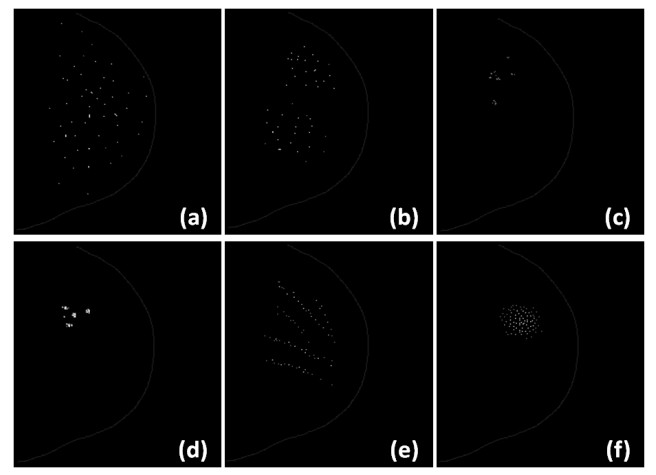
Citation: Yali Ouyang, Zhuhuang Zhou, Weiwei Wu, Jin Tian, Feng Xu, Shuicai Wu, Po-Hsiang Tsui. A review of ultrasound detection methods for breast microcalcification[J]. Mathematical Biosciences and Engineering, 2019, 16(4): 1761-1785. doi: 10.3934/mbe.2019085
[1] | Xiao Zou, Jintao Zhai, Shengyou Qian, Ang Li, Feng Tian, Xiaofei Cao, Runmin Wang . Improved breast ultrasound tumor classification using dual-input CNN with GAP-guided attention loss. Mathematical Biosciences and Engineering, 2023, 20(8): 15244-15264. doi: 10.3934/mbe.2023682 |
[2] | Qun Xia, Yangmei Cheng, Jinhua Hu, Juxia Huang, Yi Yu, Hongjuan Xie, Jun Wang . Differential diagnosis of breast cancer assisted by S-Detect artificial intelligence system. Mathematical Biosciences and Engineering, 2021, 18(4): 3680-3689. doi: 10.3934/mbe.2021184 |
[3] | Xiaoyue Fang, Ran Zhou, Haitao Gan, Mingyue Ding, Ming Yuchi . Time-of-flight completion in ultrasound computed tomography based on the singular value threshold algorithm. Mathematical Biosciences and Engineering, 2022, 19(10): 10160-10175. doi: 10.3934/mbe.2022476 |
[4] | Jun Gao, Qian Jiang, Bo Zhou, Daozheng Chen . Convolutional neural networks for computer-aided detection or diagnosis in medical image analysis: An overview. Mathematical Biosciences and Engineering, 2019, 16(6): 6536-6561. doi: 10.3934/mbe.2019326 |
[5] | Yihua Song, Chen Ge, Ningning Song, Meili Deng . A novel dictionary learning-based approach for Ultrasound Elastography denoising. Mathematical Biosciences and Engineering, 2022, 19(11): 11533-11543. doi: 10.3934/mbe.2022537 |
[6] | Xi Lu, Xuedong Zhu . Automatic segmentation of breast cancer histological images based on dual-path feature extraction network. Mathematical Biosciences and Engineering, 2022, 19(11): 11137-11153. doi: 10.3934/mbe.2022519 |
[7] | Thaweesak Trongtirakul, Sos Agaian, Adel Oulefki . Automated tumor segmentation in thermographic breast images. Mathematical Biosciences and Engineering, 2023, 20(9): 16786-16806. doi: 10.3934/mbe.2023748 |
[8] | Jiajia Jiao, Xiao Xiao, Zhiyu Li . dm-GAN: Distributed multi-latent code inversion enhanced GAN for fast and accurate breast X-ray image automatic generation. Mathematical Biosciences and Engineering, 2023, 20(11): 19485-19503. doi: 10.3934/mbe.2023863 |
[9] | Salman Lari, Hossein Rajabzadeh, Mohammad Kohandel, Hyock Ju Kwon . A holistic physics-informed neural network solution for precise destruction of breast tumors using focused ultrasound on a realistic breast model. Mathematical Biosciences and Engineering, 2024, 21(10): 7337-7372. doi: 10.3934/mbe.2024323 |
[10] | Bo An . Construction and application of Chinese breast cancer knowledge graph based on multi-source heterogeneous data. Mathematical Biosciences and Engineering, 2023, 20(4): 6776-6799. doi: 10.3934/mbe.2023292 |
Breast cancer has become a serious health problem around the world. The latest report by the World Health Organization and International Agency for Research on Cancer showed that the incidence and mortality of breast cancer ranked the first place among female cancer patients [1]. Breast microcalcifications (MCs) are the first indication in more than 40% of breast cancers, and they are sometimes the only indication of malignancy [2,3]. In addition, 95% of breast ductal carcinoma in situ (DCIS) is diagnosed by analyzing MCs [4]. The early detection of breast MCs can increase the possibility of breast cancer survival, making their diagnosis critical [5].
Breast MCs are small calcium deposits, with size of 0.1-1.0 mm shown on ultrasound or mammography images [6]. MCs are majorly categorized as two types which differ in chemical composition. The first type is composed of calcium oxalate, which is mainly found in benign breast lesions; the second type is hydroxyapatite, a kind of calcium phosphate, which can be found in both benign and malignant tumors [7,8]. The size, number, distribution and morphology of MCs contain important information about the malignancy and benignity of breast lesions. According to the Breast Imaging Reporting and Data System (BI-RADS), imaging based diagnosis of breast MCs mainly include three types: low risk of malignancy, intermediate risk of malignancy, and high risk of malignancy [9,10]. Amorphous calcifications with round, thick rod, diffuse and non-catheter distribution are low risk of malignancy calcifications (Figures 1a, b). Large and heterogeneous calcifications and amorphous or isolated calcifications are more associated with indeterminate MCs (Figures 1c, d). Calcifications showing fine and pleomorphic, or fine linear branching distribution are associated with highly suspicious malignant MCs (Figures 1e, f).
Among different imaging modalities, mammography has been taken as the reference standard for evaluation of MCs and is able to detect clustered MCs which have a size of about 100 μm or greater [11,12]. However, mammography exposes patients to ionizing radiation, and its diagnostic performance is relatively poor for dense and massive glands of breast [13]. Many studies have shown that Asian women have dense breast tissue and are fit for screening by ultrasound rather than mammography [13]. Ultrasound imaging is one of the most frequently used diagnostic tools to detect and classify abnormalities of the breast, because of its low cost, nonionizing radiation, and real-time capability. Ultrasound has potential for detecting breast MCs, especially for detecting MCs in dense breasts. Many researchers have strived to improve the capability of ultrasound in detecting breast MCs. However, a review of current ultrasound detection methods for breast MCs is still lacking.
This paper aims to review the state-of-the-art ultrasound methods for breast MC detection. These methods were broadly divided into high-frequency B-mode ultrasound imaging techniques, B-mode ultrasound image processing techniques, ultrasound elastography techniques, time reversal techniques, high spatial frequency techniques, second-order ultrasound field (SURF) imaging techniques, and photoacoustic imaging (PAI) techniques. Table 1 summarizes the major ultrasound detection techniques of breast MCs in this review according to the following conditions: detection method, study type, number of cases, ultrasound transducer type, transducer center frequency, size of an individual MC, MC distribution pattern, reference standard, and major findings.
Authors | Year | Method | Study | N | T | TCF (MHz) | Size* (mm) | Pattern | Reference standard | Findings |
Authors | Year | Method | Study | N | T | TCF (MHz) | Size* (mm) | Pattern | Reference standard | Findings |
Yang et al. [14] | 1997 | HF B-mode US | MCs in tumors in vivo | 84 | LAT | 10-5 | < 1 | Clustered | Mammography | Sensitivity: 95%, specificity: 87.8%, accuracy: 91%. |
Gufler et al. [15] | 2000 | HF B-mode US | MCs in non-palpable invasive carcinomas, in situ carcinomas and benign lesions in vivo | 46 | LAT | 7.5 | 0.1-1 | Clustered | Mammography /histology | MCs in malignant lesions were reliably identified by HF B-mode US, but they were difficult to detect in fibrocystic breast lesions. |
Teh et al. [16] | 2000 | HF B-mode US | MCs without associated mammographic or palpable masses in vivo | 44 | LAT | 13 | - | Clustered | Mammography /histology | Isolated clustered MCs were visualized by HF B-mode US in 41 cases. |
Moon et al. [17] | 2000 | HF B-mode US | MCs in hypoechoic benign and malignant masses in vivo | 94 | LAT | 10, 12 | - | Clustered/linear /segmental | Mammography /histology | The visibility at high-frequency ultrasound was much higher in malignant MCs compared to benign MCs. |
Cheung et al. [18] | 2002 | HF B-mode US | Mammographically detected MCs without a mass in vivo | 66 | LAT | 7, 5-9 | - | Clustered/linear /segmental | Mammography /histology | High-frequency ultrasound could depict MCs without an associated mass on mammography. |
Nagashima et al. [4] | 2005 | HF B-mode US | Mammographically detected MCs in DCIS in vivo | 73 | LAT | 7.5-13 | - | Clustered/linear/segmental | Mammography /histology | The lesions associated with MCs were identified in 74% by HF B-mode US. |
Stoblen et al. [19] | 2011 | HF B-mode US | Mammographically detected MCs in BI-RADS 4a patients in vivo | 52 | LAT | 13 | - | Clustered/linear/segmental | Mammography / histology | Detection rate: HF B-mode US: 98.1%, ApliPureTM: 100%, MicroPureTM: 25%. |
Huang et al. [20] | 2017 | HF B-mode US | MCs in screening mammography in vivo | 133 | - | - | - | —— | Mammography | Sensitivity: 87.5%, specificity: 75%, accuracy for malignancy: 82.1%. |
Authors | Year | Method | Study | N | T | TCF (MHz) | Size* (mm) | Pattern | Reference standard | Findings |
Machado et al. [11] | 2012 | MicroPureTM | Mammographically identified MCs in vivo | 20 | LAT | 14 | - | Isolated | Mammography | MicroPureTM images showed more MCs than HF B-mode US, but still less than mammography. |
Grigoryev et al. [30] | 2014 | MicroPureTM | Lesions with suspicious MCs in vivo | 104 | LAT | 9 | - | —— | Mammography | The detection of MCs with MicroPureTM imaging in breasts with a hypoechoic focal lesion correlated well with digital mammography. |
Tan et al. [24] | 2015 | MicroPureTM | Female patients with suspicious lesions | 135 | LAT | 5-14 | - | —— | Histology | MicroPureTM imaging yielded better performance in MC detection than HF B-mode US and Doppler US did. |
Park et al. [25] | 2016 | MicroPureTM | Grouped MCs without a mass on screening mammography | 9 | LAT | 7-18 | - | Clustered | Mammography/histology | MicroPureTM imaging was a promising US technique that could improve the sensitivity for detecting grouped MCs that were not associated with mass in the breast on mammography. |
Machado et al. [31] | 2018 | MicroPureTM | Surgical breast specimens in vitro | 22 | LAT | 14 | - | Isolated | Mammography | MicroPureTM identified more breast MCs than B-mode US in surgical breast specimens in vitro. |
Machado et al. [32] | 2018 | MicroPureTM | Lesions with suspicious MCs in vivo | 100 | LAT | 14 | - | Isolated | Mammography | MicroPureTM may represent a new imaging method for guiding a biopsy to areas of MCs. |
Fatemi et al. [44] | 2002 | Elastography | MCs in excised tissue samples ex vivo | 3 | TCT | 3 | ≥ 0.11 | —— | Mammography/histology | VA was capable of detecting small breast MCs, which could be delineated from within dense and sclerotic tissues. |
Alizad et al. [45] | 2004 | Elastography | MCs in excised tissue samples ex vivo | 74 | TCT | 3 | ≥ 0.11 | —— | Radiograph/histology | Visual inspection of VA guided by radiograph confirmed 78.4% of MCs. |
Gregory et al. [48] | 2015 | Elastography | Mammographically identified MCs in benign masses in vivo | 3 | LAT | 5, 5-14 | - | Clustered/isolated | Mammography | The presence of large isolated MCs and highly concentrated clusters of MCs could introduce areas with apparent high elasticity in SWE. |
Authors | Year | Method | Study | N | T | TCF (MHz) | Size* (mm) | Pattern | Reference standard | Findings |
Labyed et al. [54] | 2011 | TR-MUSIC | Phantom experiments | - | LAT | - | 0.25 | Isolated | Synthetic aperture US | Synthetic-aperture US had great potential for detecting breast MCs. |
Labyed et al. [57] | 2013 | PC-MUSIC | Computer simulation/phantom experiments | - | LAT | 7.5 | 0.25 | Isolated | Mammography | The accuracy of target localization and image resolution were improved. |
Huang et al. [58] | 2013 | PC-MUSIC | Mammographically identified MCs in cysts, masses or breast tissues in vivo | 40 | LAT | 7.5 | - | —— | Mammography | The super-resolution US PC-MUSIC imaging with synthetic-aperture US data could provide a new imaging modality for detecting breast MCs in clinic. |
Bahramian et al. [62] | 2014 | High spatial frequency | Computer simulation | - | LAT | 6.81 | - | Isolated | —— | The complement images that recovered the missing information in the display stage of B-mode imaging could resolve MCs. |
Bahramian et al. [64] | 2018 | High spatial frequency | Computer simulation | - | - | - | - | Isolated | —— | Supplementary intensity processing provided about twice the spatial information of the center frequency of the ultrasound system, which significantly enhanced the contrast of MCs. |
Denarie [68] | 2010 | SURF | Computer simulation | - | LAT, CAT | 0.9(LF); 8, 10(HF) | - | —— | —— | Using simulated data, the linear scattering components in the image was reduced by 29 dB, ensuring a 4 dB contrast level between MCs and surrounding tissues. |
Florenaes et al. [70] | 2017 | SURF | Breast phantom (CIRS model 073) | 1 | LAT | 8, 0.8 | - | Isolated | Mammography | By transmitting multiple dual frequency band pulse complexes, the scattering from normal tissue was suppressed and the signal from the MCs was enhanced. |
Kang et al. [74] | 2011 | PAI | Ex vivo experiments | 7 | LAT | 7 | < 1 | —— | Mammography | The feasibility of PAI to construct the images of MCs with high spatial and contrast resolutions was proved. |
Authors | Year | Method | Study | N | T | TCF (MHz) | Size* (mm) | Pattern | Reference standard | Findings |
Kang et al. [75] | 2012 | PAI | Three-dimensional ex vivo data | 2 | LAT | 7.2 | < 1 | Clustered | Mammography | PAI could provide optical contrast on breast MCs and their locations agreed well with their real positions. |
Kim et al. [73] | 2014 | PAI | Core-biopsied specimens | 21 | LAT | 7 | - | —— | Mammography | Photoacoustic imaging might serve as an alternative in overcoming the limitations of the conventional US imaging. |
Kang et al. [76] | 2015 | PAI | Three-dimensional ex vivo data | - | LAT | 7.5 | - | Clustered/isolated | Mammography | PAI might be more sensitive and specific in breast cancer screening than mammography. |
Taki et al. [83] | 2012 | Correlation technique | MC phantoms (spherical glass beads in swine breast tissue) | 4 | LAT | 7.5 | 0.1, 0.2, 0.3 | —— | —— | Using small glass beads to mimic MCs, the method based on the deterioration in the cross-correlation between adjacent scan lines had the potential to depict small MCs without acoustic shadowing. |
Huang et al. [87] | 2014 | Beamforming | Volunteer in vivo | - | LAT | - | - | Isolated | —— | The beamforming technique had potential for MC detection. |
Shankar [90] | 2013 | Envelope statistics | Phantom data modified to include bright spots to represent MCs | 2 | LAT | 6 | - | Isolated | —— | Higher speckle factors (seen with the McKay density) could be used to isolate and display MCs. |
Liao et al. [91] | 2014 | strain-compounding speckle factor imaging | Lesions with suspicious MCs in vivo | 26 | LAT | 7.5 | - | —— | B-mode US | The strain-compounding speckle factor imaging method was more effective at discriminating between MCs and false MCs. |
*: Mean size (diameter) of individual MCs; MC: Microcalcification; US: ultrasound; HF: High-frequency; VA: Vibro-acoustography; SWE: shear wave elastography; TR: time reversal; PC: phase coherent; MUSIC: multiple signal classification; SURF: second order ultrasound field; PAI: photoacoustic imaging. N: number of patients or specimens; T: transducer; TCF: transducer center frequency; LAT: linear array transducer; CAT: curvilinear array transducer; TCT: two-element confocal transducer. |
MCs appear as bright spots on B-mode ultrasound. However, the speckle pattern and some tissue structures seen on B-mode ultrasound may look like MCs. High-frequency B-mode ultrasound increases the imaging resolution by increasing the transducer center frequency to generally above 7 MHz.
Yang et al. reported that, high-frequency B-mode ultrasound achieved a sensitivity of 95%, a specificity of 87.8% and an accuracy of 91% in the detection of MCs when they were within a breast mass [14]. Gufler et al. showed that high-frequency B-mode ultrasound could reliably identify MCs in malignant breast lesions, but it is difficult to detect MCs in fibrocystic lesions [15]. Teh et al. demonstrated that clustered MCs could be visualized using high-frequency B-mode ultrasound in over 90% of cases [16]. Moon et al. reported that high-frequency B-mode ultrasound could depict more breast masses associated with malignant MCs, especially for clustered MCs larger than 10 mm [17]. Cheung et al. reported that high-frequency B-mode ultrasound could depict MCs without a mass on mammography [18]. Nagashima et al. reported that the lesions associated with MCs were identified by high-frequency B-mode ultrasound in 54 of 73 cases (74%) [4]. Stoblen et al. evaluated the diagnostic value of high-frequency B-mode ultrasound for the detection of MCs in BI-RADS 4a patients. It was shown that the detection rate of MCs was 98.1% [19]. Huang et al. reported that the high-frequency B-mode ultrasound has high sensitivity, specificity, and accuracy for malignancy (87.5%, 75%, and 82.1%, respectively) [20]. By using in vivo patient imaging, Huang et al. demonstrated that high-frequency synthetic-aperture B-mode ultrasound was a promising imaging modality for detecting breast MCs [21].
It is easier to visualize MCs when they are located inside solid masses, because the solid masses can provide a hypoechoic background that improves the visualization of the bright echo associated with MCs, and the detection rate of MCs in malignant tumors is significantly higher than that in benign ones [4,17,18,19]. However, identifying isolated MCs within normal breast tissues remains challenging. Because MCs are so small, normal fibroglandular tissues or speckle artifacts can interfere with their visualization [17,18,19,22].
MicroPureTM imaging (Toshiba America Medical Systems, Tustin, CA, USA) is an innovative ultrasound imaging technology, which improves the visualization of breast MCs on B-mode ultrasound [23,24,25,26,27,28,29]. MicroPureTM imaging is based on a nonlinear imaging and speckle suppression technique, log-(constant false alarm rate) (log-CFAR). The brightness of each pixel was compared with the average brightness of the surrounding pixels [26]. Let A(i, j) denote the brightness of a pixel at the coordinate (i, j); a new density B(i, j) was computed from the brightness of the surrounding n pixels (Ck):
B(i,j)=A(i,j)−1nn∑k=1Ck | (1) |
By optimizing the horizontal direction, isolated bright echoes were extracted from a heterogeneous background [23,24,25]. The filtered image of breast MCs was compounded with the original image, and the final MicroPureTM image showed high-brightness dots (suspicious MCs) within a dark blue color overlay on the B-mode ultrasound image.
Machado et al. evaluated MicroPureTM and traditional B-mode ultrasound imaging for identifying breast MCs, using mammography as the reference standard [11]. It was shown that MicroPureTM images identified more MCs than high-frequency B-mode ultrasound, but still less than mammography [11]. Grigoryev et al. compared ultrasound and mammography imaging for the detection of MCs in women with dense breast. It was shown that the detection of MCs in a hypoechoic focal lesion by MicroPureTM imaging was closely related to mammography [30]. Tan et al. evaluated 70 pathologically proven breast lesions with suspected MCs and showed that MicroPureTM imaging yielded better performance in MC detection than high-frequency B-mode ultrasound and Doppler ultrasound did [24]. Park et al. showed that MicroPureTM imaging could improve the sensitivity in detecting grouped MCs that were not associated with a mass in the breast on mammography, and showed a larger number of MCs than high-frequency B-mode ultrasound did [25]. A recent study by Machado et al. used a total of 22 breast surgical specimens obtained from patients with diffuse MCs, and showed that MicroPureTM (mean number of MCs detected: 14.0 ± 12.0) can identify more breast MCs and fewer artifacts than high-frequency B-mode ultrasound (mean number of MCs detected: 3.0 ± 3.2) [31]. Machado et al. further investigated 100 female patients with breast MCs in vivo [32]. It was shown that MicroPureTM could be used to identify suspicious areas with breast MCs, but it was difficult to effectively characterize these areas. Instead, MicroPureTM had a potential for guiding a biopsy to areas of MCs.
The MicroPureTM technique has shown its capability in improving the visualization of MCs ex vivo and in vivo [11,23,31,32]. It overcomes the disadvantage that traditional B-mode ultrasound is difficult to identify isolated MCs in normal breast tissues, and quickly determines the number and distribution of MCs. However, not all bright echoes are MCs. Because the high echoes of fibrous tissues in gland are easily confusing with those of MCs, it will cause a higher false positive rate.
In addition to MicroPureTM, there are some other B-mode ultrasound image processing techniques for breast MC detection. Chang et al. employed three-dimensional (3D) ultrasound to detect breast MCs, using a slice by slice strategy [33]. In each slice, the top-hat filter was adopted to detect bright spots and four criteria were designed to select the spots as candidate MCs. Those spots appearing in sequent slices at the same position were detected as MCs. Chang et al. also proposed using adaptive speckle reduction and top hat filter for detecting breast MCs [34]. The sensitivity of MC detection was 80.3%, and the false positive rate was 3.1% [34]. Cho et al. used image enhancement and threshold adjacency statistics to improve the detection rate of MCs in ultrasound images, with an accuracy of 82.75% [35]. Islam combined multiple image processing techniques to improve the performance of MC detection [36]. Zhuang et al. employed a pattern recognition kernel to distinguish the small hyperechoic spots in B-mode ultrasound images collected from a concave automated breast ultrasound scanner [37]. The B-mode ultrasound image post-processing techniques can effectively improve the visualization of MCs, but they are limited by the inherent limitations of B-mode ultrasound imaging.
Ultrasound elastography was first proposed in the early 1990s [38] and started to be used in the clinical setting in 1997 [39]. Elastography usually consists of three steps: (ⅰ) create a distortion (displacement) in the tissue, (ⅱ) track and process the tissue response to infer the mechanical properties of the tissue, and (ⅲ) display the results to the operator, usually as an image [40,41]. Elastography maps the elastic properties and stiffness of tissues, providing diagnostic information about the presence or status of disease. Breast calcifications have very different elastic behavior than normal or abnormal breast tissues do. Studies reported that the Young's modulus of breast calcifications was 20-117 kPa, while the Young's modulus of benign and malignant breast masses ranged from 10 to 80 kPa and 30 to 180 kPa, respectively [42,43].
Fatemi et al. proposed using acoustic radiation force with low frequency (kHz) to vibrate breast tissue; the obtained response was used to generate images related to tissue stiffness [44]. It was shown that vibro-acoustography (VA) could detect breast MCs with diameters as small as 110 μm, and dense breast tissues might not interfere with the imaging. Alizad et al. evaluated the performance of VA in detecting MCs in 74 breast tissue samples; 78.4% of MCs were correctly detected [45,46]. Gregory et al. investigated the effects of large isolated macrocalcifications and grouped MCs on shear wave elastography (SWE) by three sets of phantoms with different sizes and distributions [47]. It was shown that macrocalcifications had a higher effect on SWE than MCs did. In addition, the dispersion of MCs could decrease elasticity values and had less bias effect than concentrated clusters of MCs. Gregory et al. demonstrated that the presence of clustered MCs and macrocalcifications in benign breast masses in vivo could induce the appearance of high stiffness regions when they were evaluated by SWE [48]. Consequently, the method was easy to misdiagnose benign breast tumors containing MCs as malignant.
The advantage of ultrasound elastography is its ability to classify breast tissues according to hardness. Conventional B-mode ultrasound imaging is susceptible to the interference of various factors when detecting breast MCs. Ultrasound elastography may overcome this limitation. However, there still is a need to quantify the elasticity elevations of benign masses with different types of MCs.
The principle of TR is briefly described as follows. An ultrasound transducer transmits a plane wave that propagates toward the target and is reflected off it. The reflected wave returns to the ultrasound transducer, where it looks as if the target has emitted a weak signal. The ultrasound transducer reverses and retransmits the signal, and a more focused wave propagates toward the target. As the process is repeated, the waves become more and more focused on the target.
The TR with multiple signal classification (TR-MUSIC) imaging algorithm, developed by Devaney, was used to estimate the position of one or more coherent scatterers [49]. The major characteristic of TR is that it does not need to know the propagation model of the sound beam in the medium or the signal delay of the transducer array, but it can generate an automatic focusing effect on the signal [50,51]. TR-MUSIC can separate the target with high scattering amplitude from the inhomogeneous medium; it can also achieve super-resolution in the presence of multiple scattering [52,53]. Therefore, this method has potential for detecting MCs. Consider an array of N ultrasound transducer elements interrogating inhomogeneous media. Each element is excited sequentially and the backscattered signals are received by all elements, yielding the inter-element response matrix K at each frequency. The matrix K is then used to compute the TR matrix T=K∗K, where the superscript * denotes the complex conjugate. TR-MUSIC can be implemented by performing singular value decomposition (SVD) on the array response matrix K. The pseudo-spectrum PS for generating the image is given by [54].
PS(r)=(N∑m0=M+1|<μ∗m0,Ar>|2)−1 | (2) |
where PS(r) is the intensity at any point r in the imaging area; μm0 is the m0th eigenvector; Ar is the vector Green's function of the array element Rj to an arbitrary point r and written as
Ar=[A(R1,r),A(R1,r),...,A(RN,r)] | (3) |
The inner product <u∗m0,Ar> will vanish whenever r corresponds to the location of one of the scatterers.
When the number of point scatterers in the imaging plane is larger than the number of transducer elements, the TR-MUSIC algorithm is no longer valid [49]. To this end, Labyed et al. developed a windowed TR-MUSIC method to image small targets with high resolution [54]. The imaging plane was divided into several sub-regions, and each sub-region was imaged separately. The number of targets within the sub-regions was less than the number of transducer elements. Islam and Kaabouch investigated the impact of sampling frequency and speed of sound on TR-MUSIC by using numerical phantoms that mimic breasts with MCs, and showed that the quality of images produced by TR-MUSIC increased with increasing sampling frequency and speed of sound [55]. TR-MUSIC can accurately locate point targets and has higher lateral resolution than synthetic-aperture ultrasound imaging. However, when there is noise, the TR-MUSIC super-resolution capability will be seriously weakened.
The method of phase-coherent MUSIC (PC-MUSIC) was developed by Asgedom et al. [56]. This method modified TR-MUSIC and utilized the phase information from multiple frequencies to reduce noise effects and to maintain the super-resolution capability. Compared with TR-MUSIC, PC-MUSIC has almost no improvement in lateral resolution, but the axial resolution is significantly improved. However, due to neglecting the phase response between transducer elements, the target location is not accurate. Subsequently, Labyed et al. compensated for the phase response of the transducer elements to improve the accuracy of target localization and image resolution [57]. Huang et al. applied this method to clinical patients, and showed that it could get clear images of breast MCs [58].
TR-MUSIC imaging shows an elongation artifact that decreases the axial resolution. Consequently, it cannot identify axially adjacent point targets accurately. The artifacts depend on the point spread function and signal-to-noise ratio (SNR) of the transducer array at the scatterer position [57]. Although PC-MUSIC imaging obtains better imaging performance, the specific location of the scatterers must be known in advance to compensate for the phase factor. Additionally, PC-MUSIC has a high computational complexity.
The principle is to recover high spatial frequency information which is lost in the processing of conventional B-mode ultrasound imaging. Comparing the information in the original radiofrequency (RF) data with the available information in B-mode ultrasound images, it was found that B-mode processing can eliminate up to 50% of the information that can be helpful for diagnosis [59]. Nguyen et al. showed that the RF signal can contain valid echo information about tissue scattering at spatial frequencies twice that of the pulse-envelope bandwidth [60]. Bahramian et al. measured the performance efficiency of B-mode images with ideal RF data, and showed that the task-energy efficiency could predict the performance efficiency, especially for large-area tasks [61]. Compared with the corresponding RF data, the loss of diagnostic information in B-mode images was verified. For small-area tasks, such as MC detection, the loss of information in the B-mode image was more significant [59].
In order to recover the lost high spatial frequency information related to MCs, Bahramian et al. analyzed the flow of signal energy in various stages of image formation [61,62]. The signal processing method of task-energy efficiency was used to retrieve the missing information, which was displayed as a complement image. The original B-mode image was combined with the complement image. The combined image ideally contained all the task information captured in the RF data. Computer simulation and phantom experiments showed that the small MC in the heterogeneous lesion area was detectable in the complement images. Moreover, with the increase of ultrasound pulse frequency, more information could be captured in both the original B-mode image and the complement image, which further enhanced the detection of MCs. Recently, on the basis of Abbey et al.'s work [63], Bahramian et al. proposed using complement intensity imaging to recover the high spatial frequency information loss at the display stage [64]. Because an image composed of intensity signals provided the same diagnostic performance as B-mode (envelope) signals for lesion detection, analysis of either signal was related to the quality of the ultrasound image [65]. The complement intensity processing could provide spatial information about around twice the central frequency of the ultrasound system; the contrast of MCs was significantly enhanced [64]. In addition, the spatial information provided by compensated intensity processing could be extracted from the autocorrelation of RF signals sampled at the Nyquist rate, without additional processing cost.
It is important to note that the methods of high spatial frequency were all realized under ideal conditions [59,62,63]. In the process of analyzing the energy efficiency of transmission tasks in the whole imaging stage, it is not considered that some tasks of scattering objects may lose energy in the acquisition process, and it is assumed that the filter in the display stage does not introduce any noise. In addition, in the processing of echo signal intensity, it is difficult to record multiple scattering signals from space-registered tissues. Usually, only one kind of scattering object is captured, and the information expressed by this separate implementation may be very weak.
The principle is to combine the advantages of low-frequency (LF) and high-frequency (HF) imaging pulses. The SURF imaging technique was developed to improve the contrast resolution for ultrasound contrast agents [66]; it has potential in detection and characterization of nonlinear scattering in tissues, including the imaging of MCs.
HF pulse imaging has a high resolution, but a short wavelength and a weak penetration. On the contrary, LF pulse imaging is less affected by damping attenuation and has a stronger penetration, but the resolution is lower than HF pulse imaging. SURF is based on the transmission of dual-frequency band pulses from the same acoustic source using an LF manipulation pulse and an HF imaging pulse, while conventional B-mode ultrasound imaging uses a single frequency band pulse, as shown in Figure 2. The frequency ratio between the LF and the HF is typically 1:8-10 [67]. The HF is used to image tissues or nonlinear scatterers under the influence of the manipulation pulse. The purpose of the LF pulse is to manipulate the scattering and propagation of the HF imaging pulse; the LF pulse is only transmitted but not received.
However, imaging of low-intensity nonlinear scatterers such as MCs requires higher manipulation pressures. Denarie proposed to characterize and correct the nonlinear forward propagation effect [68]. The simulation results showed that the linear scattering components in the image were reduced by 29 dB, ensuring a 4 dB contrast level between MCs and surrounding tissues. Jahren et al. effectively suppressed reverberation noise by time delay correction [69]. The SNR for scatterers from stiff particles was increased. But nonlinear scattering did not increase by suppression of linear scattering. Florenaes et al. used a phantom, which had 100-300 μm MCs embedded in some spherical regions mimicking dense lesions to verify SURF imaging performance [70]. Nonlinear scattering was detected by transmitting multiple pulse complexes with different LF amplitudes, and received signals were processed by the delay correction subtraction method. The results showed that the background scattering could be suppressed by 20-40 dB, improving the detection capability of MCs.
One of the challenges of SURF imaging techniques is to control the time interval between LF and HF pulses in the propagation process of the pulse complex. The co-propagating HF pulses will distort under non-homogeneous LF pressure. The distortion of HF pulses masks the nonlinear scattering. How to optimize the radiation aperture of LF and HF pulses, to produce minimum pulse distortion, and to improve the ability of SURF reverberation suppression still is a challenge.
PAI is an emerging hybrid imaging modality with high spatial and contrast resolutions [71]. PAI can potentially overcome the shortcomings of mammography and ultrasound, and take the advantages of wavelength selective with high optical contrast and high ultrasonic resolution to detect breast lesions and MCs. Photoacoustic (PA) signal is generated by a laser (excitation source) irradiation of the biological tissue [72,73]. The irradiated tissue area and its adjacent area will absorb the pulsed laser energy and convert it into thermal energy. The pressure or stress changes due to thermo-elastic expansion will excite and propagate ultrasound waves. An ultrasound transducer is used to detect ultrasound signals, which are reconstructed to form images representative of optical absorption distribution.
Kang et al. investigated the effects of transmitting laser pulse by ex vivo experiments with different wavelengths ranging from 680 to 1000 nm [74]. When the laser wavelength ranged from 690 to 700 nm, the PA signals from MCs had the maximum amplitude. The feasibility of PAI to construct the images of MCs with high spatial and contrast resolutions was demonstrated. They also showed that PAI could provide optical contrast on breast MCs and the locations of MCs agreed well with their real positions [75]. Kim et al. compared PAI of breast specimens containing MCs (n = 11) and a non-MC group (n = 10), using laser pulses with two wavelengths (700 and 800 nm) [73]. It was shown that the PA signal amplitude of MCs was different from that of normal breast tissues. The PAI ratio (the ratio of signal amplitude at 700 and 800 nm) in the MC group was significantly higher than the PAI ratio of cores included in the control group. The sensitivity and specificity of PAI were 90.91% and 80.0%, respectively. Kang et al. showed that the intensity of PA signals from MCs decreased with increasing laser wavelength from 700 to 800 nm, while the composition of surrounding tissues such as fat and glandular tissues remained almost unchanged [76]. However, the number of scattered MCs in the sample became less and less with increasing wavelength. The scattered MCs generally belong to calcium oxalate and mostly are benign, which means that PAI may be more sensitive and specific in breast cancer screening than mammography.
PAI overcomes the limitations of conventional B-mode ultrasound imaging and can distinguish between normal tissues and MCs in a breast. It has important application prospects for improving early diagnosis of breast cancer. However, PAI is susceptible to noise interference, and the choice of wavelengths will have an important impact on imaging performance.
Taki et al. reported a correlation technique for ultrasound calcification depiction, which utilized the decrease of correlation between adjacent scan lines caused by the echo waveform change [77]. Because calcification has greater acoustic impedance than soft tissue, the ultrasound pulse waveform changes greatly at the calcified position. The existence of small calcifications in a heterogeneous medium was supposed to suppress the correlation between adjacent scan lines [77,78,79,80,81,82]. When the region of interest contains small calcification, the variance of the correlation coefficient in inhomogeneous media will increase. The correlation method improves the ultrasonic sensitivity and has the potential to describe small calcifications without acoustic shadowing. However, the correlation coefficients are also susceptible to noise. The effect of SNR on the distribution of correlation coefficients between adjacent scan lines may be considered in future developments. In 2014, Taki et al. proposed another imaging method based on frequency domain interferometry (FDI) to improve the performance of B-mode ultrasound in depicting small calcifications [83]. The FDI imaging method suppresses the contribution of undesired echo signals when their waveforms differ from the waveform of a small calcification echo. The results showed that when the SNR is 0 dB, the FDI method may depict small calcifications with a diameter of 0.2 mm that are difficult to identify in conventional B-mode images.
The twinkling artifact in color Doppler ultrasound was employed to detect breast MCs [84,85]. The twinkling artifact is a well-known color Doppler artifact [86]. Tsujimoto investigated the twinkling artifact of breast MCs and found that the twinkling artifact may assist in the detection of calcified foci [84]. A prospective study of 46 patients demonstrated that the twinkling artifact on color Doppler ultrasound is useful in the ultrasound management of suspicious breast MCs [85].
The ultrasound channel data contains more information than the B-mode images obtained after the processing of beamforming and envelope detection [87]. Therefore, channel-data-based beamforming techniques can provide additional information related to MCs. Huang et al. developed MC detection algorithms based on coherence factor and dominance of the first eigenvalue of covariance matrices [87]. These two parameters were used to yield a binary MC map that assisted in indicating the presence of MCs. The preliminary results showed the promise of the algorithm for MC detection. However, the performance needs to be further validated. Huang et al. proposed a super-resolution ultrasound imaging method for detecting breast MCs; the method was based on SVD, a factorization scheme, and a wave-equation reflection imaging scheme, and could detect MCs at a full spatial resolution for numerical phantoms [88].
Liao et al. proposed using a strain-compounding technique with Nakagami imaging to detect breast MCs [89]. Shankar proposed a three-parameter statistical model of McKay density for the ultrasonic backscattered echo from tissues containing MCs [90]. The method could be used to separate and display MCs according to the observed high speckle factor. However, Shankar [90] did not investigate the use of ultrasound speckle factor to detect actual MCs within breast lesions. To this end, Liao et al. proposed a strain-compounding technique with speckle factor (SF) imaging to detect MCs in human breast tissues [91]. Their method could be used to distinguish between the relative contributions of scattering from MCs versus false MCs. However, the detection capability was limited by the unfitting scattering statistical models, and estimating error was caused by manual compression conditions.
MC is an important indicator of early breast cancers. In this review, we summarized the current ultrasonic detection methods for breast MCs. To the best of our knowledge, this paper is the first to provide a comprehensive review on the state-of-art ultrasound techniques for detecting breast MCs.
At present, high-frequency B-mode ultrasound imaging, MicroPureTM imaging, and ultrasound elastography techniques have been commercialized. Other detection techniques are mainly involved with experimental studies including computer simulation, phantom experiments, and experiments of breast tissue specimens containing MCs. Refer to Table 1 again. Most researchers used mammography as the reference standard; linear array transducers were mostly used to detect breast MCs. For the pattern of calcification distribution, most researchers have improved the detection rate of isolated MCs over conventional B-mode ultrasound. However, there still is a high false positive rate. How to distinguish between normal breast tissues and isolated MCs with hyperechoic background remains a challenge. In addition, clustered MCs are easy to detect as macrocalcifications, which are likely benign, thus causing misdiagnosis. In addition, the size of MCs has an important indication of the performance of detection, but only a few studies have reported it.
Table 2 shows the advantages and limitations of various methods for ultrasonic detection of breast MCs. The advantages and disadvantages of some detection methods are briefly discussed as follows. The TR-MUSIC and PAI techniques show the feasibility for MC detection, but they are vulnerable to noise interference. The PC-MUSIC method retains phase information, but it cannot locate the scatterers accurately. Although PC-MUSIC improves the positioning accuracy after compensating for the phase response of the transducer elements, its computational complexity is high as it uses TR-MUSIC to pre-locate the scatters. SURF enhances the nonlinear scattering characteristics of MCs and enhances the contrast against surrounding tissues. However, to design a dual-band ultrasound transducer for SURF imaging is a challenge. We should consider not only the noise suppression but also the minimum distortion of imaging pulses. Retrieving high spatial frequency information is easy to implement. However, it should be noted that the experimental study is conducted in an ideal situation and the accuracy needs to be further validated. The correlation, FDI, and beamforming techniques have shown the potential for detecting MCs and have improved the sensitivity of conventional B-mode ultrasound, but they are susceptible to noise and the accuracy needs further verification. Ultrasound elastography is based on the different elastic modulus of breast MCs against surrounding breast tissues. The elastic modulus of breast MCs is much higher than that of glandular tissues. Therefore, dense tissues may have little influence on the detection of MCs. It may overcome the limitations of some detection techniques and has important application prospects.
Examination method | Advantages | Limitations |
HF B-mode US | High specificity. | The total detection rate of breast MCs is low. |
MicroPureTM | Enhancement of contrast of MC against background. | False positive rate is high. |
Ultrasound elastography | Effective detection of coarse calcification and clustered MCs in breast masses. | It is easy to misdiagnose benign tumor with MCs as malignant. |
TR-MUSIC | High precision in positioning scatterers and good horizontal resolution. | It is easily affected by noise and the axial resolution is low. |
PC-MUSIC | Improve axial resolution of TR-MUSIC. | The positioning is not accurate. |
High spatial frequency | It is easy to implement. | The method is too idealized and the accuracy needs further verification. |
SURF | It can suppress the linear scattering of background and increase the nonlinear scattering of MCs. | Imaging pulse is easily distorted. |
PAI | It combined the high contrast of optical imaging and high resolution of acoustic imaging. | It is easily affected by noise. |
Correlation technique | The sensitivity of conventional B-mode ultrasound is improved. | It is easily affected by noise. |
FDI | The sensitivity of conventional B-mode ultrasound is improved. | It is easily affected by noise. |
Beamforming | The sensitivity of conventional B-mode ultrasound is improved. | The accuracy needs further verification. |
Strain-compounding speckle factor imaging | Improve the effective identification of MCs and false MCs. | Selection of scattering statistical models and manual compression can cause errors. |
MC: microcalcification; US: ultrasound; HF: high-frequency; VA: Vibro-acoustography; SWE: shear wave elastography; TR: time reversal; PC: phase coherent; MUSIC: multiple signal classification; SURF: second order ultrasound field; PAI: photoacoustic imaging; FDI: frequency domain interferometry |
It is interesting to discuss different imaging features for the differing ultrasonic MC detection methods reviewed in this article. In high-frequency B-mode ultrasound imaging, detected breast MCs have a higher echogenicity than surrounding tissues do, thus appearing as bright spots. In MicroPureTM imaging, detected breast MCs are high-brightness dots. In ultrasound elastography, detected breast MCs may have a different elasticity and thus show a contrast compared with surrounding tissues. In TR-MUSIC and PC-MUSIC imaging and high spatial frequency imaging, breast MCs appear as brighter spots, compared with their appearance in conventional B-mode ultrasound imaging. Breast MCs are brighter spots in SURF imaging with a higher contrast, compared with their appearance in conventional B-mode ultrasound imaging. Breast MCs produce PA signals with their amplitude different from normal breast tissues, so there is an imaging contrast in PAI.
The detection methods reviewed in this paper have improved the performance of traditional B-mode ultrasound for MC detection to a certain extent, but there still is a gap compared with mammography. The future developments of ultrasonic detection methods for breast MCs are suggested as below.
1. Real-time ultrasound detection of breast MCs. Ultrasound is widely used, partially because of its real-time performance. Because some detection algorithms are too complex, even if MCs can be characterized, it may not be suitable for real-time clinical application. Therefore, an increase in the time efficiency of ultrasonic breast MC detection methods may be considered in future developments.
2. The comparison and evaluation of ultrasonic breast MC detection methods. Due to the difference in experimental methods, reference standards, and MC distributions, various detection techniques lack intuitive comparability. In addition, the use of histology as the reference standard is also limited by the fact that histologic processing can lead to the loss of MCs. A unified reference standard can be set up to detect the same MCs using different ultrasonic detection techniques to find out a more reliable detection method.
3. Extensive in vivo validation. Although some ultrasonic detection techniques have shown satisfying performance for computer simulation, phantom experiments, and experiments of breast tissue specimens containing MCs, the extensive in vivo validation of ultrasonic detection techniques remains an issue. Comparison of biopsy examinations with noninvasive validations of different ultrasonic MC detection methods in vivo may be considered in future clinical research.
4. Integration of different ultrasonic detection techniques. Because conventional B-mode ultrasound imaging is difficult to detect breast MCs, researchers have begun to introduce new concepts and methods to the ultrasound detection of breast MCs, such as PAI and SURF techniques. In future developments, the effective combination of different ultrasonic signal/image processing techniques can be considered, in order to obtain a high-contrast visualization of breast MCs.
5. Diagnosis and classification of MC malignancy. Current ultrasound detection methods only determine the existence of breast MCs, but there are few reports on how to evaluate the malignancy of MCs. Therefore, it is a challenge to develop ultrasound methods that can not only detect MCs, but also identify the malignancy of MCs and classify their pathological types.
6. Combination of computer-aided diagnosis (CAD) systems with automatic detection of MCs in breast ultrasound. Ultrasound is more dependent on operators than mammography, and good imaging results require well-trained and experienced radiologists. However, well-trained experts may have a high inter-observer variation rate. The computerized techniques for MC detection on breast ultrasound can reduce inter-observer variability. However, there are few reports on ultrasound CAD systems for breast MCs. Therefore, CAD systems of breast ultrasound [92,93,94,95,96,97,98,99,100,101,102] should be investigated further in future developments to incorporate automatic MC detection. Ultrasonographic features correlating with mammographic findings are valuable for differentiating between malignant and benign lesions, including the shape, orientation, margin, boundary, echo pattern, and posterior features of breast lesions [9]. A breast ultrasound CAD system is typically composed of four parts: preprocessing, segmentation, feature extraction and selection, and classification [92]. The incorporation of computerized breast MC detection/classification can enhance the capability of breast ultrasound CAD systems [92,93,94,95,96,97,98,99,100,101,102], especially for breast tumors with MCs. However, it should be noted that current breast ultrasound CAD systems [92,93,94,95,96,97,98,99,100,101,102] mainly use B-mode ultrasound images, while some of the ultrasonic MC detection techniques reviewed in this article are based on backscattered RF signals, such as ultrasound elastography. Therefore, breast ultrasound CAD systems incorporating quantitative ultrasound techniques using ultrasound RF signals [95] may be considered in future developments.
7. MC detection in 3D breast ultrasound. Conventional diagnostic ultrasound systems usually use two-dimensional (2D) ultrasound imaging, which can only show a cross-section of the tissue. A 2D image can result in a loss of spatial information and may cause superimposition of individual calcifications within the cluster. 3D breast ultrasound can offer an enhanced ultrasound tissue characterization, especially for detecting breast lesions and MCs [103,104,105,106]. Note that there are automated breast volume scanning (ABVS) 3D ultrasound systems that have been commercialized. Computerized detection and segmentation of breast tumors in ABVS ultrasound images have been investigated [107,108]. In future developments, 3D ultrasonic detection of breast MCs may be considered. In addition, the real-time performance of 3D ultrasonic breast MC techniques should be considered.
The authors would like to thank the anonymous reviewers for their valuable comments and suggestions. This work was supported in part by the Beijing Natural Science Foundation (Grant No. 4184081), the National Natural Science Foundation of China (Grant Nos. 11804013, 61871005, 71661167001, and 61801312), the Basic Research Fund of Beijing University of Technology, the International Research Cooperation Seed Fund of Beijing University of Technology (Grant No. 2018A15), and the Intelligent Physiological Measurement and Clinical Translation, Beijing International Base for Scientific and Technological Cooperation.
The authors declared they have no competing interests. The authors alone are responsible for the content and writing of the paper.
[1] | F. Bray, J. Ferlay and I. Soerjomataram, et al., Global cancer statistics 2018: GLOBOCAN estimates of incidence and mortality worldwide for 36 cancers in 185 countries, CA. Cancer J. Clin., 68 (2018), 394–424. |
[2] | M. E. Anderson, M. S. Soo and R. C. Bentley, et al., The detection of breast microcalcifications with medical ultrasound, J. Acoust. Soc. Am., 101 (1997), 29–39. |
[3] | R. Baker, K. D. Rogers and N. Shepherd, et al., New relationships between breast microcalcifications and cancer, Br. J. Cancer, 103 (2010), 1034–1039. |
[4] | T. Nagashima, H. Hashimoto and K. Oshida, et al., Ultrasound demonstration of mammographically detected microcalcifications in patients with ductal carcinoma in situ of the breast, Breast Cancer, 12 (2005), 216–220. |
[5] | S. Obenauer, K. P. Hermann and E. Grabbe, Applications and literature review of the BI-RADS classification, Eur. Radiol., 15 (2005), 1027–1036. |
[6] | J. R. Cleverley, A. R. Jackson and A. C. Bateman, Pre-operative localization of breast microcalcification using high-frequency ultrasound, Clin. Radiol., 52 (1997), 924–926. |
[7] | L. Frappart, I. Remy and H. C. Lin, et al., Different types of microcalcifications observed in breast pathology. Correlations with histopathological diagnosis and radiological examination of operative specimens, Virchows. Arch. A. Pathol. Anat. Histopathol., 410 (1986), 179–187. |
[8] | M. J. Radi, Calcium oxalate crystals in breast biopsies. An overlooked form of microcalcification associated with benign breast disease, Arch. Pathol. Lab. Med., 113 (1989), 1367–1369. |
[9] | I. Thomassin-Naggara, A. Tardivon and J. Chopier, Standardized diagnosis and reporting of breast cancer, Diagn. Interv. Imaging, 95 (2014), 759–766.10. P. L. Arancibia, T. Taub and A. López, et al., Breast calcifications: description and classification according to BI-RADS 5th Edition, Rev. Chil. Radiol., 22 (2016), 80–91. |
[10] | 11. P. Machado, J. R. Eisenbrey and B. Cavanaugh, et al., New image processing technique for evaluating breast microcalcifications: a comparative study, J. Ultrasound Med., 31 (2012), 885–893. |
[11] | 12. M. Ruschin, B. Hemdal and I. Andersson, et al., Threshold pixel size for shape determination of microcalcifications in digital mammography: A pilot study, Radiat. Prot. Dosimetry., (2005), 415–423. |
[12] | 13. F. Pediconi, C. Catalano and A. Roselli, et al., The challenge of imaging dense breast parenchyma: is magnetic resonance mammography the technique of choice? A comparative study with x-ray mammography and whole-breast ultrasound, Invest. Radiol., 44 (2009), 4421. |
[13] | 14. W. T. Yang, M. Suen and A. Ahuja, et al., In vivo demonstration of microcalcification in breast cancer using high resolution ultrasound, Br. J. Radiol., 70 (1997), 685–690. |
[14] | 15. H. Gufler, C. H. Buitrago-Tellez and H. Madjar, et al., Ultrasound demonstration of mammographically detected microcalcifications, Acta. Radiol., 41 (2000), 217–221. |
[15] | 16. W. L. Teh, A. R. Wilson and A. J. Evans, et al., Ultrasound guided core biopsy of suspicious mammographic calcifications using high frequency and power Doppler ultrasound, Clin. Radiol., 55 (2000), 390–394. |
[16] | 17. W. K. Moon, J. G. Im and Y. H. Koh, et al., US of mammographically detected clustered microcalcifications, Radiology, 217 (2000), 849–854. |
[17] | 18. Y. C. Cheung, Y. L. Wan and S. C. Chen, et al., Sonographic evaluation of mammographically detected microcalcifications without a mass prior to stereotactic core needle biopsy, J. Clin. Ultrasound, 30 (2002), 323–331. |
[18] | 19. F. Stoblen, S. Landt and R. Ishaq, et al., High-frequency breast ultrasound for the detection of microcalcifications and associated masses in BI-RADS 4a patients, Anticancer Res., 31 (2011), 2575–2581. |
[19] | 20. W. H. Huang, I. W. Chen and C. C. Yang, et al., The sonogaphy is a valuable tool for diagnosis of microcalcification in screening mammography, Ultrasound Med. Biol., 43 (2017), S28. |
[20] | 21. L. Huang, Y. Labyed and Y. Lin, et al., Detection of breast microcalcifications using synthetic-aperture ultrasound, SPIE Medical Imaging 2: Ultrasonic Imaging, Tomography, and Therapy, (2012), 83200H. |
[21] | 22. S. Bae, J. H. Yoon and H. J. Moon, et al., Breast microcalcifications: diagnostic outcomes according to image-guided biopsy method, Korean. J. Radiol., 16 (2015), 996–1005. |
[22] | 23. T. Fischer, M. Grigoryev and S. Bossenz, et al., Sonographic detection of microcalcifications - potential of new method, Ultraschall. Med., 33 (2012), 357–365. |
[23] | 24. R. Tan, Y. Xiao and Q. Tang, et al., The diagnostic value of Micropure imaging in breast suspicious microcalcificaion, Acad. Radiol., 22 (2015), 1338–1343. |
[24] | 25. A. Y. Park, B. K. Seo and K. R. Cho, et al., The utility of MicroPure ultrasound technique in assessing grouped microcalcifications without a mass on mammography, J. Breast Cancer, 19 (2016), 83–86. |
[25] | 26. N. Kamiyama, Y. Okamura and A. Kakee, et al., Investigation of ultrasound image processing to improve perceptibility of microcalcifications, J. Med. Ultrason., 35 (2008), 97–105. |
[26] | 27. P. Machado, J. R. Eisenbrey and B. Cavanaugh, et al., Evaluation of a new image processing technique for the identification of breast microcalcifications, Ultrasound Med. Biol., 39 (2013), S87. |
[27] | 28. P. Machado, J. R. Eisenbrey and B. Cavanaugh, et al., Ultrasound guided biopsy of breast microcalcifications with a new ultrasound image processing technique, Ultrasound Med. Biol., 39 (2013), S41. |
[28] | 29. P. Machado, J. R. Eisenbrey and B. Cavanaugh, et al., Microcalcifications versus artifacts: Initial evaluation of a new ultrasound image processing technique to identify breast microcalcifications in a screening population, Ultrasound Med. Biol., 40 (2014), 2321–2324. |
[29] | 30. M. Grigoryev, A. Thomas and L. Plath, et al., Detection of microcalcifications in women with dense breasts and hypoechoic focal lesions: Comparison of mammography and ultrasound, Ultraschall. Med., 35 (2014), 554–560. |
[30] | 31. P. Machado, J. R. Eisenbrey and M. Stanczak, et al., Ultrasound detection of microcalcifications in surgical breast specimens, Ultrasound Med. Biol., 44 (2018), 1286–1290. |
[31] | 32. P. Machado, J. R. Eisenbrey and M. Stanczak, et al., Characterization of breast microcalcifications using a new ultrasound image-processing technique, J. Ultrasound Med., (2019), In press, doi: 10.1002/jum.14861. |
[32] | 33. R. F. Chang, S. F. Huang and L. P. Wang, et al., Microcalcification detection in 3-D breast ultrasound, 27th Annual International Conference of the IEEE Engineering in Medicine and Biology Society, (2005), 6297–6300. |
[33] | 34. R. F. Chang, Y. L. Hou and C. S. Huang, et al., Automatic detection of microcalcifications in breast ultrasound, Med. Phys., 40 (2013), 102901. |
[34] | 35. B. H. Cho, C. Chang and J. H. Lee, et al., Fast microcalcification detection in ultrasound images using image enhancement and threshold adjacency statistics, SPIE Medical Imaging 2013: Computer-Aided Diagnosis, (2013), 86701Q. |
[35] | 36. M. S. Islam, Microcalcifications detection using image and signal processing techniques for early detection of breast cancer, Ph.D. Dissertation, University of North Dakota, Grand Forks, North Dakota, USA, (2016). |
[36] | 37. B. Zhuang, T. Chen and C. Leung, et al., Microcalcification enhancement in ultrasound images from a concave automatic breast ultrasound scanner, 2012 IEEE International Ultrasonics Symposium (IUS), (2012), 1662–1665. |
[37] | 38. J. Ophir, I. Cespedes and H. Ponnekanti, et al., Elastography: A quantitative method for imaging the elasticity of biological tissues, Ultrason. Imaging, 13 (1991), 111–134. |
[38] | 39. K. J. Parker, M. M. Doyley and D. J. Rubens, Imaging the elastic properties of tissue: The 20 year perspective, Phys. Med. Biol., 56 (2011), R1–R29. |
[39] | 40. R. G. Barr, K. Nakashima and D. Amy, et al., WFUMB guidelines and recommendations for clinical use of ultrasound elastography: Part 2: breast, Ultrasound Med. Biol., 41 (2015), 1148–1160. |
[40] | 41. J. Carlsen, C. Ewertsen and S. Sletting, et al., Ultrasound elastography in breast cancer diagnosis, Ultraschall. Med., 36 (2015), 550–562. |
[41] | 42. K. H. Ko, H. K. Jung and S. J. Kim, et al., Potential role of shear-wave ultrasound elastography for the differential diagnosis of breast non-mass lesions: preliminary report, Eur. Radiol., 24 (2014), 305–311. |
[42] | 43. J. M. Chang, J. K. Won and K. B. Lee, et al., Comparison of shear-wave and strain ultrasound elastography in the differentiation of benign and malignant breast lesions, AJR. Am. J. Roentgenol., 201 (2013), W347–W356. |
[43] | 44. M. Fatemi, L. E. Wold and A. Alizad, et al., Vibro-acoustic tissue mammography, IEEE. Trans. Med. Imaging, 21 (2002), 1–8. |
[44] | 45. A. Alizad, M. Fatemi and L. E. Wold, et al., Performance of vibro-acoustography in detecting microcalcifications in excised human breast tissue: a study of 74 tissue samples, IEEE. Trans. Med. Imaging, 23 (2004), 307–312. |
[45] | 46. A. Alizad, D. H. Whaley and J. F. Greenleaf, et al., Critical issues in breast imaging by vibro-acoustography, Ultrasonics., 44 (2006), e217–e220. |
[46] | 47. A. Gregory, M. Bayat and M. Denis, et al., An experimental phantom study on the effect of calcifications on ultrasound shear wave elastography, 2015 37th Annual International Conference of the IEEE Engineering in Medicine and Biology Society (EMBC), (2015), 3843–38 |
[47] | 48. A. Gregory, M. Mehrmohammadi and M. Denis, et al., Effect of calcifications on breast ultrasound shear wave elastography: an investigational study, PLoS. One, 10 (2015), e0137898. |
[48] | 49. A. J. Devaney, Super-resolution processing of multi-static data using time reversal and MUSIC, Preprint: Northeastern University, Boston, USA, (2000). |
[49] | 50. M. Fink, D. Cassereau and A. Derode, et al., Time-reversed acoustics, Rep. Prog. Phys., 63 (2000), 1933–1995. |
[50] | 51. J. L. Robert, M. Burcher and C. Cohen-Bacrie, et al., Time reversal operator decomposition with focused transmission and robustness to speckle noise: Application to microcalcification detection, J. Acoust. Soc. Am., 119 (2006), 3848–3859. |
[51] | 52. Y. Labyed and L. Huang, Ultrasound time-reversal MUSIC imaging of extended targets, Ultrasound Med. Biol., 38 (2012), 2018–2030. |
[52] | 53. Y. Labyed and L. Huang, TR-MUSIC inversion of the density and compressibility contrasts of point scatterers, IEEE. Trans. Ultrason. Ferroelectr. Freq. Control, 61 (2014), 16–24. |
[53] | 54. Y. Labyed and L. Huang, Detecting small targets using windowed time-reversal MUSIC imaging: A phantom study, 2011 IEEE International Ultrasonics Symposium (IUS), (2011), 1579–1582. |
[54] | 55. M. S. Islam and N. Kaabouch, Evaluation of TR-MUSIC algorithm efficiency in detecting breast microcalcifications, 2015 IEEE International Conference on Electro/Information Technology (EIT), (2015), 617–620. |
[55] | 56. E. Asgedom, L. J. Gelius and A. Austeng, et al., Time-reversal multiple signal classification in case of noise: A phase-coherent approach, J. Acoust. Soc. Am., 130 (2011), 2024–2034. |
[56] | 57. Y. Labyed and L. Huang, Super-resolution ultrasound imaging using a phase-coherent MUSIC method with compensation for the phase response of transducer elements, IEEE. Trans. Ultrason. Ferroelectr. Freq. Control., 60 (2013), 1048–1060. |
[57] | 58. L. Huang, Y. Labyed and K. Hanson, et al., Detecting breast microcalcifications using super-resolution ultrasound imaging: A clinical study, SPIE Medical Imaging 2013: Ultrasonic Imaging, Tomography, and Therapy, (2013), 86751O. |
[58] | 59. S. Bahramian, C. K. Abbey and M. F. Insana, Analyzing the performance of ultrasonic B-mode imaging for breast lesion diagnosis, SPIE Medical Imaging 2014: Physics of Medical Imaging, (2014), 90332A. |
[59] | 60. N. Q. Nguyen, C. K. Abbey and M. F. Insana, Objective assessment of sonographic: Quality II acquisition information spectrum, IEEE. Trans. Med. Imaging, 32 (2013), 691–698. |
[60] | 61. S. Bahramian and M. F. Insana, Retrieving high spatial frequency information in sonography for improved microcalcification detection, SPIE Medical Imaging 2014: Image Perception, Observer Performance, and Technology Assessment, (2014), 90370W. |
[61] | 62. S. Bahramian and M. F. Insana, Improved microcalcification detection in breast ultrasound: phantom studies, 2014 IEEE International Ultrasonics Symposium (IUS), (2014), 2359–2362. |
[62] | 63. C. K. Abbey, Y. Zhu and S. Bahramian, et al., Linear system models for ultrasonic imaging: intensity signal statistics, IEEE. Trans. Ultrason. Ferroelectr. Freq. Control., 64 (2017), 669–678. |
[63] | 64. S. Bahramian, C. K. Abbey and M. F. Insana, Method for recovering lost ultrasonic information using the echo-intensity mean, Ultrason. Imaging, 40 (2018), 283–299. |
[64] | 65. J. M. Thijssen, B. J. Oosterveld and R. F. Wagner, Gray level transforms and lesion detectability in echographic images, Ultrason. Imaging, 10 (1988), 171–195. |
[65] | 66. R. Hansen and B. A. Angelsen, SURF imaging for contrast agent detection, IEEE. Trans. Ultrason. Ferroelectr. Freq. Control., 56 (2009), 280–290. |
[66] | 67. R. Hansen, S. E. Masoy and T. F. Johansen, et al., Utilizing dual frequency band transmit pulse complexes in medical ultrasound imaging, J. Acoust. Soc. Am., 127 (2010), 579–587. |
[67] | 68. B.E. Denarie, Using SURF imaging for efficient detection of micro-calcifications, M.S. Thesis, Norwegian University of Science and Technology, Trondheim, Norway, (2010). |
[68] | 69. T.S. Jahren, Suppression of multiple scattering and imaging of nonlinear scattering in ultrasound imaging, M.S. Thesis, Norwegian University of Science and Technology, Trondheim, Norway, (2013). |
[69] | 70. E. Florenaes, S. Solberg and J. Kvam, et al., In vitro detection of microcalcifications using dual band ultrasound, 2017 IEEE International Ultrasonics Symposium (IUS), (2017), 1–4. |
[70] | 71. H. F. Zhang, K. Maslov and G. Stoica, et al., Functional photoacoustic microscopy for high-resolution and noninvasive in vivo imaging, Nat. Biotechnol., 24 (2006), 848–851. |
[71] | 72. Y. Y. Cheng, T. C. Hsiao and W. T. Tien, et al., Deep photoacoustic calcification imaging: feasibility study, 2012 IEEE International Ultrasonics Symposium (IUS), (2012), 2325–2327. |
[72] | 73. G. R. Kim, J. Kang and J. Y. Kwak, et al., Photoacoustic imaging of breast microcalcifications: a preliminary study with 8-gauge core-biopsied breast specimens, PLoS. One, 9 (2014), e105878. |
[73] | 74. J. Kang, E. K. Kim and J. Y. Kwak, et al., Optimal laser wavelength for photoacoustic imaging of breast microcalcifications, App. Phys. Lett., 99 (2011), 153702. |
[74] | 75. J. Kang, E. K. Kim and T. K. Song, et al., Photoacoustic imaging of breast microcalcifications: A validation study with 3-dimensional ex vivo data, 2012 IEEE International Ultrasonics Symposium (IUS), (2012), 28–31. |
[75] | 76. J. Kang, E. K. Kim and G. R. Kim, et al., Photoacoustic imaging of breast microcalcifications: A validation study with 3-dimensional ex vivo data and spectrophotometric measurement, J. Biophotonics., 8 (2015), 71–80. |
[76] | 77. H. Taki, T. Sakamoto and M. Yamakawa, et al., Small calcification depiction in ultrasonography using correlation technique for breast cancer screening, Acoustics 2012, (2012), 847–851. |
[77] | 78. H. Taki, T. Sakamoto and M. Yamakawa, et al., Small calcification indicator in ultrasonography using correlation of echoes with a modified Wiener filter, J. Med. Ultrason., 39 (2012), 127–135. |
[78] | 79. H. Taki, T. Sakamoto and T. Sato, et al., Small calculus detection for medical acoustic imaging using cross-correlation between echo signals, 2009 IEEE International Ultrasonics Symposium (IUS), (2009), 2398–2401. |
[79] | 80. H. Taki, T. Sakamoto and M. Yamakawa, et al., Indicator of small calcification detection in ultrasonography using decorrelation of forward scattered waves, Int. Conf. Comput. Electr. Syst. Sci. Eng., (2010), 175–1 |
[80] | 81. H. Taki, T. Sakamoto and M. Yamakawa, et al., Calculus detection for ultrasonography using decorrelation of forward scattered wave, J. Med. Ultrason., 37 (2010), 129–135. |
[81] | 82. H. Taki, T. Sakamoto and M. Yamakawa, et al., Small calcification depiction in ultrasound B-mode images using decorrelation of echoes caused by forward scattered waves, J. Med. Ultrason., 38 (2011), 73–80. |
[82] | 83. H. Taki, M. Yamakawa and T. Shiina, et al., Small calcification depiction in ultrasonography using frequency domain interferometry, 2014 IEEE International Ultrasonics Symposium (IUS), (2014), 1304–1307. |
[83] | 84. F. Tsujimoto, Microcalcifications in the breast detected by a color Doppler method using twinkling artifacts: Some important discussions based on clinical cases and experiments with a new ultrasound modality called multidetector-ultrasonography (MD-US), J. Med. Ultrason., 41 (2014), 99–108. |
[84] | 85. A. Relea, J. A. Alonso and M. González, et al., Usefulness of the twinkling artifact on Doppler ultrasound for the detection of breast microcalcifications, Radiología., 60 (2018), 413–423. |
[85] | 86. A. Rahmouni, R. Bargoin and A. Herment, et al., Color Doppler twinkling artifact in hyperechoic regions, Radiology., 199 (1996), 269–271. |
[86] | 87. S. W. Huang, J. L. Robert and E. Radulescu, et al., Beamforming techniques for ultrasound microcalcification detection, 2014 IEEE International Ultrasonics Symposium (IUS), (2014), 2193–2196. |
[87] | 88. L. Huang, F. Simonetti and P. Huthwaite, et al., Detecting breast microcalcifications using super-resolution and wave-equation ultrasound imaging: A numerical phantom study, SPIE Medical Imaging 2010: Ultrasonic Imaging, Tomography, and Therapy, (2010), 762919. |
[88] | 89. Y. Y. Liao, C. H. Li and C. K. Yeh, An approach based on strain-compounding technique and ultrasound Nakagami imaging for detecting breast microcalcifications, 2012 IEEE-EMBS International Conference on Biomedical and Health Informatics (BHI), (2012), 515–518. |
[89] | 90. P. M. Shankar, A statistical model for the ultrasonic backscattered echo from tissue containing microcalcifications, IEEE. Trans. Ultrason. Ferroelectr. Freq. Control., 60 (2013), 932–942. |
[90] | 91. Y. Y. Liao, C. H. Li and P. H. Tsui, et al., Discrimination of breast microcalcifications using a strain-compounding technique with ultrasound speckle factor imaging, IEEE. Trans. Ultrason. Ferroelectr. Freq. Control., 61 (2014), 955–965. |
[91] | 92. H. D. Cheng, J. Shan and W. Ju, et al., Automated breast cancer detection and classification using ultrasound images: A survey, Pattern. Recogn., 43 (2010), 299–317. |
[92] | 93. Q. Huang, Y. Chen and L. Liu, et al., On combining biclustering mining and AdaBoost for breast tumor classification, IEEE. Trans. Knowl. Data Eng. (2019), In press, doi: 10.1109/TKDE.2019.2891622. |
[93] | 94. Q. Huang, X. Huang and L. Liu, et al., A case-oriented web-based training system for breast cancer diagnosis, Comput. Methods Programs Biomed., 156 (2018), 73–83. |
[94] | 95. S. M. Hsu, W. H. Kuo and F. C. Kuo, et al., Breast tumor classification using different features of quantitative ultrasound parametric images, Int. J. Comput. Assist. Radiol. Surg., (2019), In press, doi: 10.1007/s11548-018-01908-8. |
[95] | 96. Q. Zhang, S. Song and Y. Xiao, et al., Dual-modal artificially intelligent diagnosis of breast tumors on both shear-wave elastography and B-mode ultrasound using deep polynomial networks, Med. Eng. Phys., 64 (2019), 1–6. |
[96] | 97. Q. Huang, Y. Luo and Q. Zhang, Breast ultrasound image segmentation: a survey, Int. J. Comput. Assist. Radiol. Surg., 12 (2017), 493–507. |
[97] | 98. Q. Huang, F. Zhang and X. Li, Machine learning in ultrasound computer-aided diagnostic systems: a survey, Biomed. Res. Int., 2018 (2018), 5137904. |
[98] | 99. Q. Huang, F. Zhang and X. Li, A new breast tumor ultrasonography CAD system based on decision tree and BI-RADS features, World. Wide. Web., 21 (2018), 1491–1504. |
[99] | 100. Q. Huang, F. Zhang and X. Li, Few-shot decision tree for diagnosis of ultrasound breast tumor using BI-RADS features, Multimed. Tools. Appl., 77 (2018), 25–29918. |
[100] | 101. Z. Zhou, W. Wu and S. Wu, et al., Semi-automatic breast ultrasound image segmentation based on mean shift and graph cuts, Ultrason. Imaging, 36 (2014), 256–276. |
[101] | 102. Z. Zhou, S. Wu and K. J. Chang, et al., Classification of benign and malignant breast tumors in ultrasound images with posterior acoustic shadowing using half-contour features, J. Med. Biol. Eng., 35 (2015), 178–187. |
[102] | 103. Q. Huang, J. Lan and X. Li, Robotic arm based automatic ultrasound scanning for three-dimensional imaging, IEEE. Trans. Industr. Inform., 15 (2019), 1173–1182. |
[103] | 104. Q. Huang and Z. Zeng, A review on real-time 3D ultrasound imaging technology, Biomed Res. Int., 2017 (2017), 6027029. |
[104] | 105. Q. Huang, B. Wu and J. Lan, et al., Fully automatic three-dimensional ultrasound imaging based on conventional B-scan, IEEE. Trans. Biomed. Circuits. Syst., 12 (2018), 426–436. |
[105] | 106. Z. Zhou, S. Wu and M. Y. Lin, et al., Three-dimensional visualization of ultrasound backscatter statistics by window-modulated compounding Nakagami imaging, Ultrason. Imaging, 40 (2018), 171–189. |
[106] | 107. E. Kozegar, M. Soryani and H. Behnam, et al., Mass segmentation in automated 3-D breast ultrasound using adaptive region growing and supervised edge-based deformable model, IEEE. Trans. Med. Imaging, 37 (2018), 918–928. |
[107] | 108. T. C. Chiang, Y. S. Huang and R. T. Chen, et al., Tumor detection in automated breast ultrasound using 3-D CNN and prioritized candidate aggregation, IEEE. Trans. Med. Imaging, 38 (2019), 240–249. |
1. | Wei-Hsin Yuan, Hui-Chen Hsu, Ying-Yuan Chen, Chia-Hung Wu, Supplemental breast cancer-screening ultrasonography in women with dense breasts: a systematic review and meta-analysis, 2020, 123, 0007-0920, 673, 10.1038/s41416-020-0928-1 | |
2. | Juan Wu, Rong Kong, Shen Tian, Hao Li, Jia-shuo Liu, Zhou Xu, Bao-shan Zou, Kai-nan Wu, Ling-quan Kong, Advances in Ultrasound-Guided Vacuum-Assisted Biopsy of Breast Microcalcifications, 2021, 47, 03015629, 1172, 10.1016/j.ultrasmedbio.2021.01.008 | |
3. | Yali Ouyang, Po-Hsiang Tsui, Shuicai Wu, Weiwei Wu, Zhuhuang Zhou, Classification of Benign and Malignant Breast Tumors Using H-Scan Ultrasound Imaging, 2019, 9, 2075-4418, 182, 10.3390/diagnostics9040182 | |
4. | Xiao-Long Li, Feng Lu, An-Qi Zhu, Dou Du, Yi-Feng Zhang, Le-Hang Guo, Li-Ping Sun, Hui-Xiong Xu, Multimodal Ultrasound Imaging in Breast Imaging-Reporting and Data System 4 Breast Lesions: A Prediction Model for Malignancy, 2020, 46, 03015629, 3188, 10.1016/j.ultrasmedbio.2020.08.003 | |
5. | Jinbum Kang, Kanghee Han, Kang‐Sik Kim, Won Seuk Jang, Min Jung Kim, Yangmo Yoo, 3D microcalcification detection using a color Doppler twinkling artifact with optimized transmit conditions: Preliminary results, 2020, 47, 0094-2405, 6171, 10.1002/mp.14342 | |
6. | Li Ren, Yangyang Liu, Ying Tong, Xuehong Cao, Yiyun Wu, Calcification segmentation based on a different scales superpixels saliency detection algorithm, 2020, 46, 03015629, 3404, 10.1016/j.ultrasmedbio.2020.08.013 | |
7. | Tao Jiang, Yanling Zhang, Shanshan Wu, Jujiang Mao, 2021, Chapter 4, 978-3-030-56347-9, 103, 10.1007/978-3-030-56348-6_4 | |
8. | Kun Lan, Gloria Li, Yang Jie, Rui Tang, Liansheng Liu, Simon Fong, Convolutional neural network with group theory and random selection particle swarm optimizer for enhancing cancer image classification, 2021, 18, 1551-0018, 5573, 10.3934/mbe.2021281 | |
9. | P D Karunia, P Prajitno, D S Soejoko, Automatic Detection of Breast Calcification in Ultrasound Imaging with Convolutional Neural Network, 2021, 2019, 1742-6588, 012077, 10.1088/1742-6596/2019/1/012077 | |
10. | Giulia Matrone, Muyinatu A. Lediju Bell, Alessandro Ramalli, Spatial Coherence Beamforming With Multi-Line Transmission to Enhance the Contrast of Coherent Structures in Ultrasound Images Degraded by Acoustic Clutter, 2021, 68, 0885-3010, 3570, 10.1109/TUFFC.2021.3099730 | |
11. | Jinyi Bian, Jili Zhang, Xiukun Hou, Diagnostic accuracy of ultrasound shear wave elastography combined with superb microvascular imaging for breast tumors, 2021, 100, 0025-7974, e26262, 10.1097/MD.0000000000026262 | |
12. | Xiuling Zhou, Ye Zhang, Le Liu, Xiaochun Feng, Hongshi Zhang, Therapeutic effect of acupuncture combined montelukast sodium on cough variant asthma in children, 2021, 100, 0025-7974, e28048, 10.1097/MD.0000000000028048 | |
13. | Jinbum Kang, Kanghee Han, Ilseob Song, Kang-Sik Kim, Won Seuk Jang, Min Jung Kim, Yangmo Yoo, Real-Time Ultrasound Detection of Breast Microcalcifications Using Multifocus Twinkling Artifact Imaging, 2022, 41, 0278-0062, 1300, 10.1109/TMI.2021.3136901 | |
14. | Chengbin Wang, Chenglin Yan, Lu An, Huifeng Zhao, Shaoli Song, Shiping Yang, Fe3O4 assembly for tumor accurate diagnosis by endogenous GSH responsive T2/T1 magnetic relaxation conversion, 2021, 9, 2050-750X, 7734, 10.1039/D1TB01018B | |
15. | Angela Logullo, Karla Prigenzi, Cristiane Nimir, Andreia Franco, Mario Campos, Breast microcalcifications: Past, present and future (Review), 2022, 16, 2049-9450, 10.3892/mco.2022.2514 | |
16. | Ayaka Naka, Toshinori Morisaku, Shu-hei Urashima, Hiroharu Yui, Characterization of the Elasticity of Small Objects Buried in Media Based on the Measurements of Photoacoustic Temporal Waveforms, 2020, 36, 0910-6340, 1487, 10.2116/analsci.20P076 | |
17. | Stine Hverven Thon, Roy Edgar Hansen, Andreas Austeng, Detection of Point Scatterers in Medical Ultrasound, 2022, 69, 0885-3010, 617, 10.1109/TUFFC.2021.3129619 | |
18. | Wenhui Ren, Xuelian Zhao, Xiaowei Zhao, Huijiao Yan, Shangying Hu, Youlin Qiao, Zhijian Xu, Fanghui Zhao, The Potential of Adding Mammography to Handheld Ultrasound or Automated Breast Ultrasound to Reduce Unnecessary Biopsies in BI-RADS Ultrasound Category 4a: A Multicenter Hospital-Based Study in China, 2023, 30, 1718-7729, 3301, 10.3390/curroncol30030251 | |
19. | Ethan O. Cohen, Rachel E. Perry, Ravinder S. Legha, Hilda H. Tso, Kyungmin Shin, Megan E. Speer, Kanchan A. Phalak, Jia Sun, Jessica W. T. Leung, Suspicious Ultrasound-Occult Non-Calcified Mammographic Masses, Asymmetries, and Architectural Distortions Are Moderate Probability for Malignancy, 2024, 16, 2072-6694, 655, 10.3390/cancers16030655 | |
20. | Zhengchang Kou, Trevor H. Park, Rita J. Miller, Michael L. Oelze, Detection of microcalcifications using nonlinear beamforming techniques, 2023, 49, 03015629, 1709, 10.1016/j.ultrasmedbio.2023.03.011 | |
21. | Seda Aladag Kurt, Fusun Taskin, Yasemin Kayadibi, Tulin Ozturk, İbrahim Adaletli, Gul Esen Icten, Role of Combining Grayscale Findings With Superb Microvascular Imaging and Shear Wave Elastography in Standardization and Management of NON-MASS Breast Lesions, 2024, 40, 1536-0253, 10.1097/RUQ.0000000000000689 | |
22. | Shahriar Mahmud Kabir, Mohammed Imamul Hassan Bhuiyan, Md. Alfew Rahman, Md. Abdul Aziz Prodhan, Md Faysal Miah, ASM Shihavuddin, 2022, Contourlet Transformed Nakagami Image-based Breast Tumor Classification Using Deep CNN, 978-1-6654-9045-0, 1, 10.1109/STI56238.2022.10103332 | |
23. | Xuening Cao, Hao Yang, Zu-Lei Wu, Bei-Bei Li, Ultrasound sensing with optical microcavities, 2024, 13, 2047-7538, 10.1038/s41377-024-01480-8 | |
24. | Li-Fang Yu, Chao-Chao Dai, Luo-Xi Zhu, Xiao-Jing Xu, Hong-Ju Yan, Chen-Xiang Jiang, Ling-Yun Bao, Detection and diagnosis of automated breast ultrasound in patients with BI-RADS category 4 microcalcifications: a retrospective study, 2024, 24, 1471-2342, 10.1186/s12880-024-01287-4 | |
25. | Esther Chiadikaobi Ugwueke, Dimitrios Palogiannidis, 2023, Preparation of Patient Mammogram Images for Segmentation, 979-8-3503-2248-4, 305, 10.1109/SCM58628.2023.10159092 | |
26. | Doaa Youssef, Somia A.M. Soliman, Jala El-Azab, Tawfik Ismail, 2024, Optical Screening System Using Biospeckle Imaging and Texture-Based Analysis for Breast Cancer Detection, 979-8-3503-7732-3, 1, 10.1109/ICTON62926.2024.10647593 | |
27. | Babak Mohammadzadeh Asl, Roya Paridar, 2024, Chapter 7, 978-981-99-7527-3, 307, 10.1007/978-981-99-7528-0_7 | |
28. | Roya Paridar, Babak Mohammadzadeh Asl, A Low-Complexity Compressive Sensing Algorithm for Point Target Detection Using Ultrafast Plane Wave Imaging, 2024, 12, 2169-3536, 2977, 10.1109/ACCESS.2023.3349348 | |
29. | Cuijuan Lou, Zhaohui Liu, Ming Yuchi, Mingyue Ding, Normalized Spatial Autocorrelation in Ultrasound B-Mode Imaging for Point-Scatterer Detection, 2024, 50, 03015629, 690, 10.1016/j.ultrasmedbio.2024.01.009 | |
30. | Yusuke Miyashita, Kumi Yanagida, Naoki Shirafuji, Ryuji Hoshide, Katsuyuki Sato, Keiichi Taku, Kimitoshi Nakamura, Ultrasonography is an effective tool for breast cancer screening in individuals with severe motor and intellectual disabilities, 2024, 37, 1360-2322, 10.1111/jar.13234 | |
31. | Doaa Youssef, Tawfik Ismail, Somia A.M. Soliman, Jala El-Azab, 2023, A Pilot Study Using Biospeckle Photography for Optical Breast Cancer Screening, 979-8-3503-0303-2, 1, 10.1109/ICTON59386.2023.10207319 | |
32. | Miguel Alejandro Hernández-Vázquez, Yazmín Mariela Hernández-Rodríguez, Fausto David Cortes-Rojas, Rafael Bayareh-Mancilla, Oscar Eduardo Cigarroa-Mayorga, Hybrid Feature Mammogram Analysis: Detecting and Localizing Microcalcifications Combining Gabor, Prewitt, GLCM Features, and Top Hat Filtering Enhanced with CNN Architecture, 2024, 14, 2075-4418, 1691, 10.3390/diagnostics14151691 | |
33. | Maria Chatrasingh, Cholatip Wiratkapun, Jackrit Suthakorn, A generalized closed-form solution for 3D registration of two-point sets under isotropic and anisotropic scaling, 2023, 51, 22113797, 106746, 10.1016/j.rinp.2023.106746 | |
34. | Haiyan Song, Cuihong Liu, Shengnan Li, Peixiao Zhang, TS-GCN: A novel tumor segmentation method integrating transformer and GCN, 2023, 20, 1551-0018, 18173, 10.3934/mbe.2023807 | |
35. | Massimo Ferrucci, Francesco Milardi, Daniele Passeri, Matteo Cagol, Paola Del Bianco, Ugo Grossi, Alberto Marchet, Intraoperative ultrasound-guided breast-conserving surgery: A performance analysis on the basis of novel cancer lesion classification and patients' cosmetic satisfaction, 2025, 180, 00396060, 109037, 10.1016/j.surg.2024.109037 | |
36. | Chao Xu, Xu Chen, Shuxiao Wang, Yan Cai, Xiaopeng Shao, 2025, Design of optomechanical ultrasound sensor based on Si and SiN composite waveguide, 9781510688834, 246, 10.1117/12.3057314 |
Authors | Year | Method | Study | N | T | TCF (MHz) | Size* (mm) | Pattern | Reference standard | Findings |
Authors | Year | Method | Study | N | T | TCF (MHz) | Size* (mm) | Pattern | Reference standard | Findings |
Yang et al. [14] | 1997 | HF B-mode US | MCs in tumors in vivo | 84 | LAT | 10-5 | < 1 | Clustered | Mammography | Sensitivity: 95%, specificity: 87.8%, accuracy: 91%. |
Gufler et al. [15] | 2000 | HF B-mode US | MCs in non-palpable invasive carcinomas, in situ carcinomas and benign lesions in vivo | 46 | LAT | 7.5 | 0.1-1 | Clustered | Mammography /histology | MCs in malignant lesions were reliably identified by HF B-mode US, but they were difficult to detect in fibrocystic breast lesions. |
Teh et al. [16] | 2000 | HF B-mode US | MCs without associated mammographic or palpable masses in vivo | 44 | LAT | 13 | - | Clustered | Mammography /histology | Isolated clustered MCs were visualized by HF B-mode US in 41 cases. |
Moon et al. [17] | 2000 | HF B-mode US | MCs in hypoechoic benign and malignant masses in vivo | 94 | LAT | 10, 12 | - | Clustered/linear /segmental | Mammography /histology | The visibility at high-frequency ultrasound was much higher in malignant MCs compared to benign MCs. |
Cheung et al. [18] | 2002 | HF B-mode US | Mammographically detected MCs without a mass in vivo | 66 | LAT | 7, 5-9 | - | Clustered/linear /segmental | Mammography /histology | High-frequency ultrasound could depict MCs without an associated mass on mammography. |
Nagashima et al. [4] | 2005 | HF B-mode US | Mammographically detected MCs in DCIS in vivo | 73 | LAT | 7.5-13 | - | Clustered/linear/segmental | Mammography /histology | The lesions associated with MCs were identified in 74% by HF B-mode US. |
Stoblen et al. [19] | 2011 | HF B-mode US | Mammographically detected MCs in BI-RADS 4a patients in vivo | 52 | LAT | 13 | - | Clustered/linear/segmental | Mammography / histology | Detection rate: HF B-mode US: 98.1%, ApliPureTM: 100%, MicroPureTM: 25%. |
Huang et al. [20] | 2017 | HF B-mode US | MCs in screening mammography in vivo | 133 | - | - | - | —— | Mammography | Sensitivity: 87.5%, specificity: 75%, accuracy for malignancy: 82.1%. |
Authors | Year | Method | Study | N | T | TCF (MHz) | Size* (mm) | Pattern | Reference standard | Findings |
Machado et al. [11] | 2012 | MicroPureTM | Mammographically identified MCs in vivo | 20 | LAT | 14 | - | Isolated | Mammography | MicroPureTM images showed more MCs than HF B-mode US, but still less than mammography. |
Grigoryev et al. [30] | 2014 | MicroPureTM | Lesions with suspicious MCs in vivo | 104 | LAT | 9 | - | —— | Mammography | The detection of MCs with MicroPureTM imaging in breasts with a hypoechoic focal lesion correlated well with digital mammography. |
Tan et al. [24] | 2015 | MicroPureTM | Female patients with suspicious lesions | 135 | LAT | 5-14 | - | —— | Histology | MicroPureTM imaging yielded better performance in MC detection than HF B-mode US and Doppler US did. |
Park et al. [25] | 2016 | MicroPureTM | Grouped MCs without a mass on screening mammography | 9 | LAT | 7-18 | - | Clustered | Mammography/histology | MicroPureTM imaging was a promising US technique that could improve the sensitivity for detecting grouped MCs that were not associated with mass in the breast on mammography. |
Machado et al. [31] | 2018 | MicroPureTM | Surgical breast specimens in vitro | 22 | LAT | 14 | - | Isolated | Mammography | MicroPureTM identified more breast MCs than B-mode US in surgical breast specimens in vitro. |
Machado et al. [32] | 2018 | MicroPureTM | Lesions with suspicious MCs in vivo | 100 | LAT | 14 | - | Isolated | Mammography | MicroPureTM may represent a new imaging method for guiding a biopsy to areas of MCs. |
Fatemi et al. [44] | 2002 | Elastography | MCs in excised tissue samples ex vivo | 3 | TCT | 3 | ≥ 0.11 | —— | Mammography/histology | VA was capable of detecting small breast MCs, which could be delineated from within dense and sclerotic tissues. |
Alizad et al. [45] | 2004 | Elastography | MCs in excised tissue samples ex vivo | 74 | TCT | 3 | ≥ 0.11 | —— | Radiograph/histology | Visual inspection of VA guided by radiograph confirmed 78.4% of MCs. |
Gregory et al. [48] | 2015 | Elastography | Mammographically identified MCs in benign masses in vivo | 3 | LAT | 5, 5-14 | - | Clustered/isolated | Mammography | The presence of large isolated MCs and highly concentrated clusters of MCs could introduce areas with apparent high elasticity in SWE. |
Authors | Year | Method | Study | N | T | TCF (MHz) | Size* (mm) | Pattern | Reference standard | Findings |
Labyed et al. [54] | 2011 | TR-MUSIC | Phantom experiments | - | LAT | - | 0.25 | Isolated | Synthetic aperture US | Synthetic-aperture US had great potential for detecting breast MCs. |
Labyed et al. [57] | 2013 | PC-MUSIC | Computer simulation/phantom experiments | - | LAT | 7.5 | 0.25 | Isolated | Mammography | The accuracy of target localization and image resolution were improved. |
Huang et al. [58] | 2013 | PC-MUSIC | Mammographically identified MCs in cysts, masses or breast tissues in vivo | 40 | LAT | 7.5 | - | —— | Mammography | The super-resolution US PC-MUSIC imaging with synthetic-aperture US data could provide a new imaging modality for detecting breast MCs in clinic. |
Bahramian et al. [62] | 2014 | High spatial frequency | Computer simulation | - | LAT | 6.81 | - | Isolated | —— | The complement images that recovered the missing information in the display stage of B-mode imaging could resolve MCs. |
Bahramian et al. [64] | 2018 | High spatial frequency | Computer simulation | - | - | - | - | Isolated | —— | Supplementary intensity processing provided about twice the spatial information of the center frequency of the ultrasound system, which significantly enhanced the contrast of MCs. |
Denarie [68] | 2010 | SURF | Computer simulation | - | LAT, CAT | 0.9(LF); 8, 10(HF) | - | —— | —— | Using simulated data, the linear scattering components in the image was reduced by 29 dB, ensuring a 4 dB contrast level between MCs and surrounding tissues. |
Florenaes et al. [70] | 2017 | SURF | Breast phantom (CIRS model 073) | 1 | LAT | 8, 0.8 | - | Isolated | Mammography | By transmitting multiple dual frequency band pulse complexes, the scattering from normal tissue was suppressed and the signal from the MCs was enhanced. |
Kang et al. [74] | 2011 | PAI | Ex vivo experiments | 7 | LAT | 7 | < 1 | —— | Mammography | The feasibility of PAI to construct the images of MCs with high spatial and contrast resolutions was proved. |
Authors | Year | Method | Study | N | T | TCF (MHz) | Size* (mm) | Pattern | Reference standard | Findings |
Kang et al. [75] | 2012 | PAI | Three-dimensional ex vivo data | 2 | LAT | 7.2 | < 1 | Clustered | Mammography | PAI could provide optical contrast on breast MCs and their locations agreed well with their real positions. |
Kim et al. [73] | 2014 | PAI | Core-biopsied specimens | 21 | LAT | 7 | - | —— | Mammography | Photoacoustic imaging might serve as an alternative in overcoming the limitations of the conventional US imaging. |
Kang et al. [76] | 2015 | PAI | Three-dimensional ex vivo data | - | LAT | 7.5 | - | Clustered/isolated | Mammography | PAI might be more sensitive and specific in breast cancer screening than mammography. |
Taki et al. [83] | 2012 | Correlation technique | MC phantoms (spherical glass beads in swine breast tissue) | 4 | LAT | 7.5 | 0.1, 0.2, 0.3 | —— | —— | Using small glass beads to mimic MCs, the method based on the deterioration in the cross-correlation between adjacent scan lines had the potential to depict small MCs without acoustic shadowing. |
Huang et al. [87] | 2014 | Beamforming | Volunteer in vivo | - | LAT | - | - | Isolated | —— | The beamforming technique had potential for MC detection. |
Shankar [90] | 2013 | Envelope statistics | Phantom data modified to include bright spots to represent MCs | 2 | LAT | 6 | - | Isolated | —— | Higher speckle factors (seen with the McKay density) could be used to isolate and display MCs. |
Liao et al. [91] | 2014 | strain-compounding speckle factor imaging | Lesions with suspicious MCs in vivo | 26 | LAT | 7.5 | - | —— | B-mode US | The strain-compounding speckle factor imaging method was more effective at discriminating between MCs and false MCs. |
*: Mean size (diameter) of individual MCs; MC: Microcalcification; US: ultrasound; HF: High-frequency; VA: Vibro-acoustography; SWE: shear wave elastography; TR: time reversal; PC: phase coherent; MUSIC: multiple signal classification; SURF: second order ultrasound field; PAI: photoacoustic imaging. N: number of patients or specimens; T: transducer; TCF: transducer center frequency; LAT: linear array transducer; CAT: curvilinear array transducer; TCT: two-element confocal transducer. |
Examination method | Advantages | Limitations |
HF B-mode US | High specificity. | The total detection rate of breast MCs is low. |
MicroPureTM | Enhancement of contrast of MC against background. | False positive rate is high. |
Ultrasound elastography | Effective detection of coarse calcification and clustered MCs in breast masses. | It is easy to misdiagnose benign tumor with MCs as malignant. |
TR-MUSIC | High precision in positioning scatterers and good horizontal resolution. | It is easily affected by noise and the axial resolution is low. |
PC-MUSIC | Improve axial resolution of TR-MUSIC. | The positioning is not accurate. |
High spatial frequency | It is easy to implement. | The method is too idealized and the accuracy needs further verification. |
SURF | It can suppress the linear scattering of background and increase the nonlinear scattering of MCs. | Imaging pulse is easily distorted. |
PAI | It combined the high contrast of optical imaging and high resolution of acoustic imaging. | It is easily affected by noise. |
Correlation technique | The sensitivity of conventional B-mode ultrasound is improved. | It is easily affected by noise. |
FDI | The sensitivity of conventional B-mode ultrasound is improved. | It is easily affected by noise. |
Beamforming | The sensitivity of conventional B-mode ultrasound is improved. | The accuracy needs further verification. |
Strain-compounding speckle factor imaging | Improve the effective identification of MCs and false MCs. | Selection of scattering statistical models and manual compression can cause errors. |
MC: microcalcification; US: ultrasound; HF: high-frequency; VA: Vibro-acoustography; SWE: shear wave elastography; TR: time reversal; PC: phase coherent; MUSIC: multiple signal classification; SURF: second order ultrasound field; PAI: photoacoustic imaging; FDI: frequency domain interferometry |
Authors | Year | Method | Study | N | T | TCF (MHz) | Size* (mm) | Pattern | Reference standard | Findings |
Authors | Year | Method | Study | N | T | TCF (MHz) | Size* (mm) | Pattern | Reference standard | Findings |
Yang et al. [14] | 1997 | HF B-mode US | MCs in tumors in vivo | 84 | LAT | 10-5 | < 1 | Clustered | Mammography | Sensitivity: 95%, specificity: 87.8%, accuracy: 91%. |
Gufler et al. [15] | 2000 | HF B-mode US | MCs in non-palpable invasive carcinomas, in situ carcinomas and benign lesions in vivo | 46 | LAT | 7.5 | 0.1-1 | Clustered | Mammography /histology | MCs in malignant lesions were reliably identified by HF B-mode US, but they were difficult to detect in fibrocystic breast lesions. |
Teh et al. [16] | 2000 | HF B-mode US | MCs without associated mammographic or palpable masses in vivo | 44 | LAT | 13 | - | Clustered | Mammography /histology | Isolated clustered MCs were visualized by HF B-mode US in 41 cases. |
Moon et al. [17] | 2000 | HF B-mode US | MCs in hypoechoic benign and malignant masses in vivo | 94 | LAT | 10, 12 | - | Clustered/linear /segmental | Mammography /histology | The visibility at high-frequency ultrasound was much higher in malignant MCs compared to benign MCs. |
Cheung et al. [18] | 2002 | HF B-mode US | Mammographically detected MCs without a mass in vivo | 66 | LAT | 7, 5-9 | - | Clustered/linear /segmental | Mammography /histology | High-frequency ultrasound could depict MCs without an associated mass on mammography. |
Nagashima et al. [4] | 2005 | HF B-mode US | Mammographically detected MCs in DCIS in vivo | 73 | LAT | 7.5-13 | - | Clustered/linear/segmental | Mammography /histology | The lesions associated with MCs were identified in 74% by HF B-mode US. |
Stoblen et al. [19] | 2011 | HF B-mode US | Mammographically detected MCs in BI-RADS 4a patients in vivo | 52 | LAT | 13 | - | Clustered/linear/segmental | Mammography / histology | Detection rate: HF B-mode US: 98.1%, ApliPureTM: 100%, MicroPureTM: 25%. |
Huang et al. [20] | 2017 | HF B-mode US | MCs in screening mammography in vivo | 133 | - | - | - | —— | Mammography | Sensitivity: 87.5%, specificity: 75%, accuracy for malignancy: 82.1%. |
Authors | Year | Method | Study | N | T | TCF (MHz) | Size* (mm) | Pattern | Reference standard | Findings |
Machado et al. [11] | 2012 | MicroPureTM | Mammographically identified MCs in vivo | 20 | LAT | 14 | - | Isolated | Mammography | MicroPureTM images showed more MCs than HF B-mode US, but still less than mammography. |
Grigoryev et al. [30] | 2014 | MicroPureTM | Lesions with suspicious MCs in vivo | 104 | LAT | 9 | - | —— | Mammography | The detection of MCs with MicroPureTM imaging in breasts with a hypoechoic focal lesion correlated well with digital mammography. |
Tan et al. [24] | 2015 | MicroPureTM | Female patients with suspicious lesions | 135 | LAT | 5-14 | - | —— | Histology | MicroPureTM imaging yielded better performance in MC detection than HF B-mode US and Doppler US did. |
Park et al. [25] | 2016 | MicroPureTM | Grouped MCs without a mass on screening mammography | 9 | LAT | 7-18 | - | Clustered | Mammography/histology | MicroPureTM imaging was a promising US technique that could improve the sensitivity for detecting grouped MCs that were not associated with mass in the breast on mammography. |
Machado et al. [31] | 2018 | MicroPureTM | Surgical breast specimens in vitro | 22 | LAT | 14 | - | Isolated | Mammography | MicroPureTM identified more breast MCs than B-mode US in surgical breast specimens in vitro. |
Machado et al. [32] | 2018 | MicroPureTM | Lesions with suspicious MCs in vivo | 100 | LAT | 14 | - | Isolated | Mammography | MicroPureTM may represent a new imaging method for guiding a biopsy to areas of MCs. |
Fatemi et al. [44] | 2002 | Elastography | MCs in excised tissue samples ex vivo | 3 | TCT | 3 | ≥ 0.11 | —— | Mammography/histology | VA was capable of detecting small breast MCs, which could be delineated from within dense and sclerotic tissues. |
Alizad et al. [45] | 2004 | Elastography | MCs in excised tissue samples ex vivo | 74 | TCT | 3 | ≥ 0.11 | —— | Radiograph/histology | Visual inspection of VA guided by radiograph confirmed 78.4% of MCs. |
Gregory et al. [48] | 2015 | Elastography | Mammographically identified MCs in benign masses in vivo | 3 | LAT | 5, 5-14 | - | Clustered/isolated | Mammography | The presence of large isolated MCs and highly concentrated clusters of MCs could introduce areas with apparent high elasticity in SWE. |
Authors | Year | Method | Study | N | T | TCF (MHz) | Size* (mm) | Pattern | Reference standard | Findings |
Labyed et al. [54] | 2011 | TR-MUSIC | Phantom experiments | - | LAT | - | 0.25 | Isolated | Synthetic aperture US | Synthetic-aperture US had great potential for detecting breast MCs. |
Labyed et al. [57] | 2013 | PC-MUSIC | Computer simulation/phantom experiments | - | LAT | 7.5 | 0.25 | Isolated | Mammography | The accuracy of target localization and image resolution were improved. |
Huang et al. [58] | 2013 | PC-MUSIC | Mammographically identified MCs in cysts, masses or breast tissues in vivo | 40 | LAT | 7.5 | - | —— | Mammography | The super-resolution US PC-MUSIC imaging with synthetic-aperture US data could provide a new imaging modality for detecting breast MCs in clinic. |
Bahramian et al. [62] | 2014 | High spatial frequency | Computer simulation | - | LAT | 6.81 | - | Isolated | —— | The complement images that recovered the missing information in the display stage of B-mode imaging could resolve MCs. |
Bahramian et al. [64] | 2018 | High spatial frequency | Computer simulation | - | - | - | - | Isolated | —— | Supplementary intensity processing provided about twice the spatial information of the center frequency of the ultrasound system, which significantly enhanced the contrast of MCs. |
Denarie [68] | 2010 | SURF | Computer simulation | - | LAT, CAT | 0.9(LF); 8, 10(HF) | - | —— | —— | Using simulated data, the linear scattering components in the image was reduced by 29 dB, ensuring a 4 dB contrast level between MCs and surrounding tissues. |
Florenaes et al. [70] | 2017 | SURF | Breast phantom (CIRS model 073) | 1 | LAT | 8, 0.8 | - | Isolated | Mammography | By transmitting multiple dual frequency band pulse complexes, the scattering from normal tissue was suppressed and the signal from the MCs was enhanced. |
Kang et al. [74] | 2011 | PAI | Ex vivo experiments | 7 | LAT | 7 | < 1 | —— | Mammography | The feasibility of PAI to construct the images of MCs with high spatial and contrast resolutions was proved. |
Authors | Year | Method | Study | N | T | TCF (MHz) | Size* (mm) | Pattern | Reference standard | Findings |
Kang et al. [75] | 2012 | PAI | Three-dimensional ex vivo data | 2 | LAT | 7.2 | < 1 | Clustered | Mammography | PAI could provide optical contrast on breast MCs and their locations agreed well with their real positions. |
Kim et al. [73] | 2014 | PAI | Core-biopsied specimens | 21 | LAT | 7 | - | —— | Mammography | Photoacoustic imaging might serve as an alternative in overcoming the limitations of the conventional US imaging. |
Kang et al. [76] | 2015 | PAI | Three-dimensional ex vivo data | - | LAT | 7.5 | - | Clustered/isolated | Mammography | PAI might be more sensitive and specific in breast cancer screening than mammography. |
Taki et al. [83] | 2012 | Correlation technique | MC phantoms (spherical glass beads in swine breast tissue) | 4 | LAT | 7.5 | 0.1, 0.2, 0.3 | —— | —— | Using small glass beads to mimic MCs, the method based on the deterioration in the cross-correlation between adjacent scan lines had the potential to depict small MCs without acoustic shadowing. |
Huang et al. [87] | 2014 | Beamforming | Volunteer in vivo | - | LAT | - | - | Isolated | —— | The beamforming technique had potential for MC detection. |
Shankar [90] | 2013 | Envelope statistics | Phantom data modified to include bright spots to represent MCs | 2 | LAT | 6 | - | Isolated | —— | Higher speckle factors (seen with the McKay density) could be used to isolate and display MCs. |
Liao et al. [91] | 2014 | strain-compounding speckle factor imaging | Lesions with suspicious MCs in vivo | 26 | LAT | 7.5 | - | —— | B-mode US | The strain-compounding speckle factor imaging method was more effective at discriminating between MCs and false MCs. |
*: Mean size (diameter) of individual MCs; MC: Microcalcification; US: ultrasound; HF: High-frequency; VA: Vibro-acoustography; SWE: shear wave elastography; TR: time reversal; PC: phase coherent; MUSIC: multiple signal classification; SURF: second order ultrasound field; PAI: photoacoustic imaging. N: number of patients or specimens; T: transducer; TCF: transducer center frequency; LAT: linear array transducer; CAT: curvilinear array transducer; TCT: two-element confocal transducer. |
Examination method | Advantages | Limitations |
HF B-mode US | High specificity. | The total detection rate of breast MCs is low. |
MicroPureTM | Enhancement of contrast of MC against background. | False positive rate is high. |
Ultrasound elastography | Effective detection of coarse calcification and clustered MCs in breast masses. | It is easy to misdiagnose benign tumor with MCs as malignant. |
TR-MUSIC | High precision in positioning scatterers and good horizontal resolution. | It is easily affected by noise and the axial resolution is low. |
PC-MUSIC | Improve axial resolution of TR-MUSIC. | The positioning is not accurate. |
High spatial frequency | It is easy to implement. | The method is too idealized and the accuracy needs further verification. |
SURF | It can suppress the linear scattering of background and increase the nonlinear scattering of MCs. | Imaging pulse is easily distorted. |
PAI | It combined the high contrast of optical imaging and high resolution of acoustic imaging. | It is easily affected by noise. |
Correlation technique | The sensitivity of conventional B-mode ultrasound is improved. | It is easily affected by noise. |
FDI | The sensitivity of conventional B-mode ultrasound is improved. | It is easily affected by noise. |
Beamforming | The sensitivity of conventional B-mode ultrasound is improved. | The accuracy needs further verification. |
Strain-compounding speckle factor imaging | Improve the effective identification of MCs and false MCs. | Selection of scattering statistical models and manual compression can cause errors. |
MC: microcalcification; US: ultrasound; HF: high-frequency; VA: Vibro-acoustography; SWE: shear wave elastography; TR: time reversal; PC: phase coherent; MUSIC: multiple signal classification; SURF: second order ultrasound field; PAI: photoacoustic imaging; FDI: frequency domain interferometry |