 2003–2012. AIMS Environmental Science, 2018, 5(1): 35-46. doi: 10.3934/environsci.2018.1.35 |
[2] | Carolyn Payus, Siti Irbah Anuar, Fuei Pien Chee, Muhammad Izzuddin Rumaling, Agoes Soegianto . 2019 Southeast Asia Transboundary Haze and its Influence on Particulate Matter Variations: A Case Study in Kota Kinabalu, Sabah. AIMS Environmental Science, 2023, 10(4): 547-558. doi: 10.3934/environsci.2023031 |
[3] | Pasquale Avino, Maurizio Manigrasso . Ozone formation in relation with combustion processes in highly populated urban areas. AIMS Environmental Science, 2015, 2(3): 764-781. doi: 10.3934/environsci.2015.3.764 |
[4] | Nikolaos Barmparesos, Vasiliki D. Assimakopoulos, Margarita Niki Assimakopoulos, Evangelia Tsairidi . Particulate matter levels and comfort conditions in the trains and platforms of the Athens underground metro. AIMS Environmental Science, 2016, 3(2): 199-219. doi: 10.3934/environsci.2016.2.199 |
[5] | Andrea L. Clements, Matthew P. Fraser, Pierre Herckes, Paul A. Solomon . Chemical mass balance source apportionment of fine and PM10 in the Desert Southwest, USA. AIMS Environmental Science, 2016, 3(1): 115-132. doi: 10.3934/environsci.2016.1.115 |
[6] | Meher Cheberli, Marwa Jabberi, Sami Ayari, Jamel Ben Nasr, Habib Chouchane, Ameur Cherif, Hadda-Imene Ouzari, Haitham Sghaier . Assessment of indoor air quality in Tunisian childcare establishments. AIMS Environmental Science, 2025, 12(2): 352-372. doi: 10.3934/environsci.2025016 |
[7] | Tabaro H. Kabanda . Investigating PM2.5 pollution patterns in South Africa using space-time analysis. AIMS Environmental Science, 2024, 11(3): 426-443. doi: 10.3934/environsci.2024021 |
[8] | Barend L. Van Drooge, David Ramos García, Silvia Lacorte . Analysis of organophosphorus flame retardants in submicron atmospheric particulate matter (PM1). AIMS Environmental Science, 2018, 5(4): 294-304. doi: 10.3934/environsci.2018.4.294 |
[9] | Jordan Finch, Daniel W. Riggs, Timothy E. O’Toole, C. Arden Pope III , Aruni Bhatnagar, Daniel J. Conklin . Acute exposure to air pollution is associated with novel changes in blood levels of endothelin-1 and circulating angiogenic cells in young, healthy adults. AIMS Environmental Science, 2019, 6(4): 265-276. doi: 10.3934/environsci.2019.4.265 |
[10] | Scheitel M, Stanic M, Neuberger M . PM10, PM2.5, PM1, number and surface of particles at the child’s seat when smoking a cigarette in a car. AIMS Environmental Science, 2016, 3(4): 582-591. doi: 10.3934/environsci.2016.4.582 |
Short-term air quality monitoring in a coastal area, Naklua Subdistrict, Pattaya, Thailand is an activity to support the designated area under Thailand's sustainable tourism development. This study provided a short-term monitoring data analysis on time series and Bivariate Polar Plot (BVP) to provide the status of air quality and to determine the potential source area of air pollution. The result showed that NO2, SO2, CO and PM10 were not higher than the national air quality standards, while the 24-hour average of PM2.5 and the 8-hour average of O3 were slightly higher than the World Health Organization (WHO) air quality guideline values. The nighttime PM2.5 concentration was higher than the daytime concentration, and its potential source area is urban areas in the south. However, the daytime O3 concentration is higher than the nighttime concentration. Its potential source area is from the northwest, where Sichang island is located. This result could be used to support air pollution management by controlling and reducing emissions in the potential source areas as the first priority. Also, the study revealed that the BVP technique could be used to determine the source area of air pollution in the coastal area, where wind circulation is more complex than that over the land.
The United Nation World Tourism Organization declared the term, "sustainable tourism" in 1996 based on the concept of sustainable development, which "takes into account the needs of present tourists, and travelers, needs of future generations as well" [1]. Air quality, the impact of air quality on tourists, and the impact of the tourism sector on air quality are indicators to be considered in the measure of sustainable tourism [2]. Pattaya is a designated area for sustainable tourism, a seaside city located in Chonburi Province, Thailand. The city is famous for tourism with many beaches, e.g., Naklua and Pattaya Beaches and related daytime and nighttime activities. During the daytime, what tourists can travel to visit interesting places such as the Sanctuary of Truth, the Pattaya floating market, Art in Paradise, et ceteras. Visit the seafood market, have lunch at a seafood restaurant, and rest at the beach are tourists prefer to do. The Walking Street is welcome for strolling around during nighttime. Tourists are also going to watch and enjoy the Tiffany's Cabaret Show. Get a hangout and party at various bars. Transportation, traffic, cooking, industry, chemical reactions in the atmosphere, etc. in this area and surrounding areas would be causes to increase air pollution level, and would affect air pollution exposure level of human. Therefore, air quality data and its related analysis are ingredients to support sustainable tourism management in the area.
Although there is Covid-19 outbreak in Thailand, the difference in air pollution levels in Bangkok, the capital city of Thailand, to the previous year is less different. The air quality index (AQI) mean value is slightly decreased and the concentrations of PM10 and PM2.5 are a small increase [3]. Therefore, air pollution problems still inevitably affect people's quality of life. Disease is one of the factors affecting the quality of life. The level of air pollution in the atmosphere is related to diseases such as cardiovascular disease, respiratory infection, and mortality rate [4]. Improving air quality in tourism locations, resting spots, and general access sites will allow people to enjoy the benefits of a good environment. In terms of management, identifying potential source areas of air pollution first is beneficial. This would be better than spending resources to reduce emissions in many areas without air pollution sources. Many receptor modeling methods have been utilized for source apportionment and contribution of pollution source types such as chemical mass balance (CMB), the principal component analysis (PCA), and positive matrix factorization (PMF). The methods related to incorporating wind data and back trajectories are the conditional probability function (CPF) and potential source contribution function (PSCF) [5]. To identify potential source areas of air pollutants many techniques are available using statistical measures revealing the relevant polar coordinates. The bivariate polar plot (BVP) uses the observed air quality data, wind speed, and wind direction to pinpoint areas of air pollutant potential sources in various places [6,7,8,9,10]. The polar plot technique can identify potential source areas of air pollution such as coke ovens and a plants in different locations [10], and biomass power plants [8]. The potential source areas representing mobile (transportation) and area sources (mining) can be distinguished from each other [9]. Also, the potential source areas in urban locations were identified [7]. Therefore, this technique is likely to be used to determine the potential source areas of air pollution management.
However, the study areas in related studies included inland areas [6,7,8,9,10] governed by the prevailing winds. On the contrary, coastal areas are not only be influenced by the prevailing winds, but also from land and sea breezes resulting in more complex wind circulation than the wind flow over inland areas [11]. It would be better to obtain more information on applying the BVP technique to identify potential source areas in coastal districts affected by sea and land breezes. According to scientific and sustainable tourism issues, this paper would like to apply BVP in a coastal area, and also provide air quality information to support sustainable tourism management.
Naklua Subdistrict is part of Pattaya City, Chonburi Province, Thailand, comprising a population of 49,005 people. A popular coastal park named Lanpho Naklua Park accommodates tourists and residents to sit and eat seafood including a sunset ambiance. Car parks and seafood markets are located near Lanpho Naklua Park. The western side of the park adjoins the Gulf of Thailand, the northern and southern sides are coastline, and the eastern side comprises urban areas, presenting traffic and other activities related to air pollutant emissions. Short-term air quality monitoring was performed at the Lanpho Naklua Park (Figure 1) by the mobile air quality monitoring unit belonging to the Environmental Research and Training Center from November 3–12, 2020. The study period was selected under discussion with local authorities. We take into account the short monitoring period should be within the high concentration season of air pollution, which many places in Thailand face an air pollution problem [7,8,9,12] during winter season (October-February) [13]. Furthermore, the readiness of related parties was a factor taking into consideration. Since Thailand was in lockdown due to Covid-19 pandemic, this study was carried out after the situation was unlocked on June 30, 2020 by the Regulation Issued under Section 9 of the Emergency Decree on Public Administration in Emergency Situations B.E. 2548 (2005) No. 11.
The observed parameters consisted of particulate matter less than 10 micron (PM10), particulate matter 2.5–10 micron (PM2.5–10), particulate matter less than 2.5 micron (PM2.5), nitrogen dioxide (NO2), sulfur dioxide (SO2), carbon monoxide (CO), ozone (O3), wind speed, and wind direction. Thermo Scientific model 1405-DF was used to measure PM10, PM2.5–10, and PM2.5. The 1405-DF instrument uses the tapered element oscillating microbalances (TEOM) and Filter Dynamics Measurement System (FDMS) techniques to measure the particulate matter concentration. The measured concentration is updated every 6 minutes. The 42i and the 43i models were used for NO2 and SO2 monitoring, respectively. The O3 and CO concentrations were measured using Teledyne analyzers model T400 and T300, respectively. The 42i analyzer operates on the principle of chemiluminescence to measure NO2, and the 43i analyzer operates on the principle of the fluorescence technique for SO2 measurement. T400 and T300 analyzers use ultraviolet-absorption photometry and gas filter correlation techniques for O3 and CO measurements, respectively. The time resolution of instrumentation to measure the concentration of the pollutant depends on each analyzer. The response times for NO2, SO2, O3 and CO measurements are about 40, 20, 30 and 70 seconds, respectively. These automatic analyzers are listed in the list of designated reference and equivalent methods of US EPA. Zero and span calibrations were performed with the pollutant standard gaseous before and after measurement. The span concentration is 80% of the full-scale value. The criteria to accept gaseous calibrations of this study is zero and span drifts are not greater than 7%. For meteorological parameters, wind speed and wind direction were measured using LSI LASTEM instruments. During the measurement period, the instruments sent their signal to the Advantech data logger, and then the data was calculated and recorded on an hourly basis by the Envidas environmental data acquisition system. The observed hourly missing data were 12.89%, 12.89%, 8.44%, 8.00%, 8.44%, and 8.00% for PM10, PM2.5, NO2, SO2, CO, and O3, respectively.
The OpenAir and other packages embedded in the R program were used to analyze data, reveal time series, and detect the potential source areas of pollutants. The BVP function of the OpenAir package can plot mean concentrations on polar coordinates related to wind direction and wind speed. The graphical output of BVP provides a result in spatial concentration-wind speed dependencies. Wind involves atmospheric advection processes resulting in the concentration change of ambient air pollutant. Therefore, the concept of BVP is based on the relation in concurrent existing concentration and wind. First, concentration data were partitioned in bins associated with each wind direction and wind speed range. Wind direction interval is of 10 degree and wind speed range is divided in 30 intervals. Concentrations in each bin were calculated to gain the relevant statistical metrics such as mean. Each bin (a cell) consisted of wind direction, wind speed, and mean concentration. To provide smooth concentration surface, the Generalized Additive Model with the isotropic smooth function was used, and expresses as √Ci=s(u,v)+ei. Where, Ci is the ith pollutant concentration, s is the smooth function, ei is the ith residual, u=¯ws.sin(2π/¯wd), and v=¯ws.cos(2π/¯wd). Wind direction (wd) and wind speed (ws) corresponded to θ and r in polar coordinates, respectively [14,15,16]. Thus, each mean concentration could be plotted using polar coordinates. Shading surface of concentrations on the polar coordinate could highlight the potential source areas of pollutants [10].
Hourly air quality from 3–12 November 2020 at Lan Pho-Naklua Park were monitored. Figure 2 shows that the concentration ranges of PM10, PM2.5–10, PM2.5, NO2, O3 and CO were wider than SO2. The ranges of PM10, PM2.5, NO2, O3 and CO ranged from 3.30–66.10 µg/m3, 3.00–44.40 µg/m3, 4.80–28.50 ppb, 5.60–59.40 ppb, and 110–750 ppb while SO2 ranged from and 0.60–3.8 ppb, respectively. Mean concentrations with standard deviation comprised 33.64±11.57 µg/m3, 13.60±6.18 µg/m3, 20.04±7.31 µg/m3, 1.23±0.55 ppb, 11.20±5.11 ppb, 270±120 ppb, and 31.46±11.20 ppb, for PM10, PM2.5–10, PM2.5, SO2, NO2, CO, and O3, respectively. However, air quality in Bangkok during a Covid-19 episode, 1 January–30 March 2020, revealed mean concentrations of 51.70±24.09 µg/m3, 32.19±17.51 µg/m3, 1.46±0.75 ppb, 15.46±10.49 ppb, 570±210 ppb, and 28.01±8.06 ppb for PM10, PM2.5, SO2, NO2, CO, and O3, respectively, reported by Sangkham et al. [3]. These data indicated that air quality at Lan Pho-Naklua Park, Pattaya was better than that in Bangkok and had less impact of air quality on tourists and residents. The air quality at Lan Pho-Naklua Park was compared with the national air quality standard of Thailand (NAAQs), the WHO air quality guidelines [17], and the standards given by the US EPA. Table 1 presents that none of the pollutants exceeded the NAAQs and US EPA standard values. When they were compared to the WHO guidelines, the PM2.5 and O3 pollutants showed maximum concentrations of 24-hour average and 8-hour average were higher than corresponding standards. In terms of AQI, the maximum concentrations were used to calculate the quality of ambient air. It found that Thai AQI value is 58 respecting to the 8-hour average O3 and US AQI value is 88 respecting to the 24-hour average PM2.5. The quality of ambient air is moderate based on both the Thai AQI and the US AQI. These signify the tourists and visitors in Lan Pho-Naklua Park perceived air quality at an acceptable level, with less pollution, and all pollutants lower than the air quality standard. Nonetheless, air quality at Lan Pho-Naklua Park was not lower than all the standard or guideline values; the O3 and PM2.5 concentrations slightly exceeded the WHO guideline values. O3 is a secondary pollutant formed by photochemical reactions during daytime. PM2.5 is directly emitted from pollution sources, and is formed by chemical reactions in the atmosphere. Investigations into the relationship between air pollutants discussed below will provide information to consider whether they originate from the same source or not.
Pollutant | Average Period | Maximum Concentration | Standard/Guideline | ||
NAAQs | WHOa | US.EPAb | |||
PM10 (g/m3) | 24 hours | 45.54 | 120 | 50 | 150 |
PM2.5 (g/m3) | 24 hours | 29.66 | 50 | 25 | 35 |
SO2 (ppb) | 1 hour | 3.80 | 300 | - | - |
24 hours | 1.88 | 120 | 20 g/m3 (7.6 ppb) | 75 | |
NO2 (ppb) | 1 hour | 28.50 | 170 | 200 g/m3 (106.4 ppb) | 100 |
CO (ppm) | 1 hour | 0.75 | 30 | - | 35 |
8 hours | 0.64 | 9 | - | 9 | |
O3 (ppb) | 1 hour | 59.40 | 100 | - | - |
8 hours | 53.85 | 70 | 100 g/m3 (50.9 ppb) | 70 | |
Notes: aWHO Air quality guidelines for particulate matter, ozone, nitrogen dioxide and sulfur dioxide-Global update 2005. bThe primary standard of US NAAQs obtained from https://www.epa.gov/criteria-air-pollutants/naaqs-table. |
Among observed ambient air pollutants, correlation analysis was performed and results presented in Figure 3. PM10 showed good correlation with PM2.5, PM2.5–10, and NO2 of 0.88, 0.83, and 0.62, significantly at 0.001. High correlation between particulate matter and NO2 revealed the emission source to be related to fuel combustion. Also, correlation between NO2 and CO was 0.71. This implied they would be emitted from a similar source. The emissions would stem from anthropogenic sources related to fuel combustion emitting NO2, CO, PM10, PM2.5–10, and PM2.5. The possible source related to activities in urban areas. Many studies in urban areas indicated the ratio of PM2.5/PM10 be 0.6, approximately [7,18]. Figure 4 reveals the distribution of the PM2.5/PM10 ratio of this study. Statistical indices, consisting of minimum, the 1st quartile, median, mean, the 3rd quartile, and maximum, are 0.30, 0.53, 0.60, 0.60, 0.69, and 0.91. The median and mean are similar connoting the distribution was quite symmetrical. About 50% of the ratio data fell within a range from 0.53 to 0.69. This ratio implied anthropogenic sources related to fuel combustion in urban areas and similar to related studies.
For O3, correlation to NO2 was negative, for which Pearson correlation coefficient was –0.61, and its correlation to wind speed was positive with r equal to 0.75. The negative correlation denotes increasing O3 related to decreasing NO2. This relation stems from the photochemical reaction in atmosphere, for which NO2 is a precursor of O3 formation. The concise reaction is NO2hv↔NO+O3 [19]. We also observed a high positive correlation between O3 and wind speed (WS) as presented in Figure 3. The high positive correlation denotes increasing WS corresponding to enhanced O3 concentrations at the monitoring station. During the same period, an increase in wind speed conveyed that the air mass movement was longer distanced from the low wind speed. Thus, it could be assumed that O3 was carried from another remote area to the monitoring station by wind.
Diurnal variations of PM2.5 and O3 pollutants and wind speed (Figure 5) were constructed from averaging hourly concentrations to view each fluctuation in time series whether they changed or not as we observed from the correlation analysis. Also, the diurnal variations were used to consider high and low concentration periods for both pollutants. The PM2.5 concentration was high at midnight and continuously decreased until 11:00. Then PM2.5 level started rising from 15:00 to high concentrations at 19:00, and remained at high concentration conditions until midnight (Figure 5a). Notably, many air pollution emission sources, including traffic and other activities during the daytime could emit PM2.5, but the concentration variation revealed low levels during that time. Meteorological factors are related to atmospheric advection process, in which wind played a role in reducing PM2.5 concentration. Figure 5c presents diurnal variation of wind speed indicating strong wind during the daytime resulting in a large dilution of PM2.5 caused by transported air mass. This is a reason PM2.5 is low during daytime. Also, weak transportation of air mass during nighttime enhanced PM2.5 concentrations in the air. Therefore, high PM2.5 period was during the nighttime. Another factor was the height of the Planet Boundary Layer (PBL) resulting in the ability of substances to mix in the atmosphere. Deep and shallow PBL present during the daytime and nighttime, respectively [20,21,22,23,24]. The layer is known as the mixing height [25], and could be used as an indicator of air quality. The deep mixing height during the daytime implied a large volume of air in the atmosphere. As the PM2.5 mass remains constant and the air volume increases, the concentration remains low.
Figure 5b shows the O3, concentration is low during in the nighttime and high during the daytime. Its concentration increases at 7:00, becomes highest around 13:00–14:00, decreasing until 19:00, and then remaining at low concentration levels until sunrise onward (Figure 3b). However, Figure 3c shows wind speed is high during the daytime, but the O3, concentration is also high. The reason can be explained by a related study. Negative correlations exist between many pollutants and mixing height, except O3 pollutant. The correlation of O3 to mixing height showed a positive correlation because of its sunrise dependency [20]. The O3 pollutant is formed by photochemical reactions with the presence of NO and NO2 in the air. The volatile organic compounds (VOCs) and CO are also ozone precursors, [19]. Strong sunlight during daytime enhances O3 level in the ambient air. This differs from PM2.5 in that the deep mixing height layer during daytime results in increasing air volume that lowers PM2.5 concentration.
The wind rose plot was performed to present prevailing wind during the study, high concentration, and low concentration periods. Daytime wind rose showed strong winds of 4.87 m/s (mean wind speed), and most of the wind blows from the northern section as shown in Figure 6. The contribution of north-northeast, east-northeast, north, north-northwest, and west-northwest directions were 55%, 18%, 12%, 10%, and 5%, respectively. However, the nighttime wind rose showed weaker winds (2.67 m/s). The contribution of north-northeast, east-northeast, and east directions were 45%, 36%, and 13%, respectively. These agreed with the prevailing wind during the study period that Thailand was governed by the winter (northeasterly) wind [26]. As shown in Figure 1, the west side of the monitoring station constitutes sea. Notably, more wind comes from the east and none of wind comes from the west side during nighttime than those during the daytime. This results from the influence of sea breeze during the daytime and land breeze during the nighttime.
Diurnal variation of O3 showed (as reported in Figure 5) high and low concentration periods from 7:00–19:00 and 20:00–6:00, respectively. The high and low concentration periods of PM2.5 presented from 17:00–8:00 and 9:00–16:00, respectively. However, high concentration periods of PM2.5 differs from high concentration periods of O3. Also, low concentration periods differ. Analyzing these two different periods related to PM2.5 and O3 concentrations, as reported in Figure 7, the low and high PM2.5 periods are affected by strong and weak northeasterly wind, respectively (Figure 7a, b). Whereas the low and high O3 periods show weak and strong northeasterly wind, respectively (Figure 7c, d). The result given by wind rose analysis could be used to suggest the pollutant movement pathway. Strong northeasterly winds during daytime would imply air pollutants are carried from remote air pollution source areas to the monitoring station receptor due to high wind speed. On the other hand, well mixed ambient air caused by turbulence from high wind speed could reduce the pollutant concentration in the air. Thus, presence of low PM2.5 concentration during strong northeasterly wind implied that the potential PM2.5 source was not located at remote areas in the northeast direction. The source was perhaps located near the monitoring station and the PM2.5 emitted to the ambient air would be diluted by the strong wind. For O3, high concentrations present during strong winds. This implied the source of O3 precursor would be locate far from the monitoring station. After O3 formation, the wind carried it to the monitoring station. This assumption would be analyzed further to present potential source areas that could either increase or decrease the concentration level at the monitoring station as we expected.
To improve air quality in the park and surrounding area for people and tourists to sit, rest, and take dinner during afternoon to evening, identifying source areas is required to support the management of the relevant authorities.
The statistical analysis, the BVP technique, can provide an image to identify potential source areas using the monitoring data at the receptor location as an input. As mentioned in related studies, analysis of BVP on PM2.5 and O3 could identify potential source areas during three periods of time: 1) analysis covering all study periods, 2) analysis of high concentration periods, and 3) analysis of low concentration periods. Moreover, the PM2.5/PM10 ratio was analyzed to provide more information related to potential source areas of PM2.5. The mean concentrations on polar coordinates provided by the BVP function are shown in Figure 8. During monitoring periods, the result revealed the potential source areas of PM2.5 were located south of the monitoring station as indicated by red shading on the polar coordinate (Figure 8a). The red shade area is not over 4 m/s in the radial coordinate. In case of straight movement over one hour, frictionless air mass can travel a distance of 14.4 km at 4 m/s of wind speed. The potential source area should be located far from the monitoring station at this distance. We compared the result with a map and found that the potential source area during the study period was located south of the monitoring station, an urban area named Pattaya city. Also, the BVP plot of NO2 showed similar areas (not present here). This implied the source was relate to traffic in the area. For the high PM2.5 concentration period (Figure 8b), the potential source area corresponded to the mean concentration of 35–40 µg/m3, closer to the pole of polar coordinates than the previous results for the entire monitoring period. The potential source area during high PM2.5 periods covers the residential area and roads around the North Pattaya area. This result agreed with wind rose analysis during the high PM2.5 concentration period presenting weak northeasterly winds. The weak wind was less than the atmospheric advection and produced more stable conditions resulting in greater accumulation of PM2.5 emitted from sources nearby. The potential source areas related to 20–30 µg/m3 appeared in areas nearby and west of the monitoring station during strong northeasterly winds presenting the low PM2.5 concentration. Strong northeasterly wind resulted in an absence of source areas to the south of the monitoring station. The potential source areas located to the west side of the monitoring station was the nearshore and sea. Studies have reported particles are emitted from the sea including sea salt and sea spray [27,28,29]. Sea salt aerosols are largely composed of NaCl, and other chemicals such as K+, Mg2+, Ca2+, SO42−. Sea spray aerosol contains not only sea salt, but other organic compositions [29]. The breaking waves can produce particles in sizes of 10 nm to greater than 10 µm with the number of particles peaking around 0.03–0.40 µm [27]. The size distribution of sea spray aerosol was reported ranging from 0.05 to 1.1 µm [28]. Thus, one possible source of PM2.5 during low concentration could be aerosols from the sea to the west side of the monitoring station.
Interestingly, another potential area of the PM2.5/PM10 ratio could be related to urban traffic areas because one study reported a high PM2.5/PM10 ratio of 0.6 [18]. Previous studies suggested that a ratio greater than 0.6 was related to emissions from industrial and vehicular fuel combustion, whereas low ratios of PM2.5/PM10 were related to more coarse particulate matter (PM2.5–10) contribution, and their sources involved mechanical processes [30]. The polar plot of PM2.5/PM10 ratio though the period (Figure 8d) revealed most areas contributed to PM2.5. The southern and eastern areas comprised urban areas showing a ratio of 0.6 similar to the related study, but the western area (offshore area) also revealed a ratio of 0.6. Moreover, remote areas in the north presented low PM2.5 concentrations (Figure 7a) and low PM2.5/PM10 ratios (Figure 8d). This area comprised agricultural and paddy fields, unpaved roads, and reservoirs locates in Ban Bang Phra area nearby the Khao Khiao Hill. Wind-blown dust and soil from this source area could transfer more PM2.5–10 to the monitoring station than PM2.5, one reason for the small PM2.5/PM10 ratio over this area. Figure 8e shows the red circular shading (the PM2.5/PM10 ratio is of 0.6–0.7) in the northeast direction during the high PM2.5 period. This circular shading corresponds to the major crossing between two highly traffic expressways (Figure S1 in supplementary), an interesting potential source area of PM2.5 during the high concentration period. Another potential source area during this period is located in the east side, an urban area with many roads bypassing the area. Also, low concentrations of PM2.5 indicated high ratio over the sea located to the west of the station. This signified PM2.5 was more abundant than PM2.5–10.
For ozone, the potential source area was not nearby the monitoring station. The potential source area (red shade in Figure 8g) was in the northwest direction. The area covers Sichang Island. The island has an oil and gas tank farm and related activities could emit volatile organic compounds (VOCs) in the atmosphere (Figure S2 in supplementary). VOCs and NOx are known as precursors of ozone formation [19,31,32]. Formation of O3 by photochemical reactions during daytime results in high O3 concentrations as shown in Figure 8h, caused by the potential source areas related to petrochemical terminals in Sichang Island. During low concentration periods of O3, the potential source area shows in the northeast direction with mean concentration around 40 ppb. The high O3 over the area during this period at nighttime, stems from less NO2. As we know, O3 can be formed by photochemical reactions with the presence of its precursor and sunlight. Some O3 produced in daytime could remain until the nighttime, and nighttime O3 reacts with NO2 yielding nitrate radical (NO3) resulting in reduced concentration [33]. Thus, the amount of NO2 controls O3 levels denoting less NO2 could remove small amounts of O3 at nighttime, and vice versa for high NO2 level. This constitutes one reason in presenting the potential source areas of O3 in the northeast direction due to fewer amounts of NO2 than that to the south of the monitoring station.
This study demonstrated that the analysis of short-term air quality monitoring data in coastal areas could provide useful results to support air quality management. The study area, the Lanpho Naklua Park, located in Pattaya Chonburi Province, Thailand is the designated area for sustainable tourism. The analysis firstly provided the situation of air quality from November 3–12, 2020. The status of air quality showed the air quality level was lower than those of the NAAQs of Thailand and USEPA. Nevertheless, 24-hour average PM2.5 and 8-hour average O3 slightly exceeded the guideline values suggested by the WHO. Both pollutants showed different high concentration periods corresponding to potential source areas.
The PM2.5 concentration was high during the nighttime, and low during the daytime. The high and low PM2.5 concentrations related to weak and strong northeasterly wind, respectively. The potential source areas given by BVP analysis during high concentration periods included the area to the south of the monitoring station covering residential areas and roads around the North Pattaya area. Also, the analysis of PM2.5/PM10 ratio revealed the major crossing between two expressways and urban areas in the east side could potentially contribute to abundant PM2.5. However, during the low concentration period, the park received PM2.5 from the sea on the west side. The O3 concentration was high during daytime caused by photochemical reactions with its precursors. The potential source area of O3 during high O3 concentration period covered the Sichang Island where an oil and gas tank farm is located. This constituted a source of VOCs, are O3 precursors. The result also showed that the BVP technique could be used to analyze ambient air monitoring data, including primary and secondary pollutants, in coastal areas.
Nevertheless, there is a study limitation, which is the short monitoring period that was performed to obtain data for analysis. The results can represent this short-term period, such as the potential source area given by this analysis cannot be used to identify for other periods influenced by different factors. The result given by short-term monitoring is not only utilized to present whether exceeding the NAAQs or not, but it can be analyzed for other purposes such as for understanding the extreme episode [34] and giving the scientific result to solve air pollution conflict between communities and enterprises [8]. Whereas, the long-term air quality analysis can present the state of environment, seasonal variation, and trend [9,12], which is the short-term analysis cannot provide them. This would be careful in analyzing and interpreting results in different time scales.
To improve air quality in Lanpho Naklua Park, Pattaya, policy makers should firstly focus on the identified potential source areas of PM2.5 and O3. Relevant emissions controls should be applied including improving the quality of the oil storage tank to reduce evaporation of VOCs. Improving traffic management in the city, along intersections, reducing the traffic volume, and improving engine efficiency would also reduce PM2.5 and O3 precursors. A long-term air quality analysis is also required in order to further confirm and fulfill the state of environment, seasonal variation, and the potential source area for various periods.
The authors would like to thank the Designated Areas for Sustainable Tourism Administration (Public Organization), Region 3, who supported researchers during the monitoring period with their kind cooperation. This paper is a part of a study under the approved project (ID: 2537805) by Thailand Science Research and Innovation (TSRI) with support from the annual government statement of expenditure via the TSRI and Department of Environmental Quality Promotion, Thailand.
The authors declare no conflict of interest.
[1] |
Janusz GK, Bajdor P (2013) Towards to Sustainable Tourism – Framework, Activities and Dimensions. Procedia Econ Finance 6: 523–529. doi: 10.1016/S2212-5671(13)00170-6
![]() |
[2] | World Tourism Organization (Ed.) (2004) Indicators of sustainable development for tourism destinations: a guidebook, Madrid. |
[3] |
Sangkham S, Thongtip S, Vongruang P (2021) Influence of air pollution and meteorological factors on the spread of COVID-19 in the Bangkok Metropolitan Region and air quality during the outbreak. Environ Res 197: 111104. doi: 10.1016/j.envres.2021.111104
![]() |
[4] |
Darçın M (2014) Association between air quality and quality of life. Environ Sci Pollut Res 21: 1954–1959. doi: 10.1007/s11356-013-2101-3
![]() |
[5] |
Hopke PK (2016) Review of receptor modeling methods for source apportionment. J Air Waste Manag Assoc 66: 237–259. doi: 10.1080/10962247.2016.1140693
![]() |
[6] |
Grange SK, Lewis AC, Carslaw DC (2016) Source apportionment advances using polar plots of bivariate correlation and regression statistics. Atmos Environ 145: 128–134. doi: 10.1016/j.atmosenv.2016.09.016
![]() |
[7] |
Kanchanasuta S, Sooktawee S, Patpai A, et al. (2020) Temporal Variations and Potential Source Areas of Fine Particulate Matter in Bangkok, Thailand. Air Soil Water Res 13: 1–10. doi: 10.1177/1178622120978203
![]() |
[8] |
Sooktawee S, Kanchanasuta S, Boonyapitak S, et al. (2020) Distinguish Potential Source Areas of PM2.5 and PM10 by Statistical Data Analysis. IOP Conf Ser Earth Environ Sci 489: 012024. doi: 10.1088/1755-1315/489/1/012024
![]() |
[9] |
Sooktawee S, Kanabkaew T, Boonyapitak S, et al. (2020) Characterising particulate matter source contributions in the pollution control zone of mining and related industries using bivariate statistical techniques. Sci Rep 10: 21372. doi: 10.1038/s41598-020-78445-5
![]() |
[10] |
Uria-Tellaetxe I, Carslaw DC (2014) Conditional bivariate probability function for source identification. Environ Model Softw 59: 1–9. doi: 10.1016/j.envsoft.2014.05.002
![]() |
[11] |
Lu R, Turco RP (1994) Air Pollutant Transport in a Coastal Environment. Part I: Two-Dimensional Simulations of Sea-Breeze and Mountain Effects. J Atmospheric Sci 51: 2285–2308. doi: 10.1175/1520-0469(1994)051<2285:APTIAC>2.0.CO;2
![]() |
[12] |
Chirasophon S, Pochanart P (2020) The Long-term Characteristics of PM10 and PM2.5 in Bangkok, Thailand. Asian J Atmospheric Environ 14: 11. doi: 10.5572/ajae.2020.14.1.073
![]() |
[13] | Wongsaming P, Exell RHB (2011) Criteria for Forecasting Cold Surges Associated with Strong High Pressure Areas over Thailand during the Winter Monsoon. J Sustain Energy Environ 2: 145–156. |
[14] |
Carslaw D, Beevers S, Ropkins K, et al. (2006) Detecting and quantifying aircraft and other on-airport contributions to ambient nitrogen oxides in the vicinity of a large international airport. Atmos Environ 40: 5424–5434. doi: 10.1016/j.atmosenv.2006.04.062
![]() |
[15] |
Carslaw DC, Ropkins K (2012) openair — An R package for air quality data analysis. Environ Model Softw 27–28: 52–61. doi: 10.1016/j.envsoft.2011.09.008
![]() |
[16] |
Carslaw DC, Beevers SD (2013) Characterising and understanding emission sources using bivariate polar plots and k-means clustering. Environ Model Softw 40: 325–329. doi: 10.1016/j.envsoft.2012.09.005
![]() |
[17] | World Health Organization (Ed.) (2006) WHO Air quality guidelines for particulate matter, ozone, nitrogen dioxide and sulfur dioxide Global update 2005 Summary of risk assessment, Switzerland, World Health Organization. |
[18] |
Munir S (2017) Analysing Temporal Trends in the Ratios of PM2.5/PM10 in the UK. Aerosol Air Qual Res 17: 34–48. doi: 10.4209/aaqr.2016.02.0081
![]() |
[19] | Seinfeld JH, Pandis SN (2006) Atmospheric chemistry and physics: from air pollution to climate change, Hoboken, N.J, J. Wiley. |
[20] |
Geiß A, Wiegner M, Bonn B, et al. (2017) Mixing layer height as an indicator for urban air quality? Atmospheric Meas Tech 10: 2969–2988. doi: 10.5194/amt-10-2969-2017
![]() |
[21] |
Guo J, Miao Y, Zhang Y, et al. (2016) The climatology of planetary boundary layer height in China derived fromradiosonde and reanalysis data. Atmospheric Chem Phys 16: 13309–13319. doi: 10.5194/acp-16-13309-2016
![]() |
[22] |
Liu S, Liang X-Z (2010) Observed Diurnal Cycle Climatology of Planetary Boundary Layer Height. J Clim 23: 5790–5809. doi: 10.1175/2010JCLI3552.1
![]() |
[23] |
Solanki R, Macatangay R, Sakulsupich V, et al. (2019) Mixing Layer Height Retrievals From MiniMPL Measurements in the Chiang Mai Valley: Implications for Particulate Matter Pollution. Front Earth Sci 7: 308. doi: 10.3389/feart.2019.00308
![]() |
[24] |
Nakoudi K, Giannakaki E, Dandou A, et al. (2019) Planetary boundary layer height by means of lidar and numerical simulations over New Delhi, India. Atmospheric Meas Tech 12: 2595–2610. doi: 10.5194/amt-12-2595-2019
![]() |
[25] |
Feng X, Wu B, Yan N (2015) A Method for Deriving the Boundary Layer Mixing Height from MODIS Atmospheric Profile Data. Atmosphere 6: 1346–1361. doi: 10.3390/atmos6091346
![]() |
[26] |
Sooktawee S, Humphries U, Limsakul A, et al. (2014) Spatio-Temporal Variability of Winter Monsoon over the Indochina Peninsula. Atmosphere 5: 101–121. doi: 10.3390/atmos5010101
![]() |
[27] |
Clarke A, Kapustin V, Howell S, et al. (2003) Sea-Salt Size Distributions from Breaking Waves: Implications for Marine Aerosol Production and Optical Extinction Measurements during SEAS. J Atmospheric Ocean Technol 20: 1362–1374. doi: 10.1175/1520-0426(2003)020<1362:SSDFBW>2.0.CO;2
![]() |
[28] |
Saliba G, Chen C-L, Lewis S, et al. (2019) Factors driving the seasonal and hourly variability of sea-spray aerosol number in the North Atlantic. Proc Natl Acad Sci 116: 20309–20314. doi: 10.1073/pnas.1907574116
![]() |
[29] |
Schiffer JM, Mael LE, Prather KA, et al. (2018) Sea Spray Aerosol: Where Marine Biology Meets Atmospheric Chemistry. ACS Cent Sci 4: 1617–1623. doi: 10.1021/acscentsci.8b00674
![]() |
[30] | Zhao D, Chen H, Yu E, et al. (2019) PM2.5/PM10 Ratios in Eight Economic Regions and Their Relationship with Meteorology in China. Adv Meteorol 2019: 1–15. |
[31] |
Kleinman LI, Daum PH, Lee YN, et al. (2001) Sensitivity of ozone production rate to ozone precursors. Geophys Res Lett 28: 2903–2906. doi: 10.1029/2000GL012597
![]() |
[32] |
Reid N, Yap D, Bloxam R (2008) The potential role of background ozone on current and emerging air issues: An overview. Air Qual Atmosphere Health 1: 19–29. doi: 10.1007/s11869-008-0005-z
![]() |
[33] |
Awang NR, Ramli NA, Yahaya AS, et al. (2015) High Nighttime Ground-Level Ozone Concentrations in Kemaman: NO and NO2 Concentrations Attributions. Aerosol Air Qual Res 15: 1357–1366. doi: 10.4209/aaqr.2015.01.0031
![]() |
[34] |
Velasco E, Rastan S (2015) Air quality in Singapore during the 2013 smoke-haze episode over the Strait of Malacca: Lessons learned. Sustain Cities Soc 17: 122–131. doi: 10.1016/j.scs.2015.04.006
![]() |
1. | Sirapong Sooktawee, Suwimon Kanchanasuta, Natthaya Bunplod, Assessment of 24-h moving average PM2.5 concentrations in Bangkok, Thailand against WHO guidelines, 2023, 33, 2468-2039, 10.1186/s42834-023-00165-y | |
2. | José Abel Espinoza-Guillen, Marleni Beatriz Alderete-Malpartida, Franchesco David Roncal-Romero, Joycy Claudia Vilcanqui-Sarmiento, Identification of particulate matter (PM10 and PM2.5) sources using bivariate polar plots and k-means clustering in a South American megacity: Metropolitan Area of Lima-Callao, Peru, 2025, 197, 1573-2959, 10.1007/s10661-025-13696-1 | |
3. | Khalisha Meliana Qatrunnada, Hafidz Abdillah, Puji Lestari, Study on black carbon concentrations and potential sources across DKI Jakarta during the wet season, 2025, 1448, 1755-1307, 012005, 10.1088/1755-1315/1448/1/012005 |
Pollutant | Average Period | Maximum Concentration | Standard/Guideline | ||
NAAQs | WHOa | US.EPAb | |||
PM10 (g/m3) | 24 hours | 45.54 | 120 | 50 | 150 |
PM2.5 (g/m3) | 24 hours | 29.66 | 50 | 25 | 35 |
SO2 (ppb) | 1 hour | 3.80 | 300 | - | - |
24 hours | 1.88 | 120 | 20 g/m3 (7.6 ppb) | 75 | |
NO2 (ppb) | 1 hour | 28.50 | 170 | 200 g/m3 (106.4 ppb) | 100 |
CO (ppm) | 1 hour | 0.75 | 30 | - | 35 |
8 hours | 0.64 | 9 | - | 9 | |
O3 (ppb) | 1 hour | 59.40 | 100 | - | - |
8 hours | 53.85 | 70 | 100 g/m3 (50.9 ppb) | 70 | |
Notes: aWHO Air quality guidelines for particulate matter, ozone, nitrogen dioxide and sulfur dioxide-Global update 2005. bThe primary standard of US NAAQs obtained from https://www.epa.gov/criteria-air-pollutants/naaqs-table. |
Pollutant | Average Period | Maximum Concentration | Standard/Guideline | ||
NAAQs | WHOa | US.EPAb | |||
PM10 (g/m3) | 24 hours | 45.54 | 120 | 50 | 150 |
PM2.5 (g/m3) | 24 hours | 29.66 | 50 | 25 | 35 |
SO2 (ppb) | 1 hour | 3.80 | 300 | - | - |
24 hours | 1.88 | 120 | 20 g/m3 (7.6 ppb) | 75 | |
NO2 (ppb) | 1 hour | 28.50 | 170 | 200 g/m3 (106.4 ppb) | 100 |
CO (ppm) | 1 hour | 0.75 | 30 | - | 35 |
8 hours | 0.64 | 9 | - | 9 | |
O3 (ppb) | 1 hour | 59.40 | 100 | - | - |
8 hours | 53.85 | 70 | 100 g/m3 (50.9 ppb) | 70 | |
Notes: aWHO Air quality guidelines for particulate matter, ozone, nitrogen dioxide and sulfur dioxide-Global update 2005. bThe primary standard of US NAAQs obtained from https://www.epa.gov/criteria-air-pollutants/naaqs-table. |