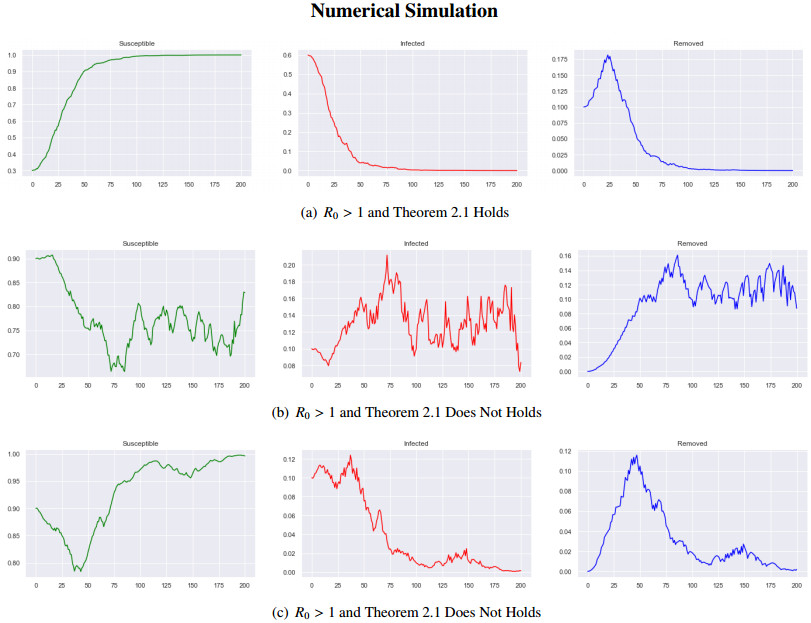
Citation: Andrew Vlasic, Troy Day. Poisson integral type quarantine in a stochastic SIR system[J]. Mathematical Biosciences and Engineering, 2020, 17(5): 5534-5544. doi: 10.3934/mbe.2020297
[1] | Yu Zhu, Liang Wang, Zhipeng Qiu . Threshold behaviour of a stochastic SIRS Lˊevy jump model with saturated incidence and vaccination. Mathematical Biosciences and Engineering, 2023, 20(1): 1402-1419. doi: 10.3934/mbe.2023063 |
[2] | Tingting Ding, Tongqian Zhang . Asymptotic behavior of the solutions for a stochastic SIRS model with information intervention. Mathematical Biosciences and Engineering, 2022, 19(7): 6940-6961. doi: 10.3934/mbe.2022327 |
[3] | Yang Chen, Wencai Zhao . Dynamical analysis of a stochastic SIRS epidemic model with saturating contact rate. Mathematical Biosciences and Engineering, 2020, 17(5): 5925-5943. doi: 10.3934/mbe.2020316 |
[4] | Zhongwei Cao, Jian Zhang, Huishuang Su, Li Zu . Threshold dynamics of a stochastic general SIRS epidemic model with migration. Mathematical Biosciences and Engineering, 2023, 20(6): 11212-11237. doi: 10.3934/mbe.2023497 |
[5] | Tingting Xue, Xiaolin Fan, Zhiguo Chang . Dynamics of a stochastic SIRS epidemic model with standard incidence and vaccination. Mathematical Biosciences and Engineering, 2022, 19(10): 10618-10636. doi: 10.3934/mbe.2022496 |
[6] | Meng Gao, Xiaohui Ai . A stochastic Gilpin-Ayala mutualism model driven by mean-reverting OU process with Lévy jumps. Mathematical Biosciences and Engineering, 2024, 21(3): 4117-4141. doi: 10.3934/mbe.2024182 |
[7] | Ying He, Yuting Wei, Junlong Tao, Bo Bi . Stationary distribution and probability density function analysis of a stochastic Microcystins degradation model with distributed delay. Mathematical Biosciences and Engineering, 2024, 21(1): 602-626. doi: 10.3934/mbe.2024026 |
[8] | Katarzyna Pichór, Ryszard Rudnicki . Stochastic models of population growth. Mathematical Biosciences and Engineering, 2025, 22(1): 1-22. doi: 10.3934/mbe.2025001 |
[9] | Qingshan Yang, Xuerong Mao . Stochastic dynamics of SIRS epidemic models withrandom perturbation. Mathematical Biosciences and Engineering, 2014, 11(4): 1003-1025. doi: 10.3934/mbe.2014.11.1003 |
[10] | Ying He, Junlong Tao, Bo Bi . Stationary distribution for a three-dimensional stochastic viral infection model with general distributed delay. Mathematical Biosciences and Engineering, 2023, 20(10): 18018-18029. doi: 10.3934/mbe.2023800 |
Many authors have considered continuous time stochastic epidemiological models. For instance, the papers [1], [2], and [3] analyzed various but similar SIR models perturbed by a Gaussian term in the form of an Iô integral, [4] considered a two-group SIR model with similar Gaussian perturbations, and [5] analyzed a SIS model also perturbed by an Iô integral. However, none of these models have taken into account anomalies that affect the system. Recently, there have been some efforts to incorporate anomalies into the dynamic by incorporating a Poisson integral to capture the instantaneous impact. The paper [6] assumed temporary immunity was captured by random jumps in a SIR model, while [7] and [8] both considered general environmental that may instantaneously affect different SIR models, and [9] assumed a SIS model with a Poisson integral capturing outside effects. Excluding the manuscript in [9], all of the models consider a compensated-Poisson integral. While there is a clear effect on the dynamic, the compensated-Poisson integral disappears in expectation and may not properly capture the phenomena.
Kuske, Gordillo and Greenwood in [3] investigated an SIR model perturbed by multiple and independent Brownian terms, and through a linear approximation, give conditions for a unique stationary measure establishing that an epidemic will occur. Roa, Wang, and Li [1] also considered an SIR, but the authors assume a nonlinear incident rate, and after analyzing the deterministic model, perturb the system twice with a two-dimensional Brownian motion, one for an endemic and the other for the disease free equilibrium. The authors then show that these systems are well defined and give conditions for almost sure convergence to an endemic and the disease free equilibrium. Yu, Jiang, and Shi [4] analyze a Brownian perturbed two-group SIR model with a stationary point at the deterministic endemic and determine sufficient conditions for the endemic equilibrium to be stochastically stable. The models examined in [2] and [3] are very close to the models considered in this paper and are explored in detail below.
For a fixed population of size N, we consider the following SIR model of the form
S′(t)=−βS(t)I(t)−μS(t)+μI′(t)=βS(t)I(t)−(λ+μ)I(t)R′(t)=λI(t)−μR(t) | (1.1) |
where S(t), I(t), and R(t) denote respectively the frequencies of the susceptible, infected, and removed; ergo, S(t)+I(t)+R(t)=1. The constant μ represents the birth and death rate (newborns are assumed to be susceptible), λ is the recovery rate for the individuals that are infected, and β represents the transmission rate. (Please see [10] for further information.)
In this system, when β≤λ+μ the disease free equilibrium is globally asymptotically stable. If β>λ+μ then the endemic equilibrium (μ+λβ,μβ(βμ+λ−1),λβ(βμ+λ−1)) is globally asymptotically stable.
We investigate a stochastic version of this SIR model under a Gaussian noise of interactions and include quarantine as a random event described by a Poisson process. Take the function j(y) to be continuously differentiable where minj(y)>0, and maxj(y)<1. Define N(dt,dy) as a Poisson measure with ν(⋅) as its intensity measure, and W(t) as an independent standard Brownian motion. This system is described by the right-continuous stochastic differential equation
dS(t)=(−βS(t)I(t)−μS(t)+μ)dt−σS(t)I(t)dW(t),dI(t)=(βS(t)I(t)−(λ+μ)I(t))dt+σS(t)I(t)dW(t)−∫Rj(y)I(t−)N(dt,dy),dR(t)=(λI(t)−μR(t))dt+∫Rj(y)I(t−)N(dt,dy). | (1.2) |
Since the Iô term is well known and represents the noise in the transmission process, we focus on the Poisson integral and give intuition for this term. Suppose that a specific percentage of the population is quarantined randomly with respect to a Poisson distribution. Denoting this percentage as C and N(⋅) as a Poisson distribution then the stochastic effect of the quarantine at time t is C⋅N(t). However, this kind of modeling is not robust enough in capturing the lack of efficiently of placing a subpopulation in quarantine. This is especially true of a population adjusting to an endemic.
To incorporate different impact sizes into the evolution, we briefly discuss the Poisson process as a temporal and spatial random measure, and interpret an integral under this measure. For further information, please see Applebaum [11]. For a Lévy process X(s) and B∈B(R∖{0}), define ΔX(s):=X(s)−X(s−), N(t,B):=#{0≤s≤t:ΔX(s)∈B}, and ν(B):=E[N(1,B)]. The measure ν(⋅) is denoted as the intensity measure. If ν(R)=∞ it is possible for an anomaly to happen an infinite number of times in a finite time interval. Therefore, the assumption that ν(R)<∞ is quite natural.
Taking χB(⋅) to be the identity function over the measurable set B then at time t the total effect is
∫t0∫Bj(y)N(ds,dy)=∑0≤s≤tj(ΔX(s))χB(ΔX(s)). |
When the deterministic integral −∫t0∫Rj(y)ν(dy)ds, (ds is the Lebesgue measure) is added to the Poisson integral the expectation of this effect is zero. Specifically, for ˜N(ds,dy):=N(ds,dy)−ν(dy)ds, the expected net effect of the “adjusted” quarantine E[−∫t0∫Rj(y)I(t−)˜N(ds,dy)]=0. See Sato [12], Bertoin [13], or Applebaum [11] for further information. This observation will be utilized in the analysis of the dynamic.
For completeness of the model, for the triple (Ω,F,P) we assume the filtration {Ft}t∈R+ is right-continuous and F0 contains all of the null sets of F.
Tornatore, Buccellato, and Vetro [2] analyzed System (1.2) when j≡0 and displayed when β<min{λ+μ−σ22, 2μ} the dynamic converged to the disease free equilibria of the noiseless version of the system. The authors displayed through simulations that this inequality is a good threshold. A stochastic SIR with delay was then analyzed and determined that if the same condition holds the process will converge to the disease free equilibria almost surely. Further, Tornatore and Buccellato [14] show that if β>λ+μ+σ22 then a strong endemic will occur.
Since this seminal paper [2], many authors have further refined their results by considering similar dynamics (see [15], [16], and [17]). When the various parameters were set to zero, the models in [15] and [16] showed that the model given in [2] converges to the disease free equilibria when β<λ+μ−σ22. Furthermore, for R0:=βλ+μ the deterministic endemic equilibrium, the authors in [16] showed that the dynamics stay within a neighborhood of R0 if β>λ+μ, i.e., if R0>1. Interestingly, Chen, Li and Liu [17] showed that the dynamics are stable to the disease free equilibrium when β<λ+μ+σ22.
In this paper it will be shown that System (1.2) is well-defined, and we give conditions for stability of the disease free equilibrium for both dynamics, as well as give conditions for System (1.2) to be within a neighborhood of the endemic equilibrium of the underlying deterministic system.
While the methods applied are well-known in the analysis of stochastic differential equations, analysis of the dynamic are not necessarily restricted these and similar methods. For instance, Calatayud, Cortés and Jornet [18] establish an algorithm that will quickly approximate the probability density function. The authors utilize polynomial expansions, the random variable transformation (RVT) technique, and multidimensional integration schemes to derive a solution with an algorithm. The authors display the efficacy of their algorithm with an application to a SIR model. The authors in [19] also focus on the probability density function in a more rigorous manner via a randomized Markov chain SIR-type model.
Define E(t)=(S(t),I(t),R(t)) and Δ3={x∈R3:x1,x2,x3>0 and x1+x2+x3=1} as the simplex. To ensure the process is well-defined, we show that System (1.2) is invariant to the simplex. In other words, given an initial condition that lies in simplex, System (1.2) will stay in the simplex for all finite time.
Denoting L as the infinitesimal generator for System (1.2), L has the form
Lg(x)=(−βx1x2−μx1+μ)∂g∂x1(x)+(βx1x2−(λ+μ)x2)∂g∂x2(x)+(λx2−μx3)∂g∂x3(x)+12σ2x21x22∂2g∂x21(x)+12σ2x21x22∂2g∂x22(x)−12σ2x21x22∂2g∂x1∂x2(x)−12σ2x21x22∂2g∂x2∂x1(x)+∫R[g(x+(0,−j(y)x2,j(y)x2))−g(x)]ν(dy), |
where g(x) is a twice differentiable function.
Proposition 2.1. For all finite t, given that x∈Δ3, Px(E(t)∈Δ3)=1.
Proof. We will invoke Theorem 2.1 in Meyn and Tweedie [20] and apply a norm-like function (V:Δ3→R+ that is measurable and V(x)→∞ when x tends to the boundary) such that, for some c>0, LV≤cV.
Define V(x)=−log(x1)−log(x2)−log(x3). Then for x∈Δ3
LV(x)=−1x1(−βx1x2−μx1+μ)−1x2(βx1x2−(λ+μ)x2)−1x3(λx2−μx3)+1x21σ22x21x22+1x22σ22x21x22+∫R[−log(x2−j(y)x2)−log(x3+j(y)x2)+log(x2)+log(x3)]ν(dy)=βx2+μ−μx1−βx1+λ+μ+−λx2x3+μ+σ22x22+σ22x21+∫R[log(11+j(y)x2/x3)−log(1−j(y))]ν(dy)≤β+3μ+λ+σ2+∫R−log(1−j(y))ν(dy). |
Clearly, there exists a positive constant c such that LV(x)≤cV(x).
To determine conditions for convergence to the disease free equilibrium, we use the stochastic Lyapunov method. Examining the process, one can see that the only stationary position is the point e1=(1,0,0).
Theorem 2.1. If β<min{λ+μ+∫Rj(y)ν(dy)−∫Rj2(y)2ν(dy)−σ22, 2μ} then for x∈Δ3,
Px(limt→∞E(t)=e1)=1. |
Proof. Define the positive function f(x)=c1(x1−1)2+c2x22+c3x23, where c3(λ+∫Rj(y)ν(dy))2μ−β<c1 and c1(σ2+2β)+c3∫Rj2(y)ν(dy)2λ+2μ+2∫Rj(y)ν(dy)−∫Rj2(y)ν(dy)−σ2−2β<c2. Then
Lf(x)=(−βx1x2−μx1+μ)2c1(x1−1)+(βx1x2−(λ+μ)x2)2c2x2+(λx2−μx3)2c3x3+12σ2x21x222c1+12σ2x21x222c2+∫R[c2(x2−j(y)x2)2−c2x22]ν(dy)+∫R[c3(x3+j(y)x2)2−c3x23]ν(dy)=−2μc1(x1−1)2−2μc3x23+2c1βx1x2(1−x1)+{2c2(βx1−(λ+μ)+σ22x21+∫R[−j(y)+j2(y)2]ν(dy))+c1σ2x21+c3∫Rj2(y)ν(dy)}x22. |
Noticing (x1−1)2=(1−x1)2=(x2+x3)2=x22+2x2x3+x23 it is clear that c3(∫Rj(y)ν(dy)+λ)2x2x3≤c3(∫Rj(y)ν(dy)+λ)(x1−1)2, and also that 2c1βx1x2(1−x1)≤2c1βx2(1−x1)≤2c1βx22+c1β(x1−1)2. Hence
Lf(x)≤{−c1(2μ−β)+c3(∫Rj(y)ν(dy)+λ)}(x1−1)2−2μc3x23+{2c2(β−(λ+μ)+σ22+∫R[−j(y)+j2(y)2]ν(dy))+c1(β+σ2)+c3∫Rj2(y)ν(dy)}x22. |
Therefore there exists a positive constant k such that Lf≤−kf. Theorem 4 and Remark 2 in [21] (page 325) yields that for an ϵ>0 there exists a neighborhood of e1, say U, such that for x∈U∩Δ3 we have Px(limt→∞E(t)=e1)≥1−ϵ.
Fix an arbitrary ϵ>0 and define τϵ=inf{t≥0:S(t)≥1−ϵ}. We will show that Ex[τϵ]<∞, which will assert our theorem. Define ϵ0=sup{S(τϵ)}. Since the process does not hit the boundary in finite time, we have that ϵ0<1 a.s. For the function f above, Lf(x)≤−α(1−x1)2, for some α>0. Now for x∈Δ3, such that x1<1−ϵ, and 0<t<∞, Dynkin's formula yields
0≤Ex[f(τϵ∧t)]=f(x)−Ex[∫τϵ∧t0Lf(E(t))ds]≤f(x)−Ex[∫τϵ∧t0(1−S(t))2ds]≤f(x)−α(1−ϵ0)2Ex[τϵ∧t]. |
Thus Ex[τϵ]<∞.
Now take an arbitrary ϵ>0 and x∈Δ3 and define M={limt→∞E(t)=e1}. The strong Markov property then yields
Px(M)=Ex[EE(τϵ)[χM]]≥1−ϵ. |
Since ϵ was arbitrary, the theorem follows.
Following the method applied in [16], we will show that if the deterministic condition R0>1 holds, i.e., βλ+μ>1, then the dynamic remains within a neighborhood of the deterministic epidemic equilibrium. Although the result in [17] might seem to contradict this result, since β<λ+μ+σ22 is the condition needed for almost sure convergence to the disease free equilibria, this does not mean this equilibrium is not in the neighborhood given by [16]. What we can say for certain is that when the disease free equilibria is not in this neighborhood, an endemic will occur.
Define (S∗,I∗,R∗) as the deterministic endemic equilibrium. Since our process is in the simplex, we will show that process is “near” (S∗,I∗), which would imply that the process is in a neighborhood of (S∗,I∗,R∗). The result is very similar to [16], where the jump perturbation adds to the radius of the ball around the deterministic endemic equilibria. In particular, the term kJ displays how when a random percentage of the infected population is consistently quarantined this decreases the impact to the general population.
Clearly, when both perturbations are small, an endemic will occur.
Theorem 3.1. If R0>1, then
lim supt→∞1t∫t0[(S(t)−S∗)2+(I(t)−I∗)2]dt≤kI∗2μ(σ22+kJ), |
where k=2(λ+2μ)/β and kJ=∫R(j(y)2/kI∗+2j(y)(S∗+I∗)/kI∗−log(1−j(y)))ν(dy).
Proof. Define f(t)=(S(t)−S∗+I(t)−I∗)2+k(I(t)−I∗−I∗log(I(t)/I∗)), (which is similar to the function defined in [16]). Then by Iô's extended lemma we have
df(t)=2(S(t)−S∗+I(t)−I∗)(−μS(t)+μ−(λ+μ)I(t))dt+k(1−I∗/I(t))(βS(t)I(t)−(λ+μ)I(t))dt+kI∗σ22S(t)2dt+σS(t)(I(t)−I∗)dW(t)+∫R[(S(t)−S∗+I(t)−j(y)I(t)−I∗)2−(S(t)−S∗+I(t)−I∗)2]N(dt,dy)+∫R[k(I(t)−I∗−I∗log((I(t)−j(y)I(t))/I∗)−k(I(t)−j(y)I(t)−I∗−I∗log(I(t)/I∗))]N(dt,dy). |
First we simplify and manipulate the drift, then we will simplify and manipulate the Poisson integrand. Notice that k(1−I∗/I(t))(βS(t)I(t)−(λ+μ)I(t))=βk(I(t)−I∗)(S(t)−S∗) and
(S(t)−S∗+I(t)−I∗)(−μS(t)+μ−(λ+μ)I(t))=(S(t)−S∗+I(t)−I∗)(−μS(t)+μλ+μβ−(λ+μ)I(t)+−μλ+μβ+μ)=(S(t)−S∗+I(t)−I∗)(−μ(S(t)−S∗)−(λ+μ)(I(t)−I∗)). |
Hence
2(S(t)−S∗+I(t)−I∗)(−μS(t)+μ−(λ+μ)I(t))+k(1−I∗/I(t))(βS(t)I(t)−(λ+μ)I(t))+kI∗σ22S(t)2=−2μ(S(t)−S∗)2−2(λ+μ)(I(t)−I∗)2+[−2(λ+2μ)+βk](S(t)−S∗)(I(t)−I∗)+kI∗σ22S(t)2=−2μ(S(t)−S∗)2−2(λ+μ)(I(t)−I∗)2+kI∗σ22S(t)2. | (3.1) |
For the Poisson integrands, we have
∫R[(S(t)−S∗+I(t)−j(y)I(t)−I∗)2−(S(t)−S∗+I(t)−I∗)2]N(dt,dy)=∫R[j(y)2I(t)2−2j(y)I(t)(S(t)−S∗+I(t)−I∗)]N(dt,dy) |
and
∫R[k(I(t)−I∗−I∗log((I(t)−j(y)I(t)/I∗)−k(I(t)−j(y)I(t)−I∗−I∗log(I(t)/I∗))]N(dt,dy)=∫Rk[−j(y)I(t)−I∗log(1−j(y)I(t))]N(dt,dy). |
Thus the Poisson integral is
∫R[j(y)2I(t)2−2j(y)I(t)(S(t)−S∗+I(t)−I∗)+k[−j(y)I(t)−I∗log(1−j(y)I(t))]]N(dt,dy)=∫R[j(y)2I(t)2−2j(y)I(t)(S(t)−S∗+I(t)−I∗)+k[−j(y)I(t)−I∗log(1−j(y)I(t))]]˜N(dt,dy)+∫R[j(y)2I(t)2−2j(y)I(t)(S(t)−S∗+I(t)−I∗)+k[−j(y)I(t)−I∗log(1−j(y)I(t))]]ν(dy)dt. | (3.2) |
The last equality comes from the integrand being bounded and ν(R)<∞.
Taking (3.1) and the deterministic measure in (3.2) we see that
−2μ(S(t)−S∗)2−2(λ+μ)(I(t)−I∗)2+kI∗σ22S(t)2+∫R[j(y)2I(t)2−2j(y)I(t)(S(t)−S∗+I(t)−I∗)+k[−j(y)I(t)−I∗log(1−j(y)I(t))]]ν(dy)≤−2μ[(S(t)−S∗)2+(I(t)−I∗)2]+kI∗[σ22+∫R(j(y)2/kI∗+2j(y)(S∗+I∗)/kI∗−log(1−j(y)))ν(dy)]. |
By the Strong Law of Large Numbers for local martingales, we have almost surely that
limt→∞1t∫t0∫R[j(y)2I(t)2−2j(y)I(t)(S(t)−S∗+I(t)−I∗)+k[−j(y)I(t)−I∗log(1−j(y)I(t))]]˜N(dt,dy)=0 |
and
limt→∞1t∫t0σS(t)(I(t)−I∗)dW(t)=0. |
Therefore, since
0≤f(t)−f(0)≤−2μ∫t0[(S(t)−S∗)2+(I(t)−I∗)2]dt+∫t0kI∗(σ22+kJ)dt+∫t0σS(t)(I(t)−I∗)dW(t)+∫t0∫R[j(y)2I(t)2−2j(y)I(t)(S(t)−S∗+I(t)−I∗)+k[−j(y)I(t)−I∗log(1−j(y)I(t))]]˜N(dt,dy), |
we are able to conclude that
lim supt→∞1t∫t0[(S(t)−S∗)2+(I(t)−I∗)2]dt≤(λ+2μ)I∗βμ(σ22+kJ). |
In this paper quarantine of infected individuals was modeled with a Poisson measure. Due to the imperfections of human interactions, we have given a model that better represents the effect of quarantine. After the derivation of the model, we determined that this dynamic was well defined. In Theorem 2.1 we showed that if the inequality β<min{λ+μ+∫Rj(y)ν(dy)−∫Rj2(y)2ν(dy)−σ22, 2μ} holds then the dynamic will converge almost surely to the disease free equilibrium. The condition for the disease free equilibrium maybe counterintuitive since the term in the right side of the inequality, −∫Rj(y)22ν(dy), shows that quarantine does not fully affect the dynamic. However, what this tells us is that the quarantine does not have a complete effect on the dynamic of the disease and, in some instances, may only slightly dampen the impact to the population.
In Theorem 3.1, computational conditions for an endemic where given. The condition for an endemic is rather encompassing, as it includes all possible strengths of recurrence. The result shows the complexity the quarantine adds to the dynamic encompassed in the complex term kJ. The term kJ displays how the quarantine has an effect on the entire populace, and in particular how a large quarantine does not completely stop the evolution of the disease and how a small quarantine has a larger effect than expected.
For the computer simulations below, Figures 1(a) and 1(b) display the subtleties with Theorem 2.1 on the long run behavior of the system. The last simulation, Figure 1(c), shows intuition behind the random quarantine on how and this term severely affects the dynamic and increases the size of the neighborhood of the deterministic endemic equilibrium, as shown in Theorem 3.1.
The authors declare no conflicts of interest in this paper.
[1] | F. Rao, W. Wang, Z. Li, Stability analysis of an epidemic model with diffusion and stochastic perturbation, Comm. Nonlinear Sci. Num. Simul., 17 (2012), 2551-2563. |
[2] | E. Tornatore, S. Buccellato, P. Vetro, Stability of a stochastic SIR system, Phys. A, 354 (2005), 111-126. |
[3] | R. Kuske, L. F. Gordillo, P. Greenwood, Sustained oscillations via coherence resonance in SIR, J. Theor. Biol., 245 (2007), 459-469. |
[4] | J. Yu, D. Jiang, N. Shi, Globaly stability of the two-group SIR model with random perturbation, J. Math. Anal. App., 360 (2009), 235-244. |
[5] | A. Gray, D. Greenhalgh, L. Hu, X. Mao, J. Pan, Stochastic differential equation SIS epidemic model, SIAM J. Appl. Math., 71 (2011), 876-902. |
[6] | Q. Liu, D. Jiang, T. Hayat, B. Ahmad, Analysis of a delayed vaccinated SIR epidemic model with temporary immunity and Lévy jumps, Nonlinear Anal. Hybrid Sys., 27 (2018), 29-43. |
[7] | X. Zhang, K. Wang, Stochastic SIR model with jumps, Appl. Math. Letters, 26 (2013), 867-874. |
[8] | Y. Zhou, W. Zhang, Threshold of a stochastic SIR epidemic model with Lévy jumps, Phys. A, 446 (2016), 204-216. |
[9] | A. Vlasic, D. Troy, Modeling stochastic anomalies in an SIS system, Stoch. Anal. App., 35 (2017), 27-39. |
[10] | H. Hethcote, The mathematics of infectious diseases, SIAM Rev., 42 (200), 599-653. |
[11] | D. Applebaum, Lévy processes and stochastic calculus, 2nd edition, Cambridge Studies in Advanced Mathematics, Cambridge, 2004. |
[12] | K. Sato, Lévy Processes and Infinitely Divisible Distributions, 1st edition, Cambridge University Press, Cambridge, 1999. |
[13] | J. Bertoin, Lévy Processes, 1st edition, Cambridge University Press, Cambridge, 1996. |
[14] | E. Tornatore, S. Buccellato, On a stochastic SIR model, Appl. Math. (Warsaw), 34 (2007), 389-400. |
[15] | Q. Lu, Stability of SIR system with random perturbations, Phys. A, 388 (2009), 3677-3686. |
[16] | C. Ji, D. Ji, N. Shi, The behavior of an SIR epidemic model with stochastic perturbation, Stoch. Anal. App., 30 (2012), 755-773. |
[17] | G. Chen, T. Li, Ch. Liu, Lyapunov exponent of a stochastic SIR model, Comptes Rendus Math., 351 (2013), 33-35. |
[18] | J. Calatayud, J. C. Cortés, M. Jornet, Computing the density function of complex models with randomness by using polynomial expansions and the RVT technique. Application to the SIR epidemic model, Chaos Solit. Fract., 133 (2020), 109639. https://doi.org/10.1016/j.chaos.2020.109639. |
[19] | J. C. Cortés, S. K. El-Labany, A. Navarro-Quiles, M. M. Selim, H. Slama, A comprehensive probabilistic analysis of SIR-type epidemiological models based on full randomized DiscreteTime Markov Chain formulation with applications, Math. Methods Appl. Sci., (2020), https://doi.org/10.1002/mma.6482. |
[20] | S. P. Meyn, R. L. Tweedie, Stability of Markovian processes Ⅲ: Foster-Lyapunov criteria for continuous-time processes, Adv. Appl. Prob., 25 (1993), 518-548. |
[21] | I. Gihman, A. V. Skorohod, Stochastic differential equations, 2nd edition, Springer-Verlag, New York, 1972. |
[22] | D. Down, S. P. Meyn, R. L. Tweedie, Exponential and uniform ergodicity of Markov Processes, Ann.Appl. Prob., 23 (1995), 1671-1691. |
[23] | A. V. Skorohod, Asymptotic Methods in the Theory of Stochastic Differential Equations, 1st edition, American Mathematical Society, Moscow, 1989. |
[24] | X. Mao, Stochastic Differential Equations and Applications, 1st edition, Horwood, Chichester, 2007. |